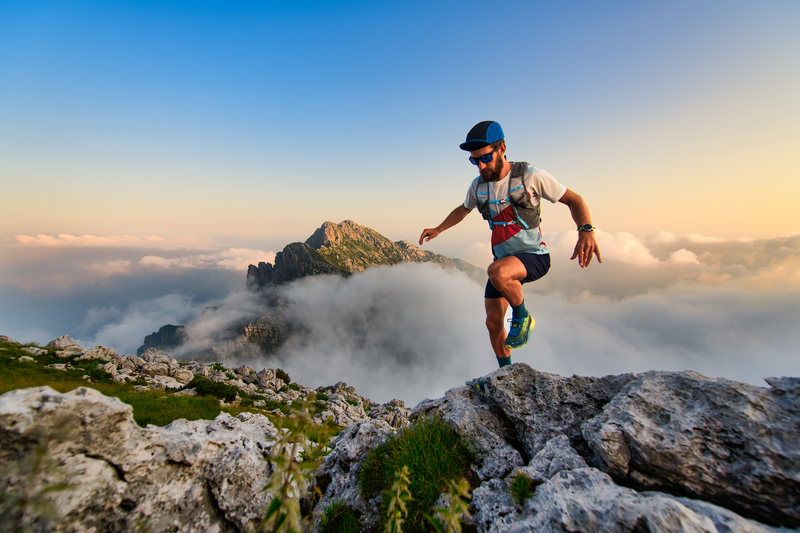
95% of researchers rate our articles as excellent or good
Learn more about the work of our research integrity team to safeguard the quality of each article we publish.
Find out more
ORIGINAL RESEARCH article
Front. Clim. , 12 December 2024
Sec. Climate Services
Volume 6 - 2024 | https://doi.org/10.3389/fclim.2024.1441647
This article is part of the Research Topic Climate Services for Africa View all 4 articles
Skilful monsoon onset forecasts are highly sought after in West Africa, due to the importance of monsoon onset for supporting agriculture, disease prevalence and energy provision. With the sub-seasonal timescale bridging the gap between weather and seasonal forecasts, sub-seasonal forecasts have the potential to provide useful decision-making information about the onset of the monsoon rainfall. This study explores sub-seasonal monsoon onset forecasts over Ghana using the European Centre for Medium Range Weather Forecasting (ECMWF) operational ensemble prediction systems. Three commonly used methods for defining monsoon onset are compared, and the benefits, challenges, and potential of real-time, sub-seasonal onset forecasting using these methods are examined. Monsoon onset forecasting at sub-seasonal timescales, and associated decision-making with shorter-term information can be challenging due to the typically single occurrence of rainfall onset within a season. Sub-seasonal forecasts of monsoon onset are shown to be particularly useful for confirming onset occurrence, at least 1–2 weeks earlier than when observations are used. Earlier confirmation of monsoon onset provided by sub-seasonal forecasts, has the potential for earlier decision-making and action that would positively impact sectors such as agriculture.
The onset of the annual wet season is of key importance to agriculturalists in sub-Saharan Africa, as it marks the start of the agricultural growing season; a key event in the social and agricultural calendar. A range of important agricultural decisions are made based upon the timing of the onset, including when to prepare the fields, and when and which seeds to plant. Preparing and planting too early, before consistent, reliable rainfall has arrived can mean seedlings die, and extra expenditure for additional seeds, while planting too late may mean crops fail to reach maturity before season cessation and yields are reduced. Thus, information and forecasts detailing the timing of wet season onset is of key importance to farmers both in sub-Saharan Africa, and across the tropics, and skilful onset forecasts are highly sought after. In addition to agriculture, forecasts of the timing and duration of wet season onset can provide vital information for decision-making within many sectors, including disease prevention and energy planning (Owusu et al., 2015; Laux et al., 2008; Acharya and Bennett, 2021).
A range of studies have explored the prediction skill of onset in seasonal forecasts across Africa and the wider tropics. MacLeod (2018) and Scheuerer et al. (2024) used seasonal simulations to produce onset forecasts for the East African wet seasons; generally it was found that skill was better for the short rains (October to December), compared to the long rains (March to May). MacLeod (2018) also explored the value of adding sub-seasonal forecasts for the first 46 days of the forecast period; this was found to bring a small improvement. Zampieri et al. (2023) also used seasonal forecasts, but analysed the prediction skill of onset forecasts across Africa; they found positive skill in South Africa and Eastern Africa, but less skill over West Africa. Chevuturi et al. (2021) used European Centre for Medium Range Weather Forecasting (ECMWF) seasonal forecasts to produce monsoon onset forecasts for India; they found the model exhibited good skill in forecasting monsoon onset 1 month in advance. Vellinga et al. (2013) used the UK Met Office seasonal forecast model to produce rainfall onset forecasts over West Africa, and found modest skill at 2–3 months lead time. While these studies show that seasonal onset forecasts are possible, they mostly offer a broad indication of the likelihood of an early or late onset.
Sub-seasonal timescales bridge the gap between short-range and seasonal weather forecasts, and may provide useful information in the period preceding monsoon onset. Thus sub-seasonal forecasts offer an exciting opportunity to provide decision relevant onset forecasts to users across the tropics, including in West Africa. Bombardi et al. (2017) used a hybrid methodology, combining observations with sub-seasonal forecasts, to produce onset forecasts for across the tropics; they found that the models outperform the climatology in predicting rainy season onset across West Africa, and for two models [National Centers for Environmental Prediction (NCEP) and China Meteorological Administration (CMA) models] the onset forecasts exhibit good skill more than 3 weeks in advance for the region. Conversely, they found limited skill over East Africa; this may be because the region they have used for East Africa is different to the region used in other studies. Olaniyan et al. (2019) also used sub-seasonal forecasts to produce onset forecasts over Nigeria; they found poor performance, with the ECMWF model performing best (compared to the CMA and UKMO model). While Bombardi et al. (2017) and Olaniyan et al. (2019) explored the skill of onset forecasts from sub-seasonal forecast products, the operational application, including how sub-seasonal onset forecasts can provide decision-relevant information, was not considered.
In this paper we present an approach for the creation of onset forecasts using sub-seasonal rainfall forecasts in real-time, and detail how new developments in sub-seasonal rainfall prediction can assist with the decision-making processes associated with the onset of monsoon rains. We will analyse different methods of defining monsoon onset, and will examine the benefits and challenges of subseasonal-to-seasonal onset forecasting using each method. Methodologies similar to those used by Bombardi et al. (2017) and Olaniyan et al. (2019) for determining monsoon onset are used, alongside other locally-defined methodologies. In contrast to other studies, we specifically explore the real-time operational application of the methodologies for determining onset. With the introduction of the World Meteorological Organisation Sub-seasonal to Seasonal (S2S). Real-time pilot project, this study was granted the opportunity to explore the application of sub-seasonal forecasts for use in decision-making in real-time. Applying traditionally seasonally monitored metrics such as onset on shorter timescales provided unique challenges and this study is able to demonstrate these challenges as well as the potential benefit that the application of sub-seasonal real-time forecasts can provide within the decision-making process.
In this study, we chose to focus on Ghana, in West Africa. Agriculture in Ghana is of high societal importance, with around 40% of the employees in Ghana working in the agricultural sector, and agriculture making up around 20% of Ghana’s GDP, in 2021 (Statista, 2024a, 2024b). Much of this agriculture is rain-fed, and dependent upon the annual wet season. Ghana experiences an annual dry season, from November to February, followed by an annual wet season, from March to October (Figure 1), associated with the northern movement of the tropical rainband during the boreal summer. In the northern parts of the country the wet season is shorter (starting later and ending earlier), while in the southernmost parts of the country the main March–October wet season is split into two seasons, with the “Little Dry Season” in July–August (Dunning et al., 2016). Thus onset forecasts are important for agriculturalists across all of Ghana. In particular, this study focuses on the coastal Savannah region around the capital city Accra, as the majority of the population are located in the southern part of the country.
Figure 1. Map of average annual rainfall (mm) over West Africa. The inset shows the mean monthly annual cycle of rainfall for Accra (black dot), located in the Greater Accra region, which is mostly coastal Savannah.
This work was completed as part of the Global Challenges Research Fund (GCRF) African Science for Weather Information and Forecasting Techniques (SWIFT) sub-seasonal forecasting testbed (Hirons et al., 2021, 2023); a two-year forum where prototype S2S forecast products were co-produced and operationally trialled in real time. Working with Ghana Meteorological Agency (GMet) and the Ministry of Food and Agriculture (MoFA) in Ghana, S2S forecast products were co-developed and distributed to local farmers via agricultural extension workers. Through this process the importance of onset forecasting was continuously highlighted by stakeholders, resulting in this work exploring the operational use of S2S forecasts for forecasting onset (Hirons et al., 2023).
Through the GCRF African SWIFT forecasting testbed S2S forecast products were being co-produced with in-country national meteorological agencies and agricultural bodies to inform agricultural decision-making. Within the iterative dialogue between agricultural stakeholders, operational forecast producers and researchers it was abundantly clear that reliable and actionable forecasts of rainy season onset would be hugely beneficial for stakeholders.
The aim of this study is to demonstrate the challenges and potential added benefit to decision-making that real-time sub-seasonal forecasts of rainfall onset can provide.
The remainder of the paper is structured as follows; in section 2 the methodology, data processing and verification techniques are outlined. Section 3 presents the comparative results between the three rainfall onset definitions, as well as the methods for applying real-time sub-seasonal forecasts to onset forecasting. Sections 4 and 5 include a discussion of the implications of these results and conclusions, respectively.
The precipitation observation data used for this study is the Tropical Applications of Meteorology using SATellite data and ground observations (TAMSAT) version 3.1 daily estimates (Maidment et al., 2014; Tarnavsky et al., 2014; Maidment et al., 2017). TAMSAT is a widely used gridded rainfall dataset, based on thermal infrared imagery; previous studies have shown that TAMSAT accurately represents rainfall over Ghana (Atiah et al., 2020). For consistency with the ECMWF hindcast period, data from 1998 to 2019 is used for the TAMSAT climatology. For comparison with the forecast data, the observations are used for the period from 31st January to the 18th April each year, with the full year used to calculate the climatological daily mean rainfall for each day of the year. The spatial resolution of daily TAMSAT data was interpolated from the regular 0.0375° × 0.0375° grid to 1.5° × 1.5° to facilitate the comparison with the ECMWF S2S forecast data.
The ECMWF sub-seasonal model, version CY45R1, is used in this study. This is the ECMWF model from the sub-seasonal to seasonal (S2S) Prediction Project database (Vitart et al., 2017). This model configuration has a spatial resolution of Tco639/319L91, which corresponds to a horizontal resolution of ~16 km up to day 15 and ~32 km after day 15, with 91 levels in the vertical. The forecast length is 46 days, with 51 forecast ensemble members and 11 hindcast (or re-forecast) ensemble members. For the purposes of this study, weekly Monday initialisation dates are examined between the 17th February 2020 to the 13th April 2020. A 20-year hindcast period from 1998 to 2019 was used to compare the model with the observations, as well as to examine model bias. The ECMWF data was analysed on a 1.5 degree grid, with a day 15–16 correction to remove any anomalies caused by the horizontal resolution change after day 15. This correction involved removing 1 day of data on day 15 (which is erroneous due to the resolution change) and replacing it with a daily mean value from the 46-day forecast data.
Global weather and climate models have systematic errors (biases) in their output which can be caused by a range of different factors. For example, limited spatial resolution, simplified physical and thermodynamic processes represented by sub-grid parameterisation schemes or an incomplete understanding of the global climate system. Using uncorrected model outputs, especially in applications where decisions are being based on forecast information, could be misleading. Various bias correction or bias adjustment methods have been developed to overcome these model deficiencies. It is important to note that a good, reliable observational dataset is required for effective bias correction. In this study several bias correction techniques were explored, including scaling using the mean (Osei et al., 2021), scaling using the mean as well as the standard deviation, and quantile mapping (Teutschbein and Seibert, 2012). The quantile mapping method, which is widely accepted, was determined to be the most appropriate here, as the scaling techniques did not account for interannual variability within the model. It provides a way to adjust the whole forecast distribution to align better with the observed distribution.
The quantile mapped, leave-one-out method of bias correction was applied. The forecast and hindcasts were combined into a one 21 year dataset, and de-accumulated into daily and weekly total rainfall time series, prior to onset date calculation.
The quantile mapping was conducted by calculating the quantiles for the observed and hindcast datasets (sections 2.1 and 2.2, respectively). This technique maps the cumulative distribution function (CDF) of the ECMWF model forecast time series against the observed CDF time series (Rajulapati and Papalexiou, 2023). This bias correction method was applied separately to daily total data and to weekly totals, as bias-corrected daily rainfall does not accurately account for biases in weekly rainfall values.
This study uses the Equitable Threat Score (ETS) to analyse the skill of the ECMWF onset forecasts. The ETS is a verification metric commonly used for the analysis of precipitation forecasts (ECMWF Confluence, 2024). It measures the ratio of correctly predicted events adjusted for random chance, showing how well the correctly forecasted events correspond to the observed event occurrence. This calculates the number of hits the forecast is achieving without the hits that would have been correct purely by chance. The ETS is calculated as follows:
where:
This study examines the challenges associated with operationally forecasting monsoon onset on sub-seasonal timescales. Specifically, it compares three definitions of monsoon onset over Ghana in West Africa (Figure 1). The three methods of defining monsoon onset are the cumulative daily rainfall anomaly method (ACCUM), the daily rainfall threshold method (3DAY), and the weekly rainfall threshold method (WKLY). These methods are described in detail below and summarised in Table 1.
The cumulative daily rainfall anomaly method (hereafter referred to as “ACCUM”) is adapted from a definition of monsoon onset developed by Dunning et al. (2016), based on Liebmann et al. (2012). This method calculates the monsoon onset by first finding the long term daily mean annual precipitation, and then using this to calculate the rainfall anomaly for each day for the year of interest. This time series is accumulated to get the cumulative daily rainfall anomaly. The monsoon onset is identified as the day of the year at which the minimum cumulative anomaly occurs. However, with a 46-day forecast, it is not yet possible to confirm if a minima is the annual “global minima.” Thus, in order to apply this method to sub-seasonal forecasts in real time, we used an additional criterion that the onset is the day of the year at which the minimum cumulative anomaly occurs, with no lower minima forecast in the next 30 days.
The operational daily rainfall threshold method (hereafter referred to as “3DAY”) is used for agricultural purposes by the Ghana Meteorological Agency (Sivakumar, 1988; Fitzpatrick et al., 2015). This method identifies the monsoon onset as the first day, after 1st February, of three consecutive days over which the cumulative rainfall is greater than 20 mm, and after which there are no periods of 10 days or more with less than 1 mm of rainfall within 30 days of onset. This dry-spell constraint ensures that onset is not falsely declared after a singular heavy precipitation event.
The third method of rainfall onset is the weekly rainfall threshold method (hereafter referred to as “WKLY”), adapted from the AGRHYMET (1996) and Mounkaila et al. (2014) definitions, for use with weekly sub-seasonal forecasts. Previous methodologies are based on 3 consecutive decades (10-day periods) with total rainfall over a given threshold. Our adaptation of the method requires a week 1 rainfall total greater than 20 mm, with the three consecutive weeks of more than 15 mm in total. The onset date is defined as the first day of week 1.
In order to ensure this comparative analysis study is relevant to current practices it includes onset definitions (3DAY and WKLY) which are currently operational for agricultural applications in the West African region. However, a potential disadvantage of such definitions is their dependence upon specific rainfall thresholds. Although these thresholds have been chosen to be appropriate for the regional rainfall characteristics, they may need calibration to reliably be applied to a forecast model with inherent biases (section 2.3). In contrast, the accumulated method (ACCUM) was designed such that it is not threshold dependent, and can be applied to biased, spatially aggregated data. The ACCUM method was originally designed for use after the season, when the full seasonal rainfall evolution is known; here we applied modifications so we could utilise it with 46 day forecast data (added additional criterion that the onset is the day of the year at which the minimum cumulative anomaly occurs, with no lower minima forecast in the next 30 days). While defining and communicating rainy season onset to stakeholders is clearly strongly desired, this comparative analysis of three onset methods gives insight to the challenges of operationally defining monsoon onset in real-time.
Figure 2 shows the annual wet season onset date for Accra, calculated using the three onset methodologies applied to TAMSAT data for 2000–2020. Annual wet season onset ranges from early February (e.g., in 2011) to late April (WKLY definition in 2004 and 2007), with the majority of onset dates occurring during March. In some years, all three onset definitions show good agreement, for example for 2000, 2002, 2005, 2010–2013 and 2016 (8 years) the three onset dates are all within a week of each other. In other years two of the methods agree to within a few days, while the third is notably different. In these cases, the onset methodology that gives the date that differs is not consistent, for example, in 2004, 2008, 2009 and 2014 the onset dates given by ACCUM and 3DAY methodologies agree to within a week, while the WKLY method is much later, while in 2003, 2006 and 2020 the WKLY and ACCUM onset dates agree to within a week, while the 3DAY onset date is much earlier. For 4 years (2001, 2007, 2017 and 2019) the 3 onset methodologies give 3 different dates, all differing by at least a week; the largest spread is found in 2007 where the range in onset dates is greater than 60 days. 2007 and 2017 are explored further below. 2018 is the only year when the requirements for onset are not met for two of the onset methodologies (ACCUM and WKLY).
Figure 2. Observed onset dates calculated from TAMSAT data for the hindcast period (2000–2020) for the three onset definitions shown in Table 1: ACCUM (black triangle), 3DAY (blue dot) and WKLY (green cross). The mean onset date from each method across the hindcast period is shown by the horizontal dashed lines. Case study years from Figure 3 are highlighted by grey and black boxes, with further examination of 2012 and 2017 in Figures 4, 5 represented by the black boxes.
Figure 2 also shows the difference in the long term average onset, indicated by the horizontal dashed lines. This figure indicates that the 3DAY method gives earlier onset dates than the other two methods, with the WKLY method tending toward later onsets.
Figure 2 highlights the sensitivity in the three onset definitions. The characteristics of particular years, highlighted by the grey and black boxes in Figure 2, are explored further here.
Figure 3 shows the cumulative rainfall anomaly (ACCUM), daily rainfall (3DAY), and weekly (WKLY) rainfall from TAMSAT observations for four example years (2007, 2012, 2016, 2017), represented by the grey and black boxes in Figure 2.
Figure 3. Observed onset dates from TAMSAT for example years of (a) 2007, (b) 2012, (c) 2016 and (d) 2017, highlighted by grey and black boxes in Figure 2. The weekly (WKLY), daily (3DAY) and accumulated anomaly (ACCUM) onset methods (Table 1) are shown in the bottom, middle and top parts of each subplot, respectively. The dashed vertical line in each section indicates the onset date for each definition. The dates along the x-axis indicate the start of the week.
These 4 years were chosen as examples where the three methodologies display a diversity in comparative behavior; where they have similar monsoon onset dates (2012 and 2016), and where they have a large difference in onset date (2007 and 2017). In 2012 (Figure 3b) all three onset dates agreed to within 1 day; rainfall started during the week of the 23rd March, with regular rainfall and no long dry spells after this. For 2016 (Figure 3c) all three onset dates agree to within 1 week; rainfall started in the week beginning 2nd March and was consistent after this. While there was a drier week in late April (20th April), with a 7 day dry spell, this was over 4 weeks after the onset, and did not have a large impact on the cumulative rainfall anomaly.
2007 and 2017 exhibit a larger spread of onset dates (Figures 3a,d). In 2017 (Figure 3d) there was a very heavy rainfall event (~30 mm) at the end of January (22nd Jan) which met the threshold for the 3DAY onset definition; however this was before 1st February so was not considered. In early March a few days of rainfall triggered the 3DAY onset definition, and while rainfall was low after this there were no 10 day dry spells, so this was defined as onset for the 3DAY method. However, the low rainfall totals in the following weeks meant that this did not satisfy the criteria for the other methodologies. The WKLY and ACCUM methodology have the onset occurring in late March, when rainfall totals were generally consistently higher.
In 2007 (Figure 3a) a few days of rainfall in mid-February met the threshold for the 3DAY onset definition. Similar to 2017, rainfall after this date was generally low, but since there were no 10 day dry spells, this was the onset date for the 3DAY methodology. The period from mid-February to mid-March was generally dry, with increased rainfall from mid-late March onwards; the ACCUM methodology has the onset as 25th March. While the weeks in mid-late March/early April did meet the threshold for the WKLY methodology, a dry week in mid-April (w/b 14th April) meant that this did not meet the onset criteria for the WKLY method. The onset for the WKLY method is later on 5th May, when the 20 mm threshold for week 1 was exceeded. This week also contained a minima in the cumulative rainfall anomaly, but since it was after 30 days after the first minima, it was not the ACCUM onset.
This analysis demonstrates the differences and sensitivities of the different onset methodologies. The 3DAY methodology may be triggered by a large rainfall event and then followed by low rainfall (without 10 day dry spells) which gives an earlier onset date than the other methodologies. Figure 2 shows a few years when the 3DAY onset date is earlier than the date given by the other methodologies (e.g., 2003, 2006, 2007, 2017, 2019, 2020). In 2018 the 3DAY methodology was the only definition that was met. The WKLY methodology is sometimes later due to 1 week of lower rainfall, or the absence of a week with 20 mm to trigger the definition. The ACCUM methodology can contain multiple minima, with slight differences in magnitude, which may lead to a notable difference in onset date. It is important to note that none of these onset definitions are wrong, rather they are all designed to capture slightly different aspects of the rainfall variability during the onset period. Which method is most appropriate is likely to depend on the particular stakeholder decision that it is being applied to. Different applications will have different sensitivities to extended dry spells, for example. That said, these methodological sensitivities are important when considering their application to ensemble forecasts.
As previously stated, forecasting rainfall onset is of crucial importance to stakeholders who need this information to make important weather-related decisions in their sectors. However, providing this information in real-time is extremely challenging because onset definitions rely on forecasting both the immediate conditions (i.e., next week), and also future conditions over the next month (i.e., next 30 days). Reliably forecasting this extended range is challenging (de Andrade et al., 2021), turning that information into an actionable forecast for farmers even more so.
Here, the three monsoon onset definitions are applied to a state-of-the-art sub-seasonal forecast model (ECMWF; section 2.2) to assess if reliable and actionable information can be extracted in real-time. The example years 2012 (Figure 4) and 2017 (Figure 5), highlighted by the black boxes in Figure 2, are used to explore the real-time application.
Figure 4. Forecasts of onset for the three onset methods within 2012, for ECMWF model initialisation dates starting (a) 17th February, (b) 23rd March, (c) 30th March and (d) 6th April. The top panels in each subplot show the accumulated anomaly (ACCUM) for each ensemble member (coloured lines), with observations (black line). The second panel shows the weekly rainfall (WKLY) in 3 categories (<15 mm, 15–20 mm, >20 mm) for the observations (black line) and the forecasts (coloured lines). Please note that the position within each box has no meaning, the lines are simply separated to make the observations and each ensemble member visible. The third panel shows the onset states for the 3DAY method for the observations and forecasts. In the 3DAY panel “A” represents the state where the 3 day accumulation of 20 mm rainfall has not been met or has been met but there has been a subsequent 10 dry spell; “B” represents that there has been a 3 day period with 20 mm rainfall in the last 30 days with no subsequent 10 day dry spell, the transition to “B” occurs on the first day of the 3 day spell; “C” represents that there has been a 3-day period with more than 20 mm of rainfall and no 10 dry spell in the following 30 days. Monsoon onset is defined by the 1st day of the transition to B with no subsequent transition to A in the next 30 days. The bottom panel shows the daily observed TAMSAT rainfall for interpretation purposes. As in Figure 3, the black dotted vertical lines in each plot panel show the observed monsoon onset date for the respective onset method definition. The data is plotted at the beginning of the week period, e.g., 06-01 corresponds to rainfall from the 6–12th January.
Figure 5. As Figure 4, but for initialisation dates from (a) 2nd March, (b) 9th March, (c) 16th March, and (d) 6th April for 2017.
In the 17th February initialisation (Figure 4a) the forecast ensemble members contain no indication of monsoon onset occurrence prior to or during the forecast period for any method. For the WKLY method most weeks have rainfall <15 mm, and for the 3DAY method all ensemble members remain in category A or B. For the ACCUM methodology there is some suggestion that onset may occur at the end of the forecast period, but it is too close to the end of the forecast period to tell if this is the minima for the next 30 days. The 23rd March initialisation (Figure 4b) shows early indications of a potential monsoon onset according to the 3DAY method and WKLY methods, however no ensemble members predict onset for the ACCUM method. By the 30th March initialisation (Figure 4c), the 3DAY method confirms all ensemble members agree that onset occurred on 22nd March, just over a week before initialisation. The ACCUM method now also indicates that all but one ensemble member predicts onset to have occurred on 22nd March. The WKLY method shows that more than half the ensemble members also predict onset to have occurred, the week beginning 23rd March. For the 6th April initialisation (Figure 4d), the 3DAY and ACCUM still confirm onset occurrence on 22nd March, with the majority of ensemble members confirming onset occurrence for the WKLY method on 23rd March.
It is interesting to note that in early February there was a false onset, with initial rainfall meeting the first criteria for the 3DAY as well as the first two criteria for the WKLY method, however this was followed swiftly by a dry period of little to no rainfall. The 17th February forecast suggests that this was not the onset.
Figure 4 indicates that when onset occurrence is in close agreement across the three methods, as with the 2012 case study (all three methods indicate an onset date within 1 day), the onset can be confirmed in the forecast after approximately 1 week. The 30th March initialisation can be used to strongly indicate that the 22nd March was the onset, long before the 30 day/4 week period after onset, when the onset can be confirmed in observations. However, forecasts in advance of onset offer much less information; the forecast initialised on 23rd March did not strongly suggest that onset was about to occur. After the potential onset (when the first condition is met), there is a 30 day/4 week window where other conditions (no dry spells/no other minima etc.) must be met for the date to be confirmed as onset. The results in Figure 4 suggest that the main strength of the sub-seasonal onset forecasts may be in confirming an onset date within this 30 day/4 week confirmation window (before onset can be confirmed in observations), rather than forecasting the onset date in advance.
Unlike in 2012, in 2017 the three onset methods have a larger spread in the onset date, with the 3DAY onset occurring on the 4th March, the WKLY method on the 31st March and the ACCUM method indicating onset occurrence on the 20th March.
For the 3DAY methodology, in the 9th March initialisation most ensemble members agree on an onset on 4th March, however a later initialisation on 16th March then suggests 10 day dry spells, meaning that ensemble members move back to category A. It is not until the initialisation on the 6th April, that onset can be confirmed, when the observations confirm the occurrence of onset anyway. For the other methods (WKLY and ACCUM) there is limited consensus on onset dates, even for the later initialisation dates, after onset has occurred.
Figure 5 shows that when there is a larger spread in the observed onset date across the three onset methods, the forecasts do not capture or confirm onset reliably, even after the onset has occurred. The disagreement in observed onset dates is likely to be a result of the sensitivity of these methods to small rainfall changes in amount and consistency. These are years with “borderline” rainfall, meaning some onset definitions are met, while others are not. Small differences in rainfall can mean thresholds are/are not met, and can lead to subtle differences in minima for the ACCUM method. Such small and subtle rainfall variations are unlikely to be captured by the sub-seasonal ensemble forecasts. For example there was not quite enough rainfall (a matter of millimetres) in the week of 24th March, which caused a delay in the observed onset within the WKLY method. It is likely that the intermittent dry days around this time also influenced the model prediction for the ACCUM and the 3DAY methods. In years where ACCUM method has a minimum but does not then increase notably from the minima for a long time, small variations in model rainfall may lead to further minima, which results in high forecast sensitivity to model spread.
Section 3.2 has shown that there are discrepancies between these onset definitions when they are applied to rainfall observations. Section 3.3 has explored how these different definitions can be applied to sub-seasonal forecasts in real-time and again highlighted the challenges within examples where the onset date differs considerably. Receiving and interpreting conflicting information like this could be extremely confusing and misleading for a decision-maker. The landscape of stakeholders making onset-related decisions is extremely broad and represents decision-makers with a wide variety of technical backgrounds, access to information, and training. In this section the analysis goes beyond highlighting the differing characteristics between methods and explores their practical application to decision-making. For an onset forecast to be useful, it must be both useable and used. It is necessary that the sub-seasonal forecast has skill, but this is not sufficient for it to be useful. To add value the onset forecast must also be actionable and inform decisions.
Figure 6b uses the structure of Figures 4, 5 to represent the onset forecast information from all the onset methods. However, to help interpret the complex information, it is now overlaid with and accompanied by a decision making process tree (Figure 6a). This figure describes the complex decision-making process a sub-seasonal forecast users may take (Figure 6a), in relation to when in the season the sub-seasonal information suggests onset has occurred (Figure 6b). A user would ask themselves whether the onset has already occurred in the observations, and whether this needs to be confirmed by further data from the sub-seasonal forecast. If onset has not occurred, they would then consult the latest sub-seasonal data to explore whether an onset threshold is forecasted to occur, and whether this onset can also be validated with the same forecast. Finally, if onset is not forecasted or cannot be validated in the observations or latest available forecast, this is still useful information for a decision-maker. Figure 6 showcases how categories from the decision-making tree (Figure 6a) can be used to analyse a sub-seasonal forecast (Figure 6b) to retrieve useful information of a seasonal event at sub-seasonal timescales.
Figure 6. (a) Decision making process tree. (b) 2012 example from Figure 4 with the decision categories overlain. Decision category 1 is when the onset occurs in the observations and can be validated also by the observations. Decision category 2 is when the onset occurs in the observations but requires the forecast to validate or confirm the onset conditions. Decision category 3 is when the onset can be seen early in the forecast and can be validated or confirmed also in the forecast. Decision category 4 is when the onset is seen in the later weeks of the forecast but the forecast cannot be confirmed or validated. Decision category 5 is when the onset cannot yet be seen in the observations or forecasted rainfall.
Since monsoon onset is typically a climatological event that occurs once in a season, it can be difficult to utilise shorter-term information, such as sub-seasonal forecasts, when making decisions about onset within that season. Figure 6 demonstrates the potential application of sub-seasonal forecasts for use in real-time decision-making, within the early part of the rainy season. Weekly forecasts can provide updates on the occurrence, or lack-thereof, of rainfall onset. This can be broken into several categories that explore whether the forecast can confirm and support a potential declaration of monsoon onset within the observed record, provide early prediction of potential onset, or provide information regarding the lack of evidence for an upcoming monsoon onset occurrence.
By utilising decision-making categories such as those in Figure 6, in combination with scientific evaluation techniques, we can determine the reliability as well as the practical usefulness of sub-seasonal forecasting for longer term event determination. Conducting meteorological verification is important in building producer and user confidence in the forecast system as well as confidence in the output usefulness for decision-making application. Figure 7 shows the results of equitable threat score (ETS) analysis of the forecasts by lead time and decision-making category.
Figure 7. Equitable threat score (with a 50% threshold) for Accra, for the ACCUM method (a), 3DAY method (b), and the WKLY method (c). The forecast initialisation dates are shown on the y-axis and onset categories, which align with the decision categories from Figure 5, on the x-axis. These categories are: onset shown in the observations (aligned with decision category 1), onset occurring in weeks-3 and -4 (aligned with decision category 2), onset occurring in weeks -2 and -1 (also aligned with decision category 2), onset occurring in weeks 1 and 2 (aligned with decision category 3), and onset occurring after week 3 (aligned with decision categories 4 and 5). The values within the boxes show hits, misses, false alarms and correct negatives. The darker blue colours indicate more favourable ETS scores, with hatching over the boxes indicating where the forecast gives only correct negatives.
The equitable threat scores (Figure 7) demonstrate that within each onset method there is good skill in the ECMWF forecasts confirming onset having occurred in the observations as well as in the weeks immediately preceding forecast initialisation. Dark blue colours in weeks-3 and -4 for all methods show that when onset is triggered 3–4 weeks before the forecast, there is good forecast skill for confirming onset (within the 30 day/4 week confirmation window). For weeks-1 and -2 the skill is better for the 3DAY method; when the onset is triggered 1–2 weeks before the forecast, again, there is good forecast skill for confirming onset (within the 30 day/4 week confirmation window). While the skill is lower for ACCUM and WKLY there is some forecast skill here.
The WKLY method shows poor skill compared to the 3DAY and ACCUM methods week 1 and 2 of the forecast but appears to better predict later onset occurrence, especially within the later initialisation dates. The 3DAY method has poor skill during later initialisation dates. The ACCUM method appears to have more consistent skill for prediction onset within the forecasted period, and across initialisation dates.
The skill analysis shown in Figure 7 confirms what was suggested in Figures 4, 5. For both the ACCUM and WKLY methods, when the onset occurs 2 or more weeks prior to the forecast, the forecast has good skill in confirming that onset has occurred. For the 3DAY method this confirmation of onset happens even earlier, with good skill in the forecast’s ability to determine onset more than 1 week after onset occurs within the observations. Using this ETS analysis, we can have reasonable and actionable confidence when the 3DAY method has onset occurring in week-1 and -2, that onset has indeed occurred that season. This is especially true when the onset methods are in better agreement that season, as discussed in Figures 4, 5.
Although there is limited skill for reliably forecasting onset within the forecast itself, the forecast’s ability to confirm onset occurrence after 1–2 weeks is still 2 weeks earlier than current operational techniques, which require 30 days or more to accurately assess if onset has occurred. It is important to note that these results, and forecasts, provide just one piece of valuable and useful information among several within an effective decision-making and actionable process.
We have presented an approach for the creation of onset forecasts using ECMWF sub-seasonal rainfall forecasts in real-time, and have detailed how such onset forecasts could be used in decision-making processes associated with the onset of monsoon rains. For the most part, monsoon onset is a climatological event that occurs once in a season, thus it can be difficult to utilise shorter-term information, such as sub-seasonal forecasts, when making decisions about onset within that season.
Three commonly used methods for defining onset were used. While two are used in operational contexts (3DAY and WKLY), the ACCUM method has been used in a range of studies on seasonal and sub-seasonal forecasting of monsoon onset [e.g., Bombardi et al., 2017; MacLeod, 2018], due to its applicability to biased, spatially aggregated data. All of the methodologies have different strengths and weaknesses when applied to the sub-seasonal forecasts, and exhibit different sensitivities.
The 3DAY and WKLY methods both rely on rainfall over a period of days, which make these methods more sensitive to exactly when the rainfall occurs temporally. The WKLY onset determination method can be sensitive to the starting day of the week, due to the method calculation constraints. For example, a single day of high rainfall falling on a Sunday, with the week starting on Monday, can result in a large change in the weekly total and thereby impact the onset date. Small differences in the timing of rainfall in the forecast can lead to large differences in the timing of wet season onset. Small differences in rainfall amount (0.9 mm vs. 1.1 mm) and timing can alter the length of dry spells, and the timing of the 3DAY onset.
All three methods can be sensitive to small differences in rainfall amount, meaning a threshold is or is not met, or a minima is reached. These methodological sensitivities are important when considering their application to ensemble forecasts.
The decision-making context demands careful consideration of the application of a specific onset method, as there can be a large difference in the onset dates observed (Figure 2). Particularly, the average observed onset using the WKLY method was later than the other two.
The onset method should be carefully designed relative to the application, and which method is most appropriate for a decision-making context is likely to depend on the particular stakeholder decision that it is being applied to. For example, what rainfall amount and distribution is required for seedlings to survive, or for a reservoir supplying water to a hydroelectric dam to fill, or for populations of malarial mosquitoes to increase? Different applications will have different sensitivities to extended dry spells. This may create challenges for a National Meteorological Agency providing onset forecasts to users; which onset date will best meet the decision-making context for most users? In addition, for which onset method does the sub-seasonal forecast exhibit the highest prediction skill t? Figure 7 suggests that the ECMWF forecast has the most skill for the 3DAY method, and can confirm onset just 1–2 weeks after the initial trigger. Overall, the forecast based on the 3DAY method is able to determine onset earlier, reliably, than the other two methods.
It is also important to note that, although it was not discussed in detail during this analysis, the geographical scale or resolution of the data can make a big difference to the determination of onset dates. Fitzpatrick et al. (2015, 2016) show differences in onset dates when calculated at different scales; this may be a challenge of using forecasts at 1.5° scale to forecast local onset. Further work should explore how coarse-scale sub-seasonal forecasts can be used for local scale decision making.
For operational use in a decision-making context, the primary benefit of sub-seasonal forecasting of monsoon onset using the three methods described within this study is the earlier confirmation of onset occurrence when compared to examination of observations only. When solely using observations, onset is not confirmed until 30 days/4 weeks after the initial trigger. Here we have shown that sub-seasonal forecasts from ECMWF can be used within that 30 day/4 weeks window to confirm if an initial trigger was the wet season onset. This may be of particular use for agriculturalists, who can plant earlier with confidence that the wet season has started, offering a longer growing period.
We have also shown that sub-seasonal forecasts cannot be used to forecast onset in advance of the onset date. This may be due to the onset definition depending on at least 30 days/4 weeks of rainfall, and sub-seasonal forecast skill is lower after week 3–4 (de Andrade et al., 2021).
Here we have demonstrated an approach for the creation of onset forecasts using ECMWF sub-seasonal rainfall forecasts in real-time; further work should explore with stakeholders and operational forecasters how this can be used in an operational setting, including a forecast testbed for trialling the production and use of sub-seasonal onset forecasts in real time.
Publicly available datasets were analyzed in this study. This data can be found here: https://apps.ecmwf.int/datasets/data/s2s-realtime-instantaneous-accum-ecmf/levtype=sfc/type=cf/.
ET: Writing – original draft, Writing – review & editing. CW: Writing – original draft, Writing – review & editing. LH: Writing – original draft, Writing – review & editing. SW: Writing – original draft, Writing – review & editing.
The author(s) declare that financial support was received for the research, authorship, and/or publication of this article. This work, and its authors, were supported by UK Research and Innovation as part of the Global Challenges Research Fund, African SWIFT Programme, Grant No. NE/P021077/1. LH and SW were also supported by National Centre of Atmospheric Science (NCAS) ACREW (Atmospheric hazard in developing Countries: Risk assessment and Early Warning). CW acknowledges funding of the Grantham Research Fellowship from the Grantham Foundation. The ECMWF Monthly forecast data used in this study has been provided through the Subseasonal-to-Seasonal (S2S) Prediction Project Real-time Pilot. The S2S Prediction Project is a joint initiative of the World Weather Research Programme (WWRP) and the World Climate Research Research Programme (WCRP).
The authors would also like to thank the Ghana Meteorological Agency for their contributions to the African SWIFT programme and the information they were able to provide with regard to operational procedure.
The authors declare that the research was conducted in the absence of any commercial or financial relationships that could be construed as a potential conflict of interest.
All claims expressed in this article are solely those of the authors and do not necessarily represent those of their affiliated organizations, or those of the publisher, the editors and the reviewers. Any product that may be evaluated in this article, or claim that may be made by its manufacturer, is not guaranteed or endorsed by the publisher.
Acharya, N., and Bennett, E. (2021). Characteristic of the regional rainy season onset over Vietnam: tailoring to agricultural application. Atmosphere 12:198. doi: 10.3390/atmos12020198
AGRHYMET (1996) Methodologic de Suivi des zones a risque AGRHYMET Flash, Bulletin de la campague Agricoleou Sahel, centre regional AGRHYMENT, Niamey, Niger 2: 1–96
Atiah, W. A., Amekudzi, L. K., Aryee, J. N. A., Preko, K., and Danuor, S. K. (2020). Validation of satellite and merged rainfall data over Ghana, West Africa. Atmosphere 11:859. doi: 10.3390/atmos11080859
Bombardi, R. J., Pegion, K. V., Kinter, J. L., Cash, B. A., and Adams, J. M. (2017). Sub-seasonal predictability of the onset and demise of the rainy season over monsoonal regions. Front. Earth Sci. 5:14. doi: 10.3389/feart.2017.00014
Chevuturi, A., Turner, A. G., Johnson, S., Weisheimer, A., Shonk, J., Stockdale, T. N., et al. (2021). Forecast skill of the Indian monsoon and its onset in the ECMWF seasonal forecasting system 5 (SEAS5). Clim. Dyn. 56, 2941–2957. doi: 10.1007/s00382-020-05624-5
de Andrade, F. M., Young, M. P., Macleod, D., Hirons, L. C., Woolnough, S. J., and Black, E. (2021). Subseasonal precipitation prediction for Africa: forecast evaluation and sources of predictability. Weather Forecast. 36, 265–284. doi: 10.1175/WAF-D-20-0054.1
Dunning, C. M., Black, E. C. L., and Allan, R. P. (2016). The onset and cessation of seasonal rainfall over Africa. J. Geophys. Res. Atmos. 121, 11405–11424. doi: 10.1002/2016JD025428
ECMWF Confluence. (2024) Section 6.2.3 Equitable Threat Score. Available at: https://confluence.ecmwf.int/display/FUG/Section+6.2.3+Equitable+Threat+Score. (Accessed May 28, 2024).
Fitzpatrick, R., Bain, C., Knippertz, P., Marsham, J., and Parker, D. (2015). The West African monsoon onset: a concise comparison of definitions. J. Clim. 28, 8673–8694. doi: 10.1175/JCLI-D-15-0265.1
Fitzpatrick, R. G. J., Bain, C. L., Knippertz, P., Marsham, J. H., and Parker, D. J. (2016). On what scale can we predict the agronomic onset of the West African monsoon? Mon. Weather Rev. 144, 1571–1589. doi: 10.1175/MWR-D-15-0274.1
Hirons, L., Thompson, E., Dione, C., Indasi, V. S., Kilavi, M., Nkiaka, E., et al. (2021). Using co-production to improve the appropriate use of sub-seasonal forecasts in Africa. Clim. Serv. 23:100246. doi: 10.1016/j.cliser.2021.100246
Hirons, L., Wainwright, C. M., Nying'uro, P., Quaye, D., Ashong, J., Kiptum, C., et al. (2023). Experiences of co-producing sub-seasonal forecast products for agricultural application in Kenya and Ghana. Weather 78, 148–153. doi: 10.1002/wea.4381
Laux, P., Kunstmann, H., and Bardossy, A. (2008). Predicting the regional onset of the rainy season in West Africa. Int. J. Climatol. 28, 329–342. doi: 10.1002/joc.1542
Liebmann, B., Bladé, I., Kiladis, G. N., Carvalho, L. M. V., Senay, G. B., Allured, D., et al. (2012). Seasonality of African precipitation from 1996 to 2009. J. Clim. 25, 4304–4322. doi: 10.1175/JCLI-D-11-00157.1
MacLeod, D. (2018). Seasonal predictability of onset and cessation of the east African rains. Weather Clim. Extrem. 21, 27–35. doi: 10.1016/j.wace.2018.05.003
Maidment, R., Grimes, D., Allan, R. P., Tarnavsky, E., Stringer, M., Hewison, T., et al. (2014). The 30 year TAMSAT African rainfall climatology and time series (TARCAT) data set. J. Geophys. Res. 119, 10619–10644. doi: 10.1002/2014JD021927
Maidment, R. I., Grimes, D., Black, E., Tarnavsky, E., Young, M., Greatrex, H., et al. (2017). A new, long-term daily satellite-based rainfall dataset for operational monitoring in Africa. Sci. Data 4:170063. doi: 10.1038/sdata.2017.63
Mounkaila, M. S., Abiodun, B. J., and Omotosho, J. B. (2014). Assessing the capability of CORDEX models in simulating onset of rainfall in West Africa. Theor. Appl. Climatol. 119, 255–272. doi: 10.1007/s00704-014-1104-4
Olaniyan, E., Adefisan, E. A., Balogun, A. A., and Lawal, K. A. (2019). The influence of global climate drivers on monsoon onset variability in Nigeria using S2S models. Modeling Earth Systems and Environment. 5, 1405–1428. doi: 10.1007/s40808-019-00606-x
Osei, M. A., Amekudzi, L. K., and Quansah, E. (2021). Characterisation of wet and dry spells and associated atmospheric dynamics at the Pra River catchment of Ghana, West Africa. J. Hydrol. Reg. Stud. 34:100801. doi: 10.1016/j.ejrh.2021.100801
Owusu, K., Obour, P. B., and Asare-Baffour, S. (2015). “Climate variability and climate change impacts on smallholder farmers in the Akuapem North District, Ghana” in Handbook of climate change adaptation. ed. W. Leal Filho (Berlin: Springer), 1791–1806.
Rajulapati, C. R., and Papalexiou, S. M. (2023). Precipitation bias correction: a novel semi-parametric quantile mapping method. AGU Adv. Earth Space Sci. 10:e2023EA002823. doi: 10.1029/2023EA002823
Scheuerer, M., Bahaga, T. K., Segele, Z. T., and Thorarinsdottir, T. L. (2024). Probabilistic rainy season onset prediction over the greater horn of Africa based on long-range multi-model ensemble forecasts. Clim. Dyn. 62, 3587–3604. doi: 10.1007/s00382-023-07085-y
Sivakumar, M. V. K. (1988). Predicting rainy season potential from the onset of rains in southern Sahelian and Sudanian climatic zones of West Africa. Agric. For. Meteorol. 42, 295–305. doi: 10.1016/0168-1923(88)90039-1
Statista (2024a) Ghana—employment by economic sector from 2011 to 2021. Available at: https://www.statista.com/statistics/447530/employment-by-economic-sector-in-ghana/. (Accessed May 28, 2024)
Statista (2024b) Ghana—share of economic sectors in the gross domestic product 2012–2022. Available at: https://www.statista.com/statistics/447524/share-of-economic-sectors-in-the-gdp-in-ghana/. (Accessed May 28, 2024)
Tarnavsky, E., Grimes, D., Maidment, R., Black, E., Allan, R., Stringer, M., et al. (2014). Extension of the TAMSAT satellite-based rainfall monitoring over Africa and from 1983 to present. J. Appl. Meteorol. Clim. 53, 2805–2822. doi: 10.1175/JAMC-D-14-0016.1
Teutschbein, C., and Seibert, J. (2012). Bias correction of regional climate model simulations for hydrological climate-change impact studies: review and evaluation of different methods. J. Hydrol. 456–457, 12–29. doi: 10.1016/j.jhydrol.2012.05.052
Vellinga, M., Arribas, A., and Graham, R. (2013). Seasonal forecasts for regional onset of the West African monsoon. Clim. Dyn. 40, 3047–3070. doi: 10.1007/s00382-012-1520-z
Vitart, F., Ardilouze, C., Bonet, A., Brookshaw, A., Chen, M., Codorean, C., et al. (2017). The subseasonal to seasonal (S2S) prediction project database. Bull. Am. Meteorol. Soc. 98, 163–173. doi: 10.1175/BAMS-D-16-0017.1
Keywords: sub-seasonal, forecasting, decision-making, onset, monsoon, rainfall, Ghana, West Africa
Citation: Thompson EM, Wainwright CM, Hirons LC and Woolnough SJ (2024) Practical application of real-time sub-seasonal monsoon onset forecasting and monitoring for decision-making. Front. Clim. 6:1441647. doi: 10.3389/fclim.2024.1441647
Received: 31 May 2024; Accepted: 07 November 2024;
Published: 12 December 2024.
Edited by:
Ricardo de Camargo, University of São Paulo, BrazilReviewed by:
Srinivas Desamsetti, National Centre for Medium Range Weather Forecasting, IndiaCopyright © 2024 Thompson, Wainwright, Hirons and Woolnough. This is an open-access article distributed under the terms of the Creative Commons Attribution License (CC BY). The use, distribution or reproduction in other forums is permitted, provided the original author(s) and the copyright owner(s) are credited and that the original publication in this journal is cited, in accordance with accepted academic practice. No use, distribution or reproduction is permitted which does not comply with these terms.
*Correspondence: Elisabeth M. Thompson, ZWxpc2FiZXRoLnRob21wc29uQG1ldG9mZmljZS5nb3YudWs=
Disclaimer: All claims expressed in this article are solely those of the authors and do not necessarily represent those of their affiliated organizations, or those of the publisher, the editors and the reviewers. Any product that may be evaluated in this article or claim that may be made by its manufacturer is not guaranteed or endorsed by the publisher.
Research integrity at Frontiers
Learn more about the work of our research integrity team to safeguard the quality of each article we publish.