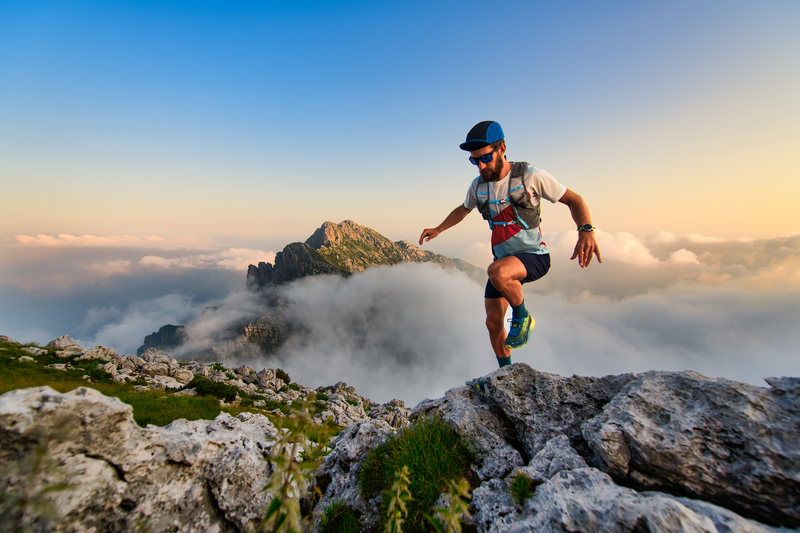
94% of researchers rate our articles as excellent or good
Learn more about the work of our research integrity team to safeguard the quality of each article we publish.
Find out more
ORIGINAL RESEARCH article
Front. Commun. , 20 July 2018
Sec. Psychology of Language
Volume 3 - 2018 | https://doi.org/10.3389/fcomm.2018.00025
Contextual predictability variation affects phonological and phonetic structure. Reduction and expansion of acoustic-phonetic features is also characteristic of prosodic variability. In this study, we assess the impact of surprisal and prosodic structure on phonetic encoding, both independently of each other and in interaction. We model segmental duration, vowel space size and spectral characteristics of vowels and consonants as a function of surprisal as well as of syllable prominence, phrase boundary, and speech rate. Correlates of phonetic encoding density are extracted from a subset of the BonnTempo corpus for six languages: American English, Czech, Finnish, French, German, and Polish. Surprisal is estimated from segmental n-gram language models trained on large text corpora. Our findings are generally compatible with a weak version of Aylett and Turk's Smooth Signal Redundancy hypothesis, suggesting that prosodic structure mediates between the requirements of efficient communication and the speech signal. However, this mediation is not perfect, as we found evidence for additional, direct effects of changes in surprisal on the phonetic structure of utterances. These effects appear to be stable across different speech rates.
Language offers speakers a multitude of choices of how to encode their messages, ranging from the temporal and spectral properties of sub-word units, to the choice of words, the structuring of syntactic elements, and sequencing sentences in discourse. In recent years, a body of psycholinguistic evidence has accumulated suggesting that the ease of processing of a linguistic expression is correlated with the predictability of the expression given its context. Contextual predictability is often quantified in terms of an information-theoretic measure known as surprisal.
On the phonetic level, effects such as shortening, deletion, lenition, a higher degree of coarticulation and reduced vowel dispersion are understood as manifestations of increased phonetic encoding density and are associated with higher predictability (low surprisal). Lower predictability (high surprisal) is associated with less dense phonetic encoding manifested in lengthening, fortition, a lower degree of coarticulation, and increased vowel dispersion.
Therefore, the phonetic encoding of a linguistic expression appears to be in a relation of covariation with the expression's contextually determined predictability. Words that are predictable from context tend to be produced with less phonetic detail, shorter duration, and even reductions on the phonological level, such as neutralization of features or elision of phonemes (Bell et al., 2003, 2009; Aylett and Turk, 2004, 2006).
The work reported in this paper explores the effects of surprisal and prosodic structure, separately and in interaction, on phonetic encoding. We study the impact of prosody and surprisal on duration, vowel dispersion, spectral emphasis and consonantal center of gravity. These phonetic variables are known correlates of prosodic variability and have also been shown to be sensitive to predictability effects. We analyze six languages that differ in their prosodic characteristics and come from different genetic sub-families: American English, Czech, Finnish, French, German, and Polish. Apart from answering to the need of more cross-linguistic studies on probabilistic effects (Jaeger and Buz, 2016), we look at the interplay of surprisal with prosodic structure (prominence and boundaries) and systematic speech rate variation. We also discuss how these interactions might be constrained by language-specific factors.
A particular direction of variation based on predictability is often used as an explanation for a specific phonetic phenomenon. For instance, studies on phonetic reduction concentrate on the effects of high contextual predictability while hypotheses concerning prosodic prominence often refer to acoustic enhancement of unpredictable elements. Despite these differences in focus, the nature and symmetry of probabilistic effects suggest that they complement each other as part of the same mechanism (Jaeger and Buz, 2016).
The mechanism is most generally defined as the mapping of information onto speech signal variability. The ratio of information content of a unit per amount of linguistic signal is often called information density (Levy and Jaeger, 2007). The co-variation has been hypothesized to be optimized by speakers in order to achieve a uniform ratio between information and signal encoding, i.e., a uniform information density (UID, Aylett and Turk, 2004; Levy and Jaeger, 2006).
In psycholinguistic literature and in the present study, information content of a unit is defined as the local, context-dependent likelihood, or predictability, of that unit. A common operationalization of such likelihood is surprisal. Surprisal quantifies the amount of information (in terms of bits) as the inverse of the units log probability given a local context:
Surprisal Theory (Hale, 2001; Levy, 2008) has been quite successful as an account of word-by-word processing difficulty. The theory posits that the processing difficulty incurred by a word is inversely proportional to the surprisal, or unexpectedness, of a word, which is typically estimated using probabilistic language models. Language models are most frequently trained on the word level but can in principle be applied to other types of units on different levels of the linguistic description. In this paper, we will use language models on a sub-word level, in fact, on the segmental level, to quantify the contextual predictability of speech sounds.
Surprisal is a local estimate of predictability, that is, it varies from one context to another. Another example of a local variable is phonological neighborhood density (PND, Munson and Solomon, 2004; Wright, 2004; Munson, 2007; Gahl et al., 2012; Gahl, 2015; Buz and Jaeger, 2016). PND measures the number of words in the lexicon that are phonologically similar to a target word, indicating how many words exist in the lexicon that are potentially confusable with the target word. Phonological neighbors are words that differ from the target word by one phoneme, i.e., one phoneme substitution, deletion, or addition (Luce and Pisoni, 1998).
There are other probabilistic factors that in turn refer to the systemic, global, or context-independent likelihood. Measures of global effects encode the unit's information status that comes with the properties of a given linguistic system: the lexicon, in case of words, or the sound system, in case of phonemes. In other words, such measures indicate the unit's a priori likelihood (Ernestus, 2014). A well-studied example of a global effect is the frequency of a word's occurrence in the lexicon based on corpus counts, i.e., lexical frequency.
For instance, Jurafsky et al. (2001, 2002) reported vowel reduction and shortening, arising from frequent production usage. However, a corpus study on English found differences in coarticulation between high- and low-frequency syllables embedded in nonce words but failed to find frequency effects on duration (Croot and Rastle, 2004). Frequency effects have also been demonstrated in studies analyzing syllable durations in large speech corpora (Schweitzer and Möbius, 2004), corroborated by computational simulations (Walsh et al., 2007, 2010). Moreover, frequency of occurrence can affect the shape of the fundamental frequency (F0) contour, and there are interactions between pitch accent type frequency and the frequency of the information status category of the word carrying the pitch accent (Schweitzer et al., 2009, 2010).
Speech production has been shown to be sensitive to lexical frequency (absolute frequency count in a corpus) and unigram probability (probability relative to other unigrams, as estimated from large corpora, with all probabilities summing up to unity). For instance, the speech production model proposed by Levelt and colleagues assumes an interaction between frequency of occurrence and the encoding of articulatory processes (Levelt and Wheeldon, 1994; Levelt, 1999). Frequent syllables, which tend to occur in frequent words, are produced faster than rare ones (Carreiras and Perea, 2004; Cholin et al., 2006), frequent past tense verbs are produced faster than rare ones (Losiewicz, 1992), frequent collocations (with don't, such as don't know) are more reduced than infrequent ones (Bybee and Scheibman, 1999), and words with high relative frequency—i.e., with high probability of occurrence given their neighbors—are more reduced than words with low relative frequency (Jurafsky et al., 2001). High-frequency syllables exhibit more coarticulation than rare syllables (Whiteside and Varley, 1998; Croot and Rastle, 2004). In experiments investigating coarticulatory effects on laboratory and acted speech, significant differences between frequent and infrequent syllables in words and pseudowords were found for several parameters defining vowels in perceptual space, including formant trajectories within the syllable, and formant transitions at syllable boundaries (Benner et al., 2007).
Apart from lexical frequency, there is evidence of other systemic effects, such as informativity (Seyfarth, 2014; Cohen Priva, 2015). Informativity (Piantadosi et al., 2011; Cohen Priva, 2015) is the average predictability of a segment given its language-specific distribution in the lexicon. In other words, informativity captures the amount of information a segment usually provides, i.e., how informationally useful it is across the entire language.
There are interactions between the effects of local and global likelihood measures. Informativity appears to modulate the impact of local predictability of a segment, given its context. For instance, the relative frequency of occurrence of the phoneme /ŋ/ in English is low but it is highly predictable when following /stændɪ-/, as in standing (Cohen Priva, 2015). Cohen Priva (2015) has shown that segments that are globally unpredictable have longer durations and are less likely to be elided even when they are locally predictable. On the basis of these results, Cohen Priva (2015) proposes that informativity provides a link between the language-specific variability observed in the duration of segments and universal mechanisms that co-determine it.
Interpretations of probabilistic effects usually take a speaker-oriented or a listener-oriented perspective. As pointed out by Gahl et al. (2012), both perspectives refer to the speed of retrieval: either retrieval in production or retrieval in comprehension. In production, increased difficulty of lexical access may be related to less frequent and contextually less predictable units, leading to longer or more spectrally distinctive realizations (cf. Buz and Jaeger, 2016). However, longer and more distinctive realizations have also been suggested to result from explicit encodings by speakers who choose to facilitate the intelligibility of difficult target units (Wright, 2004; Levy and Jaeger, 2007; Gahl et al., 2012; Gahl, 2015). Speaker-oriented and listener-oriented accounts are not mutually exclusive. Hypotheses and interpretations found in psycholinguistic literature fall on a continuum between the two views (Jaeger and Buz, 2016), especially if seen as a consequence of the constraints imposed by communication (Gambi and Pickering, 2017), i.e., the tension between production ease and robust message transmission (Zipf, 1935; Lindblom, 1990; Levy and Jaeger, 2007; Jaeger and Buz, 2016).
Probabilistic properties of the speech communication process have sometimes been interpreted as evidence for communicative efficiency. Studies taking the communicative efficiency perspective often directly refer to Shannon's information theory (Shannon, 1948) as a meta-theory. Information theory is the basis of a rational perspective of language use, which assumes that speakers are aiming at an optimal distribution of information across the linguistic signal. This optimal distribution avoids local peaks and troughs in information, which would exceed or under-utilize the capacity of the communication channel or the cognitive capacity of the interlocutor (e.g., Aylett and Turk, 2004; Levy and Jaeger, 2007). It has become a methodological standard to use quantitative measures of information derived within the mathematical paradigm of information theory, such as density, entropy, etc., to relate to structural properties of written language and to human language processing.
A recent example of this line of reasoning is Pate and Goldwater (2015) who have offered a re-interpretation of predictability effects “through the lens of information theory,” by regarding particular effects as either reflecting source coding or channel coding. The expected effect of source coding is to use shorter signals for more common messages by eliminating redundancy. The well-known property of the lexicon to use shorter encodings for high-probability words (Zipf, 1935; Piantadosi et al., 2011) is an example of source coding. Conversely, channel coding is expected to preserve or add redundancy to signals to avoid communication errors.
In this paper, we are investigating aspects of the relation between contextual predictability, operationalized as surprisal, and phonetic encoding. Our underlying hypothesis is that speakers modulate the density of phonetic encoding in the service of maintaining a balanced distribution of information: information and phonetic encoding are assumed to be in an inverse relationship (Aylett and Turk, 2004, 2006; Levy and Jaeger, 2007). We aim to analyze how phonetic encoding is modulated by systematic changes in the phonetic structure and some of its acoustic-phonetic features as a function of the predictability of a linguistic expression.
Several authors have proposed that the mapping of information onto speech signal variability is mediated by prosodic structure. For instance, the Hyper- & Hypoarticulation (H&H) theory (Lindblom, 1990) suggests that prosody reflects predictability effects. The theory explains variation between weak and strong elements as a function of contextual predictability. It posits that the speaker attempts to achieve a balance between clarity of perception and ease of articulation. The H&H theory thus integrates a speaker-oriented and a listener-oriented account. However, the H&H theory stops short of incorporating frequency-based concepts such as probability of occurrence or information-theoretic concepts such as surprisal.
In contrast, the Smooth Signal Redundancy (SSR) hypothesis proposed by Aylett and Turk (2004, 2006) is in essence an information-theoretic account of phonetic variation (cf. the UID byLevy and Jaeger, 2007 for syntactic variation). The SSR hypothesis emphasizes the role of prosodic structure in the mapping between contextual predictability and the speech signal explicitly, by positing that prosodic variation encodes predictability. According to this account, languages have evolved to distribute the recognition likelihood of each element of the utterance evenly. The recognition likelihood of an element depends on linguistic and acoustic redundancy. Linguistic redundancy is defined as the lexical, syntactic, semantic and pragmatic contextual “clues to identity” of the element (Turk, 2010), or the element's predictability profile (Turk and Shattuck-Hufnagel, 2014). Acoustic redundancy, i.e., the set of acoustic clues to identity, is implemented by prosodic variation (Turk, 2010) or the prosodic profile. The inverse relationship between predictability and the prosodic profile results in a Smooth Signal Redundancy (SSR) profile, that is, in the smooth spread of information over the utterance, thereby globally optimizing the recognition likelihood of the utterance.
For example, vowels in highly predictable contexts are assumed to show less dispersion than vowels in less predictable contexts. Aylett and Turk (2006) investigated the influence of prosodic structure and predictability on vowel characteristics in General American English read speech. Results of the study showed that vowels were more centralized with increased language redundancy (i.e., higher predictability), vowel quality in prominent syllables was more distinct than in syllables that were not prominent, and spectral characteristics of vowels were also more distinct in syllables before prosodic boundaries than in syllables at word- or at no boundary. Aylett and Turk (2006) concluded that language redundancy and acoustic redundancy show an inverse relationship which is mediated and implemented through prosodic structure.
On the other hand, many authors consider prosodic factors as important predictors of signal variability, however, independent from predictability. For example, Bell et al. (2009), Gahl et al. (2012), Gahl (2015) and Cohen Priva (2015) also include prosodic effects such as speech rate or lexical stress as control factors, but crucially without attributing prosodic variation with a function that mediates, for instance, retrieval speed.
Turk (2010) and Turk and Shattuck-Hufnagel (2014) also suggested a link between prosodic constituency and predictability. They proposed that signaling word boundary strength can be exploited to complement language redundancy. In this way, predictability explains the observation that the probability that a boundary occurs is higher as the utterance becomes longer. As utterance length increases, it becomes exceedingly more difficult to parse the words, which lowers the recognition likelihood of words in such a sequence. Breaking up long, low-probability sequences by adding a boundary improves parsing and thus the likelihood of success in recognizing the words.
The SSR hypothesis in its strong form implies that prosody acts as an interface to redundancy effects. That is, for example, local duration variability in speech originates from the relationship between language and acoustic redundancy and the primary role of prosody would be to manage the information density. Empirically, however, Aylett and Turk (2004) showed that acoustic variance is only partially explained by this relationship. There was a unique contribution of prosodic factors to duration variance, which Aylett and Turk (2004) explained by possible effects of phonologization and conventionalization of the relationship between redundancy and prominence. This explanation seems to relate to the systematic encoding of information in languages and lexicons, i.e., global probabilistic effects. It suggests that prosodic structure encodes the a priori variation in redundancy.
There are inter-relations between the number and strength of boundaries and prominences in an utterance and speech rate. From the perspective of the SSR hypothesis, the question arises whether the modulation of acoustic redundancy by means of prosody results in a change of speech rate as well: does increased (decreased) acoustic redundancy correlate with faster (slower) speech rate? There are possible inter-relations with speaking style, too. For instance, boundaries and prominences, i.e., structural properties of prosody, subserve communicative goals such as the need to speak clearly. In clear speech, speech rate is slow and the number and strength of boundaries and prominences increases. The result is a higher degree of acoustic redundancy: the recognition likelihood of an element from the acoustic signal is increased. Conversely, producing fewer pauses and therefore fewer boundaries, results in faster speech rates (Quené, 2008) and lower acoustic redundancy. Such an option may be desirable when the linguistic predictability of the units spoken faster is high. A third option is also possible: as Cohen Priva (2017) shows, speakers might limit information content and provide less informative syntactic structures overall in order to speak quickly.
Turk (2010) and Turk and Shattuck-Hufnagel (2014) suggest that effects of speech rate and clarity on the frequency of boundaries and prominences are general, non-grammatical factors. These effects should globally constrain the relationship of linguistic predictability with acoustic redundancy by adding a “fixed amount” of acoustic redundancy proportionally to all boundaries and prominences in an utterance. They suggest that this general “magnification” of acoustic redundancy via speech rate and discourse factors is independent of the local modulation of prominence and boundaries.
In a similar vein, van Son and van Santen (2005) noted that language redundancy exerting a larger direct effect on phonetic encoding, over and above prosodic effects, can be observed if the analysis is performed on a different level than the syllable, which has been the reference level for studies in the SSR paradigm (Aylett and Turk, 2004, 2006). van Son and van Santen (2005) analyzed the center of gravity (COG) of sentence-medial, intervocalic consonants in accented American English words as a function of segment predictability, which was operationalized as frequency of occurrence. They did not find a straightforward relationship between segment frequency and COG. However, they observed that after correcting for consonant quality factors influencing COG (e.g., place of articulation) there was a correlation between higher segment frequency and higher COG and longer duration in specific word positions for specific segment classes.
It appears that the way in which predictability is encoded phonetically differs across languages. The acoustic parameters that encode predictability in one language may not be susceptible to such effects in another language. Moreover, the inter-relationships between prosodic encoding and predictability encoding in acoustic variability are language-specific as well.
For instance, Turnbull et al. (2015) offered evidence that the relationship between prosodic prominence and local predictability is constrained by language-specific structure. Turnbull et al. (2015) studied two genetically unrelated languages, viz. American English and Tupi-Guarani, in which they hypothesized an interdependency between focus and predictability in their effect on acoustic exponents of prominence, such as f0 and duration. Specifically, they expected that unpredictable context would lead to the enhancement of acoustic prominence under focus, relative to focus exponents in predictable context. Pitch accent types, f0 and duration were analyzed as a function of visual contextual predictability. In American English, the effect of focus was found to be greater in an unpredictable context than in a predictable context, as reflected in the variability of f0 peaks, accent types and duration of the target words. However, in Tipi-Guarani, contextual predictability did not modulate the effect of focus on acoustic correlates of prominence. Instead, an effect of focus on pitch accenting and duration was observed, independent of the main effect of predictability on f0 slope variability.
van Son et al. (2004) reported on a corpus study of three typologically different languages, viz. Dutch, Finnish and Russian. They used word frequency as a global predictability measure, and the probability of a vowel given the preceding word onset as a local, segment-based probability measure. They measured the effect of these measures on correlates of vowel reduction: duration, center of gravity, vowel dispersion and intensity. They showed robust, if low, correlation coefficients (6% or less of the variance explained) for all correlates in relationship to word frequency. However, the information content of the segment showed larger cross-linguistic differences in its effect on acoustic reduction. van Son et al. (2004) attributed the difficulty to attest cross-linguistically consistent effects of the more local measure to the noisy character of the specific measure used in the study. It is, however, conceivable that on the level of segments, prosodic, phonotactic and morphological effects specific to the studied languages interact with the degree of exponence of predictability. Interestingly, van Son et al. (2004) did not find an effect of segmental information content on vocalic reduction in Finnish and Russian expressed in terms of vowel dispersion. Additionally, they showed that predictability effects also depend on discourse, in that the observed correlations were stronger in read speech than in spontaneous speech.
Recently, Athanasopoulou et al. (2017) examined the fixed vs. movable phonological stress parameter as a manifestation of predictability. In languages with movable stress, such as Brazilian Portuguese (or English), its placement is relatively inconsistent and hence, much less predictable than in fixed-stress languages. This contrasts with languages with fixed stress, such as Armenian, Turkish or French studied in Athanasopoulou et al. (2017) that canonically place lexical stress on a specified syllable. At the same time, fixed-stress languages also allow for some non-canonical placements that show degrees of predictability depending on the morphological and phonological aspects of stress location in that language. Athanasopoulou et al. (2017) analyzed f0, intensity and vowel dispersion and found that the hypothetically redundant, canonical positions do not decrease the distinctiveness of the acoustic properties, relative to the non-canonical positions. However, the overall position predictability did affect the acoustic manifestation in all of the studied languages.
Clearly, much remains to be studied with respect to how probabilistic effects, prosodic effects and prosodic systems relate to, and possibly interact with, one another and influence observable signal variability. The present study is intended as a step toward clarifying this relationship cross-linguistically and under different intended speech rates.
The primary goal of this study is to investigate the effects of prosody and contextual predictability (defined as surprisal) on segmental duration, vowel dispersion, vocalic spectral emphasis and consonantal center of gravity. These phonetic variables are known correlates of prosodic variability and have also been shown to be sensitive to predictability effects.
The study includes six languages from different sub-families and with different prosodic, phonological and grammatical characteristics: American English, Czech, Finnish, French, German, and Polish. We expect that these languages will primarily show differences related to the phonetic encoding of information in those acoustic parameters that are affected by prominence. In other words, where in a specific language a parameter is not used for marking prominence, it will also not be available as a correlate of predictability. For example, contrary to English, we do not expect surprisal to greatly affect duration in Polish because of the weak correlation of duration with prominence in this language (Malisz and Wagner, 2012; Malisz and Żygis, 2018).
We also aim to shed more light on the research questions posed by Turk (2010), Turk and Shattuck-Hufnagel (2014), and Pellegrino et al. (2011) regarding the relationship between speech rate and surprisal. Specifically, we address the hypothesis that the effect of surprisal does not depend on speech rate but is robustly positive across speech rate levels in all studied languages. In other words, we expect that the higher the surprisal of a segment, the more phonetically distinct the segment is, regardless of speech rate. Phonetic distinctiveness is expressed by longer duration, an expanded vowel space, and specific spectral energy distributions. The precise combination of the acoustic variables that convey the degree of distinctiveness is expected to depend more on how the language-specific prosodic structure is expressed phonetically, rather than on speech rate.
The broader question we are asking is whether contextual predictability affects phonetic encoding at all levels of the linguistic structure, including prosody, or is fully moderated by prosody, as posited by the strong version of the SSR hypothesis (Aylett and Turk, 2004, 2006). Similarly to the original SSR studies we analyse the proportion of observed effects that is explained by the prosodic structure while looking further at the effects of surprisal that are not mediated by prosody. In general, we expect these effects to be subtle but significant.
In comparison to other studies that directly or indirectly explored similar research questions (Aylett and Turk, 2004, 2006; van Son et al., 2004; van Son and van Santen, 2005), we analyze a dataset that varies systematically in speech rate but is otherwise more consistent and less noisy than what is typically the case in corpus studies. We use semi-automatic methods of segmentation and annotation of the data, meaning that each observation, unlike what is possible for large corpora, was verified by experts using consistent annotation instructions. Additionally, we exploited the steadily growing text corpus resources that allowed us to build more accurate language models than, e.g., models based on CELEX. We also use a statistical analysis method, viz. linear mixed models (Bates et al., 2015) that allows for a comprehensive regression modeling of fixed and random effects, in addition to correlation analysis (van Son et al., 2004; van Son and van Santen, 2005) and multiple regression (Aylett and Turk, 2004, 2006) used in previous studies.
The remainder of this paper is organized as follows. Section 2 presents the languages under investigation as well as the pertinent text and speech corpora. It explains the methods of extracting the acoustic-phonetic features that are considered to be affected by changes in surprisal, the language models that serve as the basis for quantifying surprisal, and the structure of the prosodic model that is assumed to modulate its effects on the speech signal. The results of the acoustic-phonetic and statistical analysis is presented in section 3, in terms of both correlations and linear mixed models. The results are discussed in section 4, with a special emphasis on the language-specific interaction between surprisal and the prosodic structure.
To examine the impact of surprisal on segmental variability we analyzed production data from six languages: Czech (CES), American English (ENG), Finnish (FIN), French (FRA), German (DEU) and Polish (POL). Apart from Finnish, a Finno-Ugric language, the other languages belong to the Indo-European language family. All studied languages but Finnish have a complex syllable structure. The research consensus on French is that it does not possess lexical stress. Instead, accent is assumed to be regular on the last syllable of a phrase with a full vowel, often acoustically realized as lengthening (Jun and Fougeron, 1995; Di Cristo, 1998; Michelas et al., 2000). English and German have a bounded weight-sensitive lexical stress system, where stress location depends on syllable weight, morphological structure, and lexical marking. The primary accent in underived words falls within a three-syllable window (Goedemans and van der Hulst, 2013). Word stress in Czech and Polish is fixed. In Czech, it is assigned to the leftmost syllable of the prosodic word, in Polish it is predominantly fixed on the penultimate syllable. All analyzed languages but Polish have a phonological vowel length contrast. In Finnish and Czech the vowel length contrast exists in stressed as well as unstressed syllables. Finnish also contrasts phonological consonant length.
Large text corpora were processed and language models for the six languages were built (Table 1). First, each corpus was cleaned by removing erroneous entries with non-alphabetic characters. Then, the text was phonetically transcribed into IPA or, if a syllabified annotation was not already provided with the corpus, it was processed by means of an automatic syllabification program custom-scripted in bash shell.
For French, Lexique 3.80 (New et al., 2001), which provides phonetic transcription and syllabification, was retrieved online. For German, the Frankfurter Rundschau corpus1 was transcribed and syllabified using a tool for grapheme-to-phoneme conversion (Reichel and Kisler, 2014). For American English, the same procedure was applied to process the Corpus of Contemporary American English2. For Finnish, the Finnish Parole Corpus was acquired online3. The text was automatically converted into IPA by the eSpeak speech synthesizer (Duddington, 2015) and automatically syllabified using the custom script. For Polish, a frequency dictionary derived from a large-scale web corpus (Zséder et al., 2012) was converted into IPA and syllabified by an automatic tool for transcription and syllabification (Zeldes, 2008–2014). For Czech, a frequency dictionary acquired from a large-scale web corpus (Zséder et al., 2012) was processed using the same methods as in the case of Finnish.
A subset of the BonnTempo corpus (Dellwo et al., 2004) was analyzed with three female and three male speakers of male speakers of CES, DEU, ENG, FIN, FRA, and POL. FIN recordings were added to the BonnTempo corpus using the original instructions (Dellwo et al., 2004). Informed consent forms were signed by all participants. An ethics committee approval process was not required per any involved institutions' guidelines or per national regulations in the recording of the corpus. Speakers were given an excerpt of a novel in their native language and were asked to familiarize themselves with the text. Next, speakers were recorded at what they considered to be reading at their preferred, normal pace. Then, they were asked to slow down their repeated reading, and to slow down even more. In a third step, fast speech rate was recorded asking speakers to speak fast, and speed up their speech rate until they considered they could not speed up any more. From these acceleration steps, recordings at the normal speech rate as well as the first increments toward slow and fast speech tempo were used for analysis.
The speech data were automatically segmented using SPPAS for FRA (Bigi, 2013), and WebMaus (Kisler et al., 2012) for the other languages. Since there was no automatic segmentation tool available for CES, WebMaus implementations for other languages were tested. Hungarian WebMaus proved to be the most effective for CES because both languages have a largely similar consonant inventory, and vowel length is phonemic in both CES and Hungarian. The manual verification process of the automatic segmentation of CES data was completed by a Slavic languages expert (the fifth author of the paper). For all the other languages, the automatic segmentation was also manually verified by phonetic experts using criteria such as to facilitate a comparative analysis between the different languages in the corpus. For example, the beginnings of vowels were marked when F1 was clearly visible, and ends of vowels were marked using the end of visible F2 structure.
The phonetic variables under investigation include segmental duration and vowel space expansion (i.e., vowel dispersion), both of which have previously been identified as correlates of prosodic variability and as being sensitive to predictability effects. Moreover, two related measures of spectral variability are also included: spectral emphasis for vocalic segments and center of gravity for consonantal segments. These measures identify the frequency regions in which energy is concentrated, either in the form of a ratio (spectral emphasis) or, somewhat simplified, the mean (COG).
Segmental duration was measured in the recordings of six speakers per language in the BonnTempo audio corpus (frequencies: CES = 3620, DEU = 3515, ENG = 3398, FIN = 4990, FRA = 3293, POL = 3292; total number of observations = 22108).
In an F1/F2 space, the location of the vowel can be defined by its dispersion (Bradlow et al., 1996), often operationalized as the Euclidean distance between the average center of the vowel space and formant values for each vowel token (Bradlow et al., 1996). Vowels with a large vowel dispersion are more distinct from vowels produced with a neutral vertical and horizontal tongue position. Furthermore, increased vowel dispersion is associated with increased intelligibility (Bradlow et al., 1996).
Recently, the interpretation of vowel dispersion has been broadened with respect to vowel specific movement within the vowel space with regard to competitor vowels. Wedel et al. (2018) argued that vowels are under competition from neighboring vowels depending on their position in the vowel space. Peripheral vowels, such as /i, e/, for instance, are under competition from interior vowels, i.e., /ɪ, ɛ/. Wedel et al. (2018) showed that in cases of lexical competition peripheral vowels move further away from the vowel space center to the periphery, while interior vowels move closer to the center. In the light of these findings, one could argue that vowel dispersion is not an ideal measure of vowel space expansion. However, only ENG and DEU from the language investigated in our corpus, and CES to some extent, have contrastive interior vowels in their phonemic systems. In addition, Wedel et al. (2018) limited their study to vowels in stressed position. Surely, in unstressed position DEU interior vowels, and /ɪ/ in ENG, face competition from mid central vowels. Admittedly, the F1/F2 Euclidean distance from the vowel space center cannot possibly capture all vowel movements within the vowel space, and vowels might behave differently with regard to the amount of dispersion from the center. We have included vowel identity as a control factor to account for these differences.
F1 and F2 formant analysis was conducted at the temporal midpoint of vocalic nuclei with the Burg algorithm implemented in Boersma and Weenink (2015). Default settings of the software were used, i.e., maximum of five formants, window size of 0.025 s, pre-emphasis from 50 Hz. The maximum formant threshold was changed as advised by Boersma and Weenink (2015) depending on the speaker's sex, 5000 Hz for male speakers and 5500 Hz for female speakers. The total number of analyzed tokens was 5198. Table 2 shows the token frequency and vowel qualities in this analysis. The number of analyzed items varied across languages. If available in the data, tense and lax vowels in closed front, closed back, low and front mid position were used for the analysis to allow for comparability of the vowel spaces across languages. The different lax and tense vowel phonemes in Table 2 were summarized under four umbrella vowel identities /i/ for closed front vowels, /e/ for mid front vowels, /a/ for open mid/back vowels, and /u/ for closed back vowels. Vowel identity was included in the statistical models to inspect the vowel specific effect on the vowel space expansion measure.
Table 2. Vowel and consonant qualities and token frequencies per language for vowel dispersion (Vowels) and consonantal center of gravity analyses (Consonants).
Formant values were cleaned and manually checked when they were 200 Hz above or below average values for each language, if the range of dispersion in the data was not provided in the literature (Wiik, 1965; Majewski and Hollien, 1967; Hillenbrand et al., 1995; Liénard and Di Benedetto, 1999), and below or above attested measures of variability otherwise (Sendlmeier and Seebode, 2006; Skarnitzl and Volin, 2012). Then, speaker-dependent standard normalization was applied to control for differences in formant values due to speaker identity and sex (Lobanov, 1971). As a measure for vowel distinctiveness, the Euclidean distance between the midpoint of the vowel space and formant values for each vowel token were calculated for each speaker (Bradlow et al., 1996), to facilitate comparisons with previous studies on vowel dispersion (Munson and Solomon, 2004; Wright, 2004; Gahl, 2015; Buz and Jaeger, 2016). This measure of vowel dispersion is independent of differences in vowel inventory between the languages because it is a relative measure of vowel space expansion within the individual vowel space of each speaker.
Spectral emphasis and COG approximate loudness and, possibly, articulatory effort—factors involved in the perception and production of prominence. Both lower spectral emphasis and lower COG indicate less high-frequency power (van Son and van Santen, 2005). Higher values indicate a wider frequency range contributing to the clarity and loudness of the sound. Compared to Euclidean distances in the F1/F2 dimension, these variables provide more information about segment distinctiveness in the auditory domain.
Spectral emphasis expresses the contribution of higher frequency bands to the overall intensity, relative to the lower frequency bands. It was measured by subtracting the sum of energy in the higher frequencies (1200–5000 Hz) from the sum of energy in the lower frequencies (70–1000 Hz) over the whole vowel interval. Vowel spectral emphasis analysis involved all available vocalic segments in the BonnTempo corpus (n = 9422).
Center of gravity was analyzed in nasal stops and the majority of continuant consonants, viz. approximants and fricatives, with the exception of glottal fricatives (van Son and Pols, 1999; van Son and van Santen, 2005). The frequencies and qualities of the analyzed consonantal segments for each language are presented in Table 2. Some consonants from this set did not occur in the corpus data, e.g., /ʃ, ʒ, j/ in the English text, so we deal with partially crossed factors. The total number of consonant tokens in the COG analysis was 7126.
COG was measured using a 25 ms Hamming window in the initial, medial and final phase of the sound and then averaged over these phases. In order to equalize the variances between fricative continuants and the other sounds in the subset, we express the mean COG in semitones, following van Son and van Santen (2005).
Following Aylett and Turk (2006), the prosodic model used in the current study comprises prominence and boundary. Prominence was defined as a binary factor using primary lexical stress (stressed vs. unstressed) based on the BonnTempo corpus. In French, accent was marked on the last syllable of a phrase with a full vowel (Jun and Fougeron, 1995; Féry, 2014). If monosyllabic, function words were counted as unstressed, whereas content words were identified as stressed. We did not include secondary stress as a factor since some of the languages tested do not have secondary stress i.e. Czech (Dogil et al., 1999) and French (Di Cristo, 1998). Besides, the number of polysyllabic words in the text that would bear secondary stress is too low. We note, however, that the automatic transcription (e.g.,: using e-speak) we used for calculating tri-phone probabilities is allophonic and dependent on lexical stress. The factor Boundary involved two levels: none and a high likelihood of a prosodic boundary. A high likelihood of a prosodic boundary was marked when a pause of at least 100 ms followed the syllable that included the segments under analysis (Aylett and Turk, 2006).
A three level categorical factor was coded according to the acceleration or deceleration condition in which the speaker was reading the text material. This is equivalent to the Intended Speech Rate variable studied by Dellwo et al. (2004) in BonnTempo. We will henceforth refer to this factor as speech tempo. We selected the preferred rate coded as “normal,” the first acceleration step coded as “fast” and the first deceleration step coded as “slow.” Figure 1 shows the mean differences measured in syllables per second (cf. Laboratory Speech Rate, Dellwo et al., 2004) across these intended speech tempo conditions for each of the six languages in the BonnTempo subset.
Figure 1. Speech rate in syllables per second for the analyzed subset of the BonnTempo corpus, differentiated by tempo condition and language.
To study the relationship between surprisal and phonetic structure, we trained language models (LMs) on the phone level. Models were computed on the basis of large text corpora in the six languages under study (cf. section 2.2.1). The surprisal variable derived from each model was used as a predictor of the phonetic variables. Overall, we estimated surprisal values from tri-phone and bi-phone models (2)–(4). The bi-phone-based surprisal models were further differentiated depending on whether the right or the left context of the target segment was taken into account.
Tri-phone based surprisal model centered on the target segment:
Bi-phone model, preceding context:
Bi-phone model, following context:
Bi-phone and tri-phone LMs were tested for relationships with segmental duration and the vocalic and consonantal spectral variables. Due to the restricted number of vowel data points in the BonnTempo corpus, only bi-phone LMs were used in vowel space analyses.
We use exploratory correlation analyses to inspect the cross-linguistic aspects of surprisal effects on the acoustic variables in the BonnTempo corpus. Moreover, correlational data was reported previously in studies involving some of the languages we analyze (e.g., Finnish, Russian van Son et al., 2004); we include correlations for comparative purposes. We build statistical models in section 3.2 to analyze general tendencies across languages.
We calculate Pearson's r to identify the surprisal model that has the strongest and most consistent relationship with the target phonetic variable. We refer to Table 3 for details on the correlation coefficients regarding all used surprisal models.
Table 3. Pearson's correlation coefficients and tests (α = 0.05) between phonetic encoding variables (duration, vocalic spectral emphasis and consonantal center of gravity) and surprisal values estimated from bi-phone models taking the following or preceding context into account and from a tri-phone model.
We found that the tri-phone based surprisal model had the strongest and most consistent relationship with duration. We show the correlation between segment duration and the surprisal values for these segments based on the tri-phone language model in Figure 2, differentiated by language and tempo condition. The correlation between these two variables shows their dependence relative to language. The coefficients are statistically significant in each language and the coefficient for all six languages equals r = 0.16 [t(22108) = 24.0, p < 0.001]. The strongest correlation is found for American English [r = 0.28, t(3398) = 18.18, p < 0.001]. The correlation is slightly negative and weakest for Polish [r = −0.04, t(3292) = −2.19, p < 0.01].
Figure 2. Correlations (Pearson's r) between segment duration in milliseconds and log-transformed surprisal values of segments based on tri-phone language models in the BonnTempo corpus, differentiated by tempo condition.
For vowel dispersion, surprisal values were estimated from bi-phone LMs for each language taking the previous context into account. Figure 3 presents the correlation of the Euclidean distance values for the vocalic segments with surprisal values, differentiated by language and tempo condition. Averaged over all languages, there is a significant positive correlation between vowel dispersion and bi-phone surprisal relative to the preceding context [r = 0.16, t(5196) = 11.75, p < 0.01]. When analyzed separately, this general observation also holds for German, American English, French, Czech and Polish. For Finnish, however, there is no significant relationship between vowel dispersion and surprisal. The correlation between both variables is the least strong in Polish [r = 0.12, t(788) = 3.37, p < 0.01], and the strongest for German vowels [r = 0.30, t(823) = 9.05, p < 0.01]. The positive relationship and coefficient values appear not to change with tempo class for the duration and vowel distinctiveness variables. We further test the effect of tempo in section 3.2.
Figure 3. Correlations (Pearson's r) between Lobanov-normalized Euclidean distance as a measure of vowel dispersion and log-transformed surprisal values of segments based on bi-phone language models in the BonnTempo corpus, differentiated by tempo condition.
Vocalic spectral emphasis and consonantal COG were correlated with both bi-phone models (modeling preceding or following context) and the tri-phone model (modeling both contexts). All coefficients are listed in Table 3. The results indicate that only German and American English show a consistent positive relationship between vocalic spectral emphasis and surprisal derived from all three language models. Regarding consonantal COG, the pattern is the same for German, whereas English shows a positive relationship only for the preceding context model.
Looking at the other, less straightforward correlation patterns in the other languages, we see that Czech shows evidence of significant relations between vocalic spectral emphasis and surprisal variation. This is the case when surprisal is influenced by preceding segmental context, and both contexts at the same time, but not by the following context. On the other hand, the center of gravity of the analyzed Czech consonants does not appear to depend on surprisal. Finnish shows a significant inverse relationship for both spectral measures if preceding context or both contexts influence the value of surprisal. The following context bi-phone model does not produce a significant dependence.
French contrasts with most languages discussed so far with regard to spectral measures and displays only significant, positive correlations between surprisal derived from the following context language model. This applies to the variation of spectral energy in both vowels and consonants. Polish, the only clear outlier in this sample of languages regarding correlations with duration, shows a positive dependency between the following context model and spectral emphasis as well as a weak inverse correlation for the preceding context model. Not surprisingly, tri-phone based surprisal does not produce a significant correlation here. Regarding COG, Polish somewhat follows the Finnish pattern in that we see a very weak, to weak, inverse relationship for the preceding and bi-directional context.
In this section we analyze several dependent variables using linear mixed models4. We study the following correlates of prosodic variability: duration, vocalic dispersion, spectral emphasis as well as consonantal center of gravity, and the impact of the prosodic model and the surprisal model on these variables.
In each model reported below we used backwards model selection by first formulating a maximum model (including interaction terms, if so stated). The maximum model also included a maximal random structure involving random slopes and intercepts for all fixed factors (and their interactions, if so stated). Random variables included Language, Speaker, Word and Segment, Preceding and Following segment as hierarchical (nested) factors. We coded Language as a random effect in our models to test the hypothesis, motivated by the study by Pellegrino et al. (2011), that information encoding strategies are apparent across all speech rates and languages. In case there were convergence errors, these led to simplifications of the random structure: we removed higher terms in the random structure first, then simple random slopes, one by one. The same procedure applied when correlations of 1 or 0 were found for the random slopes. The final random structure is stated in the descriptions of the final models arrived at for each dependent variable.
Significance of fixed effects was evaluated by performing maximum likelihood t-tests using Satterthwaite approximations to degrees of freedom (using the lme4 Bates et al., 2015 and lmerTest packages Kuznetsova et al., 2016).
The continuous variable Surprisal was log-transformed due to positive skewness. All categorical factors were treatment-coded.
We first tested for the baseline condition that Surprisal is a significant predictor of duration in an additive model. We entered prosodic fixed factors: Tempo, Stress, Boundary plus the information theoretic factor Surprisal as predictors and included the control variable speaker Gender. The maximal random structure and the model backward selection principles are described in section 3.2. Table 4 shows the estimates and coefficients of model comparisons via maximum likelihood t-tests. As expected, duration is significantly and positively affected by stress, the presence of a boundary and slowing tempo. Our contextual predictability measure, the tri-phone surprisal, also significantly lengthens the segments.
Once we established that both the prosodic and the surprisal factors are significant predictors of duration, we aimed to verify whether the impact of Surprisal on duration is contingent on the effect of Tempo. If such a relationship between these predictors exists, we should see a significant interaction between these variables in a duration model.
We formulated the maximum interaction model and selected the final model using the procedure described in 3.2. For duration, we included interaction terms for each prosodic fixed factor: Tempo, Stress, Boundary with Surprisal and included the control variable speaker Gender. The full model also included a maximal random structure involving random slopes and intercepts for all the interactions. The final random structure for the duration model included (a) random intercepts for Segment, Preceding, Following segment, Word and Language, as well as (b) random slopes for Stress, Boundary, Tempo and log(Surprisal) per Speaker as well as Stress and Boundary per Word.
According to the tests, the interaction of Tempo with Surprisal is not significant for the duration variable. For the fixed factors, we report the regression coefficients, the standard errors, the t-test values as well as the corresponding p-values associated with the maximum likelihood tests in Table 5.
The marginal pseudo-R2, indicating how much variance is explained by the fixed factors, showed that the baseline prosodic model explains 33% of the duration variance alone. The explained variance increases by 3% when Surprisal is included in the additive model and by further 1% when Surprisal interacts with Stress and Boundary. The conditional pseudo-R2 for the variance explained by both fixed and random effects equaled 77% in the final interaction model.
Formant values F1 and F2 were normalized using speaker-dependent standard normalization (Lobanov, 1971). Surprisal values were log-transformed due to positive skewness (γ = 0.63). The predictor variables Surprisal and Stress were slightly collinear (r = 0.24). The categorical variables Stress, Tempo and Boundary were treatment coded, while sum coding (effect coding) was used for the factor Vowel identity.
For the baseline vowel dispersion model, the fixed effects Surprisal of the preceding bi-phone, Vowel identity, Stress, Speech Rate, Boundary, and Gender were entered. The maximal random structure included random intercepts for Following and Preceding segment, Speaker, Language and Word, as well as random slopes for all fixed effects. Because of convergence errors the model was simplified in a backward selection procedure following the procedure explained in section 3.2. First, the random structure was reduced removing random slopes. The random intercept for Speaker did not explain any variance in the data. Stepwise simplification resulted in a final model with random intercepts for Preceding and Following Segment, Language and Word and random slopes for Surprisal per Word.
In the baseline surprisal–prosody analysis, all fixed effects but Boundary, Stress, and Gender reached significance level in explaining variability in vowel dispersion. Table 6 shows the estimates of the model obtained by lme4 and lmerTest. As expected, vowel dispersion was positively affected by tempo: as the speech rate gets slower, the vowel dispersion measure increases. We found a tendency for an effect for vowels in stressed syllables to be more dispersed than vowels in unstressed syllables. Regarding the contextual predictability measure, vowels with high bi-phone surprisal values were significantly more dispersed than vowels with lower surprisal values. Vowel dispersion also differed with the vowel that was investigated. On average, /i/ was significantly more dispersed than the grand mean, while vowels /a/ and /e/ were less dispersed than the grand mean. Post-hoc analysis revealed that there were significant differences between vowel dispersion at normal, fast, and slow speech rate. Vowels at slow speech rate were more dispersed than vowels at fast and normal speech rate, and vowels produced at normal intended speech rate were significantly more dispersed than fast vowels (Table 7).
In a second step, interactions were entered into the baseline surprisal–prosody analysis of vowel dispersion. Similar to the segmental duration analysis, interactions between all prosodic factors and Surprisal were tested comparing the interaction model to the baseline model. None of the interactions in the model reached statistical significance (Table 8).
The marginal pseudo-R2 indicating how much variance is explained by the fixed factors showed that the baseline prosodic factors explain 0.6% of the vowel dispersion variance. The explained variance increases by 2.5% when Surprisal was included in the additive model. A large amount of variance was explained when Vowel identity was added to the model (16.48% increase). The conditional pseudo-R2 for the variance explained by both fixed and random effects equaled 87% in the final model.
In the linear mixed model analyzing effects on spectral emphasis of vowels listed in Table 2, the maximal random structure was reduced stepwise and the models were selected following the procedure explained in section 3.2. The simplified structure resulted in random intercepts for Preceding and Following Segment, Vowel and Language, as well as random intercepts and slopes for Boundary, Stress, Tempo and Surprisal both per Word and per Speaker.
Table 9 presents the results of the prosodic model for the variable. We see a weak but statistically significant positive effect of Stress on vocalic spectral emphasis, indicating that energy in stressed vowels is amplified in the higher frequencies. The Tempo condition and the Boundary factor have no effect on this variable.
Regarding the effect of Surprisal, we tested all three measures of Surprisal as predictors of vocalic spectral emphasis added one by one to the baseline prosodic model. The three models included Surprisal measures stemming from (a) a bi-phone preceding context language model, (b) a bi-phone following context model and (c) a tri-phone model. The interaction term between Stress and Surprisal was not tested, since the main effect of Surprisal as tested in additive models (a), (b), or (c) was not significant. Table 10 presents the statistically non-significant estimates for the surprisal factors found in the three models. We conclude that we were not able to show evidence for an effect of Surprisal on this correlate of prosodic structure, at least not with the amount of data at our disposal (n = 9422 vocalic intervals).
Table 10. Vocalic spectral emphasis: effects of three surprisal measures as estimated in separate models.
In the following model we analyzed the center of gravity of consonants listed in Table 2. The maximal random structure was reduced stepwise following the procedure explained in section 3.2. The simplified structure resulted in random intercepts for Preceding and Following Segment, Vowel, Word and Language, as well as random intercepts and slopes for Boundary, Stress, Tempo per Speaker and per Word. Surprisal also remained as a random slope per Speaker. The Surprisal measure was based on the bi-phone language model taking the following segment as context into account.
The main effects of Stress and Tempo on COG were not significant. The factor Boundary had a significant positive effect. Surprisal, however, had a significant negative effect, namely, there was less energy concentrated in the higher frequencies of the studied consonants when they were surprising given the following segmental context. Table 11 presents the estimates and results of the maximum likelihood t-tests. As an additional check, we formulated a model with solely Surprisal as the predictor of COG in order to see whether its effect is positive but only changes sign in the presence of other variables entered in the maximum model. Such a model still provided a negative estimate of the simple effect of Surprisal on COG (Coeff. = −5.4, St. Err = 0.86, t = −6.3, p < 0.001).
The interaction model was selected as described in section 3.2. In order to test for the consonant identity effects indicated in van Son and van Santen (2005), we entered the sum-coded factor Place (coronal, labial, palatal) and let it interact with prosodic factors and Surprisal. The analysis showed that Surprisal was a significant positive predictor of consonantal center of gravity when its effect was moderated by Stress. The effect of Surprisal was also different depending on the level of the factor Place (Table 12). The value of COG (in semitones) was higher when the target consonant was a coronal and higher in surprisal, given the following context. This result agrees with van Son and van Santen (2005), who found a linear relationship between coronals and information content.
Table 12. Interaction model of consonantal COG: interaction of surprisal with prosodic and place of articulation factors.
One of the goals of the present study was to analyze the potential influence of tempo changes on contextual predictability effects. Our working hypothesis was that consistent encoding strategies should be observed in all speech rates, based on the evidence that languages show a systematic relationship between information transmission and speech rate as part of phonetic encoding (Pellegrino et al., 2011). In other words, the relationship between linguistic redundancy and acoustic redundancy should remain apparent across speech rates as well as across languages.
Our findings show that in accordance with Pellegrino et al. (2011), tempo does not appear to interact with local segmental modulations of duration that encode information. These results might also speak in favor of Turk's suggestion (Turk, 2010) that tempo encodes acoustic redundancy globally, without interfering with local modulations or with the surprisal effects on, for instance, duration. Our data contains tempo conditions in which the speakers were reading the same text in three intended speech rates, normal, slow and fast. Due to the nature of the task, the speech rate, within a given tempo condition, remains relatively stable. There are, however, clear differences between tempo conditions in terms of measured speech rate (cf. Figure 1). The models also show that tempo changes have the expected effects on duration in our self-controlled tempo task, i.e., the segments are longer in the decelerated condition and shorter in the accelerated condition. But as we do not have a quantification of speech rate that would dynamically measure the influence of tempo segment by segment, it is difficult to definitively answer if and how predictability might interact with the speed of articulation. We can only conclude that tempo does not appear to, for example, invert the positive relationship between segmental contextual predictability and the acoustic parameters we studied. Such an effect would not be consistent with the general hypotheses we considered. We also aimed to shed further light onto how language-specific factors might relate to the effects of contextual predictability. Most studies so far have been conducted on Germanic data or on typologically closely related languages (Jaeger and Buz, 2016), with the exception of studies we discussed in section 1.4. Our data includes examples of three major subfamilies of Indo-European (Germanic, Slavic, and Romance) and a Finno-Ugric language, Finnish. Importantly, these languages exhibit several differences in the way in which they encode prosodic information and other related structural properties phonetically. We assumed that these properties will influence the relationships between contextual predictability measures and acoustic measures of phonetic encoding. We did not search the space of all possible acoustic parameters that might play a role in information encoding but we restricted ourselves to those that have been implicated in prosodic structuring.
Given Aylett and Turk's hypothesis (Aylett and Turk, 2004, 2006) that probabilistic effects have been phonologized over time in prosodic structure, we expected that those acoustic parameters that are known to be reliable exponents of prosodic structure in a given language will correlate most strongly with surprisal. These parameters were duration, vowel dispersion, spectral emphasis (Sluijter and van Heuven, 1995; Sluijter and Van Heuven, 1996; Heldner, 2003) and consonantal center of gravity (van Son and van Santen, 2005).
It is known that duration is strongly related to the expression of prominence in English but not in Polish (Malisz and Wagner, 2012). In line with this, we find stronger dependence of surprisal on duration in the former language than in the latter. Moreover, since Finnish does not have significant vowel reduction in unstressed positions, we do not find a positive correlation between surprisal and F1/F2 Euclidean distance measure of vowel dispersion, similar to van Son et al. (2004) (who studied stressed word-initial Finnish vowels). With the exception of robust correlations in Czech, in general those languages in our set that espouse weak acoustic expression of prominence, i.e., French and Polish, show the weakest relationships evidenced in our correlation analysis of duration and vowel dispersion.
A complex picture emerges from correlations of spectral features with predictability measures. This is in a sense expected, if the relationship between prosody and surprisal is considered, since parameters such as spectral balance are not the most robust correlates of prosodic prominence for example. We separated the broadly conceived spectral balance characteristics into spectral emphasis for vowels and center of gravity for consonants. Note, the majority of the six languages showed a positive correlation between duration and vowel dispersion and surprisal, which was not always the case in the spectral analyses (Figures 2, 3, and Table 3).
The Germanic languages in our study, English and German, showed robust effects here too (with the exception of English COG–surprisal correlations). French, however, showed a positive effect only in the case of a bi-phone surprisal model taking the following context into account, while Czech for example, did not present a significant relationship between surprisal and COG at all. Finnish and Polish, languages with outlying tendencies in the vowel dispersion and duration analyses, also exhibited either non-significant correlations or significant negative dependencies between different models of surprisal (bi- and tri-phone) and vocalic spectral emphasis and consonantal COG, respectively.
One possible explanation for the moderately strong inverse dependencies between, e.g., surprisal and COG in Polish, as well as the lack of any relationship in Czech, is the interaction of place of articulation with the effect of surprisal. In their study of prosodic and predictability effects on consonantal COG, van Son and van Santen (2005) showed that the effect of stress on COG (and duration) strongly depends on the primary consonantal articulator and the phone's position in the word. Specifically, they found a positive effect of stress on COG only for word-medial coronals. A significant correlation between their systemic predictability measure, i.e., frequency, and COG and duration was also evidenced. Specifically, coronals, as consonants produced with the tongue, which is the more agile articulator, were strongly reduced when they were systemically predictable.
Similar to van Son and van Santen (2005), the result of our modeling is that the variability of COG under prosodic and predictability variation is complex. The spectral bandwidth expressed by COG in our data is lower as a function of segmental surprisal and does not vary under stress, but it is higher when stressed segments are more surprising given the following segmental context. Coronals, in particular, are more susceptible to the effect of surprisal, as it was also the case for the high-front vowels in the vowel dispersion study.
We also tested for local probabilistic effects on vocalic spectral emphasis. We are not aware of a cross-linguistic study that looked at vocalic spectral emphasis in the context of predictability—our analysis points to a null effect of surprisal on this measure. van Son et al. (2004) suggested via a correlation analysis of a read speech corpus that a local measure of segmental predictability was positively related to root mean square intensity and COG in Finnish and Russian and only COG in Dutch. All three measures, COG, spectral emphasis and root mean square intensity are measures of the variation in loudness differentiated on the basis of spectral characteristics of different basic speech sounds. However, we found surprisal effects on a measure relevant for consonants (COG) but not for vowels (spectral emphasis).
Motivated by the work by Aylett and Turk (2004, 2006) on English and van Son and van Santen (2005) on Russian, Dutch, Finnish we also asked how far contextual predictability effects can be responsible for phonetic variation, independently of prosody. Our findings are generally in line with a weak version of the Smooth Signal Redundancy hypothesis (Aylett and Turk, 2004, 2006), and they highlight multiple and complex interactions between segmental and suprasegmental factors and information-theoretic factors related to predictability in context.
The contributions of surprisal and prosodic predictors to the overall duration variance are in accordance with the results in Aylett and Turk (2004), who analyzed the impact of a different local measure on syllabic duration in corpora, namely the probability of a syllable given the previous two syllables. We find similar effects in that regard using a tri-phone model centered on the target segment and analyzing the relationship between surprisal and duration using linear mixed models rather than multiple regression.
Our model explained 77% of variance in the semi-controlled material. A contextual segment-based surprisal measure explained 3% of the variance in addition to the variance accounted for by the prosodic model. Aylett and Turk's (2004) results also showed a relatively small contribution of redundancy factors at 6%. Furthermore, in our analysis, the best fitting model for duration included a significant dependency of surprisal upon stress, which further strengthens the hypothesis that its variability is contingent upon prosodic structure.
The ratio of variance explained by prosodic factors vs. surprisal is considered informative in Aylett and Turk (2004, 2006) and in our study, as relatively more focus is put on the hypothesis that the linguistic function of prosody and surprisal is similar, possibly shared. Namely, prosody is seen as a modulator of information density. Other studies on duration variability and the influence of information theoretic factors, e.g., Jaeger (2010); Seyfarth (2014) and Cohen Priva (2015) included prosodic factors as important control variables with the assumption that the effects of both factors are largely independent.
We found a significant positive effect of surprisal on vowel dispersion. Vowels in high-surprisal contexts were more dispersed in their spectral characteristics than in low-surprisal contexts, as expected (Jurafsky et al., 2001, 2002; Aylett and Turk, 2006; Benner et al., 2007). Based on marginal pseudo-R2 values, surprisal explained a larger quantity of the vowel dispersion variance than the prosodic factors used here. This result was contrary to findings in Aylett and Turk (2006), who reported an overall smaller effect of language redundancy on vowel formants F1 and F2 than for the prosodic model.
The current study replicates results regarding differences between vowel dispersion as a function of speech rate. Vowel dispersion increases with decreasing speech rate (Turner et al., 1995; Weiß, 2007; Weirich and Simpson, 2014). Vowel formants move to a more central position in the F1/F2 vowel space under fast speech rate when investigated in intended tempo deviations (Turner et al., 1995) and in naturally occurring differences in speech rate (Weiß, 2007).
In contrast to Schulz et al. (2016) and Aylett and Turk (2006), we found a positive but non-significant effect of stress on vowel dispersion. This difference might be due to the weak positive correlation between surprisal and stress (r = 0.23). Effects for both variables cannot be fully separated in a statistical model. In addition, Schulz et al. (2016) analyzed only five languages of the BonnTempo corpus, DEU, CES, POL, FIN, and FRA. The present study also includes (American) English, a language which shows a relatively strong correlation between surprisal and vowel dispersion [r = 0.26, t(558) = 6.39, p < 0.001]. The factor Boundary in the prosodic model was not significant in the LMM, in contrast to previous studies which showed that word and phrase boundaries complement effects of language redundancy (Turk, 2010), and that vowels tend to be more distinct in syllables preceding a phrase boundary (Aylett and Turk, 2006).
Aylett and Turk (2006) emphasized the large degree of variability of unique or shared contributions of their redundancy and prosodic model in explaining variance in F1 and F2 of ENG vowels among different vowel phonemes. The current study also showed that the impact of surprisal and prosody largely depended on the investigated vowel identity, although a different measure of vowel dispersion was used than in Aylett and Turk (2006). The factor Vowel identity explained 17.5% of variance in the vowel dispersion measurements. In addition, vowel identities differed in the magnitude of their dispersion compared to the mean. The phoneme /i/ was significantly more dispersed than the grand mean, while vowels /a/ and /e/ were less dispersed than the grand mean.
Finally, we consider empirical aspects that usually constitute caveats and limitations of studies similar to the present one: the estimation quality of prosodic and information theoretic variables. Regarding the latter, since we used a domain denser than that of words, namely, phones, it could be of benefit to use larger n sizes in the language models. This question is particularly relevant given our results that indicate tri-phone models to show more robust results than bi-phone models. Despite the fact that phones are small units and there are only about 40 types per language, we nevertheless run into data sparsity problems when n is increased for n-gram model training, especially given the limited size of available corpora for spoken language. Moreover, hierarchical structural information such as syllable and word boundaries, which affect the properties of units on the lower level (i.e., phones), is captured by contexts of sequences on the same level (i.e., phone sequences) only if we have models for very long sequences and extremely large annotated corpora; in practice, neither is available. We also believe that smaller contexts facilitate the comparison across languages that have quite diverse syllable structures. In addition, the relationship between information density and phonetic structures is assumed to be better reflected by phoneme language models (Oh et al., 2015).
It would be worth to investigate methods for combining language models trained on specific levels of linguistic representation with models for other levels. This approach is motivated by the insight that, for instance, the phonetic encoding of a target phone depends not only on the sequence of phones preceding and following it (cf. Van Son and Pols, 2003) but also on the surprisal of the syllable to which it belongs and on that of the word, etc. This is a major research effort and beyond the scope of this paper.
There is one crucial limitation of the present study that concerns read speech. In the BonnTempo task, the speakers read the material in different tempos several times. Our motivation to use this type of data was the controlled variation of speech tempo that would be difficult to elicit in spontaneous speech. At the same time, the repetition of the same textual material probably has the most attenuating impact on predictability effects due to possible familiarization and memorization effects. Whether read speech is a sub-optimal register to evidence local predictability effects is not clear.
In a study on final /t/ reduction in Dutch, Hanique and Ernestus (2011) found that effects of predictability on phonetic structure were more pronounced in spontaneous speech than in other speech registers including read speech. Such findings suggest at the same time that the patterns found by us in read speech may also have an effect in more spontaneous registers. On the other hand, van Son et al. (2004) report on correlational data where they found stronger effects of information content in read speech than in spontaneous speech. Another correlation analysis in Van Son and Pols (2003) did not show differences in speech registers that were repeated (retold stories and repeated sentences) compared to ad hoc speech. Clearly the interdependence of speech register, prosody and predictability harbors unexplored complexities.
To conclude, our findings are generally compatible with a weak version of Aylett and Turk's Smooth Signal Redundancy hypothesis (Aylett and Turk, 2004, 2006), suggesting that the prosodic structure mediates between requirements of efficient communication and the speech signal. However, this mediation is not perfect, as we found evidence for additional, direct effects of changes in predictability on the phonetic structure of utterances. These effects appear to be stable across different speech rates in models fit to data derived from six different European languages.
ZM, EB, and BM drafted the manuscript with critical revisions by YO and BA. ZM, EB, and BA annotated; ZM, EB, and YO processed; and ZM and EB analyzed the data. All authors designed the experiment and approved the final version of the manuscript.
The authors declare that the research was conducted in the absence of any commercial or financial relationships that could be construed as a potential conflict of interest.
We wish to thank both reviewers for their insightful and constructive comments. This research was funded by the Deutsche Forschungsgemeinschaft (DFG) as part of SFB 1102 Information Density and Linguistic Encoding at Saarland University.
1. ^European Corpus Initiative Multilingual Corpus I (ECI/MCI), http://www.elsnet.org/eci.html
2. ^http://corpus.byu.edu/coca/
3. ^http://kaino.kotus.fi/sanat/taajuuslista/parole.php
4. ^The model was formulated and evaluated using the lme4 package (1.1-12) (Bates et al., 2015) and lmerTest package (2.0-33) (Kuznetsova et al., 2016) in R (3.3.3) (R Core Team, 2017), a software environment for statistical computing.
Athanasopoulou, A., Vogel, I., and Dolatian, H. (2017). “Acoustic properties of canonical and non-canonical stress in French, Turkish, Armenian and Brazilian Portuguese,” in Proceeding of Interspeech 2017 (Stockholm), 1398–1402.
Aylett, M., and Turk, A. (2004). The smooth signal redundancy hypothesis: a functional explanation for relationships between redundancy, prosodic prominence, and duration in spontaneous speech. Lang. Speech 47, 31–56. doi: 10.1177/00238309040470010201
Aylett, M., and Turk, A. (2006). Language redundancy predicts syllabic duration and the spectral characteristics of vocalic syllable nuclei. J. Acoust. Soc. Am. 119, 30–48. doi: 10.1121/1.2188331
Bates, D., Mächler, M., Bolker, B., and Walker, S. (2015). Fitting linear mixed-effects models using lme4. J. Statist. Softw. 67, 1–48. doi: 10.18637/jss.v067.i01
Bell, A., Brenier, J., Gregory, M., Girand, C., and Jurafsky, D. (2009). Predictability effects on durations of content and function words in conversational English. J. Memory Lang. 60, 92–111. doi: 10.1016/j.jml.2008.06.003
Bell, A., Jurafsky, D., Fosler-Lussier, E., Girand, C., Gregory, M., and Gildea, D. (2003). Effects of disfluencies, predictability, and utterance position on word form variation in English conversation. J. Acoust. Soc. Am. 113, 1001–1024. doi: 10.1121/1.1534836
Benner, U., Flechsig, I., Dogil, G., and Möbius, B. (2007). “Coarticulatory resistance in a mental syllabary,” in Proceedings of the International Congress of Phonetic Sciences (Saarbrücken), 485–488.
Bigi, B. (2013). SPPAS – Automatic Annotation of Speech. Available online at: http://www.lpl-aix.fr/~bigi/software.html
Boersma, P., and Weenink, D. (2015). Praat: doing phonetics by computer [computer program]. Version 5.4.22. Available online at: http://www.fon.hum.uva.nl/praat/
Bradlow, A. R., Torretta, G. M., and Pisoni, D. B. (1996). Intelligibility of normal speech I: Global and fine-grained acoustic-phonetic talker characteristics. Speech Commun. 20, 255–272. doi: 10.1016/S0167-6393(96)00063-5
Buz, E., and Jaeger, T. F. (2016). The (in)dependence of articulation and lexical planning during isolated word production. Lang. Cogn. Neurosci. 31, 404–424. doi: 10.1080/23273798.2015.1105984
Bybee, J., and Scheibman, J. (1999). The effect of usage on degrees of constituency: the reduction of don't in English. Linguistics 37, 575–596. doi: 10.1515/ling.37.4.575
Carreiras, M., and Perea, M. (2004). Naming pseudowords in Spanish: effects of syllable frequency. Brain Lang. 90, 393–400. doi: 10.1016/j.bandl.2003.12.003
Cholin, J., Levelt, W. J., and Schiller, N. O. (2006). Effects of syllable frequency in speech production. Cognition 99, 205–235. doi: 10.1016/j.cognition.2005.01.009
Cohen Priva, U. (2015). Informativity affects consonant duration and deletion rates. Lab. Phonol. 6, 243–278. doi: 10.1515/lp-2015-0008
Cohen Priva, U. (2017). Not so fast: Fast speech correlates with lower lexical and structural information. Cognition 160, 27–34. doi: 10.1016/j.cognition.2016.12.002
Croot, K., and Rastle, K. (2004). “Is there a syllabary containing stored articulatory plans for speech production in English?” in Proceedings of the 10th Australian International Conference on Speech Science and Technology, (Sydney), 376–381.
Dellwo, V., Steiner, I., Aschenberner, B., Dankovicova, J., and Wagner, P. (2004). “BonnTempo-corpus and BonnTempo-tools: a database for the study of speech rhythm and rate,” in Proceedings of Interspeech 2004 (Jeju Island, Korea), 777–780.
Di Cristo, A. (1998). “Intonation in French,” in Intonation Systems: A Survey of Twenty Languages, eds D. Hirst, and A. Di Cristo (Cambridge: Cambridge University Press), 195–218.
Dogil, G., Gvozdanovic, J., and Kodzasov, S. (1999). Slavic languages. Emp. Approac. Lang. Typol. 20-4, 813–876. doi: 10.1515/9783110197082.2.813
Duddington, J. (2015). eSpeak text to speech. Available online at: http://espeak.sourceforge.net/, Retrieved on 1 February 2015.
Ernestus, M. (2014). Acoustic reduction and the roles of abstractions and exemplars in speech processing. Lingua 142, 27–41. doi: 10.1016/j.lingua.2012.12.006
Féry, C. (2014). “Final compression in French as a phrasal phenomenon,” in Perspectives on Linguistic Structure and Context: Studies in Honour of Knud Lambrecht, eds S. Katz Bourns, and L. L. Myers (Amsterdam: John Benjamins), 133–156.
Gahl, S. (2015). Lexical competition in vowel articulation revisited: Vowel dispersion in the Easy/Hard database. J. Phonet. 49, 96–116. doi: 10.1016/j.wocn.2014.12.002
Gahl, S., Yao, Y., and Johnson, K. (2012). Why reduce? Phonological neighborhood density and phonetic reduction in spontaneous speech. J. Mem. Lang. 66, 789–806. doi: 10.1016/j.jml.2011.11.006
Gambi, C., and Pickering, M. J. (2017). “Models linking production and comprehension,” in The Handbook of Psycholinguistics, eds E. M. Fernández and H. Smith Cairns (John Wiley & Sons), 157–181.
Goedemans, R., and van der Hulst, H. (2013). “Fixed stress locations,” in The World Atlas of Language Structures Online, Chapter 14. eds M. S. Dryer, and M. Haspelmath (Leipzig: Max Planck Institute for Evolutionary Anthropology).
Levy, R. (2001). “A probabilistic Earley parser as a psycholinguistic model,” in Proceedings of the second meeting of the North American Chapter of the Association for Computational Linguistics on Language technologies, NAACL 01 (Stroudsburg, PA), 1–8. doi: 10.3115/1073336.1073357
Hanique, I., and Ernestus, M. (2011). “Final/t/reduction in dutch past-participles: The role of word predictability and morphological decomposability,” in Proceedings of Interspeech 2011 (Florence), 2849–2852.
Heldner, M. (2003). On the reliability of overall intensity and spectral emphasis as acoustic correlates of focal accents in Swedish. J. Phonet. 31, 39–62. doi: 10.1016/S0095-4470(02)00071-2
Hillenbrand, J., Getty, L. A., Clark, M. J., and Wheeler, K. (1995). Acoustic characteristics of American English vowels. J. Acoust. Soc. Am. 97, 3099–3111. doi: 10.1121/1.411872
Jaeger, T. F. (2010). Redundancy and reduction: speakers manage syntactic information density. Cognit. Psychol. 61, 23–62. doi: 10.1016/j.cogpsych.2010.02.002
Jaeger, T. F., and Buz, E. (2016). “Signal reduction and linguistic encoding,” in Handbook of Psycholinguistics, eds E. M. Fernández, and H. S. Cairns (Hoboken, NJ: Wiley-Blackwell), 38–81.
Jun, S.-A., and Fougeron, C. (1995). “The accentual phrase and the prosodic structure of French,” in Proceedings of the International Congress of Phonetic Sciences (Stockholm), 722–725.
Michelas, A., Portes, C., and Champagne-Lavau, M. (2000). “A phonological model of French intonation,” in Intonation ed A. Botinis (Netherlands: Springer), 209–242.
Jurafsky, D., Bell, A., and Girand, C. (2002). “The role of the lemma in form variation,” in Laboratory Phonology 7, eds C. Gussenhoven, and N. Warner (Berlin: Mouton de Gruyter), 1–34.
Jurafsky, D., Bell, A., Gregory, M., and Raymond, W. D. (2001). “Probabilistic relations between words: evidence from reduction in lexical production,” in Frequency and the Emergence of Linguistic Structure, eds J. Bybee, and P. Hopper (Amsterdam: Benjamins), 229–254.
Haak, D., Samsel, C., Gehlen, J., Jonas, S., and Deserno, T. M. (2012). “Signal processing via web services: the use case WebMAUS,” in Proceedings of Digital Humanities 2012, (Hamburg).
Kuznetsova, A., Bruun Brockhoff, P., and Haubo Bojesen Christensen, R. (2016). lmerTest: Tests in Linear Mixed Effects Models. R package version 2.0-33. doi: 10.18637/jss.v082.i13
Levelt, W. J. M. (1999). “Producing spoken language: a blueprint of the speaker,” in The Neurocognition of Language, eds C. M. Brown, and P. Hagoort (Oxford: Oxford University Press), 83–122. doi: 10.1093/acprof:oso/9780198507932.003.0004
Levelt, W. J., and Wheeldon, L. (1994). Do speakers have access to a mental syllabary? Cognition 50, 239–269.
Levy, R. (2008). Expectation-based syntactic comprehension. Cognition 106, 1126–1177. doi: 10.1016/j.cognition.2007.05.006
Levy, R., and Jaeger, T. F. (2006). “Speakers optimize information density through syntactic reduction,” in Advances in Neural Information Processing Systems 19, eds B. Schölkopf, J. Platt, and T. Hofmann (Cambridge, MA: MIT Press), 849–856.
Levy, R., and Jaeger, T. F. (2007). “Speakers optimize information density through syntactic reduction,” in Proceedings of the 20th Conference on Neural Information Processing Systems (NIPS) 849–856.
Liénard, J. S., and Di Benedetto, M. G. (1999). Effect of vocal effort on spectral properties of vowels. J. Acoust. Soc. Am. 106, 411–422. doi: 10.1121/1.428140
Lively, S. E., Pisoni, D. B., Van Summers, W., and Bernacki, R. H. (1990). “Explaining phonetic variation: a sketch of the H & H theory,” in Speech Production and Speech Modelling, eds W. J. Hardcastle and A. Marchal (Dordrecht: Kluwer), 403–439.
Lobanov, B. M. (1971). Classification of Russian vowels spoken by different speakers. J. Acoust. Soc. Am. 49, 606–608. doi: 10.1121/1.1912396
Losiewicz, B. L. (1992). The Effect of Frequency on Linguistic Morphology. PhD thesis, University of Texas, Austin, TX.
Luce, P. A., and Pisoni, D. B. (1998). Recognizing spoken words: the neighborhood activation model. Ear Hear. 19, 1–36. doi: 10.1097/00003446-199802000-00001
Majewski, W., and Hollien, H. (1967). Formant frequency regions of Polish vowels. J. Acoust. Soc. Am. 42, 1031–1037. doi: 10.1121/1.1910685
Malisz, Z., and Wagner, P. (2012). Acoustic-phonetic realisation of Polish syllable prominence: A corpus study. Speech Lang. Technol. 14/15, 105–114.
Malisz, Z., and Żygis, M. (2018). “Lexical stress in Polish: evidence from focus and phrase-position differentiated production data,” in Proceedings of Speech Prosody 2018 (Poznań), 1008–1012.
Munson, B. (2007). “Lexical access, lexical representation, and vowel production,” in Laboratory Phonology 9, eds J. Cole and J. I. Hualde (Berlin: Mouton de Gruyter), 201–228.
Munson, B., and Solomon, N. P. (2004). The effect of phonological neighborhood density on vowel articulation. J. Speech Lang. Hear. Res. 47, 1048–1058. doi: 10.1044/1092-4388(2004/078)
New, B., Pallier, C., Ferrand, L., and Matos, R. (2001). Une base de données lexicales du français contemporain sur internet: LEXIQUE 3.80. L'Année Psychol. 101, 447–462. doi: 10.3406/psy.2001.1341
Oh, Y. M., Coupé, C., Marsico, E., and Pellegrino, F. (2015). Bridging phonological system and lexicon: insights from a corpus study of functional load. J. Phonet. 53, 153–176. doi: 10.1016/j.wocn.2015.08.003
Pate, J. K., and Goldwater, S. (2015). Talkers account for listener and channel characteristics to communicate efficiently. J. Mem. Lang. 78, 1–17. doi: 10.1016/j.jml.2014.10.003
Pellegrino, F., Coupé, C., and Marsico, E. (2011). A cross-language perspective on speech information rate. Language 87, 539–558. doi: 10.1353/lan.2011.0057
Piantadosi, S. T., Tily, H., and Gibson, E. (2011). Word lengths are optimized for efficient communication. Proc. Natl. Acad. Sci. U.S.A. 108, 3526–3529. doi: 10.1073/pnas.1012551108
Quené, H. (2008). Multilevel modeling of between-speaker and within-speaker variation in spontaneous speech tempo. J. Acoust. Soc. Am. 123, 1104–1113. doi: 10.1121/1.2821762
R Core Team (2017). R: A Language and Environment for Statistical Computing. R Foundation for Statistical Computing, Vienna, Austria.
Reichel, U. D., and Kisler, T. (2014). “Language-independent grapheme-phoneme conversion and word stress assignment as a web service,” in Elektronische Sprachverarbeitung, Studientexte zur Sprachkommunikation 71, ed R. Hoffmann (Dresden: TUDpress).
Schulz, E., Oh, Y. M., Malisz, Z., Andreeva, B., and Möbius, B. (2016). “Impact of prosodic structure and information density on vowel space size,” in Proceedings of Speech Prosody 2016, (Boston, MA), 350–354.
Schweitzer, A., and Möbius, B. (2004). “Exemplar-based production of prosody: evidence from segment and syllable durations,” in Proceedings of Speech Prosody 2004, (Nara), 459–462.
Schweitzer, K., Walsh, M., Möbius, B., Riester, A., Schweitzer, A., and Schütze, H. (2009). “Frequency matters: Pitch accents and information status,” in Proceedings of EACL 2009, (Athens), 728–736.
Schweitzer, K., Walsh, M., Möbius, B., and Schütze, H. (2010). “Frequency of occurrence effects on pitch accent realisation,” in Proceedings of Interspeech 2010, (Makuhari, Chiba), 138–141. doi: 10.18419/opus-2994
Sendlmeier, W. F., and Seebode, J. (2006). Formantkarten Des Deutschen Vokalsystems. Available online at: https://www.kw.tu-berlin.de/fileadmin/a01311100/Formantkarten_des_deutschen_Vokalsystems_01.pdf, retrieved on 1 September 2015.
Seyfarth, S. (2014). Word informativity influences acoustic duration: effects of contextual predictability on lexical representation. Cognition 133, 140–155. doi: 10.1016/j.cognition.2014.06.013
Shannon, C. E. (1948). A mathematical theory of communication. Bell Syst. Tech. J. 27, 379–423, 623–656. doi: 10.1002/j.1538-7305.1948.tb00917.x
Skarnitzl, R., and Volin, J. (2012). Reference values of vowel formants in young adult speakers of standard Czech. Akustické Listy 18, 7–11.
Sluijter, A. M., and van Heuven, V. J. (1995). Effects of focus distribution, pitch accent and lexical stress on the temporal organization of syllables in dutch. Phonetica 52, 71–89. doi: 10.1159/000262061
Sluijter, A. M., and Van Heuven, V. J. (1996). Spectral balance as an acoustic correlate of linguistic stress. J. Acoust. Soc. Am. 100, 2471–2485. doi: 10.1121/1.417955
Turk, A. (2010). Does prosodic constituency signal relative predictability? A smooth signal redundancy hypothesis. Lab. Phonol. 1, 227–262. doi: 10.1515/labphon.2010.012
Turk, A., and Shattuck-Hufnagel, S. (2014). Timing in talking: what is it used for, and how is it controlled? Philos. Trans. Roy. Soc. B Biol. Sci. 369:1658. doi: 10.1098/rstb.2013.0395
Turnbull, R., Burdin, R. S., Clopper, C. G., and Tonhauser, J. (2015). Contextual predictability and the prosodic realisation of focus: a cross-linguistic comparison. Lang. Cogn. Neurosci. 30, 1061–1076. doi: 10.1080/23273798.2015.1071856
Turner, G. S., Tjaden, K., and Weismer, G. (1995). The influence of speaking rate on vowel space and speech intelligibility for individuals with amyotrophic lateral sclerosis. J. Speech Hear. Res. 38, 1001–1013. doi: 10.1044/jshr.3805.1001
van Son, R., Bolotova, O., Pols, L. C., and Lennes, M. (2004). “Frequency effects on vowel reduction in three typologically different languages (Dutch, Finnish, Russian),” in Proceedings of Interspeech 2004, (Jeju Island), 1277–1280.
Van Son, R. J. J. H., and Pols, L. C. W. (2003). “How efficient is speech?” in Proc. Inst. Phonet. Sci. 25, 171–184.
van Son, R. J. J. H., and Pols, L. C. W. (1999). An acoustic profile of consonant reduction. Speech Commun. 28, 125–140. doi: 10.1016/S0167-6393(99)00009-6
van Son, R. J. J. H., and van Santen, J. P. H. (2005). Duration and spectral balance of intervocalic consonants: a case for efficient communication. Speech Commun. 47, 100–123. doi: 10.1016/j.specom.2005.06.005
Walsh, M., Möbius, B., Wade, T., and Schütze, H. (2010). Multi-level Exemplar Theory. Cogn. Sci. 34, 537–582. doi: 10.1111/j.1551-6709.2010.01099.x
Walsh, M., Schütze, H., Möbius, B., and Schweitzer, A. (2007). “An exemplar-theoretic account of syllable frequency effects,” in Proceedings of the International Congress of Phonetic Sciences, (Saarbrücken), 481–484.
Wedel, A., Nelson, N., and Sharp, R. (2018). The phonetic specificity of contrastive hyperarticulation in natural speech. J. Mem. Lang. 100, 61–88. doi: 10.1016/j.jml.2018.01.001
Weirich, M., and Simpson, A. P. (2014). Differences in acoustic vowel space and the perception of speech tempo. J. Phonet. 43, 1–10. doi: 10.1016/j.wocn.2014.01.001
Weiß, B. (2007). “Rate dependent vowel reduction in German,” in Proceedings of the 12th SPECOM, (Moscow), 300–305.
Whiteside, S. P., and Varley, R. A. (1998). “Dual-route phonetic encoding: some acoustic evidence,” in Proceedings of the 5th International Conference on Spoken Language Processing, (Sydney), 3155–3158.
Wiik, K. (1965). Finnish and English Vowels: A Comparison With Special Reference to the Learning Problems Met by Native Speakers of Finnish Learning English. PhD thesis, University of Turku.
Wright, R. (2004). “Factors of lexical competition in vowel articulation,” in Papers in Laboratory Phonology VI, eds J. J. Local, R. Ogden, and R. Temple (Cambridge: Cambridge University Press), 75–87.
Zeldes, A. (2008–2014). Automatic Phonetic Transcription and Syllable Analysis. Available online at: http://corpling.uis.georgetown.edu/amir/phon.php.
Keywords: speech rate, information density, surprisal, duration, vowel distinctiveness, spectral emphasis
Citation: Malisz Z, Brandt E, Möbius B, Oh YM and Andreeva B (2018) Dimensions of Segmental Variability: Interaction of Prosody and Surprisal in Six Languages. Front. Commun. 3:25. doi: 10.3389/fcomm.2018.00025
Received: 23 May 2017; Accepted: 30 May 2018;
Published: 20 July 2018.
Edited by:
Pia Knoeferle, Humboldt-Universität zu Berlin, GermanyReviewed by:
Rob Van Son, The Netherlands Cancer Institute (NKI), NetherlandsCopyright © 2018 Malisz, Brandt, Möbius, Oh and Andreeva. This is an open-access article distributed under the terms of the Creative Commons Attribution License (CC BY). The use, distribution or reproduction in other forums is permitted, provided the original author(s) and the copyright owner(s) are credited and that the original publication in this journal is cited, in accordance with accepted academic practice. No use, distribution or reproduction is permitted which does not comply with these terms.
*Correspondence: Zofia Malisz, bWFsaXN6QGt0aC5zZQ==
Disclaimer: All claims expressed in this article are solely those of the authors and do not necessarily represent those of their affiliated organizations, or those of the publisher, the editors and the reviewers. Any product that may be evaluated in this article or claim that may be made by its manufacturer is not guaranteed or endorsed by the publisher.
Research integrity at Frontiers
Learn more about the work of our research integrity team to safeguard the quality of each article we publish.