- 1Department of Computational and Data Sciences, George Mason University, Fairfax, VA, United States
- 2Department of Computer Science, Engineering, and Physics, University of Michigan-Flint, Flint, MI, United States
Attribution of responsibility and blame are important topics in political science especially as individuals tend to think of political issues in terms of questions of responsibility, and as blame carries far more weight in voting behavior than that of credit. However, surprisingly, there is a paucity of studies on the attribution of responsibility and blame in the field of disaster research.
In this work, we investigate the attribution of responsibility and blame through social media in the case of Flint water crisis. We form hypotheses based on social scientific theories in disaster research and then operationalize them on public responses available on social media rather than employing traditional data collection methods such as interviewing and surveying. In particular, we investigate the source for blame, the partisan predisposition, the concerned geographies, and the contagion of complaining by testing our hypotheses on data collected from Twitter.
Our results demonstrate the utility of social media data in testing those hypotheses, which are rooted in sociology of disasters. Our findings are not only aligned with official reports listing the responsible officials for the source blame, but also reveal a partisan predisposition in regards to Democratic and Republican stances. We also confirm that closer geographies are more concerned and complaining seems contagious in social media conversations.
This paper adds to the sociology of disasters research by exploiting a new, rarely used data source (the social web), and by employing new computational methods (such as sentiment analysis and retrospective cohort study design) on this new form of data. In this regard, this work can be seen as the first step toward drawing more challenging inferences on the sociology of disasters from “big social data”.
1. Introduction
Although altruistic behaviors are common in times of disasters (Glasgow et al., 2016), in the recovery phase, when the community is pressed by difficult living conditions and when there is a lack of short-term improvement, public increase their criticism of administrators, and attribute blame to whom they perceive as the agents of responsibility. Among other psychological and social reasons, a political explanation for this act is that in democracies the public acts as a watchdog and actively participates in discussions to control and influence the decision makers. To this end, use of Twitter hashtags in citizen protests has already become a common apparatus the public leverages to grab attention to their concerns (Feenstra and Casero-Ripollés, 2014). This online activism, sometimes called slacktivism, is defined as “as low-risk, low-cost activity via social media, whose purpose is to raise awareness, produce change, or grant satisfaction to the person engaged in the activity” (Rotman et al., 2011).
Attribution of responsibility is a key issue in political decision making as blame carries far more weight in voting behavior than that of credit (Lau, 1985). Moreover, “individuals tend to simplify political issues by reducing them to questions of responsibility and their issue opinions flow from their answers to these questions” (Iyengar, 1994). Besides, attributions formed during states of national emergencies are of particular importance, especially because these attributions become shared memories of the entire nation and are long used as concrete examples of severity of consequences of wrong policy decisions.
Complaint, another concept related to disasters, is defined as “an expression of dissatisfaction for the purpose of drawing attention to a perceived misconduct by an organization and for achieving personal or collective goals” and these goals could be personal like “anxiety reduction, vengeance, advice seeking, self-enhancement” or they could be collective such as “helping others and the organization” (Einwiller and Steilen, 2015). While Einwiller and Steilen (2015) study how large companies (“the blamed”) handle complaints on their social media pages, in this study we are interested in the ways citizens (“the blamers”) raise their voice against the agents in the government as a response to the violation of a basic human right, access to clean city water.
Information and communication technologies can be used to address social scientific inquiries of disaster research and we call this field as computational disaster research. Computational Disaster research is a field in which disaster related data is collected and/or analyzed computationally to address traditional social science inquiries (i.e., problems of social psychology, anthropology, sociology, economics, and political science) at every phase of disaster events. A typical design for computational disaster research is as follows: Big crisis data containing various kinds of information are collected mainly from online sources such as news reports and social media platforms; these new forms of data guide formation of hypotheses, which in the first place are built upon the findings of traditional social science disaster research; these hypotheses are then operationalized by identifying useful information in the data, and by finding ways to represent and integrate them into the models; these quantitative or computational models are then calibrated and re-run; finally, as in all scientific work, the limitations and generalizability as well as implications of the findings are discussed.
There are several notable computational disaster research that use social media to infer responses to terror attacks and disasters. Lin and Margolin (2014) examined inter-communal emotions and expressions during the 2013 Boston bombings and found that the extent to which residents of a city visit the affected-city has the most predictive power for the level of fear, sympathy and solidarity in that city. Similarly, Wen and Lin (2016) studied the factors (geographic proximity, media exposure, social support and gender) of distress (anxiety, sadness, and anger) after the 2015 Paris terror attacks, and compared the immediate acute responses and the ones before the attacks. Glasgow et al. (2016) compared the expressions of gratitude for social support received after 2011 Alabama tornado and 2012 Sandy Hook school shooting (Newtown, CT), and found that there were proportionally fewer expressions of gratitude for support received in Alabama although community “quantitatively” suffered more.
In this study, we examine theories of attribution of responsibility and blame in the recovery phase of a crisis using observational data from social media in the case of Flint water crisis. Namely, we take a similar approach to aforementioned computational studies by employing keyword search and sentiment analysis (Hutto and Gilbert, 2014). However, we are focused on the public responses in terms attribution of blame and responsibility rather than physical protests or how social support is expressed online. Another difference of our work is the phase of disaster we are studying. Instead of studying emotions right after a terrorist attack (i.e., during the response phase), our study focuses on political responsibility attribution later in the recovery phase in which attribution of blame and responsibility usually take place. Finally, instead of trying to solve a software engineering or a disaster management problem, here we study the sociology of disasters from a computational social science perspective. In order to achieve this, we first construct theoretical hypotheses on top of existing social theories, and then operationalize them on unobtrusive, observational social media data via computational methods.
2. Related Work
In the last decade of disaster research, there has been a proliferation of studies exploiting information and communication technologies (ICT) for advancing emergency response (Alexander, 2013). While some attempts were specifically focused on software development for social networking in crisis situations (Plotnick et al., 2009; Reuter et al., 2012), there have been efforts reporting the successful use of social media by the public for disaster response and risk reduction in disasters such as tsunami (Hjorth and Kim, 2011) and flash-flooding (Bird et al., 2012). In addition to its self-reporting features, social media has been utilized as a monitoring tool (Cheong and Lee, 2010) and checked against disinformation (Ratkiewicz et al., 2011).
We can list social network analysis, geospatial analysis, online crowdsourcing and field experiments, agent-based simulations, and informational retrieval and data analysis as the common methods under computational disaster research.
2.1. Social Networks
Approaches to disaster network research could be classified into as socio-centric (mostly about inter-organizations) and ego-centric (mostly about individuals or intra-organization). One major topic in disaster research is mobilization for social support, the process through which people decide to whom in their network to turn when they need help (material, informational, or emotional) (Small and Sukhu, 2016; Faas and Jones, 2017). In this regard, some studies have differentiated the types of support provided by kin and non-kin in different disaster contexts (e.g., Shavit et al., 1994; Hurlbert et al., 2000; Casagrande et al., 2015). For example, it has been argued that while the former provided immediate aid in life threatening situations and in long-term recovery, the latter provided emotional support during preparedness and short-term recovery (Casagrande et al., 2015). Risk perception is another disaster related topic that social networks play an important role. While some researchers have examined networks of emergency organizations during disasters (e.g., Kapucu, 2006, others focused on networks of victims (e.g., Small and Sukhu, 2016). Kapucu (2006) studied inter-agency communication networks during emergencies. Burger et al. (2017) embedded individuals into multiplex social networks (e.g., with family ties, school ties, and work ties).
2.2. Geospatial Analysis
Availability of new collaboration technologies and geographical data opens up a wealth of applications for geospatial analysis along with the rise of digital humanitarians and crisis mapping (Meier, 2015), in the sense that of much data contributed during disasters has a geographic component in the form of GPS tag tweets or place names associated with Flickr (Panteras et al., 2015), for example. Such social media has been used to assess the extent of earthquakes (Crooks and Wise, 2013) or to assess how people are impacted by disasters (Vieweg et al., 2010). Also advances with Web 2.0 technologies (see Crooks et al., 2014 for a review) has also led to the emergence of volunteered geographical information (VGI, Goodchild, 2011) which allow for people to act as sensors. Perhaps the most prominent VGI example is OpenStreetMap. OpenStreetMap has been used to provide detailed and up to data maps after disasters such as the 2010 Haiti Earthquake (Zook et al., 2010), the recent Ebola crisis (Mao, 2015). Using geospatial analysis techniques, computational disaster researchers can look at changes to the physical environment caused by a disaster (e.g., in the form of map edits in OpenStreetMap) or assess the impacted population through their contributions on social media.
2.3. Online Crowdsourcing and Field Experiments
Meier (2015) discusses how digital humanitarians help make sense of big crisis data by crowdsourcing social media messages, satellite and aerial imagery smartly along with the artificial intelligence for disaster response. The emergence of digital humanitarians, their organizational behaviors and interactions are great interest of social scientists. In this regard, Mao (2015) experimented on a realistic crisis mapping task to test the relationship between team size and productivity, a question of broad relevance across many disciplines including economics, psychology, and management science. We should also note that disaster researchers sometimes use crowdsourcing sites for cheap labor. For example, Olteanu et al. (2015) used crowdsourcing to label 1,000 tweets from each of 26 different crisis situations that took place in 2012 and 2013. Using crowdsourcing, they identified six broad categories for information communicated over Twitter during disasters (affected individuals, infrastructure and utilities, donation and volunteers, caution and advice, sympathy and emotional support, and other useful information).
2.4. Agent-Based Simulations
In disaster research literature we find studies addressing social complexity of disasters verbally, mathematically, as well as by designing computer simulations. To highlight the ways new ICT based data sources can be integrated into simulation forts to aid humanitarian response efforts, using crowdsourced data (volunteered geographic information), Crooks and Wise (2013) explored how people react to the distribution of aid, and how rumors relating to aid availability propagate through the population in a spatially explicit agent-based model. To assess the longer-term welfare impacts of urban disasters, (Grinberger et al., 2015; Grinberger and Felsenstein, 2016) made several simulations spanning three years after an earthquake. They simulated the urban dynamics (residential and non-residential capital stock and population dynamics) using both bottom-up (locational choice for workplace, residence and daily activities) and top-down (land use and housing price) protocols. Realistic population synthesis is another important aspect of social simulations of disaster response. In this regard, Burger et al. (2017) proposed an agent-based model that simulates human behavior in the event of a nuclear explosion in a megacity.
2.5. Information Retrieval and Data Analysis
One of the major advantages of working with digital traces is that event time or location has almost no effect in collecting disaster related information. Although there are other issues in the ways disaster related data collected and processed, researchers know that at least some information about disasters are being recorded somewhere (e.g., servers of social media platforms). This help researchers overcome a challenging task in disaster research, unobservability, as Wallace puts it (Wallace, 1956): “An anthropologist can watch or participate in a religious ritual; a sociologist can attend a union meeting; the psychiatrist can see his patient a few hours or minutes after a family quarrel. But disasters, generally speaking, are so unpredictable as to place and time, that it is unlikely that any given team of trained observers will be in an impact area, before and during an impact of the appropriate type.” Palen et al. (2007) also emphasize the advantages of crisis informatics in quick response research. On the other hand, availability of big data may also obscure the most relevant piece of information needed for an accurate conclusion (Spence et al., 2016), which appears to be a major limitation in social media research.
We would like to note two fields which both use big crisis data –but for different purposes: while computational disaster researchers use it as an instrument, crisis informatics researchers see it as a new object of study (Hargittai and Sandvig, 2016). The difference is in the kinds of questions researchers are interested in, i.e., the inquiry of “what can be learned about social behaviors from ICT data” and that of “how to design ICT for better disaster response” serve different purposes.
3. Materials and Methods
3.1. Historical Background
Flint is a postindustrial city with a population of approximately 100,000 people and has been suffering from high unemployment rate and poverty mostly due to the shrinking automotive industry in the region since 1980 (Jacobs, 2009). Therefore, the city has gone through state of financial emergency multiple times. As the city was struggling with the financial problems under the state-appointed emergency management, tap water that has been purchased from Detroit for decades became the most expensive option (Longley, 2011). Then on March 25, 2014 Flint city council approved buying water from Karegnondi Water Authority (KWA) when it becomes active. Upon this decision the “water war” started according to Detroit Water and Sewerage Department (DWSD), and DWSD gave a notice that it would terminate its contract with Flint in one year (Fonger, 2013; Wright, 2013). Flint had to find a temporary primary water source until KWA becomes effective, and by late April 2014, changed its water supply from Detroit-supplied Lake Huron water to the Flint River as a temporary solution. The complaints about the tap water related to its color, taste, and odor reportedly started right after this change (Force, 2016). Bacteria were another issue which further implicitly caused elevation in trihalomethane levels due to disinfection attempts1.
In contrast to the water provided by DWSD, chloride level and chloride-to-sulfate mass ratio were high in Flint River water which had no corrosion inhibitor (Edwards et al., 2015). The city infrastructure was also old and consisting of high percentage of lead pipes (Fonger, 2015) and an analysis of 120 samples from Flint homes showed that water lead level increased (Roy, 2015). This was followed by the reveal of significantly high blood lead levels in children (Hanna-Attisha et al., 2016; Kennedy, 2016) in September 2015 for whom quality of life was poor already2.
On Saturday, January 16, 2016, President Obama declared a federal state of emergency for an area in Michigan affected by contaminated water and authorized the Department of Homeland Security, Federal Emergency Management Agency (FEMA) to “coordinate all disaster relief efforts” (The White House Office of the Press Secretary, 2016). When he later visited Flint, the most adversely affected city in Genesee County, he described the water crisis as “a man-made disaster” that was “avoidable” and “preventable” (Shear and Bosman, 2016), while not naming who in particular were responsible.
According to Flint Water Advisory Task Force (FWATF) (Force, 2016), the following seven entities are responsible for the Flint water crisis at various levels: Michigan Department of Environmental Quality (MDEQ), Michigan Department of Health and Human Services (MDHHS), Michigan Governor's Office, State-appointed emergency managers (EMs), Genesee County Health Department's (GCHD), and U.S. Environmental Protection Agency (EPA).
3.2. Data
We used TweetTracker (Kumar et al., 2011) as our data collection tool, and the data collected starts on the day before the President declared a state of emergency for Flint. In order to have most possible amount of data, we did not restrict ourselves to tweets with geocoded information or that contained a particular hashtag only. Furthermore, to not include irrelevant postings, we filtered the Twitter stream by keywords Flint and #FlintWaterCrisis.
From Jan. 15 to Jun. 29, 2016 (163 days3), we obtained 664,775 tweets by 281,535 unique users. Figure 1 illustrates the activity on Twitter by highlighting some of the major events that draw public's attention4. It appears that the public interest in the Flint water crisis has been limited, and peaked at times of major political events. In this regard, the only day we hit the 50,000 daily tweet collection limit of TweetTracker was the day of the Democratic presidential debate that was held in Flint on March 6. We calculated sentiments of the tweets in our dataset using NLTK implementation of VADER because it is particularly designed for sentiment analysis for social media text5. In the rest of this section, we discuss how we operationalize the theoretical hypotheses put forward in section 3.3.
3.3. Hypotheses
In “An Inventory of Sociological Findings,” Drabek (1986) discusses “blame assignation processes” at the community-level in the disaster reconstruction phase of his typology, in which he lists hypotheses on three topics: (i) when blame occurs, (ii) purposes of blaming and how they work out, and (iii) who those blamers are. Here, we build our hypotheses on top of this existing sociology of disasters research. Drabek also notes the scarcity of studies on blame assignment behavior in disaster research and we hope our research helps reduce this gap of knowledge in the field by forming and testing hypotheses.
3.3.1. Source for Blame
“Animated by a desire for prevention of future occurrences”, blame occurs especially when (i) conventional explanations failed, (ii) when the responsible agents are perceived to be unwilling to take action to remedy the situation, and (iii) when they violate moral standards (Bucher, 1957). All of the conditions are present in the case of Flint water crisis; (i) there is no conventional explanation for this man-made disaster, (ii) almost all of the agents of responsibility were reluctant to respond in time, and (iii) the public was deprived of a basic human right, the right to safe water. Yet, per condition (ii), every primarily responsible officer in the state “somehow payed the price” by leaving their posts, but Governor Snyder6 (Force, 2016). Moreover, both Democratic presidential candidates demanded the governor to resign. Therefore, our first hypothesis goes:
H1. The amount of blame directed toward Governor Snyder exceeds any other agent.
3.3.2. Partisan Predisposition
Blaming an entire party or an ideology upon a particular crisis predisposes him against that party. In disasters, sometimes blame is not seen as “a function of the immediate crisis, but that reflect pre-existing conflicts and hostilities”, and when biased or irrational factors play a role in the process of blaming, it is called “scapegoating” (Singer, 1982) (cited in Drabek, 1986). One can relate this to the social identity theory, which suggests that if someone is guilty then (s)he must be among the out-group (Simon and Klandermans, 2001). Theories on partisan bias project this socio-psychological bias onto the political plane, suggesting that partisanship has an important influence on attitudes toward political elements (Bartels, 2002). So we expect people blaming a particular party or ideology to express more negative sentiments toward representatives of that party. In our case, some of these representatives are Democrat while others are Republican7, and for some, Flint poisoning is primarily a partisan issue (e.g., Krugman, 2016). Hence, our second hypothesis is:
H2a. Individuals who assign responsibility to the Republican party or ideology show greater negative feeling toward the Governor (R) than those who blame Democratic party or ideology.
H2b. Individuals who assign responsibility to the Republican party or ideology express less negative sentiment toward the Mayor (D) than those who blame Democratic party or ideology.
3.3.3. Concerned Geographies
Tobler's first law of geography says “everything is related to everything else, but near things are more related than distant things” (Tobler, 1970). In the case of Flint water crisis, this also relates to environmental vulnerability, suggesting that individuals who are at greater risk are more likely to express their concerns. Flint residents are under the highest threat, followed by the Genesee residents, followed by Michiganders. Therefore we expect:
H3. Expression of concern per capita is to be the highest for the city of Flint, followed by other cities in the Genesee county, followed by other cities and counties in Michigan.
3.3.4. Contagion of Complaining
Twitter is not only used as a social network but also as a news media (Kwak et al., 2010). In the former case, individuals befriend with similar others (homophily) and influence each other (McPherson et al., 2001). When Twitter serves as a news media, we expect ideological similarity between the user and who he follows as selective exposure suggests (Sears and Freedman, 1967). Besides, one who hears a complaint is more likely to start complaining (positive feedback), and Kowalski offers three explanations for this in times of disasters (Kowalski and Western Carolina U, 1996). Accordingly:
H4. Individuals who express negative emotions on the Flint water crisis have friends more negative than that of individuals who talk more positively about the crisis.
3.4. Hypothesis Testing
3.4.1. Source for Blame
Our first hypothesis questions whether most of the blames are directed toward Governor Snyder. To learn if a tweet, or a phrase in a tweet, attributes blame or responsibility to any specific person or a group, we employed manual curation. First, based on the roles of government entities in the Flint water crisis listed in Sec. 3 and from our preliminary observation of our dataset we came up with eight candidates that are likely to be blamed. Then, we randomly selected five chunks of 200 tweets from our original dataset and asked voluntary coders to label every tweet in a chunk with at least one of these predefined labels (candidates). If there is no blame attributed to any specific person or a group in a tweet, then it is labeled no blame. If a person or group is blamed but happens not to be in the candidates list, then those tweets are labeled as other. Multiple labeling was allowed in case a tweet assigns blame to several persons or groups. Curators were instructed not to label a tweet if they are unsure of the person blamed, and to indicate so. Distribution of these eleven cases is captured in Figure 2. To measure inter-rater reliability, each of the samples is created with approximately 10% overlap with any other sample (σ = 19.1, μ = 2.5)8. Then, to operationalize our first hypothesis we simply evaluate the number of tweets coded per category by the curators, for which we calculate a Fleiss' kappa statistic for every possible coder pair. As visualized in the heatmap in Figure 2, most of the rater pairs are in the 0.41–0.60 kappa range, which is interpreted as moderate agreement9 (Landis and Koch, 1977).
3.4.2. Partisan Predisposition
Our second hypothesis is about the relationship between explicitly blamed parties or ideologies and the sentiments expressed toward their representatives at administrative positions. We expect users who blame the Republican (Democratic) party or ideology to have a more negative sentiment toward the Republican governor (Democratic mayor) than those who blame the Democratic (Republican) party or ideology. In our manually coded tweets sample (Figure 2) two of the labels indicate tweets explicitly blaming parties or ideologies. A total of 62 (24) of the 892 labeled tweets found to be blaming Republicans (Democrats) for the crisis. After identifying these tweets, we look for the individuals (Twitter accounts) who (re)tweeted at least one of those tweets. In total, 165 such users are identified in our main dataset, 136 of which blamed the Republicans, and 29 blamed the Democrats.
We used keyword filtering to identify the tweets mentioning the governor of Michigan (G), the mayor of Flint (M), and the emergency managers (EM)10, and then selected tweets exclusively mentioning the mayor or the governor as such: Let Mo: = M\(G∪EM) represent the set of tweets that has only mayor-related tweets. Similarly, Go: = G\(M∪EM) gives the exclusively governor-related tweets. Within each of those sets, we looked at the sentiments of individuals blaming Republicans (R) and Democrats (D) separately. To measure statistical difference between those who blame R and D in their sentiments expressed toward G and M, we performed Kolmogorov-Smirnov test, which is a non-parametric test that does not rely on any probability distribution.
3.4.3. Concerned Geographies
Rather than working with geocoded tweets, which are rarely available for our collection, we make use of the location field in Twitter user profiles, from which we managed to get geographic coordinates using regular expressions. Then to measure cities' level of interest in the Flint water crisis, we normalize total number of tweets originated at each city by its population. For counties, we normalize total tweet counts originated from cities in a county with the square root of sum of city populations. We do square root transformation to account for larger standard deviations at county level11.
3.4.4. Contagion of Complaining
Some Flinters have posted positive messages about the crisis (cohort), while most others have expressed negative sentiments (control). We expect friends of a user in any of these two groups reflect sentiments similar to the user. To test this hypothesis, we designed a retrospective cohort study in which we compared the sentiments of the friends of the cohort group on the Flint water crisis to that of the control group. To rule out the geographic effect, we form both of the groups only by Flinters, the Flinters that have at least three but no more than 20 tweets in our dataset12. We found 223 such Flinters in our dataset (115 of who had a negative and 101 of who had a positive sentiment on average on the Flint water crisis)13. We then performed two-sample Kolmogorov-Smirnov statistical test that rejects the null hypothesis if the two samples (the average sentiments of the friends of each group) were drawn from the same distribution.
3.4.5. Emotional Assortativity
The assortativity measure refers to the correlation of degrees in a network (Newman, 2002) which can infer whether the number of connections for any two nodes play a role in building the relationship. Inspired by this, we extend our analysis for contagion of complaining by investigating the emotional assortativity which we define as the correlation of sentiment values of individuals in a given pair. Namely, we aim to investigate how sadness or negative expressions distributed in the case of Flint Water Crisis in addition to the number of connections. Therefore, we focus on sentiment correlation, rs, as well as degree correlation, rd, over time to further examine our hypothesis.
For the months of January through May of the year 2016, we cumulatively go through the data month-by-month and derive three variations of interactions corresponding to retweets only, replies only, and either method. Each of the aforementioned interactions lead to three networks: retweet-network, reply-network, combined-network (the combination of the former two networks) as illustrated in the first column of Figure 3.
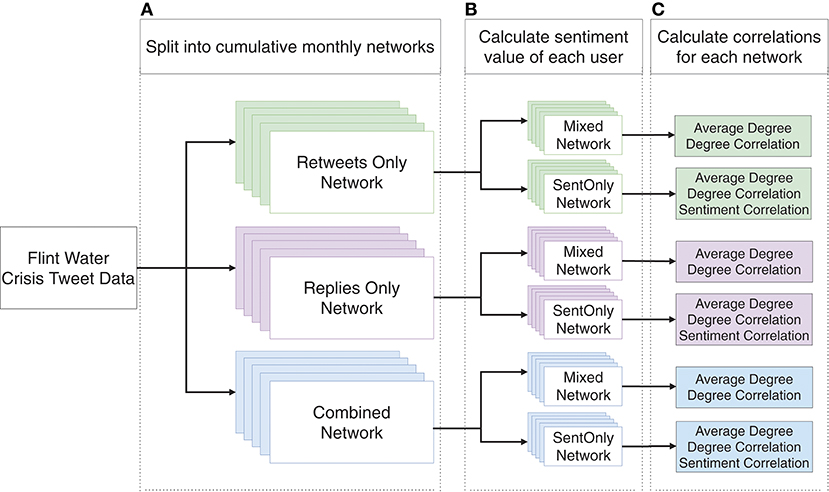
Figure 3. Network creation and analysis steps. (A) Three criteria used to link users, (B) Two types of networks created for each criterion, (C) Network measures applied.
We then calculate the average user sentiment for each user in an individual pair. Some users do not have any sentiment value because they did not express any opinion on the subject but were mentioned in others' tweets –we still keep them in the interaction networks. Because of this discrepancy we create two other variations of those networks: mixed networks and sentiment-only networks as shown in Figure 3. The mixed networks include all pairs, even those with users with no sentiment values while the sentiment-only networks include only pairs with valid average sentiment values for both users in a pair.
For each interaction criterion, we end up with 10 networks over the course of 5 months that include five mixed and five sentiment-only networks. Then we calculate average degrees, degree correlations, and sentiment correlations which eventually account for the nature of interactions in terms of topology and sentiment. Whereas topological measures examine whether the number of interactions of users in a pair play a role, sentiment correlations measure emotional agreement of interacting users. In the latter case, we aim to determine if users have tendency to interact with others who have similar sentiment value.
4. Results
4.1. Source for Blame
Our first hypothesis expected the Governor of Michigan to be the most blamed in the Twitter posts. As shown in Figure 2, he is blamed 3.5 times more than the second most blamed agent.
4.2. Partisan Predisposition
We asked if those who blame the Democratic party or ideology (D) is any different from those who blame the Republican party or ideology (R) in their sentimental expressions toward the governor, and the mayor14. Figure 4 shows that individuals blaming R have more negative sentiment toward the governor than those individuals who blame D. The null hypothesis that the two samples (blaming R and blaming D) are from the same distribution is rejected for the governor by the two-sample Kolmogorov-Smirnov test (for α = 0.001, D = 0.36, p-value = 3.5e−6). Similarly, Figure 4 shows that individuals blaming R have less negative sentiment toward the mayor than those individuals who blame D. However, due to small sample size, we cannot statistically claim any effect of partisan predisposition on the sentiments expressed about the mayor. Thus, our statistical tests support H2a but not H2b.
4.3. Concerned Geographies
We expected that the cities expressed interest in the Flint water crisis the most to be from the county of Genesee and from the state of Michigan. As expected, most concerned cities are found to be from the county of Genesee (four of the top ten) and counties (six of the top ten) are from Michigan (Table 1).
4.4. Contagion of Complaining
The friends of the Flinters who expressed negative sentiments on the Flint water crisis (cohort's friends) are expected to be more negative than the friends of those Flinters who talk positively (control's friends). Figure 5 illustrates that the mean sentiments of the tweets of the cohort's friends are more negative than that of control's friends. Furthermore, the Kolmogorov-Smirnov test statistically shows that the distributions of the sentiment scores of tweets of the two groups' friends are significantly different from each other with 95% confidence level (p-value = 0.017). This discrepancy supports our hypothesis.
4.5. Emotional Assortativity
We have an increasing number of users in the networks throughout the 5-month time course as presented in Table 2. We present a snapshot of degree distributions in Figure 6 for the sentiment-only network that includes replies and retweets (combined). We observe that not only this particular network, but also other cumulative networks show scale free behavior (Supplementary Figure 1) with α and xmin values listed in Table 2.
We also observe an increase in the average degree in each network (Figure 7) until March 2016 in parallel with the network growth. For the following months, we do not observe a drastic change which might be an implication of fewer new interactions for the existing users despite the new individuals sharing tweets over the water crisis. Since sentiment-only networks are subgraphs of mixed networks, we see almost the same pattern in their average degrees.
Degree correlations in Figure 8 have negative values for all possible networks, suggesting that topologically we have diassortative mixing. In other words, interactions among users over the Flint Water Crisis is not proportional to their number of connections. Even though we observe fluctuations, there is a consistency among degree correlations and reply tweets in sentiment-only networks show a slight increase in assortativity.
Over the cumulative months, we also notice a consistently moderately positive sentiment correlation indicating that individuals within the network are slightly more inclined to interact with those who shared a similar sentiment. Figure 9 shows the consistency of sentiment correlations within each network configuration where reply networks have less sentiment correlation compared to retweet networks.
5. Discussion
In this work, we demonstrated how social media data could be analyzed to assess responsibility and blame in the aftermath of a disaster in accordance with social theories. While earlier work for similar purposes mostly benefited data collected through traditional means such as surveys (Driedger et al., 2014), we took a different approach that totally relied on microblog postings in the social media, i.e., Twitter, to test our hypotheses rooted in sociology of disasters. However, we should note that we did not form our hypotheses upon conflicting views on the topics in the first place, and they do not challenge the findings in the literature. The nature of our hypotheses also does not require complex or multivariate analysis.
By studying different kinds of crises, Olteanu et al. (2015) categorize information types shared on social media during these events. Following their topology, Flint water crisis is an instantaneous human-induced accidental hazard diffused over a county. It is a man-made disaster that might have started as an accident but evolved into “a story of government failure, intransigence, unpreparedness, delay, inaction, and environmental injustice” (Force, 2016); and it is an instantaneous crisis because no notices were given before it happened. Reviewing the earlier work in the literature, they also identify six broad categories for information communicated over Twitter during disasters. These information categories are (i) affected individuals, (ii) infrastructure and utilities, (iii) donations and volunteers, (iv) caution and advice, (v) sympathy and emotional support, and (vi) other useful information. They do not consider attribution of responsibility and blame as a distinct category; “updates about the investigation and suspects” is the most related phenomenon mentioned, which is addressed in the “other useful information” category (expressed vis-a-vis shooting and bombing events).
Although about thirty years ago Neal found it surprising that the process of blame was a neglected topic in disaster research (Neal, 1984), tracing over the citations that his paper has received to date and still not seeing any article particularly discussing blame, made us even more worrisome. In this paper, we contribute to this neglected field by testing theories of attribution of blame and responsibility on the Flint water crisis using new forms of data (the social web) and methods. In particular, we add to the disaster research by addressing the issues of: (i) sources for blame regarding a disaster, (ii) partisan predispositions in the blaming behavior, (iii) geographies that shows interest in the crisis the most, and (iv) the contagion of complaining (homophily, peer or network effect, and selective exposure).
Our findings agreed with the facts listed in the FWATF report which pointed the Governor of Michigan as the source of blame. We believe the consensus on an official report is more reliable than to be aligned with news on other media outlets which might be biased due to the framing (Thomas et al., 2016). In other words, social media is a venue for citizen journalism where contributing parties are individuals, who might be going through the disaster, as opposed to other news sources. Therefore, confirmation of the source of blame is an important proof of objectivity and reliability of the social media analysis.
In accordance with the source of blame, we discovered a one-way partisan predisposition based on sentiment analysis of the tweets targeting state officials. Namely, those who are blaming the Republican governor seem to have a milder or positive stance against the Democratic mayor which might be attributed to pre-existing conflicts and hostilities as Singer suggested (Singer, 1982). However, we were not able to confirm our hypothesis regarding the Republican stances toward the mayor due to the limited number of tweets.
Positive sentiment correlations reinforced the findings regarding the contagion of complaining as they demonstrated that individuals sharing the same emotions tend to interact more. In contrast to earlier studies which measured the correlation of happiness at different levels of social network neighborhoods (Bollen et al., 2011; Bliss et al., 2012), we proposed to study emotional assortativity since any kind of emotion, i.e., sadness or happiness, was our interest. We also believe emotional assortativity is another useful quality like obesity, disease, and habits in social networks which have been widely studied by Christakis, Fowler, and others (Christakis and Fowler, 2007, 2008, 2013; Fowler and Christakis, 2008; Hill et al., 2010; Rosenquist et al., 2010). Although the sentiment correlations are not that high, we can claim a moderate level of emotional assortativity which implies the higher likelihood of interaction between users of similar sentiments. On the other hand, lower correlations in Figure 9 for replies might be a sign of discussion of opposing parties that have more discrepant emotions.
Despite the abundance of data and ease of access in social media, we encountered some other limitations in this study such as the sample size which was limited to 50,000 tweets per day due to the crawling tool and Twitter regulations. When we applied the keyword filters to detect the relevant tweets to our hypotheses, we had a smaller corpus, which in turn was not enough to test the second hypothesis on partisan predisposition. In addition, our study is focused on a 5-month window where we observed a decreasing interest in the crisis toward the end, which limited a fair temporal analysis. Sentiment analysis was another challenge because of the length of the text in tweets which we tried to overcome by using an appropriate tool.
6. Conclusion
This study investigates attribution of responsibility and blame through online social media in the case of a disaster. In particular, we first form several theoretical hypotheses on attribution of responsibility and blame by building on the existing research in sociology of disasters. Then we operationalize these hypotheses on unobtrusive, observational social media data via computational methods. Our results demonstrate the effectiveness of social media data in revealing the source of blame, partisan predisposition, concerned geographies, and contagion of complaining in the aftermath of a crisis. Furthermore, this work adds to the few number of studies on this topic. It contributes to the sociology of disasters research also by exploiting a new, rarely used data source (the social web), and employing new computational methods (e.g., sentiment analysis and retrospective cohort study design) on this new form of data15. In this regard, this work can be seen as the first step toward drawing more challenging inferences on the sociology of disasters from social media data.
Author Contributions
HB and TO performed all calculations and data analysis, and wrote the first draft of manuscript. HB and TO developed the methods and had the original idea and guided the data analysis and presentation of results. RH contributed to the data analysis and assisted with writing the manuscript. All authors read and approved the final manuscript.
Funding
This work has been supported in part by the University of Michigan-Flint Provost's 60th Anniversary Research Grant.
Conflict of Interest Statement
The authors declare that the research was conducted in the absence of any commercial or financial relationships that could be construed as a potential conflict of interest.
Acknowledgments
Most of this work was done when TO was working at the George Mason University Center for Social Complexity and was supported by the Defense Threat Reduction Agency (DTRA) grant HDTRA1-16-0043.
Supplementary Material
The Supplementary Material for this article can be found online at: https://www.frontiersin.org/articles/10.3389/fcomm.2018.00045/full#supplementary-material
Supplementary Figure 1. Degree distributions for different types of networks over five months.
Footnotes
1. ^City of Flint 2014 Annual Water Quality Report. Available online at: https://www.cityofflint.com/wp-content/uploads/CCR-2014.pdf (Accessed July 04, 2018).
2. ^County Health Rankings and Roadmaps: Building a Culture of Health, County by County. Available online at: http://www.countyhealthrankings.org/app/michigan/2015/overview (Accessed July 04, 2018).
3. ^Data for the following days are missing due to collection issues: 01:23,24; 02:14,17-19; 04:28-30; 05:1-3,7,13-25.
4. ^Since there is no major political event taking place after the President's visit on May 4th, we truncate the figure for the sake of better visualization.
5. ^VADER's sentiment lexicon includes emoticons, common slang words, and accounts for punctuation and capitalization.
6. ^They either resigned (e.g., EPA officials and emergency managers), were fired (e.g., the head of MDEQ's drinking water unit), or their effective terms ended (e.g., the mayor).
7. ^The city council is made up of Democrats, the state of Michigan is ruled by a Republican governor, the Congress is controlled by Republicans, and the President is a Democrat.
8. ^Therefore, instead of 1000 tweets we ended up with 892 unique tweets labeled in total.
9. ^That is, pairs calculated. A perfect agreement would equate to a kappa of 1, and a chance agreement would equate to 0.
10. ^Tweets labeled for G (97577), M (11609) and E (6028) using keyword sets “governor, Snyder, onethoughnerd”, “mayor, Dayne, Walling”, and “mgr, manager, Kurtz, Earley, Darnell”, respectively.
11. ^This is due to our normalization factor. In normalizing Flint-related tweets per county, instead of using true population of counties we simply use sum of population of cities from which at least three tweets originated and available in our dataset.
12. ^Location field in the Twitter user profile is used to detect the Flinters
13. ^Following Twitter's convention, we use the term friends to refer to the users who someone follows. These Flinters in total follow 122,953 unique accounts, and 8,339 of those happen to be in our dataset.
14. ^When we examined the expressions toward the mayor and the governor without separating the parties blamed, we found out that average sentiment scores are negative for both officials, though at different levels (–0.12, –0.31).
15. ^The analysis code is available at github.com/oztalha/Flint.
References
Alexander, D. E. (2013). Social media in disaster risk reduction and crisis management. Sci. Eng. Ethics 20, 717–733. doi: 10.1007/s11948-013-9502-z
Bartels, L. M. (2002). Beyond the running tally: partisan bias in political perceptions. Polit. Behav. 24, 117–150. doi: 10.1023/A:1021226224601
Bird, D., Ling, M., and Haynes, K. (2012). Flooding facebook-the use of social media during the queensland and victorian floods. Aus. J. Emerg. Manag. 27, 27–33. Available online at: https://search.informit.com.au/documentSummary;dn=046814266005608;res=IELAPA
Bliss, C. A., Kloumann, I. M., Harris, K. D., Danforth, C. M., and Dodds, P. S. (2012). Twitter reciprocal reply networks exhibit assortativity with respect to happiness. J. Comput. Sci. 3, 388–397. doi: 10.1016/j.jocs.2012.05.001
Bollen, J., Gonçalves, B., Ruan, G., and Mao, H. (2011). Happiness is assortative in online social networks. Artif. Life 17, 237–251. doi: 10.1162/artl_a_00034
Burger, A., Oz, T., Crooks, A., and Kennedy, W. (2017). “Generation of realistic mega-city populations and social networks for agent-based modeling,” in Proceedings of the 2017 International Conference of The Computational Social Science Society of the Americas (New York, NY: ACM), 15:1–15:7.
Casagrande, D. G., McIlvaine-Newsad, H., and Jones, E. C. (2015). Social networks of help-seeking in different types of disaster responses to the 2008 Mississippi River Floods. Hum. Organ. 2015, 351–361. doi: 10.17730/0018-7259-74.4.351
Cheong, M., and Lee, V. (2010). “Twitmographics: learning the emergent properties of the twitter community,” in From Sociology to Computing in Social Networks, eds H. Chen, Y. Zhou, and E. Reid (Springer), 323–342.
Christakis, N. A., and Fowler, J. H. (2007). The spread of obesity in a large social network over 32 years. N. Engl. J. Med. 357, 370–379. doi: 10.1056/NEJMsa066082
Christakis, N. A., and Fowler, J. H. (2008). The collective dynamic of smoking in a large social network. N. Engl. J. Med. 358, 2249–2258. doi: 10.1056/NEJMsa0706154
Christakis, N. A., and Fowler, J. H. (2013). Social contagion theory: examining dynamic social networks and human behavior. Stat. Med. 32, 556–577. doi: 10.1002/sim.5408
Crooks, A., Hudson-Smith, A., Croitoru, A., and Stefanidis, A. (2014). “The evolving GeoWeb,” in GeoComputation, eds R. J. Abrahart and M. Linda (Boca Raton, FL: CRC Press), 69–96.
Crooks, A. T., and Wise, S. (2013). GIS and agent-based models for humanitarian assistance. Comput. Environ. Urban Syst. 41, 100–111. doi: 10.1016/j.compenvurbsys.2013.05.003
Drabek, T. E. (1986). Human System Responses to Disaster: An Inventory of Sociological Findings. Springer Series on Environmental Management. New York, NY: Springer.
Driedger, S. M., Mazur, C., and Mistry, B. (2014). The evolution of blame and trust: an examination of a canadian drinking water contamination event. J. Risk Res. 17, 837–854. doi: 10.1080/13669877.2013.816335
Edwards, M., Falkinham, J., and Pruden, A. (2015). Synergistic Impacts of Corrosive Water and Interrupted Corrosion Control on Chemical/Microbiological Water Quality. Flint, MI: National Science Foundation Grant Abstract.
Einwiller, S. A., and Steilen, S. (2015). Handling complaints on social network sites–An analysis of complaints and complaint responses on Facebook and Twitter pages of large US companies. Public Relat. Rev. 41, 195–204. doi: 10.1016/j.pubrev.2014.11.012
Faas, A. J., and Jones, E. C. (eds.). (2017). “Chapter 2 - Social network analysis focused on individuals facing hazards and disasters,” in Social Network Analysis of Disaster Response, Recovery, and Adaptation (Cambridge, MA: Butterworth-Heinemann), 11–23. doi: 10.1016/B978-0-12-805196-2.00002-9
Feenstra, R. A., and Casero-Ripollés, A. (2014). Democracy in the digital communication environment: a typology proposal of political monitoring processes. Int. J. Commun. 8:21. Available online at: http://ijoc.org/index.php/ijoc/article/view/2815 (Accessed September 29, 2018).
Fonger, R. (2013). Detroit Gives Notice: It's Terminating Water Contract Covering Flint, Genesee County in One Year. Available online at: http://www.mlive.com/news/flint/index.ssf/2013/04/detroit_gives_notice_its_termi.html (Accessed June 14, 2016).
Fonger, R. (2015). Flint Data on Lead Water Lines Stored on 45,000 Index Cards. Available online at: https://www.mlive.com/news/flint/index.ssf/2015/10/flint_official_says_data_on_lo.html (Accessed July 04, 2018).
Force, F. W. A. T. (2016). Flint Water Advisory Task Force Final. eds M. Davis, C. Kolb, L. Reynolds, E. Rothstein, and K. Sikkema. Report.
Fowler, J. H., and Christakis, N. A. (2008). Dynamic spread of happiness in a large social network: longitudinal analysis over 20 years in the framingham heart study. BMJ 337:a2338. doi: 10.1136/bmj.a2338
Glasgow, K., Vitak, J., Tausczik, Y., and Fink, C. (2016). “ ‘With Your Help…We Begin to Heal’: social edia expressions of gratitude in the aftermath of disaster,” in Proceedings of Social, Cultural, and Behavioral Modeling: 9th International Conference, SBP-BRiMS 2016, Vol. 9708 (Washington, DC: Springer) 226.
Goodchild, M. F. (2011). “Citizens as sensors: the world of volunteered geography,” in The Map Reader, eds M. Dodge, R. Kitchin, and C. Perkins (Wiley-Blackwell), 370–378.
Grinberger, A. Y., and Felsenstein, D. (2016). Dynamic agent based simulation of welfare effects of urban disasters. Comput. Environ. Urban Syst. 59, 129–141. doi: 10.1016/j.compenvurbsys.2016.06.005
Grinberger, A. Y., Lichter, M., and Felsenstein, D. (2015). “Simulating urban resilience:14 disasters, dynamics and (synthetic) data,” in Planning Support Systems and Smart Cities, Lecture Notes in Geoinformation and Cartography eds S. Geertman, J. Ferreira, R. Goodspeed, and J. Stillwell (Cham: Springer International Publishing), 99–119.
Hanna-Attisha, M., LaChance, J., Sadler, R. C., and Champney Schnepp, A. (2016). Elevated blood lead levels in children associated with the flint drinking water crisis: a spatial analysis of risk and public health response. Am. J. Public Health 106, 283–290. doi: 10.2105/AJPH.2015.303003
Hargittai, E., and Sandvig, C. (2016). “How to think about digital research,” in Digital Research Confidential: The Secrets of Studying Behavior Online, eds E. Hargittai and C. Sandvig (Cambridge, MA: MIT Press), 288.
Hill, A. L., Rand, D. G., Nowak, M. A., and Christakis, N. A. (2010). Emotions as infectious diseases in a large social network: the sisa model. Proc. R. Soc. Lond. B Biol. Sci. 277, 3827–3835. doi: 10.1098/rspb.2010.1217
Hjorth, L., and Kim, K.-h. Y. (2011). The mourning after: a case study of social media in the 3.11 earthquake disaster in japan. Telev. New Media 12, 552–559. doi: 10.1177/1527476411418351
Hurlbert, J. S., Haines, V. A., and Beggs, J. J. (2000). Core networks and tie activation: what kinds of routine networks allocate resources in nonroutine situations? Am. Sociol. Rev. 65, 598–618. Available online at: http://www.jstor.org/stable/2657385
Hutto, C. J., and Gilbert, E. (2014). “VADER: a parsimonious rule-based model for sentiment analysis of social media text,” in Eighth International AAAI Conference on Weblogs and Social Media (Ann Arbor, MI).
Iyengar, S. (1994). Is Anyone Responsible?: How Television Frames Political Issues. Chicago, IL: University of Chicago Press.
Jacobs, A. J. (2009). The impacts of variations in development context on employment growth: a comparison of central cities in michigan and ontario, 1980-2006. Economic Develop. Q. 23, 351–371. doi: 10.1177/0891242409343304
Kapucu, N. (2006). Interagency communication networks during emergencies boundary spanners in multiagency coordination. Am. Rev. Public Administ. 36, 207–225. doi: 10.1177/0275074005280605
Kennedy, C. (2016). Blood lead levels among children aged <6 years? flint, michigan, 2013–2016. MMWR Morb. Mortal. Wkly. Rep. 65, 650–654. doi: 10.15585/mmwr.mm6525e1
Kowalski, R. M., and Western Carolina, U. (1996). Complaints and complaining: Functions, antecedents, and consequences. Psychol. Bull. 119, 179–196. doi: 10.1037/0033-2909.119.2.179
Kumar, S., Barbier, G., Abbasi, M. A., and Liu, H. (2011). “TweetTracker: an analysis tool for humanitarian and disaster relief,” in The International Conference on Weblogs and Social Media (Barcelona).
Kwak, H., Lee, C., Park, H., and Moon, S. (2010). “What is Twitter, a Social Network or a News Media?” in Proceedings of the 19th International Conference on World Wide Web (New York, NY: ACM), 591–600.
Landis, J. R., and Koch, G. G. (1977). The Measurement of Observer Agreement for Categorical Data. Biometrics 33, 159–174. doi: 10.2307/2529310
Lau, R. R. (1985). Two explanations for negativity effects in political behavior. Am. J. Polit. Sci. 29, 119–138. doi: 10.2307/2111215
Lin, Y.-R., and Margolin, D. (2014). The ripple of fear, sympathy and solidarity during the Boston bombings. EPJ Data Sci. 3:31. doi: 10.1140/epjds/s13688-014-0031-z
Longley, K. (2011). Report: Buying in to New Water Pipeline From Lake Huron Cheaper for Flint Drinking Water Than Treating River Water. Report. Available online at: http://www.mlive.com/news/flint/index.ssf/2011/09/water_treatment.html (Accessed June 14, 2016).
Mao, Q. (2015). Experimental Studies of Human Behavior in Social Computing Systems. PhD thesis, Harvard University.
McPherson, M., Smith-Lovin, L., and Cook, J. M. (2001). Birds of a feather: homophily in social networks. Ann. Rev. Sociol. 27, 415–444. doi: 10.1146/annurev.soc.27.1.415
Meier, P. (2015). Digital Humanitarians: How Big Data Is Changing the Face of Humanitarian Response. Boca Raton, FL: Routledge.
Neal, D. M. (1984). Blame assignment in a diffuse disaster situation: a case example of the role of an emergent citizen group. Int. J. Mass Emergen. Disast. 2, 251–266.
Newman, M. E. (2002). Assortative mixing in networks. Phys. Rev. Lett. 89:208701. doi: 10.1103/PhysRevLett.89.208701
Olteanu, A., Vieweg, S., and Castillo, C. (2015). “What to expect when the unexpected happens: social media communications across crises,” in Proceedings of the 18th ACM Conference on Computer Supported Cooperative Work & Social Computing CSCW '15 (New York, NY: ACM), 994–1009.
Palen, L., Vieweg, S., Sutton, J., Liu, S. B., and Hughes, A. L. (2007). “Crisis informatics: studying crisis in a networked world,” in Third International Conference on e-Social Science (Ann Arbor, MI).
Panteras, G., Wise, S., Lu, X., Croitoru, A., Crooks, A., and Stefanidis, A. (2015). Triangulating social multimedia content for event localization using flickr and twitter. Trans. GIS 19, 694–715. doi: 10.1111/tgis.12122
Plotnick, L., White, C., and Plummer, M. M. (2009). “The design of an online social network site for emergency management: a one stop shop,” in AMCIS 2009 Proceedings, Vol. 420. Available online at: https://aisel.aisnet.org/amcis2009/420
Ratkiewicz, J., Conover, M., Meiss, M., Gonçalves, B., Patil, S., Flammini, A., et al. (2011). “Truthy: mapping the spread of astroturf in microblog streams,” in Proceedings of the 20th International Conference Companion on World Wide Web, WWW '11 (New York, NY: ACM), 249–252.
Reuter, C., Marx, A., and Pipek, V. (2012). Crisis management 2.0: Towards a systematization of social software use in crisis situations. Int. J. Inform. Syst. Crisis Respon. Manag. 4, 1–16. doi: 10.4018/jiscrm.2012010101
Rosenquist, J. N., Murabito, J., Fowler, J. H., and Christakis, N. A. (2010). The spread of alcohol consumption behavior in a large social network. Ann. Inter. Med. 152, 426–433. doi: 10.7326/0003-4819-152-7-201004060-00007
Rotman, D., Vieweg, S., Yardi, S., Chi, E., Preece, J., Shneiderman, B., et al. (2011). “From slacktivism to activism: participatory culture in the age of social media,” in CHI '11 Extended Abstracts on Human Factors in Computing Systems CHI EA '11 (New York, NY), 819–822. ACM.
Roy, S. (2015). Analysis of Water Samples from an Additional 72 Flint Homes Are Concerning. Flint Water Study Updates. Available online at: http://flintwaterstudy.org/2015/08/analysis-of-water-samples-from-an-additional-72-flint-homes-are-concerning-as-well/ (Accessed September 29, 2018).
Sears, D. O., and Freedman, J. L. (1967). Selective Exposure to Information: A Critical Review. Public Opin. Q. 31, 194–213. doi: 10.1086/267513
Shavit, Y., Fischer, C. S., and Koresh, Y. (1994). Kin and nonkin under collective threat: Israeli networks during the gulf war. Soc. Forces 72, 1197–1215. doi: 10.1093/sf/72.4.1197
Shear, M. D., and Bosman, J. (2016). “I've Got Your Back,” Obama Tells Flint Residents. New York, NY: The New York Times.
Simon, B., and Klandermans, B. (2001). Politicized collective identity: a social psychological analysis. Am. Psychol. 56, 319–331. doi: 10.1037/0003-066X.56.4.319
Singer, T. J. (1982). An introduction to disaster: Some considerations of a psychological nature. Aviat. Space Environ. Med. 53, 245–250.
Small, M. L., and Sukhu, C. (2016). Because they were there: access, deliberation, and the mobilization of networks for support. Soc. Netw. 47, 73–84. doi: 10.1016/j.socnet.2016.05.002
Spence, P. R., Lachlan, K. A., and Rainear, A. M. (2016). Social media and crisis research: data collection and directions. Comput. Hum. Behav. 54, 667–672. doi: 10.1016/j.chb.2015.08.045
The White House Office of the Press Secretary. (2016). President Obama Signs Michigan Emergency Declaration.
Thomas, T. L., Kannaley, K., Friedman, D. B., Tanner, A. H., Brandt, H. M., and Spencer, S. M. (2016). Media coverage of the 2014 west virginia elk river chemical spill: a mixed-methods study examining news coverage of a public health disaster. Sci. Commun. 38, 574–600. doi: 10.1177/1075547016662656
Tobler, W. R. (1970). A computer movie simulating urban growth in the detroit region. Economic Geogr. 46, 234–240. doi: 10.2307/143141
Vieweg, S., Hughes, A. L., Starbird, K., and Palen, L. (2010). “Microblogging during two natural hazards events: what twitter may contribute to situational awareness,” in Proceedings of the SIGCHI Conference on Human Factors in Computing Systems, CHI '10 (New York, NY: ACM), 1079–1088.
Wallace, A. F. C. (1956). Human Behavior in Extreme Situations; A Study of the Literature and Suggestions for Further Research. Washington, DC: National Academy of Sciences, National Research Council.
Wen, X., and Lin, Y.-R. (2016). “Sensing distress following a terrorist event,” in Social, Cultural, and Behavioral Modeling, Vol. 9708, eds K. S. Xu, D. Reitter, D. Lee, and N. Osgood (Cham: Springer International Publishing), 377–388.
Wright, J. (2013). Genessee County Drain Commissioner's Office. Available online at: http://media.mlive.com/newsnow_impact/other/Genesee%20County%20news%20release.pdf (Accessed June 14, 2016).
Keywords: sociology of disasters, computational social science, blame, responsibility, flint water crisis, social media, big data
Citation: Oz T, Havens R and Bisgin H (2018) Assessment of Blame and Responsibility Through Social Media in Disaster Recovery in the Case of #FlintWaterCrisis. Front. Commun. 3:45. doi: 10.3389/fcomm.2018.00045
Received: 16 October 2017; Accepted: 20 September 2018;
Published: 15 October 2018.
Edited by:
Nitin Agarwal, University of Arkansas at Little Rock, United StatesReviewed by:
Beverly Ann Cigler, Penn State Harrisburg, United StatesFantina Maria Santos Tedim, Universidade do Porto, Portugal
Copyright © 2018 Oz, Havens and Bisgin. This is an open-access article distributed under the terms of the Creative Commons Attribution License (CC BY). The use, distribution or reproduction in other forums is permitted, provided the original author(s) and the copyright owner(s) are credited and that the original publication in this journal is cited, in accordance with accepted academic practice. No use, distribution or reproduction is permitted which does not comply with these terms.
*Correspondence: Halil Bisgin, bisgin@umich.edu