- Phonetics Lab, University of California, Davis, CA, United States
The current study tests whether individuals (n = 53) produce distinct speech adaptations during pre-scripted spoken interactions with a voice-AI assistant (Amazon’s Alexa) relative to those with a human interlocutor. Interactions crossed intelligibility pressures (staged word misrecognitions) and emotionality (hyper-expressive interjections) as conversation-internal factors that might influence participants’ intelligibility adjustments in Alexa- and human-directed speech (DS). Overall, we find speech style differences: Alexa-DS has a decreased speech rate, higher mean f0, and greater f0 variation than human-DS. In speech produced toward both interlocutors, adjustments in response to misrecognition were similar: participants produced more distinct vowel backing (enhancing the contrast between the target word and misrecognition) in target words and louder, slower, higher mean f0, and higher f0 variation at the sentence-level. No differences were observed in human- and Alexa-DS following displays of emotional expressiveness by the interlocutors. Expressiveness, furthermore, did not mediate intelligibility adjustments in response to a misrecognition. Taken together, these findings support proposals that speakers presume voice-AI has a “communicative barrier” (relative to human interlocutors), but that speakers adapt to conversational-internal factors of intelligibility similarly in human- and Alexa-DS. This work contributes to our understanding of human-computer interaction, as well as theories of speech style adaptation.
Introduction
People dynamically adapt their speech according to the communicative context and (apparent) barriers present. In the presence of background noise, for example, speakers produce speech that is louder, slower, and higher pitched (“Lombard speech”) (for a review, see Brumm and Zollinger, 2011), argued by some to be an automatic, non-socially mediated response (Junqua, 1993; Junqua, 1996). Other work has shown that people adapt their speech to the type of listener they are engaging with. One stance is that speakers presume certain types of interlocutors to have greater communicative barriers (Clark and Murphy, 1982; Clark, 1996; Oviatt et al., 1998b; Branigan et al., 2011). Supporting this account, prior work has shown that people use different speech styles when talking to non-native speakers (Scarborough et al., 2007; Uther et al., 2007; Hazan et al., 2015), hearing impaired adults (Picheny et al., 1985; Scarborough and Zellou, 2013; Knoll et al., 2015), and computers (Oviatt et al., 1998a; Oviatt et al., 1998b; Bell and Gustafson, 1999; Bell et al., 2003; Lunsford et al., 2006; Stent et al., 2008; Burnham et al., 2010; Mayo et al., 2012; Siegert et al., 2019). For example, computer-directed speech (DS) has been shown to be louder (Lunsford et al., 2006), with durational lengthening (Burnham et al., 2010; Mayo et al., 2012), greater vowel space expansion (Burnham et al., 2010), and smaller pitch range (Mayo et al., 2012) than speech directed to a (normal hearing adult) human.
This paper explores whether speakers use a specific speech style (or “register”) when talking to a voice-activated artificially intelligent (voice-AI) assistant. Voice-AI assistants (e.g., Amazon’s Alexa, Apple’s Siri, Google Assistant) are now a common interlocutor for millions of individuals completing everyday tasks (e.g., “set a timer for 5 min”, “turn on the lights”, etc.) (Bentley et al., 2018; Ammari et al., 2019). A growing body of research has begun to investigate the social, cognitive, and linguistic effects of humans interacting with voice-AI (Purington et al., 2017; Arnold et al., 2019; Cohn et al., 2019b; Burbach et al., 2019). For example, recent work has shown that listeners attribute human-like characteristics to the text-to-speech (TTS) output used for modern voice-AI, including personality traits (Lopatovska, 2020), apparent age (Cohn et al., 2020a; Zellou et al., 2021), and gender (Habler et al., 2019; Loideain and Adams, 2020). While the spread of voice-AI assistants is undeniable—particularly in the United States—there are many open scientific questions as to the nature of people’s interactions with voice-AI.
There is some evidence for a different speech style used in interactions with voice-AI assistants: several studies have used classifiers to successfully identify “device-” and “non-device-directed” speech from users’ interactions with Amazon Alexa (Mallidi et al., 2018; Huang et al., 2019). Yet, in these cases, the linguistic content, physical distance from the device, and other factors were not controlled and might have contributed to differences that are not speech-style adaptations per se. Critically, holding the interaction constant across a voice-AI and human interlocutor can reveal if individuals have a distinct voice-AI speech style. Some groups have aimed to compare human and voice-AI speech styles in more similar contexts. For instance, the Voice Assistant Conversation Corpus (VACC) had participants complete the same type of communicative task (setting an appointment on a calendar and doing a quiz) with an Alexa Echo and a real human confederate (Siegert et al., 2018). Several studies measuring the acoustic-phonetic features of human- and Alexa-DS in the corpus found productions toward Alexa were louder (Raveh et al., 2019; Siegert and Krüger, 2021), higher in fundamental frequency (f0, perceived pitch) (Raveh et al., 2019), and contained different vowel formant characteristics1 (Siegert and Krüger, 2021). Yet, similar to studies of individuals using Alexa in their homes (e.g., Huang et al., 2019), differences observed in the VACC might also be driven by physical distance from the device and conversational variations. The current study holds context and physical distance from the microphone constant for the two interlocutors to address these limitations in prior work.
Making a direct human- and Alexa-DS comparison in a scripted task can speak to competing predictions across different computer personification accounts: if speech styles differ because speakers have a “routinized” way of talking to computers (in line with routinized interaction accounts) or if speech styles are the same (in line with technology equivalence accounts). Routinized interaction accounts propose that people have a “routinized” way of interacting with technological systems (Gambino et al., 2020), borne out of real experience with the systems, as well as a priori expectations. As mentioned, there is ample evidence for a computer-DS register (e.g., Bell and Gustafson, 1999; Bell et al., 2003; Burnham et al., 2010). Specifically, some propose that the computer faces additional communicative barriers, relative to humans (Oviatt et al., 1998b; Branigan et al., 2011). These attitudes appear to be a priori, developed before any evidence of communicative barriers in an interaction. For example, people rate TTS voices as less “communicatively competent” (Cowan et al., 2015). Therefore, one prediction for the current study is that speakers might have overall different speech styles in human- and Alexa-DS, reflecting this presumed communicative barrier and a “routinized” way of talking to voice-AI.
Technology equivalence accounts, on the other hand, propose that people automatically and subconsciously apply social behaviors from human-human interaction to their interactions with computer systems (e.g., Lee, 2008). For example, “Computers are Social Actors” (CASA) (Nass et al., 1994; Nass et al., 1997) specifies that this transfer of behaviors from human-human interaction is triggered when people detect a “cue” of humanity in the system, such as engaging with a system using language. For example, people appear to apply politeness norms from human-human interaction to computers: giving more favorable ratings when a computer directly asks about its own performance, relative to when a different computer elicits this information (Nass et al., 1994; Hoffmann et al., 2009). In line with technology equivalence accounts, there is some evidence for applied social behaviors to voice-AI in the way people adjust their speech, such as gender-mediated vocal alignment (Cohn et al., 2019b; Zellou et al., 2021). In the present study, one prediction from technology equivalence accounts is that people will adjust their speech patterns when talking to voice-AI and humans in similar ways if the communicative context is controlled.
Different Strategies to Improve Intelligibility Following a Misrecognition?
To probe routinized interaction and technology equivalence accounts, the present study further investigates if speakers adapt their speech differently after a human or a voice-AI assistant “mishears” them. There is evidence that speakers monitor communicative pressures during an interaction, varying their acoustic-phonetic output to improve intelligibility when there is evidence listeners might mishear them (Smiljanić and Bradlow, 2009; Hazan and Baker, 2011). Lindblom’s (1990) Hyper- and Hypo-articulation (H&H) model proposes a real-time trade-off between speakers’ needs (i.e., to preserve articulatory effort) and listeners’ needs (i.e., to be more intelligible). While the majority of prior work examining speakers’ adaptations following a computer misrecognition has lacked a direct human comparison, many of the adjustments parallel those observed in human-human interaction; for example, speakers produce louder and slower speech after a dialogue system conveys that it “heard” the wrong word (Oviatt et al., 1998a; Bell and Gustafson, 1999; Swerts et al., 2000). Additionally, some studies report vowel adaptations in response to a misunderstanding that are consistent with enhancements to improve intelligibility, including vowel space expansion (Bell and Gustafson, 1999; Maniwa et al., 2009) and increase in formant frequencies (Vertanen, 2006). There is also evidence of targeted adjustments: speakers produce more vowel-specific expansion (e.g., high vowels produced higher) in response to misrecognitions by a dialogue system (Stent et al., 2008). Will speakers use different strategies to improve intelligibility following a staged word misrecognition based on who their listener is? One possibility is that speakers might have a “routinized” way of improving their intelligibility following a misrecognition made by a voice-AI assistant, which would support routinized interaction accounts. At the same time, Burnham et al. (2010) found no difference between speech adjustments post-misrecognition for an (apparent) human and digital avatar, but only more global differences for the computer interlocutor (i.e., speech with longer segmental durations and with greater vowel space expansion). Therefore, it is possible that speakers will produce similar intelligibility adjustments in response to a staged misrecognition made by either a voice-AI or human listener, supporting technology equivalence accounts.
Additionally, the current study adds a novel manipulation in addition to intelligibility pressures: emotional expressiveness. When an interlocutor “mishears”, they might be disappointed and express it (e.g., “Darn! I think I misunderstood.”); when they get it correct, they might be enthusiastic and convey that in their turn (e.g., “Awesome! I think I heard boot.”). Emotional expressiveness is a common component of naturalistic human conversations, providing a window into how the listener is feeling (Goffman, 1981; Ameka, 1992). This “socio-communicative enhancement” might increase the pressure for speakers to adapt their speech for the listener. On the one hand, this enhanced emotional expressiveness might result in even more similar adjustments for voice-AI and human interlocutors, since adding expressiveness might increase the perception of human-likeness for the device, which could strengthen technology equivalence. Indeed, there is some work to suggest that emotional expressiveness in a computer system is perceived favorably by users. For instance, Brave and colleagues (2005) found when computer systems expressed empathetic emotion, they were rated more positively. For voice-AI, there is a growing body of work testing how individuals perceive emotion in TTS voices (Cohn et al., 2019a; Cohn et al., 2020a). For example, an Amazon Alexa Prize socialbot was rated more positively if it used emotional interjections (Cohn et al., 2019a). Alternatively, the presence of emotionality might lead to distinct clear speech strategies for the human and voice-AI interlocutors. For example, a study of phonetic alignment (using the same corpus in the current study) found that vowel duration alignment differed both by the social category of interlocutor (human vs. voice-AI) and based on emotionality (Zellou and Cohn, 2020): participants aligned more in response to a misrecognition, consistent with H&H theory (Lindblom, 1990), which increased even more when the voice-AI talker was emotionally expressive when conveying their misunderstanding (e.g., “Bummer! I’m not sure I understood. I think I heard sock or sack.”). Still, that study examined just one acoustic difference in speech behavior (vowel duration alignment). The present study investigates whether emotionality similarly mediates targeted speech adjustments to voice-AI, an underexplored research question.
Current Study
The present study examines a corpus of speech directed at a human and voice-AI interlocutor which crossed intelligibility factors (staged misrecognitions) and emotionality of the interlocutor’s responses in identical pre-scripted tasks (Zellou and Cohn, 2020). This is the first study, to our knowledge, to test both intelligibility and emotional expressiveness factors in speech style adaptations for a voice-AI assistant and human. Here, the Amazon Alexa voice (US-English, female) was selected for its ability to generate emotionally expressive phrases recorded by the voice actor, common in Alexa Skills Kit apps (“Speechcons”). To determine overall differences between Alexa- and human-DS, as well as more local intelligibility adjustments in response to a staged misrecognition, we measure several acoustic features associated with computer-DS and/or “clear” speech: intensity, speech rate, mean f0, f0 variation, and vowel formant characteristics (F1, F2).
Methods
Participants
Data were taken from a corpus (Zellou and Cohn, 2020) containing 53 native English speaking participants (27 female, 26 male; mean age of 20.28 years old, sd = 2.42 years; range: 18–34) talking to a voice-AI and human interlocutor in an identical interactive task. None reported having any hearing impairment. Nearly all participants (n = 49) reported using a voice-AI system: Alexa (n = 35), Siri (n = 13), Google Assistant (n = 1). Participants were recruited from the UC Davis psychology subjects pool and completed informed consent, in pursuance with the UC Davis Institutional Review Board (IRB).
Target Words
Sixteen target words, presented in Table 1, were selected from Babel (2012) who had chosen the items for being low frequency in American English; higher frequency items have been shown to be more phonetically reduced in production (e.g., Pluymaekers et al., 2005). Target words were all CVC words containing either /i, æ, u, ow, a/ and a word-final obstruent (e.g., /z/, /p/) (a subset of the words used in Babel, 2012). In addition, we selected a real-word vowel minimal pair, differing in vowel backness, to be used in the interlocutor responses in the misrecognition condition.
Interlocutor Recordings
The human and voice-AI interlocutor responses were pre-recorded. For the human, a female native California English speaker recorded responses in a sound attenuated booth, with a head-mounted microphone (Shure WH20 XLR). The Alexa productions were generated with the default female Alexa voice (US-English) with the Alexa Skills Kit. Both interlocutors generated introductions (“Hi! I’m Melissa. I’m a research assistant in the Phonetics Lab.”/“Hi! I’m Alexa. I’m a digital device through Amazon.”) and voice-over instructions for the task. We recorded each interlocutor producing two responses for each target word: a “correctly understood” response (“I think I heard bat”) and an “misrecognition” response (“I’m not sure I understood. I think I heard bought or bat.”). Figure 1 provides an example of the different interlocutor responses. Order of target word and misheard word was counterbalanced across sentences, such that the “correct” word did not always occur in the same position in these response types.
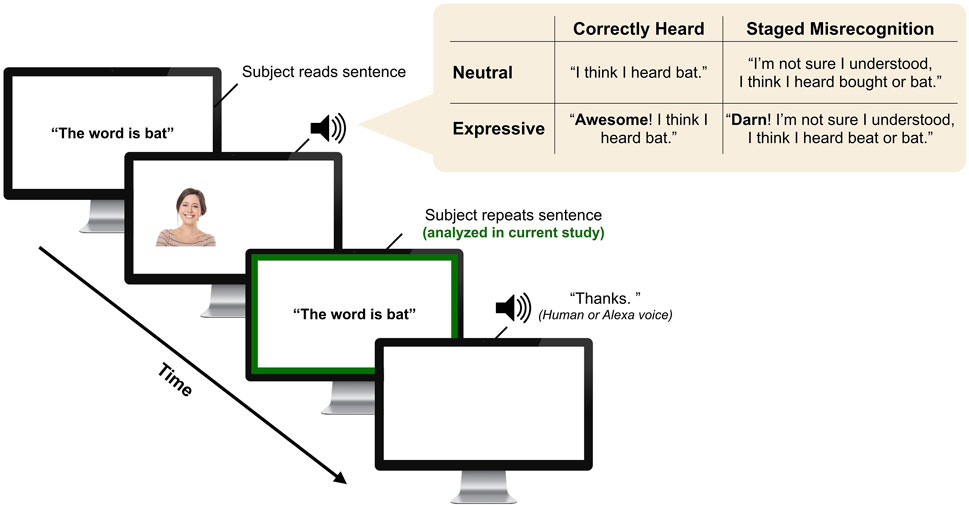
FIGURE 1. Interaction trial schematic. After participants read a sentence, the interlocutor (human or Alexa) responds in one of the Staged Misunderstanding Conditions (correctly heard, misrecognition) and Emotionality Conditions (neutral, emotionally expressive). Then, the subject responds (the production we analyze). Finally, the interlocutor provides a follow-up response.
Both interlocutors generated 16 emotionally expressive interjections as well: eight positive interjections (bam, bingo, kapow, wahoo, zing, awesome, dynamite, yipee) and eight negative interjections (argh, baa, blarg, oof, darn, boo, oy, ouch) selected from the Speechcons website2 at the time of the study. We generated these interjections for the Alexa text-to-speech (TTS) output using synthesis markup language (SSML) tags. The human produced these interjections in an expressive manner (independently, not imitating the Alexa productions). We randomly assigned each interjection to the interlocutor responses, matching in whether the response was correctly understood (positive interjection) or misunderstood (negative interjection). The full set of interjections was used twice in each block (e.g., eight positive interjections randomly concatenated to 16 correct productions). The full set of interlocutor productions are available on Open Science Framework3.
Procedure
Participants completed the experiment while seated in a sound-attenuated booth, wearing a head-mounted microphone (Shure WH20 XLR) and headphones (Sennheiser Pro), and facing a computer screen. First, we collected citation forms of the target words produced in sentences. Participants read the word in a sentence (“The word is bat.”) presented on the screen. Target words were presented randomly.
Following the Citation block, participants completed identical experimental blocks with both a human talker and an Alexa talker (block order counterbalanced across subjects). First, the interlocutor introduced themselves and then went through voice-over instructions with the participant. Participants saw an image corresponding to the interlocutor category: stock images of “adult female” (used in prior work; Zellou et al., 2021) and “Amazon Alexa” (2nd Generation Black Echo).
Each trial consisted of four turns. Participants first read a sentence aloud containing the target word sentence-finally (e.g., “The word is bat.”). Then, the interlocutor responded in one of four possible Staged Misunderstanding (correctly heard/misrecognition) and Emotionality (neutral/expressive) Conditions (see Figure 1). Next, the participant responded to the interlocutor by repeating the sentence (e.g., “The word is bat.”). This is the response that we acoustically analyze. Finally, the interlocutor provides a confirmation, randomized (“Thanks”, “Perfect”, “Okay”, “Uh huh”, “Got it”, etc.).
In 50% of trials, the interlocutor (human, Alexa) “misunderstood” the speakers, while in the other 50% they heard correctly. Additionally, in 50% of trials, the interlocutor responded with an expressive production (distributed equally across correctly heard and misrecognition trials). Order of target words was randomized, as well as trial correspondence to the Misunderstanding and Emotionality Conditions. In each block, participants produced all target sentences once for all conditions for a total of 128 trials for each interlocutor (16 words x two misunderstanding conditions x two emotionality conditions). Participants completed the task with both interlocutors (256 total target sentences). After the speech production experiment ended (and while still in the soundbooth), participants used a sliding scale (0–100) to rate how human-like each interlocutor sounded (order of interlocutor was randomized) (“How much like a real person did [Alexa/Human] sound?” (0 = not like a real person, 100 = extremely realistic)”. The overall experiment took roughly 45 min.
Acoustic Analysis
Four acoustic measurements were taken over each target sentence in both the Citation and Interaction blocks using Praat scripts (DiCanio, 2007; De Jong et al., 2017): intensity (dB), speech rate (syllables/second), mean fundamental frequency (f0) (semitones, ST, relative to 100 Hz), and f0 variation (ST). We centered the measurements from the Interaction blocks within-speaker, subtracting their Citation speech mean value (within-speaker, within-word). This measurement indicates changes from the speakers’ citation form for that feature.
To extract vowel-level features, recordings were force-aligned (using the Forced Alignment and Vowel Extraction (FAVE) suite) (Rosenfelder et al., 2014). Next, vowel boundaries were hand-corrected by trained research assistants: vowel onsets and offsets were defined by the presence of both higher format structure and periodicity. Following hand-correction, we measured vowel duration and vowel formant frequency values (F1, F2) at vowel midpoint with FAVE-extract (Rosenfelder et al., 2014) for the subset of 13 words containing corner vowels: /i/ (cheek, weave, deed), /u/ (boot, hoop, toot, dune), /a/ (pod, cot, sock, tot), and /æ/ (bat, tap). We additionally scaled the formant frequency values (from Hertz) using a log base-10 transformation and centering each value to the subject’s citation production values for that word (Nearey, 1978).
In order to assess whether speech changes made by participants were not simply alignment toward the interlocutors, the same sentence-level (rate, mean f0, f0 variation) and target vowel measurements (duration, F1, F2) were also taken over each interlocutor’s production in Turn 2 (e.g., “I think I heard weave.”). In order to compare across the interlocutors, formant frequency values (F1, F2) were centered relative to each interlocutor’s mean value for that word (log mean normalization: Nearey, 1978).
Statistical Analysis
Participants’ sentence-level values for each acoustic feature (centered to speaker citation form values) were modeled in separate linear mixed effects models with the lme4 R package (Bates et al., 2015), with identical model structure: fixed effects of Interlocutor (voice-AI, human), Staged Misunderstanding Condition (correctly heard, misrecognition), Expressiveness (neutral, expressive), and all possible interactions, with by-Sentence and by-Speaker random intercepts.
Participants’ vowel-level features (F1, F2) were also modeled in separate linear mixed effects models with a similar structure as in the sentence-level models: Interlocutor, Staged Misunderstanding Condition, Expressiveness Condition, with by-Word and by-Speaker random intercepts. In both the F1 and F2 model, we included an additional predictor of Vowel Category (For the F1 (height) model, this factor included two height levels: high vs. low vowels; for the F2 (backness) model, this factor included two levels: front vs. back vowels) and all possible interactions with the other predictors (Vowel Category*Interlocutor*Misunderstanding*Emotion). The formant models (F1, F2) additionally included a fixed effect of Vowel Duration (centered within speaker).
Results
Human-likeness Rating
Figure 2 provides the mean values for participants’ human-like ratings of the voices. A t-test on participants’ ratings of the voices confirmed that the Alexa voice was perceived as less human-like (x̄ = 31.06) than the human (x̄ = 87.67) [t (104.87) = −12.84, p < 0.001].
Interlocutor Stimuli Acoustics
T-tests of the interlocutors’ productions found no overall difference between the Alexa and Human speaking rate (Human x̄ = 2.53 syll/s; Alexa x̄ = 2.68 syll/s) [t (124.27) = −1.87, p = 0.06], but there was a significant difference in mean f0: the human had a higher mean f0 (x̄ = 14.42 ST) than Alexa (x̄ = 13.16 ST) [t (106.25) = 9.21, p < 0.001]. Additionally, the human produced greater f0 variation (x̄ = 3.27 ST) than Alexa (x̄ = 2.86 ST) [t (132.97) = 7.06, p < 0.001]. T-tests comparing formant frequency characteristics revealed no difference in vowel height (F1) for the interlocutors for high vowels (Human x̄ = −0.37 log Hz; Alexa x̄ = −0.41 log Hz) [t (35.28) = −1.38, p = 0.18] or low vowels (Human x̄ = 0.43 log Hz; Alexa x̄ = 0.47 log Hz) [t (45.42) = 1.75, p = 0.09]. Additionally, there was no difference in vowel fronting (F2) for the interlocutors for front vowels (Human x̄ = 0.30; Alexa x̄ = 0.35)[t (34.66) = 0.67, p = 0.51] or back vowels (Human x̄ = -0.18; Alexa x̄ = −0.22)[t (47.73) = 0.71, p = 0.48].
T-tests comparing the Expressiveness Conditions (neutral vs. emotionally expressive) confirmed differences: expressive productions were produced with a slower speaking rate (Expressive x̄ = 2.45 syll/s; Neutral x̄ = 2.76 syll/s) [t (153.88) = −4.25, p < 0.001] and with a lower mean f0 (Expressive x̄ = 13.55 ST; Neutral x̄ = 14.03 S T) [t (145.44) = −2.89, p < 0.01]. However, there was no difference for f0 variation (Expressive x̄ = 3.04 ST; Neutral x̄ = 3.09 ST) [t (157.44) = −0.60, p = 0.55].
T-tests comparing the Misunderstanding Conditions (correctly heard vs. misrecognition) showed no significant difference in speaking rate (Correct x̄ = 2.64 syll/s; misunderstood x̄ = 2.57 syll/s) [t (139.38) = 0.89, p = 0.37] or mean f0 (Correct x̄ = 13.92 ST; misunderstood x̄ = 13.65 ST) [t (114.62) = 1.60, p = 0.11]. However, they did vary in terms of f0 variation: larger for correctly understood (x̄ = 3.15 ST) than misrecognized (x̄ = 2.98 ST) [t (141.59) = 2.58, p < 0.05].
Participants’ Sentence-Level Measurements
Figure 3 displays the mean acoustic values for participants’ sentence-level measurements (centered to speakers’ Citation form values). Model output tables are provided in Supplementary Data Sheet 3, Appendices A1–A4.
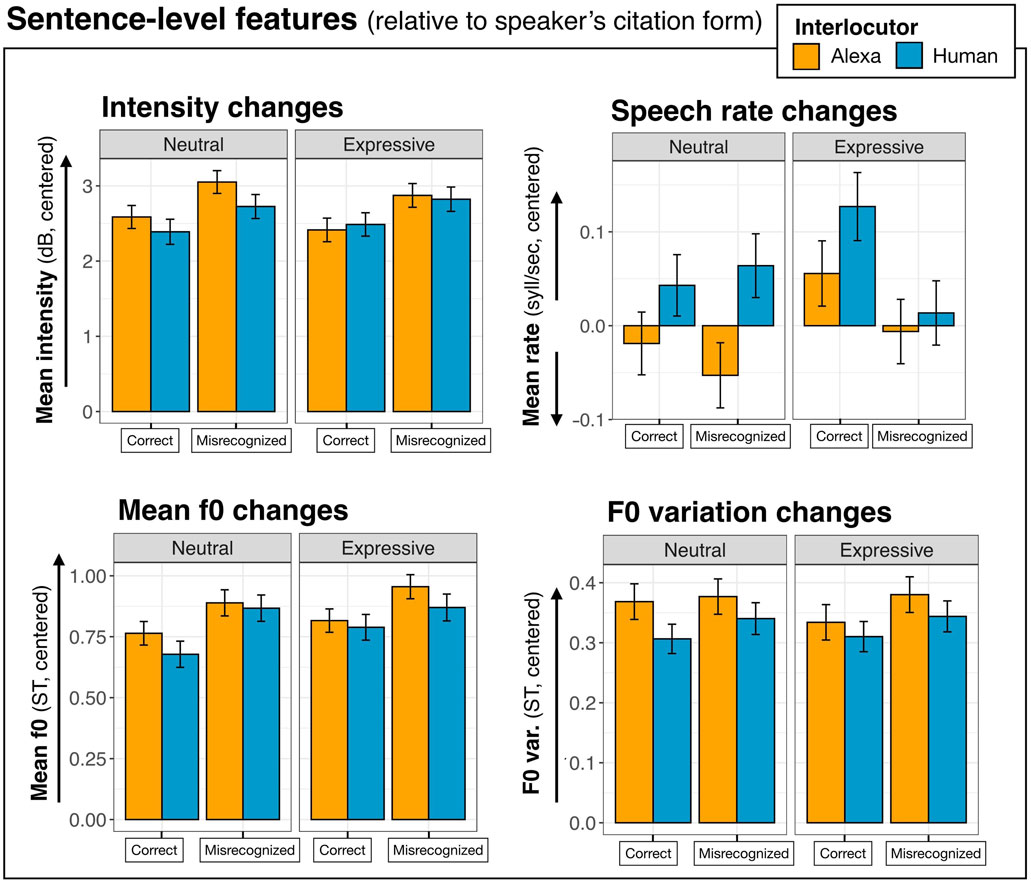
FIGURE 3. Mean acoustic changes from speakers’ citation form productions to the interaction with the Interlocutors (Alexa vs. human) for sentence intensity (in decibels, dB), speech rate (syllables per second), f0 (semitones, ST, rel. to 100 Hz), and f0 variation (ST). The x-axis shows Staged Misunderstanding Condition (correctly heard vs. misrecognized), while Expressiveness Condition is faceted. Values higher than 0.0 indicate an increase (relative to speakers’ citation form), while values lower than 0.0 indicate a relative decrease. Error bars depict the standard error.
The Intensity model showed a significant intercept: participants increased their intensity in the interaction (relative to their citation form) [Coef = 2.64, SE = 0.45, t = 5.86, p < 0.001]. There was also a main effect of Misunderstanding Condition: as seen in Figure 3, participants’ productions of sentences that the system did not understand correctly were louder than repetitions of utterances that the system understood correctly [Coef = 0.20, SE = 0.04, t = 5.13, p < 0.001]. No other effects or interactions were significant in the Intensity model.
The Speech Rate model showed no difference from 0 for the intercept: overall, speakers did not speed up or slow down their speech in interlocutor interactions, relative to their citation form productions. The model also revealed a main effect of Interlocutor, producing a slower speech rate (indicated by fewer syllables per second) in Alexa-DS [Coef = −0.03, SE = 0.01, t = −2.87, p < 0.01]. There was also a main effect of Misunderstanding Condition wherein speakers decreased their speech rate in response to a misrecognition [Coef = -0.02, SE = 0.01, t = −1.96, p < 0.05]. These effects can be seen in Figure 3. No other effects or interactions were significant in the model.
The Mean F0 model had a significant intercept, indicating that speakers increased their mean f0 in the interactions relative to the citation form productions [Coef = 0.83, SE = 0.15, t = 5.65, p < 0.001]. The model also showed an effect of Interlocutor: speakers produced a higher mean f0 toward the Alexa interlocutor [Coef = 0.03, SE = 0.01, t = 2.40, p < 0.05]. Additionally, there was an effect of Misunderstanding wherein responses to misunderstood utterances were produced with a higher f0 [Coef = 0.06 SE = 0.01, t = 5.04, p < 0.001], as seen in Figure 3. Furthermore, there was a main effect of Expressiveness Condition wherein speakers produced a higher mean f0 in response to emotionally expressive utterances [Coef = 0.03, SE = 0.01, t = 2.49, p < 0.05]. No other effects or interactions were observed in the Mean f0 model.
The F0 Variation model also had a significant intercept: relative to their citation form productions, speakers increased their f0 variation in the interaction [Coef = 0.34, SE = 0.07, t = 4.94, p < 0.001]. There was also a main effect of Interlocutor: speakers produced greater f0 variation in responses directed to the Alexa voice [Coef = 0.02, SE = 0.01, t = 2.79, p < 0.01]. Additionally, there was an effect of Misunderstanding: responses to misrecognitions were produced with greater f0 variation [Coef = 0.01, SE = 0.01, t = 1.98, p < 0.05]. No other effects or interactions were significant in the F0 Variation model.
Participants’ Vowel-Level Measurements
Figure 4 displays participants’ mean vowel-level values across conditions. Model output tables are provided in Supplementary Data Sheet 3, Appendices A5 and A6.
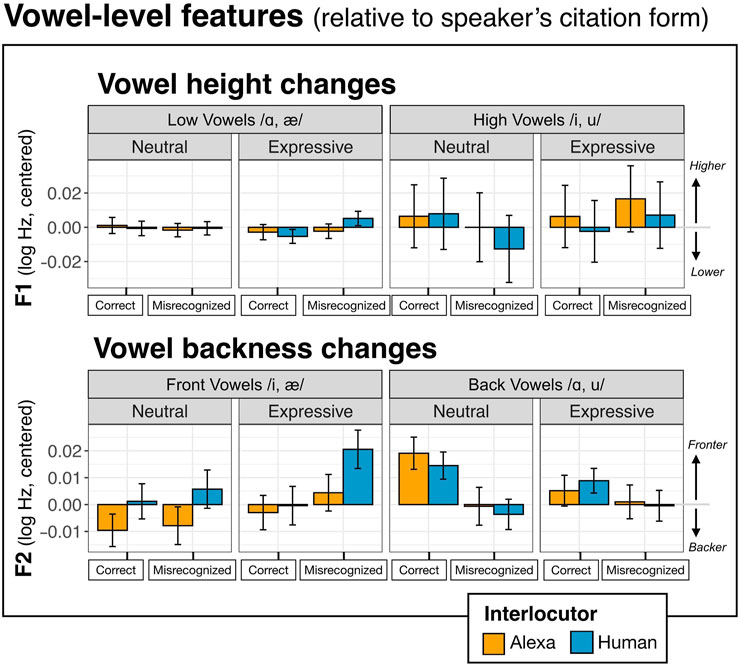
FIGURE 4. Mean acoustic changes from speakers’ citation form productions to the interaction with the Interlocutors (Alexa vs. human) for vowel duration (milliseconds, ms), F1 (log Hertz, Hz), and F2 (log Hertz, Hz). Formant plots are additionally faceted by Vowel Category: F1 (by vowel height: low vs. high vowels) and F2 (by vowel backness: front vs. back vowels). The x-axis shows Staged Misunderstanding Condition (correctly heard vs. misrecognized), while Expressiveness Condition is faceted. Values higher than 0.0 indicate an increase (relative to speakers’ citation form), while values lower than 0.0 indicate a relative decrease. Error bars depict the standard error.
The F1 model testing changes in vowel height (where a smaller F1 values indicate raising) showed no significant intercept; relative to the citation forms, speakers did not change their vowel height. The model revealed only an effect of Vowel Duration: speakers produce lower vowels (higher F1) with increasing duration [Coef = 2.1e-04, SE = 7.8e-05, t = 2.62, p < 0.01]. No other effects or interactions were significant.
The F2 model, testing changes in vowel backness, showed several significant effects. While there was no significant intercept (indicating no general change in vowel backness from citation form), participants produced more backed vowels (i.e., lower F2 values) with increasing vowel duration [Coef = −1.8e-04, SE = 3.4e-05, t = −5.41, p < 0.001]. There was also an interaction between Misunderstanding Condition and Vowel Category. As seen in Figure 4, back vowels were produced even farther back (lower F2) in response to a staged word misrecognition [Coef = −0.01, SE = 1.5e-03, t = −3.46, p < 0.001]. No other effects or interactions were observed4.
Discussion
The current study examined whether participants use a different speech style when talking to an Alexa interlocutor, relative to a human interlocutor, in a computer-mediated interaction (a summary of the main effects is provided in Table 2). We systematically controlled functional and socio-communicative pressures in real-time during interactions with both interlocutors who made the same types and rates of staged word misrecognitions, and responded in emotionally expressive and neutral manners. This approach serves to complement studies done with users talking to devices in their home (e.g., Mallidi et al., 2018; Huang et al., 2019) and also pinpoint differences that might be present due to other factors in the situation (e.g., physical distance from the microphone; rate and type of automatic speech recognition (ASR) errors). While TTS methods have advanced in recent years (e.g., Wavenet in Van Den Oord et al., 2016), our participants rated the two talkers as distinct in their human-likeness: Alexa was less human-like than the human voice, consistent with prior work (Cohn et al., 2020b; Cohn and Zellou, 2020).
Overall, we found prosodic differences across Alexa- and human-DS, consistent with routinized interaction accounts that propose people have a “routinized” way of engaging with technology (Gambino et al., 2020), and in line with prior studies finding differences in computer and voice-AI speech registers (e.g., Burnham et al., 2010; Huang et al., 2019; Siegert and Krüger, 2021). In the present study, speakers showed a systematic Alexa-DS speech style: when talking to Alexa, speakers produced sentences with a slower rate, higher mean f0, and higher f0 variation, relative to human-DS. These differences align with prior work showing slowed speech rate toward Alexa socialbot (Cohn et al., 2021), increased higher mean f0 in speech toward voice-AI (Raveh et al., 2019), and greater segmental lengthening in computer-DS (Burnham et al., 2010). Furthermore, both an increased mean f0 and f0 variation are consistent with increased vocal effort in response to a presumed communicative barrier; for instance, prior work has reported that speakers produce greater f0 variation in response to a word misrecognition in computer-DS (Vertanen, 2006), as well as higher mean f0 and a larger f0 range in Lombard speech (Brumm and Zollinger, 2011; Marcoux and Ernestus, 2019). Furthermore, in contrast to other work reporting greater intensity in Alexa-DS (Raveh et al., 2019; Siegert and Krüger, 2021), we did not see a difference in intensity in the present study. This might reflect the controlled interaction, where participants were recorded with a head-mounted microphone (such that it was equidistant from their mouths for the entire experiment) and heard amplitude normalized stimuli over headphones. Additionally, the lack of an intensity effect suggests that adjustments in Alexa-DS differ from strict “Lombard” effects (e.g., louder in Brumm and Zollinger, 2011).
While one possibility was that these adjustments reflect alignment toward the Alexa talker, we did not find support for this: acoustic analyses demonstrated that the Alexa productions had lower mean f0 and less f0 variation than the human productions (speech rate did not significantly differ for the Alexa and human productions). Hence, speakers appear to produce more effortful prosodic adjustments in response to an interlocutor with presumed communicative barriers (Clark and Murphy, 1982; Oviatt et al., 1998a; Branigan et al., 2011; Cowan et al., 2015), even while the “actual” misunderstandings were matched across the two talker types.
Do the differences in human- and Alexa-DS reflect distinct functionally oriented speech registers? Examining responses to misrecognized utterances suggests that some of these adjustments might be part of a more general speech intelligibility strategy. When either interlocutor “misheard” the word, participants responded by producing many of the same adjustments they did in Alexa-DS, including slower rate, higher f0, and higher f0 variation. These adjustments are in line with proposals that the speech adjustments people make in communicatively challenging contexts are listener-oriented (Lindblom, 1990; Smiljanić and Bradlow, 2009; Hazan and Baker, 2011). Thus, for these particular features, the adjustments made when there is a local communicative pressure parallel those made globally in Alexa-DS, suggesting that speakers make adjustments following misrecognitions and toward Alexa to improve intelligibility.
Yet, we see other adjustments in response to word misrecognitions not seen globally in Alexa-DS: increased intensity and F2 adjustments. These F2 adjustments, in particular, are predicted based on the type of misunderstanding created in the experimental design: when the interlocutor “misheard” the participant, they always produced the correct target word alongside its minimal pair counterpart which differed in backness (e.g., “mask” (front vowel) vs. “mosque” (back vowel)). Producing back vowels further back is consistent with vowel space expansion. In particular, one possibility is that these F2 adjustments are targeted specifically for clarity, making the vowels more distinct from the distractor minimal pair. This aligns with findings from Stent et al. (2008) who found that speakers repaired misrecognitions of high vowels by a dialogue system (e.g., “deed”) by producing even higher vowels. That the same effect is not seen for front vowels in the current study could come from the dialectal variety of the speakers: participants were California English speakers, a variety with back vowel fronting (Hall-Lew, 2011). Thus, it is possible that there is more room for these speakers to make back vowels more back, rather than to adjust the front vowels, though further work exploring dialect-specific intelligibility strategies can shed light on this question (cf. Clopper et al., 2017; Zellou and Scarborough, 2019). Future work varying vowel height, as well as hyperarticulation of consonants (e.g., flapping vs. /t/ release in Stent et al., 2008) can further explore targeting effects in response to word misunderstandings.
However, if people produce global register differences in speech toward Alexa that parallel those seen in response to misrecognitions, why don’t we see greater speech adjustments in response to misrecognitions made by Alexa? One possible explanation for the similarities is the rate: in the current study, the interlocutors both had staged word misrecognitions in 50% of trials. Related work has shown that rate of misrecognition can change speakers’ global and local adaptations (Oviatt et al., 1998b; Stent et al., 2008); at a high rate of word misrecognitions, speakers might produce more similar intelligibility-related adjustments across interlocutors. Additionally, this high misrecognition rate—as well as random occurrence of the misunderstandings—might be interpreted by the speaker that the listener (human or Alexa) is not benefiting from these adjustments, which might drive similarities. In the current study, speakers might produce a word as clearly as they can and the human/voice-AI listener still misunderstands them half the time. The extent to which these patterns hold at a lower misrecognition rate—or an adaptive misrecognition rate, improving as the speaker produces “clearer” speech—are avenues for future work.
Furthermore, another possible reason for the similar intelligibility adjustments in response to a misunderstanding (in both Alexa- and human-DS) is that the speakers did not have access to information about the source of these perceptual barriers. For example, Hazan and Baker (2011) found that speakers dynamically adjust their speech to improve intelligibility when they are told their listener is hearing them in competing background speakers or as noise-vocoded speech (simulating the auditory effect of cochlear implants), relative to when the listener experienced no barrier. Furthermore, the type of adjustments varied according to the type of barrier (e.g., more f0 adjustments when the listener was in “babble” than “vocoded speech”). In the present study, speakers were left to “guess” what the source of the communicative barrier was, based on observed behavior of the human or voice-AI interlocutor. Indeed, when the speaker does not have information about the listener, adaptations might not be advantageous. For example, computer-DS adaptations have been shown in some work to lead to worse outcomes for some ASR systems, leading to a cycle of misunderstanding (e.g., Wade et al., 1992; for a discussion, see Stent et al., 2008; Oviatt et al., 1998b). Future work examining intelligibility for the intended listener (here, a human or ASR system) can further shed light on the extent local intelligibility adjustments in Alexa- and human-DS are equally beneficial.
Another possible factor why we see similar local intelligibility adjustments in response to misunderstandings (across Alexa- and human-DS) is that the experiment was computer-mediated. Recent work has shown differences in linguistic behavior across contexts: for example, participants show stronger style convergence toward their interlocutor in the in-person condition, relative to a (text-based) computer-mediated interaction (Liao et al., 2018). In line with this possibility, Burnham et al. (2010) found similar adjustments in response to a misrecognition made by a computer- and human-DS (but overall differences in computer-DS, paralleling our findings). At the same time, in the current study, the human-likeness ratings for the interlocutors collected at the end of study suggest that the participants found the interlocutors to be distinct. Future work manipulating rate of misunderstanding and embodiment (Staum Casasanto et al., 2010; Cohn et al., 2020a) can investigate what conditions lead to greater targeted intelligibility strategies for distinct interlocutor types.
We also explored whether emotional expressiveness mediates speech styles for Alexa- and human-DS. Here, we found the same speech adjustments in response to expressiveness by both interlocutors: higher mean f0 in response to utterances containing emotional expressiveness. First, speakers’ overall higher f0 in their sentences does not appear to reflect an alignment toward the interlocutors (who actually produced lower mean f0 in their expressive productions). One possible explanation for the increased f0 following the expressive responses is that it reflects a positivity bias in reaction to stimuli (but see Jing-Schmidt (2007) for work on biases toward negative valence). Indeed, work has shown that smiling is associated with higher mean f0 (Tartter, 1980; Tartter and Braun, 1994) (but we did not see formant shifts, which are also associated with smiled speech, in response to Expressiveness). Here, one explanation for similarities in response to emotion by both interlocutors is that speakers are applying the social behaviors toward voice-AI as they do toward humans, as proposed by technology equivalence accounts (Nass et al., 1994; Nass et al., 1997; Lee, 2008). For instance, here people are reacting to emotional expressiveness by both types of interlocutors similarly. This explanation is consistent with work showing similar affective responses to computers as seen in human-human interaction (e.g., Brave et al., 2005; Cohn et al., 2019a; Cohn and Zellou, 2019).
Additionally, we did not observe differences in how participants adapted their speech following an emotionally expressive or neutral word misrecognition. This contrasts with related work on this same corpus (Zellou and Cohn, 2020) that found greater vowel duration alignment when participants responded to an emotionally expressive word misunderstanding made by a voice-AI system. Thus, it is possible that emotional expressiveness might shape vocal alignment, but it might not influence speech style adjustments. That emotion appears to have an effect on vocal alignment toward humans and voice-AI (e.g., Vaughan et al., 2018; Cohn and Zellou, 2019) could be explained by proposals that alignment is used as a means to communicate social closeness (Giles et al., 1991). While conveying affect is thought to be part of infant- and pet-DS registers (Trainor et al., 2000), listener-oriented speech styles directed toward human adults (non-native speakers, hearing impaired speakers) and computers are generally not associated with increased emotionality. Furthermore, conveying affect is generally not associated with clear speech strategies. Indeed, classic perspectives on clear speech (H&H theory) do not account for emotionality in predicting hyperspeech behavior (e.g., Lindblom, 1990). Yet, one possibility for a lack of difference in the current study is based on how emotion was added in the stimuli: emotional expressiveness was conveyed only in the interjection. Since the time this study was run, there are now more ways to adapt the Alexa voice in terms of positive and negative emotionality (at low, medium, and high levels5), which can serve as avenues for future research.
There were also several limitations of the present study which open directions for future work. For instance, one possible factor in the lack of difference detected for emotionality across Alexa- and human-DS is the communicative context: the current study consisted of fully scripted interactions in a lab setting. While this controlled interaction was intentional as we were interested in word misrecognitions (which might otherwise be difficult to control in voice-AI interactions), it is possible that differences based on emotional expressiveness might be seen in a non-scripted conversation with voice-AI, as well as one conducted outside a lab context (e.g., Cohn et al., 2019b). Additionally, the present study used two types of voices; it is possible that other paralinguistic features of those voices might have mediated speech style adjustments. For example, recent work has shown that speakers align speech differently toward TTS voices that “sound” older (e.g., Apple’s Siri voices, rated in their 40 and 50s) (Zellou et al., 2021). Furthermore, there is work showing that introducing “charismatic” features from human speakers’ voices shapes perception of TTS voices (Fischer et al., 2019; Niebuhr and Michalsky, 2019). The extent to which individual differences in speakers (human and TTS) and participants remain avenues for future research.
While here the findings align with those for another Germanic language (e.g., German in Raveh et al., 2019; Siegert and Krüger, 2021), the extent to which the same effects might be observed with other languages and other cultures is another open question for future work. For example, cultures might vary in terms of acceptance of voice-AI technology, such as due to privacy concerns (e.g., GDPR in Europe: Voss, 2016; Loideain and Adams, 2020). Additionally, cultures vary in terms of their expressions of emotion (Shaver et al., 1992; Mesquita and Markus, 2004; Van Hemert et al., 2007). How emotional expressiveness and “trust” in voice-AI (Shulevitz, 2018; Metcalf et al., 2019) might interact remains an open question for future work.
Conclusion
Overall, this work adds to our growing understanding of the dynamics of human interaction with voice-AI assistants—still distinct from how individuals talk to human interlocutors. As these systems and other AI robotics systems are even more widely adopted, characterizing these patterns across different timepoints—and with diverse populations of participants—is important in our ability to track the trajectory of the influence of voice-AI on humans and human speech across languages and cultures.
Data Availability Statement
The original contributions presented in the study are included in the Supplementary Material, further inquiries can be directed to the corresponding author.
Ethics Statement
The studies involving human participants were reviewed and approved by the University of California Institutional Review Board (IRB). The participants provided their written informed consent to participate in this study.
Author Contributions
MC and GZ contributed to conception and design of the study. MC programmed the experiment and performed the statistical analysis. Both authors contributed to manuscript drafting and revision. Both have read, and approved the submitted version.
Funding
This material is based upon work supported by the National Science Foundation SBE Postdoctoral Research Fellowship under Grant No. 1911855 to MC and an Amazon Faculty Research Award to GZ.
Conflict of Interest
The authors declare that the research was conducted in the absence of any commercial or financial relationships that could be construed as a potential conflict of interest.
Publisher’s Note
All claims expressed in this article are solely those of the authors and do not necessarily represent those of their affiliated organizations, or those of the publisher, the editors and the reviewers. Any product that may be evaluated in this article, or claim that may be made by its manufacturer, is not guaranteed or endorsed by the publisher.
Supplementary Material
The Supplementary Material for this article can be found online at: https://www.frontiersin.org/articles/10.3389/fcomm.2021.675704/full#supplementary-material
Footnotes
1They do not report a directionality of difference.
2https://developer.amazon.com/en-US/docs/alexa/custom-skills/speechcon-reference-interjections-english-us.html
4Note that while there is a numerical F2 increase in the Front Vowels in response to Misrecognized Expressive productions, this was not significant in the main model or in a post hoc model (with the subset of Front Vowels).
5https://developer.amazon.com/en-US/docs/alexa/custom-skills/speech-synthesis-markup-language-ssml-reference.html#amazon-emotion
References
Ameka, F. (1992). Interjections: The Universal yet Neglected Part of Speech. J. Pragmatics 18 (2–3), 101–118. doi:10.1016/0378-2166(92)90048-g
Ammari, T., Kaye, J., Tsai, J. Y., and Bentley, F. (2019). Music, Search, and IoT. ACM Trans. Comput.-Hum. Interact. 26 (3), 1–28. doi:10.1145/3311956
Arnold, R., Tas, S., Hildebrandt, C., and Schneider, A. (2019). “Any Sirious Concerns yet?–An Empirical Analysis of Voice Assistants’ Impact on Consumer Behavior and Assessment of Emerging Policy,” in TPRC47: Research Conference on Communications, Information and Internet Policy, Washington, DC, September 20–21, 2019.
Babel, M. (2012). Evidence for Phonetic and Social Selectivity in Spontaneous Phonetic Imitation. J. Phonetics 40 (1), 177–189. doi:10.1016/j.wocn.2011.09.001
Bates, D., Mächler, M., Bolker, B., and Walker, S. (2015). Fitting Linear Mixed-Effects Models Using Lme4. J. Stat. Soft. 67 (1), 1–48. doi:10.18637/jss.v067.i01
Bell, L., Gustafson, J., and Heldner, M. (2003). “Prosodic Adaptation in Human-Computer Interaction,” in Proceedings of the 15th International Congress of Phonetic Sciences, Vol. 3, 833–836.
Bell, L., and Gustafson, J. (1999). Repetition and its Phonetic Realizations: Investigating a Swedish Database of Spontaneous Computer-Directed Speech. Proc. ICPhS 99, 1221–1224.
Bentley, F., Luvogt, C., Silverman, M., Wirasinghe, R., White, B., and Lottridge, D. (2018). Understanding the Long-Term Use of Smart Speaker Assistants. Proc. ACM Interact. Mob. Wearable Ubiquitous Technol. 2 (3), 1–24. doi:10.1145/3264901
Branigan, H. P., Pickering, M. J., Pearson, J., McLean, J. F., and Brown, A. (2011). The Role of Beliefs in Lexical Alignment: Evidence from Dialogs with Humans and Computers. Cognition 121 (1), 41–57. doi:10.1016/j.cognition.2011.05.011
Brave, S., Nass, C., and Hutchinson, K. (2005). Computers that Care: Investigating the Effects of Orientation of Emotion Exhibited by an Embodied Computer Agent. Int. J. Human-Computer Stud. 62 (2), 161–178. doi:10.1016/j.ijhcs.2004.11.002
Brumm, H., and Zollinger, S. A. (2011). The Evolution of the Lombard Effect: 100 Years of Psychoacoustic Research. Behav. 148 (11–13), 1173–1198. doi:10.1163/000579511x605759
Burbach, L., Halbach, P., Plettenberg, N., Nakayama, J., Ziefle, M., and Valdez, A. C. (2019). ““ Hey, Siri”,“ Ok, Google”,“ Alexa”. Acceptance-Relevant Factors of Virtual Voice-Assistants,” in 2019 IEEE International Professional Communication Conference (ProComm), Aachen, Germany, July 2019 (IEEE), 101–111. doi:10.1109/procomm.2019.00025
Burnham, D. K., Joeffry, S., and Rice, L. (2010). “Computer-and Human-Directed Speech before and after Correction,” in Proceedings of the 13th Australasian International Conference on Speech Science and Technology, Melbourne, Australia, December 14–16, 2010, 13–17. Available at: http://handle.uws.edu.au:8081/1959.7/504796.
Clark, H. H., and Murphy, G. L. (1982). “Audience Design in Meaning and Reference,” in Advances in Psychology. Editors J. F. Le Ny, and W. Kintsch (North-Holland, The Netherlands: Elsevier), Vol. 9, 287–299. doi:10.1016/s0166-4115(09)60059-5
Clark, H. H. (1996). Using Language. Cambridge, United Kingdom: Cambridge University Press. doi:10.1017/cbo9780511620539
Clopper, C. G., Mitsch, J. F., and Tamati, T. N. (2017). Effects of Phonetic Reduction and Regional Dialect on Vowel Production. J. Phonetics 60, 38–59. doi:10.1016/j.wocn.2016.11.002
Cohn, M., Chen, C.-Y., and Yu, Z. (2019a). “A Large-Scale User Study of an Alexa Prize Chatbot: Effect of TTS Dynamism on Perceived Quality of Social Dialog,” in Proceedings of the 20th Annual SIGdial Meeting on Discourse and Dialogue, Stockholm, Sweden, September 11–13, 2019 (Association for Computational Linguistics), 293–306. Available at: https://www.sigdial.org/files/workshops/conference20/proceedings/cdrom/pdf/W19-5935.pdf.
Cohn, M., Ferenc Segedin, B., and Zellou, G. (2019b). “Imitating Siri: Socially-Mediated Alignment to Device and Human Voices,” in Proceedings of International Congress of Phonetic Sciences, Melbourne, Australia, August 5–9, 2019, 1813–1817. Available at: https://icphs2019.org/icphs2019-fullpapers/pdf/full-paper_202.pdf.
Cohn, M., Jonell, P., Kim, T., Beskow, J., and Zellou, G. (2020a). “Embodiment and Gender Interact in Alignment to TTS Voices,” in Proceedings of the Cognitive Science Society, Toronto, Canada, July 31–August 1, 2020, 220–226. Available at: https://cogsci.mindmodeling.org/2020/papers/0044/0044.pdf.
Cohn, M., Liang, K.-H., Sarian, M., Zellou, G., and Yu, Z. (2021). Speech Rate Adjustments in Conversations with an Amazon Alexa Socialbot. Front. Commun. 6, 1–8. doi:10.3389/fcomm.2021.671429
Cohn, M., Sarian, M., Predeck, K., and Zellou, G. (2020b). “Individual Variation in Language Attitudes toward Voice-AI: The Role of Listeners’ Autistic-like Traits,” in Proceedings of the Interspeech 2020, Shanghai, China, October 25–29, 2020, 1813–1817. doi:10.21437/Interspeech.2020-1339
Cohn, M., and Zellou, G. (2019). “Expressiveness Influences Human Vocal Alignment toward Voice-AI,” in Proceedings of the Interspeech 2019, Graz, Austria, September 15–19, 2019, 41–45. doi:10.21437/Interspeech.2019-1368
Cohn, M., and Zellou, G. (2020). “Perception of Concatenative vs. Neural Text-To-Speech (TTS): Differences in Intelligibility in Noise and Language Attitudes,” in Proceedings of the Interspeech, Shanghai, China, October 25–29, 2020, 1733–1737. doi:10.21437/Interspeech.2020-1336
Cowan, B. R., Branigan, H. P., Obregón, M., Bugis, E., and Beale, R. (2015). Voice Anthropomorphism, Interlocutor Modelling and Alignment Effects on Syntactic Choices in Human−computer Dialogue. Int. J. Human-Computer Stud. 83, 27–42. doi:10.1016/j.ijhcs.2015.05.008
De Jong, N. H., Wempe, T., Quené, H., and Persoon, I. (2017). Praat Script Speech Rate V2. Available at: https://sites.google.com/site/speechrate/Home/praat-script-syllable-nuclei-v2 (Accessed August 30, 2020).
DiCanio, C. (2007). Extract Pitch Averages. Available at: https://www.acsu.buffalo.edu/∼cdicanio/scripts/Get_pitch.praat (Accessed May 12, 2019).
Fischer, K., Niebuhr, O., Jensen, L. C., and Bodenhagen, L. (2019). Speech Melody Matters—How Robots Profit from Using Charismatic Speech. ACM Trans. Human-Robot Interaction (Thri) 9 (1), 1–21. doi:10.1145/3344274
Gambino, A., Fox, J., and Ratan, R. A. (2020). Building a Stronger CASA: Extending the Computers Are Social Actors Paradigm. Human-Machine Commun. 1 (1), 5. doi:10.30658/hmc.1.5
Giles, H., Coupland, N., and Coupland, J. (1991). “Accommodation Theory: Communication, Context, and Consequence,” in Contexts of Accommodation (Cambridge, United Kingdom: Cambridge University Press), Vol. 1, 1–68.
Goffman, E. (1981). Forms of Talk. Philadelphia, PA: University of Pennsylvania Press, 78–122. Response Cries.
Habler, F., Schwind, V., and Henze, N. (2019). “Effects of Smart Virtual Assistants’ Gender and Language,” in Proceedings of Mensch und Computer 2019, Hamburg, Germany, September 8–11, 2019, 469–473. doi:10.1145/3340764.3344441
Hall-Lew, L. (2011). “The Completion of a Sound Change in California English,” in Proceedings of ICPhS XVII, Hong Kong, China, August 17–21, 2011, 807–810.
Hazan, V., and Baker, R. (2011). Acoustic-phonetic Characteristics of Speech Produced with Communicative Intent to Counter Adverse Listening Conditions. The J. Acoust. Soc. America 130 (4), 2139–2152. doi:10.1121/1.3623753
Hazan, V. L., Uther, M., and Granlund, S. (2015). “How Does Foreigner-Directed Speech Differ from Other Forms of Listener-Directed clear Speaking Styles?,” in Proceedings of the 18th International Congress of Phonetic Sciences, Glasgow, United Kingdom, August 10–14, 2015.
Hoffmann, L., Krämer, N. C., Lam-Chi, A., and Kopp, S. (2009). “Media Equation Revisited: Do Users Show Polite Reactions towards an Embodied Agent?,” in International Workshop on Intelligent Virtual Agents, Amsterdam, The Netherlands, September 14–16, 2009, 159–165. doi:10.1007/978-3-642-04380-2_19
Huang, C.-W., Maas, R., Mallidi, S. H., and Hoffmeister, B. (2019). “A Study for Improving Device-Directed Speech Detection toward Frictionless Human-Machine Interaction,” in Proceeding of the Interspeech 2019, Graz, Austria, September 15–19, 2019, 3342–3346. doi:10.21437/Interspeech.2019-2840
Jing-Schmidt, Z. (2007). Negativity Bias in Language: A Cognitive-Affective Model of Emotive Intensifiers. Cogn. Linguist. 18(3), 417–443. doi:10.1515/COG.2007.023
Junqua, J.-C. (1996). The Influence of Acoustics on Speech Production: A Noise-Induced Stress Phenomenon Known as the Lombard Reflex. Speech Commun. 20 (1–2), 13–22. doi:10.1016/s0167-6393(96)00041-6
Junqua, J. C. (1993). The Lombard Reflex and its Role on Human Listeners and Automatic Speech Recognizers. J. Acoust. Soc. America 93 (1), 510–524. doi:10.1121/1.405631
Knoll, M. A., Johnstone, M., and Blakely, C. (2015). “Can You Hear Me? Acoustic Modifications in Speech Directed to Foreigners and Hearing-Impaired People,” in Sixteenth Annual Conference of the International Speech Communication Association, Dresden, Germany, September 6–10, 2015. Available at: https://www.isca-speech.org/archive/interspeech_2015/i15_2987.html.
Lee, K. M. (2008). “Media Equation Theory,” in The International Encyclopedia of Communication (Malden, MA: John Wiley & Sons, Ltd), Vol. 1, 1–4. doi:10.1002/9781405186407.wbiecm035
Liao, W., Bazarova, N. N., and Yuan, Y. C. (2018). Expertise Judgment and Communication Accommodation in Linguistic Styles in Computer-Mediated and Face-To-Face Groups. Commun. Res. 45 (8), 1122–1145. doi:10.1177/0093650215626974
Lindblom, B. (1990). “Explaining Phonetic Variation: A Sketch of the H&H Theory,” in Speech Production and Speech Modelling. Editors W. J. Hardcastle, and A. Marchal (Dordrecht, The Netherlands: Springer), Vol. 55, 403–439. doi:10.1007/978-94-009-2037-8_16
Loideain, N. N., and Adams, R. (2020). From Alexa to Siri and the GDPR: the Gendering of Virtual Personal Assistants and the Role of Data protection Impact Assessments. Comp. L. Security Rev. 36, 105366. doi:10.1016/j.clsr.2019.105366
Lopatovska, I. (2020). “Personality Dimensions of Intelligent Personal Assistants,” in Proceedings of the 2020 Conference on Human Information Interaction and Retrieval, Vancouver, BC, Canada, March 14–18, 2020, 333–337. doi:10.1145/3343413.3377993
Lunsford, R., Oviatt, S., and Arthur, A. M. (2006). “Toward Open-Microphone Engagement for Multiparty Interactions,” in Proceedings of the 8th International Conference on Multimodal Interfaces, New York, NY, November 2–4, 2006, 273–280. doi:10.1145/1180995.1181049
Mallidi, S. H., Maas, R., Goehner, K., Rastrow, A., Matsoukas, S., and Hoffmeister, B. (2018). Device-directed Utterance Detection. Hyderabad, India: Interspeech 2018ISCA. doi:10.21437/interspeech.2018-1531
Maniwa, K., Jongman, A., and Wade, T. (2009). Acoustic Characteristics of Clearly Spoken English Fricatives. J. Acoust. Soc. America 125 (6), 3962–3973. doi:10.1121/1.2990715
Marcoux, K. P., and Ernestus, M. T. C. (2019). “Differences between Native and Non-native Lombard Speech in Terms of Pitch Range,” in Proceedings of the 23rd International Congress on Acoustics, Berlin, Germany, September 9–13, 2019, 5713–5720. doi:10.18154/rwth-conv-239240
Mayo, C., Aubanel, V., and Cooke, M. (2012). “Effect of Prosodic Changes on Speech Intelligibility,” in Thirteenth Annual Conference of the International Speech Communication Association, Portland, OR, September 9-13, 2012, 1706–1709. Available at: https://isca-speech.org/archive/archive_papers/interspeech_2012/i12_1708.pdf.
Mesquita, B., and Markus, H. R. (2004). “Culture and Emotion,” in Feelings and Emotions:The Amsterdam Symposium. Editors A. S. R. Manstead, N. Frijda, and A. Fischer (Cambridge, United Kingdom: Cambridge University Press), 341–358. doi:10.1017/cbo9780511806582.020
Metcalf, K., Theobald, B.-J., Weinberg, G., Lee, R., Jonsson, M., Webb, R., et al. (2019). “Mirroring to Build Trust in Digital Assistants,” in Proc. Interspeech, Graz, Austria, September 15–19, 2019, 4000–4004. doi:10.21437/Interspeech.2019-1829
Nass, C., Moon, Y., Morkes, J., Kim, E.-Y., and Fogg, B. J. (1997). Computers Are Social Actors: A Review of Current Research. Hum. Values Des. Comp. Tech. 72, 137–162.
Nass, C., Steuer, J., and Tauber, E. R. (1994). “Computers Are Social Actors,” in Proceedings of the SIGCHI Conference on Human Factors in Computing Systems, Boston, MA, April 24–28, 1994, 72–78. doi:10.1145/259963.260288
Nearey, T. M. (1978). Phonetic Feature Systems for Vowels. Bloomington, IN: Indiana University Linguistics Club.
Niebuhr, O., and Michalsky, J. (2019). “Computer-Generated Speaker Charisma and its Effects on Human Actions in a Car-Navigation System Experiment - or How Steve Jobs' Tone of Voice Can Take You Anywhere,” in International Conference on Computational Science and Its Applications, Saint Petersburg, Russia, July 1–4, 2019, 375–390. doi:10.1007/978-3-030-24296-1_31
Oviatt, S., Levow, G.-A., Moreton, E., and MacEachern, M. (1998a). Modeling Global and Focal Hyperarticulation during Human-Computer Error Resolution. J. Acoust. Soc. America 104 (5), 3080–3098. doi:10.1121/1.423888
Oviatt, S., MacEachern, M., and Levow, G.-A. (1998b). Predicting Hyperarticulate Speech during Human-Computer Error Resolution. Speech Commun. 24 (2), 87–110. doi:10.1016/s0167-6393(98)00005-3
Picheny, M. A., Durlach, N. I., and Braida, L. D. (1985). Speaking Clearly for the Hard of Hearing I. J. Speech Lang. Hear. Res. 28 (1), 96–103. doi:10.1044/jshr.2801.96
Pluymaekers, M., Ernestus, M., and Baayen, R. H. (2005). Lexical Frequency and Acoustic Reduction in Spoken Dutch. J. Acoust. Soc. America 118 (4), 2561–2569. doi:10.1121/1.2011150
Purington, A., Taft, J. G., Sannon, S., Bazarova, N. N., and Taylor, S. H. (2017). “‘Alexa is My New BFF’: Social Roles, User Satisfaction, and Personification of the Amazon Echo,” in Proceedings of the 2017 CHI Conference Extended Abstracts on Human Factors in Computing Systems, Denver, CO, May 6–11, 2017, 2853–2859. doi:10.1145/3027063.3053246
Raveh, E., Steiner, I., Siegert, I., Gessinger, I., and Möbius, B. (2019). “Comparing Phonetic Changes in Computer-Directed and Human-Directed Speech,” in Studientexte Zur Sprachkommunikation: Elektronische Sprachsignalverarbeitung, Dresden, Germany, March 3–8, 2019, 42–49.
Rosenfelder, I., Fruehwald, J., Evanini, K., Seyfarth, S., Gorman, K., Prichard, H., et al. (2014). FAVE (Forced Alignment and Vowel Extraction). Program Suite v1. 2.2. doi:10.5281/zenodo.9846 (Accessed July 13, 2020).
Scarborough, R., Dmitrieva, O., Hall-Lew, L., Zhao, Y., and Brenier, J. (2007). “An Acoustic Study of Real and Imagined Foreigner-Directed Speech,” in Proceedings of the International Congress of Phonetic Sciences, Saarbrücken, Germany, August 6–10, 2007, 2165–2168. Available at: http://icphs2007.de/conference/Papers/1673/1673.pdf.
Scarborough, R., and Zellou, G. (2013). Clarity in Communication: "Clear" Speech Authenticity and Lexical Neighborhood Density Effects in Speech Production and Perception. J. Acoust. Soc. America 134 (5), 3793–3807. doi:10.1121/1.4824120
Shaver, P. R., Wu, S., and Schwartz, J. C. (1992). “Cross-cultural Similarities and Differences in Emotion and its Representation,” in Emotion (Thousand Oaks, CA:Sage Publications, Inc), 175–212. doi:10.21236/ada248148
Shulevitz, J. (2018). “Alexa, How Will You Change Us,” in The Atlantic, Vol. 322, 94–104. Available at: https://www.theatlantic.com/magazine/archive/2018/11/alexa-how-will-you-change-us/570844/.
Siegert, I., Krüger, J., Egorow, O., Nietzold, J., Heinemann, R., and Lotz, A. (2018). “Voice Assistant Conversation Corpus (VACC): A Multi-Scenario Dataset for Addressee Detection in Human-Computer-Interaction Using Amazon’s ALEXA,” in Proceedings of the Eleventh International Conference on Language Resources and Evaluation (LREC 2018), Paris, France, May 7–12, 2018. Available at: http://lrec-conf.org/workshops/lrec2018/W20/pdf/13_W20.pdf.
Siegert, I., and Krüger, J. (2021). “Speech Melody and Speech Content Didn't Fit Together"-Differences in Speech Behavior for Device Directed and Human Directed Interactions,” in Advances in Data Science: Methodologies and Applications. Editors G. Phillips-Wren, A. Esposito, and L. C. Jain (Switzerland: Springer), Vol. 189, 65–95. doi:10.1007/978-3-030-51870-7_4
Siegert, I., Nietzold, J., Heinemann, R., and Wendemuth, A. (2019). “The Restaurant Booking Corpus–Content-Identical Comparative Human-Human and Human-Computer Simulated Telephone Conversations,” in Studientexte Zur Sprachkommunikation: Elektronische Sprachsignalverarbeitung, 126–133.
Smiljanić, R., and Bradlow, A. R. (2009). Speaking and Hearing Clearly: Talker and Listener Factors in Speaking Style Changes. Lang. Linguist Compass 3 (1), 236–264. doi:10.1111/j.1749-818X.2008.00112.x
Staum Casasanto, L., Jasmin, K., and Casasanto, D. (2010). “Virtually Accommodating: Speech Rate Accommodation to a Virtual Interlocutor,” in 32nd Annual Meeting of the Cognitive Science Society (CogSci 2010), Portland, OR, August 11–14, 2010, 127–132. Available at: http://hdl.handle.net/11858/00-001M-0000-0012-BE12-A.
Stent, A. J., Huffman, M. K., and Brennan, S. E. (2008). Adapting Speaking after Evidence of Misrecognition: Local and Global Hyperarticulation. Speech Commun. 50 (3), 163–178. doi:10.1016/j.specom.2007.07.005
Swerts, M., Litman, D., and Hirschberg, J. (2000). “Corrections in Spoken Dialogue Systems,” in Sixth International Conference on Spoken Language Processing, Beijing, China, October 16–20, 2000. Available at: https://www.isca-speech.org/archive/icslp_2000/i00_2615.html.
Tartter, V. C., and Braun, D. (1994). Hearing Smiles and Frowns in normal and Whisper Registers. J. Acoust. Soc. America 96 (4), 2101–2107. doi:10.1121/1.410151
Tartter, V. C. (1980). Happy Talk: Perceptual and Acoustic Effects of Smiling on Speech. Perception & Psychophysics 27 (1), 24–27. doi:10.3758/bf03199901
Trainor, L. J., Austin, C. M., and Desjardins, R. N. (2000). Is Infant-Directed Speech Prosody a Result of the Vocal Expression of Emotion? Psychol. Sci. 11 (3), 188–195. doi:10.1111/1467-9280.00240
Uther, M., Knoll, M. A., and Burnham, D. (2007). Do you Speak E-NG-L-I-SH? A Comparison of Foreigner- and Infant-Directed Speech. Speech Commun. 49 (1), 2–7. doi:10.1016/j.specom.2006.10.003
Van Den Oord, A., Dieleman, S., Zen, H., Simonyan, K., Vinyals, O., Graves, A., et al. (2016). WaveNet: A Generative Model for Raw Audio. arXiv. ArXiv Prepr. ArXiv160903499.
Van Hemert, D. A., Poortinga, Y. H., and van de Vijver, F. J. R. (2007). Emotion and Culture: A Meta-Analysis. Cogn. Emot. 21 (5), 913–943. doi:10.1080/02699930701339293
Vaughan, B., De Pasquale, C., Wilson, L., Cullen, C., and Lawlor, B. (2018). “Investigating Prosodic Accommodation in Clinical Interviews with Depressed Patients,” in International Symposium on Pervasive Computing Paradigms for Mental Health, Boston, January 9–10, 2018, 150–159. doi:10.1007/978-3-030-01093-5_19
Vertanen, K. (2006). “Speech and Speech Recognition during Dictation Corrections,” in Ninth International Conference on Spoken Language Processing, 1890–1893.
Voss, W. G. (2016). European union Data Privacy Law Reform: General Data protection Regulation, Privacy Shield, and the Right to Delisting. The Business Lawyer 72 (1), 221–234.
Wade, E., Shriberg, E., and Price, P. (1992). “User Behaviors Affecting Speech Recognition,” in Second International Conference on Spoken Language Processing, Banff, Alberta, Canada, October 13–16, 1992. Available at: https://www.isca-speech.org/archive/archive_papers/icslp_1992/i92_0995.pdf.
Zellou, G., Cohn, M., and Ferenc Segedin, B. (2021). Age- and Gender-Related Differences in Speech Alignment toward Humans and Voice-AI. Front. Commun. 5, 1–11. doi:10.3389/fcomm.2020.600361
Zellou, G., and Cohn, M. (2020). “Social and Functional Pressures in Vocal Alignment: Differences for Human and Voice-AI Interlocutors,” in Proceeding of the Interspeech 2020, Shanghai, China, October 25–29, 2020, 1634–1638. doi:10.21437/Interspeech.2020-1335
Keywords: voice-activated artificially intelligent (voice-AI) assistant, speech register, human-computer interaction, computer personification, speech intelligibility
Citation: Cohn M and Zellou G (2021) Prosodic Differences in Human- and Alexa-Directed Speech, but Similar Local Intelligibility Adjustments. Front. Commun. 6:675704. doi: 10.3389/fcomm.2021.675704
Received: 03 March 2021; Accepted: 21 June 2021;
Published: 29 July 2021.
Edited by:
Ingo Siegert, Otto von Guericke University Magdeburg, GermanyReviewed by:
Oliver Niebuhr, University of Southern Denmark, DenmarkHendrik Buschmeier, Bielefeld University, Germany
Volker Dellwo, University of Zurich, Switzerland
Copyright © 2021 Cohn and Zellou. This is an open-access article distributed under the terms of the Creative Commons Attribution License (CC BY). The use, distribution or reproduction in other forums is permitted, provided the original author(s) and the copyright owner(s) are credited and that the original publication in this journal is cited, in accordance with accepted academic practice. No use, distribution or reproduction is permitted which does not comply with these terms.
*Correspondence: Michelle Cohn, bWRjb2huQHVjZGF2aXMuZWR1