- Media Studies, University of Trier, Trier, Germany
With the emergence of the Internet, social media and video platforms are gaining considerable influence on the traditional media landscape in general and on science communication in particular. This has changed the role of science journalists as gatekeepers because many platforms are based on a participatory culture, in which passive consumers can become active participants. In addition to scientists, non-scientific actors also act as experts and participate in the communication process between science and the public. In contrast to the relevance of YouTube for science communication there is a lack of research focusing on the questions of how internet users receive YouTube videos to acquire information about science, how successful audiovisual media function in knowledge transfer, and what effects it has on the epistemic regime of a society. Therefore, this study combines a discourse analysis with the aim to create a typology of YouTube videos—the independent variables—and an audience study for investigating knowledge transfer—the dependent variables. In the first step, this article presents the results of a systematic analysis and categorization of 400 German science videos, from which four types of audiovisual science communication on YouTube were derived: presentation films, expert films, animation films, and narrative explanatory films. In order to clarify how powerful these new forms of science communication are in terms of knowledge transfer, attitudes, and trust toward the presentation of science, a discourse analysis of the videos is combined with a multi-level reception study and an online survey. The reception study included eye-tracking to investigate the allocation of attention and two different methods of knowledge tests (recognition and recall) of which the multiple-choice test was also applied in the online survey. The results show that the type of video has an important impact on knowledge transfer and para-social effects. One of the central results of the audience study is that the videos' gaze guidance, the recipients' allocation of attention, and the results of knowledge testing are closely intertwined. The correlation of data from eye-tracking and the two knowledge tests prove in principle that the more homogeneous the gaze patterns of the recipients are, the better they score in the multiple-choice test as well as in the concept mapping test.
Introduction
Already in 1985, the Royal Society demanded “more science in the media” in the context of the program “Public Understanding of Science” and recommended to promote scientific issues using all available media channels like broadcast programs, newspaper articles, news programs on the radio, drama series, children programs or popular science books (The Royal Society, 1985, p. 21–23). Disseminating science issues in online videos and particularly in YouTube videos can be viewed as a continuation of this program's intention to promote “awareness of the nature of science and, more particularly, of the way that science and technology pervade modern life” (The Royal Society, 1985, p. 5; see also: Hallman, 2017) via media. Since then, various new formats for science communication have been developed in all media, like TV shows, documentaries, science (fiction) novels or even science comics (see Bucher and Boy, 2018). The basic tension between the respective media logic on the one side and intentions and standards of science on the other side (Schäfer, 2017; Bucher, 2020), which characterizes all these enterprises, also affects the presentation of science in different YouTube formats. The processes resulting from these tensions between science, media and the public have been investigated in detail on an abstract level and were coined as mediatization (Rödder and Schäfer, 2010; Weingart, 2012; Bucher, 2020). Correspondingly in the case of YouTube videos the question arises how the “cultural and commercial infrastructure” of YouTube as a multichannel platform (Lobato, 2016) influences the quality of science communication. According to the approaches on mediatization, the following study focuses on the impact of typical YouTube features like audiovisual genres and discourse structures on the transfer of scientific knowledge. Despite a long-lasting debate about the concept of “public understanding of science” (Bucchi, 2008; Schäfer et al., 2020) the approach of investigating science communication on YouTube using a reception study is guided by the basic idea, that individuals' understanding of science is antecedent to public understanding of science. Applying the concept of media appropriation (Bucher and Schumacher, 2006) to the analysis or reception processes designates the shift from a top-down public understanding of science model to an interactive and constructivist model of science communication and knowledge transfer.
The increasing relevance of social media and video platforms for science communication and its participatory culture (Minol et al., 2007; Brossard, 2013; Neuberger and Jarren, 2017) has a considerable impact on the publication and dissemination of scientific content. First, the communication of scientific knowledge by scientists, research institutes, research organizations, and universities has become stepwise independent of traditional media. In addition to scientists, in online-communication non-scientific actors are also acting as experts and actively participate in the communication process between science and the public (Nisbet and Scheufele, 2009; Lo et al., 2010; Lobato, 2016; Welbourne and Grant, 2016). The range of videos on YouTube on scientific topics is correspondingly diverse, including channels operated by scientific institutions, universities, research institutions, or so-called “YouTubers,” as well as classic science journalism programs, filmed lectures and talks. The multitude of videos and actors confronts the recipients with the question which sources of information are trustworthy, reputable, and objective. Especially the “passionate amateurs,” as Welbourne and Grant (2016) call them, who neither work as journalists nor scientist, but as “YouTubers” are actors who are gaining followers (de Lara et al., 2017, p. 14–16). Given these delimitations of science communication via social media, the fundamental question arises as to whether this newly created communication space represents a democratic transformation of science communication from a distribution model to a participation model (see Gibbons et al., 1994) or whether we are dealing with an erosion of a traditional epistemic order (Schäfer et al., 2020). Despite a growing number of publications on online science videos (see inter alia Allgaier, 2016; Erviti and Stengler, 2016; Geipel, 2018; León and Bourk, 2020; Rosenthal, 2020), there is hardly any research on the reception processes triggered by the audiovisual modality of these videos.
The addressees' contact with the stimulus determines the success, efficiency, and sustainability of science communication. Thus, the reception of science communication is the starting point of this study. It examines how recipients consume videos on scientific topics, their knowledge acquisition, and how they evaluate the videos using various methods. The project takes three main steps: First, audiovisual material, distributed on YouTube and television online media centers, is collected and systemized to establish a typology of video formats based on a grounded theory approach. The identified video types are the starting point for the second research step, encompassing several reception study methods, including eye-tracking, questionnaires, interviews, and knowledge tests. This combination of product and reception analysis ensures that the results can be used as a basis for the optimization of the production of audiovisual science communication. In a third step, the consecutive postings of YouTube videos were analyzed using conversation analysis methods to get more insight into the appropriation of audiovisual content and the rationality or emotionality of scientific discourses triggered by a video. Investigating the interaction in YouTube's comment space is a prerequisite for developing strategies to moderate these discourses enabled by social media's participatory potentials.
Literature Review
Since the advent of visual media in the first half of the nineteenth century, science communication was one of the first domains of public discourse to benefit from what Jonathan Crary called “the mass visual culture of the nineteenth century” (Crary, 1990, p. 16). The adoption of visual media like Laterna Magica, woodcarving, photography and film was motivated by the conviction that visualization has the potential to enhance the communication between science and the public (for details: Bucher, 2020). The career of YouTube and in general online videos is just another chapter in this history.
According to a national survey in Germany (Koch and Beisch, 2020) online video is one of the most dynamic and fastest growing area of the Internet. Eighty-three percent of the German population watch online videos regularly, whereas for persons younger than 30 years, online videos are even more popular than television (for international data see: León and Bourk, 2020, p. 9–11). The most prominent platform in Germany for watching online videos with the highest reach in comparison to streaming services (47% of the German population) and media centers (57%) is YouTube (65%). As a bandwagon effect of this development, online videos in general and YouTube, in particular, has become “a powerful tool to communicate science and technology to the general public” (León and Bourk, 2020, p. 2; see also: de Lara et al., 2017). This growing relevance of online videos and particularly YouTube for science communication has triggered increasing research activities focusing on content, authorship, epistemic quality and impacts on science communication. The most common approaches are case studies investigating, for example, participatory aspects of YouTube (Erviti and León, 2016; Dubovi and Tabak, 2020), the role of YouTube videos for internal science communication (Kousha et al., 2012), the coverage of controversial issues like climate change or vaccines (Shapiro and Park, 2015; Allgaier, 2016, 2019; Donzelli et al., 2018; Erviti et al., 2020), the role of user comments for the scientific discourse of lay-persons (Heydari et al., 2019; Christ, 2020; Dubovi and Tabak, 2020), the motivations for watching science videos on YouTube (Rosenthal, 2018) or the differences between user-generated content and professionally generated content (de Lara et al., 2017). Besides these case studies, there are already some publications which put the single results in a nutshell by drawing some general conclusions for example on the benefits and drawback of this new media landscape (Rosenthal, 2020), on the danger of an erosion of the epistemic order of society (Neuberger and Jarren, 2017) or they discuss the impact of online videos on the transformation of science communication and the image of science and scientists (Bourk and León, 2020, p. 117–123). Particularly the publication of the international research project “Videonline” (León and Bourk, 2020) summarizes research results from different countries, giving an overview of investigations on several relevant aspects of online science videos including a classification of online science videos (Davis and León, 2020; García-Avilés and de Lara, 2020) or a discussion of criteria for the epistemic qualities of online videos (Francés and Peris, 2020). Despite the broad spectrum of issues, this comprehensive publication does not contain any empirical results on the reception of science videos and knowledge transfer.
Based on an analysis of English-language videos on 39 YouTube channels Welbourne and Grant (2016) examine which factors contribute to the distribution and reputation of science videos and channels. Differentiating between professional content from commercial media organizations and content published by amateurs (User-generated content), they conclude, consistent with other studies (de Lara et al., 2017; Davis and León, 2020), that amateurs' channels generate more views and are subscribed to more often. The study explains this success by the fact that amateurs often act as communicators themselves, presenting their content creatively and authentically, and in an informative and entertaining way (Welbourne and Grant, 2016, p. 707). As Morcillo et al. (2016) noted in their study of 190 popular science YouTube videos, a professionalization of user-generated content has already taken place. They analyzed the videos in terms of narrative structure, video editing, settings, montage, sound, special effects, etc. and find a high variation in genres and sub-genres, a high degree of complexity in montage and narration and a high expertise in storytelling (Morcillo et al., 2016, p. 22).
An interesting object of comparison for our study is the very detailed classification of online videos proposed by de Lara et al. (2017), which consists of 18 video formats divided into a group of television formats and a group of web formats. The classification is based on a sample of about 300 videos addressing the issue of climate change, which had been processed by a google search. Therefore, this classification differs from the typology proposed in this article in several respects, making it even more difficult to compare them. One reason for the differences stems from the diversity of the samples. Our sample of about 400 videos is, in some way, manifolded as it is not subjected to a special issue of science. In another way the sample is more restricted as it only contains videos in German language disseminated on YouTube channels. A second reason for the differences between the two classifications comes from our typology's theoretical foundation which is based on and legitimated by a general theory of multimodal discourse. Hence the classification criteria are inferred on the one hand from theoretical concepts of multimodality and on the other hand bottom-up from discriminating features of the videos contained in the sample (see chapter 3.1).
A systematic analysis of German web videos on science is still pending. Hence a classification of YouTube videos is the starting point of the presented project. Based on a systematic categorization of science videos, the project intends to investigate the connections between the video types and their typical features like modal orchestration on the one hand and the reception process and knowledge transfer on the other.
A systematic analysis of the scientific content uploaded to YouTube proves difficult: An exact quantification of existing channels on scientific issues is almost impossible because the platform is continuously changing (channels are deleted, new ones are added), and YouTube's algorithms for categorization and recommendation are being adapted. This was compensated by sampling the videos on different devices, in private mode and with empty cache. Another problem of the YouTube platform is that it is not a “curated moving image archive” (Allgaier, 2016). Therefore, users cannot be sure whether what they find on YouTube is scientifically authorized information. YouTube's algorithm prompts users to watch quite different videos depending on their past behavior. It also depends on how many likes a video has received. A highly rated video is more likely to be displayed on a user's home page than a video with no likes and few views. By systemizing vaccination videos, Allgaier (2016, p. 21) found that most of the information contradicts the scientific consensus and that those videos deviating from established medical knowledge also receive the most likes from users.
According to the complex and multilevel research design the study refers methodologically to a broad spectrum of theories and approaches: besides eye tracking methodology (Holmqvist et al., 2011) theories of multimodal discourse are the background of a classification of YouTube videos (see Bucher, 2017), theories of attention and knowledge transfer are used to interpret eye tracking data (Neumann, 1996; Bucher and Schumacher, 2006; Wolfe, 2015; Fairweather and Montemayor, 2017) and conversation analysis is applied for analyzing the user comments (Hutchby and Wooffitt, 2008; Herring, 2010; Clayman and Gill, 2012). For comparing the concept maps with regard to their epistemic value they are defined as cognitive networks—consisting of concepts as knots and relations as edges (Schnotz and Rasch, 2005; Schnotz, 2014)—and analyzed with tools and methods of network analysis (Wasserman and Faust, 1994; Scott, 2000). A key factor in knowledge transfer is the recipient' attention to a stimulus—a video, a text, a graphic (Fairweather and Montemayor, 2017, p. 27 ff.). Besides its double function to select the relevant aspects of a stimulus and to combine the selected elements in the process of meaning making (Neumann, 1996; Wolfe, 2015) attention is similarly intentional and unintentional: it can be bottom-up stimulus-driven or top-down recipient-driven when it is “paid” to particular cues of a stimulus (Bucher and Schumacher, 2006). According to an interactional paradigm of media reception, both directions of attention are justified (Duchowski, 2003, p. 12–14) why the architecture of the study comprises both: a systematic analysis of the stimuli—the Typology of YouTube videos—and the tracking of the reception process via eye tracking and knowledge tests.
Toward a Typology of Science Videos
Materials and Methods
Since a comprehensive analysis of German science videos is missing as well as a typology of films and providers, several hundreds of videos were examined, and a corpus of 400 videos was compiled. In the social sciences, typologies, and classification are regarded as fundamental tools of empirical research and as an intermediate between qualitative and quantitative approaches (see Lazarsfeld, 1937). The criterion for selecting a video was that it has to deal with information coming from scientific research and/or focus on the research's methodological process. Videos by scientific institutions (universities, research institutes, etc.), and non-expert persons are part of the study. So-called instructional videos or tutoring videos, which present school and university teaching material, were excluded, as they aim to impart general knowledge rather than scientific—information. In addition, filmed lectures and talks (e.g., TED Talks) were not included, as these are not formats that were produced specifically for publication on YouTube. Although there is not a clear-cut distinction in any case, the videos in the corpus have to exhibit a kind of news value and journalistic features, whereas educational videos normally contain already well-known information. In contrast to ad-hoc typologies based on a bottom-up study of a special number of videos (for example: de Lara et al., 2017; García-Avilés and de Lara, 2020) or overall features of communication (Rosenthal, 2020), our approach is rooted in a theory of multimodal discourse (Bateman and Schmidt, 2012; Bateman, 2014; Bucher, 2017). YouTube science videos are seen as well-organized multimodal arrangements consisting of a variety of visual and verbal modes like stills, moving images, text, spoken language, sounds, animations, graphics, etc. which is a much more complex system of communication than text only (for basic information see: Kress, 2012). This theoretical background makes sure that the categories of a typology are well-founded and systematically interconnected. The most basic categories like “main function” (Description, Argumentation, Explanation, Portrayal etc.), “functional elements” (Amination, Off-comment, Interview, experiment etc.), “form of presentation” (Intro, Outro, Inserts, Fast motion, Slow motion), “intermodal relations” (Visualization, Illustration, Accentuation, Foregrounding etc.), and “modal orchestration” (text-image-relation, sound-image-relation, image-image-relation etc.) are rooted in a functional theory of communication which looks at multimodal discourse as a form of complex communicative action and mutual coordination (Bateman, 2014; Bucher, 2017). This multi-layered system of categories allows a complex classification of online science videos in which the criteria are hierarchically organized und mutually discriminating. Applying this conceptual apparatus to a systematically compiled corpus leads to a typology of four basic genres of science videos each of which is assumed to trigger special reception patterns and in particular patterns of knowledge transfer. It is the basic idea behind this study's architecture to discover the regular relations between the features of the science videos and the reception process mirrored in gaze distribution, attention allocation and knowledge transfer. Genres or formats considered as “the cornerstones of the media logics” (García-Avilés and de Lara, 2020, p. 26) play a double role in media communication: they are an orientation in media production if it comes to accomplish communicative intentions and adaptability to the audience. And they are also orientations for the addressee as they trigger their expectations and organize the reception process. In so far, the classification of science videos in four different genres is a precondition for analyzing the reception process: the unique features of the formats and the differences between them can serve as basic factors for explaining differences in informational selection, gaze distribution, attention allocation, and knowledge transfer. Formats or genres of science videos must be considered idealized prototypes that often appear as hybrids or mixtures of audio-visual elements from different formats. But the experience from analyzing about 400 of science videos teaches that it is possible to assign each video to a special genre by grading the different categories of which the functional ones are the most important.
For collecting the videos, a kind of snowball sampling was applied: the footage of a prominent YouTube channel served as the starting point for searching for other channels. Furthermore, German keywords such as “Wissenschaft” (“science”), “Forschung” (“research”), or “Sozialwissenschaft” (“social sciences”) were used as search terms on the YouTube platform. Besides the keyword-based retrieval, videos were collected that were recommended on the editorially supported platform SciViews and the Fast Forward Science Competition's homepage—a competition for science videos. SciViews editors select web videos that they consider “journalistically, content-wise or aesthetically valuable [,] worth seeing or simply entertaining” and review them. The web video competition Fast Forward Science invites students, researchers, interested laypeople, and science communicators to submit their video productions as part of a competition. In addition, videos from media companies (e.g., from the channel Terra X Lesch & Co or content by funk) produced specifically for publication on YouTube were also considered to cover the area of professionally generated content. The corpus also includes videos on the bases of the participatory recommendation system of YouTube (cross-promotion). Methodologically, the study follows the principles of theoretical sampling as developed within the framework of grounded theory (Glaser and Strauss, 1998). During the encoding process, the data were continuously compared (“constant comparative method”) to work out differences and similarities between the videos. This constant comparison leads to the generation of theoretical properties and categories (Glaser and Strauss, 1998, p. 112). The categories are defined based on a comparative analysis of the phenomena occurring, in this case, the videos. The characteristics of the different videos were worked out using multimodal discourse analysis and classified according to criteria of multimodal orchestration (Bucher, 2017): how many modes are deployed to compose audiovisual scientific content and what is the primary function of the multimodal orchestration. On the baseline of this theoretical approach, detailed coding of the 400 science videos was conducted in turn to improve the coding criteria (see Glaser and Strauss, 1998) which are:
• General information: title, channel name, number of subscribers, views, number of comments, length of the video
• Communicator information: expert, layperson, institution
• Actors appearing in the video: scientist, journalist, interview-partner
• Modes applied in the video: moving image, spoken language, written text, photos, charts, music, animation, etc.
• The primary function of the video: informing, explaining, portraying, narrating, demonstrating, entertaining, etc.
• Sub-functions of single video sequences: illustrating, arguing, visualizing, labeling, asserting, etc.
• Film design: intro, outro, cuts, montage elements, experiments, laboratory images
• Topics and scientific disciplines.
Based on the categorization of 400 videos in the corpus, a typology for science videos was developed according to data-related (inductive) and theory-based (deductive) categories. The sampling was continued until no video appeared which could not be classified. Therefor the sample is complete, and the classification saturated in the sense of a Grounded Theory (Saunders et al., 2018). The typology of video genres serves two purposes: firstly, the typology should show how audiovisual formats have developed under digitization conditions. Secondly, the survey is the basis on which the science videos are selected for the reception study investigating knowledge transfer (see chapter 4).
Results: A Typology of Science Videos
Four basic types of science videos were identified based on the multimodal analysis of the videos. Two of the genres, the expert film, and the narrative explanatory film, are classical TV genres from science programs; the other two, the presentation film and the animation film, are typical YouTube genres that also appear on channels with different topics. Most of the videos in the entire corpus were professionally produced. Many apply different editing techniques and have their own intro and outro sequences and their channel logos. They are multimodal compositions, most of which contain spoken language, moving images, visualizations and elements of digital editing and design. The analyzed videos are, on average, about 5 min long. Most of the videos (214) were not produced by actively researching or teaching scientists or scientific institutions. In the following, the four different genres of science videos are briefly characterized.
Presentation Film
The classical lecture and the scientific lecture are precursor formats of this type. The lecturer/communicator is often seen in a medium closeup shot (talking head), talking directly to the camera and addressing the audience. The presentation can also take place in dialogue with two presenters. The presentation film focuses on a somewhat restricted scope of an issue and intends to answer a limited number of scientific questions. Spoken language represents the leading mode, but other modes can also be integrated, enriching the visual channel simultaneously or sequentially like, for example, text over visuals, background images, animations, or demonstrations. The presenters report on topics in which they are personally interested or which the presenters believe to be interesting and relevant for the users. The detailed analysis shows that videos of this type contain a high proportion of conspicuous or meaningful gestures and facial expressions that are applied for referring to visual features of the video, thus managing the coherence between spoken and exhibited information. The most frequently occurring actors are YouTubers, which use platform-specific actions such as asking the viewers to subscribe to their channel or leave a comment.
Expert Film
This category is characterized by the fact that the focus lies on a person—for example, a researcher—who is supposed to be portrayed in the video as an expert using the portrayed person as a kind of hook for introducing a topical field of research. Depending on the intention of the video, the focus can be more or less on the expert's person. Thus, the video can be more of a portrait or more of a research report. Expert films usually have a narrative structure: the person is characterized, her or his development is reported, and special features of the biography are narrated, which is why expert films are highly personalized. Often videos of this type are PR videos of scientific institutions. A more detailed analysis of multimodal orchestration has shown that these videos contain a high proportion of moving image material and hardly any platform-specific presentation modes (animations, insertion of user comments, addressing users, etc.). In comparison with the other types, expert films most often present scientists and research activities.
Animation Films
Animation films are characterized by the fact that—usually computer-generated—artificial moving images are shown to visually illustrate a process, a problem, an issue, or a scientific theory. The spoken language can generally be heard from off-screen synchronized with the—in many cases—dynamic visualizations. If the moving images are not computer-based, they are often live drawings and writings or whiteboard videos (illustrations on a white background), which can also be understood as animated films. Animation films make use of an above-average number of text insertions. The users are also addressed directly more often than average.
Narrative Explanatory Films
Narrative explanatory films are based on a general question that is answered in the video. They are more complex than the other three types and often contain elements that characterize the other types: Thus, they consist of functional units such as moderation, expert interviews, laboratory images, computer animations, etc. Narrative explanatory films often are structured like logical reasoning: they provide arguments about why something exists or is supposed to exist or comes to exist. They also combine narrative and informative elements by telling an entertaining story and, at the same time, give an explanation and transfer knowledge. Narrative explanatory films also use mainly moving image material. They have the highest number of cuts from the four types. The coding of the videos shows that here, too, scientists and experts frequently appear as actors. In terms of content, narrative explanatory films are the most heterogeneous group. Like the expert film, the narrative explanatory film is originally a television science programs' format whose production is rather expensive and requires an elaborate technical infrastructure.
Among the 400 sampled videos, the presentation film type is the most common (140 times), followed by the narrative explanatory film (114 times), the animated film (92 times), and the expert film (54 times). According to their background, most of the channel operators or producers of animated films or presentation films are non-scientist laypersons, so-called YouTubers. Scientific institutions are responsible for all expert films and most explanatory films (about 75%). In general, YouTubers and research institutions each account for about 30% of all videos recorded, followed by media companies (16%), YouTubers active in multi-channel networks (10%), and universities (9%). Videos by research foundations account for a share of 6%. Especially Videos from media companies are professionally generated content produced particularly for publication on the corresponding YouTube channels: Examples of this are videos produced by the funk network (ARD and ZDF) or the Terra X Lesch & Co-channel (ZDF in cooperation with objektiv media).
The number of views a video generates is a measure of how successful it is. Since some of the corpus videos have been online for several years and others were only published shortly after being included in the corpus, the average number of views per day was chosen as a comparative measure. Most views—between 3,000 and around 6,000 per day—are generated by presentation films followed by animated films. Expert films are viewed on average only 60 times per day. Narrative explanatory films receive an average of over 1,000 views. Accordingly, the most significant reach on YouTube is achieved by videos produced by laypeople (see Figure 1).
However, the views of the individual videos can vary greatly: The most popular videos in the corpus reached view numbers of over 1 million at the time of writing. Less popular videos were viewed <50 times, even if they were uploaded a long time ago. Among the 50 most popular YouTube videos in the corpus (measured by view numbers) are no scientific institutions' productions. The videos of channels such as 100SekundenPhysik, MaiLab (formerly Schönschlau), or Terra X Lesch & Co. often reach more than 500,000 hits a few weeks after publication.
In addition to the channel operators' background, it was also investigated which actors appear in the videos. Especially in the group of YouTubers, channel operators and actors are usually identical. They are the most frequent actors in presentation films and animated films (as far as persons appear in it). In narrative explanatory films and expert films, most of the people appearing are scientists (see Figure 2) and are not responsible for the channel's content.
Thus, actors without a scientific background are most often found in science video types that generate the most views. These results also show that YouTubers, i.e., those actors who do not belong to any scientific institution, dominate science communication on YouTube.
One of the reasons why non-scientific YouTubers are among the most successful producers of science videos might be that they use all resources for promotion which are typical for YouTube: They explicitly address their audience, apply typical styles of audiovisual online pieces, and interact with their viewers para-socially in their videos and the comment section. They get in touch with the community, invite their viewers to make topic suggestions for future videos, ask them to subscribe to the channel and respond to their addressee's reactions. Often, they also react to comments on their videos and thus appear more approachable than, for example, actors appearing in videos of scientific institutions. Besides presentation films, animation films are the most successful in terms of generating views. These usually shorter formats can be clearly distinguished from traditional science formats on television: they present content creatively with the help of their own illustrations and animations. They often deal with concise questions or abstract phenomena (black holes, dark matter, déjà-vu experiences) and seem to convey these more vividly or attractively.
The Reception of Science Videos
Based on the typology from the first step of the project, 18 YouTube videos were selected for the reception study. Nine videos which were originally produced for YouTube dissemination only and nine television pieces originally produced for German TV science programs and later distributed online. The television reports were selected as comparison objects, which fulfill the following criteria: They have to either cover the same topic as one of the YouTube videos and/or correspond in their multimodal composition (e.g., a presenter conveys knowledge, an animated film is used, a topic is discussed with the help of an expert) to one of the four identified types of online videos. Then, video pairs were formed so that either two different types of video deal with the same topic for type comparison or two videos of the same type deal with varying issues for the topic comparison.
A Mixed-Method Approach
When it comes to knowledge transfer, it is common sense in audience studies that there are close interrelations between the concepts of attention, selection, and knowledge acquisition (Bucher and Schumacher, 2006). For analyzing these interrelations, the study applied a multi-level approach consisting of four different methods:
• an eye-tracking study to investigate the distribution of attention,
• a guided interview to evaluate attitudes and opinions toward the science videos
• an unsupported knowledge test (concept mapping) to examine the acquisition of structural knowledge
• a questionnaire with a multiple-choice test (recognition test) to assess the acquisition of factual knowledge.
Each of the 108 test persons watched three to four videos (depending on its length) covering different types of videos (based on the typology), including at least one YouTube video and one television report. The videos were selected depending on their length, so that the test persons did not have to take part in an eye tracking experiment that was longer than 20 min. Furthermore, the video selection was based on the goal to investigate knowledge transfer, therefore, in order to avoid confusion about which video the knowledge was derived from, only videos dealing with different topics were shown to the participants. The videos were distributed among the participants in such a way that usable gaze data was collected from at least 15 test persons per video. Before showing the videos, some of the test persons (52 of the total of 108) created a concept map on a topic to ascertain previous structural knowledge. After the gaze recording and after seeing the video, the test persons made a second concept map to record stimulus-driven learning effects (for an overview over all methods and number of participants, see Picture 1). All participants had to fill out a questionnaire with multiple-choice questions and questions concerning their media usage and sociodemographic. After the videos' reception, they were interviewed to evaluate their attitudes and opinions toward the stimuli. The 108 participants in the laboratory study were, on average, around 36 years of age and evenly distributed among the age groups, with both sexes also represented approximately equally. Measured by the highest level of general education, the participants have an above-average level of education. When recruiting the test persons, care was taken to ensure that people with different socio-demographic backgrounds were chosen. One goal was to interview not only students or people from the university environment. Regarding the question whether the test persons deal with science topics privately and/or professionally, 26 stated that they neither privately nor professionally engage with science topics. Sixty-eight percent of the participants have used YouTube as a source of science related information in the past. They belong to the younger test persons (on average they are 31 years old). Those who have never used YouTube are on average 39 years old.
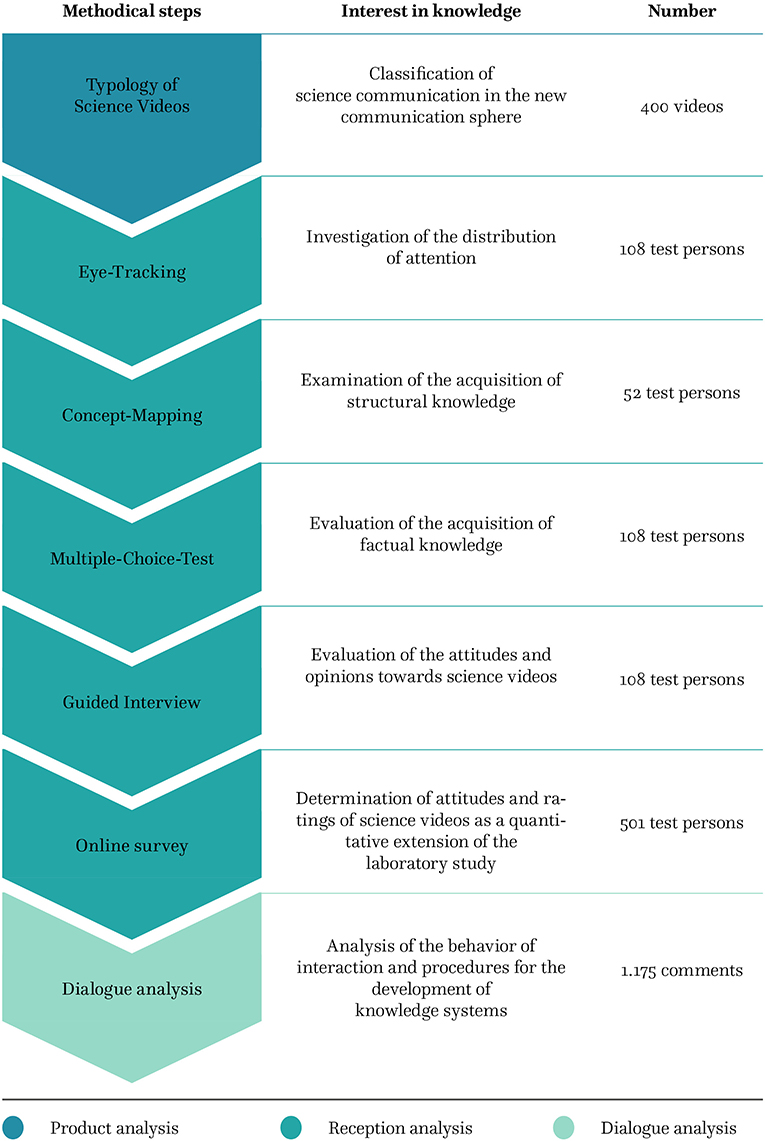
PICTURE 1. Structure of the study, the aims of the steps, and the number of participants within the parts of the study.
Since reception studies are very resource-intensive and therefore generate fewer case numbers, the laboratory study was accompanied by an online survey conducted in cooperation with the publishing house Spektrum der Wissenschaft. More than 700 people took part in the online survey, of which 501 completed questionnaires could be evaluated. The questionnaire was designed to support the laboratory study quantitatively, which is why a selection of eight of the 18 videos used in the reception study was included in the online questionnaires. Accordingly, the assessments of the epistemic quality of the different types of audiovisual science videos from the reception study could be compared with those from the online study. Since the online study also assessed knowledge transfer by multiple-choice tests, this aspect could also be evaluated comparatively. In addition to questions on sociodemographic, media usage, the relationship to science, and science communication, the participants were also asked to assess how vital entertainment, sympathy toward people appearing, the status of the actors (scientists or laypersons), and professionalism (in terms of style and actors) are to them. In terms of a control study the results of the online survey are included in the evaluation of the reception study.
Tracking Gaze Guidance and the Allocation of Attention
By applying the concept of attention to the transfer of knowledge through audiovisual stimuli, the question arises whether and how these stimuli succeed in guiding the recipients' attention to select and integrate the relevant elements appropriately. Based on the so-called eye-mind hypothesis— “the eye fixates the referent of the symbol being operated on” (Just and Carpenter, 1976, p. 441)—tracking eye movements opens a window to the mental reception process. Hence, gaze data are indicators for the allocation of attention, the evaluation of which can accordingly provide information about these selection and integration processes. Their analysis allows us to reconstruct how efficiently the “gaze guidance” (Hooge and Camps, 2013) of an audiovisual stimulus succeeds and how precisely the recipients are informed about the relevant visual aspects (see Gould, 1973; Goldberg and Helfman, 2013). Hence, comparing the eye-tracking data of different recipients allows us to determine the quality of gaze guidance of a video: “Is the scan path across AOIs directed or randomly distributed?” (Holmqvist et al., 2011, p. 341). A prerequisite for the systematic evaluation of gaze data is the definition of so-called “Areas of Interest” (AOIs), i.e., visual sections of a stimulus that contain the relevant information. With the help of these AOIs, scan paths—i.e., processes of attention distribution—can be disclosed.
Gaze data can be evaluated with two different methods that use various measures: a fixation-related evaluation according to criteria such as duration, frequency, localization, sequence, or so-called revisits of AOIs provides information about which elements (AOIs) were viewed for how long, how often, and when. A process-related evaluation, based on measures of scan paths such as their length, similarity, predictability, etc., provides information about the sequence of AOIs considered, the dynamics, and the course of the reception. The more homogeneous the recipients' gaze patterns are, the stronger is the gaze guidance of a video and the higher the probability that the recipients have caught the relevant information (Hooge and Camps, 2013; Gwizdka, 2014). In our study, the degree of homogeneity of gaze patterns was calculated employing three measures: the fixation-based criteria “dwell time” and the process related measures of matrix density and matrix entropy (Krejtz et al., 2014). The length of dwell time for an AOI indicates the intensity of reception while entropy and matrix density suggest the homogeneity of scan paths (Holmqvist et al., 2011, Chapter 10.7; 11.4; Chen and Shi, 2019).
Eye movements are detailed data for reception research because they serve as unintentional indicators for cognitive processes and provide data beyond self-reporting methods such as interviews or written surveys. Compared to data from knowledge tests, eye-tracking data have the advantage that they can be causally related to the stimulus and its characteristics. They are, therefore, the link between the reception data and the stimulus characteristics that triggered them. The present study's research design, consisting of stimulus-related approaches and stimulus-independent approaches to empirical investigations, opens the possibility of explaining reception data with specific characteristics of the science videos.
Measuring Knowledge Transfer
Two types of knowledge tests are used in the study, each of which can capture different forms of knowledge: Multiple-choice tests suitable for capturing factual knowledge (knowing that) and concept mapping, which can capture structural knowledge (knowing how and why). The concept mapping method is based on the assumption that cognitive models are organized as networks of propositions as their smallest unit, which consist of concepts and relations connecting them (see Baker et al., 1991; Ruiz-Primo, 2004). Therefore, concept maps consist of two basic elements: Concepts, which are the knots of the cognitive net, and relations like “is part of,” “causes,” “leads to” which form the edges of the net (see Novak and Gowin, 1984; Gehl, 2012). The test persons created a concept map at the beginning of the test and after having seen the video to capture the process of knowledge acquisition. The two test procedures—multiple-choice test and concept mapping—differ not only concerning the type of knowledge measured, but also the quality of the cognitive processing (Kintsch, 1968; Humphreys and Bain, 1983): The multiple-choice test belongs to the group of so-called recognition tests, in which knowledge acquired after the presentation of stimuli is reactivated or recognized. The so-called recall tests (memory tests), to which concept mapping belongs, require the test subjects to apply existing or acquired knowledge and transfer it to the test situation. Accordingly, the two test types differ in the cognitive performance necessary: “recall involves search and decision stages, while recognition involves only a decision process” (Maisto et al., 1977, p. 127). This additional search or retrieval process consists of finding the appropriate terms and the relations connecting them for an explanatory task in concept mapping (Gehl, 2012).
For comparing and evaluating the concept maps, measures from network analysis like centrality, density, or centralization were deployed (Clariana et al., 2013). Furthermore, the maps were categorized based on some assessment tools for knowledge diagnosis (Novak and Gowin, 1984). This process makes it possible to compare the concept maps according to quantitative criteria like the number of included propositions, and according to qualitative structural criteria like hierarchy or density and coherence (Freeman, 1978; Hennig et al., 2012). The test persons' concept maps were compared with each other as well as with experts' concept maps that represent all knowledge that could have potentially been acquired (see Dogusoy-Taylan and Cagiltay, 2014).
Results Concerning Knowledge Transfer, Gaze Guidance, and Attention
One of the central results of the project is that gaze guidance by the videos, the recipients' allocation of attention and the results of knowledge testing are closely intertwined. The correlation of data from eye-tracking and the two knowledge tests prove in principle that the more homogeneous the gaze patterns of the recipients are, the better they score in both knowledge tests: in the multiple-choice test as well as in the concept mapping test. To measure how successful the individual videos are in teaching factual knowledge, a multiple-choice test was conducted in both the lab study and the online survey. In both surveys, expert's solutions are the benchmarks for assessing the achievements of the test persons. In the multiple-choice test the number of correct answers is the evaluation criteria. According to the complexity of structural knowledge, which was investigated by concept mapping, the study applied a whole set of evaluation criteria which are derived from network analysis (Wasserman and Faust, 1994; Scott, 2000) and knowledge diagnostics measures (Novak and Gowin, 1984):
• Correct propositions
• Applied terminological concepts
• Centralization and density of the conceptual networks
• Hierarchy of the conceptual networks.
Results of Multiple-Choice Tests
There are apparent differences in the average number of points achieved in the multiple-choice tests regarding the different video types (both in the lab study and the online survey): The test persons in the online survey remember more factual knowledge correctly after the reception of animated films (M = 78.45; SD = 28.5)1 and narrative explanatory films (M = 76.64; SD = 23.49), while an ANOVA with pairwise post-hoc tests says that the expert film (M = 64.37; SD = 20.89) scores significantly worse (p < 0.001)2 than both, but not significantly worse than the presentation film (p = 0.243; M = 70.63; SD = 29.12). It makes hardly any difference whether videos are YouTube or television formats, in terms of remembered factual knowledge: After the reception of YouTube science videos in the laboratory, the test persons score an average of around 65% of the maximum, while the television science videos scored about 69%. The online survey results confirm the findings that there is no significant difference between the media: For YouTube videos, an average of 72% of the maximum score was achieved (SD = 26.36), and for television videos, an average of 74% of the maximum score (SD = 26.03; t(499) = −1.19, p = 0.235; see Table 1)3.
However, the acquisition of factual knowledge depends on the topics of the videos: issues that require expert knowledge differ from those that can be understood with everyday knowledge. While science videos on issues such as dark matter, black holes or STED-microscopy (stimulated emission depletion microscopy) presuppose knowledge in physics and chemistry, topics such as tap water, vaccination and the psychological problem of borderline syndrome address the subjects' everyday knowledge and experience. Science videos presupposing expert knowledge achieve, on average, only about 59% of the maximum score, whereas videos conveying everyday knowledge achieve about 73%. In the online survey, the test persons confirm that videos addressing everyday knowledge are significantly easier to understand (M = 4.55, SD = 0.72) than videos containing expert information (M = 3.92, SD = 0.96; t(432) = −7.8, p < 0.001).
The results of the online survey also indicate a significant correlation (r = 0.2, p < 0.001)4 between the relevance attributed to a video topic and the remembered factual knowledge: the more relevant the topic was rated, the better the factual questions were answered. It is noticeable that videos that primarily address everyday knowledge are considered more relevant than those that convey expert knowledge. Additionally, the online survey results document that the level of entertainment ascribed to a video is related to the score of the remembered factual knowledge (r = 0.137, p = 0.002). Moreover, the more entertaining a video is rated, the stronger the belief that the content presented is correct (r = 0.308, p < 0.001). The different types of actors appearing in the videos also influence the acquisition of factual knowledge: If journalists appear in videos (M = 82.31, SD = 21.67), the test persons remember facts significantly better than if YouTubers (M = 66.3, SD = 27.91, p < 0.001), or scientists appear (M = 64.37, SD = 20.89, p < 0.001). However, journalists and videos without actors (e.g., animated films; M = 78.54, SD = 28.5, p = 0.634) do not differ significantly, which means that both perform equally well in conveying factual knowledge. Scientists and YouTubers do not differ significantly either, which suggests that the scientific qualification of the persons appearing has no direct influence on the remembered factual knowledge, as well as aspects of personalization such as the sympathy and competence attributed to the actors involved.
The trustworthiness that the participants ascribe to the actors and familiarity with the YouTube channel or the TV program do not have a statistically significant impact on the multiple-choice test results [t(443) = −1.78, p = 0.076]. But the mean value of remembered factual knowledge rises linearly with increasing trustworthiness ascribed to the actors. Although the aforementioned personalization aspects do not influence the acquisition of factual knowledge, they affect the subjectively perceived increase of knowledge: High sympathy values attributed to the actors are accompanied by a higher perceived learning effect (r = 0.201, p < 0.001) and a higher evaluation of the comprehensibility of the explanations (r = 0.208, p < 0.001). This correlation comes up to what was coined an “illusion of understanding” (Paik and Schraw, 2013) in case of enriching learning material with animation: “Animation can keep learners from doing relevant cognitive processing, not because of increased task difficulty, but because of inappropriate facilitation of the task” (Schnotz and Rasch, 2005, p. 57).
As part of the online survey, the test persons were asked how certain they were that the scientific facts were presented correctly in the video. If one considers this question in connection with the correct factual knowledge, a slightly significant correlation becomes apparent (r = 0.124, p = 0.005.): those who were not at all sure or less sure that the facts presented were correct (n = 109) only answer about 69% of the questions correctly on average. Those who were more or less sure of the facts (n = 392) answer about 74% of the questions correctly. Accordingly, certainty about the correctness of presented facts is a significant predictor (b = 4.28, t = 2.8, p = 0.005)5 of remembered factual knowledge: it explains a significant share of 1.5% of the variance of incorrectly remembered facts [F(1,499) = 7.81, p = 0.005]6.
The findings show that the videos' epistemic reputation, the relevance of its topic, and, with reservations, some aspects of personalization of the videos' content have a distinct effect on knowledge transfer. The online survey results show that there is no significant difference in the correct answers to the factual questions regarding the sociodemographic variables age, gender, and educational level.
Results of the Concept Mapping
Compared with the findings of the multiple-choice testing of factual knowledge, the concept mapping data show a significantly less successful transfer of structural knowledge. Whereas, in the multiple-choice test, on average, about two-thirds of the correct answers are given for all videos, in the concept mapping, the test subjects achieve only about a quarter of the possible propositions (in comparison to the experts' maps). When applying the network measures for the quality of the concept maps, the test subjects remain below the limit of 40% of the expert score for all video types. Hence science videos are much better at conveying factual knowledge than structural knowledge. When comparing the video types, the narrative explanatory films prove most successful in conveying structural knowledge as measured by experts' concept maps. On average, these videos score 26.6% of the maximum number of propositions, whereas they achieve 77% of the possible correct answers in the multiple-choice tests (see Table 1). The animation video reached the highest absolute scores in the concept mapping, but only about 40% of the maximum number of points according to the network measures. The presentation film and the expert film scored worst in both knowledge tests.
As the test persons were asked to compile a concept map before and after having watched the videos, it was possible to identify the influence of prior knowledge on knowledge acquisition. In general, videos that deal with topics on which subjects have little previous knowledge achieve significantly worse results than videos that address pre-known everyday knowledge. All video types are not remarkably successful in teaching the recipients to apply terminological concepts. Less than half of the concepts which are introduced during the video are integrated into the concept maps after watching it. The measure “centralization” from network analytics determines the connectivity and coherence of a concept map: the more centralized it is, the less connectable it is to other cognitive structures. The most centralized maps were compiled by test persons watching presentation films. In contrast, the narrative explanatory films achieve the lowest centralization and the highest increase in the number of acquired propositions and the conceptual networks' density. Overall, the evaluation of the subjects' concept maps shows that there are deficits in transferring structural knowledge what go beyond all video types.
Gaze Guidance and Attention
One of the central questions of this study has been which features of a science video are responsible for allocating attention to the relevant audiovisual aspects. As mentioned above, eye movements serve as indicators for cognitive processes and provide data beyond self-reporting methods. To investigate the gaze guidance potentials of a video, three measures of eye-tracking data were applied: first, the dwell time on relevant areas of interest, which indicates the intensity of reception, matrix density and matrix entropy of eye-tracking data, which both indicate the homogeneity of scan paths and, therefore, the dynamics of reception. The study's data shows that longer dwell time on certain AOIs of a video is associated with a deeper understanding of the mediated content. To compare the different video types according to reception intensity and reception dynamics, the areas of interest (AOI) were systemized in four different groups, which fit all video types: “main person,” “graphic elements,” “text insertions,” and “additional persons” (see Appendix in Supplementary Material). There is a systematic relation between dwell time distribution to these AOI-types and the particular video type. For example, in animated videos, most dwell time is accounted for the graphic elements, in the other types for the main characters appearing in the videos. However, the dwell time is not always determined by the visible time of the AOIs, but rather by the recipients' allocated attention. This becomes particularly apparent in cases where the proportion of dwell time on a video element is greater than the proportion of visible time of this element. For example, this applies to text overlays and graphic elements, which shows that the recipients assign these elements a high relevance. The dwell time on these two elements also correlates with the knowledge tests' findings: longer dwell times on graphic elements and text insertions result in a better transfer of both structural and factual knowledge.
The analysis of dwell time reveals a dilemma of attention allocation, which is typical for presentation videos (see Wang et al., 2020): In comparison with other video types, all five of them show the worst performance in the knowledge test with concept mapping indicated by the lowest quality of the conceptual networks in terms of density and structure, the lowest increase in correct propositions and the largest distance to the expert maps. This below-average performance can be explained by a specific weakness in attentional guidance, which is expressed in the dwell time data: The simultaneous presence of a speaking person and the relevant visualizations forces the recipients to split the visual reception channel into two sources, which leads to the dilemma of attention and hence to cognitive overload (see Picture 2, in which the heat map with opaque coloring visualizes the intensity of the test persons' attention).
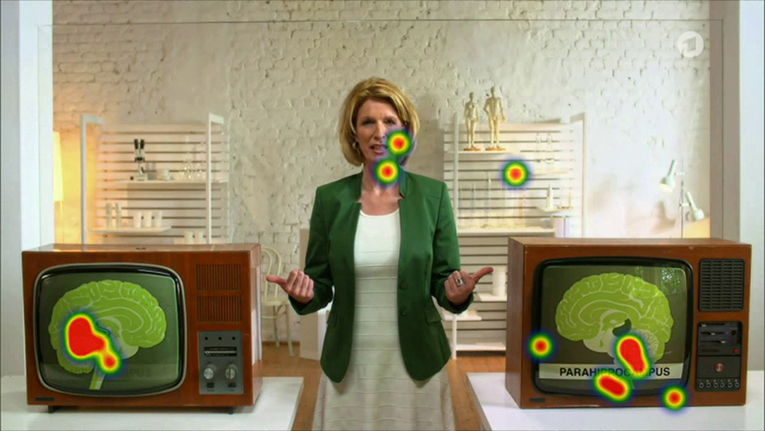
PICTURE 2. Scene from the program “Wissen vor Acht” with the speaker's verbal explanation of déjà-vu-experience and a simultaneous visualization on two TV-screens. The heatmaps of 17 test persons demonstrate the dilemma of attention allocation, which causes a quite heterogenic gaze pattern.
In the other video types, the speaking person, and other relevant parts of a video like text or visuals are organized in dual channels allowing the recipients to acquire the information simultaneously with ears and eyes. Animation videos avoid the mentioned attention dilemma by separating the relevant information into an audio channel—the spoken information of an invisible speaker from the off—and a visual channel containing the elements to gaze at (see Picture 3).
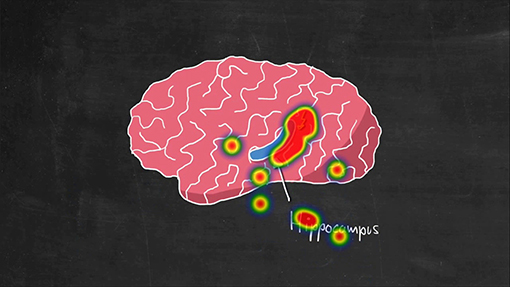
PICTURE 3. Scene from the YouTube-Video “What is a déjà-vu?” (sequential presentation of information). The heat map of 18 test persons visualizes the rather homogenic gaze pattern.
While dwell time indicates the intensity of perception, the entropy values shows the dynamics of receptions according to the scan paths' homogeneity. Scan paths are defined as “a trace of a participant's eye-movements in space and time” (Holmqvist et al., 2011, p. 253). A general result is that the lower the modal density and the higher the modal coherence of the video, the lower the entropy value. This indicates a highly homogeneous scan path and, therefore, strong gaze guidance by the video. Comparisons of different videos verify that in contrast with a simultaneous spatial presentation of informational elements on the screen, a sequential structure of informational phases promotes the acquisition of structural knowledge. The scan paths are then clearly defined so that the recipients do not have to search for the relevant information but can use their limited cognitive resources to process the content of the video sequentially. Precise attentional control helps to reduce the cognitive load. It relieves working memory resources and frees capacities for knowledge acquisition (Paas and Sweller, 2014, p. 38).
Attentional guidance and knowledge transfer are thus established differently for temporally-sequentially structured and simultaneously-spatially structured videos. A simultaneous-spatial arrangement of elements within a video increases the multimodal density. This increased external (“extraneous”) cognitive load demands cognitive resources that are lacking for processing the presented information (Mayer and Fiorella, 2014). In cases of linear stimuli like science videos, simultaneously presented additional information must be received under time pressure. In contrast, the sequential arrangement of additional information fits into the linear structure of those videos in such a way that there is no competition between spatial processing of “phases” and temporal processing of the sequential structure of the video.
Dialogue Analysis: Mapping the Participatory Space of Social Media
In contrast to television, science communication on YouTube is characterized by a participatory and interactive communication model that allows the users to comment on the initial video or comments of other users and enables the communicators to connect with their followers. The comment section turns out to be an integral part of the communication space of social media. Thus, complementary to the reception study, an investigation of this interactional space proves to be a promising, additional approach to elucidate effects and reactions to YouTube videos. The analysis of almost 2,000 user comments from the comment section of six of the examined YouTube videos shows that they are a coherent web of interactions that is composed of by mutual references such as explicit addressing, citations or thematic signals and sequence patterns like question-answer or assertion-contradiction-proof of evidence (see also Bou-Franch et al., 2012).
When it comes to knowledge transfer, these dialogues prove relevant for negotiating the videos' epistemic quality and participatory processing of the initial video's issues. Recent studies conclude that the level of civility of comments could impact users' perception of the initial video (Brossard, 2013). With regard to the assumption that the Internet plays an important role in destabilizing our society's epistemic order (Neuberger and Jarren, 2017), the question of how rational, emotional, or factual the consecutive discourses in the comment sections are is still in the foreground (Bucher and Barth, 2019). Concordant with the assumption of Dubovi and Tabak that “YouTube can offer an informal space for science deliberation” (Dubovi and Tabak, 2020, p. 2), the results of the analysis of the examined YouTube comments do not confirm such skeptical assessments: About half of the comments concern knowledge transfer or can be understood as epistemic evaluations of the video's content or the previous comment. Thematic interactions (ad-rem interaction) dominate the comment section. Ad-hominem interaction patterns based on defamation and abuse of people, which are known from other online communication spaces, occur as less frequently individual cases. The same applies to personalizing and emotionalizing patterns of action, which are less characteristic of scientific controversies but typical of online communication. With regard to the long-term development of science communication, the analyses of user comments may contribute to verify the assumption that a transition from a deficit model of science communication with a passive audience to an interaction model with active participation by the recipients takes place (for detailed results see Christ, 2020).
Discussion
As an addition to the growing amount of research on YouTube science videos, the presented study focuses on the individuals' understanding of science as a precedent of public understanding of science. It combines a typology of YouTube videos based on a multimodal discourse analysis with an audience study for investigating knowledge transfer. Hence the knowledge, acquired by watching YouTube Videos is the dependent variable; the video types are the independent ones. A typology of four audiovisual video genres was developed from the systematic analysis of 400 videos from YouTube. Two of these genres, the narrative explanatory film, and the expert film, are traditional television formats transferred to YouTube channels, especially by science institutions, universities, or media companies. The other two genres, the presentation film and the animation film, are typical YouTube genres that borrow some of their elements from other social media formats. As mentioned in other studies (de Lara et al., 2017) these new genres receive more views and comments than the television-based genres because they take up the platform's specifics and present content creatively and authentically. Their high reputation, broad distribution, and acceptance indicates a change in science communication toward more personal, more authentic, more entertaining genres that apply the full spectrum of digital tools and interactive potentials of social media. In contrast to other classifications (Morcillo et al., 2016; de Lara et al., 2017) our typology features a much smaller number of types which is conditioned both by the different sampling methods and the different classification criteria. A straightforward typology might be detrimental in terms of revealing the diversity of the classified objects, but in the case of our study it is a precondition for asserting reliable relations between the YouTube videos as audiovisual stimuli and their effects like allocated attention or acquired knowledge.
As revealed in other studies (Welbourne and Grant, 2016; Erviti et al., 2020; León and Bourk, 2020) YouTube-based science communication also indicates a transformation in terms of authorship: The platform logic provides distribution frameworks that enable laypersons to outperform science institutions (research institutes, universities) in their reach. As a result, non-scientific actors dominate science communication on YouTube, marginalizing professional authorship by scientists, science institutions, or universities. It remains open, if this leads to a long-term collapse of well-established epistemic orders or a spreading of an anti-science stance (Erviti et al., 2020), despite some optimistic results of this study's investigation of knowledge transfer and user comments. The results of the study indicate that the success of science communication depends on how its authors consider the media logic of the channel they chose.
In terms of knowledge transfer, the different types of videos have a significant impact on the recipients' quality of knowledge acquisition. Factual information conveyed in animated films and narrative explanatory films, for example, is remembered much better than the information from expert films, which are favored by universities and research institutes. However, it must be considered that expert films usually focus on conveying knowledge that has little to do with most subjects' everyday lives and therefore falls within the realm of expert knowledge. In the context of the presented study, the videos that aim to convey special scientific topics, which overlap less with the test persons' everyday life and with their previous knowledge, came off worse. Insofar the data prove a close relationship between the topic of a video and the transfer of knowledge. Together with users' bias to favor information that aligns with their pre-existing knowledge and attitudes, this relation probably favors selective exposure and epistemic filter bubbles (Landrum et al., 2019). In general, the results of the reception study attenuate the optimistic expectations which are traditionally connected with visualization in science communication and with audiovisual pieces particularly. The fact that science videos are much better at conveying factual knowledge than structural knowledge suggest that the desired Public Understanding of Science can only be achieved to a limited extent, because structural knowledge is crucial for the integration of new knowledge into existing knowledge and the integration of new information into larger contexts.
Questions concerning the relation between entertainment and information have a long history in debates about the accessibility and popularization of science communication (Myers, 2003; Shapiro and Park, 2015; Walsh, 2015). On the one hand entertainment strategies like storytelling, comic-formats, colloquial language, personalization, or visualization are assessed as counterparts to rationality and objectivity. On the other hand, they are considered to make science more attractive for an audience of non-experts. The results of our study seem to confirm the latter position, but with a cutback. The level of entertainment ascribed to a video relates to the score of the remembered factual knowledge and to the evaluation of the videos' rigor. The more entertaining a video is rated, the stronger the belief that the content presented is correct and the stronger the trust in the authors. This corresponds closely to an effect, called the “illusion of understanding” (Paik and Schraw, 2013) or “easiness effect” (Scharrer et al., 2016). Simplification—for example via infotainment—prompts the recipients to assess the content easier and more trustable and to overrate their epistemic competence. Hence the results of this study contradict the assumption that “YouTube users dissociate ‘science’ and ‘entertainment”’ (Rosenthal, 2018, p. 34) a result that might be impacted by the sample of the test person who are characterized as information-oriented. In contrast to this sample, our study considers the whole unspecified cohort of about 500 participants. Concordant with results from research on popularization of science formats like science slams and TED-Talks one can conclude that there is always a tension between entertainment and information, but that a certain amount of entertaining elements can foster knowledge acquisition (Lederman, 2016; Carlsson, 2018; Bourk et al., 2020).
Regarding the theoretical assumptions of our approach in regard to reception processes, the eye-tracking data of about one hundred recipients confirm that knowledge transfer is not only impacted by some attributes or dispositions of the recipients, but is also stimulus-driven: the allocation of attention, which is the link between a video and the acquisition of knowledge, is guided by features of the video like its modal density, modal coherence, its temporal and linear structure. In general, it can be said that according to the overall temporal structure of audiovisual material, linear organized phases of informational elements promote knowledge acquisition while simultaneous spatially organized informational elements—like a lecturer presenting visual material (see Bucher and Niemann, 2012 on the reception of PowerPoint presentations)—complicate knowledge acquisition by increasing the cognitive load.
The basic approach of the study was to combine a classification of YouTube videos with a reception study, which allows to correlate attention allocation and knowledge acquisition with video genres and their specific features. Hence it is possible to assess the different YouTube video genres regarding their appropriateness for knowledge transfer and in a wider sense for improving scientific literacy based on criteria from reception theory and cognitive science.
Due to the clear division between spoken commentary or explanatory text and visualizations into two different reception channels—hearing and seeing—animation videos have a multimodal structure in which the modes do not compete for attention but complement each other (dual-channel-assumption, Mayer, 2014, p. 47–49). The clear separation of the reception channels makes it possible to synchronize the off-screen commentary and the visualization in terms of content and time. Thus, the video supports the cognitive processes of selecting relevant information, organizing it into coherent structures, and integrating it into existing knowledge (Mayer, 2014, p. 50–52). From this one can deduct the principle that animated videos are well-suited for conveying complex and abstract facts. The strength of presentation videos lies in their personalization, which can also be used systematically to build audience loyalty by establishing anchor presenters. The opportunity to develop a para-social relationship with the addressees plays a central role in accepting YouTube videos (cf. the findings from the online survey). The study has shown two complementary characteristics for narrative explanatory films: their high-performance concerning knowledge transfer and their strong attentional control. This video format combines two functions that can complement each other in terms of knowledge transfer and attentional control: narration, by which the motivation and interest of the addressees can be gained, and explanation. The multimodal orchestration directs the attention of the addressees to the information-relevant aspects of the topic. Although the expert film impedes the addressees to identify the relevant informational elements and therefore scores low in all knowledge tests, its advantage lies in the combination of portraying a scientist and informing about scientific issues. Two disadvantages counter the advantages of the narrative explanatory film and the expert film: firstly, they are a typical television format whose reach and acceptance on YouTube is limited. And secondly, the production effort is relatively high and thus hardly feasible for YouTubers.
As the focus of this study is directed to knowledge transfer “non-knowledge objectives” (Erviti et al., 2020, p. 39), like influencing dispositions toward science, fostering excitement about science, or building trust in the scientific community had to be neglected. Although the online survey of this study reveals in some way how science videos promote attitudes or emotions toward science, the focus of this study lies on knowledge transfer, which undoubtedly is one of the main functions of science communication—but not the only one.
Data Availability Statement
The raw data supporting the conclusions of this article will be made available by the authors, without undue reservation.
Ethics Statement
Ethical review and approval was not required for the study on human participants in accordance with the local legislation and institutional requirements. The patients/participants provided their written informed consent to participate in this study.
Author Contributions
H-JB: principal investigator. BB: responsible for genre analysis and laboratory research. KC: responsible for the online-study and interaction analysis. The complexity of the approach and the multidimensional research design required a close and mutual cooperation between the BB, H-JB, and KC in all phases of the research. All authors contributed to the article and approved the submitted version.
Funding
This research project was funded by the Klaus Tschira Stiftung.
Conflict of Interest
The authors declare that the research was conducted in the absence of any commercial or financial relationships that could be construed as a potential conflict of interest.
Acknowledgments
This project, of which some results are presented in this article, was funded by the Klaus Tschira Foundation (2017–2020). The project benefitted from cooperation with the Nationales Institut für Wissenschaftskommunikation and the research group Science in Presentations at the Karlsruher Institut für Technologie (KIT). The entropy evaluation of gaze data was supported by Kerstin Wolf of iMotions and Stephan Hill, computer scientist at the University of Koblenz. The online survey was facilitated by the publishing house Spektrum der Wissenschaft.
Supplementary Material
The Supplementary Material for this article can be found online at: https://www.frontiersin.org/articles/10.3389/fcomm.2020.608620/full#supplementary-material
Footnotes
1. ^SD = standard deviation; M = mean.
2. ^p = p-value.
3. ^t = paired, t-test.
4. ^r = Pearson's r, correlation coefficient.
5. ^b = unstandardized regression coefficient.
6. ^F = f-test.
References
Allgaier, J. (2016). Wo Wissenschat auf Populärkultur trifft” in Web Video Wissenschaft – Ohne Bewegtbild läuft nichts mehr im Netz: Wie Wissenschaftsvideos das Publikum erobern, eds T. Körkel and K. Hoppenhaus (Heidelberg: Spektrum der Wissenschaft), 15–24.
Allgaier, J. (2019). Science and environmental communication on youtube: strategically distorted communications in online videos on climate change and climate engineering. Front. Commun. 4:36. doi: 10.3389/fcomm.2019.00036
Baker, E. L., Niemi, D., Novak, J., and Herl, H. (1991). “Hypertext as a strategy for teaching and assessing knowledge representation,” in Paper Presented at NATO Advanced ResearchWorkshop on Instructional Design Models for Computer-Based Learning Environments (Enschede).
Bateman, J. (2014). Text and Image. A Critical Introduction to the Visual/Verbal Divide. London, New York, NY: Routledge. doi: 10.4324/9781315773971
Bateman, J., and Schmidt, K.-H. (2012). Multimodal film analysis: how films mean. New York, NY: Routledge. doi: 10.4324/9780203128220
Bou-Franch, P., Lorenzo-Dus, N., and Blitvich, P. G.-C. (2012). Social interaction in youtube text-based polylogues: a study of coherence. J. Comput. Med. Commun. 17, 501–521. doi: 10.1111/j.1083-6101.2012.01579.x
Bourk, M., and León, B. (2020). “Conclusion: innovation and future challenges,” in Communicating Science and Technology through Online Video. Researching a New Media Phenomenon, eds B. León and M. Bourk (New York, NY; London: Routledge), 117–123.
Bourk, M., León, B., and Davis, L. S. (2020). “Entertainment in science: useful in small doses,” in Communicating Science and Technology through Online Video. Researching a New Media Phenomenon, eds B. León and M. Bourk (New York, NY; London: Routledge), 89–104.
Brossard, D. (2013). New media landscapes and the science information consumer. Proc. Natl. Acad. Sci. U. S. A. 110, 14096–14101. doi: 10.1073/pnas.1212744110
Bucchi, M. (2008). “Of deficits, deviations and dialogues. Theories of public communication of science” in Handbook of Public Communication of Science and Technology, eds M. Bucchi and B. Trench (London: Routledge), 57–76.
Bucher, H.-J. (2017). “Understanding multimodal meaning making: Theories of multimodality in the light of reception studies,” in New Studies in Multimodality. Conceptual and Methodological Elaborations, eds O. Seizov and J. Wildfeuer (London; Oxford; New York, NY; New Delhi; Sydney, NSW: Bloomsbury), 91–123.
Bucher, H.-J. (2020). “The contribution of media studies to the understanding of science communication,” in Handbook of Science Communication, eds A. Leßmöllmann, M. Dascal, and T. Gloning (Boston, MA; Berlin: De Gruyter), 51–76.
Bucher, H.-J., and Barth, C. (2019). “Zwischen Hatespeech und Deliberation: affektive Öffentlichkeiten und politische Kommunikation in den sozialen Medien,” in: Mediale Emotionskulturen, eds S. Hauser, M. Luginbühl, and S. Tienken. (Bern; Berlin; Bruxelles; New York, NY; Oxford: Peter Lang), 57–81. [Between hate speech and deliberation: affective publics and political communication in social media].
Bucher, H.-J., and Boy, B. (2018). “How informative are information comics in science communication? Empirical results from an eye-tracking study and knowledge testing,” in Empirical Comic Research. Digital, Multimodal and Cognitive Methods, eds A. Dunst, J. Laubrock, and J. Wildfeuer (New York, NY, London: Routledge), 176–196.
Bucher, H.-J., and Niemann, P. (2012). Visualizing science: the reception of powerpoint presentations. Visual Commun. 11, 283–306. doi: 10.1177/1470357212446409
Bucher, H.-J., and Schumacher, P. (2006). The relevance of attention for selecting news content. An eye-tracking study on attention patterns in the reception of print- and online media. Communications. Eur. J. Commun. Res. 31, 347–368. doi: 10.1515/COMMUN.2006.022
Carlsson, B. (2018). ‘Science Slam’ and sportification processes in science. Sport Soc. 1–15. doi: 10.1080/17430437.2018.1435030
Chen, Z., and Shi, B. E. (2019). Using variable dwell time to accelerate gaze-based web browsing with two-step selection. Int. J. Hum. Comput. Interact. 35, 240–255. doi: 10.1080/10447318.2018.1452351
Christ, K. (2020). Wissenschaft auf YouTube: mehr als nur Videos. Eine Interaktionsanalyse der Anschlusskommunikation. Gießen: Gießener Elektronische Bibliothek. [Science on Youtube: More than just videos. An interaction analysis of user comments]
Clariana, R. B., Engelmann, T., and Yu, W. (2013). Using centrality of concept maps as a measure of problem space states in computer-supported collaborative problem solving. Educ. Tech. Res. 2013, 423–442. doi: 10.1007/s11423-013-9293-6
Clayman, S. E., and Gill, V. T. (2012). “Conversation analysis,” in The Routledge Handbook of Discourse Analysis, eds J. P. Gee, P. James, and M. Handford (London; New York, NY: Routledge), 120–134.
Crary, J. (1990). Techniques of the Observer: on Vision and Modernity in the Nineteenth Century. Cambridge, MA: Massachusetts Institute of Technology.
Davis, L. S., and León, B. (2020). “New and old narratives: changing narratives of science documentary in the digital environment,” in Communicating Science and Technology through Online Video. Researching a New Media Phenomenon, eds B. León and M. Bourk (New York, NY; London: Routledge), 58–65.
de Lara, A., García-Avilés, J. A., and Revuelta, G. (2017). Online video on climate change: a comparison between television and web formats. J. Sci. Commun. 16:1–32. doi: 10.22323/2.16010204
Dogusoy-Taylan, B., and Cagiltay, K. (2014). Cognitive analysis of experts' and novices' concept mapping processes: an eye tracking study. Comput. Hum. Behav. 36, 82–93. doi: 10.1016/j.chb.2014.03.036
Donzelli, G., Palomba, G., Federigi, I., Aquino, F., Cioni, L., Verani, M., et al. (2018). Misinformation on vaccination: a quantitative analysis of YouTube videos. Hum. Vaccines Immunother. 14, 1654–1659. doi: 10.1080/21645515.2018.1454572
Dubovi, I., and Tabak, I. (2020). An empirical analysis of knowledge co-construction in YouTube comments. Comput. Educ. 156:103939. doi: 10.1016/j.compedu.2020.103939
Erviti, M. C., Codina, M., and León, B. (2020). Pro-science, anti-science and neutral science in online videos on climate change, vaccines and nanotechnology. Media Commun. 8:2. doi: 10.17645/mac.v8i2.2937
Erviti, M. C., and León, B. (2016). “Participatory culture and science communication: a content analysis of popular science on YouTube,” in Nuevas Formas de Expresión, eds C. del Valle Rojas and C. Salgado Santamaría (Madrid: Ediciones Universitarias McGraw-Hill), 271–286.
Erviti, M. C., and Stengler, E. (2016). “Online video as a science communication tool,” in 15th Annual STS Conference (Graz). doi: 10.22323/2.15040602
Fairweather, A., and Montemayor, C. (2017). Knowledge, Dexterity, and Attention. Cambridge: Cambridge University Press. doi: 10.1017/9781316105849
Francés, M., and Peris, À. (2020). “Rigour in online science videos: an initial approach,” in Communicating Science and Technology through Online Video. Researching a New Media Phenomenon, eds B. León and M. Bourk (New York, NY; London: Routledge), 66–76.
Freeman, L. C. (1978). Centrality in social networks conceptual clarification. Soc. Netw. 1, 215–239.
García-Avilés, J. A., and de Lara, A. (2020). “An overview of science online video: designing a classification of formats,” in Communicating Science and Technology through Online Video. Researching a New Media Phenomenon, eds B. León and M. Bourk (New York, NY; London: Routledge), 22–32.
Gehl, D. (2012). “Concept Mapping und Eyetracking. Eine Methodenkombination zur Diagnose medial initiierter Wissenszuwächse,” in Interaktionale Rezeptionsforschung. Theorie und Methode der Blickaufzeichnung in der Medienforschung, eds H.-J. Bucher and P. Schumacher (Wiesbaden: Springer VS), 135–155. [Concept mapping and eye tracking: Diagnosing knowledge transfer by applying method triangulation] doi: 10.1007/978-3-531-93166-1_5
Geipel, A. (2018). “Wissenschaft@YouTube,” in Knowledge in Action: Neue Formen Der Kommunikation in der Wissensgesellschaft, eds E. Lettkemann, R. Wilke and H. Knoblauch (Wiesbaden: Springer VS), 137–163. doi: 10.1007/978-3-658-18337-0_6
Gibbons, M., Limoges, C., Nowotny, H., Schwartzman, S., Scott, P., and Trow, M. (1994). The New Production of Knowledge. The Dynamics of Science and Research in Contemporary Societies. London: Sage.
Glaser, B. G., and Strauss, A. L. (1998). Grounded Theory. Strategien Qualitativer Forschung. Bern: Hans Huber.
Goldberg, J. H., and Helfman, J. I. (2013). “Eye tracking on visualizations: progressive extraction of scanning strategies,” in Handbook of Human Centric Visualization, ed W. Huang (New York, NY: Springer Science+Business Media), 337. doi: 10.1007/978-1-4614-7485-2_13
Gould, J. (1973). Eye movements during visual search and memory search. J. Exp. Psychol. 98, 184–195. doi: 10.1037/h0034280
Gwizdka, J. (2014). “Characterizing relevance with eye-tracking measures,” in Proceedings of the 5th Information Interaction in Context Symposium. Association for Computing Machinery. (Regensburg), 58–67. doi: 10.1145/2637002.2637011
Hallman, W. K. (2017). “What the public thinks and knows about science - and why it matters,” in The Oxford Handbook of the Science of Science Communication, eds K. Jamieson, K. Hall, D. Kahan, and D. A. Scheufele (Oxford; New York, NY: Oxford University Press), 61–72. doi: 10.1093/oxfordhb/9780190497620.013.6
Hennig, M., Brandes, U., Pfeffer, J., and Mergel, I. (2012). Studying Social Networks. A Guide to Empirical Research. Frankfurt: Campus.
Herring, S. C. (2010): “Computer-mediated conversation: introduction and overview.” Language@Internet 7, 1–12. Available online at: http://www.languageatinternet.org/articles/2010/2801.
Heydari, A., Zhang, J., Appel, S., Wu, X., and Ranade, G. (2019). YouTube Chatter: Understanding Online Comments Discourse on Misinformative and Political YouTube Videos. Available online at: https://arxiv.org/abs/1907.00435 (accessed July 07, 2020).
Holmqvist, K., Nyström, M., Andersson, R., Dewhurst, R., Jarodzka, H., and van de Weijer, J. (2011). Eye Tracking: A Comprehensive Guide to Methods and Measures. Oxford: Oxford University Press.
Hooge, I., and Camps, G. (2013). Scan path entropy and arrow plots: capturing scanning behavior of multiple observers. Front. Psychol. 4:996. doi: 10.3389/fpsyg.2013.00996
Humphreys, M. S., and Bain, J. D. (1983). Recognition memory: a cue and information analysis. Mem. Cogn. 11, 583–600. doi: 10.3758/BF03198283
Just, M. A., and Carpenter, P. A. (1976). Eye fixations and cognitive processes. Cognitive Psychol. 8, 441–480. doi: 10.1016/0010-0285(76)90015-3
Kintsch, W. (1968). Recognition and free recall of organized lists. J. Exp. Psychol. 78(Pt 1), 481–487. doi: 10.1037/h0026462
Koch, W., and Beisch, N. (2020). Erneut starke Zuwächse bei Onlinevideo. Ergebnisse der ARD/ZDF-onlinestudie 2020. Media Perspektiven 9, 482–500. Available online at: https://www.ard-werbung.de/fileadmin/user_upload/media-perspektiven/pdf/2020/0920_Koch_Beisch.pdf
Kousha, K., Thelwall, M., and Abdoli, M. (2012). The role of online videos in research communication: a content analysis of YouTube videos cited in academic publications. J. Am. Soc. Inform. Sci. Technol. 63, 1710–1727. doi: 10.1002/asi.22717
Krejtz, K., Szmidt, T., and Krejtz, I. (2014). Entropy-based Statistical Analysis of Eye Movement Transitions. Safety Harbor, FL: ETRA, 159–166. doi: 10.1145/2578153.2578176
Kress, G. (2012). “Multimodal discourse analysis,” in The Routledge Handbook of Discourse Analysis, eds J. P. Gee and M. Handford (London; New York, NY: Routledge), 35–50.
Landrum, A. R., Olshansky, A., and Richards, O. (2019). Differential susceptibility to misleading flat earth arguments on youtube. Media Psychol. 1–30. doi: 10.1080/15213269.2019.1669461
Lazarsfeld, P. (1937). Some remarks on the typological procedures in social research. Zeitschrift für Sozialforschung 6, 119–138. doi: 10.5840/zfs193761137
Lederman, J. (2016). Science Slams Could be the Future of Communicating Research, Popular Science. Available online at: https://www.popsci.com/science-slams-could-be-future-communicating-research (accessed October 28, 2020).
León, B., and Bourk, M. (2020). “Investigating science-related online video,” in Communicating Science and Technology through Online Video. Researching a New Media Phenomenon, eds B. León, and M. Bourk (New York, NY; London: Routledge), 8–20.
Lo, A. S., Esser, M. J., and Gordon, K. E. (2010). YouTube: a gauge of public perception and awareness surrounding epilepsy. Epilepsy Behav. 17, 541–545. doi: 10.1016/j.yebeh.2010.02.004
Lobato, R. (2016). The cultural logic of digital intermediaries: YouTube multichannel networks. Convergence 22, 348–360. doi: 10.1177/1354856516641628
Maisto, S. A., Dewaard, R. J., and Miller, M. E. (1977). Encoding processes for recall and recognition: The effect of instructions and auxiliary task performance. Bull. Psychonomic Soc. 9, 127–130. doi: 10.3758/BF03336951
Mayer, R. E. (2014). “Cognitive theory of multimedia learning,” in The Cambridge Handbook of Multimedia Learning, 2nd Edn, ed R. E. Mayer (New York, NY: Cambridge University Press), 43–71. doi: 10.1017/CBO9781139547369.005
Mayer, R. E., and Fiorella, L. (2014). “Principles for reducing extraneous processing in multimedia learning: coherence, signaling, redundancy, spatial contiguity and temporal contiguity,” in The Cambridge Handbook of Multimedia Learning, 2nd Edn, ed R. E. Mayer (New York, NY: Cambridge University Press), 278–315.
Minol, K., Spelsberg, G., Schulte, E., and Morris, N. (2007). Portals, blogs and co.: the role of the internet as a medium of science communication. Biotechnol. J. 2, 1129–1140. doi: 10.1002/biot.200700163
Morcillo, J. M., Czurda, K., and Robertson-von Trotha, C. Y. (2016). Typologies of the popular science web video. J. Sci. Commun. 15:4. doi: 10.22323/2.15040202
Myers, G. (2003). Discourse studies of scientific popularization: questioning the boundaries. Discourse Stud. 5, 265–279. doi: 10.1177/1461445603005002006
Neuberger, C., and Jarren, O. (2017). Thesen zum Wandel der Wissenschaftsöffentlichkeit und zur Wissenschaftsvermittlung im Internet, in Perspektiven der Wissenschaftskommunikation im digitalen Zeitalter, eds P. Weingart, H. Wormer, and R. F. Hüttl (Weilerswist: Velbrück Wissenschaft), 65–77. [Theses to the transformation oft the scientific public sphere and to knowledge transfer in the Internet]
Neumann, O. (1996). “Theories of attention, in Handbook of Perception and Action, Vol. 3, eds O. Neumann and A. F. Sanders (London; San diego: Academic Press), 389–446. doi: 10.1016/S1874-5822(96)80027-2
Nisbet, M. C., and Scheufele, D. A. (2009). What's next for science communication? Promising directions and lingering distractions. Am. J. Bot. 96, 1767–1778. doi: 10.3732/ajb.0900041
Novak, J. D., and Gowin, D. B. (1984). Learning How to Learn. New York, NY: Cambridge University Press. doi: 10.1017/CBO9781139173469
Paas, F., and Sweller, J. (2014). “Implications of cognitive load theory for multimedia learning,” in The Cambridge Handbook of Multimedia Learning, 2nd Edn, ed R. E. Mayer (New York, NY: Cambridge University Press), 27–42. doi: 10.1017/CBO9781139547369.004
Paik, E., and Schraw, G. (2013). Learning with animation and illusions of understanding. J. Educ. Psychol. 105, 278–289. doi: 10.1037/a0030281
Rödder, S., and Schäfer, M. S. (2010). Repercussion and resistance: an empirical study on the interrelation between science and mass media. Communications 35, 249–267. doi: 10.1515/comm.2010.014
Rosenthal, S. (2018). Motivations to seek science videos on YouTube: free-choice learning in a connected society. Int. J. Sci. Educ. Part B 8, 22–39. doi: 10.1080/21548455.2017.1371357
Rosenthal, S. (2020). Media literacy, scientific literacy, and science videos on the internet. Front. Commun. 5:581585. doi: 10.3389/fcomm.2020.581585
Ruiz-Primo, M. A. (2004). “Examining concept maps as an assesment tool. Concept maps: theory, methodology, technology,” in Proceedings of the First International Conference on Concept Mapping Pamplona (Spain), 2004.
Saunders, B., Sim, J., Kingstone, T., Baker, S., Waterfield, J., Bartlam, B., et al. (2018). Saturation in qualitative research: exploring its conceptualization and operationalization. Qual. Quantity 52, 1893–1907. doi: 10.1007/s11135-017-0574-8
Schäfer, M. (2017). “How Changing media structures are affecting science news coverage,” in The Oxford Handbook of the Science of Science Communication, eds K. Jamieson, K. Hall, D. Kahan, and D. A. Scheufele (Oxford; New York, NY: Oxford University Press). doi: 10.1093/oxfordhb/9780190497620.013.5
Schäfer, M., Kessler, S. H., and Fähnrich, B. (2020). “Analyzing science communication through the lense of communiciation science: reviewing the empirical evidence,” in Science Communiciation. Handbooks of Communication Science, Vol. 17, eds A. Leßmöllmann, M. Dascal, and T. Gloning (Boston, MA; Berlin: DeGruyter), 77–104.
Scharrer, L., Rupieper, Y., Stadtler, M., and Bromme, R. (2016). When science becomes too easy: science popularization inclines laypeople to underrate their dependence on experts. Public Understand. Sci. 26, 1003–1018. doi: 10.1177/0963662516680311
Schnotz, W. (2014). “Integrated model of text and picture comprehension,” in The Cambridge Handbook of Multimedia Learning, 2nd Edn, ed R. E. Mayer (New York, NY: Cambridge University Press), 72–103. doi: 10.1017/CBO9781139547369.006
Schnotz, W., and Rasch, T. (2005). Enabling, facilitating, and inhibiting effects of animations in multimedia learning: why reduction of cognitive load can have negative results on learning. Educ. Technol. Res. Dev. 53, 245–265. doi: 10.1007/BF02504797
Shapiro, M. A., and Park, H. W. (2015). More than entertainment: YouTube and public responses to the science of global warming and climate change. Soc. Sci. Inform. 54, 115–145. doi: 10.1177/0539018414554730
Walsh, L. (2015). The double-edged sword of popularization: the role of science communication research in the popsci.com comment shutoff. Sci. Commun. 37, 658–669. doi: 10.1177/1075547015581928
Wang, J., Antonenko, P., and Dawson, K. (2020). Does visual attention to the instructor in online video affect learning and learner perceptions? An eye-tracking analysis. Comput. Educ. 146, 103–779. doi: 10.1016/j.compedu.2019.103779
Wasserman, S., and Faust, K. (1994). Social Network Analysis. Methods and Applications. Cambridge: Cambridge University Press. doi: 10.1017/CBO9780511815478
Weingart, P. (2012). “The lure of the mass media and its repercussions on science” in The Sciences' Media Connection - Public Communication and its Repecussions, eds S. Rödder, M. Franzen, and P. Weingart (Dordrecht; Heidelberg; London; New York, NY: Springer), 17–32. doi: 10.1007/978-94-007-2085-5_2
Welbourne, D. J., and Grant, W. J. (2016). Science communication on youtube: factors that affect channel and video popularity. Public Understand. Sci. 25, 706–718. doi: 10.1177/0963662515572068
Keywords: science communication, multimodality, YouTube, reception study, knowledge transfer
Citation: Boy B, Bucher H-J and Christ K (2020) Audiovisual Science Communication on TV and YouTube. How Recipients Understand and Evaluate Science Videos. Front. Commun. 5:608620. doi: 10.3389/fcomm.2020.608620
Received: 21 September 2020; Accepted: 17 November 2020;
Published: 17 December 2020.
Edited by:
Asheley R. Landrum, Texas Tech University, United StatesReviewed by:
Sonny Rosenthal, Nanyang Technological University, SingaporeClare Wilkinson, University of the West of England, United Kingdom
André Bresges, University of Cologne, Germany
Copyright © 2020 Boy, Bucher and Christ. This is an open-access article distributed under the terms of the Creative Commons Attribution License (CC BY). The use, distribution or reproduction in other forums is permitted, provided the original author(s) and the copyright owner(s) are credited and that the original publication in this journal is cited, in accordance with accepted academic practice. No use, distribution or reproduction is permitted which does not comply with these terms.
*Correspondence: Bettina Boy, Ym95QHVuaS10cmllci5kZQ==