- Department of Civil and Environmental Engineering, Kyambogo University, Kampala, Uganda
Climate change impacts on the hydrology and water resources in East Africa require proper planning of regional adaptation measures. Past studies on the subject matter tended to focus on catchment instead of regional scale. To support actionable policy regarding regional planning of water resources management, this paper reviewed studies conducted in East Africa based on CMIP3, CMIP5, and CMIP6. Compared to the historical condition, temperature of East Africa over the mid- to late 21st century will increase by varying extents over the range 0.17–7°C with about 2.6°C on average. Future precipitation over East Africa will increase by 8.5% on average. Changes in discharge based on CMIP3, CMIP5, and CMIP6 will vary from −25.3 to +60.5%, −42.5 to 129%, and −23.4 to 69%, respectively. Ensemble means of the changes in discharge across the study area based on CMIP3, CMIP5, and CMIP6 will be by 25.2, 19.2, and 19.1%, respectively. Future peak river discharge across East Africa will increase by 8% on average. These results are important for planning regional climate change adaptation across East Africa. To support local or catchment adaptation, results of climate change impacts of temperature, precipitation and discharge of about 50 catchments across East Africa can be found summarized in this review paper. It is recommended that both regional and local policies or approaches should be considered for climate change adaptation to ensure a balanced, effective and sustainable framework for climate resilience in support of measures for tackling broad and specific needs cohesively.
1 Introduction
According to the Intergovernmental Panel on Climate Change (IPCC), various components of climate system are affected by the increasing emission of greenhouse gases (GHGs; IPCC, 2001, 2013, 2023). In fact, GHGs such as carbon dioxide, nitrous oxide and methane exist naturally in the atmosphere (IPCC, 2001). However, human activities are known to boost the accumulation of GHGs thereby leading to the alteration in the earth’s climate in terms of rising temperature, shifts in precipitation and snow patterns, as well as changes in the frequency and magnitude of hydrological extremes. Therefore, planning for water resources management should consider threats from the ongoing climate change (IPCC, 2013).
For this review paper, East Africa (Figure 1) was chosen as the study area because of the uniqueness of its complex regional climate mechanisms within the African continent. The East African region consists of complex circulation and topography in terms of some well-known highest mountains in Africa, the African Great Lakes, as well as the western and eastern rift valleys. Examples of lakes within this region include the Lake Victoria, Lake Edward, Lake Albert, Lake Tanganyika, Lake Tana, and Lake Turkana (Willis, 1938). With a maximum depth of 1,470 m, Lake Tanganyika is the deepest lake in Africa and the second deepest lake in the world (Verburga and Hecky, 2009). Lake Victoria is the largest fresh water lake in Africa. The study area also has the western rift valley (or the Albertine Rift) and eastern rift valley (or the Gregory rift; Beadle, 1968; Karp et al., 2012). These rift valleys consist of many lakes. For instance, Albert, Edward, Kivu and Tanganyika are in the western rift valley (Beadle, 1968). The eastern Gregory rift comprises Lakes such as Turkana, Nakuru, Baringo, Naivasha, Magadi. Furthermore, many of the few highest mountains in Africa are found within the Great Lakes region, for instance, Mt. Kilimanjaro (5,895 m), Mt. Kenya (5,199 m), Mt. Rwenzori (5,109 m), and Mt. Elgon (4,321 m; Onyutha et al., 2022; Osmaston, 2004). By altering the Walker circulation, the sea surface temperature from the Indian Ocean greatly influences the variation of precipitation across the East African region (Onyutha and Willems, 2015; Tierney et al., 2013). Influence from the Pacific Ocean in terms of the El Nino Southern Oscillation is also known to shape the variation of the short rains of the study area (Indeje et al., 2000). The general circulation across the study area is controlled by (Nicholson, 2000): (i) two air streams including the humid Congo airstream, and the Northeast and Southeast monsoons, and (ii) three convergence zones especially the Congo Air boundary, the Inter-Tropical Convergence Zone (ITCZ), and the zone that separates the dry, stable, Northerly flow of Saharan origin, and the moist Southerly flow. The pattern of the precipitation across the study area is dominantly influenced by the latitudinal migration of the ITCZ (Osman and Hastenrath, 1969). The migration of the ITCZ across the East African region is influenced by various regional and local factors such as mountains, lakes and rift valleys (Camberlin, 2009; Fraedrich, 1972; Onyutha et al., 2016). The circulations set up by the high mountains, and the Great Lakes affect the dynamics and stability of the atmosphere across the study area.
There have been several generations of climate models based on the respective IPCC reports (IPCC, 2001, 2013, 2023) and phases of the coupled model inter-comparison project (CMIP) including phase 3 (CMIP3; IPCC, 2001; Meehl et al., 2007), phase 5 (CMIP5; IPCC, 2013; Taylor et al., 2012), and phase 6 (CMIP6; Eyring et al., 2016; IPCC, 2023). Whereas scenarios of CMIP3 made use of the Greenhouse Gases Emissions Scenarios (GGES; IPCC, 2001; Meehl et al., 2007), those of CMIP5 considered Representative Concentration Pathways (RCP; IPCC, 2013; Taylor et al., 2012). GGES scenarios of CMIP3 included A2 (divided world), B1 (integrated and ecologically friendly), B2 (divided but ecologically friendly), and A1 (integrated world) and the detailed storylines can be found in Nakicenovic et al. (2000). CMIP5 scenarios included RCP2.6, RCP4.5, RCP6.0, and RCP8.5 and the storylines can be found in Van Vuuren et al. (2011). Scenarios of CMIP6 were based on Shared Socioeconomic Pathways (SSPs; Riahi et al., 2017). The SSP scenarios included SSP1 (taking the green road), SSP2 (middle of the road), SSP3 (a rocky road), SSP4 (a road divided), and SSP5 (taking the highway) (O’Neill et al., 2017).
Investigations of the climate change impacts on hydrology and water resources across East Africa (Figure 1) have been limited to catchment scale. For instance, some of the catchments considered in Ethiopia included Awash River catchment (Hailemariam, 1999), Kiltie catchment (Wubneh et al., 2023), the Lake Tana catchment (Taye et al., 2011), Megech catchment (Abebe and Kebede, 2017), and the Upper Blue Nile (Abay) River catchment (Mengistu et al., 2021). In Tanzania, Songwe catchment (Mwalwiba et al., 2023), Ngerengere catchment (Shagega et al., 2020), and Usangu catchment (Mollel et al., 2023) were considered. Furthermore, even within a particular country of the East Africa, studies tended to differ on the aspects of the water resources considered for analysis. For instance, in Tanzania, some studies focused on groundwater (Mollel et al., 2023), water demand (Kishiwa et al., 2018), and discharge (Mwalwiba et al., 2023; Näschen et al., 2019; Shagega et al., 2020). In Uganda, some researchers considered groundwater (Nyenje and Batelaan, 2009) while others analyzed discharge (Baraza, 2019; Nyeko-Ogiramoi, 2011). In summary, studies that considered the water resources of the entire East Africa in a climate changing context while taking into account the various generations of climate models are lacking.
This review paper is organized as follows. Section 2 deals with the methodology. Results and discussion can be found in section 3, and this is followed by conclusions. For clarity, the word discharge was used throughout the review paper instead of the various synonymous terms from the analysed articles such as runoff, flow, water yield, and streamflow. Here, water yield could be thought of in terms of the sum of lateral soil flow, surface runoff, and tile flow. Similarly, for simplicity, the word catchment was used instead of the various terms such as sub-basin, sub-catchment, basin, river basin, and watershed. Finally, the term precipitation was used in cases where the analysed studies considered rainfall.
2 Methodology
2.1 Exclusion and inclusion criteria
Articles from both open access and subscription journals were considered for analysis. Various databases such as ScienceDirect, Taylor & Francis online, Springer online, Wiley online, and Google Scholar were used to obtain required articles. Articles for review were sourced using abstract keywords such as climate change, East Africa, hydrology, water resources, and hydrological models. The Preferred Reporting Items for Systematic Reviews and Meta-Analyses (PRISMA; Moher et al., 2009) guidelines were used to obtain articles for analysis (Figure 2). The period for selecting a relevant article was relaxed to run from the 1990s to this year (2024). This period covered the various updates of the IPPC reports and generations of climate models considered in this study (IPCC, 1992, 1995, 2001, 2007, 2014, 2023).
Combinations of key words used to make searches in each database were:
i. “climate change impacts” and “water resources” and “country name,”
ii. “climate change impacts” and “hydrology” and “country name,”
iii. “climate change impacts” and “river flow” and “country name,”
iv. “climate change impacts” and “river discharge” and “country name,” and.
v. “climate change impacts” and “water balance” and “country name.”
Each of the bulleted combinations (i)-(v) was repeated for every country by changing “country name” to Uganda, Kenya, Rwanda, Burundi, Tanzania, and Ethiopia. To refine information for review, the search was limited to a few subdisciplines or subjects such as environmental management, environmental (general), environmental studies, water resources management, and hydrology. For other relevant information, simple searches especially in Google Scholar were made using the following keyword(s):
a. “IPCC climate change reports,”
b. “Hydrological modeling uncertainty,”
c. “Climate modeling uncertainty,”
d. “PRISMA in literature review,” and
e. “Water resources issues” and “transboundary water management” and “Nile basin.”
In fact, many studies investigated climate change impacts on only precipitation or evaporation but did not consider discharge. Such papers were all discarded since they were deemed to be ineligible on the ground that they did not consider discharge. Expressly, an article would qualify for inclusion if the study area fell within the East African region, and it contained results from a study that applied at least a hydrological model to some catchment(s) based on outputs of general circulation models (GCMs) or regional climate models (RCMs) from any of the three phases of CMIP especially phase 3 (CMIP3; IPCC, 2001), phase 5 (CMIP5; IPCC, 2013), and phase 6 (CMIP6; IPCC, 2023). Further criteria for qualifying an article for inclusion were linked to the clarity, elaborateness of the presented methodology, and the details of the results in line with the objective of this review. Other articles cited in this review were the seminal papers of the hydrological models applied in the reviewed studies.
2.2 Extraction of information
The process of extraction of relevant information for analysis started with the collection of the final set of articles identified based on the PRISMA guidelines (Moher et al., 2009). The pieces of information required from each article included method of bias correction, generation of climate models, hydrological models applied, change in precipitation, change in temperature, change in evaporation, change in hydrology, future water resources, catchment area, and country within East Africa. Data based on each criterion or piece of information from all the relevant articles was summarized in a data extraction table. Errors in the extracted information were checked, for instance, in terms of the order of magnitude of the values, and decimal places. During the data extraction step, if a particular article never provided most of the required pieces of information, it was excluded from analysis. Here, the numbers of excluded and included articles within the PRISMA flow chart were updated accordingly. The extracted information was summarized using tables or graphs with the subsequent analyses presented under various sub-sections of results and discussion.
3 Results and discussion
3.1 Publications based on CMIP3, CMIP5, and CMIP6
Table 1 shows overview of publications that applied climate models from CMIP3, CMIP5, and CMIP6. The number of studies varied among the countries. Rwanda had the lowest number of studies considered. This could be due to lack of observed hydro-meteorological data required for hydrological analysis of climate change impacts. The number of studies that used CMIP5 data was larger than that for CMIP3. Finally, few studies have so far used the CMIP6 data. This could be because CMIP6 was released recently.
Whereas it is difficult to directly compare projections under various sets of emission scenarios, it would be important for CMIP3-based hydrological information from a particular catchment to be evaluated with respect to the suitability of the adaptation measures. Here, focus could be given to the distant-past studies (such as those in the 1990s) that projected climate change signals over the current time horizon (for instance, the 2020s). The idea would be to evaluate, if the climate change adaptation measures based on the information from such studies were realistic or not with respect to economic viability, and safety. If a catchment was studied using some particular GCMs of the CMIP5, the findings could be updated using those similar GCMs of CMIP6. In this way, results based on CMIP6 will also need to be updated using the next phase of the CMIP. Such a procedure is important to reflect the increasing reliability of the climate change information in line with the improvements of the latest generation of climate models thereby allowing for revision or refinement of adaptation measures. To update climate change impacts for particular catchments, a project is required to conduct inventory of the relevant catchments studied to allow compilation of pertinent information to support respective or follow-up research studies.
3.2 Hydrological models applied in the analyzed articles
Table 2 shows overview of the models applied for hydrological analysis of climate change impact across East Africa. The various hydrological models fall under different categories. For instance, Nedbør-Afstrømnings-Model (NAM), Hydrological model focusing on sub-flows’ variation (HMSV), Veralgemeend Conceptueel Hydrologisch Model (VHM), TANK model, and Australian Water Balance Model (AWBM) are lumped conceptual models. They only require catchment-wide precipitation and potential evapotranspiration (PET) as meteorological inputs. These models have few parameters and are easy to calibrate. Other models such as Soil and Water Assessment Tool (SWAT), Water and Energy Transfer between Soil, Plants and Atmosphere (WetSpa) and the Hydrologic Engineering Center—Hydrologic Modeling System (HEC-HMS) are semi-distributed models. Distributed models are rarely applied in climate change context due to their huge data requirements and complexity in calibration. In the East African region, one distributed model known as Variable Infiltration Capacity (VIC) model (Liang et al., 1994) was recently applied by Gebrechorkos et al. (2023). Distributed models require both meteorological and spatial information. Meteorological inputs include gridded precipitation, minimum and maximum temperature, relative humidity, solar radiation, and wind speed. For instance, VIC was applied by Gebrechorkos et al. (2023) using 0.25° gridded station data and global runoff characteristics (Beck et al., 2015). Spatial information required by distributed models include soil map, topography (or DEM), and landuse and landcover (LULC) types.
Results of calibration and validation in many of the analyzed articles showed high values of the well-known “goodness-of-fit” metric Nash Sutcliffe efficiency (Nash and Sutcliffe, 1970) for both conceptual and semi-distributed models indicating good hydrological performance. Thus, application of satisfactorily performing conceptual models is sufficient especially when discharge at the catchment outlet is required. For applications that require spatial information on hydrology across the catchment, semi-distributed or process-based models notwithstanding their complexity can be applied.
Each of the analyzed reviewed papers except Baraza (2019) and Taye et al. (2011) did not apply two or more model. Performance of a hydrological model can vary from one catchment to another and this stems from the differences among catchments with respect to topography, weather, soil, and LULC types. Therefore, to consider the influence from the choice of a particular model, several hydrological models should be applied to a catchment. Just like for climate models where an ensemble mean is obtained, results of hydrological analysis based on various models can also be combined to obtain an ensemble.
3.3 Components of water resources analyzed
Table 3 shows the components of water resources considered in reviewed articles. Most studies focused on discharge while few researchers considered groundwater. This could be due to the complexity encountered in fully characterizing the response of groundwater to the changing climate given the influences from the human factors such as changes in LULC types and alarming exploitation of groundwater (Amanambu et al., 2020). Furthermore, the sensitivity of groundwater to changing climate is deemed to be less than that of surface water (Wada, 2016).
Surface water and groundwater (SWG) are closely linked. However, few studies separately analyzed groundwater (Abaho et al., 2011; Hersi et al., 2023; Mollel et al., 2023; Tigabu et al., 2021), and discharge (Gebremeskel and Kebede, 2018; Mengistu et al., 2021; Takele et al., 2022; Tilahun et al., 2023). Studies that analyzed SWG interactions across the East Africa are lacking. Generally, population growth, rapid industrialization and urbanization keep increasing pressure on discharge. This pressure, coupled with climate change impacts, will lead to substantial reduction in the volume of future discharge in various locations across the region. Thus, focus to meet the high-water demand will be turned to groundwater. If not carefully planned, the said focus will lead to considerable lowering of groundwater volume or table. In this line, a lot of effort is required from researchers in understanding and characterizing SWG interaction in a climate change context. This is vital to yield information that can support actionable policy towards developing regional adaptation measures.
Analysis of discharge can be linked to scale. Fine (e.g., daily) scale is required for analysis of hydrological extreme events. Analysis of coarse (e.g., monthly or seasonal) discharge can be relevant for planning agricultural practices. Changes in seasonal discharge can be indicative of the variation in rainy and dry seasons. An example of water resources applications that could depend on seasonal discharge is irrigation. However, rainfed cropping system is the commonest agricultural practice across East Africa. Nevertheless, the changes in soil moisture should guide in planning regional adaptation measures against late onset and early cessation of precipitation in support of agricultural practices (Ocen et al., 2021).
Water related catastrophes especially floods and droughts remain key hydrological challenges in East Africa (Ayugi et al., 2022; Foley, 2010; Fotso-Nguemo et al., 2019; Ngoma et al., 2022; Ongoma et al., 2018; Onyutha, 2020; Taye et al., 2015; Taye and Dyer, 2024). Floods and droughts are projected to increase in the study area (Gebrechorkos et al., 2023). Annual average daily discharge is projected to increase in various parts of the study area especially Uganda, Kenya and Tanzania within the White Nile region (Figure 3). For instance, future river discharge in Tanzania will increase by up to 25% (Gebrechorkos et al., 2023). However, in the same region like for Marabali catchment, annual average daily discharge is projected to decrease by -15.91% (Mutayoba et al., 2018). In the Blue Nile region, annual average daily discharge will increase by at least 5% in large rivers such as Awash and Bar Akobo, among others (Gebrechorkos et al., 2023). Analysis of hydrological extremes can guide in planning predictive adaptation measures against hydrology-related catastrophes such as flood and drought events. Effect of climate change on extreme low discharges should guide in planning for regulation of environmental discharge, and reservoir operations under future climatic conditions. Climate change impacts on floods should aid development of robust adaptation measures such as dams, dykes, early warning systems, flood plains, and evacuation plans.
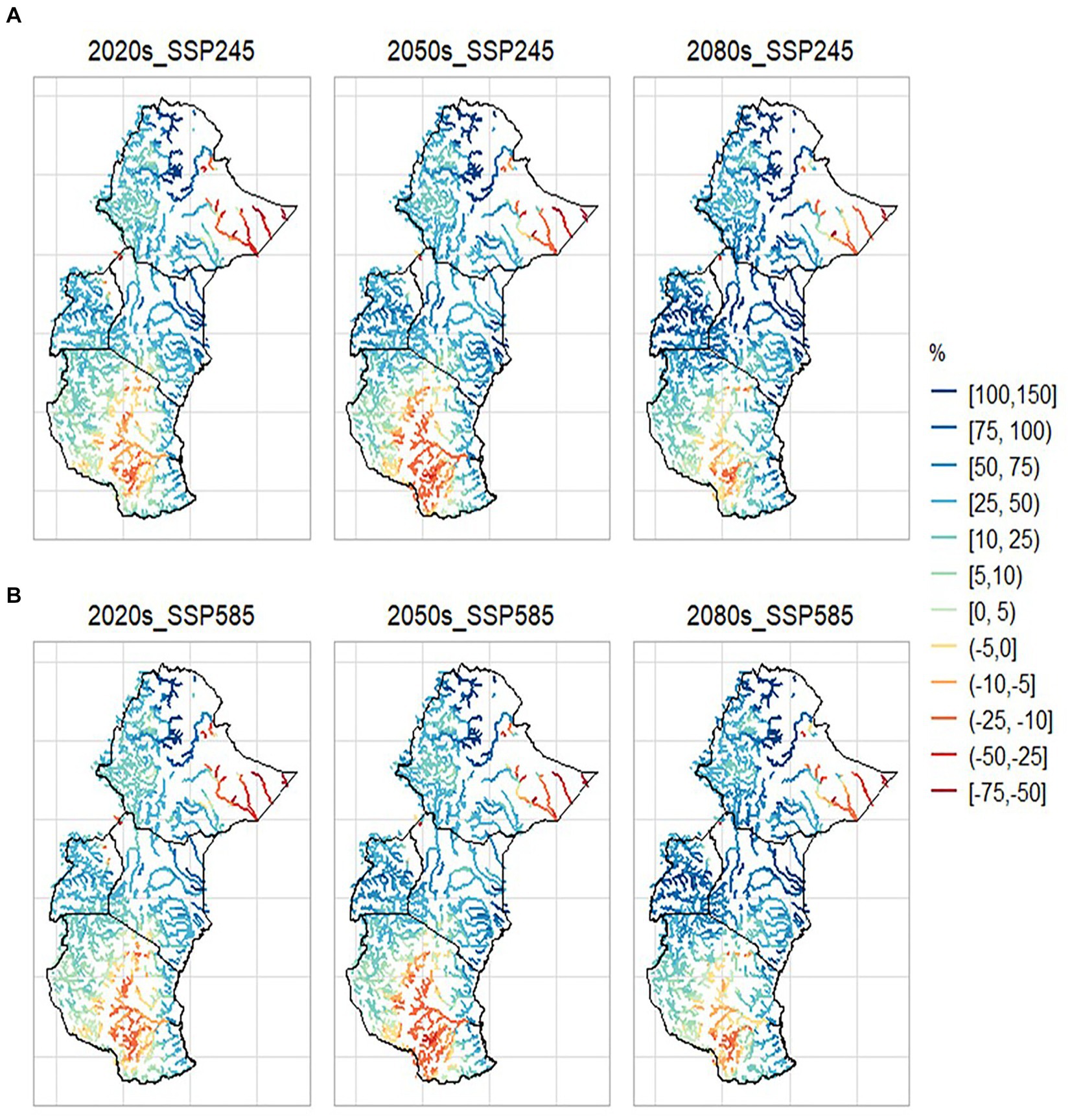
Figure 3. Future change in annual average daily discharge (%) based on (A) SSP245 and (B) SSP585 (source: Gebrechorkos et al., 2023).
3.4 Impacts of climate change on water resources components
Results of hydrological analyses can be found presented in Tables 4–7. For the Blue Nile region (Table 4), all the relevant articles showed that future temperature will be higher than that of the historical period by varying extents from 0.17 up to 6°C (with the average of 2.8°C). This information compiled from CMIP3, CMIP5, and CMIP6 agrees with the findings of a previous article (Adhikari et al., 2015) which showed using only CMIP3 that projections for temperature over the Blue Nile region will vary over the range 1.1–5.1°C with the mean of 2.7°C. For the catchments within the equatorial region (Tables 5–7) where the White Nile emanates from, temperature was again projected to generally increase by additional 2.5°C (over the range 0.9 to 7°C). Temperature projections based on only CMIP3 showed increases over the range 0.9 to 5.2°C (with the mean of 2.5°C; Adhikari et al., 2015).
On average, precipitation totals will increase over the Blue Nile and White Nile region by 10 and 7%, respectively. However, the ranges for the projected changes in precipitation over the Blue Nile and White Nile regions were (−43 to +191%) and (−61 to +100%), respectively. Previous review article (Adhikari et al., 2015) based on only CMIP3 showed that the precipitation over the Blue Nile and White Nile regions will on average increase by 10.5 and 6.4% and ranges of the projections will be (−6 to +42%) and (−15 to +42%), respectively.
Changes in temperature and precipitation affect river discharge. For instance, prolonged high temperatures with reduced precipitation imply hydrological drought. Due to climate change, monthly discharges will increase for some catchments and decrease in other areas. Even for a particular catchment, it remains possible that discharges will decrease in some months and increase for others. Previous earlier studies for instance based on CMIP3 showed the discharge will change to varying extents in the Blue Nile region. For a smaller sized catchment of the Lake Tana, discharge will decrease by −11.3% (Tarekegn and Tadege, 2006). For the Gilgel Abbay catchment, monthly discharge will change over the range −40 to 50% (Dile et al., 2013) and this could loosely approximate to the mean of 5%. Considering the entire Upper Nile, discharge will increase by 4% (Ungtae and Kaluarachchi, 2009). However, studies in the White Nile region did not focus on the monthly discharge.
Compared to the baseline conditions of different catchments across Uganda, past studies based on CMIP3 climate models showed that future recharge will increase by 27.5 and 80% (Abaho et al., 2011), and 60% (Nyenje and Batelaan, 2009), and 137% (Mileham et al., 2009). However according to Kingston and Taylor (2010), the recharge will decrease by −54%. In case the 137% is an outlier, the ensemble mean increase in recharge across Uganda becomes 28.4%. Relative to observed conditions, CMIP3-based changes in future surface runoff of various catchments across Uganda will be 60% (Nyenje and Batelaan, 2009), 53% (Mileham et al., 2009), 41% (Kingston and Taylor, 2010), −20% (Nyeko-Ogiramoi, 2011), and 27.5% (Abaho et al., 2011) and these lead to an ensemble mean of 23.6%.
In Ethiopia, analyses based on CMIP3 showed that peak discharge of the Megech catchment will increase to varying extents ranging from −17.47 to −30.58% (Abebe and Kebede, 2017). However, findings based on CMIP5 showed that the Upper Blue Nile will instead experience increase in the peak discharge over the range 10–50% (Aich et al., 2014). The percentage changes in discharge based on CMIP3 for the various catchments across Ethiopia were found to be in the ranges of −14 to −13% (Gebremeskel and Kebede, 2018), and −40 to 50% (Dile et al., 2013). Similarly, the changes considering CMIP5 were found to be in the ranges of 7 to 14.6% (Mengistu et al., 2021), −58 to 26% (Tigabu et al., 2021), −14.7 to −12.6% (Tilahun et al., 2023), −54 to −31% (Takele et al., 2022), and −54 to 26% (Tigabu et al., 2021). Thus, past studies showed that the ensemble mean changes in the discharge across Ethiopia based on CMIP3 and CMIP5 over 2050–2100 will be by −4.3 and −15.1%, respectively.
In Tanzania, analyses based on CMIP3 showed that discharge will increase on average by 30.1% (Shagega et al., 2020), and 51.5% (Kishiwa et al., 2018) thereby indicating an ensemble mean change of 40.8%. However, analyses based on CMIP5 showed that future discharge across Tanzania will change to varying extents such as −15.9% (Mutayoba et al., 2018), 23.5% (Mwalwiba et al., 2023), 9.7% (Näschen et al., 2019), and 40.4% (Adhikari et al., 2017). Thus, an ensemble mean change in future discharge based on CMIP5 will be by 14.4%. Future changes based on CMIP3 in discharge across Kenya will, on average, be by 45.5% (Taye et al., 2011), −25.3% (Mango et al., 2011), 60.5% (Wairimu, 2008), and 57.5% (Taye et al., 2011). Similarly, average changes based on CMIP5 will be 129% (Sood et al., 2017), and 68.6% (Musau et al., 2015). Analysis based on CMIP6 showed that the future discharge especially in the Narok County in Kenya will decrease by −23.4% on average (Opere et al., 2022). Based on CMIP5, increase of discharge in Ruvyironza-Kibaya and Muyinga catchments due to climate change will be by 44% on average (Rivas-López et al., 2022). However, analysis based on CMIP6 for the Ruvubu River basin showed that future discharge will increase by 35.2% on average (Kim et al., 2021).
For projections of peak discharge in the Blue Nile region from the mid- to late 21st century, the values of the mean of the minimum and maximum changes from each study were −28.5% (Abebe and Kebede, 2017), +20% (Ungtae and Kaluarachchi, 2009), and +30% (Aich et al., 2014) and this gives an average of +7.1%. The overall range of projections from the various studies of peak discharges was −30.58 to +60%. Considering the White Nile region from the mid- to late 21st century, the values of the mean of the minimum and maximum changes in peak discharge provided from each study were found to be −17.2% (Baraza, 2019), +45.15% (Baraza, 2019), +13.19% (Näschen et al., 2019), −17.5% (Paulo Mavaringana et al., 2023), −16.60% (Andersson et al., 2011), −25.30% (Andersson et al., 2011), +26.075% (Rivas-López et al., 2022). After averaging the two values from each of the two studies (Andersson et al., 2011; Baraza, 2019), the overall mean of the projections for peak discharge was +10%. The overall range of projections was from −34.1 to +86.25%.
Contrasting results on groundwater changes were obtained when different climate models were applied to the River Mitano catchment (Kingston and Taylor, 2010; Mileham et al., 2009). Thus, outputs of many climate models should be used to drive hydrological models and the ensemble mean of the results considered for analysis of future hydrological conditions. Other contrasting results were obtained in the study for River Rwizi catchment in which two hydrological models were applied. Whereas one hydrological model projected a decrease in extreme high discharge, the other model showed that there will be an increase in the high discharge quantile (Baraza, 2019). This could be explained in terms of the differences in the compatibility of hydrological models to meteorological and hydrological conditions of the catchment under consideration. This shows that the use of one hydrological model is characterized by the uncertainty due to the choice of the model (Onyutha, 2016). Therefore, it is recommended that many hydrological models should be applied in modeling climate change impacts of the same catchment and an ensemble mean is obtained by combining results of the models.
Figure 4 shows summary of changes in discharges of various catchments across the study area. The figure was obtained based on results for the various catchments and countries summarized in Tables 4–7. For each catchment, an average of the percentage changes in discharge was obtained (Figure 4A). The error bars comprise the minimum and maximum percentage changes (Figure 4A). In a few cases, the minimum and maximum changes (%) were not stipulated in the analyzed studies. For instance, the mean annual discharge of Ruvyironza-Kibaya and Muyinga will increase by 44% (Rivas-López et al., 2022). In such a case, the range comprising plus or minus 22% of the change (%) was assumed for constructing the error bars. The assumption was linked to the fact that the overall average of the mean changes for the various (or about 50) catchments considering CMIP3, CMIP5, and CMIP6 was 22%. Thus, for the change of 44%, the minimum and maximum values would become 44 ± (22/100 × 44) i.e., 34.32 and 53.68%, respectively. Considering all the catchments from past studies, the minimum, mean and maximum percentage changes (Figure 4B) were obtained. The error bars on the ensemble means (Figure 4B) comprise the standard deviations of the percentage changes. There were a total 14, 31, and 4 studies on various catchments based on climate models from CMIP3, CMIP5, and CMIP6, respectively. Based on a total of about 50 studies on various catchments, the mean changes in discharge based on CMIP3, CMIP5, and CMIP6 varied over the ranges −25.3 to +60.5%, −42.5 to 129%, and −23.4 to 69%, respectively. The ensemble of the mean changes in discharge across East Africa under future (2050–2,100) climatic conditions based on CMIP3, CMIP5, and CMIP6 were found to be 25.2, 19.2, 19.1%, respectively. The corresponding standard deviations of the percentage changes in discharge based on CMIP3, CMIP5, and CMIP6 were 33.0, 44.9, and 41.3%, respectively. The ranges of percentage changes indicate uncertainties on climate change impacts. They indicate that water resources management decisions tend to be made under large uncertainties on the findings of climate change impacts. To support actionable policies, the implications of uncertainties on climate change impacts on costs of measures required for relevant adaptation should be communicated. Finally, whereas compilation of results obtained using CMIP3, CMIP5, and CMIP6 takes into consideration the influence from the choice of a particular climate model generation, it should be noted that the derivations of future scenarios from the various phases of the CMIP were on different bases such as GGES, RCPs, and SSPs as presented in section 1. This makes direct comparison of results from the various phases of the CMIP impossible.
Ideally, the science of climate modeling aims at improving the models with respect to various aspects such as spatial resolution, validation, parameterizations, minimization of bias, and reduction of uncertainties in each new version of CMIP. In this context, CMIP5 would be expected to be more improved than CMIP3. Similarly, CMIP6 would expectedly be more improved than CMIP5. One factor that would hinder direct realization of such an improvement in latest phase of CMIP is lack of high-quality observed weather and climatic data against which outputs of climate models could be validated. Furthermore, the existence of several layers of uncertainties in hydrological modeling could also overshadow improvements of CMIP phases. Thus, a plausible way to check such improvement in CMIP phases would be with respect to the capacity of GCMs or RCMs of the current and previous generation of climate models to reproduce observed climatic variables such as precipitation. For instance, CMIP5 was found to perform better than CMIP3 in reproducing extreme precipitation events in the Lake Victoria basin in East Africa (Onyutha et al., 2016). Mean precipitation of East Africa was reproduced better by CMIP6 than CMIP5 climate models (Ayugi et al., 2021).
3.5 Bias correction or downscaling used
Climate models have coarse spatial resolution and this limits them from resolving local scale topographical and physiographic characteristics as well as granular atmospheric features (Onyutha et al., 2016). As a result, the outputs of the climate models tend to be generally biased and should first be bias-corrected before being applied for hydrological analysis of climate change impacts.
Several methods of bias correction exist including the delta method and quantile mapping (Mpelasoka and Chiew, 2009). Details as well as the advantages and disadvantages of the bias correction methods can be found in previous study (Onyutha et al., 2016). Outputs of the climate models can be estimated from the coarse (e.g., global) spatial scale to fine or local resolution through a process called downscaling. We have statistical and dynamical approaches of downscaling. In dynamical downscaling, RCM is nested into a GCM to refine the resolution of the outputs. Statistical downscaling consists of regression approach, weather generation, and weather classification.
Many methods of bias correction were applied in the reviewed studies. The delta method was applied for bias correction of precipitation scenarios in many studies (Adhikari and Nejadhashemi, 2016; Andersson et al., 2011; Baraza, 2019; Gumbo et al., 2021; Hailemariam, 1999; Hughes and Farinosi, 2020; Mileham et al., 2009; Musau et al., 2015; Wairimu, 2008). To bias-correct precipitation and temperature for driving hydrological models, quantile mapping was also applied in several studies (Adhikari et al., 2017; Adhikari and Nejadhashemi, 2016; Kim et al., 2021; Nyeko-Ogiramoi, 2011; Rivas-López et al., 2022; Taye et al., 2011; Tigabu et al., 2021; Tilahun et al., 2023; Umugwaneza et al., 2021; Wubneh et al., 2023). Trend preserving bias correction (Hempel et al., 2013) was applied by Aich et al. (2014). A common tool for bias correction is the CMhyd tool (https://swat.tamu.edu/software/cmhyd/ accessed: 28th Dec 2023) and it was applied on a number of occasions (Mfwango et al., 2022; Mutayoba et al., 2018; Takele et al., 2022).
For statistical downscaling, the SDSM tool (Wilby et al., 2002) was applied in six studies (Abebe and Kebede, 2017; Dile et al., 2013; Gebremeskel and Kebede, 2018; Mengistu et al., 2021; Nyenje and Batelaan, 2009; Takele et al., 2022). Most of the studies that applied the SDSM tool were found to have been conducted in catchments from Ethiopia. Bias correction using weather generators (such as LARS-WG and ClimGen) were applied in many studies (Kingston and Taylor, 2010; Kishiwa et al., 2018; Shagega et al., 2020; Tibangayuka et al., 2022). Statistical transfer function or linear scaling method was also applied in a few studies (Mollel et al., 2023; Mueni, 2016; Mwalwiba et al., 2023; Näschen et al., 2019; Sood et al., 2017). Finally, statistical downscaling using the bias correction-constructed analogs with quantile mapping reordering approach (Hiebert et al., 2018) was applied by Gebrechorkos et al. (2023).
The uncertainty in climate change information due to downscaling is of a magnitude that cannot be ignored especially in the study area (Gebrechorkos et al., 2023; Onyutha et al., 2016; Taye et al., 2015). This is due to lack of long-term and high-resolution observed climatic data that could be used for downscaling (Onyutha, 2018). This issue of lack of observed data is a difficult problem actually for the entire sub-Saharan Africa (Nikulin et al., 2012). An alternative is to use reanalysis downscaling to evaluate the performance of GCMs or RCMs. However, the inhomogeneity from temporal changes in the global observing system greatly affects the reanalysis products (Zhang et al., 2011). Reanalysis datasets tend to have reduced capacity in accurately capturing especially extreme climatic conditions. Thus, government of East Africa should invest in observed weather data collection through regular maintenance of recording or measurement equipment in the few available weather stations, and increasing the number or density of the weather stations across the study area.
3.6 Areas for improvements in future studies
3.6.1 The need to use many hydrological models
In each of the reviewed studies, only single hydrological model was applied. There were only two studies (Baraza, 2019; Taye et al., 2011) in each of which two models were applied. For Nyando catchment in Kenya, NAM (Madsen, 2000) and VHM (Willems, 2014) were applied by Taye et al. (2011). These two models were again applied by the same authors to the Lake Tana catchment in Ethiopia. The HMSV (Onyutha, 2019b) and AWBM (Boughton, 2004) were applied by Baraza (2019) for the Rwizi catchment in Uganda. The point is that the performance of various models applied to a given catchment can differ. Furthermore, performance of a single model can differ when applied to different catchments. Thus, application of many hydrological models to a particular catchment is recommended to even out the uncertainty due to the choice of a selected model.
3.6.2 The need for application of regional hydrological models
The analyzed articles mainly considered hydrological models applied to catchments. However, it is important for researchers to apply models that can provide regional hydrological information. For instance, Gebrechorkos et al. (2023) recently applied a distributed model referred to as VIC to entire East African region. Apart from VIC, many other models with capacities for applications for regional hydrological analysis exist such as improved SWAT version (Arnold et al., 1993, 1998), SWIM (Krysanova et al., 2015), mHM (Kumar et al., 2013), and HYPE (Lindström et al., 2010). Furthermore, building from the existing precipitation-runoff models, structures of models can be revised to increase their capacity for regional hydrological analysis. For instance, Hughes et al. (2006) modified the original version of Pitman (Pitman, 1973) for regional application using satellite data to provide insight on the surface water and groundwater interactions. For data-scarce regions, satellite data can be used along with the application of a theory-guided machine learning model (Hughes et al., 2023).
3.6.3 The use of non-changing LULC types
All the reviewed studies except (Wairimu, 2008) assumed constant LULC types under both current and future climatic conditions. This assumption may not be realistic for areas characterized by growing population, rapid urbanization and industrialization. Human factors generally affect hydrology of a catchment in a number of ways especially regarding the rates of evapotranspiration, infiltration and percolation. Thus, there is a need to project LULC types of a catchment when conducting hydrological analysis in a climate change context. Examples of distributed models which can be used in such a case include CLUE (Verburg et al., 1999), and MOLUSCE (NEXTGIS, 2023).
3.6.4 The need to update and validate results from past climate studies
There was no any single study among those reviewed that made use of at least two phases of the CMIP for hydrological modeling of the same catchment. Nevertheless, there were cases where two separate studies modeled the same catchment. For instance, the hydrology of River Rwizi catchment was modeled using both CMIP3 and CMIP5 (Baraza, 2019; Nyeko-Ogiramoi, 2011). Also the hydrology of the Gilgel Abay catchment was analyzed using both CMIP3 and CMIP5 (Dile et al., 2013; Tigabu et al., 2021). In any of these two cases, separate hydrological models were applied. For instance, Nyeko-Ogiramoi (2011) applied VHM while Baraza (2019) made use of the AWBM and HMSV. It was only in the case of the Gilgel Abay catchment when both studies applied SWAT (Dile et al., 2013; Tigabu et al., 2021). The point is that, some of the time horizons used in previous studies have either passed or are on-going. There were some studies of the 1990s that projected climate change impacts for the sub-periods 2010s, and 2020s. For instance, Hailemariam (1999) projected the discharge of the Awash River catchment to have reduced by −25% by 2010s compared to the climatic conditions of the 1970s and 1980s. Given that we are currently way after 2010, the question to answer would be on whether, indeed, the said decrease in the discharge was attained. Such an information would be important to determine if the adaptation measures in line with the findings of such a study were robust or should be adjusted in the context of adaptive water resources management under climate change. Therefore, for a particular catchment that was studied before, say, using the CMIP3, researchers need to apply the same hydrological model to update the climate change impacts based on newer or more recent generation of climate models. This will, in turn, permit updating of the adaptation measures planned in line with climate change impacts on hydrology and water resources.
3.6.5 The need for reporting on uncertainty analysis
Many of the reviewed studies did not elaborately quantify and report uncertainties on the hydrological results of climate change studies. There are various sources of uncertainties in modeling hydrological impacts of climate changes. Uncertainties are both in GCMs/RCMs and hydrological models. Uncertainties in hydrological models are due improper conceptualization of hydrological processes (Renard et al., 2010), errors in input data, and inaccuracies in parameter estimates during calibration. Specifically, calibration-related uncertainties stem from (a) errors on calibration data, e.g., observed discharge, (b) issues of initial and boundary conditions, and (c) the influence due to the choice of (i) method of calibration, (ii) objective function (Onyutha, 2024a), (iii) optimizers, and (iv) parameter sampling scheme (Onyutha, 2024b). Uncertainties in climate models are due to inter-model and intra-model variability, emission scenario, and internal variability (Dave et al., 2022). Uncertainties in climate models tend to be comparably large as those from hydrological models (Ludwig et al., 2009). Various steps in hydrological modeling of climate change impacts such as downscaling or bias correction, choice of hydrological models, and selection of climate models are characterized by uncertainties. Results from climate models can have large differences. In this line, it is acceptable to use ensemble mean of climate model results to even out the differences among the results from various GCMs or RCMs. However, bounds of uncertainties on the ensemble mean should also be provided. Implication of the model uncertainties on cost of water resources applications to be designed for adaptation to the climate change impacts should be discussed.
3.6.6 The need for integrating both regional and local approaches of dealing with water resources issues
Substantial influences of human activities such as changes of landscapes and discharge regulation negatively impact transboundary river systems (Trang et al., 2017) thereby resulting into issues of shared water resources allocation (Omer et al., 2023). Extents to which changes in landscape impact water resources vary among riparian countries. Whereas changes in LULC tend to take place upstream, the most serious impacts of the LULC on the regional water sources are felt downstream (e.g., the River Nile in this case; Omer et al., 2023). Studies that investigated impacts of LULC changes on hydrology in the East African region are mainly at catchment scales (Bewket and Sterk, 2005; Gebrehiwot et al., 2013; Guzha et al., 2018; Kizza et al., 2017; Mango et al., 2011; Mati et al., 2008; Mwangi et al., 2016). However, it is important to integrate the results of local scale changes in LULC types on discharge for understanding regional impacts of the landscape dynamics on transboundary river systems (Omer et al., 2023). In other words, instead of only catchment scale, issues of water resources could also be dealt with on a regional basis. This makes it possible to explore spatial dynamics of transboundary water resources (Senay et al., 2014). Examples of analyses that integrated results of the various spatial dynamics from different catchments to understand the regional water resources especially in the study area are few (Omer et al., 2023; Senay et al., 2014).
The regional water resources in the study area will be negatively impacted by a number of factors such as landscape dynamics, climate change, climate variability, and human interventions. For instance, landscape dynamics stemming from SSPs will expectedly lead to 3.6% decline of the future (2020–2060) annual River Nile discharge (Omer et al., 2023). Considering the two sources of the River Nile, analyses based on CMIP6 showed that the future annual discharge from the Equatorial Nile and the Eastern Nile will reduce by 8.4 and 8.9%, respectively (Omer et al., 2023). Generally, climate change will jeopardize the water security in the various sources of the River Nile (Batisha, 2023). The negative impacts of climate change on water resources in the East African region as summarized in this study indicate the need for regional water cooperation in developing adaptation measures. Cooperation in regional water management for the study area is a public good and can promote economic growth and regional stability while offering key win–win opportunities (Jägerskog et al., 2007).
For developing measures to adapt to the impacts of climate change, there should be programmes designed to maximize benefits from sharing the common water resources on regional basis. An example of such a programme that could be reinforced for climate change adaptation measures for the River Nile riparian countries is the Nile Basin Initiative (NBI) inaugurated in 1999. The six shared vision objectives of the NBI include Water security, Energy security, Food security, Environmental sustainability, Climate-change adaptation, and Transboundary water governance (NBI, 2021). Achievement of these objectives requires regional cooperation, water resources development, and water resources management. Many challenges, however, are expected in implementing the relevant initiatives or programmes such as the NBI including pressure from rapidly growing population, widespread household poverty, procedural and policies conflicts or tension stemming from the transboundary water management issues, and lack of relevant scientific knowledge on biophysical resources (Belay et al., 2010; Mohamed and Loulseged, 2008). Scientific research studies to generate information for regional predictive adaptation to the impacts of climate change, landscape dynamics, and climate variability of water resources of the East Africa should be prioritized (Mohamed and Loulseged, 2008; Omer et al., 2023).
Finally, despite the emphasis for regional adaptation for climate change in East Africa focusing on comprehensive coverage and coordinated management of transboundary resources, it is worth noting that local approaches are crucial for location-specific considerations. In other words, integrating both regional and local approaches is crucial for effectively addressing diverse challenges of water resources management in a climate change context. Regional strategies provide efficient resource allocation and unified policies but could risk overlooking specific local needs. Conversely, local adaptation allows for tailored, community-specific interventions and rapid implementation, although it can lead to fragmented efforts due to limited resources. Where necessary, combining regional policies with local actions should be considered to ensure a balanced, effective, and sustainable framework for climate resilience, with the focus of addressing both broad and specific needs cohesively.
4 Conclusion
East Africa comprises the Blue Nile and White Nile regions as the two sources of the River Nile. Over the 21st century, the average amount by which the temperature of the Blue Nile region will increase is comparable to White Nile region. However, the range over which the precipitation across the Blue Nile region (−43 − +191%) is projected to increase in future is far wider than that of the White Nile region (−61 − +100%). The change in the peak high river discharge in the Blue Nile and White Nile regions will be by +7.1% and +10%, respectively. Considering the entire study area, future temperature will increase by about 2.6°C on average with the changes varying from 0.17 to 7°C. Furthermore, future precipitation will increase by 8.5% on average. Consequentially, results of analyses from various studies based on CMIP3, CMIP5, and CMIP6 showed that the changes in discharges will vary from −25.3 to +60.5%, −42.5 to 129%, and −23.4 to 69%, thereby leading to the ensemble mean of 25.2, 19.2, 19.1%, respectively. Future peak river discharge across East Africa will increase by 8% on average.
To adjust the over reliance on surface water, policy should consider the use of both surface water and groundwater conjunctively in a climate change context. This was already demonstrated in South Africa especially for Cape Town in the context of climate variability, though (Millington and Scheba, 2021). Risk-based adaptation to the impacts of climate change on hydrological extremes should apply the integrated approach such as flood control infrastructure, and risk financing. Finally, incorporation of climate change findings from various scientists to support actionable policy in planning adaptation measures require science-policy interfacing (Onyutha, 2019a).
Many researchers modeled climate change impacts under the assumption of constant LULC types. This is unrealistic in areas typified by the impacts of human factors such as growing population, rapid urbanization and industrialization on hydrology. Thus, it is recommended that future hydrological conditions should be analyzed while using forecasted or projected or future LULC types.
It is also recommended that researchers should update information regarding climate change impacts using latest generation of climate models. The point is that some of the time horizons used in previous studies have passed or are on-going. There were some studies of the 1990s that projected climate change impacts for the sub-periods 2010s, and 2020s. Given that we are currently way after 2010, the question to answer would be on whether, indeed, the said decrease in the runoff was attained. This kind of information would be important to determine if the adaptation measures in line with the findings of such a study was robust or should be adjusted in the context of adaptive water resources management under climate change. Therefore, for a particular catchment that was studied before, say, using the CMIP3, researchers could apply the same hydrological model to update the climate change impacts based on newer or more recent generation of climate models. This will, in turn, permit updating of the adaptation measures planned in line with climate change impacts on hydrology and water resources.
Finally, in climate change impact investigations, various sources of uncertainties exist. Hydrological model uncertainties in each of the considered studies stem from flaws in conceptualization of hydrological processes, errors in observations, difficulties in the estimation of parameters. Furthermore, calibration-related uncertainties arise from the choice of (i) objective functions (Onyutha, 2024a), (ii) hydrological model, (iii) parameter sampling scheme (Onyutha, 2024b), (iv) method of calibration, and (v) optimization algorithm. Considering climate models, sources of uncertainty include the imperfections in representing physical processes in climate models, internal variability, and issues with the climate scenarios. Other uncertainties could be linked to the choice of (i) fixed of flexible model structure, bias correction and downscaling issues, and complexity in both climate and hydrological models. The extent to which the various uncertainty sources affected findings from studies reviewed varied among articles. However, the common practice of taking into account the various uncertainties while increasing reliability of the projections is the use the ensemble mean of the climate change signals.
Author contributions
CO: Writing – original draft, Writing – review & editing.
Funding
The author declares that no financial support was received for the research, authorship, and/or publication of this article.
Conflict of interest
The author declares that the research was conducted in the absence of any commercial or financial relationships that could be construed as a potential conflict of interest.
Publisher’s note
All claims expressed in this article are solely those of the authors and do not necessarily represent those of their affiliated organizations, or those of the publisher, the editors and the reviewers. Any product that may be evaluated in this article, or claim that may be made by its manufacturer, is not guaranteed or endorsed by the publisher.
References
Abaho, P., Amanda, B., Kigobe, M., Kizza, M., and Rugumayo, A. (2011). “Climate change and its impacts on river flows and recharge in the Sezibwa catchment, Uganda.” in Second International Conference on Advances in Engineering and Technology. pp. 572–578.
Abebe, E., and Kebede, A. (2017). Assessment of climate change impacts on the water resources of Megech River catchment, Abbay Basin, Ethiopia. Open J. Modern Hydrol. 7, 141–152. doi: 10.4236/ojmh.2017.72008
Adhikari, U., and Nejadhashemi, A. P. (2016). Impacts of climate change on water resources in Malawi. J. Hydrol. Eng. 21:05016026. doi: 10.1061/(ASCE)HE.1943-5584.0001436
Adhikari, U., Nejadhashemi, A. P., and Herman, M. R. (2015). A review of climate change impacts on water resources in East Africa. Trans. ASABE 58, 1493–1507. doi: 10.13031/trans.58.10907
Adhikari, U., Nejadhashemi, A. P., Herman, M. R., and Messina, J. P. (2017). Multiscale assessment of the impacts of climate change on water resources in Tanzania. J. Hydrol. Eng. 22:05016034. doi: 10.1061/(ASCE)HE.1943-5584.0001467
Aich, V., Liersch, S., Vetter, T., Huang, S., Tecklenburg, J., Hoffmann, P., et al. (2014). Comparing impacts of climate change on streamflow in four large African river basins. Hydrol. Earth Syst. Sci. 18, 1305–1321. doi: 10.5194/hess-18-1305-2014
Alemu, M. G., Wubneh, M. A., and Worku, T. A. (2022). Impact of climate change on hydrological response of mojo river catchment, awash river basin, Ethiopia. Geocarto Int. 38:2152497. doi: 10.1080/10106049.2022.2152497
Amanambu, A. C., Obarein, O. A., Mossa, J., Li, L., Ayeni, S. S., Balogun, O., et al. (2020). Groundwater system and climate change: present status and future considerations. J. Hydrol. 589:125163. doi: 10.1016/j.jhydrol.2020.125163
Andersson, L., Samuelsson, P., and Kjellström, E. (2011). Assessment of climate change impact on water resources in the Pungwe river basin. Tellus A: Dynam. Meteorol. Ocean. 63:138. doi: 10.1111/j.1600-0870.2010.00480.x
Arnold, J. G., Allen, P. M., and Bernhardt, G. (1993). A comprehensive surface-groundwater flow model. J. Hydrol. 142, 47–69. doi: 10.1016/0022-1694(93)90004-S
Arnold, J. G., Srinivasan, R., Muttiah, R. S., and Williams, J. R. (1998). Large area hydrologic modelling and assessment part I: model development. J. Am. Water Resour. Assoc. 34, 73–89. doi: 10.1111/j.1752-1688.1998.tb05961.x
Ayugi, B., Jiang, Z., Iyakaremye, V., Ngoma, H., Babaousmail, H., Onyutha, C., et al. (2022). East African population exposure to precipitation extremes under 1.5°C and 2.0°C warming levels based on CMIP6 models. Environ. Res. Lett. 17:044051. doi: 10.1088/1748-9326/ac5d9d
Ayugi, B., Zhihong, J., Zhu, H., Ngoma, H., Babaousmail, H., Rizwan, K., et al. (2021). Comparison of CMIP6 and CMIP5 models in simulating mean and extreme precipitation over East Africa. Int. J. Climatol. 41, 6474–6496. doi: 10.1002/joc.7207
Baraza, G. (2019). Assessing impacts of climate change on hydrological extremes in Rwizi catchment [Kyambogo University]. Available at: https://kyuspace.kyu.ac.ug/handle/20.500.12504/550 (Accessed September 08, 2024).
Batisha, A. (2023). A lighthouse to enhance the quality of life in the Nile River basin. Environ. Econ. Policy Stud. doi: 10.1007/s10018-023-00380-2
Beck, H. E., De Roo, A., and Van Dijk, A. I. J. M. (2015). Global maps of streamflow characteristics based on observations from several thousand catchments*. J. Hydrometeorol. 16, 1478–1501. doi: 10.1175/JHM-D-14-0155.1
Belay, A. A., Semakula, H. M., Wambura, G. J., and Jan, L. (2010). SWOT analysis and challenges of Nile Basin initiative: an integrated water resource management perspective. Chin. J. Popul. Resour. Environ. 8, 8–17. doi: 10.1080/10042857.2010.10684960
Bergstrom, S. (1995). “The HBV model” in Computer models of watershed hydrology (Highlands Ranch, CO: Water Resources Publications), 443–476.
Bewket, W., and Sterk, G. (2005). Dynamics in land cover and its effect on stream flow in the Chemoga watershed, Blue Nile basin, Ethiopia. Hydrol. Process. 19, 445–458. doi: 10.1002/hyp.5542
Boughton, W. (2004). The Australian water balance model. Environ. Model. Softw. 19, 943–956. doi: 10.1016/j.envsoft.2003.10.007
Camberlin, P. (2009). “Nile Basin Climates” in The Nile. ed. H. J. Dumont, vol. 89 (Netherlands: Springer), 307–333.
Dave, S., Belanger, J., Anis, M., Basu, S., and Stewart, S. (2022). Understanding and accommodating uncertainty in climate change data: a climate west primer. Available at: https://climatewest.ca/2022/09/27/uncertainty-101-understanding-climate-models/ (Accessed September 08, 2024).
Dile, Y. T., Berndtsson, R., and Setegn, S. G. (2013). Hydrological response to climate change for Gilgel Abay River, in the Lake Tana Basin—Upper Blue Nile Basin of Ethiopia. PLoS One 8:e79296. doi: 10.1371/journal.pone.0079296
Eder, G., Fuchs, M., Nachtnebel, H., and Loibl, W. (2005). Semi-distributed modelling of the monthly water balance in an alpine catchment. Hydrol. Process. 19, 2339–2360. doi: 10.1002/hyp.5888
Eyring, V., Bony, S., Meehl, G. A., Senior, C. A., Stevens, B., Stouffer, R. J., et al. (2016). Overview of the coupled model intercomparison project phase 6 (CMIP6) experimental design and organization. Geosci. Model Dev. 9, 1937–1958. doi: 10.5194/gmd-9-1937-2016
Foley, A. M. (2010). Uncertainty in regional climate modelling: a review. Prog. Phys. Geogra. Earth Environ. 34, 647–670. doi: 10.1177/0309133310375654
Fotso-Nguemo, T. C., Diallo, I., Diakhaté, M., Vondou, D. A., Mbaye, M. L., Haensler, A., et al. (2019). Projected changes in the seasonal cycle of extreme rainfall events from CORDEX simulations over Central Africa. Clim. Chang. 155, 339–357. doi: 10.1007/s10584-019-02492-9
Fraedrich, K. (1972). A simple climatological model of the dynamics and energetics of the nocturnal circulation at Lake Victoria. Q. J. R. Meteorol. Soc. 98, 322–335. doi: 10.1002/qj.49709841606
Gebrechorkos, S. H., Taye, M. T., Birhanu, B., Solomon, D., and Demissie, T. (2023). Future changes in climate and hydroclimate extremes in East Africa. Earth’s. Future 11:e2022EF003011. doi: 10.1029/2022EF003011
Gebrehiwot, S. G., Seibert, J., Gärdenäs, A. I., Mellander, P.-E., and Bishop, K. (2013). Hydrological change detection using modeling: half a century of runoff from four rivers in the Blue Nile Basin: hydrological change detection using modeling. Water Resour. Res. 49, 3842–3851. doi: 10.1002/wrcr.20319
Gebremeskel, G., and Kebede, A. (2018). Estimating the effect of climate change on water resources: integrated use of climate and hydrological models in the Werii watershed of the Tekeze river basin, northern Ethiopia. Agric. Nat. Resour. 52, 195–207. doi: 10.1016/j.anres.2018.06.010
Gumbo, A. D., Kapangaziwiri, E., Chikoore, H., Pienaar, H., and Mathivha, F. (2021). Assessing water resources availability in headwater sub-catchments of Pungwe River basin in a changing climate. J. Hydrol. Reg. Stud. 35:100827. doi: 10.1016/j.ejrh.2021.100827
Guzha, A. C., Rufino, M. C., Okoth, S., Jacobs, S., and Nóbrega, R. L. B. (2018). Impacts of land use and land cover change on surface runoff, discharge and low flows: evidence from East Africa. J. Hydrol. Reg. Stud. 15, 49–67. doi: 10.1016/j.ejrh.2017.11.005
Hailemariam, K. (1999). Impact of climate change on the water resources of Awash River basin, Ethiopia. Clim. Res. 12, 91–96. doi: 10.3354/cr012091
HEC-HMS (2000). Hydrologic Modeling System, HEC-HMS. Technical Reference Mannual. US Army Corps of Engineers.
Hempel, S., Frieler, K., Warszawski, L., Schewe, J., and Piontek, F. (2013). A trend-preserving bias correction – the ISI-MIP approach. Earth Syst. Dynam. 4, 219–236. doi: 10.5194/esd-4-219-2013
Hersi, N. A. M., Mulungu, D. M. M., and Nobert, J. (2023). Groundwater recharge estimation under changing climate and land use scenarios in a data-scarce Bahi (Manyoni) catchment in internal Drainage Basin (IDB), Tanzania using soil and water assessment tool (SWAT). Groundw. Sustain. Dev. 22:100957. doi: 10.1016/j.gsd.2023.100957
Hiebert, J., Murdock, T., Sobie, S., and Werner, A. (2018). ClimDown: climate downscaling in R. J. Open Source Softw. 3:360. doi: 10.21105/joss.00360
Hughes, D. A., Andersson, L., Wilk, J., and Savenije, H. H. G. (2006). Regional calibration of the Pitman model for the Okavango River. J. Hydrol. 331, 30–42. doi: 10.1016/j.jhydrol.2006.04.047
Hughes, D. A., and Farinosi, F. (2020). Assessing development and climate variability impacts on water resources in the Zambezi River basin. Simulating future scenarios of climate and development. Journal of hydrology. Reg. Stud. 32:100763. doi: 10.1016/j.ejrh.2020.100763
Hughes, D. A., Read, L., Jeuland, M., Kapangaziwiri, E., Elkurdy, M., Lambl, D., et al. (2023). Insights from a comparison of two hydrological modelling approaches in the Kwando (Cuando) river and the western tributaries of the Zambezi River basin. J. Hydrol. Reg. Stud. 48:101482. doi: 10.1016/j.ejrh.2023.101482
Indeje, M., Semazzi, F. H. M., and Ogallo, L. J. (2000). ENSO signals in east African rainfall seasons. Int. J. Climatol. 20, 19–46. doi: 10.1002/(SICI)1097-0088(200001)20:1<19::AID-JOC449>3.0.CO;2-0
IPCC (1992). IPCC supplement: Scientific assessment of climatic change. Report prepared by working group I, WMO-UNEP. Houghton, J.T., Callander, B.A. and Varney, S., eds. Cambridge University Press. Intergovernmental Panel on Climate Change (IPCC).
IPCC (1995). Climate change 1995: Impacts, adaptations, and mitigation. Summary for policymakers. Geneva: WMO/UNEP (p. 588).
IPCC (2001). Climate Change 2001: The Scientific Basis. Contribution of Working Group I to the Third Assessment Report of the Intergovernmental Panel on Climate Change. Houghton, J.T., Y. Ding, D.J. Griggs, M. Noguer, P.J. Van der Linden, X. Dai, K. Maskell, and C.A. Johnson (eds.). Cambridge University Press.
IPCC (2007). Climate Change 2007: The Physical Science Basis. Contribution of Working Group I to the Fourth Assessment Report of the Intergovernmental Panel on Climate Change. Solomon, S., D. Qin, M. Manning, Z. Chen, M. Marquis, K.B. Averyt, M.Tignor and H.L. Miller (eds.). Cambridge University Press, Cambridge, United Kingdom and New York, NY, USA. IPCC.
IPCC (2013). Climate Change 2013: The Physical Science Basis. Contribution of Working Group I to the Fifth Assessment Report of the IPCC. Cambridge University Press.
IPCC (2014). Climate Change 2014: Synthesis Report. Contribution of Working Groups I, II and III to the Fifth Assessment Report of the Intergovernmental Panel on Climate Change [Core Writing Team, R.K. Pachauri and L.A. Meyer (eds.)]. IPCC.
IPCC (2023). Climate Change 2023: Synthesis Report. Contribution of Working Groups I, II and III to the Sixth Assessment Report of the Intergovernmental Panel on Climate Change [Core Writing Team, H. Lee and J. Romero (eds.)]. IPCC, Geneva, Switzerland. (First). Intergovernmental Panel on Climate Change (IPCC).
Jägerskog, A., Granit, J., Risberg, A., and Yu, W. (2007). Transboundary water management as a regional public good. Financing Development – An Example from the Nile Basin. Report Nr. 20. SIWI, Stockholm.
Karp, T., Scholz, C. A., and McGlue, M. M. (2012). “Structure and stratigraphy of the Lake Albert rift, east AfricaObservations from seismic reflection and gravity data” in Lacustrine Sandstone Reservoirs and Hydrocarbon Systems. eds. O. W. Baganz, Y. Bartov, K. M. Bohacs, and D. Nummedal (American Association of Petroleum Geologists).
Kim, J.-B., Habimana, J. D. D., Kim, S.-H., and Bae, D.-H. (2021). Assessment of climate change impacts on the Hydroclimatic response in Burundi based on CMIP6 ESMs. Sustain. For. 13:12037. doi: 10.3390/su132112037
Kingston, D. G., and Taylor, R. G. (2010). Sources of uncertainty in climate change impacts on river discharge and groundwater in a headwater catchment of the upper Nile Basin, Uganda. Hydrol. Earth Syst. Sci. 14, 1297–1308. doi: 10.5194/hess-14-1297-2010
Kishiwa, P., Nobert, J., Kongo, V., and Ndomba, P. (2018). Assessment of impacts of climate change on surface water availability using coupled SWAT and WEAP models: case of upper Pangani River basin, Tanzania. Proc. Int. Assoc. Hydrol. Sci. 378, 23–27. doi: 10.5194/piahs-378-23-2018
Kizza, C. L., Tenywa, M. M., Majaliwa, J. G. M., Kansiime, F., Magunda, M., Nakileza, B., et al. (2017). Land use/cover change patterns in highland ecosystems of Lake Bunyonyi catchment in western Uganda. Afr. Crop. Sci. J. 25:43. doi: 10.4314/acsj.v25i1.4S
Krysanova, V., Hattermann, F., Huang, S., Hesse, C., Vetter, T., Liersch, S., et al. (2015). Modelling climate and land-use change impacts with SWIM: lessons learnt from multiple applications. Hydrol. Sci. J. 60, 606–635. doi: 10.1080/02626667.2014.925560
Kumar, R., Samaniego, L., and Attinger, S. (2013). Implications of distributed hydrologic model parameterization on water fluxes at multiple scales and locations. Water Resour. Res. 49, 360–379. doi: 10.1029/2012WR012195
Liang, X., Lettenmaier, D. P., Wood, E. F., and Burges, S. J. (1994). A simple hydrologically based model of land surface water and energy fluxes for general circulation models. J. Geophys. Res. Atmos. 99, 14415–14428. doi: 10.1029/94JD00483
Lindström, G., Pers, C., Rosberg, J., Strömqvist, J., and Arheimer, B. (2010). Development and testing of the HYPE (hydrological predictions for the environment) water quality model for different spatial scales. Hydrol. Res. 41, 295–319. doi: 10.2166/nh.2010.007
Liu, Y. B., De Smedt, F., Hoffmann, L., and Pfister, L. (2005). Assessing land use impacts on flood processes in complex terrain by using GIS and modeling approach. Environ. Model. Assess. 9, 227–235. doi: 10.1007/s10666-005-0306-7
Ludwig, R., May, I., Turcotte, R., Vescovi, L., Braun, M., Cyr, J.-F., et al. (2009). The role of hydrological model complexity and uncertainty in climate change impact assessment. Adv. Geosci. 21, 63–71. doi: 10.5194/adgeo-21-63-2009
Madsen, H. (2000). Automatic calibration of a conceptual rainfall–runoff model using multiple objectives. J. Hydrol. 235, 276–288. doi: 10.1016/S0022-1694(00)00279-1
Mango, L. M., Melesse, A. M., McClain, M. E., Gann, D., and Setegn, S. G. (2011). Land use and climate change impacts on the hydrology of the upper Mara River basin, Kenya: results of a modeling study to support better resource management. Hydrol. Earth Syst. Sci. 15, 2245–2258. doi: 10.5194/hess-15-2245-2011
Mati, B. M., Mutie, S., Gadain, H., Home, P., and Mtalo, F. (2008). Impacts of land-use/cover changes on the hydrology of the transboundary Mara River, Kenya/Tanzania. Lakes Reserv. Sci. Policy Manag. Sustain. Use 13, 169–177. doi: 10.1111/j.1440-1770.2008.00367.x
Meehl, G. A., Covey, C., Delworth, T., Latif, M., McAvaney, B., Mitchell, J. F. B., et al. (2007). THE WCRP CMIP3 multimodel dataset: a new era in climate change research. Bull. Am. Meteorol. Soc. 88, 1383–1394. doi: 10.1175/BAMS-88-9-1383
Mehdi, B., Dekens, J., and Herrnegger, M. (2021). Climatic impacts on water resources in a tropical catchment in Uganda and adaptation measures proposed by resident stakeholders. Clim. Chang. 164:10. doi: 10.1007/s10584-021-02958-9
Mengistu, D., Bewket, W., Dosio, A., and Panitz, H.-J. (2021). Climate change impacts on water resources in the upper Blue Nile (Abay) river basin, Ethiopia. J. Hydrol. 592:125614. doi: 10.1016/j.jhydrol.2020.125614
Mfwango, L. H., Ayenew, T., and Mahoo, H. F. (2022). Impacts of climate and land use/cover changes on streamflow at Kibungo sub-catchment, Tanzania. Heliyon 8:e11285. doi: 10.1016/j.heliyon.2022.e11285
Mileham, L., Taylor, R. G., Todd, M., Tindimugaya, C., and Thompson, J. (2009). The impact of climate change on groundwater recharge and runoff in a humid, equatorial catchment: sensitivity of projections to rainfall intensity. Hydrol. Sci. J. 54, 727–738. doi: 10.1623/hysj.54.4.727
Millington, N., and Scheba, S. (2021). Day zero and the infrastructures of climate change: water governance, inequality, and infrastructural politics in Cape Town’s water crisis. Int. J. Urban Reg. Res. 45, 116–132. doi: 10.1111/1468-2427.12899
Mohamed, Y. A., and Loulseged, M. (2008). The Nile Basin water resources: Overview of key research questions pertinent to the Nile Basin initiative. Colombo, Sri Lanka: International Water Management Institute. IWMI Working Paper 127. p. 34.
Moher, D., Liberati, A., Tetzlaff, J., and Altman, D. (2009). Preferred reporting items for systematic reviews and Meta-analyses: the PRISMA statement. PLoS Med. 6:e1000097. doi: 10.1371/journal.pmed.1000097
Mollel, G. R., Mulungu, D. M. M., Nobert, J., and Alexander, A. C. (2023). Assessment of climate change impacts on hydrological processes in the Usangu catchment of Tanzania under CMIP6 scenarios. J. Water Clim. Change 14, 4162–4182. doi: 10.2166/wcc.2023.542
Mpelasoka, F. S., and Chiew, F. H. S. (2009). Influence of rainfall scenario construction methods on runoff projections. J. Hydrometeorol. 10, 1168–1183. doi: 10.1175/2009JHM1045.1
Mueni, P. J. (2016). Climate change impacts on water resources over the upper Tana catchment of Kenya [university of Nairobi]. Available at: http://hdl.handle.net/11295/97575 (Accessed April 04, 2024).
Musau, J., Sang, J., Gathenya, J., and Luedeling, E. (2015). Hydrological responses to climate change in Mt. Elgon watersheds. J. Hydrol. Reg. Stud. 3, 233–246. doi: 10.1016/j.ejrh.2014.12.001
Mutayoba, E., Kashaigili, J. J., Kahimba, F. C., Mbungu, W., and Chilagane, N. A. (2018). Assessment of the impacts of climate change on hydrological characteristics of the Mbarali River sub catchment using high resolution climate simulations from CORDEX regional climate models. Appl. Phys. Res. 10:61. doi: 10.5539/apr.v10n5p61
Mwalwiba, L. G., Kifanyi, G. E., Mutayoba, E., Ndambuki, J. M., and Chilagane, N. (2023). Assessment of climate Change’s impacts on river flows in the Songwe Sub-Basin. Open J. Modern Hydrol. 13, 141–164. doi: 10.4236/ojmh.2023.132008
Mwangi, H. M., Julich, S., Patil, S. D., McDonald, M. A., and Feger, K. (2016). Modelling the impact of agroforestry on hydrology of Mara River basin in East Africa. Hydrol. Process. 30, 3139–3155. doi: 10.1002/hyp.10852
Nakicenovic, N., Alcamo, J., Davis, G., Vries, B. D., Fenhann, J. V., Gaffin, S., et al. (2000). Special report on emissions scenarios. Special report of Working Group III of the Intergovernmental Panel on Climate Change. Cambridge University Press.
Näschen, K., Diekkrüger, B., Leemhuis, C., Seregina, L., and Van Der Linden, R. (2019). Impact of climate change on water resources in the Kilombero catchment in Tanzania. Water 11:859. doi: 10.3390/w11040859
Nash, J. E., and Sutcliffe, J. V. (1970). River flow forecasting through conceptual models part I — a discussion of principles. J. Hydrol. 10, 282–290. doi: 10.1016/0022-1694(70)90255-6
NBI (2021). State of the River Nile Basin; water security in the Nile Basin 2021. Nile Basin initiative secretariat (NBI), p. 288. Available at: https://adelphi.de/system/files/document/State%20of%20Basin%202021.pdf (Accessed April 28, 2024).
NEXTGIS (2023). MOLUSCE—quick and convenient analysis of land cover changes. Available at: https://nextgis.com/blog/molusce/ (Accessed July 06, 2024).
Ngoma, H., Ayugi, B., Onyutha, C., Babaousmail, H., Lim, K., Iyakaremye, V., et al. (2022). Projected changes in rainfall over Uganda based on CMIP6 models. Theor. Appl. Climatol. 149, 1117–1134. doi: 10.1007/s00704-022-04106-4
Nicholson, S. (2000). The nature of rainfall variability over Africa on time scales of decades to millenia. Glob. Planet. Chang. 26, 137–158. doi: 10.1016/S0921-8181(00)00040-0
Nikulin, G., Jones, C., Giorgi, F., Asrar, G., Büchner, M., Cerezo-Mota, R., et al. (2012). Precipitation climatology in an ensemble of CORDEX-Africa regional climate simulations. J. Clim. 25, 6057–6078. doi: 10.1175/JCLI-D-11-00375.1
Nyeko-Ogiramoi, P. (2011). Climate change impacts on hydrological extremes and water resources in Lake Victoria catchments, upper Nile basin [Katholieke Universiteit Leuven]. Available at: https://lirias.kuleuven.be/retrieve/164653 (Accessed June 04, 2024).
Nyenje, P. M., and Batelaan, O. (2009). Estimating the effects of climate change on groundwater recharge and baseflow in the upper Ssezibwa catchment, Uganda. Hydrol. Sci. J. 54, 713–726. doi: 10.1623/hysj.54.4.713
O’Neill, B. C., Kriegler, E., Ebi, K. L., Kemp-Benedict, E., Riahi, K., Rothman, D. S., et al. (2017). The roads ahead: narratives for shared socioeconomic pathways describing world futures in the 21st century. Glob. Environ. Chang. 42, 169–180. doi: 10.1016/j.gloenvcha.2015.01.004
Ocen, E., De Bie, C. A. J. M., and Onyutha, C. (2021). Investigating false start of the main growing season: a case of Uganda in East Africa. Heliyon 7:e08428. doi: 10.1016/j.heliyon.2021.e08428
Omer, A., Yuan, X., and Gemitzi, A. (2023). Transboundary Nile basin dynamics: land use change, drivers, and hydrological impacts under socioeconomic pathways. Ecol. Indic. 153:110414. doi: 10.1016/j.ecolind.2023.110414
Ongoma, V., Chen, H., Gao, C., Nyongesa, A. M., and Polong, F. (2018). Future changes in climate extremes over equatorial East Africa based on CMIP5 multimodel ensemble. Nat. Hazards 90, 901–920. doi: 10.1007/s11069-017-3079-9
Onyutha, C. (2016). Influence of hydrological model selection on simulation of moderate and extreme flow events: a case study of the Blue Nile Basin. Adv. Meteorol. 2016, 1–28. doi: 10.1155/2016/7148326
Onyutha, C. (2018). Trends and variability in African long-term precipitation. Stoch. Env. Res. Risk A. 32, 2721–2739. doi: 10.1007/s00477-018-1587-0
Onyutha, C. (2019a). African food insecurity in a changing climate: the roles of science and policy. Food Energy Secur. 8:e00160. doi: 10.1002/fes3.160
Onyutha, C. (2019b). Hydrological model supported by a step-wise calibration against sub-flows and validation of extreme flow events. Water 11:244. doi: 10.3390/w11020244
Onyutha, C. (2020). Analyses of rainfall extremes in East Africa based on observations from rain gauges and climate change simulations by CORDEX RCMs. Clim. Dyn. 54, 4841–4864. doi: 10.1007/s00382-020-05264-9
Onyutha, C. (2024a). Pros and cons of various efficiency criteria for hydrological model performance evaluation. Proc. IAHS 385, 181–187. doi: 10.5194/piahs-385-181-2024
Onyutha, C. (2024b). Randomized block quasi-Monte Carlo sampling for generalized likelihood uncertainty estimation. Hydrol. Res. 55, 319–335. doi: 10.2166/nh.2024.136
Onyutha, C., Ayugi, B., Tabari, H., Ngoma, H., and Ongoma, V. (2022). “Drought across East Africa under climate variability” in Climate impacts on extreme weather (Elsevier), 159–173.
Onyutha, C., Tabari, H., Rutkowska, A., Nyeko-Ogiramoi, P., and Willems, P. (2016). Comparison of different statistical downscaling methods for climate change rainfall projections over the Lake Victoria basin considering CMIP3 and CMIP5. J. Hydro Environ. Res. 12, 31–45. doi: 10.1016/j.jher.2016.03.001
Onyutha, C., and Willems, P. (2015). Spatial and temporal variability of rainfall in the Nile Basin. Hydrol. Earth Syst. Sci. 19, 2227–2246. doi: 10.5194/hess-19-2227-2015
Opere, A. O., Waswa, R., and Mutua, F. M. (2022). Assessing the impacts of climate change on surface water resources using WEAP model in Narok County, Kenya. Front. Water 3:789340. doi: 10.3389/frwa.2021.789340
Osman, O. E., and Hastenrath, S. L. (1969). On the synoptic climatology of summer rainfall over Central Sudan. Archiv Für Meteorologie, Geophysik Und Bioklimatologie Serie B 17, 297–324. doi: 10.1007/BF02243371
Osmaston, H. (2004). “Quaternary glaciations in the east African mountains” in Developments in quaternary sciences, vol. 2 (Elsevier), 139–150.
Paulo Mavaringana, M. D. J., Gumindoga, W., Kileshye Onema, J.-M., and Makurira, H. (2023). Modelling future flood events under climate change scenarios in the Pungwe River basin. Water Prac. Technol. 18, 1300–1316. doi: 10.2166/wpt.2023.063
Penman, H. L. (1950). The water balance of the Stour catchment area. J. Inst. Water Eng. 4, 457–469.
Pitman, V. (1973). A mathematical model for generating monthly river flows from meteorological data in South Africa. Report No. 2/73, Hydrological Research Unit, Univ. Of the Witwatersrand. Univ. of the Witwatersrand.
Renard, B., Kavetski, D., Kuczera, G., Thyer, M., and Franks, S. W. (2010). Understanding predictive uncertainty in hydrologic modeling: the challenge of identifying input and structural errors. Water Resour. Res. 46:2009WR008328. doi: 10.1029/2009WR008328
Riahi, K., Van Vuuren, D. P., Kriegler, E., Edmonds, J., O’Neill, B. C., Fujimori, S., et al. (2017). The shared socioeconomic pathways and their energy, land use, and greenhouse gas emissions implications: an overview. Glob. Environ. Chang. 42, 153–168. doi: 10.1016/j.gloenvcha.2016.05.009
Rivas-López, M. R., Liersch, S., Menz, C., Lange, S., and Hattermann, F. F. (2022). Potential hydro-meteorological impacts over Burundi from climate change. J. Hydrol. Reg. Stud. 42:101130. doi: 10.1016/j.ejrh.2022.101130
Senay, G. B., Velpuri, N. M., Bohms, S., Demissie, Y., and Gebremichael, M. (2014). Understanding the hydrologic sources and sinks in the Nile Basin using multisource climate and remote sensing data sets. Water Resour. Res. 50, 8625–8650. doi: 10.1002/2013WR015231
Shagega, F. P., Munishi, S. E., and Kongo, V. M. (2020). Assessment of potential impacts of climate change on water resources in Ngerengere catchment, Tanzania. Physi. Chem. Earth Parts A/B/C 116:102804. doi: 10.1016/j.pce.2019.11.001
Sood, A., Muthuwatta, L., Silva, N. S., and McCartney, M. (2017). Understanding the hydrological impacts of climate change in the Tana River Basin, Kenya. International Water Management Institute (IWMI), Colombo, Sri Lanka, 40p. (IWMI Working Paper 178). Available at: https://www.iwmi.cgiar.org/Publications/Working_Papers/working/wor178.pdf (Accessed September 08, 2024).
Sugawara, M. (1995). “Tank model” in Computer models of watershed hydrology. ed. V. P. Singh (Water Resources Publications), 165–214.
Takele, G. S., Gebrie, G. S., Gebremariam, A. G., and Engida, A. N. (2022). Future climate change and impacts on water resources in the upper Blue Nile basin. J. Water Clim. Change 13, 908–925. doi: 10.2166/wcc.2021.235
Tarekegn, D., and Tadege, A. (2006). Assessing the impact of climate change on the water resources of the Lake Tana sub-basin using the WATBAL model. Discussion Paper 30. Pretoria, South Africa: University of Pretoria, Center for Environmental and Economic Policy in Africa.
Taye, M. T., and Dyer, E. (2024). Hydrologic extremes in a changing climate: a review of extremes in East Africa. Curr. Clim. Chang. Rep. 10, 1–11. doi: 10.1007/s40641-024-00193-9
Taye, M. T., Ntegeka, V., Ogiramoi, N. P., and Willems, P. (2011). Assessment of climate change impact on hydrological extremes in two source regions of the Nile River basin. Hydrol. Earth Syst. Sci. 15, 209–222. doi: 10.5194/hess-15-209-2011
Taye, M. T., Willems, P., and Block, P. (2015). Implications of climate change on hydrological extremes in the Blue Nile basin: a review. J. Hydrol. Reg. Stud. 4, 280–293. doi: 10.1016/j.ejrh.2015.07.001
Taylor, K. E., Stouffer, R. J., and Meehl, G. A. (2012). An overview of CMIP5 and the experiment design. Bull. Am. Meteorol. Soc. 93, 485–498. doi: 10.1175/BAMS-D-11-00094.1
Tibangayuka, N., Mulungu, D. M. M., and Izdori, F. (2022). Assessing the potential impacts of climate change on streamflow in the data-scarce upper Ruvu River watershed, Tanzania. J. Water Clim. Change 13, 3496–3513. doi: 10.2166/wcc.2022.208
Tierney, J. E., Smerdon, J. E., Anchukaitis, K. J., and Seager, R. (2013). Multidecadal variability in east African hydroclimate controlled by the Indian Ocean. Nature 493, 389–392. doi: 10.1038/nature11785
Tigabu, T. B., Wagner, P. D., Hörmann, G., Kiesel, J., and Fohrer, N. (2021). Climate change impacts on the water and groundwater resources of the Lake Tana Basin, Ethiopia. J. Water Clim. Change 12, 1544–1563. doi: 10.2166/wcc.2020.126
Tilahun, Z. A., Bizuneh, Y. K., and Mekonnen, A. G. (2023). The impacts of climate change on hydrological processes of Gilgel gibe catchment, Southwest Ethiopia. PLoS One 18:e0287314. doi: 10.1371/journal.pone.0287314
Trang, N. T. T., Shrestha, S., Shrestha, M., Datta, A., and Kawasaki, A. (2017). Evaluating the impacts of climate and land-use change on the hydrology and nutrient yield in a transboundary river basin: a case study in the 3S river basin (Sekong, Sesan, and Srepok). Sci. Total Environ. 576, 586–598. doi: 10.1016/j.scitotenv.2016.10.138
Umugwaneza, A., Chen, X., Liu, T., Li, Z., Uwamahoro, S., Mind’je, R., et al. (2021). Future climate change impact on the Nyabugogo catchment water balance in Rwanda. Water 13:3636. doi: 10.3390/w13243636
Ungtae, K., and Kaluarachchi, J. J. (2009). Climate change impacts on water resources in the upper Blue Nile River basin, Ethiopia. J. Am. Water Resour. Assoc. 45, 1361–1378. doi: 10.1111/j.1752-1688.2009.00369.x
Van Vuuren, D. P., Edmonds, J., Kainuma, M., Riahi, K., Thomson, A., Hibbard, K., et al. (2011). The representative concentration pathways: an overview. Clim. Chang. 109, 5–31. doi: 10.1007/s10584-011-0148-z
Verburg, P. H., De Koning, G. H. J., Kok, K., Veldkamp, A., and Bouma, J. (1999). A spatial explicit allocation procedure for modelling the pattern of land use change based upon actual land use. Ecol. Model. 116, 45–61. doi: 10.1016/S0304-3800(98)00156-2
Verburga, P., and Hecky, R. E. (2009). The physics of the warming of Lake Tanganyika by climate change. Limnol. Oceanogr. 54, 2418–2430. doi: 10.4319/lo.2009.54.6_part_2.2418
Wada, Y. (2016). Modeling groundwater depletion at regional and global scales: present state and future prospects. Surv. Geophys. 37, 419–451. doi: 10.1007/s10712-015-9347-x
Wairimu, G. F. (2008). Assessing the impacts of environmental change on the hydrology of the Nzoia catchment, in the Lake Victoria Basin [Vrije Universiteit Brussel]. Available at: http://hdl.handle.net/1834/6857 (Accessed September 04, 2024).
Wilby, R. L., Dawson, C. W., and Barrow, E. M. (2002). sdsm—a decision support tool for the assessment of regional climate change impacts. Environ. Model. Softw. 17, 145–157. doi: 10.1016/S1364-8152(01)00060-3
Willems, P. (2014). Parsimonious rainfall–runoff model construction supported by time series processing and validation of hydrological extremes – part 1: step-wise model-structure identification and calibration approach. J. Hydrol. 510, 578–590. doi: 10.1016/j.jhydrol.2014.01.017
Wubneh, M. A., Kifelew, M. S., Sahlu, D., Dzwairo, R. B., and Fikadie, F. T. (2022). Hydrological impacts of climate change in selected ungauged sub-watersheds of Lake Tana Sub-Basin, upper Blue Nile Basin, Ethiopia: a regionalization approach. Sci. Afr. 17:e01370. doi: 10.1016/j.sciaf.2022.e01370
Wubneh, M. A., Worku, T. A., and Chekol, B. Z. (2023). Climate change impact on water resources availability in the kiltie watershed, Lake Tana sub-basin, Ethiopia. Heliyon 9:e13941. doi: 10.1016/j.heliyon.2023.e13941
Yates, D. (1994). WatBal—An integrated water balance model for climate impact assessment for river runoff. IIASA Working Paper WP-94-64, IIASA, Laxenburg.
Yates, D., Sieber, J., Purkey, D., and Huber-Lee, A. (2005). WEAP21—a demand-, priority-, and preference-driven water planning model: part 1: model characteristics. Water Int. 30, 487–500. doi: 10.1080/02508060508691893
Keywords: climate change impacts, hydrology and water resources, East Africa, CMIP phases (CMIP3, CMIP5, CMIP6), bias correction
Citation: Onyutha C (2024) Climate change impacts on hydrology and water resources in East Africa considering CMIP3, CMIP5, and CMIP6. Front. Clim. 6:1453726. doi: 10.3389/fclim.2024.1453726
Edited by:
Swadhin Kumar Behera, Japan Agency for Marine-Earth Science and Technology (JAMSTEC), JapanReviewed by:
María Cleofé Valverde, Federal University of ABC, BrazilSifan A. Koriche, University of Alabama, United States
Copyright © 2024 Onyutha. This is an open-access article distributed under the terms of the Creative Commons Attribution License (CC BY). The use, distribution or reproduction in other forums is permitted, provided the original author(s) and the copyright owner(s) are credited and that the original publication in this journal is cited, in accordance with accepted academic practice. No use, distribution or reproduction is permitted which does not comply with these terms.
*Correspondence: Charles Onyutha, Y29ueXV0aGFAa3l1LmFjLnVn
†ORCID: Charles Onyutha, https://orcid.org/0000-0002-0652-3828