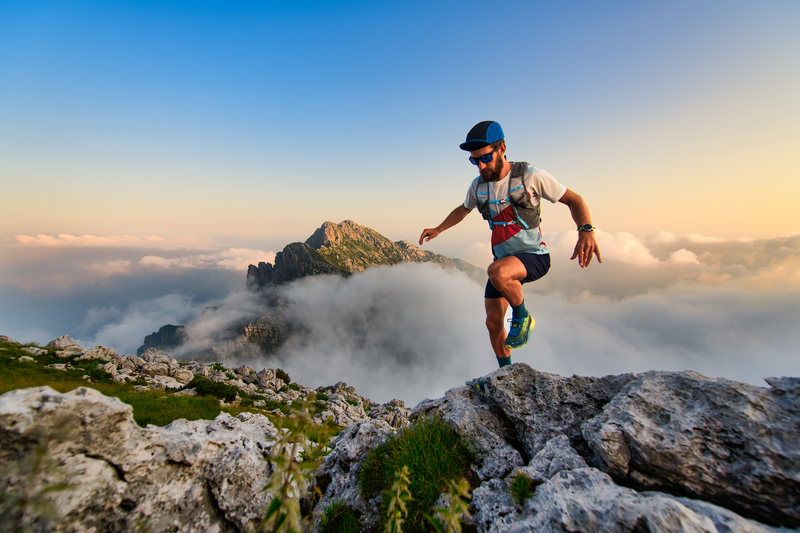
94% of researchers rate our articles as excellent or good
Learn more about the work of our research integrity team to safeguard the quality of each article we publish.
Find out more
BRIEF RESEARCH REPORT article
Front. Commun. , 07 May 2021
Sec. Psychology of Language
Volume 6 - 2021 | https://doi.org/10.3389/fcomm.2021.671429
This article is part of the Research Topic Towards Omnipresent and Smart Speech Assistants View all 12 articles
This paper investigates users’ speech rate adjustments during conversations with an Amazon Alexa socialbot in response to situational (in-lab vs. at-home) and communicative (ASR comprehension errors) factors. We collected user interaction studies and measured speech rate at each turn in the conversation and in baseline productions (collected prior to the interaction). Overall, we find that users slow their speech rate when talking to the bot, relative to their pre-interaction productions, consistent with hyperarticulation. Speakers use an even slower speech rate in the in-lab setting (relative to at-home). We also see evidence for turn-level entrainment: the user follows the directionality of Alexa’s changes in rate in the immediately preceding turn. Yet, we do not see differences in hyperarticulation or entrainment in response to ASR errors, or on the basis of user ratings of the interaction. Overall, this work has implications for human-computer interaction and theories of linguistic adaptation and entrainment.
After their introduction in the 2010s, there has been a widespread adoption of voice-activated artificially intelligent (voice-AI) assistants (e.g., Google Assistant, Amazon’s Alexa, Apple’s Siri), particularly within the United States (Bentley et al., 2018). Millions of users now speak to voice-AI to complete daily tasks (e.g., play music, turn on lights, set timers) (Ammari et al., 2019). Given their presence in many individuals’ everyday lives, some researchers have aimed to uncover the cognitive, social, and linguistic factors involved in voice-AI interactions by examining task-based interactions with voice-AI (e.g., setting an appointment on a calendar in Raveh et al., 2019), scripted interactions in laboratory settings (Cohn et al., 2019; Zellou et al., 2021), and interviews to probe how people perceive voice-AI (Lovato and Piper, 2015; Purington et al., 2017; Abdolrahmani et al., 2018). Yet, our scientific understanding of non-task based, or purely social, interactions with voice-AI is even less established.
Since 2017, the Amazon Alexa Prize competition has served as a venue for social chit-chat between users and Amazon Alexa socialbots on any Alexa-enabled device; with a simple command, “Alexa, let’s chat”, any user can talk to one of several university-designed socialbots (Chen et al., 2018; Ram et al., 2018; Gabriel et al., 2020; Liang et al., 2020). Do individuals talk to these socialbots in similar ways as they do with real humans? The Computers are Social Actors (CASA; Nass et al., 1997; Nass et al., 1994) framework proposes that people apply socially mediated, ‘rules’, from human-human interaction to computers when they detect a cue of ‘humanity’ in the system. Voice-AI systems are already imbued with multiple human-like features: they have names, apparent genders Habler et al. (2019) and interact with users using spoken language. Indeed, there is some evidence that individuals engage with voice-AI in ways that parallel the ways they engage with humans (e.g., gender-asymmetries in phonetic alignment in Cohn et al., 2019; Zellou et al., 2021). In the case of voice-AI socialbots, the cues of humanity could be even more robust since the system is designed for social interaction.
To uncover some of the cognitive and linguistic factors in how users perceive voice-AI socialbots, the current study examines two speech behaviors: ‘hyperarticulation’ and ‘entrainment’. We define ‘hyperarticulation’ as carefully articulated speech (also referred to as ‘clear’ speech; Smiljanić and Bradlow, 2009), thought by listener-oriented accounts to be tailored specifically to improve intelligibility for an interlocutor in the conversation (Lindblom, 1990). For example, there is a body of work examining acoustic adjustments speakers make when talking to computer systems, or ‘computer-directed speech’ (computer-DS) (Oviatt et al., 1998a; Oviatt et al., 1998b; Bell and Gustafson, 1999; Bell et al., 2003; Lunsford et al., 2006; Stent et al., 2008; Burnham et al., 2010; Mayo et al., 2012; Siegert et al., 2019). A common listener-oriented hyperarticulation is to slow speaking rate, produced in response to background noise (Brumm and Zollinger, 2011), as well as in interactions with interlocutors assumed to be less communicatively competent, such as computers (Oviatt et al., 1998b; Stent et al., 2008), infants (Fernald and Simon, 1984), and non-native speakers (Scarborough et al., 2007; Lee and Baese-Berk, 2020). Will users also slow their speech rate when they talk to a socialbot? One possibility that the advanced speech capabilities in Alexa socialbots (in terms of speech recognition, language understanding and generation) might lead to more naturalistic interactions, whereby users talk to the system more as they would an adult human interlocutor. Alternatively, there is work showing that listeners rate ‘robotic’ text-to-speech (TTS) voices as less communicatively competent than more human-like voices (Cowan et al., 2015) and that listeners perceive prosodic peculiarities in the Alexa voice, describing it as being ‘monotonous’ and ‘robotic’ (Siegert and Krüger, 2020). Accordingly, an alternative prediction is that speakers will use a slower speaking rate when talking to the Alexa socialbot, since robotic voices are perceived as being less communicatively competent.
In addition to hyperarticulation, we examine ‘entrainment’ (also known as ‘accommodation’, ‘alignment’, or ‘imitation’): the tendency for speakers to adopt their interlocutor’s voice and language patterns. For example, a speaker might increase their speech rate in response to hearing the socialbot’s speech rate increase. Entrainment has been previously observed both in human-human (Levitan and Hirschberg, 2011; Babel and Bulatov, 2012; Lubold and Pon-Barry, 2014; Levitan et al., 2015; Pardo et al., 2017) and human-computer interaction (Coulston et al., 2002; Bell et al., 2003; Branigan et al., 2011; Fandrianto and Eskenazi, 2012; Thomason et al., 2013; Cowan et al., 2015; Gessinger et al., 2017; Gessinger et al., 2021), suggesting it is a behavior transferred to interactions with technology. Recent work has shown that entrainment occurs in interactions with voice-AI assistants as well (Cohn et al., 2019; Raveh et al., 2019; Zellou et al., 2021). Like hyperarticulation, there are some accounts proposing that entrainment improves intelligibility (Pickering and Garrod, 2006), aligning representations between interlocutors. For example, people entrain toward the lexical and syntactic patterns of computers, lessening (presumed) communicative barriers (Branigan et al., 2011; Cowan et al., 2015). At the same time, entrainment can also reveal social attitudes: social accounts of alignment propose that people converge to convey social closeness and diverge to signal distance (Giles et al., 1991; Shepard et al., 2001), such as entraining more to interlocutors they like (Chartrand and Bargh, 1996; Levitan et al., 2012). In the current study, we predict that speakers who rate the socialbot more positively will also show more entrainment toward it.
While the vast majority of prior work examines hyperarticulation and entrainment separately (e.g., Burnham et al., 2010; Cohn et al., 2019), the current study models these behaviors in tandem. This is important as hyperarticulation and entrainment might both result in the same observed behavior: a speaker might speak slower when talking to the socialbot overall (hyperarticulation), but also slow in response to a slower speech rate by the bot (entrainment). Including both in the same model allows us to attribute observed behavior to its underlying cognitive processes. This is also important as hyperarticulation and entrainment might, at times, conflict (e.g., slowing overall speech rate, but entraining to the faster rate of the bot). Additionally, including both measures in the same model can directly test the extent hyperarticulation and entrainment are mediated by functional pressures (e.g., speech recognition errors) and social-situational pressures (e.g., presence of an experimenter).
How might hyperarticulation and entrainment vary as a function of intelligibility pressures that change dynamically within a conversation? Automatic speech recognition (ASR) mistakes are common in a spontaneous interaction with a voice-AI system. The present study investigates whether turn-by-turn dynamics of hyperarticulation and entrainment vary based on whether the Alexa system makes a comprehension error or not. There is a rich literature examining hyperarticulation toward computer interlocutors in response to an error made by the system (Oviatt and VanGent, 1996; Oviatt et al., 1998b; Bell and Gustafson, 1999; Swerts et al., 2000; Vertanen, 2006; Stent et al., 2008; Maniwa et al., 2009; Burnham et al., 2010). For example, Stent et al. (2008) found that speakers’ increased hyperarticulation in response to an ASR error lingered for several trials before ‘reverting’ back to their pre-error speech patterns; in the present study, we similarly predict slower speech rate following an ASR error. While less examined than hyperarticulation, there is some evidence suggesting that entrainment also serves a functional role (Branigan et al., 2011; Cowan et al., 2015); for example, participants show more duration alignment if their interlocutor made an error (Zellou and Cohn, 2020). Thus, we might also predict greater entrainment following an error, relative to pre-error.
How might context shape speech hyperarticulation and entrainment toward an Alexa socialbot? In the current study, half of the participants interacted with the socialbot in-person in a laboratory setting with experimenters present, while the other half interacted at home1 using the Amazon Alexa app. While many studies of voice-AI are conducted in a laboratory setting (e.g., Cohn et al., 2019; Zellou et al., 2021), there is evidence that the presence of an experimenter influences how participants complete a task (Orne, 1962; Belletier et al., 2015; Belletier and Camos, 2018). Indeed, Audience Design theory proposes that people tailor their speech style for their intended addressee, as well as for ‘overhearers’ (i.e., individuals listening to the conversation, but not directly taking part) (Clark and Carlson, 1982). For example, speakers are more polite when there is a bystander present (Comrie, 1976). As a result, we might predict more careful, hyperarticulated speech in a lab setting with overhearers. Prior work has also shown that engaging with additional interlocutors shapes entrainment: Raveh et al. (2019) found that speakers entrained less toward an Alexa assistant if they had interacted with a third interlocutor (a human confederate), compared to dyadic interactions only between the user and Alexa. Therefore, we might predict that participants will display less entrainment in the laboratory setting (relative to at-home).
In the current study, we use a socialbot system originally designed for Amazon Alexa Prize (Chen et al., 2018; Liang et al., 2020). In-lab user studies were conducted on the same day (pre-social isolating measures) in a quiet room. At-home user studies occurred across nine days in April-June, where speakers participated in an online experiment, activating the socialbot from home and recording their interaction with their computer microphone in a quiet room.
Participants (n = 35) were native English speakers, recruited from UC Davis (mean age = 20.94 years old ±2.34; age range 18–30 years; 22 female, 13 male). The in-lab user condition, consisting of 17 participants (mean age = 20.76 years ±2.66; 14 female, 3 male). An additional 18 participants (mean age = 21.11 years ±2.03, 9 female, 9 male) completed an at-home user condition. A t-test revealed that there was no significant difference in ages between these groups [t (29.9) = −0.43, p = 0.67]. Nearly all participants (34/35) reported using voice-AI assistants in the past. All participants consented to the study (following the UC Davis Institutional Review Board) and received course credit for their participation.
In-lab participants completed the experiment in a quiet room, with an Amazon Echo located in front of them on a table. Their interactions were recorded using a microphone (Audio-Technica AT 2020) facing the participant. An experimenter initiated the socialbot, and 1-2 experimenters were present in the room to listen to the conversation. Those in the at-home condition completed the experiment online via a Qualtrics survey which was used to record their speech (via AddPipe2 and their computer microphone). For the at-home condition, participants were given instructions to install the Alexa app to their phones and activate a Beta version of the socialbot.
All participants began with a baseline recording of an utterance: “The current month is [current month]. Test of the sound system complete.” Then, they initiated the socialbot conversation and were instructed to have two conversational interactions with the system for roughly 10 min each (see Table 1 for an example excerpt). If the bot crashed before the 10 min, they were asked to re-engage the Alexa Skill again. Dialogue flows included multiple domains (e.g., movies, sports, animals, travel, food, music, and books), as well as general chit-chat and questions about Alexa’s ‘backstory’ (e.g., favorite color, animal, etc.) (Chen et al., 2018; Liang et al., 2020). At the end of the interaction, participants rated the Alexa socialbot across three dimensions, on a scale of 1–5: “How engaging did you find the bot? 1 = not engaging, 5 = extremely engaging”, “How likely would you talk to the bot again? 1 = not likely, 5 = extremely likely”, “How coherent was the bot? 1 = not coherent, 5 = extremely coherent”.
Baseline and conversation recordings were initially transcribed with Amazon ASR or Sonix3. Trained research assistants confirmed the accuracy of the transcripts and annotated the sound files in a Praat Textgrid (Boersma and Weenink, 2018), labeling the interlocutor turns and the presence of ASR errors made by the socialbot. Errors included ‘long pause’ errors, such as when the socialbot took a long pause and then used an interjection or responded with phrases like “Tik tok! Did I confuse you?” or “Are you still there?” Other ASR errors included when the socialbot responded with a different word or topic than what the user mentioned. For instance, when the user said they were watching tv shows recently, the socialbot responded with “Great! I love talking about sports … ”
We analyze only the first continuous conversation with Alexa in order to assess differences from baseline to the bot interaction, rather than differences between bot conversations. On average, participants spoke with the socialbot for 12.48 min (sd = 5.44) including 96.63 total turns (user + Alexa) (sd = 44.58). The socialbot made an average of 6.94 errors per conversation (sd = 5.57). T-tests confirmed no difference in conversation duration [t (29.52) = −1.03, p = 0.31], overall number of turns [t (29.58) = −0.90, p = 0.37], or number of errors [t (23.49) = −0.23, p = 0.82] across in-lab and at-home contexts. In total, the corpus consisted of 1,417 productions by the human users.
Speech rate (mean number of syllables per second) was measured using a Praat script (De Jong et al., 2017) for each of the socialbot’s turns, user’s turns, and the user’s baseline productions. To measure differences in hyperarticulation in talking to the Alexa socialbot, we centered each user’s turn-level speaking rate relative to their baseline production (i.e., subtracting all ‘speech rate’ values by the user’s average baseline speech rate). This centered value is then used to ascertain change from a user’s baseline. For instance, a positive value indicates an increase in speaking rate from baseline.
To measure entrainment, we test ‘synchrony’ (Coulston et al., 2002; Levitan & Hirschberg, 2011): how speakers synchronize their productions across turns. For instance, when the Alexa produces a relatively faster speaking rate, does the user also show a relative increase in speaking rate? We used the user’s turn-level rate measurements (centered within user) and also centered the Alexa’s productions (subtracting the mean speaking rate of Alexa’s overall values for each conversation). Accordingly, comparing the ‘Alexa-prior turn’ (centered) and user’s value (centered) can capture whether users adjust their speech to match the directionality of change. Additionally, this method allows us to compare both hyperarticulation and entrainment in the same model, with the dependent variable of the (centered) user’s speaking rate.
A t-test revealed that the Alexa was rated as more engaging in the at-home condition (mean = 4.10) relative to the in-lab condition (mean = 3.35) [t (31.84) = 2.52, p < 0.05]. There was no significant difference in ratings of how coherent the bot was [t (30.52) = 0.83, p = 0.41] or in how much the participant would want to talk to the bot again [t (30.01) = −1.52, p = −0.14] based on situational context. We calculated an overall ratings value, summing users’ ratings for engagement, coherence, and desire to talk to the bot again (mean = 11.30, range = 7–14) to use in the statistical model on speaking rate change.
Mean values for speaking rate of the user’s baseline productions, users’ responses to the socialbot, as well as the socialbot’s productions are provided in Table 2. As seen, there were differences in the baseline productions based on setting, where speakers produced slower rate in-lab in their baseline production. The Alexa productions had a faster speech rate in-lab (relative to at-home)4.
We modeled speech rate (centered within user) with a linear mixed effects model using the lme4 R package (Bates et al., 2015). Fixed effects included Setting (2 levels: in-lab, at-home), Overall Rating (coherence + satisfaction + engagement, centered), and all possible two-way interactions with Alexa Prior Turn Rate (continuous, centered). We additionally added Gender as a fixed effect (2 levels: female, male)5. Random effects included by-User random intercepts6. Categorical contrasts were sum coded.
The model showed a significant negative intercept, indicating that users’ speaking rate decreases (i.e., fewer syllables/second) in the socialbot interactions relative to baseline productions [Coef = −0.62, t = −5.96, p < 0.001]. Additionally, there was a main effect of Setting, shown in Figure 1: speakers produced an even slower speech rate in-lab, relative to at-home [Coef = −0.37, t = −3.59, p < 0.01]. Furthermore, there was an effect of Gender: female users slowed their speech rate even more during socialbot interactions [Coef = −3.18, t = −2.95, p < 0.01].
FIGURE 1. Speech rate change (relative to the user’s baseline, 0.0; centered) across the at-home and in-lab settings. A decrease in the number of syllables/second (a more negative number) indicates a slower speech rate. Error bars depict standard error.
There was also an effect of Alexa Prior Turn Rate: user’s speech rate increases when the speech rate increases in the Alexa Prior Turn [Coef = 5.61, t = 11.90, p < 0.001] (see Figure 2). There was no observed effect of Overall Rating and no interactions observed.
FIGURE 2. Speech rate change (centered relative to the user’s baseline) across the at-home and in-lab settings. A decrease in the number of syllables/second (a negative number) indicates a slower speech rate. Shading depicts the standard error.
We analyzed speaker’s speech rate in a subset of the data consisting of the four user turns preceding an ASR error (Pre-Error) and four turns following an ASR error (Post-Error) (n = 771 turns, n = 327 users). Rate (centered) was modeled with a linear mixed effects model. Fixed effects included Error Condition (pre-error, post-error), Setting (in-lab, at-home), their interaction, and Gender (female, male)8, and by-User random intercepts. Contrasts were sum coded.
The model revealed a similar effect in the Pre- and Post-error subset as in the main model: an overall negative intercept [Coef = −0.71, t = −6.88, p < 0.001], an effect of Setting [Coef = 0.44 t = −4.45, p < 0.001], Alexa Prior Turn [Coef = 0.57, t = 7.91, p < 0.001], as well as Gender [Coef = −0.36, t = −3.55, p < 0.01]. However, there were no effects of Error Condition and no interactions including Error Condition observed.
This study examined users’ speech rate hyperarticulation and entrainment toward an Amazon Alexa socialbot in a conversational interaction. While generally tested and analyzed separately (e.g., Burnham et al., 2010; Cohn et al., 2019), this study highlights the importance of accounting for both hyperarticulation and entrainment to provide a fuller picture of speech interactions with voice-AI/computer interlocutors.
First, we find evidence of hyperarticulation: relative to their original baseline productions, users consistently decrease their speech rate when talking to the socialbot. This supports listener-centered accounts: speakers produce ‘clearer’ speech for listeners who might have trouble understanding them (Lindblom, 1990; Smiljanić and Bradlow, 2009). Indeed, these findings are consistent with slower speech rate observed for interlocutors presumed to have communicative difficulties, such as dialogue systems that have higher error rates (Oviatt et al., 1998b; Stent et al., 2008), as well as infants and non-native speakers (Fernald and Simon, 1984; Scarborough et al., 2007).
Above and beyond the hyperarticulation effect, we also find evidence for turn-level entrainment toward the speech rate patterns of the social bot. If Alexa produces a faster speech rate, users are more likely to speed up in the subsequent turn; conversely, if Alexa’s speech rate slows, users also slow their rate in the subsequent turn. This is consistent with prior findings in entrainment toward computers (e.g., amplitude convergence toward computer characters in Coulston et al., 2002). Yet, we did not find evidence that entrainment was linked to social ratings of the interaction, as is proposed by some alignment accounts (Giles et al., 1991; Shepard et al., 2001). One possibility is that socially mediated pressures differently affect entrainment toward voice-AI and humans in non-task oriented interactions (here, social chit-chat), but might do so in more task-oriented interactions (e.g., in a tutoring task in Thomason et al., 2013) or in less socially rich contexts (e.g., single word shadowing in (Cohn et al., 2019; Zellou et al., 2021). Another possibility is that the range of ratings might have been too narrow to detect a difference (if present), where the majority of speakers rated the interactions favorably. Future work exploring whether social sentiments influence entrainment toward socialbots can elucidate these questions.
Furthermore, we also observed differences in speech rate hyperarticulation by context: users slowed down even more in conversations in-lab than at-home. This is consistent with our prediction that participants would produce more careful, ‘clear’ speech when other observers were present—and is in line with Audience Design theory (Clark and Carlson, 1982) that productions are also tailored based on ‘overhearers’. Still, we cannot conclusively point to the overhearer as the source of this effect; it is possible that this reflects that the in-lab condition participants produced faster speech in their baseline (averaging ∼4 syllables/sec) and, possibly, had more room to hyperarticulate (slowing to an average of 2.93 syllables/sec). Future work parametrically manipulating speech rate—as well as comparing the same participants both in-lab and at-home can further tease apart these possibilities.
In addition to examining situational context, we also tested the impact of functional pressures in communication—specifically whether speakers hyperarticulate and/or entrain more following a system ASR error. We did not find effects for either behavior, contra findings human-computer interaction for post-error hyperarticulation (e.g., Oviatt et al., 1998b; Vertanen, 2006) or post-error entrainment (Zellou and Cohn, 2020). One possible explanation for why we do not observe hyperarticulation following ASR errors is that speakers were already talking in a very slow, ‘clear speech’ manner when talking to the socialbot. This explanation is consistent with studies in which, at a higher error rate, speakers maintain hyperarticulation (Oviatt et al., 1998b; Stent et al., 2008).
There were also limitations in the present study that can serve as the basis for future research. One such limitation is that we had different participants in the in-lab and at-home conditions; while one benefit to this approach was that the interaction consisted of the first socialbot conversation each user had with the system, future work examining user speech across different contexts can further tease apart the source of differences observed across settings. Furthermore, we observed differences by gender, where female participants slowed their speech even more to the socialbot; yet, as the current study was not balanced by gender, future work is needed to test whether this difference is truly socially mediated—with more hyperarticulation produced by females (e.g., increased pitch range by females in Oviatt et al., 1998b)—or possibly driven by the individual speakers in the study. Additionally, here we test one socialbot system; future work testing other systems can shed more light on how users hyperarticulate and entrain toward socialbots, more generally.
Overall, this study contributes to our broader scientific understanding of human and voice-AI interaction. Here, we find that speakers use hyperarticulation and entrainment in speech interactions with an Alexa socialbot, paralleling some patterns observed in human-human interaction. Future work directly testing a human vs. socialbot interlocutor comparison can further tease apart possible differences in social interactions with the two types of interlocutors. Additionally, human-human conversational entrainment is coordinative, with each speaker adapting their output (Levitan et al., 2015; Szabó, 2019). There is some work investigating the effects of adapting TTS output to entrain toward the user (Lubold et al., 2016). Future studies examining the extent to which speakers entrain to Alexa socialbots—as they entrain to the user—can shed light on the situational, functional, and interpersonal dynamics of human-socialbot interaction.
The datasets presented in this article are not readily available because participants cannot be deidentified in their conversations with the socialbot. Requests to access the datasets should be directed to bWRjb2huQHVjZGF2aXMuZWR1.
The studies involving human participants were reviewed and approved by UC Davis Institutional Review Board (IRB). The participants provided their written informed consent to participate in this study.
MC and K-HL contributed to the conception and design of the study. K-HL developed the socialbot with ZY. MC and MS led the acoustic analysis and received feedback from GZ. MC wrote the first draft of the manuscript. All authors contributed to the editing and revision of the manuscript.
This material is based upon work supported by the National Science Foundation SBE Postdoctoral Research Fellowship under Grant No. 1911855 to MC, an Amazon Faculty Research Award to GZ, and the Amazon Alexa Prize competition in supporting the bot development to ZY and KL. The funders were not involved in the study design, collection, analysis, interpretation of data, the writing of this article or the decision to submit it for publication.
The authors declare that this study received funding from Amazon.
1Due to the COVID-19 pandemic, all in-lab user studies were paused in Spring 2020.
4Differences in the social bot speech rate reflect the un-scripted nature of the conversations. The bot scrapes information from the relevant APIs (e.g., IMDB), leading to unique Alexa productions.
5A post-hoc model confirmed there were no interactions between Gender and the other covariates.
6More complex random effects structures resulted in singularity errors, indicating model overfit.
7We only included participants who did not have additional errors within the ± 4 turns. For example, if multiple errors occurred within four turns, we did not include those participants (n = 3).
8Posthoc models confirmed no significant interactions between Gender and the covariates.
Abdolrahmani, A., Kuber, R., and Branham, S. M. (2018). “ Siri Talks at You” an Empirical Investigation of Voice-Activated Personal Assistant (VAPA) Usage by Individuals Who Are Blind. Proceedings of the 20th International ACM SIGACCESS Conference on Computers and Accessibility, 249–258.
Ammari, T., Kaye, J., Tsai, J. Y., and Bentley, F. (2019). Music, Search, and IoT. ACM Trans. Comput.-Hum. Interact. 26 (3), 1–28. doi:10.1145/3311956
Babel, M., and Bulatov, D. (2012). The Role of Fundamental Frequency in Phonetic Accommodation. Lang. Speech 55 (2), 231–248. doi:10.1177/0023830911417695
Bates, D., Mächler, M., Bolker, B., and Walker, S. (2015). Fitting Linear Mixed-Effects Models Using Lme4. J. Stat. Softw. 67 (1), 1–48. doi:10.18637/jss.v067.i01
Bell, L., Gustafson, J., and Heldner, M. (2003). Prosodic Adaptation in Human-Computer Interaction. Proc. ICPHS 3, 833–836.
Bell, L., and Gustafson, J. (1999). Repetition and its Phonetic Realizations: Investigating a Swedish Database of Spontaneous Computer-Directed Speech. Proc. ICPhS 99, 1221–1224.
Belletier, C., and Camos, V. (2018). Does the Experimenter Presence Affect Working Memory?. Ann. N.Y. Acad. Sci. 1424, 212–220. doi:10.1111/nyas.13627
Belletier, C., Davranche, K., Tellier, I. S., Dumas, F., Vidal, F., Hasbroucq, T., et al. (2015). Choking under Monitoring Pressure: Being Watched by the Experimenter Reduces Executive Attention. Psychon. Bull. Rev. 22 (5), 1410–1416. doi:10.3758/s13423-015-0804-9
Bentley, F., Luvogt, C., Silverman, M., Wirasinghe, R., White, B., and Lottridge, D. (2018). Understanding the Long-Term Use of Smart Speaker Assistants. Proc. ACM Interact. Mob. Wearable Ubiquitous Technol. 2 (3), 1–24. doi:10.1145/3264901
Boersma, P., and Weenink, D. (2018). Praat: Doing Phonetics by Computer (6.0.37). [Computer software]. http://www.praat.org/.
Branigan, H. P., Pickering, M. J., Pearson, J., McLean, J. F., and Brown, A. (2011). The Role of Beliefs in Lexical Alignment: Evidence from Dialogs with Humans and Computers. Cognition 121 (1), 41–57. doi:10.1016/j.cognition.2011.05.011
Brumm, H., and Zollinger, S. A. (2011). The Evolution of the Lombard Effect: 100 Years of Psychoacoustic Research. Behav. 148 (11–13), 1173–1198. doi:10.1163/000579511x605759
Burnham, D. K., Joeffry, S., and Rice, L. (2010). Computer-and Human-Directed Speech before and after Correction. Proceedings of the 13th Australasian International Conference on Speech Science and Technology. Melbourne, Australia, 13–17.
Chartrand, T. L., and Bargh, J. A. (1996). Automatic Activation of Impression Formation and Memorization Goals: Nonconscious Goal Priming Reproduces Effects of Explicit Task Instructions. J. Personal. Soc. Psychol. 71 (3), 464–478. doi:10.1037/0022-3514.71.3.464
Chen, C.-Y., Yu, D., Wen, W., Yang, Y. M., Zhang, J., Zhou, M., et al. (2018). Gunrock: Building A Human-like Social Bot by Leveraging Large Scale Real User Data. 2nd Proceedings of Alexa Prize. Available at: https://developer.amazon.com/alexaprize/challenges/past-challenges/2018
Clark, H. H., and Carlson, T. B. (1982). Hearers and Speech Acts. Language 58, 332–373. doi:10.1353/lan.1982.0042
Cohn, M., Ferenc Segedin, B., and Zellou, G. (2019). Imitating Siri: Socially-Mediated Alignment to Device and Human Voices. Proceedings of International Congress of Phonetic Sciences, 1813–1817.
Comrie, B. (1976). Linguistic Politeness Axes: Speaker-Addressee, Speaker-Referent, Speaker-Bystander. Pragmatics Microfiche 1 (7), 1–12.
Coulston, R., Oviatt, S., and Darves, C. (2002). Amplitude Convergence in Children’s Conversational Speech with Animated Personas. Seventh International Conference on Spoken Language Processing.
Cowan, B. R., Branigan, H. P., Obregón, M., Bugis, E., and Beale, R. (2015). Voice Anthropomorphism, Interlocutor Modelling and Alignment Effects on Syntactic Choices in Human−computer Dialogue. Int. J. Human-Computer Stud. 83, 27–42. doi:10.1016/j.ijhcs.2015.05.008
De Jong, N. H., Wempe, T., Quené, H., and Persoon, I. (2017). Praat Script Speech Rate V2. https://sites.google.com/site/speechrate/Home/praat-script-syllable-nuclei-v2.
Fandrianto, A., and Eskenazi, M. (2012). Prosodic Entrainment in an Information-Driven Dialog System. Thirteenth Annual Conference of the International Speech Communication Association.
Fernald, A., and Simon, T. (1984). Expanded Intonation Contours in Mothers' Speech to Newborns. Dev. Psychol. 20 (1), 104–113. doi:10.1037/0012-1649.20.1.104
Gabriel, R., Liu, Y., Gottardi, A., Eric, M., Khatri, A., Chadha, A., et al. (2020). Further Advances in Open Domain Dialog Systems in the Third Alexa Prize Socialbot Grand Challenge. Proc. Alexa Prize. Available at: https://assets.amazon.science/0e/e6/2cff166647bfb951b3ccc67c1d06/further-advances-in-open-domain-dialog-systems-in-the-third-alexa-prize-socialbot-grand-challenge.pdf
Gessinger, I., Raveh, E., Le Maguer, S., Möbius, B., and Steiner, I. (2017). Shadowing Synthesized Speech-Segmental Analysis of Phonetic Convergence. Interspeech. 3797–3801. doi:10.21437/Interspeech.2017-1433
Gessinger, I., Raveh, E., Steiner, I., and Möbius, B. (2021). Phonetic Accommodation to Natural and Synthetic Voices: Behavior of Groups and Individuals in Speech Shadowing. Speech Commun. 127, 43–63. doi:10.1016/j.specom.2020.12.004
Giles, H., Coupland, N., and Coupland, I. (1991). 1. Accommodation Theory: Communication, Context, and. Contexts of Accommodation: Developments in Applied Sociolinguistics, 1.
Habler, F., Schwind, V., and Henze, N. (2019). Effects of Smart Virtual Assistants’ Gender and Language. In Proceedings of Mensch und Computer 2019. 469–473.
Lee, D.-Y., and Baese-Berk, M. M. (2020). The Maintenance of Clear Speech in Naturalistic Conversations. The J. Acoust. Soc. America 147 (5), 3702–3711. doi:10.1121/10.0001315
Levitan, R., Benus, S., Gravano, A., and Hirschberg, J. (2015). Entrainment and Turn-Taking in Human-Human Dialogue. AAAI Spring Symposia. doi:10.18653/v1/w15-4644
Levitan, R., Gravano, A., Willson, L., Beňuš, Š., Hirschberg, J., and Nenkova, A. (2012). Acoustic-prosodic Entrainment and Social Behavior. Proceedings of the 2012 Conference of the North American Chapter of the Association for Computational Linguistics: Human Language Technologies, 11–19.
Levitan, R., and Hirschberg, J. (2011). Measuring Acoustic-Prosodic Entrainment with Respect to Multiple Levels and Dimensions. Twelfth Annual Conference of the International Speech Communication Association.
Liang, K., Chau, A., Li, Y., Lu, X., Yu, D., Zhou, M., et al. (2020). Gunrock 2.0: A User Adaptive Social Conversational System. ArXiv Preprint ArXiv:2011.08906.
Lindblom, B. (1990). Explaining Phonetic Variation: A Sketch of the H&H Theory. Speech production and speech modelling. Springer, 403–439. doi:10.1007/978-94-009-2037-8_16
Lovato, S., and Piper, A. M. (2015). Siri, Is This You?: Understanding Young Children’s Interactions with Voice Input Systems. Proceedings of the 14th International Conference on Interaction Design and Children, 335–338.
Lubold, N., and Pon-Barry, H. (2014). Acoustic-prosodic Entrainment and Rapport in Collaborative Learning Dialogues. Proceedings of the 2014 ACM Workshop on Multimodal Learning Analytics Workshop and Grand Challenge, 5–12.
Lubold, N., Walker, E., and Pon-Barry, H. (2016). Effects of Voice-Adaptation and Social Dialogue on Perceptions of a Robotic Learning Companion. 2016 11th ACM/IEEE International Conference on Human-Robot Interaction (HRI), 255–262.
Lunsford, R., Oviatt, S., and Arthur, A. M. (2006). Toward Open-Microphone Engagement for Multiparty Interactions. Proceedings of the 8th International Conference on Multimodal Interfaces, 273–280.
Maniwa, K., Jongman, A., and Wade, T. (2009). Acoustic Characteristics of Clearly Spoken English Fricatives. J. Acoust. Soc. America 125 (6), 3962–3973. doi:10.1121/1.2990715
Mayo, C., Aubanel, V., and Cooke, M. (2012). Effect of Prosodic Changes on Speech Intelligibility. Thirteenth Annual Conference of the International Speech Communication Association, 1706–1709.
Nass, C., Moon, Y., Morkes, J., Kim, E.-Y., and Fogg, B. J. (1997). Computers Are Social Actors: A Review of Current Research. Hum. Values Des. Comp. Tech. 72, 137–162. doi:10.1145/259963.260288
Nass, C., Steuer, J., and Tauber, E. R. (1994). Computers Are Social Actors. Proceedings of the SIGCHI Conference on Human Factors in Computing Systems, 72–78.
Orne, M. T. (1962). On the Social Psychology of the Psychological Experiment: With Particular Reference to Demand Characteristics and Their Implications. Am. Psychol. 17 (11), 776–783. doi:10.1037/h0043424
Oviatt, S., Levow, G.-A., Moreton, E., and MacEachern, M. (1998a). Modeling Global and Focal Hyperarticulation during Human-Computer Error Resolution. J. Acoust. Soc. America 104 (5), 3080–3098. doi:10.1121/1.423888
Oviatt, S., MacEachern, M., and Levow, G.-A. (1998b). Predicting Hyperarticulate Speech during Human-Computer Error Resolution. Speech Commun. 24 (2), 87–110. doi:10.1016/s0167-6393(98)00005-3
Oviatt, S., and VanGent, R. (1996). Error Resolution during Multimodal Human-Computer Interaction. Spoken Language, 1996. ICSLP 96. Proceedings, Fourth International Conference On, 204–207.
Pardo, J. S., Urmanche, A., Wilman, S., and Wiener, J. (2017). Phonetic Convergence across Multiple Measures and Model Talkers. Atten Percept Psychophys 79 (2), 637–659. doi:10.3758/s13414-016-1226-0
Pickering, M. J., and Garrod, S. (2006). Alignment as the Basis for Successful Communication. Res. Lang. Comput. 4 (2–3), 203–228. doi:10.1007/s11168-006-9004-0
Purington, A., Taft, J. G., Sannon, S., Bazarova, N. N., and Taylor, S. H. (2017). “Alexa Is My New BFF”: Social Roles, User Satisfaction, and Personification of the Amazon Echo. Proceedings of the 2017 CHI Conference Extended Abstracts on Human Factors in Computing Systems, 2853–2859. doi:10.1145/3027063.3053246
Ram, A., Prasad, R., Khatri, C., Venkatesh, A., Gabriel, R., Liu, Q., et al. (2018). Conversational AI: The Science behind the Alexa Prize. ArXiv Preprint ArXiv:1801.03604.
Raveh, E., Siegert, I., Steiner, I., Gessinger, I., and Möbius, B. (2019). Three’s a Crowd? Effects of a Second Human on Vocal Accommodation with a Voice Assistant. Proc. Interspeech 2019, 4005–4009. doi:10.21437/Interspeech.2019-1825
Scarborough, R., Dmitrieva, O., Hall‐Lew, L., Zhao, Y., and Brenier, J. (2007). An Acoustic Study of Real and Imagined Foreigner‐directed Speech. J. Acoust. Soc. America 121 (5), 3044. doi:10.1121/1.4781735
Shepard, C. A., Robinson, W. P., and Giles, H. (2001). “Communication Accommodation Theory,” in The New Handbook of Language and Social Psychology (John Wiley & Sons), 33–56.
Siegert, I., and Krüger, J. (2020). “"Speech Melody and Speech Content Didn't Fit Together"-Differences in Speech Behavior for Device Directed and Human Directed Interactions,” in Advances in Data Science: Methodologies and Applications (Springer), 65–95. doi:10.1007/978-3-030-51870-7_4
Siegert, I., Nietzold, J., Heinemann, R., and Wendemuth, A. (2019). “The Restaurant Booking Corpus–Content-Identical Comparative Human-Human and Human-Computer Simulated Telephone Conversations,” in Studientexte Zur Sprachkommunikation: Elektronische Sprachsignalverarbeitung 2019, 126–133.
Smiljanić, R., and Bradlow, A. R. (2009). Speaking and Hearing Clearly: Talker and Listener Factors in Speaking Style Changes. Lang. Linguist Compass 3 (1), 236–264. doi:10.1111/j.1749-818X.2008.00112.x
Stent, A. J., Huffman, M. K., and Brennan, S. E. (2008). Adapting Speaking after Evidence of Misrecognition: Local and Global Hyperarticulation. Speech Commun. 50 (3), 163–178. doi:10.1016/j.specom.2007.07.005
Swerts, M., Litman, D., and Hirschberg, J. (2000). Corrections in Spoken Dialogue Systems. Sixth International Conference on Spoken Language Processing.
Szabó, I. E. (2019). Phonetic Selectivity in Accommodation: The Effect of Chronological Age. Proceedings of the 19th International Congress of Phonetic Sciences. Canberra, Australia, 3195–3199.
Thomason, J., Nguyen, H. V., and Litman, D. (2013). Prosodic Entrainment and Tutoring Dialogue Success. International Conference on Artificial Intelligence in Education, 750–753. doi:10.1007/978-3-642-39112-5_104
Vertanen, K. (2006). Speech and Speech Recognition during Dictation Corrections. Ninth International Conference on Spoken Language Processing, 1890–1893.
Zellou, G., Cohn, M., and Ferenc Segedin, B. (2021). Age- and Gender-Related Differences in Speech Alignment toward Humans and Voice-AI. Front. Commun. 5, 1–11. doi:10.3389/fcomm.2020.600361
Keywords: vocal entrainment, socialbot, voice-activated artificially intelligent assistant, non-task oriented conversations, human-computer interaction, hyperarticulation
Citation: Cohn M, Liang K-H, Sarian M, Zellou G and Yu Z (2021) Speech Rate Adjustments in Conversations With an Amazon Alexa Socialbot. Front. Commun. 6:671429. doi: 10.3389/fcomm.2021.671429
Received: 23 February 2021; Accepted: 21 April 2021;
Published: 07 May 2021.
Edited by:
Ingo Siegert, Otto von Guericke University Magdeburg, GermanyReviewed by:
Nichola Anne Lubold, Arizona State University, United StatesCopyright © 2021 Cohn, Liang, Sarian, Zellou and Yu. This is an open-access article distributed under the terms of the Creative Commons Attribution License (CC BY). The use, distribution or reproduction in other forums is permitted, provided the original author(s) and the copyright owner(s) are credited and that the original publication in this journal is cited, in accordance with accepted academic practice. No use, distribution or reproduction is permitted which does not comply with these terms.
*Correspondence: Michelle Cohn, bWRjb2huQHVjZGF2aXMuZWR1
Disclaimer: All claims expressed in this article are solely those of the authors and do not necessarily represent those of their affiliated organizations, or those of the publisher, the editors and the reviewers. Any product that may be evaluated in this article or claim that may be made by its manufacturer is not guaranteed or endorsed by the publisher.
Research integrity at Frontiers
Learn more about the work of our research integrity team to safeguard the quality of each article we publish.