- 1Leibniz-Centre General Linguistics, Berlin, Germany
- 2Language Science and Technology, Saarland University, Saarbrücken, Germany
Phonetic structures expand temporally and spectrally when they are difficult to predict from their context. To some extent, effects of predictability are modulated by prosodic structure. So far, studies on the impact of contextual predictability and prosody on phonetic structures have neglected the dynamic nature of the speech signal. This study investigates the impact of predictability and prominence on the dynamic structure of the first and second formants of German vowels. We expect to find differences in the formant movements between vowels standing in different predictability contexts and a modulation of this effect by prominence. First and second formant values are extracted from a large German corpus. Formant trajectories of peripheral vowels are modeled using generalized additive mixed models, which estimate nonlinear regressions between a dependent variable and predictors. Contextual predictability is measured as biphone and triphone surprisal based on a statistical German language model. We test for the effects of the information-theoretic measures surprisal and word frequency, as well as prominence, on formant movement, while controlling for vowel phonemes and duration. Primary lexical stress and vowel phonemes are significant predictors of first and second formant trajectory shape. We replicate previous findings that vowels are more dispersed in stressed syllables than in unstressed syllables. The interaction of stress and surprisal explains formant movement: unstressed vowels show more variability in their formant trajectory shape at different surprisal levels than stressed vowels. This work shows that effects of contextual predictability on fine phonetic detail can be observed not only in pointwise measures but also in dynamic features of phonetic segments.
1 Introduction
Probabilistic reduction of predictable words and subword units has been observed in many languages (e.g., Gahl, 2008; Bell et al., 2009; Bürki et al., 2011; Kuperman et al., 2007; Pellegrino et al., 2011; Pluymaekers et al., 2005a, b). Specifically, vowels are more reduced in their spectral distinctiveness when they are difficult to predict from their context compared to easily predictable vowels (Jurafsky et al., 2001; Wright, 2004; Aylett and Turk, 2006; Clopper and Pierrehumbert, 2008; Scarborough, 2010). This effect of contextual predictability (henceforth, for brevity—predictability) on segmental properties prevails even after controlling for known prosodic effects on phonetic structures, such as lexical stress (Brandt, 2019). For instance, stressed vowels that are difficult to predict tend to be more dispersed, that is, distant from the center of the vowel space, than unstressed vowels that are easily predictable, beyond the extent to which the dispersion would be predicted by stress alone (Brandt et al., 2019). Conversely, the degree of dispersion will be attenuated for stressed vowels in high-predictability contexts and enlarged for unstressed vowels that are hard to predict. Predictability thus affects form encoding. The smooth signal redundancy (SSR) hypothesis (Aylett and Turk, 2004, 2006) proposes that the impact of the predictability of linguistic events on the phonetic encoding of these events is mediated by the prosodic structure, in particular by lexical stress. An alternative interpretation is that the assignment of the prosodic structure is conditioned by predictability (Tang and Shaw, 2020). Both perspectives entail that predictability is tightly interwoven with the prosodic structure.
Aylett and Turk (2006) investigated the effects of predictability and stress on the first and second formants of American English vowels and observed a large amount of shared contribution of predictability and stress to explaining the formant patterns, generally supporting the SSR hypothesis. Crucially, they also found an unexpected unique contribution of predictability in their statistical models. On average, however, prominence is found to be more effective in explaining variability in F1/F2 patterns than predictability. Malisz et al. (2018) analyzed the sensitivity of different prosodic characteristics to predictability and prominence in six languages: American English, Czech, Finnish, French, German, and Polish. They observed a positive interaction effect of these two factors on the segmental duration and the consonantal center of gravity (COG): stressed segments in low-predictability contexts are longer and show higher mean COG than unstressed segments in high-predictability contexts. There was no significant interaction effect between predictability and prominence on vowel dispersion.
Taken together, there is evidence that the mediation of the effects of predictability on the segmental structure by the prosodic structure is not comprehensive and that predictability effects are not entirely consumed by prosodic prominence (Malisz et al., 2018).
However, research so far has neglected the impact of information-theoretic factors on the dynamic characteristics of vowels. The present study therefore focuses on the effect of predictability on formant dynamics using generalized additive mixed models (GAMMs) while controlling for known effects of prosodic prominence on vocalic characteristics. Most literature on predictability effects on segmental properties of speech has focused on (American) English. It is important to replicate results for other languages because of the implications they may have for explaining the production and perception of the phonetic structure. This work investigates dynamic formant trajectory patterns in German vowels in different predictability contexts.
1.1 Dynamic Structure of German Vowels
The German vowel inventory consists of a rather large number of monophthongs with seven tense/lax vowel phoneme pairs [/i–I, y–Y, e(ε)–ε, ø–œ, a–a, o–ɔ, and u–
There is, however, still considerable formant movement in German monophthongs, with distinct patterns for tense and lax pairings (Strange and Bohn, 1998). Most of the variance in dynamic formant changes in German monophthongs reflects formant movement toward the place of articulation of neighboring consonants. This coarticulatory effect is observed throughout the entire duration of the vowel and therefore is not restricted to the beginning or end of the vowel (Möbius, 1999). Lax vowels are more strongly influenced by context than tense vowels. Alveolar contexts induce stronger coarticulatory behavior in German vowels than labial contexts. Also, low and back vowels show more contextual variation than front vowels (Strange et al., 2007).
Although formant movement in German monophthongs, and especially in tense vowels, may be more subtle than that in English varieties, native German listeners show the same performance in vowel identification when listening to vocalic nuclei from CVC sequences as they do when hearing silent center syllables with only the onset and offset of the vowel being presented. Additional information about intrinsic vowel length reduces the error rate in identification and discrimination tasks (Strange and Bohn, 1998; Bohn and Polka, 2001). This indicates that German listeners rely on information about formant movement similarly to English natives, who use diphthongization as a cue to differentiate tense and lax monophthongs.
Vowel phonemes may show more or less variability and movement in their formants depending on the denseness of the vowel space in their direct vicinity (Wedel et al., 2018). This idea of competition between neighboring vowel phonemes has the following implications for German. Here, the front, close to mid-close vowel space is rather dense with a high number of vowel phonemes, while the open, mid vowels and the close, back vowels have considerably less competition from neighboring vowel phonemes (Möbius, 2001).
1.2 Information-Theoretic Measures
Information-theoretic measures (Shannon, 1948), such as frequency or predictability, have been linked to the realization of linguistic structures (for review, see Hale, 2016; Jaeger and Buz, 2017). In this context, surprisal S (uniti), which estimates the predictability of local structures, has been shown to correlate with human processing difficulty pertaining to linguistic units at different levels (Demberg et al., 2012; Levy, 2008; Hale, 2001; Levy, 2011). Surprisal is measured in bits of information and calculated as the negative log to the base two of the probability (P) of a linguistic unit (uniti) appearing in a specific context (context), which can be the preceding or following context of that unit or both (Eq. 1).
The surprisal measure reflects the intuition that linguistic units that are difficult to predict from context are more surprising when they occur, and conversely, the occurrence of easily predictable units is less surprising. Surprisal quantifies the predictability of local structures and is usually estimated from language models (LMs) based on large text corpora. In this study, we measure predictability as surprisal based on phoneme-level LMs because we investigate phonetic structures whose variability is thought to be best reflected by predictability estimated at the phoneme level (Oh et al., 2015). Hierarchical structural information, such as syllable or word boundaries, which also affect segmental properties, is implicitly reflected in sequences of phones (Raymond et al., 2006).
When investigating the impact of information-theoretic measures on linguistic structures, it is important to distinguish predictability from pure frequency effects, although frequency and predictability are not independent measures (Cohen Priva and Jaeger, 2018). Frequently used linguistic elements are under greater pressure to be efficient than less frequent ones (Zipf, 1949). More recent crosslinguistic studies have found that it is not frequency of occurrence but contextual predictability that is more efficient in explaining variability in word length, especially for lower-frequency words (Dautriche et al., 2017; Piantadosi et al., 2011). This line of research suggests that the effect of frequency is subordinate to that of predictability.
In studies on predictability effects on phonetic structures, word frequency is usually included as a control variable to tease apart effects of the two information-theoretic measures, viz. predictability and word frequency, on linguistic variability (e.g., Bell et al., 2009; Gahl et al., 2012; Jurafsky et al., 2001). On average, low-frequency words include vowels with increased dispersion, or distance from the center of the vowel space, compared to high-frequency words (Jurafsky et al., 2001; Zhao and Jurafsky, 2009). Vowels in frequent syllables have been shown to have faster formant transitions, that is, to show stronger coarticulatory influences, than vowels in infrequent syllables (Benner et al., 2007). This frequency effect has been found to be consistent in different lexical stress conditions. In accordance with the current literature, we therefore include word frequency as an additional information-theoretic measure in our models.
1.3 Research Questions and Hypotheses
The main aim of this study is to investigate whether German formant trajectories differ in their curvature when vowels stand in different surprisal contexts or appear in words with different frequencies of occurrence. We test for the effect of surprisal on formant movement by including the factor in interaction with the measurement point in the nonparametric part of our statistical model (Section 2.2.4). Given our previous findings that vowel dispersion in German is significantly affected by surprisal and word frequency (Brandt et al., 2019), we expect to find differences in formant trajectories between vowels in these different contexts, too.
Following the SSR hypothesis (Aylett and Turk, 2004, 2006), we investigate whether the effect of predictability on formant movement is modulated by a word-level effect of prominence, that is, primary lexical stress. We also control for the known effect of the place of articulation of directly preceding and following speech sounds on formant movements in the statistical models. Moreover, our models take into account that vowels located in less densely populated regions of the German vowel space are more variable in their formants, especially in F1 (Möbius, 2001), by including vowel phonemes as a predictor. We predict that the information-theoretic measure of surprisal affects formant trajectories above and beyond the effects of stress and coarticulation captured by the control factors.
2 Materials and Methods
2.1 Materials
2.1.1 Speech Corpus
The Siemens Synthesis corpus (SI1000P) (Schiel, 1997) is used as speech material. These recordings were done to provide high-quality material for concatenative speech synthesis. The corpus contains audio recordings from two professional, middle-aged, male speakers of Standard German. Both speakers are trained and experienced broadcast announcers who worked at a German local state broadcasting station (BR) at the time of the recording. They were asked to read as if in a broadcasting setting. Both speakers read the same speech material. Each speaker recorded 992 sentences selected from the Frankfurter Allgemeine newspaper corpus (SI1000) in an echo-canceling studio using a Sennheiser MKH20 omnidirectional microphone with a controlled distance of 30 cm to the mouth, at a sampling rate of 48 kHz and 16 bits, filtered and down-sampled to 16 kHz. Canonical transcriptions and automatic word and phoneme segmentations are available.
2.1.2 Language Modeling Corpus
For the purpose of language modeling and extraction of word frequency values, we used a large text corpus with a sufficient amount of data. A German language model was trained using the web-crawled DeWaC corpus (Baroni et al., 2009), which comprises 1.2 billion running words and 9.3 million lexical types from a diverse range of genres.
2.2 Data Analysis
2.2.1 Speech Data Analysis
The automatic annotations provided in the speech corpus were manually verified by two phonetically trained annotators in the Phonetics laboratory at Saarland University who showed a very strong inter-rater agreement in the choice of their segment boundaries based on a Spearman’s rho correlation test (
The first and second formants were extracted using the Burg algorithm in Praat using a time step of 0.01 s, a maximum number of five formants, a ceiling of 5,000 Hz for the formant search range which is the default for adult male speakers, a window length of 25 ms, and preemphasis from 50 Hz at every 10% of the time-normalized vowel duration, yielding a formant trajectory defined by 11 samples for each vowel. The number of measurement points is sufficient for formant trajectory estimation since male speakers produce speech at an average fundamental frequency of about 100–120 Hz, which means that formant values change at about every 8–10 ms. The average vowel duration in our data is 77 ms (SD = 33 ms), yielding a sufficiently dense sample of formant measurements per vowel.
Vowels in function words were excluded from the analysis following Bell et al. (2009). We also excluded diphthongs from the dataset because they inherently show more movement in their formants than monophthongs. The starting point at 0% and the end point at 100% of the vowel duration were discarded in the analysis because here formant extraction is potentially heavily influenced by the preceding or following speech sound. Formant values were cleaned using the interquartile ranges for F1 and F2 for German male speakers in the study by Pätzold and Simpson (1997) as a guideline. Since we model formant trajectories and are not limited to formant values at the temporal midpoint, we used more generous ranges for F1 (200–700 Hz) and F2 (450–2,400 Hz). Vowel tokens with formant values outside of these ranges were excluded from the analysis (n = 195, 0.34%). Formant values were not normalized because the statistical analysis applied here incorporates smoothing (see Section 2.2.4).
Only a subset of the German vowels was used in the modeling of German formant movement: front, close vowels: /i, I, e/; open, mid vowels: /a, a/; and back, close vowels: /u,
2.2.2 Language Modeling Procedure
Data preprocessing of the DeWaC corpus included lowercasing, punctuation removal, and grapheme-to-phoneme (g2p) conversion (Möhler et al., 2000). The transcriptions of the most frequent 1,000 words in the corpus were manually verified by the first author. Systematic errors in the g2p conversion were identified and corrected.
The training corpus (80% of the data) was used to train n-phone LMs using the SRILM toolkit (Stolcke, 2002). All LMs include sentence and word boundary markers and are based on both function and content words. By default, SRILM calculates the conditional probability of a linguistic unit based on its preceding context. In order to calculate conditional probabilities based on the following context, we used the built-in SRILM function reverse-text, which reverses the order of the linguistic units in each sentence. Models were smoothed using Witten–Bell smoothing. Because of the limited lexicon of the LM, count-of-counts statistics, such as Kneser–Ney, produced erroneous output.
The output for contextual predictability of the n-phone LMs was then transferred into surprisal (Eq. 1). We also extracted word frequency. Surprisal and word frequency were log-transformed because of their pronounced positive skewness. Surprisal values based on small n-phone sizes, as used in this study, express the probability of the phonotactic structure of a language, rather than simply giving information about preceding or following speech sounds. When segments are in word-initial or -final positions, the surprisal values reflect the word boundary marker. Other linguistic levels that potentially affect acoustic variability, even on the subword level, are only implicitly expressed in the surprisal values used here. We aimed to control for these effects by including word identity in the random structure of the statistical models.
We limit our investigation of formant movement to bi- and triphone surprisal for several reasons. First, the statistical models calculated in Section 3 explain a large quantity of deviance in the formant trajectory data (about 85%). Second, the increasing n-phone size leads to higher sparsity in the data, that is, vowels that are close to the beginning or end of a sentence are not matched with a respective surprisal value (sentences were read as separate prompts), and certain unusual combinations of longer n-phone strings are not represented in the language model. Third, in a different investigation of the effect of surprisal on vowel dispersion, we have tested different n-phone sizes up to six and shown that the correlation between these two measures drops distinctively from the triphone level to the six-phone level (Brandt, 2018).
The bi- and triphones that are used for surprisal extraction are based on a transcription of the actual produced utterance, in contrast to using the normative, dictionary forms. We follow Tucker et al. (2019) in this approach, who found that the prediction accuracy of vowel duration decreases when using diphones based on dictionary transcriptions compared to using diphones based on transcriptions of actual productions.
In addition, it should be noted that higher order n-phones always contain the string of their respective lower order n-phones, that is, the information of the biphone is contained within the triphone. For that reason, we expect biphone and triphone surprisal values that share the same context direction to be correlated to some extent.
2.2.3 Primary Lexical Stress
Prominence was coded as a binary factor based on primary lexical stress (levels: stressed vs. unstressed) in the corpus text. Monosyllabic content words were classified as stressed.
2.2.4 Generalized Additive Mixed Modeling
We used generalized additive mixed models (GAMMs) to investigate dynamic changes in the formant trajectories of F1 and F2 as provided by the R package mgcv (R Development Core Team, 2008; Wood, 2011, 2017), visualized with itsadug (van Rij et al., 2017). GAMMs combine parametric terms and smooth terms in their structure, that is, they allow investigation of the relations between a response and one or more covariates in average values and also in nonlinear terms. In addition, they incorporate random effects, that is, random intercepts, slopes, and smooths. Random smooths allow us to model nonlinear by-group variation in the response variable (Sóskuthy, 2017). Recently, GAMMs have gained popularity in phonetic studies with a focus on speech articulation (Tomaschek et al., 2018b; Carignan et al., 2020) and acoustic–phonetic measures (Kirkham et al., 2019). In addition to their advantage of modeling nonlinear data, GAMMs are also able to capture interaction effects of two continuous variables by means of tensor product interaction [ti ()]. In the field of phonetics, this is particularly useful for modeling articulatory or acoustic data because they are conditioned by the interaction of time (temporal dimension or duration) and other continuous dimensions, such as space or measurement points.
Prior to model fitting, we checked for collinearity between the variables by using the pairs. panels () function of the psych package (Revelle, 2021). As expected, surprisal values that share the same context direction were moderately correlated (preceding context: r = 0.47, following context: r = 0.62), which was why we decided to calculate separate models for each surprisal variable. Word frequency and surprisal values, however, only showed a very weak (surprisal (X
Surprisal values and word frequency were log-transformed. Vowel phonemes with three factor levels, front (/i, I, e/), mid (/a, a/), and back (/u,
We followed the modeling approach presented in the GAMM tutorial article by Wieling (2018). The model structure is given in listing 1. GAMMs were fitted using the bam () function of the mgcv package (Wood, 2019) because our dataset has more than 10,000 data points. Autocorrelation in the formant values can be expected for the temporal dimension vowel duration and also for the measurement point. Therefore, we included the autoregression function provided in the mgcv package. An AR (1) autoregressive error model for the residuals in a Gaussian model was included by using the rho parameter and setting the start event as 10% of the normalized vowel duration on an ordered dataset.
The smooth terms were fit with ‘thin plate regression splines’ (bs = ”tp”) (Wood, 2003). The interaction of the measurement point and duration and the interaction of surprisal and stress were fitted with “tensor product smooths” [ti ()], and we used “factor smooth interactions” (bs = “fs”) to fit random effects. The smoothing parameter (k) for each smooth was set via model diagnosis [gam.check ()]. Since there are less than 10 unique values for the response variable, smooths for the measurement point were set at k = 9 to avoid overfitting of the data. This approach allowed for the right amount of wiggliness in the data.
Model comparison was performed using the itsadug function compareML (), which compares two models that vary in one term using the Akaike information criterion (AIC). Models with significantly lower AIC value were preferred. Concurvity of smooth terms was checked [concurvity ()] looking at pairwise concurvity between the terms.
We included fixed effects for deviation-coded vowel phonemes (levels: front, mid, and back), treatment-coded stress (levels: yes and no), and an interaction between both terms. Smooth terms [s ()] for the measurement point were included in the model using ordered by-terms (by =) for stress and vowel phonemes as ordered factors (oVowel, oStress). We were also interested in differences in formant trajectory shape due to different surprisal values by stress and vowel phonemes. Additionally, we included a smooth for word frequency by ordered vowel phonemes. The smooth for word frequency by stress did not increase model performance significantly.
In addition, the smooth term for the measurement point, the smooth of duration, and a tensor product interaction (ti) for the measurement point and duration were added to account for the influence of the temporal structure on the trajectories (Sóskuthy, 2017). Another tensor product interaction for the measurement point and surprisal and a smooth term for surprisal were added to capture how the measurement point and surprisal interact in their effect on first and second formant trajectory. We also tested the tensor product interaction of the measurement point and word frequency, but it did not increase model performance. Including the smooth term for word frequency increased model performance.
To capture the speaker and vowel phoneme variation as well as the effect of following and preceding context on formant trajectory shape, random smooths were included in the model (bs = “fs”). The order of the nonlinearity penalty (m) for the random smooths was set to 1.
3 Results
The results of the GAMMs for F1 and F2 trajectories are presented by the terms in the models, providing a cohesive summary of the effects of surprisal and primary lexical stress and their interaction, word frequency, and the smooth terms on average formant values and the formant trajectory shapes. The GAMM output for each model is given in the supplementary material (Supplementary Tables S1–S8). Significant effects are reported when the significance level reaches p < 0.001. Since formant movement is heavily influenced by vowel duration, the average formant trajectory shapes are plotted for the mean vowel duration.
Differences in formant movement are visualized using difference smooth plots using the R package itsadug (van Rij et al., 2017). These plots convey the difference in formant trajectory shape between two factor levels (e.g., estimated difference of formant movement between unstressed and stressed vowels). Time windows with significant difference in trajectory shape are marked red and with dashed vertical lines, while those parts of the trajectory with no significant difference in shape are left unmarked. If the estimated difference with a 95% confidence interval of the dependent variable, that is, the first or second formant, is below zero in the difference smooth plot, the dependent variable in the reference level has higher values than the factor level that the reference level is compared to, and vice versa. The difference smooth plot only shows the difference between two levels of a factor, that is, multiple plots are needed if the factor has more than two levels.
Listing 1Structure of generalized additive mixed models used to model response variable (F1/F2) trajectory.
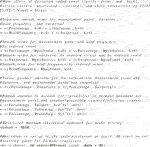
3.1 Vowel Phonemes
In our analysis of formant movement in German vowels, we focus on vowel phonemes in the periphery of the vowel space. We define three levels for the factor vowel: front: /i:, I, e:/; mid: /a:, a/:, and back vowels: /u:,
As can be seen in Figure 1, F1 is lower in back and front vowels compared to the grand mean, and F2 is lower in back vowels and higher in front vowels compared to the grand mean. Including an interaction between vowel phonemes and stress improves the model performance of all GAMMs tested in the current study. This interaction effect is also visualized in the average first and second formant trajectories given per stress condition and vowel phoneme in Figure 1. According to the GAMM output, F2 in stressed back vowels is significantly lower than in unstressed back vowels. For front vowels, F2 is higher in stressed than in unstressed vowels. F1 is lower in back and front vowels that stand in the stressed position than in those in the unstressed position, that is, these stressed vowels are more close and dispersed in the vowel space than their unstressed counterparts.
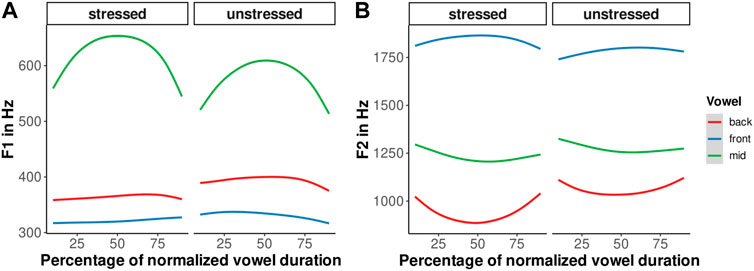
FIGURE 1. Mean first (A) and second (B) formant trajectories per vowel phoneme category (front, mid, and back) and primary lexical stress (unstressed and stressed).
The vowel is then also included as an ordered factor in a smooth term with the measurement point to compare first and second formant movement between the three factor levels. Here, “back” is set as the reference level. According to the GAMM output, both F1 and F2 formant movements differ significantly between mid and back vowels and between front and back vowels. The first formant trajectory in German open, mid /a, a/ is significantly more concave with a steeper increase and fall than in back or front vowels throughout almost the entire normalized duration of the vowel (Figures 2A,D,E,F). The estimated difference in F1 movement between front and back vowels is smaller than the difference in F1 between mid and back vowels or mid and front vowels but still statistically significant. Front vowels have higher F1 values in the first half of the normalized vowel duration than back vowels and higher F1 values in the second half of the vowel (Figure 2C). The F2 trajectory is shaped convex in mid and back vowels, while front vowels, on average, are produced with a concave F2 trajectory. These significant differences in F2 formant movement per vowel category are visualized in the difference smooth plots in Figure 2B.
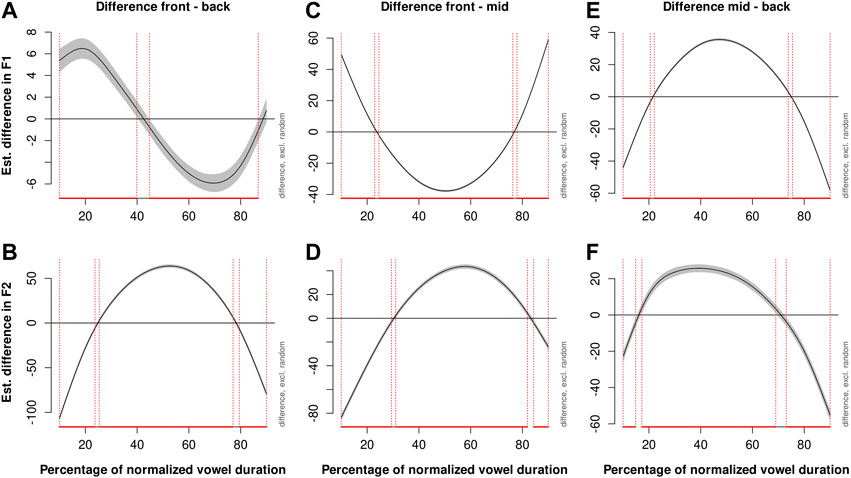
FIGURE 2. Difference smooth for first (A) and second (B) formants between front and back vowels (C), front and mid vowels (D), and mid and back vowels (E,F) with a 95% confidence interval.
3.2 Stress
The main effect of stress on average F1 and F2 reaches the significance level in almost all models calculated in the current study. Mean F1 and F2 are slightly lower in stressed vowels than in unstressed vowels. Stress is also included in the smooth term for the measurement point, accounting for variability in F1 or F2 movement in different stress conditions. This smooth term reaches the significance level in all models. However, it can be seen in Figure 3 that only a section of the formant trajectories (marked with vertical, dashed lines) in unstressed vowels is significantly different from that in stressed vowels: F1 movement differs significantly as a function of stress from around 25–50% of the normalized vowel duration (Figure 3A); F2 movement in unstressed vowels is different from that in stressed vowels only in the first part of the vowel up to 40% of its normalized duration (Figure 3B).
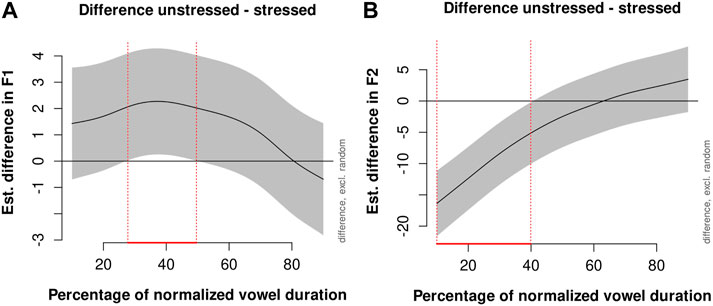
FIGURE 3. Difference smooth in first (A) and second (B) formants between vowels in unstressed and stressed positions with a 95% confidence interval.
3.3 Surprisal
We present the results for the effect of surprisal on the first and second formant trajectories of peripheral German vowels. Surprisal values are based on bi- and triphones of the preceding and following contexts of the vowel.
For the purpose of visual inspection of our data, we bin biphone surprisal of the preceding context into three equally sized categories of “low,” “mid,” and “high” and plot the frequency of the peripheral German vowels used in our subset per surprisal category (Figure 4).
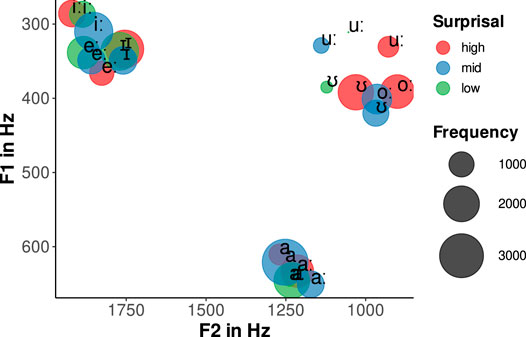
FIGURE 4. Vowel chart of the subset of peripheral vowels with frequency of vowel tokens in the three bins of high, mid, and low biphone surprisal of the preceding context.
Although Figure 4 only shows the frequency of vowel tokens in different categories of biphone surprisal of the preceding context and does not allow for general statements about the distribution of vowel phonemes in different surprisal contexts, there are two general observations that can be made. First, the average position of the vowel phoneme in the vowel space changes with regard to surprisal. Second, vowel phonemes are not equally distributed in the range of surprisal values observed in our data.
The second observation becomes even more apparent when investigating the distribution of vowel phonemes per bi- and triphone surprisal of the preceding or following context (Figure 5). On average, German back vowels have higher surprisal values, irrespective of n-phone order or direction, than mid or front vowels. Front vowels show slightly higher surprisal values than mid vowels. The difference between the distributions is more pronounced for biphone surprisal values than for triphone surprisal values.
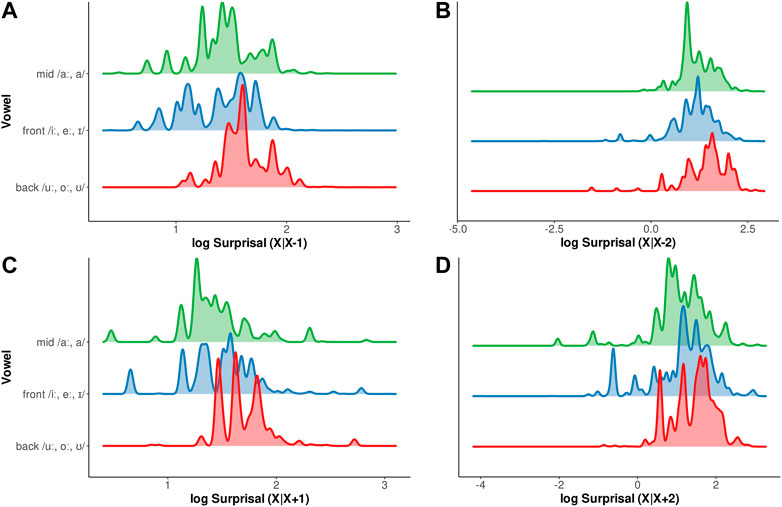
FIGURE 5. Density plot of front, mid, and back vowels in different surprisal conditions of the preceding (A,B) or following (C,D) contexts.
All GAMMs calculated here include a tensor product interaction [ti ()] of the measurement point and surprisal, as well as two separate, simple smooth terms of the measurement point and surprisal, in order to tease apart the interaction effect from the main effect of the two smooth terms. The interaction of the measurement point and surprisal on the first formant trajectory reaches the significance level in all GAMMs. This means that first and second formant movement in German vowels is significantly impacted by the interaction of the bi- and triphone surprisal of both context directions and the measurement point for formant extraction in the normalized vowel duration.
Figure 6 shows how F1 and F2 trajectory shapes for stressed vowels in the front, mid, and back positions vary. We observe that F1 shows the lowest values for all vowels in the data with high surprisal values (≥ 2.5), that is, stressed front and back vowels are more close in high surprisal contexts than in low surprisal contexts, and stressed mid vowels show more pronounced F1 movement in their previously observed concave trajectory shape due to distinctly low F1 values (around 500 Hz) in the first and last third of the normalized vowel duration.
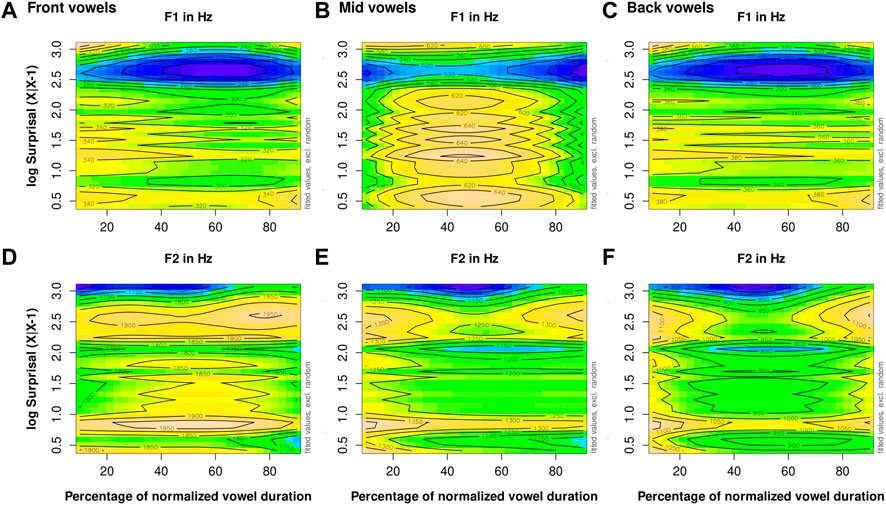
FIGURE 6. Stressed vowels: heatmap of the interaction of biphone surprisal of the preceding context (log Surprisal (X
When we plot the GAMM heatmaps (Figure 7) for the interaction of the measurement point and triphone surprisal of the preceding context for all stressed vowels in the corpus, we find quite different patterns in the formant trajectories from those observed for biphone surprisal of the preceding context (Figure 6). Stressed high surprisal (≥ −1.5) front, mid, and back vowels have lower F1 values than stressed low surprisal vowels. For F2, however, high surprisal vowels (≥ −2) overall have higher formant values than low surprisal vowels, again irrespective of their position in the vowel space. This means that high surprisal vowels are produced with more frontness than low surprisal vowels. It should be noted that triphone surprisal values of the preceding context (R = −4.5–2.8) have a larger range than biphone surprisal values of the same context direction (R = 0.4–3.1). Judging from visual inspection alone, the average first and second formant trajectories per vowel category (Figure 1) seem to be better presented by the interaction plots for the measurement point and biphone surprisal (Figure 6) than by the heatmaps displaying the interaction effect of triphone surprisal and the measurement point (Figure 7).
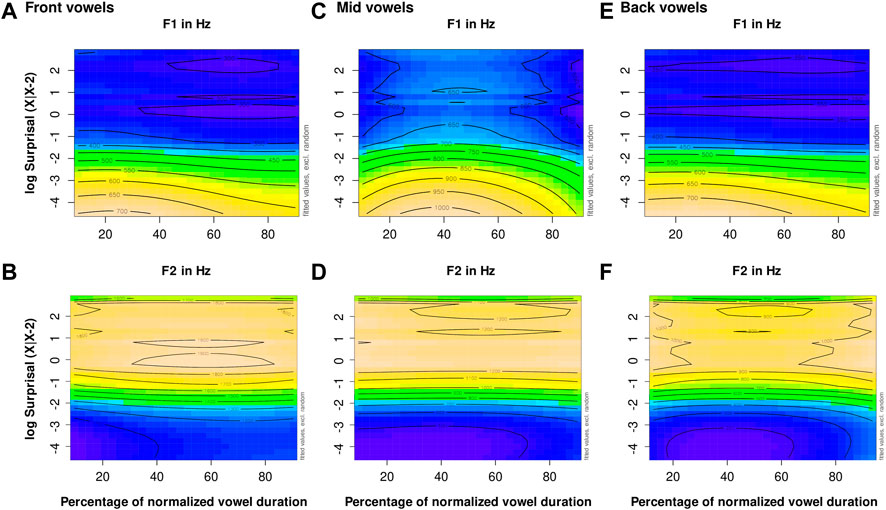
FIGURE 7. Stressed vowels: heatmap of the interaction of triphone surprisal of the preceding context (log Surprisal (X
Since we control for stress in the GAMMs, we can also investigate the impact of stress on the interaction between surprisal and the measurement point for different vowel phonemes, n-phone sizes, and forward and backward contextual predictability. For instance, Supplementary Figure S2 shows the GAMM heatmaps of the interaction of biphone surprisal of the preceding context and the measurement point for unstressed vowels. When comparing these heatmaps to their counterparts for stressed vowels (Figure 6), we see that F1 in front and back vowels has overall lower values for higher surprisal contexts in unstressed vowels compared to stressed vowels. F1 in unstressed mid vowels shows more pronounced movement than in stressed conditions, that is, lower F1 values, at the edges of the vowel. F2 values in unstressed high surprisal (≥ 2.5) vowels, especially in the beginning of the vowel, are much lower than the F2 trajectories for stressed vowels.
We proceed to make the same comparative analysis between the GAMM heatmaps of unstressed and stressed vowels for the interaction effect of triphone surprisal of the preceding context and measurement on formant movement in order to investigate potential influences of the n-phone size. Overall, we find that the relationship between the two factors surprisal and measurement point shows a higher degree of variability in formant movement in the GAMM heatmaps for unstressed vowels than that for stressed vowels. Interestingly, for stressed vowels, we observe that average first and second formant values are closely related to the triphone surprisal level (X
We test for surprisal with preceding and following context direction. The GAMM heatmaps for the interaction between surprisal and the measurement point look quite different when comparing different context directions (Supplementary Figures S1–S7). For instance, average formant values in stressed vowels are strongly influenced by biphone surprisal of the preceding context but less by the temporal domain expressed by the measurement point, while unstressed vowels in the same surprisal condition show more variability in their formant movement depending on surprisal and the measurement point. We saw a similar pattern for formant trajectories in unstressed vs. stressed vowels in models with triphone surprisal of the preceding context.
3.4 Word Frequency
During the modeling procedure, we excluded a tensor product interaction of the measurement point and word frequency and a smooth of word frequency by ordered stress from the model because they did not add to model performance. However, the simple smooth term for word frequency and the smooth for word frequency by ordered vowel added to the model. This means that F1 and F2 movements do not vary significantly per measurement point in vowels occurring in words with different frequencies, nor do they vary as a function of differences in word frequencies in stressed and unstressed vowels. The model output does, however, show that formant movement is explained by differences in word frequencies and differences in word frequencies by vowel phoneme.
3.5 Interaction Between Duration and Percentage
The interaction term between the vowel duration and measurement point adds to the explained variance in the F1 and F2 data modeled here. Formant movement is heavily influenced by the duration of the vowel and the measurement point during vowel duration.
Figure 8 shows GAMM heatmaps for the first and second formant trajectories in stressed German vowels as an interaction between the vowel duration and measurement point which is modeled by the tensor product interaction of the measurement point and duration.1
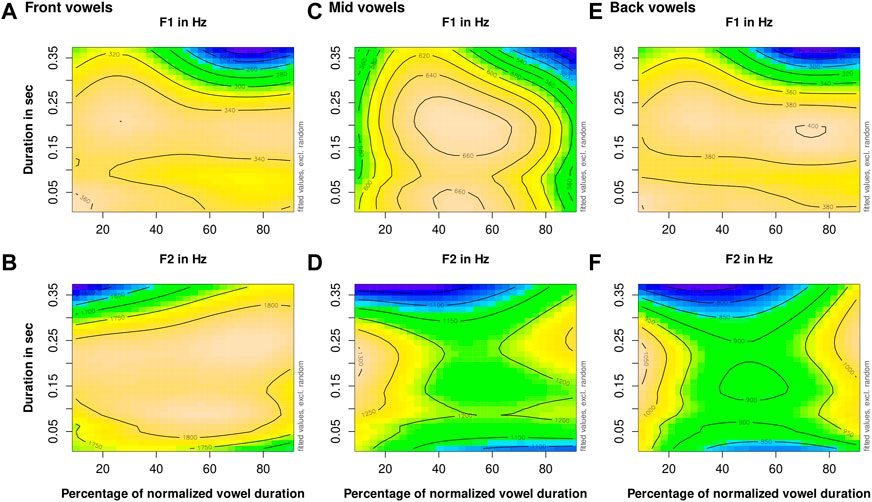
FIGURE 8. Stressed vowels: heatmap of the interaction of the vowel duration and measurement point on the trajectory of the first formant (A) and the second formant (B) per the vowel categories front (C), mid (D,E), and back (F).
In the GAMM heatmaps (Figure 8), we can observe the same overall formant trajectory shape for each vowel category that is given in Figure 1. The heat maps allow us to make more detailed observations about this overall shape, depending on vowel duration. Longer vowels above 0.25 s appear to show more pronounced first and second formant movement with lower minima than vowels with average or short duration. The peak of the F1 trajectory appears earlier in the vowel as a function of vowel duration when the vowel is longer than 0.25 s. We can also see that the average concave F2 trajectory shape for front vowels is mainly due to movement in long vowels, again above 0.25 s, while shorter front vowels show very little F2 movement. Very short vowels show surprisingly low F2 values for mid (around 1,100 Hz) and back (around 850 Hz) vowels.
3.6 Random Effects
The random smooths for the measurement point per speaker and the preceding and following contexts significantly add to the explained variability in F1 and F2 movement in all models.
4 Discussion
This study investigated whether variability in German formant trajectories can be explained by contextual predictability, measured as surprisal, and prominence, that is, primary lexical stress, as well as an interaction of both factors. We also include word frequency as an additional information-theoretic measure in our models. We use generalized additive mixed models (GAMMs) to compare the shape of formant trajectories in different surprisal contexts. Surprisal values are based on the biphone or triphone of the preceding or following context of the vowel. Only monophthongs in content words were considered in the study.
For average F1 and F2, we find expected results for different vowel phonemes that determine the position of the vowel within the acoustic vowel space. The significant interaction effect between the factors vowel and stress in the F1 and F2 models confirms that vowels in the stressed position are more dispersed in the vowel space than vowels in the unstressed position.
For the purpose of the study, we are particularly interested in the results of the smooth terms including surprisal. The GAMM output shows that the first and second formant trajectories in German are affected by surprisal in both context directions, that is, forward and backward, and by the interaction of surprisal and stress. We analyze these results in more detail via visual inspection of GAMM heatmaps that show the interaction effect of surprisal per measurement point (temporal domain) on the formant trajectory. We plot these heatmaps per vowel phoneme and stress condition because we find that these additional factors impact formant movement significantly. This procedure shows that the interaction effects of these factors on formant movement are highly complex. However, there are some general observations that we can make: unstressed vowels seem to show higher variability in their formant trajectory at different surprisal levels than stressed vowels. Differences in average formant values are also more readily expressed as a function of surprisal in stressed vowels than in unstressed vowels.
Our results show that effects of contextual predictability on formant variability are not limited to pointwise measurements of the vowel, as seen in studies on the effect of predictability on vowel dispersion (Malisz et al., 2018), but affect the dynamics throughout the entire vowel duration. When interpreted against the background of the uniform information density (UID) hypothesis (Levy and Jaeger, 2007), our findings add to the concept that the rational speaker uses optimization strategies in speech production throughout the entire utterance to ensure successful communication. This strategic behavior of the speaker also has an effect on the characteristics of formant movement and is observed while controlling for linguistic factors that are known to affect formant movement, such as vowel duration or phonetic context.
We proceed by further discussing our results with respect to the relation of prosodic prominence and predictability, especially in light of the smooth signal redundancy (SSR) hypothesis (Aylett and Turk, 2004, 2006). In addition, possible accounts of the effect of predictability on the phonetic structure are discussed.
4.1 Prosodic Prominence and Predictability Based Formant Movement
We test interaction effects between prosodic prominence and predictability on average first and second formant values and on formant movement in German vowels to investigate the effect of predictability and the prosodic structure, here primary lexical stress, on phonetic variability. This research goal is motivated by the smooth signal redundancy hypothesis (Aylett and Turk, 2004, 2006), which postulates that the effects of language redundancy or predictability on phonetic structures are moderated by the prosodic structure (prosodic prominence), that is, there are no independent or additive effects of predictability on phonetic variability. We can confirm this expected interaction effect between stress and surprisal on first and second average formant values and on formant trajectories.
Since German vowels in the stressed position and under high surprisal are known to be more dispersed in the vowel space (Malisz et al., 2018; Schulz et al., 2016), we would expect higher average F2 and lower average F1 values for front vowels in the stressed position and under high surprisal than for those in the unstressed position. Judging from the GAMM heatmaps for biphone surprisal of the preceding context, that is, the same surprisal measure as that used in our previous studies, we find the predicted pattern for front vowels. For back vowels, on the other hand, we expect lower average F1 and F2 values for stressed vowels in high surprisal contexts than for unstressed vowels. From visual inspection of the GAMM heatmaps in Figure 6 and Supplementary Figure S2, we cannot confirm this expectation for back vowels. For mid vowels /a, a/, we find that they are produced with more frontness in the unstressed condition under high surprisal than in stressed and high surprisal contexts.
We include an analysis of the impact of the temporal domain (interaction of the vowel duration and measurement point) on first and second formant trajectories, again distinguishing stress condition and vowel phoneme. While there are vast differences in formant movement depending on vowel duration, with longer vowels showing more formant movement than shorter vowels, the effect of stress on this relation appears to be small. This observation is partially in line with work that highlights the importance of time as a crucial factor for articulatory effort (Xu and Prom-on, 2010). The authors found that time constraints determine how much information speakers can convey in a conversational turn and hypothesized that speakers maximize their articulatory effort in unstressed vs. stressed vowels, which can also lead to increased dynamics for unstressed vowels compared to stressed vowels. Tang and Shaw (2020) noted that this principle applies to their findings on word duration as a function of predictability in Mandarin Chinese. The amount of time speakers allocate to a linguistic unit is a function of its importance, that is, less predictable words are produced with longer durations. In our study, we find more pronounced formant movement in unstressed vowels when investigating formant movement as a function of the surprisal and measurement point. Vowel duration and surprisal are, however, known to be correlated (Malisz et al., 2018).
Prosodic prominence, here estimated as primary lexical stress, was found to have a significant impact on the mean values of the first and second formants in German vowels in almost all GAMMs. In our models, the average F1 and F2 in stressed vowels are lower than those in unstressed vowels.
Lexically stressed American English vowels that are perceived as prominent are produced with a more open vocal tract than those vowels that are not perceived as prominent, resulting in higher F1 values for these vowels (Mo et al., 2009). Speakers are assumed to use this strategy to increase the sonority of prominent syllables (Beckman et al., 1992). For F2, or vowel frontness, vowels are hyperarticulated when they stand in a prominent position (Mo et al., 2009), supporting the hypo- and hyperarticulation hypothesis (Lindblom, 1996). This means that prominent back vowels are produced with lower F2 values and prominent front vowels are produced with higher F2 values than their non-prominent counterparts. This effect is captured by expanded vowel dispersion for stressed vowels in German (Schulz et al., 2016) and could also be replicated in our study.
The German vowel system, however, differentiates between tense and lax vowels, which can both stand in stressed or unstressed positions. German formant movement is largely influenced by vowel tenseness and frontness, that is, vowel identity. There are also known effects of stress on German tense vs. lax vowels: stressed tense vowels are longer and more peripheral in their position in the vowel space than unstressed tense vowels. Lax vowels, however, are not significantly affected by stress in their length or average formant values (Jessen, 1995; Mooshammer et al., 1999). Therefore, stress alone is possibly not an ideal factor to predict formant movement in German.
4.2 Accounts of the Effect of Predictability on Speech Variability
This study adds to previous accounts of predictability-based variability in the speech signal at the subword level. There are different accounts of these observed effects: the production ease account and the listener-oriented communicative account. Seminal work advocating the production ease account (e.g., Gahl, 2008; Bell et al., 2009) demonstrated the effect of frequency and predictability on word duration. The production planning hypothesis (Kilbourn-Ceron et al., 2020) views predictability as one of the factors that impact speech planning. Easily predictable phonological information in an upcoming word can facilitate the speech production process of pronunciation variants. The production ease account therefore relies on the contextual predictability of a linguistic structure based on both context directions, as it is known that coarticulatory processes have an effect on preceding and following neighboring phonemes. An alternative, but compatible, explanation has been offered by Tomaschek and others (Tomaschek et al., 2018a, b), who proposed that it is linguistic experience and articulation practice, rather than predictability as such, that shape articulatory trajectories.
The listener-oriented or communicative account, on the other hand, proposes that communication is a balancing act for the speaker between making the least possible amount of effort and attending to the listener’s need. As a result, predictable linguistic structures can be reduced because they are easily retrievable from their context, while structures that are difficult to predict from their context must be preserved. Therefore, both context directions (backward and forward) of contextual predictability play a role in this account. A strong interpretation of listener orientation in speech production is challenged by the finding that the speaker’s capacity to attribute mental states to others, also known as theory of mind (ToM) (Premack and Woodruff, 1978), does not necessarily lead to the phonetic reduction of predictable linguistic structures (Turnbull, 2019). It should be kept in mind however that high scores in ToM ability, as tested in the study by Turnbull (2019), estimate the speaker’s capacity of ToM but not their willingness to apply their ability to attribute mental states to others in a specific communicative setting. In our interpretation of these two accounts of the effect of predictability on speech variability, we note that both the listener-oriented and the production ease accounts rely on contextual predictability of linguistic structures that is based on the preceding and following contexts. There is also evidence from perception studies that listeners do not only utilize preceding information for word recognition in running speech but also following contextual information (Szostak and Pitt, 2013). This process seems to be modulated by contextual predictability in both directions. Listeners pay less attention to the phonetic details of easily predictable words (Manker, 2017).
With regard to our findings, surprisal based on the following context significantly explains the formant trajectory shape in German. This result is not necessarily expected since we also know from previous work that the effect of surprisal in different context directions depends on which acoustic measure is investigated. Segment duration can be explained by surprisal of the preceding and following contexts, whereas vowel dispersion is only predicted by surprisal of the preceding context (Malisz et al., 2018).
Data Availability Statement
Publicly available datasets were analyzed in this study. The monolingual German speech dataset analyzed for this study, the Siemens Synthesis Corpus (SI1000P), can be found in http://catalog.elra.info/en-us/repository/browse/ELRA-S0082/. The German text corpus used for language modeling is available at https://wacky.sslmit.unibo.it/doku.php?id = corpora.
Ethics Statement
Ethical review and approval was not required for the study on human participants in accordance with the local legislation and institutional requirements. The patients/participants provided their written informed consent to participate in this study.
Author Contributions
BM, BA, and EB contributed to the conception and design of the study. EB organized the database, performed the statistical analysis, and wrote the first draft of the manuscript. BM and BA wrote sections of the manuscript. All authors contributed to manuscript revision and read and approved the submitted version. Funding awarded to BM and BA.
Funding
This research was funded by the Deutsche Forschungsgemeinschaft (DFG, German Research Foundation)—Project-ID 232722074—SFB 1102 Information Density and Linguistic Encoding at Saarland University.
Conflict of Interest
The authors declare that the research was conducted in the absence of any commercial or financial relationships that could be construed as a potential conflict of interest.
Acknowledgments
We wish to thank Melanie Weirich for her helpful practical advice regarding GAMMs.
Supplementary Material
The Supplementary Material for this article can be found online at: https://www.frontiersin.org/articles/10.3389/fcomm.2021.643528/full#supplementary-material
Footnotes
1The equivalent GAMM heatmaps for the first and second formant trajectories in unstressed German vowels as an interaction between vowel duration and the measurement point can be found in Supplementary Figure S1. This allows us to investigate differences in formant trajectory due to the temporal domain. We include separate heatmaps of this interaction per vowel category since this factor significantly impacts formant movements (Section 3.1).
References
Aylett, M., and Turk, A. (2006). Language Redundancy Predicts Syllabic Duration and the Spectral Characteristics of Vocalic Syllable Nuclei. The J. Acoust. Soc. America 119, 3048–3058. doi:10.1121/1.2188331
Aylett, M., and Turk, A. (2004). The Smooth Signal Redundancy Hypothesis: a Functional Explanation for Relationships between Redundancy, Prosodic Prominence, and Duration in Spontaneous Speech. Lang. Speech 47, 31–56. doi:10.1177/00238309040470010201
Baroni, M., Bernardini, S., Ferraresi, A., and Zanchetta, E. (2009). The Wacky Wide Web: a Collection of Very Large Linguistically Processed Web-Crawled Corpora. Lang. Resour. Eval. 43, 209–226. doi:10.1007/s10579-009-9081-4
Beckman, M., Edwards, J., and Fletcher, J. (1992). “Prosodic Structure and Tempo in a Sonority Model of Articulatory Dynamics,” in Laboratory Phonology II: Gesture, Segment, Prosody. Editors G. J. Docherty, and D. R. Ladd (Cambridge, United Kingdom: Cambridge University Press), 68–89. doi:10.1017/cbo9780511519918.004
Bell, A., Brenier, J. M., Gregory, M., Girand, C., and Jurafsky, D. (2009). Predictability Effects on Durations of Content and Function Words in Conversational English. J. Mem. Lang. 60, 92–111. doi:10.1016/j.jml.2008.06.003
Benner, U., Flechsig, I., Dogil, G., and Möbius, B. (2007). “Coarticulatory Resistance in a Mental Syllabary,” in Proceedings of the International Congress of Phonetic Sciences (Saarbrücken, 485–488.
Bohn, O.-S., and Polka, L. (2001). Target Spectral, Dynamic Spectral, and Duration Cues in Infant Perception of German Vowels. J. Acoust. Soc. America 110, 504–515. doi:10.1121/1.1380415
Brandt, E., Andreeva, B., and Möbius, B. (2019). “Information Density and Vowel Dispersion in the Productions of Bulgarian L2 Speakers of German,” in Proceedings of the 19th International Congress of Phonetic Sciences (ICPhS 2019) Melbourne, Australia, 3165–3169.
Brandt, E. (2018). Information Density and Phonetic Structure: Explaining Segmental Variability. Ph.D. thesis. Saarbrücken: University of Saarland.
Brandt, E. (2019). Information Density and Phonetic Structure: Explaining Segmental Variability. Ph.D. thesis. Saarbrücken: Saarland University.
Bürki, A., Ernestus, M., Gendrot, C., Fougeron, C., and Frauenfelder, U. H. (2011). What Affects the Presence versus Absence of Schwa and its Duration: a Corpus Analysis of French Connected Speech. J. Acoust. Soc. America 130, 3980–3991. doi:10.1121/1.3658386
Carignan, C., Hoole, P., Kunay, E., Pouplier, M., Joseph, A., Voit, D., et al. (2020). Analyzing Speech in Both Time and Space: Generalized Additive Mixed Models Can Uncover Systematic Patterns of Variation in Vocal Tract Shape in Real-Time MRI. Lab. Phonology: J. Assoc. Lab. Phonology 11. doi:10.5334/labphon.214
Clopper, C. G., and Pierrehumbert, J. B. (2008). Effects of Semantic Predictability and Regional Dialect on Vowel Space Reduction. J. Acoust. Soc. America 124, 1682–1688. doi:10.1121/1.2953322
Cohen Priva, U., and Jaeger, T. F. (2018). The Interdependence of Frequency, Predictability, and Informativity. Linguistics Vanguard 4, 1–17. doi:10.1515/lingvan-2017-0028
Dautriche, I., Mahowald, K., Gibson, E., and Piantadosi, S. (2017). Wordform Similarity Increases with Semantic Similarity: an Analysis of 100 Languages. Cogn. Sci. 41, 2149–2169. doi:10.1111/cogs.12453
Demberg, V., Sayeed, A. B., Gorinski, P. J., and Engonopoulos, N. (2012). “Syntactic Surprisal Affects Spoken Word Duration in Conversational Contexts,” in Proceedings of the 2012 Joint Conference on Empirical Methods in Natural Language Processing and Computational Natural Language Learning (Jeju Island, Korea: Association for Computational Linguistics), 356–367.
Gahl, S. (2008). Thyme and Time Are Not Homophones: The Effect of Lemma Frequency on Word Durations in Spontaneous Speech. Language 84, 474–496. doi:10.1353/lan.0.0035
Gahl, S., Yao, Y., and Johnson, K. (2012). Why Reduce? Phonological Neighborhood Density and Phonetic Reduction in Spontaneous Speech. J. Mem. Lang. 66, 789–806. doi:10.1016/j.jml.2011.11.006
Hale, J. (2001). “A Probabilistic Early Parser as a Psycholinguistic Model,” in Proceedings of NAACL Stroudsburg, PA, 1–8.
Hale, J. (2016). Information-theoretical Complexity Metrics. Lang. Linguistics Compass 10, 397–412. doi:10.1111/lnc3.12196
Jaeger, T. F., and Buz, E. (2017). “Signal Reduction and Linguistic Encoding,” in Handbook of Psycholinguistic. Editors E. M. Fernandez, and H. M. I. Cairns (Oxford, United Kingdom: Wiley-Blackwell), 38–81. doi:10.1002/9781118829516.ch3
Jessen, M. (1995). Acoustic Correlates of Word Stress and the Tense/lax Opposition in the Vowel System of German. Int. Congress Phonetic Sci. (Stockholm) 4, 428–431.
Jurafsky, D., Bell, A., Gregory, M., and Raymond, W. D. (2001). “Probabilistic Relations between Words: Evidence from Reduction in Lexical Production,” in Frequency and the Emergence of Linguistic Structure. Editors J. Bybee, and P. Hopper (Amsterdam: Benjamins), 229–254. doi:10.1075/tsl.45.13jur
Kilbourn-Ceron, O., Clayards, M., and Wagner, M. (2020). Predictability Modulates Pronunciation Variants through Speech Planning Effects: A Case Study on Coronal Stop Realizations, Lab. Phonology: J. Assoc. Lab. Phonology, 11. doi:10.5334/labphon.168
Kirkham, S., Nance, C., Littlewood, B., Lightfoot, K., and Groarke, E. (2019). Dialect Variation in Formant Dynamics: The Acoustics of Lateraland Vowel Sequences in manchester and liverpool English. J. Acoust. Soc. America 145, 784–794. doi:10.1121/1.5089886
Kuperman, V., Pluymaekers, M., Ernestus, M., and Baayen, H. (2007). Morphological Predictability and Acoustic Duration of Interfixes in Dutch Compounds. J. Acoust. Soc. America 121, 2261–2271. doi:10.1121/1.2537393
Levy, R. (2008). “A Noisy-Channel Model of Rational Human Sentence Comprehension under Uncertain Input,” in Proceedings of the 13th Conference on Empirical Methods in Natural Language Processing. Honolulu: Waikiki, 234–243.
Levy, R. (2011). “Integrating Surprisal and Uncertain-Input Models in Online Sentence Comprehension: Formal Techniques and Empirical Results,” in Proceedings of the 49th Annual Meeting of the Association for Computational Linguistics (Portland, Oregon: Human Language Technologies), 1055–1065.
Levy, R., and Jaeger, T. F. (2007). Speakers Optimize Information Density through Syntactic Reduction. Adv. Neural Inf. Process. Syst. 19, 849–856.
Lindblom, B. (1996). Role of Articulation in Speech Perception: Clues from Production. J. Acoust. Soc. America 99, 1683–1692. doi:10.1121/1.414691
Möhler, G., Schweitzer, A., Breitenbücher, M., and Barbisch, M. (2000). IMS German Festival (Version: 1.2-os)
Malisz, Z., Brandt, E., Möbius, B., Oh, Y. M., and Andreeva, B. (2018). Dimensions of Segmental Variability: Interaction of Prosody and Surprisal in Six Languages. Front. Commun./Lang. Sci. 3, 1–18. doi:10.3389/fcomm.2018.00025
Manker, J. T. (2017). Phonetic Attention and Predictability: How Context Shapes Exemplars and Guides Sound Change. Ph.D. thesis. Berkeley: University of California.
Mo, Y., Cole, J., and Hasegawa-Johnson, M. (2009). “Prosodic Effects on Vowel Production: Evidence from Formant Structure,” in Proceedings of Interspeech. Brighton, UK), 2535–2538.
Möbius, B. (2001). German and Multilingual Speech Synthesis, Arbeitspapiere des Instituts für Maschinelle Sprachverarbeitung, AIMS, 7.
Möbius, B. (1999). The Bell Labs German Text-To-Speech System. Computer Speech Lang. 13, 319–358. doi:10.1006/csla.1999.0127
Mooshammer, C., Fuchs, S., and Fischer, D. (1999). “Effects of Stress and Tenseness on the Production of CVC Syllables in German,” in International Congress of Phonetic Sciences (San Francisco), 409–412.
Nearey, T. M., and Assmann, P. F. (1986). Modeling the Role of Inherent Spectral Change in Vowel Identification. J. Acoust. Soc. America 80, 1297–1308. doi:10.1121/1.394433
Oh, Y. M., Christophe, Coupé., Marsico, E., and Pellegrino, F. (2015). Bridging Phonological System and Lexicon: Insights from a Corpus Study of Functional Load. J. Phonetics 53, 153–176. doi:10.1016/j.wocn.2015.08.003
Pätzold, M., and Simpson, A. P. (1997). Acoustic Analysis of German Vowels in the Kiel Corpus of Read Speech. The Kiel Corpus Of Read/Spontaneous Speech Acoustic Data Base, Processing Tools and Analysis Results. Arbeitsberichte des Instituts für Phonetik und digitale Sprachverarbeitung der Universität Kiel (AIPUK) 32, 215–247.
Pellegrino, F., Coupé, C., and Marisco, E. (2011). A Cross-Language Perspective on Speech Information Rate. Language 87, 539–558. doi:10.1353/lan.2011.0057
Piantadosi, S., Tily, H., and Gibson, E. (2011). Word Lengths Are Optimized for Efficient Communication. Proc. Natl. Acad. Sci. 108, 3526–3529. doi:10.1073/pnas.1012551108
Pluymaekers, M., Ernestus, M., and Baayen, R. H. (2005a). Articulatory Planning Is Continuous and Sensitive to Informational Redundancy. Phonetica 62, 146–159. doi:10.1159/000090095
Pluymaekers, M., Ernestus, M., and Baayen, R. H. (2005b). Lexical Frequency and Acoustic Reduction in Spoken Dutch. J. Acoust. Soc. America 118, 2561–2569. doi:10.1121/1.2011150
Premack, D., and Woodruff, G. (1978). Does the Chimpanzeehave a Theory of Mind?. Behav. Brain Sci. 1, 515–526. doi:10.1017/s0140525x00076512
R Development Core Team, (2008). R: A Language and Environment for Statistical Computing. Vienna, Austria: R Foundation for Statistical Computing.
Raymond, W., Dautricourt, R., and Hume, E. (2006). Word-internal/t,d/Deletion in Spontaneous Speech: Modeling the Effects of Extra-linguistic, Lexical, and Phonological Factors. Lang. Variation Change 18, 55–97. doi:10.1017/s0954394506060042
Revelle, W. (2021). Psych: Procedures for Psychological, Psychometric, and Personality Research. Evanston, Illinois: Northwestern University.R package version 2.1.3.
Scarborough, R. (2010). “Lexical and Contextual Predictability: Confluent Effects on the Production of Vowels,” in Laboratory Phonology 10. Editors C. Fougeron, B. Kühnert, M. D’Imperio, and N. Vallee (Scarborough: Berlin: De Gruyther Mouton), 557–586.
Schulz, E., Oh, Y. M., Malisz, Z., Andreeva, B., and Möbius, B. (2016). “Impact of Prosodic Structure and Information Density on Vowel Space Size,” in Proceedings of Speech Prosody Boston, 350–354.
Shannon, C. E. (1948). A Mathematical Theory of Communication. Bell Syst. Tech. J. 27 (379–423), 623–656. doi:10.1002/j.1538-7305.1948.tb00917.x
Sóskuthy, M. (2017). Generalised Additive Mixed Models for Dynamic Analysis in Linguistics: a Practical Introduction. Working Paper 1–47.
Strange, W., and Bohn, O.-S. (1998). Dynamic Specification of Coarticulated German Vowels: Perceptual and Acoustical Studies. J. Acoust. Soc. America 104, 488–504. doi:10.1121/1.423299
Strange, W., Bohn, O.-S., Trent, S. A., and Nishi, K. (2004). Acoustic and Perceptual Similarity of North German and American English Vowels. J. Acoust. Soc. America 115, 1791–1807. doi:10.1121/1.1687832
Strange, W., Weber, A., Levy, E. S., Shafiro, V., Hisagi, M., and Nishi, K. (2007). Acoustic Variability within and across German, French, and American English Vowels: Phonetic Context Effects. J. Acoust. Soc. America 122, 1111–1129. doi:10.1121/1.2749716
Szostak, C. M., and Pitt, M. A. (2013). The Prolonged Influence of Subsequent Context on Spoken Word Recognition. Attention, Perception, Psychophysics 75, 1533–1546. doi:10.3758/s13414-013-0492-3
Tang, K., and Shaw, J. A. (2020). Prosody Leaks into the Memory of Words. Cognition 210, 104601. doi:10.1016/j.cognition.2021.104601
Tomaschek, F., Arnold, D., Bröker, F., and Baayen, R. H. (2018a). Lexical Frequency Co-determines the Speed-Curvature Relation in Articulation. J. Phonetics 68, 103–116. doi:10.1016/j.wocn.2018.02.003
Tomaschek, F., Tucker, B. V., Fasiolo, M., and Baayen, H. (2018b). Practice Makes Perfect: the Consequences of Lexical Proficiency for Articulation. Linguistic Vanguard 4. doi:10.1515/lingvan-2017-0018
Tucker, B. V., Sims, M., and Baayen, H. (2019). Opposing Forces on Acoustic Duration. doi:10.31234/osf.io/jc97w
Turnbull, R. (2019). Listener-oriented Phonetic Reduction and Theory of Mind. Lang. Cogn. Neurosci. 34, 747–768. doi:10.1080/23273798.2019.1579349
van Rij, J., Wieling, M., Baayen, R. H., and van Rijn, H. (2017). Itsadug: Interpreting Time Series and Autocorrelated Data Using Gamms. R package version 2.3.
Wedel, A., Nelson, N., and Sharp, R. (2018). The Phonetic Specificity of Contrastive Hyperarticulation in Natural Speech. J. Mem. Lang. 100, 61–88. doi:10.1016/j.jml.2018.01.001
Wieling, M. (2018). Analyzing Dynamic Phonetic Data Using Generalized Additive Mixed Modeling: A Tutorial Focusing on Articulatory Differences between L1 and L2 Speakers of English. J. Phonetics 70, 86–116. doi:10.1016/j.wocn.2018.03.002
Wood, S. N. (2011). Fast Stable Restricted Maximum Likelihood and Marginal Likelihood Estimation of Semiparametric Generalized Linear Models. J. R. Stat. Soc. 73, 3–36. doi:10.1111/j.1467-9868.2010.00749.x
Wood, S. N. (2003). Thin-plate Regression Splines. J. R. Stat. Soc. (B) 65, 95–114. doi:10.1111/1467-9868.00374
Wright, R. (2004). “Factors of Lexical Competition in Vowel Articulation,” in Papers in Laboratory Phonology VI. Editors J. Local, R. Ogden, and R. Temple (Cambridge: Cambridge University Press), 26–50.
Xu, Y., and Prom-on, S. (2010). Economy of Effort or Maximum Rate of Information? Exploring Basic Principles of Articulatory Dynamics. Front. Psychol. doi:10.3389/fpsyg.2019.02469
Keywords: information theory, surprisal, predictability, formant trajectories, German, read speech, prominence
Citation: Brandt E, Möbius B and Andreeva B (2021) Dynamic Formant Trajectories in German Read Speech: Impact of Predictability and Prominence. Front. Commun. 6:643528. doi: 10.3389/fcomm.2021.643528
Received: 18 December 2020; Accepted: 31 May 2021;
Published: 21 June 2021.
Edited by:
Rory Turnbull, Newcastle University, United KingdomReviewed by:
Fabian Tomaschek, University of Tübingen, GermanyJoey Stanley, Brigham Young University, United States
Copyright © 2021 Brandt, Möbius and Andreeva. This is an open-access article distributed under the terms of the Creative Commons Attribution License (CC BY). The use, distribution or reproduction in other forums is permitted, provided the original author(s) and the copyright owner(s) are credited and that the original publication in this journal is cited, in accordance with accepted academic practice. No use, distribution or reproduction is permitted which does not comply with these terms.
*Correspondence: Erika Brandt, YnJhbmR0QGxlaWJuaXotemFzLmRl