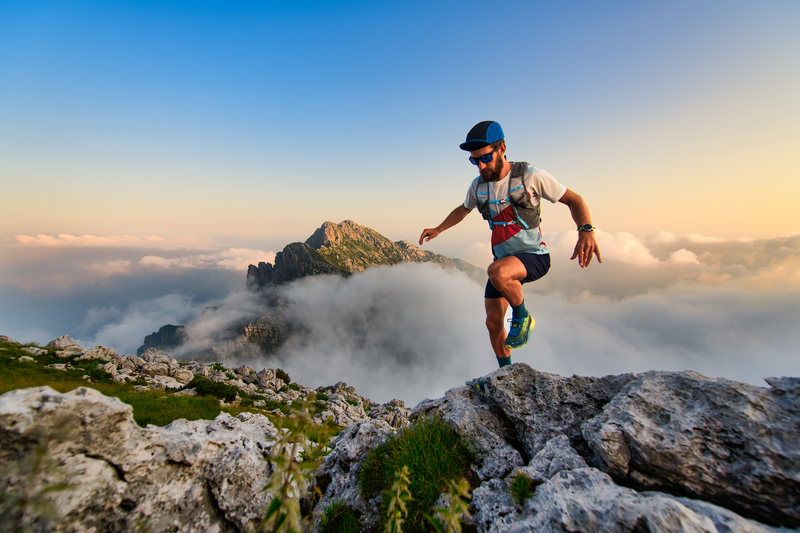
94% of researchers rate our articles as excellent or good
Learn more about the work of our research integrity team to safeguard the quality of each article we publish.
Find out more
REVIEW article
Front. Commun. , 24 May 2021
Sec. Media Governance and the Public Sphere
Volume 6 - 2021 | https://doi.org/10.3389/fcomm.2021.632317
This article is part of the Research Topic The Age of Mass Deception: Manipulation and Control in Digital Communications View all 7 articles
We review the phenomenon of deepfakes, a novel technology enabling inexpensive manipulation of video material through the use of artificial intelligence, in the context of today’s wider discussion on fake news. We discuss the foundation as well as recent developments of the technology, as well as the differences from earlier manipulation techniques and investigate technical countermeasures. While the threat of deepfake videos with substantial political impact has been widely discussed in recent years, so far, the political impact of the technology has been limited. We investigate reasons for this and extrapolate the types of deepfake videos we are likely to see in the future.
Since the invention of photography in the 19th century, visual media have enjoyed a high level of trust by the general public, and unlike audio recordings, photos and videos have seen a widespread use as evidence in court cases (Meskin and Cohen, 2008), and it is widely accepted that visual media are a particularly effective propaganda tool (Winkler and Dauber, 2014). Consequently, the incentives for creating forged visual documents have always been high. Extensive use of manipulated images for political purposed is documented as early as the 1920s by the Soviet Union (Dickerman, 2000; King, 2014).
On the other hand, video manipulation took skilled experts and a significant amount of time to create, since every frame had to be changed individually. The technology for manipulating videos was perfected in Hollywood in the 1990s (Pierson, 1999), but it was so expensive that only a few movies made full use of it. Consequently, creating manipulated videos for the purpose of political propaganda was rare. However, a technology known as deepfake that allows manipulation of entire videos with limited effort and consumer-grade computing hardware has recently become available. It leverages modern artificial intelligence to automate repetitive cognitive tasks such as identifying the face of a person in every frame of a video and swapping it for a different face, thus making the creation of such a manipulated video rather inexpensive.
Thus, the fundamental change does not lie in the quality of manipulated media, but in the easy accessibility. Given moderate technical skills, input video material, and consumer grade computer equipment, almost anyone can create manipulated videos today (Hall, 2018; Beridze and Butcher, 2019). However, so far deepfake videos have not played the prominent role in politics that many initially feared (Chesney and Citron, 2019a), even though the year 2020 has seen a massive amount of other misinformation, especially connected to the COVID-19 pandemic and the US presidential election.
In this article we investigate possible explanations for this development and discuss the likely future developments and identify areas in which deepfakes are expected to be effective and thus likely to appear in the future. We review the deepfake technology, present the latest developments in an accessible manner, and discuss its implications in the context of historical media manipulation. We focus only on the manipulation of visual media in the sense of creating or changing media directly such that they show contents that does not match physical reality, and we exclude misinformation created by incorrect labelling, suggestive editing, and similar techniques.
Individual images are relatively easy to manipulate via the technique of retouching. The technique was already in widespread use in the 1920s, most prominently in the Soviet Union (King, 2014). A prominent example is the removal of Alexander Malchenko from official photos after his execution in 1930 (Dickerman, 2000; King, 2014) (Figure 1). While this example, along with many others, and the possibility of manipulating photos in general has been commonly known for a long time, the effort involved in the creation of manipulated images was comparatively high until recently. Furthermore, experts were typically capable of detecting such manipulations. Consequently, photos, unlike audio recordings, generally retained public confidence. Even today, the term “photographic evidence” is somewhat commonly used, even though this confidence seems to be in decline (Meskin and Cohen, 2008).
FIGURE 1. Left: Alexander Malchenko, P. Zaporozhets, and Anatoly Vaneyev (standing); Victor V. Starkov, Gleb Krzhizhanovsky, Vladimir Lenin, and Julius Martov (sitting). Right: manipulated image with Malchenko removed. Source: Wikipedia (Wikipedia, 2020).
The manipulation of images has been available to the general public since the 1990s. In fact, it is so common today that the term “to photoshop,” named after the Adobe Photoshop program (Adobe, 2020), is being used as a verb for the act of manipulating images. Typically, this refers to minor manipulations such as making a person conform to some beauty ideal, but larger manipulations for commercial or entertainment purposes are abundant (Reddit, 2020). Naturally, these techniques can be and have been used for the purposes of propaganda. With the help of image processing software, the manipulation shown in Figure 1, i.e., the removal of a person from an individual image, requires a few minutes of work by a skilled user nowadays.
Today, manipulations such as removing a person can be facilitated further for both individual images as well as larger numbers of images via the use of artificial intelligence (AI). For example, NVIDIA Image Inpainting (NVIDIA, 2020) is a simple tool for removing persons or objects from photos. Figure 2 shows the original image with Alexander Malchenko (left), alongside a second version (right) where we had marked Malchenko for removal by the NVIDIA Inpainting tool. The effort required by the user is very small. First, the part of the image to be removed must be specified. Then, the system determines how to fill the created gap by analyzing the remaining picture.
FIGURE 2. Operation of NVIDIA Image Inpainting. Left: Image selection. Right: Selection of the contents to be removed. Source: Created by the authors using NVIDIA InPaint (NVIDIA, 2020). Image from Wikipedia (Wikipedia, 2020).
The result is shown in Figure 3. The manipulation is not perfect, mostly due to the fact that the part of the image specified for removal is replaced by an artificial background created from scratch. There exist commercial services that perform essentially the same function but deliver better results. However, they make use of human judgement and they charge for each image to be manipulated (Business Insider, 2017), which limits this approach to small numbers of pictures. In a similar manner, instead of removing a person, it is also possible to replace them with a different person, typically by replacing the face. Automated tools that can do so, e.g., a program called Reflect by NEOCORTEXT (Reflect, 2020), are available, but they have similar limitations when working on individual images.
FIGURE 3. Output image of NVIDIA Image Inpainting. The result shows some signs of manipulation. Source: Wikipedia. Created by the authors using NVIDIA Inpainting (NVIDIA, 2020). Image from Wikipedia (Wikipedia, 2020).
On the other hand, it is much easier for AI systems to remove a person from a video if the person is moving. In that case, the video will contain images of the background, and all the AI has to do is take the correct background from one video frame and place it over the person in a different frame, thereby effectively removing the person. Figure 4 shows an example of this. It was created using Inpainting from a video showing two people walking by. Because the entire static background is contained in the video, the persons can be removed perfectly, i.e., a human observer would not detect such a manipulation. Thus, the additional information of the video makes it easier to create a believable manipulation automatically. A similar manipulation would be possible with multiple photos of the same scene from different angles.
FIGURE 4. Removal of persons from a video. A manipulation of the image on the right is not visible here. Source: Still from a video created by the authors using NVIDIA Inpainting (NVIDIA, 2020).
In the 1940s, the total number of images taken worldwide was comparatively low. Thus, the number of pictures that documented a single event was typically so small that by manipulating individual photos, it was possible to establish a contrafactual narrative. On the other hand, today the manipulation of an individual image carries little weight for political purposes. With the proliferation of digital photography and smartphones that are carried constantly, the number of pictures taken per year has exploded to an estimated 1.2 trillion pictures taken in 2017 (Business Insider, 2017). Thus, for important events, it is usually no longer possible to establish a narrative by censoring or manipulating individual images when thousands of original images exist. Doing so would require manipulating a large number of images automatically. On the other hand, if a large number of images or videos of an event is available, it becomes possible to create believable manipulations using artificial intelligence automatically, assuming most of the material can be accessed and changed. Simple versions of such systems have been used for a while to, e.g., censor pornographic content in online platforms (Gorwa et al., 2020). Similarly, Google Street View automatically detects and blurs faces and license plates for privacy reasons. But the same technology could be used for far more malicious purposes such as automatically removing or replacing people or events from all accessible video documents.
Thus, as long as the same entity has access to many or all images of an event, it could conceivably use advanced AI to manipulate or censor most images taken of a given event. Due to the fact that today most photos are taken by smartphones that are permanently connected to cloud computing servers, in a country that has central control of the internet this is technologically feasible. According to the University of Hong Kong, some variants of this technology have been implemented in China (University of Hong Kong, 2020). However, very few other governments have both the technological capability as well as the constitutional right to implement such a system. Thus, in the following we focus on videos by individuals or small organizations rather than state actors.
Video manipulation traditionally requires a much higher amount of effort and technical skill than photo manipulation. The large number of frames (i.e., images) that need to be manipulated, together with the need for consistency in the manipulation, creates high technological barriers to successful video manipulation. However, just as in the case of photo manipulation, the cost of doing so can be reduced greatly due to the use of new machine learning techniques.
Until recently, direct manipulation of the video material was rare, and a more common way of video-based misinformation was mislabeling, i.e., videos that claim to show something different from what they actually show, or suggestive editing, i.e., cutting authentic video material in such a way that it misrepresents the situation that was filmed (Matatov et al., 2018). In order to separate such techniques from deepfakes, the term shallowfakes has been suggested (European Science Media Hub, 2019). A third case is the manipulation of the actual video contents at a level that does not require AI. Such videos are sometimes referred to as cheapfakes. A video showing a politician that was reencoded at reduced speed, thus giving the impression of slurred speech, is the most well-known example of a cheapfake (Donovan and Paris, 2019). While such techniques can be very effective, they are not new and thus they will not be discussed here as we only focus on AI based manipulation of video contents.
To a large degree, the technology for directly manipulating video material was developed for and by the movie industry, mainly in Hollywood. Milestones include Jurassic Park (1993), that added believable computer-generated dinosaurs to filmed scenes, and Forest Gump (1995), where Tom Hanks is inserted into historic footage of John F. Kennedy. Avatar (2009) showed that given a large enough budget, almost anything can be brought to the screen. However, with the exception of political campaign advertisement, which does not fall under most standard definitions of misinformation, there are very few known cases of the use of this technology for propaganda. A likely reason is the fact that the cost of deploying this technology was very high. Movies containing a large number of high-quality CGI effects typically cost more than US$ 1 million per minute of footage. However, this has radically changed due to the introduction of AI based methods for video manipulation. In the following, we will discuss the methods that make this possible.
In 2017, users of the online platform Reddit presented videos of celebrities whose faces were swapped with those of different persons. While the effect was novel, the software that created these images relied on a technology that had been developed a few years earlier.
In a landmark paper in 2012, deep learning, a refinement of artificial neural networks, was established as a superior technology for image recognition (Krizhevsky et al., 2012). From there, an immense body of work has emerged in the recent years, proposing both refinements of the method and extensions to other application areas. In addition, this development has had a considerable impact outside the scientific community, and brought the topic of artificial intelligence to the attention of politics, industry, and media (Witness Lab, 2020).
While the mathematical foundations had been known for decades, the 2012 paper demonstrated that when trained with a large number of suitable input images, convolutional neural networks (CNNs) can categorize the contents of a picture with high accuracy. The key to do so lies in the abstract representation of a type of object (e.g., chair) in the higher levels of the deep neural network, whose structure resembles that of the visual cortex in the human brain. A CNN can be trained to recognize specific people and to reliably tell them apart on a wide range of images. The prerequisites for doing so are a powerful computer and a large number of images from which a CNN learns.
Once trained, CNNs and other neural networks can be inverted. This is done by specifying an output, and then performing the inverse mathematical operations for each layer in reverse order. Strictly speaking, not all operations can be inverted, but this does not limit the applicability of the concept. The result is an image being created from the abstract features that the network has learned. The original input layer then acts as the output layer. It produces an image of the same resolution as the images that were originally used to train the CNN. A neural network requires a fixed image resolution, but images can easily be scaled. We call a CNN that has been trained to recognize a specific person a detector network, and the inverted version of it a generator network. The deepfake technology relies on combining both types.
It is possible to link a detector and its corresponding generator. Such a system is called an autoencoder, because it learns to encode an image–in our case the face of a person–in some abstract way as a result of its training. Given enough training data, the network can also recognize a face in a noisy image or an unusual angle. Because it represents the face internally in an abstract manner, it can output the face without noise using the generator network.
Deepfakes for face swapping are created by training two such autoencoders. One network is trained to recognize the target person whose face is to be replaced by that of the source person, while the other network is trained to recognize the source person. Then, the detector for the source person is linked to the generator for the target person, thus creating a new autoencoder. When applied to a video of the source person, the result is that the face of the target person appears instead of the face of the source person. The design is shown in Figure 5. This creates the impression that the target person is doing whatever the source person was doing in the input video, thereby creating a substantial potential for manipulation and misinformation.
FIGURE 5. Basic technology for the creation of deepfakes. Network A is trained to recognize the source person even from distorted or noisy images, and configured such that it outputs a noiseless picture of the target person. Network B is trained on the target person in the same manner. After training, the output of the detector of the source person is fed as input to the generator of the target person. Thus, wherever the source person is detected in an input video, it is replaced by the target person.
The technology described above became publicly available in 2017. Programs that implement it formed the first generation of deepfake software, which was followed by two further generations of increasing sophistication. We will discuss the software generations here. Individual programs are presented in the Supplementary Appendix.
As discussed above, the first generation of deepfake software required a large number of training images to function properly. Consequently, these programs are impractical for creating manipulated videos of an average person. For that reason, most deepfake videos that were created for entertainment purposes featured famous actors of which many images are publicly available. The first-generation software is described in detail in Supplementary Appendix A.
However, the second generation of deepfake software no longer has this restriction. This is due to the use of generative adversarial networks (GANs) (The Verge, 2020). GANs are a type of neural network similar to autoencoders. However, in a GAN the detector and the generator networks work against each other. The task of the generator is to create variants of images that are similar but not identical to original inputs by adding random noise. Using these generated images, the detector network, which is also called discriminator in this context, is trained as shown in Figure 6. In this manner, the discriminator network becomes very good at recognizing variants of the same image, such as a face seen from many different angles, even if there are few original images to train from.
FIGURE 6. Basic setup of a generative adversarial network. The generator creates images by adding random noise to input images, while the discriminator network is fed both original input images and generated images. Source: Image created by authors from photo taken by authors.
Note that unlike the first generation, which contains easy to use software, most of the second generation of deepfake generators are research codes which require considerable technical skill to be used successfully. However, it would certainly be possible to create user-friendly software from them. The most prominent programs making use of this concept for the second generation of deepfake software are described in detail in Supplementary Appendix B.
Typically, creating a manipulative video requires the creation of a manipulative audio track. However, compared to video, manipulating audio is a fairly simple task. In fact, in many judicial systems it is significantly harder to establish audio recordings as evidence (Al-Sharieh and Bonnici, 2019). Furthermore, imperfect audio quality can always be masked to appear as a lack of microphone quality in the original recording. Thus, for creating convincing deepfake, audio manipulation is a minor challenge. Nonetheless, the effect can be considerable, as manipulated audio has been used successfully for cybercrime (Stupp, 2019). Two programs that can generate audio for deepfake are described in Supplementary Appendix C.
The latest generation of deepfake software builds upon the second generation, but extends its capabilities in several ways. It significantly increases model complexity, combines multiple generator networks in one model, and extracts features from linked time-series. This allows working in a face-features space rather than in 2D-frame space and simulating changes from frame-to-frame in a naturally looking way. They usually include audio directly in order to generate natural lip movements. We present the two most important software packages in Supplementary Appendix D.
Given the potential threat of deepfakes, different types of countermeasures against them have been considered. We discuss both technical and legal countermeasures here.
Reacting to the threat posed by manipulated visual media, the Defense Advanced Research Projects Agency (DARPA) of the United States established the media forensics project with the goal of developing tools for recognizing manipulation in videos and images (Darpa, 2020), using a wide variety of tools such as semantic analysis. In that context, Adobe, creator of the popular Photoshop software, announced a program capable of detecting most image manipulations Photoshop is capable of (Adobe Communications Team, 2018). The technology builds on long term research at the University of Maryland (Zhou et al., 2018). Furthermore, large sets of data for training and testing detection algorithms have recently become available (Dolhansky et al., 2019; Guan et al., 2019; Rossler et al., 2019).
Many ideas for AI based deepfake detectors have been tested in a competitive challenge organized by Facebook in 2020 (Dolhansky et al., 2020; Ferrer et al., 2020). The challenge resulted in several new approaches for detectors (Mishra, 2020). An overview over the field of media forensics in relation to fake news was recently given by Verdoliva (Verdoliva, 2020).
While these approaches show promise, their success depends ultimately on their mode of deployment. Furthermore, recent research (Gandhi and Jain, 2020; Neekhara et al., 2020) using adversarial strategies (Goodfellow et al., 2014) indicates that even the current best detectors can be fooled. Adversarial strategies consist of adding noise to a video or image. This noise is imperceptible to the human eye, but it is sufficient to confuse a fake news detector.
Thus, it is likely that many of these systems are ultimately flawed in their practical application because they do not offer a 100% detection accuracy, and if they are available to the general public, they will also be available to the creators of misinformation. Even a 99% detection accuracy means that the system can be defeated in some way. For state-of-the-art image recognition, it is known that a large number of adversarial examples, i.e. pairs of input images that will be recognized as the same object by humans, but as very different objects by neural networks, exist (Szegedy et al., 2013; Gu and Rigazio, 2014). Thus, a manipulation detector built on the same technology would exhibit the same weaknesses, and could thus be conceivably defeated with minimal changes to the manipulated content. Figure 7 illustrates the situation.
FIGURE 7. Two different scenarios for the deployment of manipulation filters. Left: the assumed scenario is that the user has a 99% accurate detector that filters out almost all manipulated content. Right: in a realistic scenario, the source of the misinformation has access to the same detector. Consequently, only manipulated content that passes the detector is released to the public, rendering the detector almost useless. Source: Image created by authors from MS ClipArt.
The problem is similar to that of virus detection software (Fedler et al., 2013; Rastogi et al., 2013). Within the last 3 decades, the problem of malware was not solved by the use of detection software running on user systems. By the same token, it is unlikely that the situation will be different for manipulated media. The following statement concisely describes the phenomenon: “Currently it is trivial for malware authors to slightly alter existing malware, with the effect that it will not be detected by antivirus software until new signatures have been released.” (Fedler et al., 2013). For the detection of malware and spam, centralized instances that cannot be accessed and tested in an unlimited manner by malware creators have seen a measure of success. For example, software on smartphones can typically be installed only through controlled channels (Apple App Store, Google Play), and the measures to circumvent this limitation are generally restricted to technically savvy users. Confirmed malware is regularly being removed from these channels. This approach can be considered comparatively successful. A likely reason for this is that the providers of smartphone operating systems have a strong incentive to keep malware away from their systems, and thereby provide value for their customers.
However, for the distribution of news, such a centralized system controlled by one company is clearly not a desirable solution. The hallmark of a healthy media landscape in a democratic society is a diverse selection of independent sources of news. Thus, central control over what is “truth,” even in the benign form of manipulation detection, carries an immense risk of abuse. In addition, such an instance would likely be subject to political pressure, in the same way that social networks such as Facebook or Twitter are often subject to pressure from private or state actors to remove certain kinds of contents from their services. Thus, a preferable solution would be open, decentralized, and accessible to all. The technical challenge lies in building such a solution that is nonetheless effective.
In addition to detection software, there are also other technological approaches, such as the New York Times' News Provenance Project (Koren, 2019) that makes use of blockchains to ensure that a video has not been tampered with (Hasan and Salah, 2019b). As they are based on cryptography rather than artificial intelligence, they do not suffer from the accuracy problems discussed above. However, they would need wide adoption to be effective.
Aside from technological solutions, there might be leagal approaches to tackle the problem of abuse of deepfake software. One could argue that banning the technology would fix the problem, but such a heavy-handed approach would make a large portion of satire, humor, internet memes, and possibly even movies illegal and is thus inconceivable in a democratic society. Neither the spreading of fake images nor the use of deepfake software is inherently harmful, and a lot of benign applications exist. For the same reason, it is rather unlikely that social media companies will ban their use or distribution entirely (Chesney and Citron, 2019b). Still, there is a noticeable legislative push in the United States towards restricting harmful use of deepfakes (Ruiz, 2020).
However, experience with new laws against cyberbullying, cyberstalking, online harassment and hate speech, etc., has shown, introducing new laws or changing existing laws to deal with deepfakes might lead to a whole new set of problems, such as hastily executed self-censorship by social media companies to avoid fines (Chesney and Citron, 2019b). Since the intent of a deepfake is more important than the technology itself, it might be possible to deal with fakes by applying already existing laws against fraud, harassment, or other forms of defamatory statements (Brown, 2020).
Considering the limitations of technological and legal countermeasures, it seems that first and foremost, societies need to develop resilience to deepfakes as formulated by Robert Chesney and Danielle Citron (Chesney and Citron, 2019b):
In short, democracies will have to accept an uncomfortable truth: in order to survive the threat of deepfakes, they are going to have to learn how to live with lies.
To date, a whole subgenre of deepfake parody videos has established itself on video platforms like YouTube, with switching the main actors of a movie being the most common manipulation. For example, a short film named Home Stallone (Face, 2020)—a reference to the 1990 comedy movie Home Alone—features an 8 year-old AI generated Sylvester Stallone instead of the real actor, Macaulay Culkin. Many similar videos are available on the internet.
In 2020, several cases of politically motivated deepfake videos made appearance on the news. On February 7, 2020, during the Legislative Assembly elections in Delhi, two video messages surfaced, showing the leader of the Delhi Bharatiya Janata Party (BJP), Manoj Tiwari, addressing his potential voters in English and Haryanvi. According to VICE India, the videos were shared in over 5,800 WhatsApp groups, reaching about 15 million people (Vice, 2020). Surprisingly, Manoj Tiwari does not speak Haryanvi, nor has he ever recorded the video message in English or any other language. An Indian PR company called “The Ideaz Factory” had taken an older video message of Manoj Tiwari where he spoke about a totally different topic–in Hindi. Then they trained an AI with videos of Manoj Tiwari speaking, until it was able to lip synchronize arbitrary videos of him. Then they used a voice artist to record the English and Haryanvi and merged both audio and video (Khanna, 2020).
On April 14, 2020, the hashtag #TellTheTruthBelgium caught media attention. A video showed a nearly 5 min speech of Belgian premier Sophie Wilmès, depicting the COVID-19 pandemic as a consequence of environmental destruction. The environmental movement Extinction Rebellion used deepfake technology to alter a past address to the nation that Sophie Wilmès held previously (Extinction Rebellion, 2020; Galindo, 2020).
Another example is an appeal addressed to Mexican president Andrés Manuel López Obrador. In the video published on October 29, 2020, Mexican author and journalist Javier Valdez prompts president López Obrador and his administration, to fight harder against corruption and organized crime. Javier Valdez was murdered on May 15, 2017, as his digital alter ego explains in the beginning of the video. Presumably he was killed as a consequence of his investigations into organized crime. For 1 min and 39 s, the “Defending Voices Program for the Safety of Journalists” brought Mr. Valdez back to life using deepfake technology to demand justice for killed and missing journalists. The Defending Voices Program for the Safety of Journalists is a cooperation between the Mexican human rights NGO “Propuesta Cívica” and the German journalists association “Reporter ohne Grenzen” (Journalists without Borders) (Reporter ohne Grenzen, 2020).
While many applications of deepfake technology are humorous or benign, they harbor an inherent possibility of misuse. The above examples are not malicious by intent, the case of Manoj Tiwari shows that it is indeed possible to mislead voters by using synthetic media. The other two examples were published accompanied by a disclaimer, stating that deepfake technology was used. It has been widely suspected that deepfakes would be used to influence the 2020 United States presidential election. However, at the time of this writing, video manipulation has relied more on conventional techniques (Politifact, 2020; The Verge, 2020). Instead, deepfake videos that warn about threats to democracy have been released (Technology Review, 2020). Thus, so far it seems that outright fabrications rarely make it to the public sphere where they can be debunked, but it is possible that such videos are circulated in closed groups with the intent of mobilizing supporters. Videos involving foreign politicians seem to be especially viable since it is harder for people to judge whether the depicted behavior is believable for that person (Schwartz, 2018).
For now, deepfakes do not primarily target the political sphere. The Dutch startup Sensity. ai (Sensity, 2020), which traces and counts deepfake videos available on the internet, reports that more than 85% of all deepfake videos target female celebrities in the sports, entertainment, and fashion industries. Some of these constitute cases of so-called involuntary pornography where the face of the target person is placed in a pornographic source video. Initially, this required large amounts of images of the target person and thus, only celebrities were targets. However, recent deepfake technology based on generative adversarial networks makes it possible to target persons of whom only a small number of images exist.
Thus, considering the wide availability of this technology, using deepfakes for cyberbullying has become a relevant threat. Such an event, called the Nth Room scandal (International Business Times, 2020), happened in South Korea in 2019. The event involved the production and distribution of pornographic deepfake videos using the faces of female celebrities, along with other exploitative practices. Similarly, an extension for the popular messaging app Telegram, called DeepNude (Burgess, 2020), became available in 2019 and reappeared in 2020. Given a photo of the target person, it essentially replaces the clothed body with a naked body, thereby creating the likeness of a naked picture of the target person. The program is relatively unsophisticated, and was apparently only trained to work for white women. However, its design essentially removes all remaining barriers from the creation of potentially harmful contents. Since it relies on external servers, it is conceivable that more sophisticated programs that deliver high quality images or videos will appear in the future.
A different application of deepfake technology is the impersonation of other individuals in online communication. In 2019, criminals used audio manipulation to impersonate a CEO on a phone call and order an employee to transfer a large amount of money to a private account (Stupp, 2019). Recently, a software that allows impersonation in video calls using deepfake technology was released (Siarohin et al., 2019). Clearly such a technology has a wide range of possible criminal applications, the most concerning among them being the use by sexual predators to impersonate minors (Foster, 2019; Hook, 2019).
We have seen that the deepfake technology has the potential to create severely damaging videos. On the other hand, so far, the number of cases where this happened is limited. This may be due to the novelty of the technique. In that case, we should expect a massive increase of deepfake videos in the near future. However, it is also possible that they will replace traditional disinformation only in a fairly limited number of situations.
In order to gain a deeper understanding of the likely future cases, it is necessary to consider the differences between deepfakes and other disinformation. Deepfakes are visual in nature, and they contain a large amount of information. This can increase credibility, but it can also provide more details that discredit the disinformation, such as visible artifacts.
The COVID-19 pandemic has been the dominant news event of the year 2020, and it has been accompanied by a large amount of misinformation. In spring 2020, the European External Action Service’s East StratCom Task Force pointed out that “in times of a pandemic medical disinformation can kill” (EU vs DisInfo, 2020). However, to the best of our knowledge, there are no impactful cases of deepfake videos spreading COVID-19 misinformation.
Since the virus is invisible, it is difficult to spread visual misinformation about it, such as the claim that COVID-19 is caused by the 5G wireless network radiation (Temperton, 2020) (which is also invisible). The 5G-COVID misinformation was predominantly spread by YouTube videos that show people who talk about such conspiracy theories. Clearly, the effectiveness of such misinformation depends on the credibility and persuasiveness of the speakers, which cannot be enhanced by deepfakes. However, it is possible to create footage of a credible speaker to spread misinformation. So far, there are few recorded cases. It remains to be seen whether this becomes a significant vector for spreading disinformation.
However, deepfakes are more suited for spreading disinformation in cases where video recordings are typically effective. Such situations depict actions of recognizable persons whose significance is comprehensible without a lot of specific context and which elicit an emotional response. The reason for these conditions is based on what deepfake technology is able to do. Swapping faces is not necessary if the persons involved are not recognizable individuals (e.g. police or soldiers in uniform), although it is conceivable that deepfake technology will be used in future war propaganda by replacing uniforms or nationality markings. This may be even more relevant to for civil wars and conflicts involving militant groups that are not formal armies (Cameroon, 2020).
Furthermore, for eliciting a rational response, textual disinformation can often be as effective, and it is much easier to produce at a high volume, but video is effective at creating an emotional response (Nelson-Field et al., 2013).
Furthermore, as deepfake videos may contain visual clues to the fact that they do not depict reality, they could even be less effective than text. Thus, the gain from deepfake produced for this purpose is unlikely to outweigh the cost. On the other hand, emotional responses are likely to be amplified by seeing a video. And in order to elicit an emotional response, the video itself must lead the viewers to this response, rather than relying on additional explanations. Thus, we can formulate five conditions which characterize deepfakes that are effective for political manipulation. We expect that most significant future deepfakes will fulfill these conditions:
1. Involve recognizable persons
2. Depict human actions
3. Can be understood with limited additional context
4. Elicit an emotional response
5. The response is significant enough to affect opinions or incite action
Clearly videos involving nudity or sexual activity of known individuals fulfill these conditions, and such incidents have happened (Ayyub, 2018; Walden, 2019). While the case involving a Malaysian cabinet minister (Walden, 2019) was apparently not based on an actual deepfake, it is clear that an actual deepfake video would have been even more effective if it had been available to the perpetrators. Thus, it is to be expected that similar cases will happen again in the near future. However, since the effect of such disinformation campaigns depends to a large extent on cultural norms, the effect may only be restricted to parts of the world. Another likely area would be violent behavior or some other physical transgression of social norms by a known individual. On the other hand, videos that only show a known person speaking are less likely to be effective deceptions, at least not significantly more effective than other disinformation techniques.
An example of a video manipulation that fulfills all the above criteria happened in Germany in 2015 where a video of an obscene gesture and its subsequent denial by Greek Finance Minister Yanis Varoufakis caused an uproar, with major German newspapers writing hostile articles until a satirical TV show host revealed that the video was a forgery he created (The Guardian, 2015). The video was manipulated professionally, and it was widely considered to be genuine. While the incident was essentially benign, with the goal of entertainment as well as cautioning the public against premature judgement, it showed that manipulated videos can deepen existing tensions between countries. Using deepfake technology, the ability to perform such manipulations becomes available to almost everyone.
In addition to cyberbullying and political disinformation, another possible area in which deepfakes might be used in the future is market manipulation. In the past, even tweets containing misinformation have had a significant impact on market prices. For example, in July 2012, a Twitter message falsely claimed the death of the Syrian President Bashar al-Assad, causing crude oil prices to temporarily rise by more than US$ 1/barrel (Zero Hedge, 2020). In 2013, an exclusionary statement made by Abercrombie and Fitch CEO Mike Jeffries in 2006 about the target audience of the Abercrombie and Fitch brand gained widespread attention, and caused the brand value of Abercrombie and Fitch to plummet as a result (Bradford, 2017). Thus, a deepfake video that shows a CEO making a socially unacceptable statement or perform unacceptable actions (e.g., sexual harassment) could conceivably be used to damage a value of a company. Since it is possible to benefit from such an event via financial markets, it is sufficient that enough people believe the disinformation after its release, even if it is universally accepted to be false later.
We have seen that while photo and even video manipulation has a long history, it is only recent technological developments that have undermined the trustworthiness of video evidence. Modern deepfake software can easily generate videos that a casual observer might perceive as perfectly real. Furthermore, automated systems can be defeated easily if the attacker can test and, if needed, modify, the manipulated video. Thus, with no easy technical solution in sight, and much like with disinformation and fake news in general, education and media literacy remain the foremost defense against disinformation.
However, the impact of deepfakes should not be overestimated. On the one hand, disinformation is widespread, and conventional disinformation has proven effective. Thus, even though the creation of deepfakes is cheap, it still requires more effort than producing texts. Consequently, they will only replace other methods of disinformation where an enhanced effect can be expected.
On the other hand, just because an artificially created video cannot be distinguished from a recorded video does not mean that people can be made to believe that arbitrary event happened. Today, video content creators on video platforms such as YouTube, TikTok, and Reddit compete for attention. Due to significant monetary incentives, content creators are pushed towards presenting dramatic or shocking videos, which in turn leads them to stage dramatic or shocking situations, and staged videos are often labeled as such by viewers. Thus, regular users of these video platforms are becoming increasingly aware of manipulative videos. By the same token, increased proliferation of deepfake videos will eventually make people more aware that they should not always believe what they see.
JL is the main author of this paper. KP investigated and formulated the research trends in deepfake technology. SB collected numerous examples and contributed substantial editing work. PF revised the interdisciplinary aspect of the article and provided additional editing. DS contributed additional ideas.
This work was funded by the Norwegian SAMRISK-2 project “UMOD” (No. 272019). The research presented in this paper has benefited from the Experimental Infrastructure for Exploration of Exascale Computing (eX3), which is financially supported by the Research Council of Norway under contract 270053.
The authors declare that the research was conducted in the absence of any commercial or financial relationships that could be construed as a potential conflict of interest.
The Supplementary Material for this article can be found online at: https://www.frontiersin.org/articles/10.3389/fcomm.2021.632317/full#supplementary-material
Adobe (2020). Photoshop. Available at: https://www.adobe.com/products/photoshop.html (Accessed April 1, 2020).
Adobe Communications Team (2018). Spotting image manipulation with AI. Available at: https://theblog.adobe.com/spotting-image-manipulation-ai/ (Accessed April 1, 2020).
Al-Sharieh, S., and Bonnici, J. M. (2019). “STOP, you’re on camera: the evidentiary admissibility and probative value of digital records in europe,” in Synergy of community policing and technology. Editors G. Leventakis, and M. R. Haberfeld (Cham, Switzerland: Springer), 41–52.
Ayyub, R. (2018). I was the victim of A deepfake porn plot intended to silence me. Available at: https://www.huffingtonpost.co.uk/entry/deepfake-porn_uk_5bf2c126e4b0f32bd58ba316 (Accessed April 1, 2020).
Beridze, I., and Butcher, J. (2019). When seeing is no longer believing. Nat. Mach Intell. 1 (8), 332–334. doi:10.1038/s42256-019-0085-5
Bradford, H. (2017). Abercrombie and fitch’s reputation takes A hit after CEO’s ‘fat’ comments resurface. Available at: https://www.huffpost.com/entry/abercrombie-reputation-ceo-comments_n_3288836 (Accessed April 1, 2020).
Burgess, M. (2020). A deepfake porn bot is being used to abuse thousands of women. Available at: https://www.wired.co.uk/article/telegram-deepfakes-deepnude-ai (Accessed April 1, 2020).
Business Insider (2017). People will take 1.2 trillion digital photos this year—thanks to smartphones. Available at: https://www.businessinsider.com/12-trillion-photos-to-be-taken-in-2017-thanks-to-smartphones-chart-2017-8?r=US&IR=T (Accessed April 1, 2020).
Cameroon, B. H. (2020). The bellingcat podcast season 2-the executions. Available at: https://www.bellingcat.com/resources/podcasts/2020/07/21/the-bellingcat-podcast-season-2-the-executions/ (Accessed April 1, 2020).
Chesney, B., and Citron, D. (2019a). Deep fakes: a looming challenge for privacy, democracy, and national security. Calif. L. Rev. 107, 1753. doi:10.2139/ssrn.3213954
Chesney, R., and Citron, D. (2019b). Deepfakes and the new disinformation war. Available at: https://www.foreignaffairs.com/articles/world/2018-12-11/deepfakes-and-new-disinformation-war (Accessed April 1, 2020).
Darpa (2020). Media forensics (MediFor). Available at: https://www.darpa.mil/program/media-forensics (Accessed April 1, 2020).
Dickerman, L. (2000). Camera obscura: socialist realism in the shadow of photography. October 93, 139–153. doi:10.2307/779160
Dolhansky, B., Bitton, J., Pflaum, B., Lu, J., Howes, R., Wang, M., et al. (2020). The deepfake detection challenge dataset. Preprint repository name [Preprint]. Available at: arXiv:2006.07397.
Dolhansky, B., Howes, R., Pflaum, B., Baram, N., and Ferrer, C. C. (2019). The deepfake detection challenge (dfdc) preview dataset. Preprint repository name [Preprint]. Available at: arXiv:1910.08854.
Donovan, J., and Paris, B. (2019). Beware the cheapfakes. Available at: https://slate.com/technology/2019/06/drunk-pelosi-deepfakes-cheapfakes-artificial-intelligence-disinformation.html (Accessed April 1, 2020).
EU vs DisInfo (2020). Disinformation can kill. Available at: https://euvsdisinfo.eu/disinformation-can-kill/ (Accessed April 1, 2020).
European Science Media Hub (2019). Deepfakes, shallowfakes and speech synthesis: tackling audiovisual manipulation. Available at: https://sciencemediahub.eu/2019/12/04/deepfakes-shallowfakes-and-speech-synthesis-tackling-audiovisual-manipulation/ (Accessed April 1, 2020).
Extinction Rebellion (2020). The prime minister’s speech by our rebels. Available at: https://www.extinctionrebellion.be/en/tell-the-truth/the-prime-ministers-speech-by-the-rebels (Accessed April 1, 2020).
Face, C. S. (2020). Home Stallone [DeepFake]. Available at: https://www.youtube.com/watch?v=2svOtXaD3gg (Accessed April 1, 2020).
Fedler, R., Schütte, J., and Kulicke, M. (2013). On the effectiveness of malware protection on android. Fraunhofer AISEC 45.
Ferrer, C. C., Dolhansky, B., Pflaum, B., Bitton, J., Pan, J., and Lu, J. (2020). Deepfake detection challenge results: an open initiative to advance AI. Available at: https://ai.facebook.com/blog/deepfake-detection-challenge-results-an-open-initiative-to-advance-ai/ (Accessed April 1, 2020).
Foster, A. (2019). How disturbing AI technology could be used to scam online daters. Available at: https://www.news.com.au/technology/online/security/how-disturbing-ai-technology-could-be-used-to-scam-online-daters/news-story/1be46dc7081613849d67b82566f8b421 (Accessed April 1, 2020).
Galindo, G. (2020). XR Belgium posts deepfake of Belgian premier linking Covid-19 with climate crisis. Available at: https://www.brusselstimes.com/news/belgium-all-news/politics/106320/xr-belgium-posts-deepfake-of-belgian-premier-linking-covid-19-with-climate-crisis/ (Accessed April 1, 2020).
Gandhi, A., and Jain, S. (2020). Adversarial perturbations fool deepfake detectors. Preprint repository name [Preprint]. Available at: arXiv:2003.10596. doi:10.1109/ijcnn48605.2020.9207034
Goodfellow, I. J., Shlens, J., and Szegedy, C. (2014). Explaining and harnessing adversarial examples. Preprint repository name [Preprint]. Available at: arXiv:1412.6572.
Gorwa, R., Binns, R., and Katzenbach, C. (2020). Algorithmic content moderation: technical and political challenges in the automation of platform governance. Big Data Soc. 7 (1), 2053951719897945. doi:10.1177/2053951719897945
Gu, S., and Rigazio, L. (2014). Towards deep neural network architectures robust to adversarial examples. Preprint repository name [Preprint]. Available at: arXiv:1412.5068.
Guan, H., Kozak, M., Robertson, E., Lee, Y., Yates, A. N., Delgado, A., et al. (2019). “MFC datasets: large-scale benchmark datasets for media forensic challenge evaluation,” in IEEE winter applications of computer vision workshops (WACVW), Waikoloa, HI, January 7–11, 2019 (IEEE), 63–72.
Hasan, H. R., and Salah, K. (2019). Combating deepfake videos using blockchain and smart contracts. IEEE Access 7, 41596–41606. doi:10.1109/access.2019.2905689
Hook, C. (2019). Warning snapchat ‘baby’ filter could be a gift to online child predators. Available at: https://7news.com.au/technology/snapchat/warning-snapchat-baby-filter-could-be-a-gift-to-online-child-predators-c-114074 (Accessed April 1, 2020).
International Business Times (2020). Nth Room scandal: here is how Jo Joo Bin victimized popular female idols with deepfake pornography. Available at: https://www.ibtimes.sg/nth-room-scandal-here-how-jo-joo-bin-victimized-popular-female-idols-deepfake-pornography-42238 (Accessed April 1, 2020).
Khanna, M. (2020). How BJP used deepfake for one of its Delhi campaign videos and why it’s dangerous. Available at: https://www.indiatimes.com/technology/news/how-bjp-used-deepfake-for-one-of-its-delhi-campaign-videos-and-why-its-dangerous-506795.html (Accessed April 1, 2020).
King, D. (2014). The commissar vanishes: the falsification of photographs and art in stalin's Russia. London, United Kingdom: Tate Publishing.
Koren, S. (2019). Introducing the news provenance project. Available at: https://open.nytimes.com/introducing-the-news-provenance-project-723dbaf07c44 (Accessed April 1, 2020).
Krizhevsky, A., Sutskever, I., and Hinton, G. E. (2012). “Imagenet classification with deep convolutional neural networks,” in Advances in neural information processing systems, Lake Tahoe, Nevada, December 3–6, 2012, 1097–1105.
Matatov, H., Bechhofer, A., Aroyo, L., Amir, O., and Naaman, M. (2018). “DejaVu: a system for journalists to collaboratively address visual misinformation,” in Computation+ journalism symposium (Miami, FL: Harvard University).
Meskin, A., and Cohen, J. (2008). “Photographs as evidence,” in Photography and philosophy: essays on the pencil of nature. Editor S. Walden (Hoboken, New Jersey: Wiley), 70–90.
Mishra, R. (2020). “Fake news detection using higher-order user to user mutual-attention progression in propagation paths,” in Proceedings of the IEEE/CVF conference on computer vision and pattern recognition workshops, Seattle, WA, June 16–18, 2020 (IEEE), 652–653.
Neekhara, P., Hussain, S., Jere, M., Koushanfar, F., and McAuley, J. (2020). Adversarial deepfakes: evaluating vulnerability of deepfake detectors to adversarial examples. Preprint repository name [Preprint]. Available at: arXiv:2002.12749.
Nelson-Field, K., Riebe, E., and Newstead, K. (2013). The emotions that drive viral video. Australas. Marketing J. 21 (4), 205–211. doi:10.1016/j.ausmj.2013.07.003
NVIDIA (2020). Image inpainting. Available at: https://www.nvidia.com/research/inpainting/ (Accessed April 1, 2020).
Pierson, M. (1999). CGI effects in hollywood science-fiction cinema 1989-95: the wonder years. Screen 40 (2), 158–176. doi:10.1093/screen/40.2.158
Politifact (2020). Video of ‘ballot stuffing’ is not from a flint, mich., polling place. it’s from Russia. Available at: https://www.politifact.com/factchecks/2020/nov/05/facebook-posts/video-ballot-stuffing-filmed-russia-not-flint-mich/ (Accessed April 1, 2020).
Rastogi, V., Chen, Y., and Jiang, X. (2013). “DroidChameleon: evaluating android anti-malware against transformation attacks,” in Proceedings of the 8th ACM symposium on information, computer and communications security, Hangzhou, China, May 8–10, 2013 (ASIACCS).
Reddit (2020). Photoshopbattles: a place to have fun with everyone's favorite graphic software. Available at: https://www.reddit.com/r/photoshopbattles/ (Accessed April 1, 2020).
Reflect (2020). Realistic face swap. Available at: https://reflect.tech/ (Accessed April 1, 2020).
Reporter ohne Grenzen (2020). Mexiko javier valdez fordert gerechtigkeit für ermordete medienschaffende reporter ohne grenzen. Available at: https://www.youtube.com/watch?v=ZdIHUrCobuc (Accessed April 1, 2020).
Rossler, A., Cozzolino, D., Verdoliva, L., Riess, C., Thies, J., and Nießner, M. (2019). “Faceforensics++: learning to detect manipulated facial images,” in Proceedings of the IEEE international conference on computer cision, Seoul, Korea, October 27–November 2, 2019, 1–11.
Ruiz, D. (2020). Deepfakes laws and proposals flood United States. Available at: https://blog.malwarebytes.com/artificial-intelligence/2020/01/deepfakes-laws-and-proposals-flood-us/ (Accessed April 1, 2020).
Schwartz, O. (2018). You thought fake news was bad? deep fakes are where truth goes to die. Guardian, November2018.
Sensity (2020). The world's first detection platform for deepfakes. Available at: https://sensity.ai/ (Accessed April 1, 2020).
Siarohin, A., Lathuilière, S., Tulyakov, S., Ricci, E., and Sebe, N. (2019). “First order motion model for image animation,” in Advances in neural information processing systems, Vancouver, Canada, December 8–14, 2019, 7137–7147.
Stupp, K. (2019). Fraudsters used AI to mimic CEO’s voice in unusual cybercrime case. Available at: https://www.wsj.com/articles/fraudsters-use-ai-to-mimic-ceos-voice-in-unusual-cybercrime-case-11567157402.
Szegedy, C., Zaremba, W., Sutskever, I., Bruna, J., Erhan, D., Goodfellow, I., et al. (2013). Intriguing properties of neural networks. Preprint repository name [Preprint]. Available at: arXiv:1312.6199.
Technology Review (2020). Deepfake putin is here to warn americans about their self-inflicted doom. Available at: https://www.technologyreview.com/2020/09/29/1009098/ai-deepfake-putin-kim-jong-un-us-election/ (Accessed April 1, 2020).
Temperton, J. (2020). The rise and spread of a 5G coronavirus conspiracy theory. Available at: https://www.wired.com/story/the-rise-and-spread-of-a-5g-coronavirus-conspiracy-theory/ (Accessed April 1, 2020).
The Guardian (2015). I faked Yanis Varoufakis middle-finger video, says german TV presenter. Available at: https://www.theguardian.com/world/2015/mar/19/i-faked-the-yanis-varoufakis-middle-finger-video-says-german-tv-presenter. (Accessed April 1, 2020).
The Verge (2020). Bloomberg debate video would violate Twitter’s deepfake policy, but not facebook’s. Available at: https://www.theverge.com/2020/2/20/21146227/facebook-twitter-bloomberg-debate-video-manipulated-deepfake (Accessed April 1, 2020).
Thies, J., Zollhofer, M., Stamminger, M., Theobalt, C., and Nießner, M. (2016). “Face2face: real-time face capture and reenact- ment of rgb videos,” in Proceedings of the IEEE conference on computer vision and pattern recognition, Las Vegas, Nevada, June 26–July 1, 2016 (IEEE), 2387–2395.
University of Hong Kong (2020). Weiboscope project. Available at: https://weiboscope.jmsc.hku.hk/64CensoredPics/ (Accessed April 1, 2020).
Verdoliva, L. (2020). Media forensics and deepfakes: an overview. IEEE J. Sel. Top. Signal. Process. 14 (5), 910–932. doi:10.1109/JSTSP.2020.3002101
Vice (2020). Here’s how deepfakes, like the one BJP used, twist the truth. Available at: https://www.vice.com/en/article/939d4p/bjp-manoj-tiwari-deepfake-twists-truth (Accessed April 1, 2020).
Walden, M. (2019). Malaysian cabinet minister allegedly depicted in gay sex video, accused of corruption. Available at: https://mobile.abc.net.au/news/2019-06-12/gay-sex-video-sparks-scandal-for-malaysian-government/11201834?pfmredir=sm (Accessed April 1, 2020).
Wikipedia (2020). Files. Available at: https://en.wikipedia.org/wiki/File:Union-de-Lucha.jpg (Accessed April 1, 2020).
C. K. Winkler, and C. E. Dauber (Editors) (2014). Visual propaganda and extremism in the online environment. Carlisle, PA: Army War College Carlisle Barracks Pa Strategic Studies Institute.
Witness Lab (2020). Prepare, don’t panic: synthetic media and deepfakes. Available at: https://lab.witness.org/projects/synthetic-media-and-deep-fakes/ (Accessed April 1, 2020).
Zero Hedge (2020). Supposedly fake tweets about Syrian president Assad’s death cause all-too-real spike crude. Available at: http://www.zerohedge.com/news/supposedly-fake-tweets-about-syrian-president-assads-death-cause-all-too-real-spike-crude-and-s (Accessed April 1, 2020).
Keywords: deepfakes, visual media manipulation, fake news, disinformation, generative adversarial networks
Citation: Langguth J, Pogorelov K, Brenner S, Filkuková P and Schroeder DT (2021) Don't Trust Your Eyes: Image Manipulation in the Age of DeepFakes. Front. Commun. 6:632317. doi: 10.3389/fcomm.2021.632317
Received: 23 November 2020; Accepted: 25 January 2021;
Published: 24 May 2021.
Edited by:
Daniel Broudy, Okinawa Christian University, JapanReviewed by:
Vian Bakir, Bangor University, United KingdomCopyright © 2021 Langguth, Pogorelov, Brenner, Filkuková and Schroeder. This is an open-access article distributed under the terms of the Creative Commons Attribution License (CC BY). The use, distribution or reproduction in other forums is permitted, provided the original author(s) and the copyright owner(s) are credited and that the original publication in this journal is cited, in accordance with accepted academic practice. No use, distribution or reproduction is permitted which does not comply with these terms.
*Correspondence: Johannes Langguth, bGFuZ2d1dGhAc2ltdWxhLm5v
Disclaimer: All claims expressed in this article are solely those of the authors and do not necessarily represent those of their affiliated organizations, or those of the publisher, the editors and the reviewers. Any product that may be evaluated in this article or claim that may be made by its manufacturer is not guaranteed or endorsed by the publisher.
Research integrity at Frontiers
Learn more about the work of our research integrity team to safeguard the quality of each article we publish.