- School of New Media and Communication, Tianjin University, Tianjin, China
Social media has enabled misinformation to circulate with ease around the world during the novel coronavirus disease 2019 (COVID-19) pandemic. This study applies the Crisis and Emergency Risk and Communication model (CERC) to understand the themes and evolution of misinformation on the Internet during the early phases of the COVID-19 outbreak in China, when the epidemic developed rapidly with mysteries. Drawing on 470 misinformation rated as false by three leading Chinese fact-checking platforms between 1 January and 3 February 2020, the analysis demonstrated five major misinformation themes surrounding COVID-19: prevention and treatment, crisis situation updates, authority action and policy, disease information, and conspiracy. Further trend analyses found that misinformation emerged only after the nationwide recognition of the crisis, and appeared to evolve relating to crisis stages, government policies, and media reports. This study is the first to apply the CERC model to investigate the primary themes of misinformation and their evolution. It provides a standard typology for crisis-related misinformation and illuminates how misinformation of a particular topic emerges. This study has significant theoretical and practical implications for strategic misinformation management.
Introduction
The Internet, especially social media, has caused considerable concerns on their roles in promoting misinformation during health crises like disease outbreaks (Tandoc et al., 2018; Waszak et al., 2018). Misinformation can be broadly defined as “information presented as truthful initially but that turns out to be false later on” (Lewandowsky et al., 2013). It may inhibit effective outbreak communication by amplifying public fear and misleading the public to develop practices that might harm their health (Poland and Spier, 2010; Swire and Ecker, 2018). Particularly, in the current coronavirus disease 2019 (COVID-19) pandemic, the United Nations has warned a “misinfo-demic” that is spreading harmful health advice on the Internet1.
A rapidly growing body of literature has investigated the misinformation surrounding disease outbreaks, such as the current COVID-19 pandemic. Research has found that a quarter of social media information (e.g., Twitter, YouTube) contained medical misinformation and unverified content pertaining to the COVID-19 pandemic (Kouzy et al., 2020; Li et al., 2020). Over two-thirds of the top 110 popular websites from the Google Search engine were found to have a low quality of COVID-19 information (Cuan-Baltazar et al., 2020).
Different typologies of misinformation were also identified in previous studies (Bastani and Bahrami, 2020; Brennen et al., 2020). Brennen et al. (2020) found 9 topics of COVID-19 misinformation, with falsehoods about “public authority action,” “community spread,” “general medical advice,” and “prominent actors” as the most prevalent topics. Bastani and Bahrami (2020) identified 5 main categories of misinformation, including “disease statistics,” “treatments, vaccines and medicines,” “prevention and protection methods,” “dietary recommendations,” and “disease transmission ways.” Similarly, studies surrounding the Ebola and Zika outbreak found misinformation about disease health impacts, vaccinations, and disease transmission mechanisms (Oyeyemi et al., 2014; Sommariva et al., 2018; Vijaykumar et al., 2018).
However, two essential research gaps can be identified. First, none of the studies utilized a theoretical model to guide the development of their misinformation typologies. Particularly, it is essential to develop a topology of crisis-related misinformation based on a crisis communication model. A theoretical typology of crisis-related misinformation can facilitate comparisons and integration of previous findings by providing a standard framework. This can also inform better health communication and in turn improve crisis management.
Second, how misinformation emerges and evolves during disease outbreaks remain unclear, preventing strategic crisis management on combating misinformation. Theories indicate that misinformation like rumors is induced during health crises because of the public's unsatisfied information needs (Rosnow, 1991; DiFonzo and Bordia, 2007). Rumors can often play crucial roles in reducing public feelings of anxiety and uncertainty that are triggered by the unknown and threatening circumstances (Rosnow, 1991; DiFonzo and Bordia, 2007). Hence, as the situations develop swiftly during disease outbreaks like COVID-19, it is likely that different types of misinformation emerge and evolve in different time frames corresponding to the information needs of the public. Nevertheless, none of the existing research has explored the temporal patterns of misinformation.
An Application of the Crisis and Emergency Risk Communication Model
This research aims to address the above research gaps by adopting the Crisis and Emergency Risk Communication Model (CERC) to examine the typology and evolution of misinformation during the early phases of a disease outbreak. The CERC is a communication model that guides authorities' communication strategies at different stages of the risk and crisis lifecycle, “from risk, to eruption, to clean-up and recovery, and on into evaluation (p. 51)” (Reynolds and Seeger, 2005). The latter three stages mark the containment and the end of the crisis, when misinformation may not be prevalent. Therefore, this study focuses only on the first two stages of a health crisis. As the model is developed based on the audiences' information needs (Reynolds and Seeger, 2005), it provides the temporal patterns of those needs that can be used to theorize the typology and evolution of crisis-related misinformation.
The pre-crisis stage is the first stage of the CERC model when the crisis is yet to occur. The authorities will need to heighten the public awareness of the potential crisis by warning the public, providing risk information, and educating self-preventions. However, in the context of crisis-related misinformation, as the CERC model suggests that the public is generally not aware of the crisis at this stage (Reynolds and Seeger, 2005), it can be expected that there will be no misinformation during the pre-crisis stage.
The second stage of CERC is the initial stage when the crisis occurs and when the public is first aware of the crisis. The model demonstrates uncertainty reduction, self-efficacy, and reassurance as the three main communication strategies at this stage (Reynolds and Seeger, 2005). In the context of an infectious disease outbreak (Lazard et al., 2015; Lwin et al., 2018), reducing uncertainty is to provide information on case reports and crisis-related events. Messages on self-efficacy are to communicate personal preventions and treatments. Reassurance is information about government interventions. That is, the initial stage is characterized by three urgent information needs: crisis situation updates, self-preventions, and reassurance from authority-initiated actions. Accordingly, misinformation about crisis situations, self-preventions, and authority actions will be prominent at this stage. Specifically, the public requires more accurate understandings of the disease as the crisis continues to develop (Centers for Disease Control and Prevention, 2018; Lwin et al., 2018). Hence, misinformation about disease natures is expected to surge at the later period of the initial stage.
Though the CERC model suggests that misinformation will develop surrounding the four themes during the initial stage of an outbreak, it does not provide detailed predictions on how these four themes of misinformation emerge and evolve within the stage. It is important to understand the rapid evolution of misinformation because disease outbreaks often develop swiftly with many mysteries within the initial stage. Also, breaking news and government responses will be put forth rapidly within this period. It is crucial to explore if government actions and media reports will affect the emergence of misinformation.
This study takes the COVID-19 outbreak in China as a valuable opportunity for applying the CERC to understand the typology and evolution of crisis-related misinformation. Particularly, this research aims to analyse the themes and temporal patterns of COVID-19 misinformation by utilizing data from fact-checking platforms.
The COVID-19 and Fact-Checking Platforms in China
On 31 December 2019, the first cases of COVID-19 were reported in Wuhan, China (Zhu et al., 2020). The disease has been put under national surveillance since 11 January (Tu et al., 2020). A week later, Guangdong, Shanghai, and Beijing reported their first imported cases from Wuhan. As of 23 January, all but two provinces of China reported confirmed cases2. The following days witnessed the quarantine of Wuhan and other cities in the Hubei province3. The disease continued to spread and has seen a broader outreach in China since 27 January, but the number of daily new cases began to drop after 3 February4.
The COVID-19 outbreak has triggered massive amounts of misinformation on social media within China. Fake reports on new cases and unverified information about prevention from the disease (e.g., Taking vitamin C can prevent the disease) have been circulated with ease. Fact-checking platforms, which aim to debunk fake news and online falsehoods, provide novel data sources for misinformation surveillance during the outbreak. This study analyses data from three such platforms, including Jiaozhen, Ding Xiang Yuan, and Toutiao.
Jiaozhen is the leading Chinese fact-checking platform that aims to fight against health-related falsehoods5. It is jointly run by the Health Communication Working Committee of China Medical Doctor Association and Tencent, the company that hosts the most active social media application, WeChat. This platform is providing real-time information services during the COVID-19 outbreak in China, by curating and reviewing hot topics in public health from news and social media with the help of artificial algorithms. Ding Xiang Yuan6, which is the leading social networking site for health professionals in China, has developed a fact-checking platform specifically for the COVID-19 outbreak. The platform is specializing in debunking medical-related misinformation surrounding the disease. Finally, Toutiao is the Chinese leading online news media. It provides services to counter fake news during the crisis by gathering falsehoods from news media7.
Hypotheses and Research Questions
H1: Misinformation will emerge surrounding crisis situation updates, prevention and treatment, authority action and polity, and disease information during the initial stage of the COVID-19 outbreak in China.
RQ1: How did different themes of misinformation emerge during the first two stages of the COVID-19 outbreak suggested by the CERC model?
Methods
Data Extraction
All fact-checking articles published between 1 January and 3 February 2020 were extracted from the three platforms, namely Jiaozhen, Ding Xiang Yuan, and Toutiao. The investigation period marked the first two stages of the outbreak. The pre-outbreak stage was from 1 January to 20 January; the initial stage was from 21 January till the end of the investigation. The two stages were categorized as above because the China government recognized the COVID-19 crisis by confirming human-to-human transmission of COVID-19 and starting daily national reports on the outbreak on 20 January, and the disease began to be contained after 3 February4. The extraction yielded 524 articles, of which 225 on Jiaozhen, 69 on Ding Xiang Yuan, and 230 on Toutiao.
Data Coding Procedures
Data coding of the articles involved several procedures. First, the author and a student assistant independently scanned all articles with their titles and full texts and identified 470 articles that are related to the COVID-19 outbreak. The interrater agreement of the scanning was 100%. Of those relevant articles, 434 rated their fact-checked stories as false. These include 155 articles on Jiaozhen, 64 on Ding Xiang Yuan, and 215 on Toutiao.
Second, the 434 articles were then thematically analyzed with two steps. In step 1, a codebook was developed by the author. The CERC was applied to develop the typology of COVID-19 related misinformation. As discussed, four major themes were derived from the CERC (Reynolds and Seeger, 2005; Lwin et al., 2018): (1) prevention and treatment: misinformation on measures or medication for disease prevention or treatment; (2) crisis situation updates: misinformation on updates or events related to new or existing cases, or other crisis-related situations; (3) authority action and policy: misinformation on government policies taken against the disease or other public policies; and (4) disease information: misinformation pertaining to disease spreading mechanisms, diagnosis, and other disease-related information.
To ensure all themes of misinformation would be captured, an open coding procedure was also employed (Bernard et al., 2016). The fifth theme was derived from the open coding procedure: (5) conspiracy: false statements or accusations that the virus is human-made or that some countries utilized the virus as a bioengineered weapon. The author and the student assistant then read the posts independently and identified finer topics under each of the five themes. The team met to discuss disagreements and agreed on the final codebook.
In step 2, the author and another student assistant independently went through the title and full text of each article and categorized them into themes and topics guided by the codebook. The interrater agreement between the two coders was 87.1%. The coding disagreement was solved by discussions.
Results
Themes of Misinformation (H1)
Table 1 demonstrates the five themes and examples of misinformation. Several topics were identified under the major themes. First, the theme of “prevention and treatment” was predominant, accounting for 31.6% of all misinformation. “Folk medicine” surfaced as one of the most prevalent topics, presenting folk beliefs of alternative medicines such that taking vitamin C can prevent people from the disease. The second common theme was misinformation pertaining to “crisis situation updates,” representing a share of 27.9%. Examples in this theme included fake reports that claimed someone had confirmed with the infection or died of the virus.
“Authority action and policy” emerged as another type of misinformation that was widely spreading (23.5%). Particularly, fake news of isolation controls claiming city quarantine plans was the most frequent topic, making up 15.7% of all misinformation. The fourth common theme was “disease information” (14.7%). An example of this theme is the rumor that the COVID-19 could be transmitted through eye contact between people. Conspiracy (2.3%), such that the coronavirus was bioengineered in the lab, was also circulated extensively within China (Cohen, 2020).
Evolution of Misinformation (RQ1)
A trend analysis was conducted utilizing Jiaozhen as the only data source. The platform aims to provide timely facts within 24 h after a piece of impactful misinformation emerges online. As such, its data allows investigations of the evolution of misinformation. However, data from the other two platforms are not optimal for trend analysis. Ding Xiang Yuan did not provide a timestamp for its articles, and Toutiao revealed a significant time lag of fact-checking during the investigation period.
Figure 1 shows the numbers of COVID-19 misinformation checked by Jiaozhen between 1 January and 3 February 2020. Fact-checking articles that are not related to the disease were also examined for comparison purposes, presenting the baseline fact-check frequency of the platform. Though the virus infections have been put under national surveillance as early as 11 January, misinformation pertaining to the disease emerged only on 21 January, the day when the crisis was nationally recognized. The number of misinformation peaked at the first 2 days of Chinese New Year (i.e., 25 and 26 January).
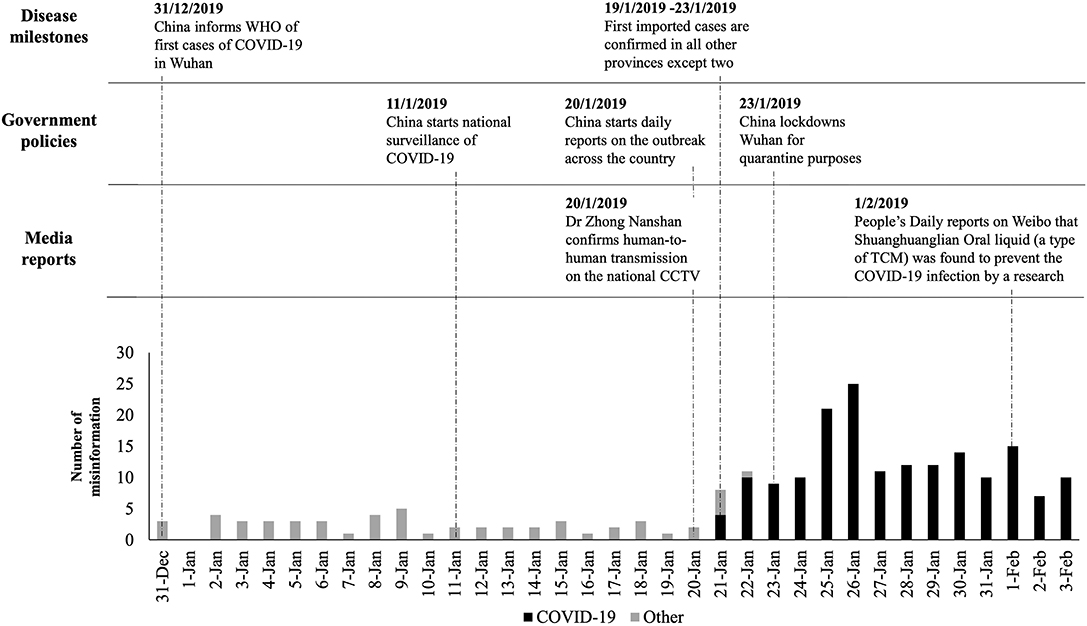
Figure 1. The numbers of COVID-19 misinformation in relation to other misinformation, disease milestones, government policies, and media reports in China.
Figure 2 demonstrates how different themes of misinformation developed during the initial stage of the outbreak. Particularly, three short meaningful phases were identified within this stage based on disease developments and government actions. The preparation phase (Phase 1; 21–23 January) witnessed the period when the disease was first recognized as a national crisis by the public. Misinformation of prevention and treatment (e.g., folk medicine) and crisis situation updates (e.g., case reports) was predominant at this phase.
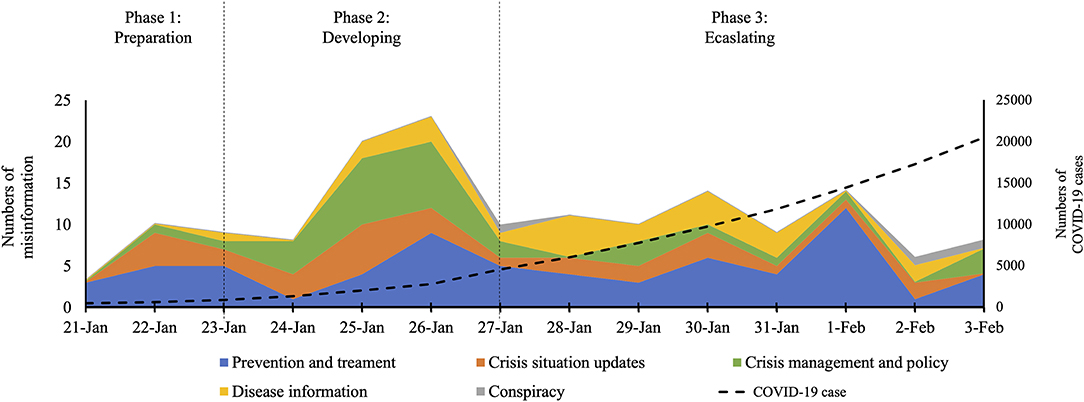
Figure 2. Evolution of misinformation themes in relation to crisis phases and the COVID-19 cases in China.
The developing phase (Phase 2; 24–27 January) started from the day when the Wuhan city was quarantined. The number of misinformation surrounding authority actions and policies surged at this phase, becoming the most frequent theme. Rumors of isolation measures accounted mostly for the increase.
The escalating phase (Phase 3) has seen an upsurge of confirmed cases across the country from 27 January to 3 February. Mysteries surrounding the disease, especially its spreading dynamics, become salient. Interestingly, on 1 February, People's Daily, the official newspaper of the Central Committee of the Communist Party of China, reported a study that argued the effectiveness of Shuanghuanglian Oral Liquid, one of traditional Chinese medicine that is familiar to the public, in preventing the disease infection. This media report coincided with an escalation of misinformation on folk medicine.
Discussion
This study is the first to apply the CERC model to investigate the primary themes of misinformation and their evolution during the early stages of the COVID-19 outbreak in China. Across three platforms, the study demonstrated five major themes of misinformation. The four predominant themes were derived from the CERC model, including crisis situation updates, prevention and treatment, authority action and polity, and disease information, supporting H1. From a health communication perspective, this study provides a standard typology for crisis-related misinformation. This is helpful as the framework can guide future systematic reviews to summarize and compare previous findings. From the crisis communication perspective, the findings suggest that combating crisis-related misinformation and communicating crisis information are two sides of a coin. Though this insight seems intuitive, it illuminates the theoretical possibilities for future integration for the two distinct research fields. It also suggests that the containment of crisis-related misinformation should be implemented simultaneously along with crisis communication.
However, the CERC model did not predict the emergence of conspiracies, though conspiracies were commonly found during health crises (Sommariva et al., 2018; Wood, 2018). This is likely because conspiracies do not emerge from a particular information need; instead, it serves to provide an immediate and holistic understanding of the situation: why the crisis happened, who benefits from it, and who should be blamed (Bessi et al., 2015; Wood, 2018). Though conspiracies accounted for only a tiny proportion of misinformation, they can significantly tarnish the reputations of health authorities and prevent effective health and crisis communication (Cohen, 2020). Future studies should investigate how they can be efficiently prevented and addressed during health crises.
Regarding temporal patterns (RQ1), misinformation emerged only after the national recognition of the crisis, supporting the intuitive prediction from the CERC. Importantly, government policies and media reports appear to elicit misinformation under some circumstances at the initial stage of the COVID-19 outbreak in China (21 January to 3 February). The findings clearly showed the concurrence between the city quarantine and the upsurge of fake news about government policies, and between People Daily's reports and the circulation of misinformation about folk medicine. Given the relatively short investigation period in this study, the causality of their associations cannot be claimed. Future studies should focus on a longer period and conduct time series analysis to understand the effects of government policies and media reports on misinformation.
Nevertheless, those concurrences suggest that misinformation might not emerge randomly or evenly across time. Rather, misinformation of a topic may be induced by an event or information on the same topic. This is likely because ongoing events and information can act as circumstantial evidence for misinformation of a similar topic if they are not communicated effectively. This insight goes beyond the current research that predominantly examines when misinformation emerges (Rosnow, 1980, 1988), by suggesting how misinformation of a particular topic emerges. This suggestion is particularly critical for practitioners as it can strategize the allocations of limited communication resources for misinformation debunking. Future research should investigate how misinformation of a topic emerges and spreads along with ongoing events and information.
Additionally, as misinformation often emerge when official information is lacking (DiFonzo and Bordia, 2007), the findings suggest that crisis management policies, especially strong or extreme ones, should be supported by follow-up communication to ease the public from fear and uncertainty. News media should also frame their reports rigorously and scientifically to avoid misunderstandings.
This study has two limitations. First, the trend analysis was conducted with data of only one platform. As Table 1 clearly shows that different fact-checking platforms tend to gather different themes of misinformation, future research should try to generalize the study results regarding the evolution of misinformation. Second, as this study utilized publicly available data on fact-checking platforms, it is unable to discover mechanisms why particular misinformation is made and circulated. Future studies should conduct surveys and experiments to understand how people create and spread misinformation during a disease crisis.
Conclusions
This study is the first to apply the CERC model to investigate the themes and evolution of misinformation during the early stages of an infectious disease outbreak. Though the study focused on misinformation that emerged surrounding COVID-19 in China, the findings are expected to be generalized into other public health emergencies because they are largely corresponding to the CERC model. This research is of theoretical and practical interest to communication scholars and practitioners who seek to maximize the effectiveness of outbreak communication by combating misinformation surrounding health crises. Future research should examine how and why misinformation is made and circulated by particular groups of people in specific crisis stages, to achieve successful crisis communication through combating misinformation.
Data Availability Statement
The raw data supporting the conclusions of this article will be made available by the authors, without undue reservation.
Author Contributions
JL conceptualized the manuscript, analyzed the data, and contributed to the manuscript writing, reviewed the content, and agreed with submission. All authors contributed to the article and approved the submitted version.
Conflict of Interest
The author declares that the research was conducted in the absence of any commercial or financial relationships that could be construed as a potential conflict of interest.
Footnotes
1. ^United Nations. Hatred going viral in ‘dangerous epidemic of misinformation’ during COVID-19 pandemic. Available online at: https://news.un.org/en/story/2020/04/1061682 (accessed June 26, 2020).
2. ^National Health Commission of the People's Republic of China. Situation reports. Available online at: http://www.nhc.gov.cn/xcs/yqtb/list_gzbd.shtml (accessed June 26, 2020).
3. ^General Office of Hubei Provincial People's Government. Wu Han Shi Xin Xing Guan Zhuang Bing Du Gan Ran De Fei Yan Yi Qing Fang Kong Zhi Hui Bu Tong Gao (Di Yi Hao) Statement on the novel coronavirus infection pneumonia outbreak preparedness in Wuhan (No. 1). Available online at: http://www.gov.cn/xinwen/2020-01/23/content_5471751.htm (accessed June 26, 2020).
4. ^COVID-19 Dashboard by the Center for Systems Science and Engineering (CSSE) at Johns Hopkins University. Available online at: https://coronavirus.jhu.edu/map.html (accessed June 26, 2020).
5. ^Jiaozhen. Available onlin at: https://vp.fact.qq.com/home (accessed June 26, 2020).
6. ^Ding Xiang Yuan. Available onlin at: http://ncov.dxy.cn/ncovh5/view/pneumonia_rumors?from=dxyandsource=andlink=andshare= (accessed June 26, 2020).
7. ^Toutiao. Available onlin at: https://www.toutiao.com/c/user/62596297771/#mid=1585938857001998 (accessed June 26, 2020).
References
Bastani, P., and Bahrami, M. A. (2020). COVID-19 related misinformation on social media: a qualitative study from Iran. J. Med. Internet Res. doi: 10.2196/18932. [Epub ahead of print].
Bernard, H. R., Wutich, A., and Ryan, G. W. (2016). Analyzing Qualitative Data: Systematic Approaches. New York, NY: SAGE Publications.
Bessi, A., Coletto, M., Davidescu, G. A., Scala, A., Caldarelli, G., and Quattrociocchi, W. (2015). Science vs conspiracy: collective narratives in the age of misinformation. PLoS ONE 10:e0118093. doi: 10.1371/journal.pone.0118093
Brennen, J. S., Simon, F. M., Howard, P. N., and Nielsen, R. K. (2020). Types, Sources, and Claims of Covid-19 Misinformation. Reuters Institute. Available online at: http://www.primaonline.it/wp-content/uploads/2020/04/COVID-19_reuters.pdf (accessed June 26, 2020).
Centers for Disease Control Prevention (2018). Crisis and Emergency Risk Communication−2014 Edition. Available online at: https://emergency.cdc.gov/cerc/manual/index.asp (accessed June 26, 2020).
Cohen, J. (2020). Scientists “Strongly Condemn” Rumors and Conspiracy Theories About Origin of Coronavirus Outbreak. Science Magazine. Available online at: https://www.sciencemag.org/news/2020/02/scientists-strongly-condemn-rumors-and-conspiracy-theories-about-origin-coronavirus (accessed June 26, 2020).
Cuan-Baltazar, J. Y., Muñoz-Perez, M. J., Robledo-Vega, C., Pérez-Zepeda, M. F., and Soto-Vega, E. (2020). Misinformation of COVID-19 on the internet: infodemiology study. JMIR Public Health Surveill. 6:e18444. doi: 10.2196/18444
DiFonzo, N., and Bordia, P. (2007). Rumor Psychology: Social and Organizational Approaches. Washington, DC: American Psychological Association.
Kouzy, R., Abi Jaoude, J., Kraitem, A., El Alam, M. B., Karam, B., Adib, E., et al. (2020). Coronavirus goes viral: quantifying the COVID-19 misinformation epidemic on Twitter. Cureus 12:e7255. doi: 10.7759/cureus.7255
Lazard, A. J., Scheinfeld, E., Bernhardt, J. M., Wilcox, G. B., and Suran, M. (2015). Detecting themes of public concern: a text mining analysis of the Centers for Disease Control and Prevention's Ebola live Twitter chat. Am. J. Infect. Control 43, 1109–1111. doi: 10.1016/j.ajic.2015.05.025
Lewandowsky, S., Stritzke, W. G., Freund, A. M., Oberauer, K., and Krueger, J. I. (2013). Misinformation, disinformation, and violent conflict: from Iraq and the “War on Terror” to future threats to peace. Am. Psychol. 68:487. doi: 10.1037/a0034515
Li, H. O., Bailey, A., Huynh, D., and Chan, J. (2020). YouTube as a source of information on COVID-19: a pandemic of misinformation?. BMJ Glob. Health 5:e002604. doi: 10.1136/bmjgh-2020-002604
Lwin, M. O., Lu, J., Sheldenkar, A., and Schulz, P. J. (2018). Strategic uses of Facebook in Zika outbreak communication: implications for the crisis and emergency risk communication model. Int. J. Environ. Res. Public Health 15:1974. doi: 10.3390/ijerph15091974
Oyeyemi, S. O., Gabarron, E., and Wynn, R. (2014). Ebola, Twitter, and misinformation: a dangerous combination?. BMJ 349:g6178. doi: 10.1136/bmj.g6178
Poland, G. A., and Spier, R. (2010). Fear, misinformation, and innumerates: how the Wakefield paper, the press, and advocacy groups damaged the public health. Vaccine 28:2361. doi: 10.1016/j.vaccine.2010.02.052
Reynolds, B., and Seeger, M. W. (2005). Crisis and emergency risk communication as an integrative model. J. Health Commun. 10, 43–55. doi: 10.1080/10810730590904571
Rosnow, R. L. (1980). Psychology of rumor reconsidered. Psychol. Bull. 87, 578–591. doi: 10.1037/0033-2909.87.3.578
Rosnow, R. L. (1988). Rumor as communication: a contextualist approach. J. Commun. 38, 12–28. doi: 10.1111/j.1460-2466.1988.tb02033.x
Rosnow, R. L. (1991). Inside rumor: a personal journey. Am. Psychol. 46:484. doi: 10.1037/0003-066X.46.5.484
Sommariva, S., Vamos, C., Mantzarlis, A., Ðào, L. U., and Martinez Tyson, D. (2018). Spreading the (fake) news: exploring health messages on social media and the implications for health professionals using a case study. Am. J. Health Educ. 49, 246–255. doi: 10.1080/19325037.2018.1473178
Swire, B., and Ecker, U. (2018). “Misinformation and its correction: Cognitive mechanisms and recommendations for mass communication,” in Misinformation and Mass Audiences, eds B. Southwell, E. A. Thorson, and L. Sheble (Austin, TX: University of Texas Press), 195–211.
Tandoc, E. C. Jr, Lim, Z. W., and Ling, R. (2018). Defining “fake news”: a typology of scholarly definitions. Digit J. 6, 137–153. doi: 10.1080/21670811.2017.1360143
Tu, Y., Qing, L., Yi, Z., and Xian, S. J. (2020). People's Daily. Available online at: https://news.sina.com.cn/c/2020-01-31/doc-iimxyqvy9378767.shtml?cre=tianyiandmod=pcpager_finandloc=16andr=9andrfunc=100andtj=noneandtr=9 (accessed June 26, 2020).
Vijaykumar, S., Nowak, G., Himelboim, I., and Jin, Y. (2018). Virtual Zika transmission after the first US case: who said what and how it spread on Twitter. Am. J. Infect. Control 46, 549–557. doi: 10.1016/j.ajic.2017.10.015
Waszak, P. M., Kasprzycka-Waszak, W., and Kubanek, A. (2018). The spread of medical fake news in social media–the pilot quantitative study. Health Policy Tech. 7, 115–118. doi: 10.1016/j.hlpt.2018.03.002
Wood, M. J. (2018). Propagating and debunking conspiracy theories on Twitter during the 2015–2016 Zika virus outbreak. Cyberpsychol. Behav. Soc. Netw. 21, 485–490. doi: 10.1089/cyber.2017.0669
Keywords: COVID-19, misinformation, internet, surveillance, crisis communication, fact-checking
Citation: Lu J (2020) Themes and Evolution of Misinformation During the Early Phases of the COVID-19 Outbreak in China—An Application of the Crisis and Emergency Risk Communication Model. Front. Commun. 5:57. doi: 10.3389/fcomm.2020.00057
Received: 14 May 2020; Accepted: 06 July 2020;
Published: 14 August 2020.
Edited by:
Rukhsana Ahmed, University at Albany, United StatesReviewed by:
Raihan Jamil, Zayed University, United Arab EmiratesZhenyi Li, Royal Roads University, Canada
Copyright © 2020 Lu. This is an open-access article distributed under the terms of the Creative Commons Attribution License (CC BY). The use, distribution or reproduction in other forums is permitted, provided the original author(s) and the copyright owner(s) are credited and that the original publication in this journal is cited, in accordance with accepted academic practice. No use, distribution or reproduction is permitted which does not comply with these terms.
*Correspondence: Jiahui Lu, dGtkaHVpaHVpMjhAZ21haWwuY29t