- 1Department of Psychology, University of Windsor, Windsor, ON, Canada
- 2Department of Linguistics, University of Alberta, Edmonton, AB, Canada
This study examines listening effort, as indexed by pupil dilation, needed for processing foreign-accented speech that varies in intelligibility. Previous research has shown that the magnitude of pupil dilation is influenced by various factors, crucially the amount of noise added to speech. However, the method has not yet been used to examine foreign-accented speech. Here, we determine if the full range of foreign accent intelligibility induces a similar increase in cognitive processing effort as that seen for speech in noise. Further, we examine whether listener experience with the accent mitigates this increase in cognitive effort. The results indicate that as speech becomes less intelligible due to accent, pupil dilation increases. Additionally, experience not only reduces the overall magnitude of pupil dilation, it shifts the threshold at which decreased intelligibility begins to incur additional processing effort. We discuss the present results in terms of listening effort when processing spoken variability. The present study establishes pupillometry as an informative method for investigating the processing demands associated with foreign-accented speech.
Introduction
With recent increases in global travel and immigration, interactions with foreign-accented speakers has become a part of the everyday experience of many language users. Foreign-accented speech is highly variable and can lead to differences in the intelligibility of the message intended by non-native speakers (see Bradlow and Bent, 2008). However, despite the challenges, native listeners can accommodate this variability, generally understanding what their conversation partner is attempting to communicate. While this may initially require additional effort and concentration, comprehension can improve over time (see Bradlow and Bent, 2008). Because effective communication relies on successful comprehension, it is important to understand how accented speech is processed and ultimately understood by listeners. In this study, we use pupil dilation as a measure of the listening effort associated with cognitive processing load to examine how varying intelligibility—due to foreign accentedness—influences the extent and time-course of processing. We further examine how the amount of experience listeners have interacting with non-native speakers also influences processing.
Significant research has been conducted in the area of accented speech processing (see Cristia et al., 2012, for a general overview). That body of research has repeatedly demonstrated that the presence of a foreign accent generally leads to a disruption in processing, which has been observed in both offline processing (i.e., transcription accuracy, see, for example, Bradlow and Bent, 2008) and online processing, including reaction time and eye-tracking (see, for example, Floccia et al., 2009; Porretta et al., 2016), and EEG (see, for example, Hanulíková et al., 2012; Porretta et al., 2017). Importantly, the observed processing costs associated with non-native speech appear to depend on the strength of the accent (see Porretta et al., 2016 for an example using accentedness ratings; Witteman et al., 2013, for an example using vowel substitutions). While processing costs have been repeatedly demonstrated, the precise nature of these costs is still not fully understood. However, recent work has indicated that these processing costs may be due to the underlying dynamics of lexical competition; namely, increased accentedness leads to strong and diffuse activation throughout the lexicon which requires more processing time and effort to resolve the additional competition among words (Porretta and Kyröläinen, 2019).
Despite the processing challenges introduced by foreign-accented speech, both rapid, talker-specific adaptation (Clarke and Garrett, 2004) and gradual, talker-independent adaptation (Bradlow and Bent, 2008) have been shown using online and offline measures. For example, in a cross-modal word verification task—in which reaction time was measured for decisions assessing if a written word matched the final word of an auditory sentence—Clarke and Garrett (2004) showed that participants adapted to a new accent in 2–4 sentences, indicating rapid adaptation to one specific accented talker. Further, Bradlow and Bent (2008) showed that transcription accuracy to a new talker improved if participants had previously been exposed to multiple other talkers of the same accent, suggesting a more generalized adaptation to the accent more broadly, rather than to the specific talker (see Kleinschmidt and Jaeger, 2015, for discussion on generalized adaptation). These studies suggest the importance of listener experience with the accent in question, which has been further investigated using online measures (see Witteman et al., 2013; Porretta et al., 2016).
In both cross-modal identity priming and visual world paradigm eye-tracking to native- and Chinese-accented English, Porretta et al. (2016) showed a significant interaction between gradient foreign accentedness and listener experience interacting with Chinese-accented speakers. With regard to reaction times in the cross-modal identity priming task, stronger accentedness of the auditory prime led to longer reaction times to the written form of the word, indicating that greater accentedness reduced the effectiveness of the prime. Further, regarding looking behavior in the visual world paradigm eye-tracking task, the likelihood of fixating the written form of the word, decreased as accentedness of the auditory token increased, further indicating that accent strength influences certainty within the word recognition process. Both of those patterns interacted with the amount of experience listeners had with Chinese-accented speakers. Specifically, reaction times decreased for listeners with more experience, and likelihood of fixating the written word increased for listeners with greater experience.
Foreign-accents have been likened to a type of noise imposed on the speech stream (see Goslin et al., 2012), because listeners must still perceive sufficient cues in the signal in order to understand the intended message. Research on speech comprehension has shown that the presence of various types of noise (both energetic, in which the mask causes the nature of the target signal to become imperceptible, and informational, in which the mask competes with the target signal) generally leads to diminished intelligibility (see, for example, Sumby and Pollack, 1954). Intelligibility is one way of norming samples of speech (including non-native speech) by calculating the likelihood that the intended message will be understood correctly. As indicated by Munro and Derwing (1995b), while intelligibility and accentedness are related and partially correlated dimensions of foreign-accented speech, they are not one and the same. Using a transcription task, Porretta and Tucker (2015) underscored the partially independent relationship of these two dimensions. In that study they found that the relationship between intelligibility and accentedness is nonlinear, such that intelligibility decreases at a faster rate at higher levels of accentedness. While foreign-accented speech has been shown to influence both intelligibility and processing speed, it is unclear how it is related to the amount of effort listeners must exert in order to process non-native speech.
Previous research on foreign-accented speech has used both offline measures (e.g., transcriptions, ratings) and online measures (e.g., reaction times, eye-tracking, and EEG). These measures all provide interesting perspectives on the processing of foreign-accented speech. Relatively recently, pupillometry—the measurement of pupil dilation—has been employed as a measure of cognitive processing associated with spoken language comprehension. Specifically, pupil dilation represents a physiological correlate of cognitive load which allows for a measurement of cognitive effort during spoken language processing that does not depend on a behavioral response (see Mathôt, 2018, for overview). To the best of our knowledge, pupillometry has not yet been applied to the study of foreign-accented speech processing. Applying pupil dilation to accented speech allows us to gain insight into the magnitude and duration of the cognitive load incurred during processing which, in behavioral studies (e.g., Floccia et al., 2009; Porretta et al., 2016), has been shown to manifest as pervasive processing cost. In particular, the present study applies pupillometry to address questions regarding the extent to which a foreign accent impacts the amount of effort listeners must exert in order to understand non-native speech, and if (and how) challenges related to understanding this naturally occurring form of variability can be overcome through daily life experience.
Cognitive load is generally defined as the extent to which task demands consume available resources for successful completion of the task (Pichora-Fuller et al., 2016). The pupil is commonly known to constrict and dilate with changes in luminance. However, early work in the cognitive domain showed that dilation can measure emotional arousal (Hess and Polt, 1960; Hess et al., 1965; Fitzgerald, 1968; Beatty, 1982). This work was later extended to show that small changes (<1 mm) in dilation also reflect changes in cognitive processing (Beatty and Lucero-Wagoner, 2000; Kafkas and Montaldi, 2012). This pupillary response is believed to be related to neural activity in the locus coeruleus (Kahneman and Beatty, 1966; Kahneman, 1973). This structure, located in the brainstem, controls part of the central nervous system and plays an important role in attentional processes (Laeng et al., 2012). The response is linked to two distinct modes of activity: tonic and phasic. Tonic (or baseline) dilation is slow-changing and is related to general state of arousal or vigilance. Phasic (or event-related) dilation is fast-changing and is related to the processing of task relevant events and stimuli. Generally, this time-locked, stimulus-related phasic response is the variable of interest in psycholinguistic studies. This is typically measured using peak dilation amplitude (maximal dilation value within a given time window), peak latency (the time point of the maximum dilation within a given time window), and mean pupil dilation (average dilation within a given window).
With regard to language processing, pupillary response is sensitive to a host of linguistic variables. Kuchinke et al. (2007) showed that pupillary response varies with lexical frequency such that peak dilation is greater for low frequency words than for high frequency words. Pupillary response is also sensitive to temporary syntactic ambiguity; Engelhardt et al. (2010) showed that when prosodic structure conflicts with syntactic structure, pupil dilation increases, and this is modulated by the presence of a visual context which is either consistent or inconsistent with the correct interpretation. The results of those studies indicate that aspects of language influence the allocation of processing resources and that pupil dilation can index processing load and effort.
It has been shown that pupillary response is larger for auditory stimuli than for visual stimuli (Klingner et al., 2011), and more specifically, pupil dilation can be used to measure cognitive processing load for speech perception (Beatty, 1982). Pertinent to the present research questions, pupillary response has been shown to change with listening effort (see Winn et al., 2018, for discussion on the application of pupillometry to the study of listening effort). These changes have been shown to be systematic and relate to the intelligibility of speech in noise (Zekveld et al., 2010; Kramer et al., 2013; Zekveld and Kramer, 2014). Specifically, peak dilation amplitude, peak latency, and mean pupil dilation all increase as noise reduces intelligibility. This indicates that the presence and intensity of noise leads to greater listening effort. It should be noted that this type of noise can be characterized as signal degradation related to listening condition.
As pointed out by Van Engen and McLaughlin (2018), currently, little-to-no research has been done examining the use of pupillometry to study talker-related challenges (e.g., accented speech) in speech recognition. However, one study has examined pupil dilation in response to native speaker mispronunciations. Tamási et al. (2017) showed that pupillary response in 30-month-old children changes with the detection of mispronunciations. In their study, pictures of objects were followed by a spoken word which was manipulated for the number of articulatory feature changed in the word-initial consonant. For example, the word baby was paired with three mispronunciations: daby (1 feature change), faby (2 feature changes), and shaby (3 feature changes). The results showed that mispronounced words elicit a larger dilation than correct forms and this dilation increased for mispronunciations that were more distant from the correct form.
Lastly, pupil dilation in response to linguistic stimuli may also reflect individual differences. Lõo et al. (2016) showed that pupil dilation during a word naming task reflects individual differences in the frequency effect among participants. In that study, variation was found in both the magnitude and the direction of dilation, suggesting that subjects engaged differently with the task. In a listening task, Zekveld and Kramer (2014) found that individual differences in text reception threshold influenced pupil dilation to spoken stimuli in noise. Specifically, they found that participants who were better at understanding text presented with a visual mask displayed greater dilation. The authors suggested that participants who performed better on the text reception put in more effort, which was then reflected in their pupillary response.
In summary, foreign accent can be described as a type of signal-intrinsic noise, i.e., variability in the signal that is attributable to the speaker, and likely requires increased listening effort. Because of this, accented speech must also be considered within a framework of listening effort, as pointed out by Van Engen and Peelle (2014). Pupillary response has been shown to provide an objective physiological measure of online cognitive processing during listening and has, at least for speech in noise, been shown to reflect listening effort (Zekveld and Kramer, 2014). Given that non-native speech has been likened to speech in noise, pupillometry may provide an informative means for studying the cognitive load and listening effort induced by foreign-accented speech, as suggested by Van Engen and McLaughlin (2018). To the best of our knowledge, this is the first study to apply this method to the study of non-native speech. Further, given that experience with foreign-accented speech has been shown to influence behavioral and electrophysiological measures of processing, and that individual differences may also be indexed by pupil dilation, pupillometry may provide a window into how linguistic experience influences cognitive load and listening effort.
These points taken together lead to our primary research question: Does pupillary response to Chinese-accented speech correspond to previous work examining the processing load induced by reduced intelligibility due to noise? If the listening effort required for foreign-accented speech of varying intelligibility coincides with that required for speech in noise, we expect that as intelligibility falls, pupil dilation will increase. As noted by Zekveld and Kramer (2014), it may be that at very low levels of intelligibility, pupil dilation may diminish, reflecting an overload in which processing demands exceed the available resources. To test this we employ a listen-and-repeat task (similar to that of Zekveld et al., 2010 and Zekveld and Kramer, 2014), in which participants hear a token and must repeat the word they heard. This task provides a simple way for listeners to focus on the stimulus while attempting to comprehend it, in order to ensure that the pupil dilation corresponds to the processing associated with listening effort.
Subsequent to the first question, we ask: Is the observed pattern influenced by experience with the accent in question? If listeners with greater experience with the accent are better able to decode the signal, thus requiring less effort, we expect that as experience increases, the overall effect of intelligibility will be reduced. Additionally, if cognitive overload is reflected in pupil dilation, this may be reflected only in participants with the least amount of experience. To measure experience we ask participants to report the frequency with which they interact with non-native speakers and, more specifically, speakers with a Chinese accent.
With regard to both intelligibility and listener experience, we also examine the time course of the pupillary response. Therefore, we further ask if the time course of pupil dilation—rather than simply peak dilation or peak latency—is modulated by both intelligibility and listener experience. If reduced intelligibility requires additional processing time, we expect that listening effort (as indexed by pupil dilation) will be sustained relative to fully intelligible speech (as measured by transcription accuracy), following the peak of dilation. However, if listener experience attenuates peak pupil dilation, we also expect that the pattern of sustained dilation will be reduced for listeners with greater experience, indicating reduced listening effort later in time. To address these questions we present a pupil dilation study using foreign-accented speech which has been normed for intelligibility. As a part of this, we assess the amount of experience listeners have interacting with non-native speakers.
Methodology
Participants
Eighty-five native speakers of North American English (65 female) were recruited from the University of Alberta Department of Linguistics participant pool and ranged in age from 17 to 42 years old (M = 20.01, SD = 3.80). All reported having normal hearing. All participants provided written informed consent in accordance with study approval by the Research Ethics Board at the University of Alberta.
Stimuli
The auditory stimuli used here were a subset of those used by Porretta et al. (2015) and Porretta and Tucker (2015). These consisted of 40 monosyllabic English words retrieved from the NU Wildcat Corpus of native- and foreign-accented English (Van Engen et al., 2010). This subset contained 5 talkers (1 male native English speaker and 4 male native Mandarin Chinese speakers), sampled to reflect the characteristics of the larger group in terms of the distribution of both accentedness and intelligibility. The items included in the present study (n = 200) had mean intelligibility scores ranging from zero to one (M = 0.72, SD = 0.33) and mean accentedness ratings ranging from 1.03 to 8.73 (M = 4.51, SD = 1.87), on a scale from one (completely native) to nine (completely non-native). Five counterbalanced lists were created to ensure that lexical items were not repeated, while still guaranteeing that each participant would hear words spoken by each talker. Specifically, each participant heard a total of 40 words, eight from each talker. Stimuli were blocked by talker and the presentation of these blocks was randomized. Further, items within blocks were presented in a random order.
Procedure
A listen-and-repeat task, similar to the one used by Zekveld et al. (2010), was employed here. Stimuli were presented using SR Research Experiment Builder (version 1.10.165) via ER-1 insert earphones (Etymotic Research, Inc.), with gaze and pupil data time-locked to the onset of the auditory stimulus. Gaze location and pupil size were sampled at 250 Hz, using an Eyelink II head-mounted eye-tracker (SR Research Ltd.) Prior to beginning the experiment, the system was calibrated to the participants' right eye using a 9-point calibration procedure. A head-mounted Countryman microphone and Korg digital recorder were used to record participants' repetitions.
Participants completed the task in a quiet, windowless room seated in front of a 23-inch LCD computer monitor. Written instructions were provided along with three practice items (from the Native English talker) for familiarization with the task. Prior to each trial, a drift correction was performed. A black fixation cross was displayed on a gray background prior to the onset of the auditory stimulus and remained on screen for the duration of the trial. Following the offset of the auditory stimulus there was a pause of 2,500 ms to allow the pupil dilation to subside. A 500 ms beep then prompted the participant to repeat the word. After the experiment, participants responded to a brief language experience questionnaire—identical to the one used by Porretta et al. (2016)—which was designed to gather information regarding participants' interactions with non-native (specifically Chinese-accented) speakers.
Dependent Variable
The sample data were exported using SR Research Data Viewer (version 2.2.1), relative to the onset of the auditory stimulus, and were further processed in the statistical environment R, version 3.3.3 (R Development Core Team, 2018) using the package PupilPre, version 0.5.0 (Kyröläinen et al., in preparation). Blinks in the pupillary data were first cleaned semi-automatically in 100 ms windows around marked blinks by removing data points in which the participant was entering or exiting a blink. These semi-cleaned data were then manually checked and remaining blink artifacts were cleaned by hand. Trials with more than 20% missing data due to blinks were removed, resulting in the loss of 6.26% of the data set. The data were then downsampled to a rate of 25 Hz and the dilation was baseline normalized (individually by trial) using the average of the 500 ms preceding the onset of the stimulus. Figure 1 shows the average pupil dilation, displaying a typical phasic pupil dilation in response to the auditory stimulus (see Beatty, 1982). These preprocessed pupil dilation data were taken as the dependent variable of interest.
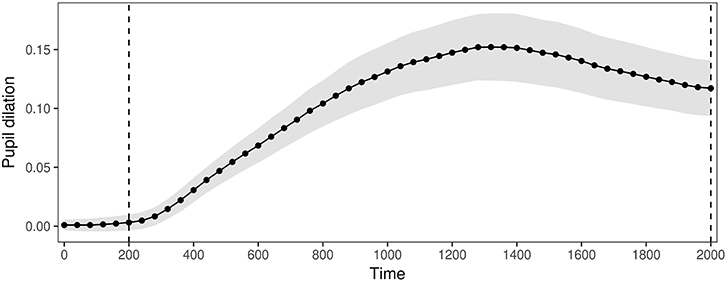
Figure 1. Time-course of pupil dilation. Zero ms represents onset of the auditory stimulus. Shaded bands represent 95% simultaneous confidence intervals. Vertical lines at 200 and 2,000 ms indicate the subsequent analysis window.
Independent Variables
The primary variables of interest were intelligibility and listener experience. As stated above, the intelligibility scores of the auditory stimuli relate to the mean intelligibility of each token and were obtained from Porretta and Tucker (2015). In that study, mean intelligibility was calculated by taking the average of transcription accuracy; that is, word transcriptions were scored as correct (1) or incorrect (0), and this was averaged by token across respondents1. Listener experience was evaluated using participant responses to the language experience questionnaire, following Porretta et al. (2016). Specifically, the answers to two questions—“On a weekly basis, how often do you interact with non-native speakers of English?” and “What percentage of those interactions include speakers with a Chinese accent?”—were used to calculate a composite score, representing the amount of experience interacting with Chinese-accented speakers on a scale from zero to 100. The measure (0–100, M = 22.08, SD = 27.92) contained a right skew. Therefore, as in Porretta et al. (2016), a log transformation was employed with a constant of one added prior to the transformation to accommodate zero values (0–4.62, M = 2.09, SD = 1.67). As the time course of processing was of critical interest, time (in milliseconds) was included as a covariate. A window from 200 to 2,000 ms was selected for analysis. Two hundred milliseconds is the point at which the pupil can begin to respond to auditory stimuli (see Aston-Jones and Cohen, 2005). Two thousand milliseconds was chosen based on visual inspection of the results and it was deemed sufficiently long to capture the decline of the dilation after the peak while still temporally preceding the response prompt.
Additionally, control variables were included, namely, list (with five levels), trial, log frequency of the item (obtained from the Corpus of Contemporary American English, COCA, Davies, 2008), gaze coordinates, and baseline dilation. While the lists were fully counterbalanced, list was included to control for the possibility of differences among the lists. Trial order was included to control for possible habituation to the task. Log word frequency was included to account for any differences in lexical frequency (see Kuchinke et al., 2007). Gaze coordinates (X and Y positions on the screen) were included to account for changes in recorded pupil size due solely to eye gaze relative to the eye-tracking camera. The baseline dilation of each trial was included to control for differential dilation, as high baseline values restrict the upper limit of dilation during the trial and may be related to decreased phasic response (see Aston-Jones and Cohen, 2005).
Statistical Considerations
To investigate processing load incurred by varying foreign accent intelligibility, baseline-normalized pupil dilation (200–2,000 ms) was modeled using Generalized Additive Mixed Modeling (mgcv, version 1.8–17, Wood, 2018) in R. Generalized Additive Mixed Modeling provides a straightforward and robust framework for modeling possible nonlinear effects along continuous variables while also allowing for the inclusion random-effect structure. This method has been employed in modeling the time course pupillometric data (van Rij, forthcoming; Lõo et al., 2016). The input variables described above were fitted to the response variable with by-subject and by-item factor smooths for time as well as by-event random intercepts. Factor smooths allow for the shape of the average time-course to vary by participant and item. Random intercepts for event (the combination of subject and trial, indexing each unique time-series) allow each unique time-course to have its own intercept in the model. List was included as a parametric component. For log frequency, trial, and baseline value, nonlinear functional relations with the response variable were allowed using smooth functions (Wood, 2006; Baayen, 2010). The interaction of X and Y gaze coordinates was included using an isometric smooth interaction. Intelligibility, time and experience were included as a three-way interaction using a tensor product. Additionally, as autocorrelation in the time-series data can lead to overconfidence of model estimates (Baayen et al., 2018), an AR-1 correlation parameter, ρ = 0.937, estimated from the data, was included.
The model was fit using a backwards step-wise elimination procedure (Zuur et al., 2009). The inclusion of predictors in the model was evaluated using two criteria. The first criterion was the estimated p-value of the smoothing parameter or parametric component. The second criterion was Maximum Likelihood (ML) score comparison of model variants. Through this process, only list was eliminated from the model. This model was refitted for data within ±2.5 standard deviations of the residuals of the model−2.40% removed, 3,524 data points—(see Baayen, 2008).
Results
The final model accounted for 77.80% of deviance explained, indicating that the model was able to capture important facets of variation in pupil dilation over time. The estimated parameters of the final model are found in Table 1. After controlling for frequency of the word, trial, baseline dilation, and gaze coordinates, there was a significant three-way interaction between time, intelligibility, and experience. Here we focus on this interaction, however, the significant effects of the control variables are presented in the Appendix.
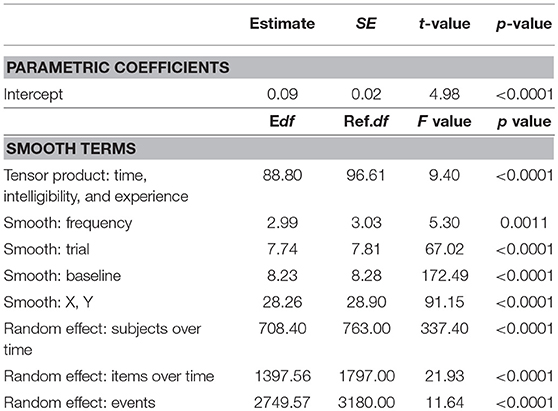
Table 1. Generalized additive mixed model reporting parametric coefficient and Estimated degrees of Freedom (Edf), Reference Degrees of Freedom (Ref.df), F, and p-Values for the Tensor Product, Smooth Terms, and Random Effects for the Final Model.
Figure 2 displays the effect of the three-way interaction on pupil dilation. In order to best characterize the central aspects of this interaction for the purposes of the present study, it is presented as a multi-panel plot showing the contour surfaces of predicted pupil dilation across time and intelligibility at four values of experience, namely the minimum, first tercile, second tercile, and maximum. In the visualization, dark blue/purple indicate smaller dilation while yellow/white indicate larger dilation. The contour lines represent the model-predicted pupil dilation values. Readily noticeable in all four plots is that, as time progressed, pupil dilation increased. Two important aspects of the dilation are also visible in each plot. First, as intelligibility decreased, pupil dilation increased. For example, looking between 1,000 and 2,000 ms in each plot, a clear increase in dilation occurred as the intelligibility of the stimulus decreased. Second, the duration of the dilation tended to persist longer for low intelligibility stimuli. Following the full time-course for high intelligibility items (near 1 on the y-axis), the dilation rises and then falls, as indicated by the values on the contour lines which increase and then decrease as time progresses. By comparison, the dilation rises and stays large for the time-course for low intelligibility items (near 0 in the y-axis).
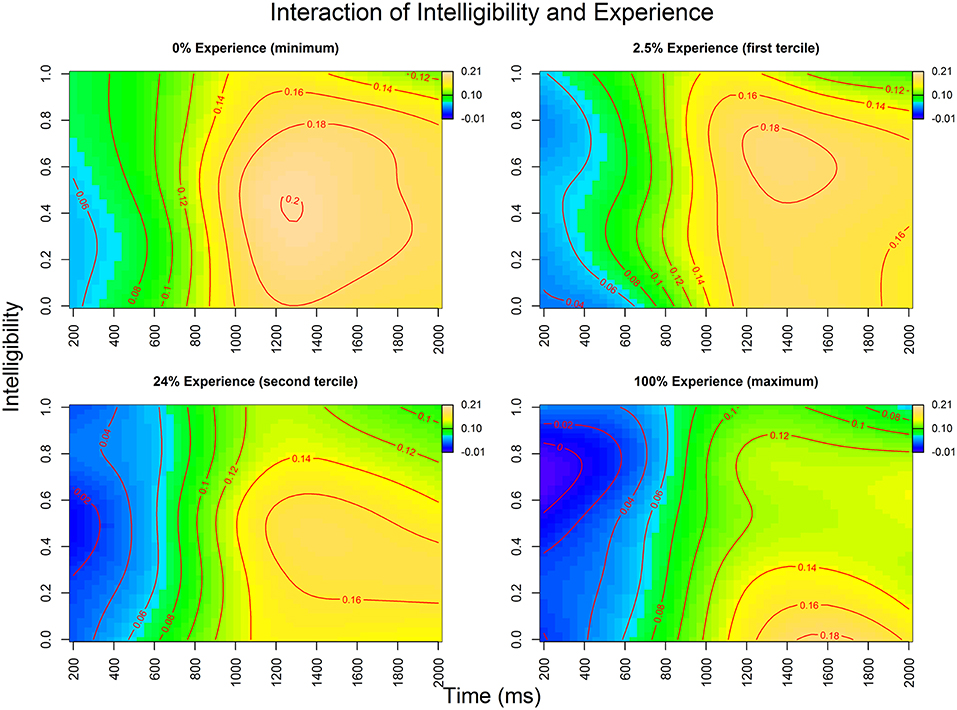
Figure 2. Contour plots of the interaction between intelligibility and time at different values of experience. The plots presented represent experience at the minimum value, the first tercile, the second tercile, and the maximum value. Dark blue/purple indicate smaller dilation while yellow/white indicate larger dilation. The contour lines represent pupil dilation values predicted by the model.
Also noticeable is that the patterns of dilation related to intelligibility were not uniform across the values of listener experience. Compare, for example, the minimum and maximum experience plots after 1,000 ms. The shape of the effect appears very different, particularly along intelligibility. In the minimum experience plot, this increase in pupil dilation began to increase as soon as intelligibility began to drop. However, in the maximum experience plot, the increase in pupil dilation was slight until intelligibility was at its lowest values. Examining the plots for experience values in between the two extremes, this effect was gradual, with the threshold for the increase of pupil dilation slowly shifting down the intelligibility continuum.
To examine more closely aspects of this three-way interaction we present Figures 3, 4. Each makes post-hoc comparisons based on predicted values from the model indicating differences between curves presented with 95% confidence intervals. The difference between the estimated curves is statistically significant when the curves do not lie within each other's confidence intervals. Figure 3 presents the effect of intelligibility by experience. Specifically, it presents the difference between minimum and maximum experience at three values of intelligibility, namely, zero, 0.5, and one. As can be seen in the middle panel (when intelligibility is 0.5), the difference is significant from ~1,000–1,400 ms, i.e., the span of time capturing the average peak dilation of the data. Figure 4 presents the effect of experience by intelligibility. That is, it presents the difference between items that were fully intelligible (i.e., when intelligibility is one) and completely unintelligible (i.e., when intelligibility is zero) for both minimum experience listeners and maximum experience listeners. In the maximum experience panel, a significant difference arose starting at ~1400 ms. Taken together, the results indicate that the shape of the time-course of pupil dilation depends both on an item's overall intelligibility and the experience of the listener.
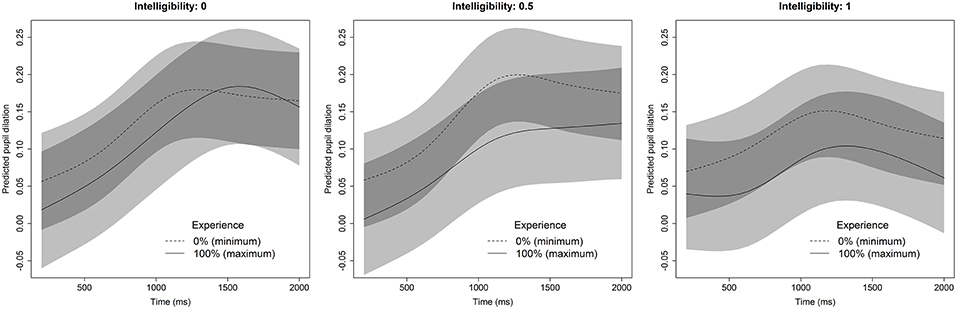
Figure 3. Time-course plots of pupil dilation values predicted by the model at three values of intelligibility (0, 0.5, and 1), each comparing minimum experience and maximum experience. Shaded bands indicate 95% confidence intervals.
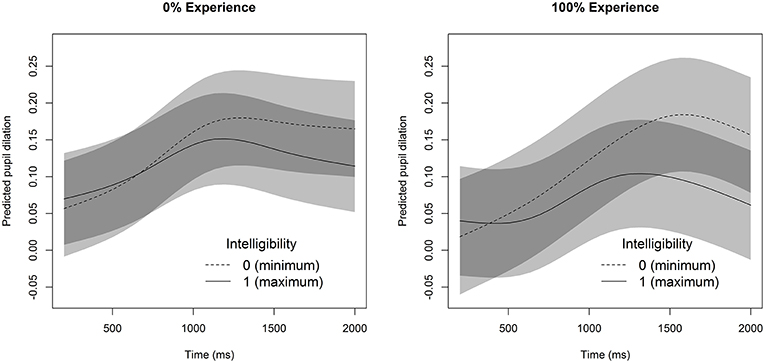
Figure 4. Time-course plots of pupil dilation values predicted by the model for minimum experience (left panel) and maximum experience (right panel), each comparing completely unintelligible and fully intelligible stimuli. Shaded bands indicate 95% confidence intervals.
Discussion
The present study examined the pupillary response elicited during the comprehension of spoken words that varied in intelligibility due to foreign accentedness. Based on work examining speech in noise (see Zekveld et al., 2010; Kramer et al., 2013; Zekveld and Kramer, 2014), we predicted that as intelligibility decreases, processing load, as indexed by pupil dilation would increase. Additionally, we asked whether individual differences—in listener experience interacting with speakers with a Chinese accent—would modulate any effect of intelligibility on pupil dilation. Given previous work showing the influence of listener experience on other measures of online processing (see Witteman et al., 2013; Porretta et al., 2016, 2017), we predicted that greater reported accent experience would modulate the effect of intelligibility.
The analysis of the pupil dilation data indicated that as foreign-accent related intelligibility began to fall, pupil dilation increased in a gradual pattern. This indicates that the processing load related to listening effort increased as it became more difficult to understand non-native talkers. Importantly, this mirrors the results obtained for speech in noise. Zekveld and Kramer (2014) found a similar effect in which pupil dilation increases as more noise was added to the speech signal. Zekveld and Kramer (2014) anticipated reduced dilation for speech in the greatest amount of noise, based on previous research (Granholm et al., 1996) suggesting that in conditions of cognitive overload, the pupil would not respond. This was not the case in Zekveld and Kramer's study; nor was it the case here. Instead, it seems that the listeners always attempt to process the speech regardless of difficulty.
Additionally, the present results suggest that the duration of the dilation also increases as intelligibility falls. Therefore, not only does the magnitude of listening effort increase, but this effort appears to be sustained in time as speech is more difficult to understand. It should be noted that Zekveld and Kramer (2014) did not test the duration the pupillary response; however, based on the plot of data they provided, it appears this would also be the case for speech in noise. The sustained effort observed here may be related to the process of eliminating competing lexical items during spoken word recognition. During spoken word recognition, multiple possible candidates for the ultimate identity of the word become activated. As more auditory information becomes available, the number of candidates becomes smaller until one of the candidates is selected. If stronger and more diffuse competition occurs in the presence of a stronger accent, this competitive process will take longer to be resolved. Porretta and Kyröläinen (2019) found that the duration of lexical competition was positively correlated with the strength of foreign accent, and a similar effect was found for speech in noise (Brouwer and Bradlow, 2016). So, this increase in the duration of cognitive load may be due to underlying processes, such as effects associated with lexical competition.
Similarly, Tamási et al. (2017) established that pupil dilation can be used as a measure of mispronunciation detection in small children. Mispronunciations elicited greater dilation, and this increase was a function of how distant the mispronunciation was from the correct form based on phonological distance. The foreign accentedness of non-native speech can be broadly thought of as the global effect of mispronunciation, which is gradient at the acoustic level (Porretta et al., 2015). Additionally, accentedness and intelligibility are inherently correlated—at least partially (see Munro and Derwing, 1995b; Porretta and Tucker, 2015). As accentedness increases, intelligibility tends to fall. Therefore, the present results also speak to pupil dilation as a measure of the processing load induced by foreign accent at large, with results in the same direction of as those of Tamási et al. (2017) who examined native speaker mispronunciations.
Previous research has shown that individual differences in listener experience influence the processing of foreign-accented speech (see Witteman et al., 2013; Porretta et al., 2016, 2017). Specifically, greater amounts of accent experience facilitates processing of non-native speech. Using the same measure of listener experience interacting with Chinese-accented speakers, the present results also indicate that individual differences influenced pupil dilation when listening to Chinese-accented speech. Listeners with more experience interacting with Chinese-accented speakers displayed reduced dilation overall. Further, high experience listeners reacted differently to decreased intelligibility; the threshold at which reduced intelligibility elicited greater dilation shifted such that these listeners better tolerated tokens with lower intelligibility.
This pattern suggests that accent-specific experience allows listeners to put forth less effort during listening. People who interact more with non-native speakers do not appear to simply put in more effort to understand; instead, it seems that experience leads to reduced effort in the long run. This is further highlighted by differences across experience early in time, which may relate to lower baseline dilation. As pointed out by Laeng et al. (2012), tonic (baseline) dilation relates to task difficulty, mental effort, and state of arousal or vigilance. These early differences suggest that low experience listeners might exert more attentional resources in order to remain engaged with the task. Thus, prior experience with the accent reduces processing load and processing duration, suggesting that listeners with the most experience have developed a generalized, accent-specific adaptation to variability in intelligibility. This type of generalized adaptation has been reported in offline measures of comprehension (see Bradlow and Bent, 2008). This effect of listener experience fits within the ideal adapter framework proposed by Kleinschmidt and Jaeger (2015). The ideal adapter model is a distributional learning model of incremental adaptation and also accounts for generalization across talkers and groups (e.g., accents), particularly when similarities exist. In this way, the measure of listener experience can be viewed as an index of incremental learning. Lastly, there was an effect of trial order (see Appendix) such that as participants progressed through the experiment, overall pupil dilation decreased. While this was not the focus of this study, the result coincides with behavioral studies (e.g., Clarke and Garrett, 2004) which have shown rapid adaptation to foreign-accented speech.
Zekveld and Kramer (2014) showed that individual differences can influence pupil dilation. Their participants completed an adaptive text reception threshold test as a measure of noise tolerance. In this test, participants are required to read sentences with varying levels of visual masking and an individual's threshold is calculated as the mean percentage of unmasked text required to correctly identify the sentence. Thus, lower thresholds indicated better performance. They found that participants who were better at understanding text presented with a visual mask displayed greater dilation in the low intelligibility condition. They explained this in terms of effort; participants performed better on the text reception because they put in more effort, which was also reflected in their patterns of dilation when listening in very difficult conditions. Importantly, the present study also demonstrates that individual differences—here, in accent experience—influence the pattern of pupil dilation. Specifically, the current results show that the accumulation of accent experience, as measured by self-reported amount of interaction with speakers with a Chinese accent, results in reduced listening effort. This difference is particularly stark in the mid-range of intelligibility (Figure 3). It should be noted that the current study was not meant to make a direct comparison with Zekveld and Kramer (2014) with regard to individual differences, given the fundamental differences between the measures. Therefore, we cannot directly compare the results related to the text reception threshold and those related to accent experience. What can be said is that individual differences in linguistic experience also modulate pupillary response for foreign-accented speech. However, further research is required to better understand how various measures of individual differences influence listening effort.
Accented speech has been compared to speech presented in noise and Van Engen and Peelle (2014) suggest that accented speech should be included with noise within the framework of listening effort. This is reasonable as noise (both energetic and informational) and foreign accent are naturally occurring forms of variability that listeners must handle during everyday communication. While research within the area of speech in noise has demonstrated dissociable effects between energetic masking and informational masking (see Garcia Lecumberri and Cooke, 2006), when comparing speech in noise (particularly energetic masking) and accented speech, there are notable similarities. Both speech in noise and accented speech have been shown to increase lexical competition (see Brouwer and Bradlow, 2016, for speech in noise, and Porretta and Kyröläinen, 2019, for accented speech). Further, the two have demonstrated increased listening effort (see Zekveld and Kramer, 2014, for speech in noise, and the present study for accented speech). Given the similarity in results—especially with regard to pupil dilation—it might seem reasonable to equate noise and foreign accent. However, while they both require increased listening effort, there may be a valuable distinction to be made with regard to the nature of what leads to these difficult listening situations: source-attributable variability vs. context-attributable variability. The perceived variability associated with foreign-accented speech can be attributed to the source of the signal (i.e., the talker), while the perceived variability associated with noise can be attributed to the listening context. Listeners may be sensitive to the distinction between these two types of variability.
Listeners make use of all available information when determining if variability in the signal is relevant or not. For example, Kraljic et al. (2008) showed, using a perceptual learning paradigm, that listeners suspend adaptation to variant forms when it is evident (based on visual information, e.g., seeing a pen in the speaker's mouth) that the variability in production is not due to a characteristic inherent to the speaker. Thus, if variability is relevant, learned statistical regularities can be used to track and later harness the regularities present within that variability (see Idemaru and Holt, 2011). Accented speech (both regional and non-native) is arguably more likely to contain regularities than various types of noise. These learned regularities likely lead to the effect of listener experience seen in the present data (see Kleinschmidt and Jaeger, 2015 for discussion related to adaptation to accents). However, further work is required to have a more fine-grained understanding of how differences and similarities between these two types of variability may affect listening effort, including a direct comparison between the subtypes of accent (regional and non-native) and noise (energetic and informational).
Finally, we would like to comment on the use of pupillometry for the investigation of foreign-accented speech and spoken language processing in general. While the measurement of pupil dilation has been used to investigate various aspects of spoken language, this study represents the first application of the method to the examination of non-native variability (Van Engen and McLaughlin, 2018). Here we focused broadly on the influence of foreign accent intelligibility for comparability with existing literature. However, foreign-accented speech encompasses many different aspects of language such as phonetic, phonotactic, phonological, lexical, and suprasegmental level (Cristia et al., 2012), which affect broader constructs like intelligibility, accentedness, and comprehensibility (see Munro and Derwing, 1995a). Pupillometry now affords researchers the possibility to investigate specific questions regarding the influence of these aspects of foreign-accented speech on the magnitude and duration of processing. Additionally, because the measurement of pupil dilation does not require an overt task or behavioral response, it can be used to investigate spoken language processing in a variety of populations (Laeng et al., 2012), including pre-verbal children or elderly adults who would have difficult performing a specific task. While there are some studies that have examined the processing of foreign-accented speech across the life span (see Cristia et al., 2012), pupillometry provides an established, non-invasive method for studying the developmental trajectory of the processing of accented speech across age groups ranging from toddlers to the elderly.
Conclusion
In summary, this study demonstrated that pupillometry can be used as an informative research tool for investigating the processing of foreign-accented speech, as suggested by Van Engen and McLaughlin (2018). Specifically, listening effort increases as intelligibility decreases due to foreign accentedness and that this effect is mitigated by prior experience with the accent in question. These results align with previous research and provide new insight into the processing of the naturalistic variability listeners encounter during daily life. The difficulty listeners experience when comprehending foreign-accented speech, which is commonly observed in behavioral response times, lies in part with the underlying effort required to overcome the cognitive load of decoding non-native speech, as well as the amount of time that effort is exerted. However, listeners who actively engage with non-native speakers on a regular basis appear to benefit in the long-run in terms of required listening effort.
Author Contributions
VP and BT jointly envisioned the project. VP was responsible for data collection and statistical analysis, and wrote a first draft of the paper. Subsequently, both authors worked on refining and revising the text. All authors approved the final version.
Conflict of Interest Statement
The authors declare that the research was conducted in the absence of any commercial or financial relationships that could be construed as a potential conflict of interest.
Footnotes
1. ^The verbal responses from the participants in the present study were coded for accuracy by the first author and averaged by token across participants. These intelligibility scores were highly correlated, r(198) = 0.85, p < 0.001) with those from Porretta and Tucker (2015).
References
Aston-Jones, G., and Cohen, J. D. (2005). An integrative theory of locus coeruleus-norepinephrine function: adaptive gain and optimal performance. Annu. Rev. Neurosci. 28, 403–450. doi: 10.1146/annurev.neuro.28.061604.135709
Baayen, R. H. (2008). Analyzing Linguistic Data: A Practical Introduction to Statistics Using R. Cambridge: Cambridge University Press.
Baayen, R. H. (2010). “The directed compound graph of English. An exploration of lexical connectivity and its processing consequences,” in New impulses in word-formation, (Linguistische Berichte Sonderheft 17) eds S. Olson (Hamburg: Buske), 383–402.
Baayen, R. H., van Rij, J., Cecile, D., and Wood, S. N. (2018). “Autocorrelated errors in experimental data in the language sciences: Some solutions offered by Generalized Additive Mixed Models,” in Mixed effects regression models in linguistics, eds D. Speelman, K. Heylan, and D. Geeraerts (Berlin: Springer), 49–69.
Beatty, J. (1982). Task-evoked pupillary responses, processing load, and the structure of processing resources. Psychol. Bull. 91, 276–292. doi: 10.1037/0033-2909.91.2.276
Beatty, J., and Lucero-Wagoner, B. (2000). “The pupillary system,” in Handbook of psychophysiology, eds J. T. Cacioppo, L. G. Tassinary, and G. Berntson (Cambridge, MA: Cambridge University Press), 142–162.
Bradlow, A. R., and Bent, T. (2008). Perceptual adaptation to non-native speech. Cognition 106, 707–729. doi: 10.1016/j.cognition.2007.04.005
Brouwer, S., and Bradlow, A. R. (2016). The temporal dynamics of spoken word recognition in adverse listening conditions. J. Psycholinguist. Res. 45, 1151–1160. doi: 10.1007/s10936-015-9396-9
Clarke, C. M., and Garrett, M. F. (2004). Rapid adaptation to foreign-accented English. J. Acous. Soc. Am. 116, 3647–3658. doi: 10.1121/1.1815131
Cristia, A., Seidl, A., Vaughn, C., Schmale, R., Bradlow, A. R., and Floccia, C. (2012). Linguistic processing of accented speech across the lifespan. Front. Psychol. 3, 1–15. doi: 10.3389/fpsyg.2012.00479
Davies, M. (2008). The Corpus of Contemporary American English (COCA): 520+ million words, 1990–present. Available online at: http://www.americancorpus.org
Engelhardt, P. E., Ferreira, F., and Patsenko, E. G. (2010). Pupillometry reveals processing load during spoken language comprehension. Q. J. Exp. Psychol. 63, 639–645. doi: 10.1080/17470210903469864
Fitzgerald, H. E. (1968). Autonomic pupillary reflex activity during early infancy and its relation to social and nonsocial visual stimuli. J. Exp. Child Psychol. 6, 470–482. doi: 10.1016/0022-0965(68)90127-6
Floccia, C., Butler, J., Goslin, J., and Ellis, L. (2009). Regional and foreign accent processing in English: can listeners adapt? J. Psycholinguist. Res. 38, 379–412. doi: 10.1007/s10936-008-9097-8
Garcia Lecumberri, M., and Cooke, M. (2006). Effect of masker type on native and non-native consonant perception in noise. J. Acoust. Soc. Am. 119, 2445–2454. doi: 10.1121/1.2180210
Goslin, J., Duffy, H., and Floccia, C. (2012). An ERP investigation of regional and foreign accent processing. Brain Lang. 122, 92–102. doi: 10.1016/j.bandl.2012.04.017
Granholm, E., Asarnow, R. F., Sarkin, A. J., and Dykes, K. L. (1996). Pupillary responses index cognitive resource limitations. Psychophysiology 33, 457–461. doi: 10.1111/j.1469-8986.1996.tb01071.x
Hanulíková, A., van Alphen, P. M., van Goch, M. M., and Weber, A. (2012). When one person's mistake is another's standard usage: the effect of foreign accent on syntactic processing. J. Cogn. Neurosci. 24, 878–887. doi: 10.1162/jocn_a_00103
Hess, E. H., and Polt, J. M. (1960). Pupil size as related to interest value of visual stimuli. Science 132, 349–350. doi: 10.1126/science.132.3423.349
Hess, E. H., Seltzer, A. L., and Shlien, J. M. (1965). Pupil response of hetero- and homosexual males to pictures of men and women: a pilot study. J. Abnorm. Psychol. 70, 165–168. doi: 10.1037/h0021978
Idemaru, K., and Holt, L. L. (2011). Word recognition reflects dimension-based statistical learning. J. Exp. Psychol. Hum. Percept. Perform. 37, 1939–1956. doi: 10.1037/a0025641
Kafkas, A., and Montaldi, D. (2012). Familiarity and recollection produce distinct eye movement, pupil and medial temporal lobe responses when memory strength is matched. Neuropsychologia 50, 3080–3093. doi: 10.1016/j.neuropsychologia.2012.08.001
Kahneman, D., and Beatty, J. (1966). Pupil diameter and load on memory. Science 154, 1583–1585. doi: 10.1126/science.154.3756.1583
Kleinschmidt, D. F., and Jaeger, T. F. (2015). Robust speech perception: recognize the familiar, generalize to the similar, and adapt to the novel. Psychol. Rev. 122, 148–203. doi: 10.1037/a0038695
Klingner, J., Tversky, B., and Hanrahan, P. (2011). Effects of visual and verbal presentation on cognitive load in vigilance, memory, and arithmetic tasks. Psychophysiology 48, 323–332. doi: 10.1111/j.1469-8986.2010.01069.x
Kraljic, T., Samuel, A. G., and Brennan, S. E. (2008). First impressions and last resorts: how listeners adjust to speaker variability. Psychol. Sci. 19, 332–338. doi: 10.1111/j.1467-9280.2008.02090.x
Kramer, S. E., Lorens, A., Coninx, F., Zekveld, A. A., Piotrowska, A., and Skarzynski, H. (2013). Processing load during listening: the influence of task characteristics on the pupil response. Lang. Cogn. Process. 28, 426–442. doi: 10.1080/01690965.2011.642267
Kuchinke, L., Võ, M. L.-H., Hofmann, M., and Jacobs, A. M. (2007). Pupillary responses during lexical decisions vary with word frequency but not emotional valence. Int. J. Psychophysiol. 65, 132–140. doi: 10.1016/j.ijpsycho.2007.04.004
Laeng, B., Sirois, S., and Gredebäck, G. (2012). Pupillometry: a window to the preconscious? Perspect. Psychol. Sci. 7, 18–27. doi: 10.1177/1745691611427305
Lõo, K., van Rij, J., Järvikivi, J., and Baayen, R. H. (2016). “Individual differences in pupil dilation during naming task,” in Proceedings of the 38th Annual Conference of the Cognitive Science Society eds A. Papafragou, D. Grodner, D. Mirman, J. C. Trueswell (Austin, TX: Cognitive Science Society), 550–555.
Mathôt, S. (2018). Pupillometry: psychology, physiology, and function. J. Cogn. 1:16. doi: 10.5334/joc.18
Munro, M. J., and Derwing, T. M. (1995a). Foreign accent, comprehensibility, and intelligibility in the speech of second language learners. Lang. Learn. 45, 73–97. doi: 10.1111/j.1467-1770.1995.tb00963.x
Munro, M. J., and Derwing, T. M. (1995b). Processing time, accent, and comprehensibility in the perception of native and foreign-accented speech. Lang. Speech 38, 289–306. doi: 10.1177/002383099503800305
Pichora-Fuller, M. K., Kramer, S. E., Eckert, M. A., Edwards, B., Hornsby, B. W. Y., Humes, L. E., et al. (2016). Hearing impairment and cognitive energy. Ear Hear. 37, 5S−27S. doi: 10.1097/AUD.0000000000000312
Porretta, V., and Kyröläinen, A.-J. (2019). Influencing the time and space of lexical competition: the effect of gradient foreign accentedness. J. Exp. Psychol. Learn. Memory Cogn. doi: 10.1037/xlm0000674. [Epub ahead of print].
Porretta, V., Kyröläinen, A.-J., and Tucker, B. V. (2015). Perceived foreign accentedness: acoustic distances and lexical properties. Attent. Percept. Psychophys. 77, 2438–2451. doi: 10.3758/s13414-015-0916-3
Porretta, V., Tremblay, A., and Bolger, P. (2017). Got experience? PMN amplitudes to foreign-accented speech modulated by listener experience. J. Neurolinguist. 44, 54–67. doi: 10.1016/j.jneuroling.2017.03.002
Porretta, V., and Tucker, B. V. (2015). “Intelligibility of foreign-accented words: acoustic distances and gradient foreign accentedness,” in Proceedings of the 18th International Congress of Phonetic Sciences (Glasgow, UK: University of Glasgow), 1–4. Available online at: https://www.internationalphoneticassociation.org/icphs-proceedings/ICPhS2015/Papers/ICPHS0657.pdf
Porretta, V., Tucker, B. V., and Järvikivi, J. (2016). The influence of gradient foreign accentedness and listener experience on word recognition. J. Phon. 58, 1–21. doi: 10.1016/j.wocn.2016.05.006
R Development Core Team (2018). R: A Language and Environment for Statistical Computing. Version 3.5.0. Vienna: R Foundation for Statistical Computing. Available online at: http://www.R-project.org/
Sumby, W. H., and Pollack, I. (1954). Visual contribution to speech intelligibility in noise. J. Acoust. Soc. Am. 26, 212–215. doi: 10.1121/1.1907309
Tamási, K., McKean, C., Gafos, A., Fritzsche, T., and Höhle, B. (2017). Pupillometry registers toddlers' sensitivity to degrees of mispronunciation. J. Exp. Child Psychol. 153, 140–148. doi: 10.1016/j.jecp.2016.07.014
Van Engen, K. J., Baese-Berk, M., Baker, R. E., Choi, A., Kim, M., and Bradlow, A. R. (2010). The Wildcat corpus of native-and foreign-accented English: communicative efficiency across conversational dyads with varying language alignment profiles. Lang. Speech 53, 510–540. doi: 10.1177/0023830910372495
Van Engen, K. J., and McLaughlin, D. J. (2018). Eyes and ears: using eye tracking and pupillometry to understand challenges to speech recognition. Hear. Res. 369, 56–66. doi: 10.1016/j.heares.2018.04.013
Van Engen, K. J., and Peelle, J. E. (2014). Listening effort and accented speech. Front. Hum. Neurosci. 8, 1–4. doi: 10.3389/fnhum.2014.00577
van Rij, J., Hendriks, P., van Rijn, H., Baayen, R. H., and Wood, S. N (forthcoming). Analyzing the time course of pupillometric data. Trends in Hearing.
Winn, M. B., Wendt, D., Koelewijn, T., and Kuchinsky, S. E. (2018). Best practices and advice for using pupillometry to measure listening effort: an introduction for those who want to get started. Trends Hear. 22:233121651880086. doi: 10.1177/2331216518800869
Witteman, M. J., Weber, A., and McQueen, J. M. (2013). Foreign accent strength and listener familiarity with an accent codetermine speed of perceptual adaptation. Attent. Percept. Psychophys. 75, 537–556. doi: 10.3758/s13414-012-0404-y
Wood, S. N. (2006). Generalized Additive Models: An Introduction With R, 2nd edn. Boca Raton, Fl: Chapman Hall/CRC Press. doi: 10.1201/9781420010404
Wood, S. N. (2018). mgcv: Mixed GAM Computation Vehicle with GCV/AIC/REML Smoothness Estimation. R Package Version 1.8-12. Available online at: https://CRAN.R-project.org/package=mgcv
Zekveld, A. A., and Kramer, S. E. (2014). Cognitive processing load across a wide range of listening conditions: insights from pupillometry. Psychophysiology 51, 277–284. doi: 10.1111/psyp.12151
Zekveld, A. A., Kramer, S. E., and Festen, J. M. (2010). Pupil response as an indication of effortful listening: the influence of sentence intelligibility. Ear Hear. 31, 480–490. doi: 10.1097/AUD.0b013e3181d4f251
Zuur, A. F., Ieno, E. N., Walker, N. J., Saveliev, A. A., and Smith, G. M. (2009). Mixed Effects Models and Extensions in Ecology with R. New York, NY: Springer.
Appendix
In the analysis of the pupil dilation data, various control predictors were included in the model. The primary purpose for their inclusion was to statistically control for their influence on pupil dilation and thus were not the focus of this study. However, because they were statistically significant predictors in the model, we report the effects here. The effects are visualized in Figure A1.
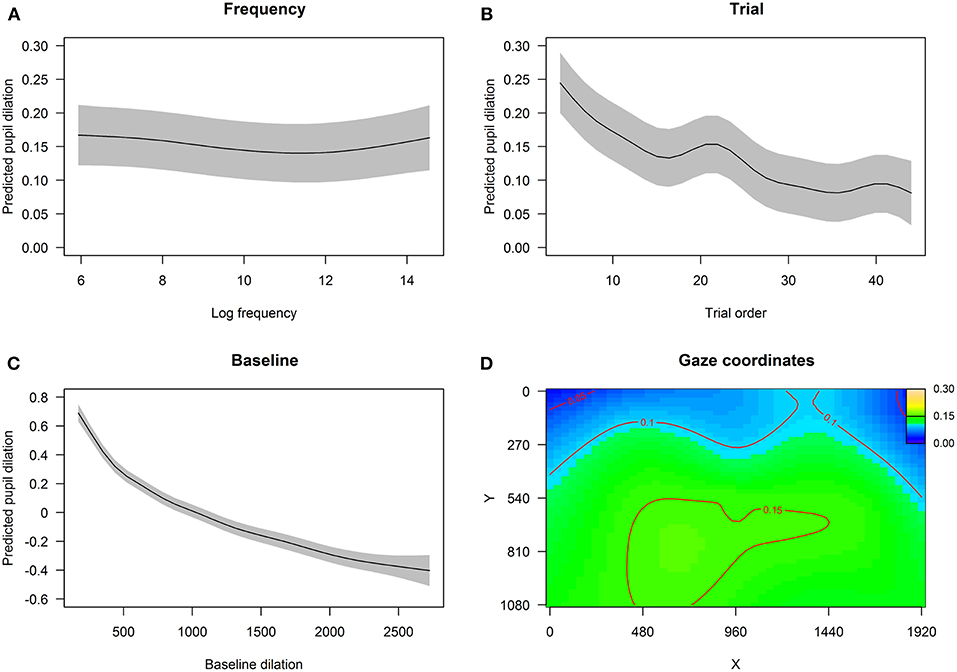
Figure 1A. Visualization of the effect of control variables on pupil dilation values predicted by the model: (A) Log word frequency, (B) Trial order, (C) Baseline dilation, (D) Gaze coordinates (X and Y). Note that the scale in (C) is different due to the size of the effect.
Figure A1A presents the main effect of log word frequency. While the effect is relatively weak, pupil dilation was greater for words with lower lexical frequency. This generally coincides with the results of Kuchinke et al. (2007). Figure A1B presents the main effect of trial order. As participants progressed through the experiment, pupil dilation decreased. This would be expected if participants habituated to the demands of the task. Figure A1C presents the main effect of baseline dilation. Baseline pupil size had an inverse relationship with pupil dilation for a given trial; that is, pupil dilation increased more when the baseline was low. This would generally be expected as the pupil cannot increase without limit and coincides with studies that indicate that increased tonic (baseline) dilation may reduce phasic dilation response (Aston-Jones and Cohen, 2005). Figure A1D presents the interaction gaze coordinates X and Y. The interaction indicates that recorded pupil dilation changed depending on gaze location on the screen. This is to be expected; when the eye moves relative to the eye-tracking camera, the recorded size of the pupil changes, even if the pupil itself does not change in dilation.
Keywords: foreign-accented speech, intelligibility, pupillometry, listening effort, cognitive load
Citation: Porretta V and Tucker BV (2019) Eyes Wide Open: Pupillary Response to a Foreign Accent Varying in Intelligibility. Front. Commun. 4:8. doi: 10.3389/fcomm.2019.00008
Received: 31 October 2018; Accepted: 04 February 2019;
Published: 22 February 2019.
Edited by:
Eva Kehayia, McGill University, CanadaReviewed by:
Karen Mulak, University of Maryland, College Park, United StatesAlba Tuninetti, Western Sydney University, Australia
Copyright © 2019 Porretta and Tucker. This is an open-access article distributed under the terms of the Creative Commons Attribution License (CC BY). The use, distribution or reproduction in other forums is permitted, provided the original author(s) and the copyright owner(s) are credited and that the original publication in this journal is cited, in accordance with accepted academic practice. No use, distribution or reproduction is permitted which does not comply with these terms.
*Correspondence: Vincent Porretta, porretta@uwindsor.ca