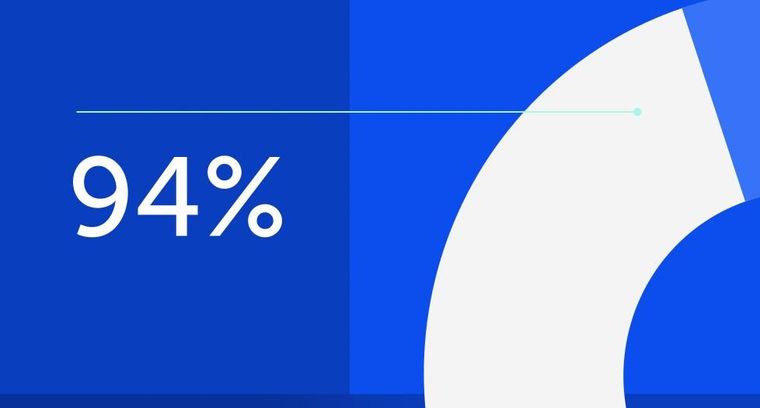
94% of researchers rate our articles as excellent or good
Learn more about the work of our research integrity team to safeguard the quality of each article we publish.
Find out more
PERSPECTIVE article
Front. Commun., 17 December 2020
Sec. Science and Environmental Communication
Volume 5 - 2020 | https://doi.org/10.3389/fcomm.2020.598454
This article is part of the Research TopicNew Directions in Science and Environmental Communication: Understanding the Role of Online Video-Sharing and Online Video-Sharing Platforms for Science and Research CommunicationView all 23 articles
With 1 billion watch-time hours per day, YouTube now plays a major role in communication. Unfortunately, a large amount of misinformation is produced and widely shared on this platform (Donzelli et al., 2018; Allgaier, 2019; Loeb et al., 2019). In this paper, after providing a brief overview of the creation of science content on YouTube, we particularly emphasize the importance of YouTube's automated recommendations. We then discuss the main challenges of making such recommendations aligned with quality science communication.
“Without our science communicators to publicly inform, explain, teach, decode, counter misinformation, and debate science matters many would remain in a space where they don't have [the] information they need, leading to poor choices being made at really crucial times,” New Zealand Prime Minister Jacinda Arden asserted in July 2020 (LeBard, 2020). The COVID-19 pandemic is surely testing the importance of science communicators and, in many cases, the lack thereof (Yong, 2020).
Our societies arguably face challenges of increasing complexity, from pandemic mitigation to climate change, social inequalities, and mass surveillance. Despite this, some views opposing the scientific consensus are proliferating at a concerning rate, and this includes various critical topics such as climate change (Allgaier, 2019), cancer (Loeb et al., 2019), and vaccination (Donzelli et al., 2018). Disturbingly, Johnson et al. (2020) fit a model that “reproduces the recent explosive growth in anti-vaccination views [on social medias] and predicts that these views will dominate in a decade.” The rise of misinformation, or simply the lack of quality information, seems to be a major risk factor for our societies in addition to numerous other social-media-related issues, such as online polarization (Tucker et al., 2018), anger pandemics (Berger and Milkman, 2012; Fan et al., 2014), and loneliness (Hunt et al., 2018) to name a few. In this context, quality science communication1 has arguably become critical for the future of humanity.
The diffusion of information is currently undergoing a major shift with the rise of online platforms. According to a survey by Shearer and Gottfried (2017), around two Americans out of three report that “they get at least some of their news on social medias.” In this paper, we will focus on the particular case of YouTube mostly because of its scale. YouTube claims2 to have 2 billion users, each with an average of 30 min of daily watch time. According to Lewis (2020), this adds up to more daily views on YouTube (6.8 billion views) than searches on Google (5.9 billion queries). Through repeated exposure (see Zajonc and Rajecki, 1969; Kahneman, 2011; Kramer et al., 2014; Jackson, 2019), a vast fraction of the population seems greatly under the influence of what YouTube exposes them to on a daily basis (Lewis, 2018). To understand today's science communication, it thus seems critical to have at least an overview of the science communication produced and shared on YouTube. This will be the topic of section 2.
An important feature of YouTube is the central role played by its recommendation algorithm, which suggests home page videos, lists videos to watch next, and responds to YouTube search queries. According to YouTube's Chief Product Officer (see Solsman, 2018), 70% of the views on YouTube result from recommendations by the YouTube algorithm. This suggests that this algorithm is particularly critical for the future of communication on any topic. If the YouTube algorithm is somehow tweaked to recommend quality science contents two times more often than it currently does, then we might expect, at a first order approximation, that quality science YouTube videos will increase their reach by 70%; it is not clear, however, whether second-order effects will decrease or increase this.
Interestingly, a 2019 survey by Shearer and Grieco (2019) suggests that a large fraction of Americans are aware of this large-scale impact of recommendation algorithms. Around two thirds of surveyed individuals believe that social media companies have “too much control over the news people see.” Given the stakes of science literacy in the twenty-first century, as will be argued in section 3, it might be urgent to demand that the algorithms that control so much of the flow of information on social medias be aligned with quality science communication, as argued by Hoang (2020a).
Unfortunately, as discussed at length by El-Mhamdi and Hoang (2019), such an alignment of recommendation systems is challenging for both technical and non-technical reasons. In section 4, we discuss several of these challenges, as well as proposals to surmount them. In particular, we will defend the need for expert-driven content recommendation like Hoang (2020c), which builds upon the collaborative ethical design framework proposed by Noothigattu et al. (2018) and Lee et al. (2019).
The early 2010s saw the rise of numerous science YouTube channels, such as Veritasium, Smarter Every Day, Numberphile, CGP Grey, Minute Physics, and ASAP Science among numerous others. Since then, thousands of channels have grown, some of which now have over 1 million subscribers. A few of these channels, such as SciShow or Mark Rober, have received over 1 billion views in total. However, most science YouTube channels remain small, with apparently a heavy tail of very small channels (Blanchard et al., 2018). Most successful channels seem mostly supported by in-video advertisement, YouTube's added advertisement, crowd-funding, and derived products such as books or goodies.
However, several channels have been supported or launched by organizations. The Public Broadcasting Service (PBS) Digital Studio hosts Crash Course, Physics Girl, It's Okay to Be Smart, PBS Space Time, PBS Infinite Series, and PBS Hot Mess, while specific series within channels, like Mind Field, Planet Slow Mo, The School of., Could You Survive the Movies?, and Sleeping with Friends, are supported directly by YouTube itself. A few journalistic organizations also produce consistently successful science videos, like Vox, Wired, and Seeker, while other institutions like NASA, the World Heath Organization, and the National Science Foundation have a less consistent success. Recordings of lectures or talks, published by foundations or institutes like TED or MIT OpenCourseWare, also have a large variance in terms of success. A few videos exceed 1 million views, but most have less than 1,000 views.
Successful videos cover a wide range of topics from theoretical physics to social sciences. Perhaps more surprisingly, they also cover a wide range of technical levels. Remarkably, The hardest problem on the hardest test, a video by 3Blue1Brown, has received over 7 million views, while The Banach–Tarski Paradox by VSauce had over 29 million views, even though these videos present the proofs of high-level mathematics. In fact, some channels like Two Minute Papers (with nearly 700,000 subscribers) are devoted to research publications.
A survey by Beautemps and Bresges (submitted) yields greater insight into the audience of science YouTube videos. According to the survey, most viewers of a selection of German science YouTube channels are young, between 13 and 24, and overwhelmingly male (88%). Around 60% of viewers are not studying natural sciences (10% provided no answer), but around 85% of them have an interest or a strong interest in natural sciences. Science videos seem to currently fail to attract viewers with little prior interest in science.
Arguably, in terms of views, subscribers, and engagement, the most successful format consists of a presentation by a host, with multiple illustrative images accompanying the hosts' explanations. The host often speaks directly on camera, though they are sometimes completely off camera. There is often a single host, or at least a main host, who may then feature extracts of interviews of experts (Welbourne and Grant, 2016). One notable exception is the format of Periodic Videos, Objectivity, and Computerphile, among others, where the host is secondary, and the explanations are essentially provided by a given expert.
Having said this, many other formats can be successful as well, including lectures, media conferences, and interviews. In particular, in more recent years, podcasts in the form of discussions between hosts, with or without guests, have gained importance, on channels like Hello Internet, Mindscapeand, Lex Fridman. Finally, there are interesting artistic takes on sometimes very rigorous science, for instance on Epic Rap Battle or acapellascience. Perhaps most iconic in this regard is a collaboration between Vietnamese health authorities and Vietnamese artists to alert the population of the COVID-19 risks. This resulted in the song Ghen Co Vy that had over 67 million views on YouTube alone (the song has been viral on TikTok too, and it has been remixed in many ways on numerous channels).
On March 11, 2020, the World Health Organization characterized COVID-19 as a pandemic. Shortly afterwards, an extraordinary collaboration of 39 French science YouTubers collectively produced and published the same Creative-Commons video, entitled “Coronavirus: Chaque JOUR compte3” and released on March 14, which urged viewers to physically distance themselves from one another and, if possible, to stay home. The video obtained a total of half a million views on at least 28 channels4.
It is quite remarkable that the equivalent of nearly 1% of the French population watched this video, which was produced for free by a large group of volunteers. Nevertheless, the fact that this video did not reach a lot more users can be seen as a missed opportunity. Given that the video was released at the heart of the exponential spread of COVID-19 in France, Hoang (2020b) estimated, based on very rough calculations5, that the video might have saved around 10 lives. But if the video made 10 million views, then perhaps hundreds of lives would have been saved.
This example highlights a critical feature of science communication. It is not sufficient for quality content to be accessible. For the content to actually be impactful—to, in this case, save many lives—it also most importantly needs to be accessed. In fact, nowadays, at least for some topics, the bottleneck of science communication is arguably no longer the production of quality contents, especially on widely covered topics, such as vaccination, climate change, and scientific methods. More often than not, the bottleneck has become the large-scale promotion of top-quality content.
In this context, especially on YouTube, one entity is overwhelmingly more influential than anyone else. This entity is YouTube's recommendation algorithm. Recall that two views out of three on YouTube result from the choice of a video to recommend by this algorithm. The algorithm can easily be designed so that a particular video makes 10 million views instead of half a million. Unfortunately, thus far, especially for outsiders, not only the algorithm is mainly a black box but so is so much of what is happening on the platform. More transparency seems critical to better understand the impacts of YouTube on society, and what can be done to avoid the nasty side effects of the platform (Taylor, 2020).
More generally, the flow of information is perhaps what shapes our societies the most in terms of economy, science, public health, politics, activism, daily habits, and beliefs. What entity controls the flow of information the most? Arguably, this entity is no longer a human; YouTube's algorithm is arguably the entity that controls the flow of information in the world the most. As a result, the future of science communication seems to be, by and large, in its hands.
Following the COVID-19 crisis, on June 11, 2020, YouTube's CEO Wojcicki (2020) announced on YouTube's official blog that, among other things, YouTube is consulting the World Health Organization and local health organizations on a regular basis to combat “harmful medical misinformation”. Wojcicki (2020) claims that, as a result of this, 200,000 videos were removed from YouTube.
While this cooperation is arguably a great news, it is noteworthy that the focus seems to be mostly on removing misleading, abusive, and hate contents. Unfortunately, such removals are often described as censorship by critics. In fact, there may be a reasonable fear that such removal decisions may fuel some conspiracy theories that already contest current authorities. This may be all the more the case when the author of the removed content is a major political figure, as in the case of the removal of President Trump's claim that children are “almost immune” to COVID-19 (see Culliford, 2020). More generally, content removal seems to be associated with high risk of backfire effects (Nyhan and Reifler, 2010; Trujillo et al., 2020).
Perhaps rather than a spectacular binary decision to remove misleading contents, a more nuanced solution could be to downgrade the recommendation rate of more problematic contents. Interestingly, in an interview by Sandlers (2019), Tessa Lyons, Facebook's Product Manager in charge of news feeds, says that Facebook used to only remove content that violated the platform policies, such as pornography, hate speech, and graphic violence. However, they found out that users were then seeking to post the most extreme content just below the removal threshold because such content was a lot more likely to go viral. Now, Lyons says, Facebook rather makes sure, using its recommendation algorithm, that contents that approach the removal threshold are widely de-recommended. Interestingly, this also incentivizes users to produce less extreme content. Arguably more research into the effects of de-recommendation, as opposed to content removal, is needed to better understand which strategy is preferrable.
But perhaps Facebook's approach is not going far enough. Recommendation algorithms have the potential to shift the battlefield of the economy of attention (Franck, 2019), where every user, influencer, advertiser, activist, web platform, and politician competes for the attention of their audience. These days, this battlefield is arguably mostly dominated by those who invested the most in hacking social media algorithms and user attention, often with clickbait, divisive, and addictive contents (Tufekci, 2017), and intent toward financial or political profit. Robustly beneficial recommendation algorithms could not only help to fight harmful contents; they could also make sure that the top-quality content will not be drowned in an ocean of sensationalism (Jackson, 2019). In fact, these days, the main misinformation may not be misleading information but rather the prevalence of unimportant information.
“In the past, censorship worked by blocking the flow of information,” Harari, 2016 argues. “In the twenty-first century, censorship works by flooding people with irrelevant information. People just don't know what to pay attention to, and they often spend their time investigating and debating side issues.” The more important news that fail to reach a large audience are sometimes known as mute news. The lack of attention to such mute news may be a greater concern than the presence of fake news (Rosling et al., 2018). Yet, to solve the problem of mute news, removing bad apples will be useless. What should perhaps be done, instead, is to identify top-quality content and flood YouTube with a stream of this content.
Evidently, one critical challenge will be to convince YouTube and other social medias to adopt such a strategy. This will not be easy. After all, it will likely at least partly conflict with companies' current desire to maximize user retention (Franck, 2019). Social pressure, and probably regulation, will likely be critical to get there. Interestingly, however, such companies seem to be giving increasing attention to the ethics of their recommendations even though this attention remains arguably largely insufficient (Nicas, 2020). Perhaps more importantly, reliable and scalable solutions to identify quality content are still lacking, which makes the advocacy for their implementations very difficult.
In fact, identifying top-quality content is a challenging endeavor in itself. For one thing, it seems important to acknowledge that even science communicators will disagree on the definition of what “quality content” is and thus on what makes content worth sharing.
Clearly, the reliability of the information presented by the content is a key feature. Surely, like quality scientific publications, quality science communication should be accompanied with reliable sources and should present the scientific consensus if it exists. Quality content should be transparent about the methods used and should perhaps also share the data it has relied on. But this is arguably far from being the only thing that matters.
As discussed earlier, it seems at least as critical to prioritize contents that address important topics. Reliable content on some anecdotal event is usually not the most urgent content to be shared on a large scale. Perhaps more interestingly, the effect size of an idea discussed in a content should also be taken into account. For instance, in terms of environmental impact, content arguing for local food consumption may not be as important to promote as a content arguing for the reduction of meat consumption (see Weber and Matthews, 2008; Ritchie and Roser, 2020). Unfortunately, fully agreeing on what is important is likely hopeless. Voting methods are probably critical to reach any agreement.
But this is not all. Arguably, quality content should also be extremely pedagogical rather than superficial or dogmatic. In fact, Muller (2008) showed that even correct and seemingly pedagogical videos in physics can fail to reliably teach concepts to viewers. Worse, such content may increase the viewer's self-confidence even when the viewer's prejudices are contradicted by the video. Overall, it seems critical to further investigate what makes pedagogical videos effective and to make sure that the results of this research are taken into account to promote videos that really have a strong positive impact.
There are still other features that may also seem critical to identifying the videos worth sharing. A top-quality video should arguably also be engaging. In fact, it seems desirable that it presents science enthusiastically, raises numerous questions, points to further contents, promotes intellectual humility and triggers genuine curiosity (Davies, 2019). In fact, the evidence collected by Kahan et al. (2017) suggests that scientific curiosity is a critical trait to fight politically motivated reasoning. Additionally, quality content should probably also minimize the risks of backfire effects, such as viewers increasing their confidence in their biased views (Taber and Lodge, 2006).
At this point, it seems clear that the content produced by recognized authorities, like the World Health Organization, are not always the contents that should be promoted in priority. The YouTube ecosystem hosts millions of videos designed by science communication talents. It would probably be greatly suboptimal not to recommend these videos.
Unfortunately, identifying these videos in the ocean of YouTube content is an extremely challenging task. Recently, the Tournesol framework has been proposed by Hoang (2020c). Tournesol aims to query experts to collect data on what experts regard as quality content according to the different features we discussed. More precisely, it asks any user from a trusted institution (universities, health agencies, NGOs, etc.) to register on the platform by confirming their certified email address. The user is then regarded as an expert by the platform. The expert is then asked to select any two videos of their choice and to say which video is more reliable, which is more pedagogical, which is more important, which is more engaging, and which is more resilient to backfire risks. Note that since Tournesol only aims at identifying quality content, it need not be exhaustive about its video reviewing process.
Tournesol then leverages a machine learning model inspired from Bradley and Terry (1952) to infer what scores the expert would assign to the videos they rated for different quality features. Tournesol then aggregates the scores from different experts using a median-like operator, akin to majority judgment (Balinski and Laraki, 2011). The global scheme is a collaborative computable ethics design inspired from Noothigattu et al. (2018) and Lee et al. (2019). Users that search contents can then adjust the importance they give to the different quality features, to obtain personalized recommendations. Perhaps this framework, which needs further research to optimize, may pave the way toward more robustly aligned recommendation algorithms6.
In this paper, we stressed the importance of science communication for the future of our societies. We also argued that today's main bottlenecks to science communication are the social media platforms' recommendation algorithms and YouTube's in particular. We discussed the challenges posed in trying to make the YouTube recommendation algorithm robustly beneficial, and we also touched on a currently investigated path to partially solve these challenges. We are facing a challenging endeavor. But, as argued by El-Mhamdi and Hoang (2019), this great endeavor may be viewed above all as a fabulous endeavor. After all, it boils down to answering what is arguably the most central question of science communication: What is quality science communication?
The author confirms being the sole contributor of this work and has approved it for publication.
The author declares that the research was conducted in the absence of any commercial or financial relationships that could be construed as a potential conflict of interest.
The author is thankful for fruitful feedback from Joachim Allgaier, Pleen Le Jeune, Sébastien Carassou, Antoine Blanchard, Erik Gustafsson, Antonin Broi, and three reviewers.
1. ^Defining quality science communication is one of the great challenges discussed in section 4. For now, it can be defined as a science communication that nearly all viewers, with expertise in the topic discussed, would describe as quality science communication.
2. ^See https://youtube.com/about/press/.
3. ^Coronavirus: Each DAY counts.
4. ^The author of the present paper is one of the participants of this massive collaboration.
5. ^Essentially, the model assumed that only half of the population was prudent, that prudence reduced R from 2 to 0.8, that 80% of the viewers were prudent, and that the video convinced half of imprudent viewers to be prudent. It then projected an exponential growth over 8 weeks. The projections should be taken with a huge grain of salt, as the results are unfortunately very sensitive to the parameters.
6. ^At the time of writing, the platform is still being developed. It will be available, however, on https://tournesol.app.
Allgaier, J. (2019). Science and environmental communication via online video: strategically distorted communications on climate change and climate engineering on youtube. Front. Commun. 4:36. doi: 10.3389/fcomm.2019.00036
Balinski, M., and Laraki, R. (2011). Majority Judgment: Measuring, Ranking, and Electing. Cambridge, MA; London: MIT Press.
Berger, J., and Milkman, K. L. (2012). What makes online content viral? J. Market. Res. 49, 192–205. doi: 10.1509/jmr.10.0353
Blanchard, A., Debove, S., Le Jeune, P., Louapre, D., and Louis, T. (2018). Que sait-on des vidéastes de science sur youtube? Le bulletin de l'amcsti.
Bradley, R. A., and Terry, M. E. (1952). Rank analysis of incomplete block designs: I. the method of paired comparisons. Biometrika 39, 324–345. doi: 10.1093/biomet/39.3-4.324
Culliford, E. (2020). Facebook, Twitter, Youtube pull Trump posts over coronavirus misinformation. Reuters.
Davies, S. R. (2019). Science communication as emotion work: negotiating curiosity and wonder at a science festival. Sci. Cult. 28, 538–561. doi: 10.1080/09505431.2019.1597035
Donzelli, G., Palomba, G., Federigi, I., Aquino, F., Cioni, L., Verani, M., et al. (2018). Misinformation on vaccination: a quantitative analysis of youtube videos. Hum. Vaccines Immunother. 14, 1654–1659. doi: 10.1080/21645515.2018.1454572
El-Mhamdi, E.-M., and Hoang, L. N. (2019). Le fabuleux chantier : rendre l'intelligence artificielle robustement bénéfique. Les Ulis: EDP Sciences.
Fan, R., Zhao, J., Chen, Y., and Xu, K. (2014). Anger is more influential than joy: sentiment correlation in weibo. PLoS ONE 9:e110184. doi: 10.1371/journal.pone.0110184
Hoang, L. N. (2020b). Thread on “coronavirus : chaque jour compte”. Twitter. Available online at: https://twitter.com/le_science4all/status/1238876966603849728
Hoang, L. N. (2020c). Tournesol: Expert-driven content recommendations. Tournesol.app. Available online at: https://bit.ly/tournesol-app
Hunt, M. G., Marx, R., Lipson, C., and Young, J. (2018). No more fomo: limiting social media decreases loneliness and depression. J. Soc. Clin. Psychol. 37, 751–768. doi: 10.1521/jscp.2018.37.10.751
Jackson, J. (2019). You Are What You Read: Why Changing Your Media Diet Can Change the World. Unbound.
Johnson, N. F., Velásquez, N., Restrepo, N. J., Leahy, R., Gabriel, N., El Oud, S., et al. (2020). The online competition between pro-and anti-vaccination views. Nature 582, 230–233. doi: 10.1038/s41586-020-2281-1
Kahan, D. M., Landrum, A., Carpenter, K., Helft, L., and Hall Jamieson, K. (2017). Science curiosity and political information processing. Polit. Psychol. 38, 179–199. doi: 10.1111/pops.12396
Kramer, A. D., Guillory, J. E., and Hancock, J. T. (2014). Experimental evidence of massive-scale emotional contagion through social networks. Proc. Natl. Acad. Sci. U.S.A. 111, 8788–8790. doi: 10.1073/pnas.1320040111
Lee, M. K., Kusbit, D., Kahng, A., Kim, J. T., Yuan, X., Chan, A., et al. (2019). Webuildai: Participatory framework for algorithmic governance. PACMHCI 3, 181:1–181:35. doi: 10.1145/3359283
Lewis, P. (2018). ‘Fiction is outperforming reality’: how youtube's algorithm distorts truth. The Guardian.
Loeb, S., Sengupta, S., Butaney, M., Macaluso, J. N. Jr., Czarniecki, S. W., Robbins, R., et al. (2019). Dissemination of misinformative and biased information about prostate cancer on youtube. Eur. Urol. 75, 564–567. doi: 10.1016/j.eururo.2018.10.056
Muller, D. A. (2008). Designing Effective Multimedia for Physics Education. Sydney, NSW: University of Sydney.
Noothigattu, R., Gaikwad, S. N. S., Awad, E., Dsouza, S., Rahwan, I., Ravikumar, P., et al. (2018). “A voting-based system for ethical decision making,” in Proceedings of the Thirty-Second AAAI Conference on Artificial Intelligence, (AAAI-18), the 30th innovative Applications of Artificial Intelligence (IAAI-18), and the 8th AAAI Symposium on Educational Advances in Artificial Intelligence (EAAI-18), eds S. A. McIlraith and K. Q. Weinberger (New Orleans, LO: AAAI Press), 1587–1594.
Nyhan, B., and Reifler, J. (2010). When corrections fail: the persistence of political misperceptions. Polit. Behav. 32, 303–330. doi: 10.1007/s11109-010-9112-2
Rosling, H., Rosling, O., and Rosling Rönnlund, A. (2018). Factfulness: Ten Reasons We're Wrong About the World–and Why Things Are Better Than You Think. New York, NY: Flatiron Books.
Shearer, E., and Gottfried, J. (2017). News use across social media platforms 2017. Pew Res. Center 7. [Epub ahead of print].
Shearer, E., and Grieco, E. (2019). Americans are wary of the role social media sites play in delivering the news. Pew Res. Center 2. [Epub ahead of print].
Taber, C. S., and Lodge, M. (2006). Motivated skepticism in the evaluation of political beliefs. Am. J. Polit. Sci. 50, 755–769. doi: 10.1111/j.1540-5907.2006.00214.x
Taylor, R. (2020). ‘it's time to crack open the black box of social media algorithms’. The Telegraph.
Trujillo, M., Gruppi, M., Buntain, C., and Horne, B. D. (2020). “What is bitchute? characterizing the “Free Speech” Alternative to YouTube,” in Proceedings of the 31st ACM Conference on Hypertext and Social Media, 139–140. doi: 10.1145/3372923.3404833
Tucker, J. A., Guess, A., Barberá, P., Vaccari, C., Siegel, A., Sanovich, S., et al. (2018). Social media, political polarization, and political disinformation: A review of the scientific literature. Political polarization, and political disinformation: a review of the scientific literature
Tufekci, Z. (2017). Twitter and Tear Gas: The Power and Fragility of Networked Protest. New Haven, CT: Yale University Press.
Weber, C. L., and Matthews, H. S. (2008). Food-Miles and the Relative Climate Impacts of Food Choices in the United States. Environ. Sci. Technol. 42, 3508–3513. doi: 10.1021/es702969f
Welbourne, D. J., and Grant, W. J. (2016). Science communication on youtube: factors that affect channel and video popularity. Public Understand. Sci. 25, 706–718. doi: 10.1177/0963662515572068
Wojcicki, S. (2020). Susan wojcicki: my mid-year update to the youtube community. YouTube Official Blog.
Keywords: youtube, science communication, recommendation, algorithm, artificial intelligence, ethics
Citation: Hoang LN (2020) Science Communication Desperately Needs More Aligned Recommendation Algorithms. Front. Commun. 5:598454. doi: 10.3389/fcomm.2020.598454
Received: 24 August 2020; Accepted: 19 November 2020;
Published: 17 December 2020.
Edited by:
Asheley R. Landrum, Texas Tech University, United StatesReviewed by:
Sibo Chen, Ryerson University, CanadaCopyright © 2020 Hoang. This is an open-access article distributed under the terms of the Creative Commons Attribution License (CC BY). The use, distribution or reproduction in other forums is permitted, provided the original author(s) and the copyright owner(s) are credited and that the original publication in this journal is cited, in accordance with accepted academic practice. No use, distribution or reproduction is permitted which does not comply with these terms.
*Correspondence: Lê Nguyên Hoang, bGUuaG9hbmdAZXBmbC5jaA==
Disclaimer: All claims expressed in this article are solely those of the authors and do not necessarily represent those of their affiliated organizations, or those of the publisher, the editors and the reviewers. Any product that may be evaluated in this article or claim that may be made by its manufacturer is not guaranteed or endorsed by the publisher.
Research integrity at Frontiers
Learn more about the work of our research integrity team to safeguard the quality of each article we publish.