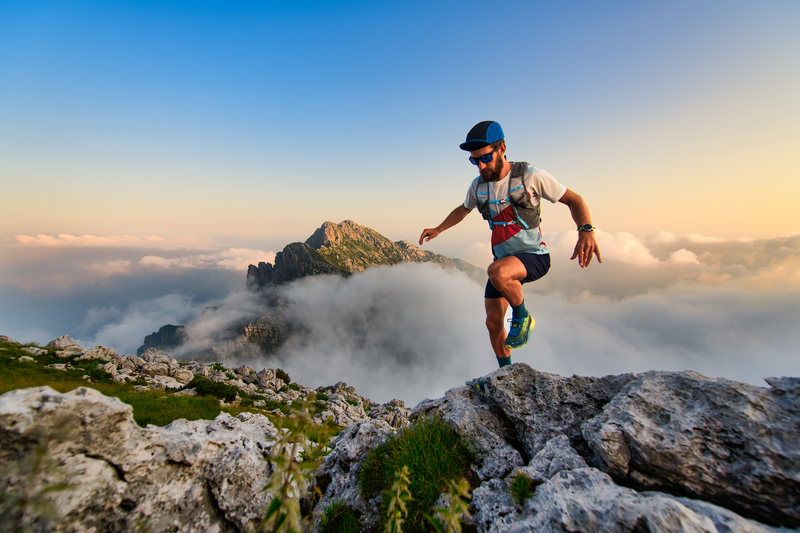
95% of researchers rate our articles as excellent or good
Learn more about the work of our research integrity team to safeguard the quality of each article we publish.
Find out more
ORIGINAL RESEARCH article
Front. Clim. , 01 November 2024
Sec. Predictions and Projections
Volume 6 - 2024 | https://doi.org/10.3389/fclim.2024.1419704
Understanding precipitation properties at regional scales and generating reliable future projections is crucial in providing actionable information for decision-makers, especially in regions with high vulnerability to climate change, where future changes impact ecosystem resilience, biodiversity, agriculture, water resources and human health. The South America Convection-Permitting Regional Climate Model experiment (SA-CPRCM) examines climate change effects in convection-permitting simulations at 4.5 km resolution, on climate time scales (10 year present-day and 10-year future RCP8.5 around 2100), over a domain covering most of South America, using the Met Office Unified Model (UM) convection-permitting RCM.
Under the RCP8.5 scenario, precipitation in the CPRCM decreases, becomes less frequent and more seasonal over the Eastern Amazon region. Dry spells lengthen, increasing the risk of drought. In the Western Amazon, precipitation increases in the wetter austral autumn (Apr. – Jun.) and decreases in the drier austral winter and spring (July – Oct.), leading to a more distinct dry season and imposing a greater risk of contraction of the tropical forest. Over South-eastern Brazil, future precipitation increases and becomes more frequent and more intense, increasing the risk of floods and landslides. A future increase in the intensity of precipitation and extremes is evident over all these regions, regardless of whether the mean precipitation is increasing or decreasing. The CPRCM and its driving GCM respond in a similar way to the future forcing. The models produce broadly similar large-scale spatial patterns of mean precipitation and comparable changes to frequency, intensity, and extremes, although the magnitude of change varies by region and season.
South America was identified in the recent IPCC AR6 reports among the regions with highest vulnerability to climate change (Castellanos et al., 2022; Intergovernmental Panel on Climate Change (IPCC), 2023). The Amazon basin is recognised as a climate change hotspot with an observed sharp increase in the frequency and intensity of severe floods and droughts in the last decades (Barichivich et al., 2018; Garcia et al., 2018; Wagner et al., 2024) together with strong evidence for warming (most notably warmer nights) (Skansi et al., 2013), and increased seasonality (Ritchie et al., 2022). These observed climatic changes, combined with reduction of large-scale precipitation due to deforestation (Smith et al., 2023), increase the forest transformation from a carbon sink to a carbon source (Bennett et al., 2023) and lead to loss of resilience of the Amazon Forest through increased risk of fire (Gatti et al., 2021; Lapola et al., 2023) and reduced evaporation and water recycling in the Amazon basin (Boulton et al., 2022).
Future projections for Amazonia show an increase in maximum temperatures as well as increased length of dry spells and drought severity over the 21st century, even at relatively modest global warming levels of 1.5 and 2 degrees (Marengo et al., 2017; Marengo et al., 2021a; Intergovernmental Panel on Climate Change (IPCC), 2022, 2023; Marengo et al., 2022; Reboita et al., 2022; Ruv Lemes et al., 2023). It has been debated as to whether these changes might lead to shifts in ecosystem structure and composition and risk of dieback (Warszawski et al., 2013; Hirota et al., 2021; Boulton et al., 2022; Parry et al., 2022; Intergovernmental Panel on Climate Change (IPCC), 2023), with much of the focus on the robustness of the precipitation response to climate change in global climate models (Baker et al., 2021).
The highly populated states of Rio de Janeiro and São Paulo in South-eastern Brazil suffer the highest total mortality rates from natural disasters, of which the most impactful are flash floods and landslides (Marengo et al., 2021a; Marengo et al., 2023). During the wet season in southeastern Brazil (Oct. – Apr.), many of these climate impacts, are related to the activity of the South Atlantic convergence zone (SACZ) and to the intensity and persistence of rainfall associated with it (da Fonseca Aguiar and Cataldi, 2021). The SACZ in itself is a part of the South American Monsoon System (SAMS) (Jones and Carvalho, 2002), and is supported by precipitation recycling over the Amazon region via local continental evaporation and moisture transportation from the Amazon to the subtropical regions and South-eastern Brazil by the low-level jet (LLJ) (Gimeno et al., 2020). Projections of future precipitation in this region vary between wetter (Marengo et al., 2012; Jeferson de Medeiros et al., 2022) and drier conditions (Lyra et al., 2018; Jeferson de Medeiros et al., 2022), depending on the ways in which different models simulate the future frequency and activity of the SACZ (Chou et al., 2014) and the location and persistence of cloud band events within it (Zilli et al., 2023). Models also differ in their capability to represent the thermodynamics of convection over the Amazon and the dynamics of southward moisture transport by the LLJ.
High temporal and spatial resolution is crucial for the representation of precipitation in order to assess the risk from these climatological features and in addressing the need for reliable and actionable climate information to inform policy and decision makers (Marengo et al., 2021b; Senior et al., 2021). In recent years, a large number of studies have been conducted using projections of future precipitation change over Brazil derived from GCMs and regional climate models (RCMs), showing a future drying signal over the Amazon basin, combined with increasing rainfall intensity over most of Brazil (Avila-Diaz et al., 2020; da Silva et al., 2023). Future drying increases the risk of drought and fire over northern and northeastern Brazil (Marengo et al., 2017; Vieira et al., 2021), while higher intensities could lead to increased risk of landslides and flash floods in Southern and Southeastern Brazil and the coastal area of Northeast Brazil (Debortoli et al., 2017; Marengo et al., 2021a).
However, most of these studies are using the current generation GCMs or RCMs, which do not explicitly represent deep convection. Furthermore, their resolution prevents them from capturing the magnitude of extreme events and other small-scale features relevant for impact studies (see the comprehensive list of regional climate simulation in Ambrizzi et al., 2019; Table 2). An exception is a recent study by Rehbein and Ambrizzi (2023), who found a reduction in frequency of mesoscale convective systems over the Amazonia in the 2040–2050 period using global NICAM cloud-resolving model (Kodama et al., 2021). Recently, the SAAG-NCAR team conducted a parallel modelling effort using convection-permitting modelling at high spatial resolution across South America (Dominguez et al., 2024). This resulted in a 22-year CPRCM historical simulation and future simulation using a “pseudo-global warming” approach, where the historical run was perturbed with climate change signals from an ensemble of CMIP6 ssp3-7 simulations (as opposed to the dynamic downscaling from a GCM technique used in this study).
Convection-permitting models (CPRCMs) are considered capable of providing climate information at local scale due to two improvements: higher horizontal resolution that enable them to better represent smaller scale geographic features like mountains, rivers, and lakes; and explicit representation of convection (Prein et al., 2013, 2015). These models provide more detailed information, which is essential for the climate impacts community who, with the aid of downstream models can provide more localised climate advice and relevant information for policy making and managements decisions (e.g., Miller et al., 2022).
A similar model configuration to the one used for this study was recently used to study the present and future climate of Africa (CP4-A; Stratton et al., 2018), with an aim to assess the roles that the high resolution and explicit representation of convection have in the ability of climate models to capture climate properties in Africa (Senior et al., 2021). In this model configuration for Africa, the CPRCM shows larger future intensification in extreme 3-hourly precipitation in the rainy season and greater increase in the length of dry spells, compared with the parameterised-convection GCM (Kendon et al., 2019; Berthou et al., 2019a). Similar larger future increases in short-duration precipitation extremes in CPRCMs were found in the UK, US and Europe (see Kendon et al., 2021 for more details).
Despite the CPRCM benefits, which are mainly at sub-daily scales and are often referred to as the added value (Lucas-Picher et al., 2021), these models still have known biases when evaluated against observed distributions of rainfall: mainly that the heavy rainfall at grid-scale can be too intense (Berthou et al., 2019b; Kendon et al., 2021; Halladay et al., 2023). Rowell and Berthou (2023) found that most regions (apart from for large mountains, and to lesser extent coastlines and urban areas) show a closer match between model and observations when spatial aggregation of the 4.5 km CPRCM data to 25 km is performed before the rainfall analysis.
We present results from the future simulation (CPRCM-2100) of the SA-CPRCM experiment. A detailed description of the model configuration and evaluation of the present-day simulations are presented in Halladay et al. (2023), who found that the CPRCM at 4.5 km grid spacing and with no parameterised convection has improved precipitation properties and smaller biases compared with the 25 km driving models which have parameterised convection. The CPRCM was found to have a more realistic precipitation intensity distribution and its diurnal cycle (timing of peak precipitation) agreed better with observations. As in CP4-A, Halladay et al. (2023) have found that these improvements are mainly in the sub-daily scales, where the CPRCM precipitation is less frequent, more intense and occurs later in the day. They also found no clear evidence for a better representation of large-scale climatological features (i.e., mean annual or seasonal precipitation) in the CPRCM (Halladay et al., 2023).
The primary motivation of this study is to provide detailed and relevant information about future climate for decision-makers in South America, from a 10-yr, high resolution, convection-permitting future climate simulation. Our aim is to examine the role that high resolution and explicit representation of convection plays in representing future projections of precipitation by comparing the future projections of different precipitation properties from the CPRCM with the driving GCM.
The CPRCM is termed MOHC-HadREM3-RAL1T-4.5 km (Met Office Hadley Centre Regional Environmental model version 3, Regional Atmosphere Land 1st tropical configuration at 4.5 km) and uses the Met Office Unified Model (UM) version 10.6. It is based on the configuration of the convection-permitting model used for the UK Climate Projections (UKCP18), with tropical setting. The experimental design is based on CP4-A (Stratton et al., 2018). We use the tropical (RAL1-T) configuration (Bush et al., 2020), adapt the CPRCM to the South America domain, update the land cover dataset and modify the aerosol optical properties (see Section 2.3 below). For a more detailed description of the model configuration see Halladay et al. (2023). The CPRCM does not include convective parameterization, and precipitation is calculated by the model dynamics and microphysics schemes. At 4.5 km resolution, deep convection is explicitly resolved, while shallow convective features (as shallow cumulus clouds) are not fully resolved by the model.
The SA-CPRCM experiment includes three simulations, each covering a 10-year period. The high-resolution CPRCM simulations were split into two segments of 6 years (1 year of spin up followed by a 5-year simulation, i.e., 1997–2003, 2002–2008) that were run in parallel, to save computing time. We assessed the continuity of temperature, precipitation and soil moisture time series across the 5-yr segments, i.e., between the end of the first segment in 2002 and the beginning of the second segment (after spinup) in 2003. The continuity indicates that the external forcing likely dominates CPRCM internal variability, particularly on daily and monthly timescales. The CPRCM initial conditions and lateral boundary conditions (LBCs) are provided by the driving RCM and GCM simulations. These simulations were completed prior to the CPRCM experiment and were not split into segments. The CPRCM uses a horizontal grid spacing of ~4.5 km at the equator and 80 vertical levels. The domain is between 400S to 150N, and 850W to 300W, covering the majority of tropical and sub-tropical South and Central America (Figure 1).
Figure 1. Present-day Köppen-Geiger climate zones (from Beck et al., 2018) in the CPRCM domain (400S-150N, 850-300 W), and selected sub-regions for Brazil: Western Amazon (WAMZ): 100S-50N, 750-650 W, Eastern Amazon (EAMZ): 100S-50N, 650-470 W and South-eastern Brazil (SEB) 250-150S, 550-380 W.
The hindcast simulation [CPRCM-ERA, as described in Halladay et al. (2023)] is driven by a reanalysis and performed for 1998–2007. The lateral boundary conditions (LBCs) are provided by the ERA-interim dataset (Dee et al., 2011), dynamically downscaled to the CPRCM 4.5 km resolution using a two-step nesting strategy: ERA-Interim data is first downscaled to 25 km with the Met Office Regional Climate Model (MOHC-HadREM3-GA71-25 km, hereafter: driving RCM) and then into 4.5 km with the CPRCM. This reanalysis-driven hindcast simulation is used to evaluate the model performance against observational datasets, results from which are presented in Halladay et al. (2023).
The present-day simulation (CPRCM-PD) covers the same 1998–2007 period. The LBCs were derived from a global atmosphere-land GCM simulation at 25 km resolution (MOHC-HadGEM3-GA7GL7-N512, hereafter: GCM), downscaled to 4.5 km with the CPRCM.
The future 2100 simulation (CPRCM-2100) is a 10-year simulation, represents a period around 2100 under the high-emissions RCP8.5 scenario, with the initial conditions and LBCs derived from the parallel GCM simulation, downscaled to 4.5 km with the CPRCM. The experimental setup follows that of Stratton et al. (2018) in that we keep the land cover and aerosol forcing fixed at present-day levels (in the CPRCM and in the driving GCM), so only the greenhouse gases (fixed at 2100 values) and sea surface temperatures (SSTs) are modified (see Section 2.3).
The present-day simulation in the GCM and in the CPRCM-PD are forced by time-varying GHGs concentrations for the simulation period (1998–2007). CO2 concentration ranges from 364 ppm in 1998 to 382 ppm in 2007. The future GHG levels are taken from the CMIP5 RCP8.5 protocol for 2100 and do not change throughout the simulation, with CO2 concentration fixed at 936 ppm.
Sea surface temperatures (SSTs) and sea ice are from the present-day daily 0.250 dataset (Reynolds et al., 2007). For the CPRCM-2100 simulation, we added the monthly SST changes between the present (1975–2005) and future (2085–2115) in HadGEM2-ES1 RCP 8.5 simulations to the daily Reynolds dataset, in the same way SSTs changes were applied to the driving GCM, and in the CPRCM simulations for Africa (Stratton et al., 2018; CP4-Africa, Kendon et al., 2019; Senior et al., 2021).
Aerosol forcing and vegetation cover do not change in the future experiment. Land cover data is from the ESA-CCI dataset (ESA, 2017) with plant functional types (PFT) mapping following Hartley et al. (2017) and C3 and C4 grasses information from Powell et al. (2012). Monthly climatology of aerosol levels is prescribed using the EasyAerosol scheme and repeated for each year of the simulation in the CPRCM. The EasyAerosol input was created from the GCM, which uses a fully interactive aerosol scheme (see Halladay et al., 2023 for more details about aerosols and land cover forcing).
The regional analysis in this paper is performed over key sub-regions in Brazil: The Amazon region, and South-eastern Brazil (Figure 1). These regions were selected for their vulnerability to future changes in climate which could lead to potentially significant impacts on their natural and social systems, facing the combined effects of biome shifts, hydrological changes (floods and droughts), landslides and increased fire risks.
The Amazon region (100S-50N, 750-470 W) is divided into the Western Amazon (WAMZ: 750-650 W) and the Eastern Amazon (EAMZ: 650-470 W) as their future precipitation signals differ markedly. The WAMZ, which includes the western part of Amazonas state, Acre and Southern parts of Colombia and Venezuela, is classified mostly as a “tropical rainforest” (Af) by the Köppen-Geiger climate classification, with a band of “tropical Monsoon” (Am) zone in the north and south (Figure 1). The climate classification of this region is projected to have a little change in the future (Beck et al., 2018). The EAMZ is a mixture of “tropical rainforest” (Af), and “tropical Monsoon” (Am). The extent of Af is projected to shrink in the future and the south and eastern parts of the region shift into Tropical Savanna with a dry winter (Aw) (Beck et al., 2018). This region includes the states of Pará, Amapá, Roraima, the eastern part of Amazonas and parts of the Guianas region.
South-eastern Brazil region (SEB: 250-150S, 550-380 W) is a mixture of Monsoon-influenced temperate climates (Cwa, Cwb) with Tropical Savannah (Aw) in the north of the region (Figure 1), which is projected to expand southward in the future. SEB includes the north-eastern part of the La Plata Basin (LPB) and the Brazilian states and federative units of Minas Gerais, Espírito Santo, Rio de Janeiro, São Paulo, North part of Paraná, and parts of Mato Grosso do Sul, Goiás and Mato Grosso.
The CPRCM data is spatially aggregated to 25 km grid space to align with the driving GCM resolution. The aggregation from 4.5 km to 25 km is expected to reduce the precipitation intensity and enhance frequency, but it was shown to be closer to the CPRCMs “effective resolution” (Rowell and Berthou, 2023), and produced a better agreement to hourly precipitation intensity distribution from the Large-Scale Biosphere Atmosphere (LBA) flux tower observations than the CPRCM at its native resolution (see Halladay et al., 2023; Figure 10). The re-gridding was performed using an area-weighted conservative method and allows a direct comparison with the driving GCM, therefore enabling us to explore the effects of high-resolution simulations and convection parameterization.
We present the projected future changes to several precipitation properties (mean precipitation, frequency, intensity, extreme rates, and dry period length) from the CPRCM and the driving GCM, based on sub-daily (3-hourly) data from the models (Table 1).
The relative seasonality index (SI) defined by Walsh and Lawler (1981) evaluates how irregular is the distribution of precipitation throughout the year and assesses the seasonal contrasts regardless of the drying or wetting trend. We found it to be a more accurate way to represent changes in seasonality compared to a simple assessment of the amplitude of the annual cycle (i.e., the difference between the mean precipitation of the wettest and the driest months), especially when a large future change is involved. For example, a future drying trend might decrease the amplitude while, in fact the relative contribution of the wet/dry season might increase, leading to increased/decreased in seasonality. The SI index calculates the monthly deviations from the “average month” and associates them to different rainfall regimes and is defined as:
Where is the mean annual precipitation and is the mean monthly precipitation of month n. The values of SI vary from 0 (when the precipitation is distributed evenly throughout the year) to 1.83 (when all precipitation is concentrated in one month). The SI index was calculated for each region and each year separately and then averaged to create a mean value for the entire 10-year period.
A comparison of the annual cycle of monthly mean precipitation for the 1998–2007 between satellite observations [from the Tropical Precipitation Measurement Mission [TMPA/3B42, TRMM V7; Huffman et al., 2007)], and the CPRCM and GCM simulations is shown in Figure 2. All models capture the annual cycle well, accurately representing the timing of driest and wettest months. The GCM has a consistent wet bias compared with the observations across most regions and months. The CPRCM-PD is wetter than the CPRCM-ERA everywhere, likely due to the wetter boundary conditions provided by its driving GCM. The GCM has a larger wet bias compared to the ERA-Interim-driven RCM, which provides LBCs for the CPRCM-ERA (see also Figure 3G, in Halladay et al., 2023). The CPRCM-PD’s wet bias is more pronounced than the CPRCM-ERA’s for the entire CPRCM domain (Figure 2A), during the drier months in WAMZ (Figure 2B) and from Jul. to Feb. in SEB (Figure 2D). Both CPRCMs accurately simulate the EAMZ monthly climatology for most of the year (Figure 2C) except for a dry bias during the wet months (Jan. – Mar.). Notably, the CPRCM-PD is wetter than its driving GCM during the dry months in the WAMZ (Jun. to Sep.), leading to a larger wet bias and a smaller (and less realistic) annual cycle amplitude in the CPRCM-PD.
Figure 2. Annual cycle of monthly mean precipitation for 1998–2007 from the TRMM V7 satellite observations compared with the CPRCM-ERA, CPRCM-PD and the GCM-PD simulations, for (A) the entire CPRCM domain; (B) Western Amazon; (C) Eastern Amazon; (D) South-eastern Brazil.
The CPRCM projection shows a marked decrease in mean annual precipitation in the Eastern Amazon region (EAMZ), and the eastern parts of tropical South America, and an increase in precipitation over South-eastern Brazil (SEB) (Figure 3C). This large-scale north–south dipole of future drying and wetting is consistent with the driving GCM that provides the lateral boundary conditions (LBCs) for the CPRCM (Figure 3F).
Figure 3. Mean annual precipitation in the CPRCM for (A) 1998–2007, (B) 2100 and (C) the future percentage change, and in the driving GCM (D–F). (G,H) Show the difference between the CPRCM and the GCM and (I) shows the magnitude of the percentage change, defined as the difference between the absolute values of the future percentage change in the CPRCM (C) and in the GCM (F). Areas with smaller percentage change in the CPRCM are marked green in (I) and those with a greater change in the CPRCM are purple (regardless of the future change direction).
Future drying is evident in the EAMZ in all months and in both models, and the relative magnitude of the drying is larger in the CPRCM compared with the driving GCM (indicated by the purple colour over EAMZ in Figures 3, 4A bottom panel and Figures 10A,B). The monthly climatology of precipitation in this region shows a distinct precipitation seasonality with a wet period from December to May and a relatively dry period between June and November (Figure 4A top panel).
Figure 4. Annual cycle of monthly precipitation for the Eastern Amazon (A), western Amazon (B) and southeast Brazil (C). Top panels show the mean monthly precipitation for the CPRCM-PD (green) and CPRCM-2100 (orange). Bottom panels show the percentage change of future precipitation in the CPRCM and the driving GCM.
The relative seasonality in EAMZ is projected to increase, even though the absolute amplitude of the annual cycle is projected to decrease with the future drying (Figure 4A). The relative seasonality index (SI) value for the present-day is 0.37, which corresponds to the rainfall regime described as “Precipitation spread throughout the year, but with a definite wetter season”. In the CPRCM-2100 simulation the SI value increases to 0.55 (“rather seasonal with a short drier season”).
In the Western Amazon (WAMZ) the CPRCM predicts a future increase in mean precipitation in the wet season (Feb. and AMJ) and a decrease in other months (Figure 4B), resulting in a small net annual increase in west of the Amazonas state, surrounded by a small overall decrease in the rest of the WAMZ (Figures 3C, 4B). The contribution of the wet (dry) months to the total precipitation increases (decreases) in the future, leading to an increase in seasonality. This implies a shift from a climate regime characterised by precipitation that is spread throughout the year (SI = 0.14) to a regime with more distinct wet and dry periods (SI = 0.27), with Aug., Sep. and Oct. becoming markedly drier. In the GCM, on the other hand, the present-day WAMZ (which is slightly drier than in the CPRCM) becomes wetter in 2100 (Figures 3G,H). Future increases to the mean precipitation extend from Feb. to Oct. (Figure 4B lower panel) and they are mainly due to larger increases to the future rainfall intensities in the GCM (see Section 3.2 below).
Seasonality in SEB is distinct, with a dry winter (May-Aug.) and wet summer (Nov.-Feb.) (Figure 4C). The annual cycle of the present-day is well simulated in both the CPRCM and the GCM (Halladay et al., 2023). An increase in mean precipitation is projected in SEB by both models, for all months except October (Figure 4C bottom panel) resulting in an increase to the amplitude of the annual cycle, but with only a minor change to seasonality. The SI index for South-eastern Brazil decreases slightly from 0.65 in CPRCM-PD to 0.63 in CPRCM-2100, both values are within the range of the “seasonal rainfall” regime. The future change towards wetter conditions over SEB is more distinct during the dry season (May, Jun., and Aug.) and the wetting is stronger in the CPRCM (Figure 4C bottom panel and Figures 10E,F).
The GCM produces precipitation more frequently than the CPRCM in the present-day and in 2100 (Figures 5G,H), except for the Cordillera Occidental (Western Cordillera of Peru) region in the Central Andes. The differences between the models are largest over Amazonia and over the northeast coast of Tropical South America (Figures 5G,H). The tendency of the GCM to rain too frequently and with lower intensities has been noted in recent studies (Berthou et al., 2019a; Halladay et al., 2023) and leads to larger precipitation bias in the GCM than the CPRCM when compared with satellite observations. Despite those large differences in precipitation frequency between the models, the spatial patterns of future change in both models are very similar (Figures 5C,F): most parts of Brazil, and especially the EAMZ, experience a future decrease in the frequency of wet 3-hourly periods. The relative decrease (i.e., the future percentage change of rainfall frequency) in the CPRCM is larger than the GCM (median for the entire domain: −21.1% and −15.5% respectively) in most regions. An exception is the WAMZ, where the relative decrease in the GCM is larger (Figures 5I, 10C,D).
Figure 5. Annual means of 3-hourly precipitation frequency in the CPRCM and the driving GCM and their future change. As in Figure 3, frequencies are shown for the CPRCM for (A) 1998–2007, (B) 2100 and (C) the future percentage change, and similarly for the driving GCM (D–F). (G,H) Show the difference between the CPRCM and the GCM and (I) shows the magnitude of the percentage change, defined as the difference between the absolute values of the future percentage change in the CPRCM (C) and in the GCM (F). The precipitation frequency is defined as the fraction of 3-hourly periods with mean precipitation rate > 0.1 mm/h.
Figure 6. Projected change in consecutive dry days (CDD), showing the differences between the multi-year mean of the monthly maximum number of consecutive dry days for 2100 and the present day, in the CPRCM (left) and in the GCM (right).
In SEB, the future annual precipitation frequency shows little change (−2% in the CPRCM, −4% in the GCM; Figures 5C,F). Both models predict more frequent precipitation during the dry season (JJA), and very small decrease to the precipitation frequency in the wet season (DJF) (Figures 10E,F), indicating that the overall increase in precipitation arises from increased intensity.
The less frequent future precipitation in Amazonia is accompanied by longer dry spells in 2100 as indicated by the increase in the monthly maximum number of consecutive dry days (CDD) (Figure 6). Both models predict an increase in dry spells length over the EAMZ, but the CPRCM signal expands further west and into the WAMZ (Figure 6).
The dry spells are projected to lengthen in the EAMZ by 3–8 days in the CPRCM (Figure 7) and by 0.5–7 days in the GCM (not shown). Smaller increases are projected for the WAMZ (0–2.5 days in the CPM, −0.5–2 days in the GCM) for most months, apart from drier months of Aug. and Sep. when the CPRCM projection indicates a doubling of the dry spell’s length (Figure 7).
In SEB dry spells are projected to shorten slightly (Figure 6). The signal is more enhanced and more widespread in the CPRCM, extending from SEB towards the Brazilian Highlands and parts of Northeast Brazil. The shortening over SEB is mainly in the dry season between May and Aug. (Figure 7), in line with the overall increase in precipitation during this season reported in Section 3.1. However, future lengthening of dry spells is evident during Mar.-Apr. and Sep.-Oct. (Figure 6), suggesting a longer period (Mar. – Oct.) where the maximum monthly CDD is larger than 10 days.
The precipitation intensity of the wet 3-hourly periods in the CPRCM is higher than in the driving GCM of both the present day and 2100 (Figures 8G,H). The highest intensities in the CPRCM-PD are over the WAMZ (Figure 8A), whereas in the GCM-PD, it does not stand out as the region of highest intensity. Halladay et al. (2023) have shown that the CPRCM overestimates the mean intensity at least compared with satellite observations, but also that the coarser 25 km resolution RCM underestimates the mean and the extreme (99th percentile) intensities over most of the domain.
Figure 8. Annual means of 3-hourly precipitation intensity in the CPRCM and the driving GCM and their future change. The precipitation intensity is defined as the mean precipitation of the “wet” 3-hourly periods (> 0.1 mm/h) in the CPRCM (upper row), the driving GCM (middle row) and the difference between them (lower row), for the present day (left), 2100 (middle) and percentage of future change (right). (I) Shows the magnitude of the percentage change (see Figure 3).
Precipitation intensity is projected to increase by ~1 mm/h. (~ 20%) over the WAMZ in the CPRCM-2100 simulation (Figures 8B,C). The GCM predicts a larger future intensification over this region in 2100 (~50%; Figure 8F), which makes the WAMZ (together with the southeastern coast of Brazil) stand out as regions of high intensity, similarly to the CPRCM. SEB shows an annual increase of ~30% in future intensities in both models (Figures 8C,F) and both seasons (Figures 10E,F). The EAMZ shows the smallest increases in annual intensities (median for the entire domain: 9% in the CPRCM and 8% in the GCM) (Figures 8C,F), with higher increases in the wet season (MAM Figure 10A).
Extreme precipitation rates are higher in the CPRCM than in the GCM for both the present-day, and in 2100 (Figures 9G,H). An increase to the future extreme rates is projected by both models and for all seasons in the CPRCM and for most regions in the GCM (Figures 9C,F, 10). The CPRCM shows largest annual increases over SEB (~45%) and WAMZ (34%) and smallest increases over the Central Andes and EAMZ (~24%). The GCM shows a similar spatial pattern, with a similar increase over SEB (45%), and a smaller increase over the EAMZ (14%) but a greater increase over WAMZ (76%) (Figures 9F,I, 10).
Figure 9. Annual means of the extreme precipitation rates in the CPRCM and the driving GCM and their future change. The extreme precipitation rates are defined as the intensity of the 99th percentile of the wet (> 0.1 mm/h) 3-hourly precipitation. Showing here for the CPRCM (upper row), the driving GCM (middle row) and the difference between them (lower row), for the present day (left), 2100 (middle) and percentage of future change (right). (I) shows the magnitude of the percentage change (see Figure 3).
Figure 10. Percentage difference for “mean precipitation”, “frequency”, “intensity” and the “99th percentile” of the wet 3-hourly periods, between the 2100 climate and the present-day simulations, for the wet season (left column) and dry season (right column) in different regions. Showing the percentage change between the regional median values. CPRCM in blue, GCM in green. Note that the wet and dry seasons can be different for each region and are defined as the nearest “climatological season” from the data presented in Figure 4.
The precipitation intensity distribution in the CPRCM-PD and CPRCM-2100 are compared with those of the GCM and with estimates from the TRMM V7 in Figure 11. We calculated the fractional contribution of different precipitation intensities using the ASoP (Analysing Scales of Precipitation) method (Klingaman et al., 2017) and presented them in a way that the area under the curve represent the total amount of precipitation (see Berthou et al., 2020 for more details). The CPRCM-PD distributions are generally closer to the TRMM satellite observation, while the GCM tends to overestimate the contribution from lower precipitation intensities (between 1 and 10 mm/3 h) in all regions.
Figure 11. Fractional contribution of 3-hourly precipitation intensity for the EAMZ (A,B), the WAMZ (C,D) and for SEB (E,F). Wet season intensity distributions are in the left column and dry season in the right. TRMM v7 satellite observations are shown in black solid line. The CPRCM data is represented as solid lines and the driving GCM as dotted lines, present day curves are green and future projections are in red. The area under the curve represents the mean precipitation.
The intensity distributions capture many of the future precipitation signals discussed above: in the EAMZ there is a slight shift towards higher intensities (although it is the smallest shift among the three regions) and a clear reduction in the total precipitation. This is evident in both the wet and dry season and in both models.
In the WAMZ the shift towards future higher intensities in the CPRCM is clearer than in the EAMZ and the enhanced contribution from heavy precipitation is more pronounced in the wet season. The reduction in total amount in the WAMZ is only evident in the dry season. The GCM shows a very strong shift towards higher intensities and extremes (at the far higher tail of the distribution) as shown earlier in this section (Figures 8, 9).
In SEB there is a clear shift towards higher intensities in the future combined with enhanced contribution from higher intensities and a general increase in the total precipitation amount in all seasons and in both models.
We have presented results from a convection-permitting simulation on a climate time scale from the SA-CPRCM experiment, performed with the Met Office Unified Model CPRCM at 4.5 km resolution. We have focused on projected precipitation changes in key regions around 2100, based on the high-end RCP 8.5 scenario (without land-use change). A detailed description of the model configuration and evaluation of the present-day simulations are presented in Halladay et al. (2023), who found that the CPRCM showed clear improvements in sub-daily precipitation characteristics and representation of precipitation extremes over Brazil and Tropical South America compared with the coarser (25 km) driving RCM and GCM simulations (which use parameterised convection).
For the present-day, the GCM shows larger wet precipitation biases from observational and satellite data (Figure 2), and it overestimates the annual mean precipitation due to the GCM’s tendency to produce precipitation too frequently and in lower intensities (Halladay et al., 2023). The intensity of extreme events is too low in the driving model, and too high in the CPRCM, when compared to gridded observational datasets. The CPRCM intensities are in better agreement with local gauging stations (Halladay et al., 2023). Despite those differences, the CPRCM and its driving GCM respond in a similar way to the future forcing. Both models project comparable patterns of change to the mean precipitation and to other precipitation properties, with some regional variations in the magnitude of changes. A notable discrepancy is in the WAMZ dry season. Here, the CPRCM-PD has a larger wet bias than the GCM, and project future drying, whereas the GCM suggests only a slight change to the mean precipitation accompanied by more pronounced intensification. The enhanced drying may be attributed to the different rates of precipitation interception by vegetation and infiltration into the ground between CPRCMs and lower-resolution models with parametrised convection, an area currently under investigation. This finding diverges from previous studies in other regions, which typically observed a more pronounced intensification of short-duration rainfall in CPRCMs relative to their driving GCMs (Berthou et al., 2019b; Kendon et al., 2021).
At the large, multi-annual scale, both models predict a future drying over Amazonia and wetter conditions over Southeastern Brazil. This large-scale north–south dipole of future drying and wetting is consistent with previous studies of CMIP5 (Alves et al., 2021; Reboita et al., 2022) and have shown it to be a robust feature (i.e., shared across at least 75% of the models) among CMIP6 models (Parsons, 2020; Almazroui et al., 2021; Ortega et al., 2021; Jeferson de Medeiros et al., 2022). The fine details, and in particular the position of the transition zone between the wetter south and the drier north are highly dependent on the model and are associated with the properties of the SACZ and with the location and persistence of cloud band events within it (Zilli et al., 2023). In our simulation the future wetter conditions are expanding northward towards the Brazilian Highlands (Figure 3C), while in most CMIP6 models they are limited to Uruguay and Argentina and to the La Plata Basin in Southern Brazil (see for example Alves et al., 2021; Figure 3A).
We found a future increase in precipitation, in both the CPRCM and the GCM in the states of Rio de Janeiro and São Paulo in South-eastern Brazil, with a larger percentage increase during the drier, austral winter (JJA) (Figures 10E,F). Increased precipitation intensity is the primary driver of wetter conditions during the wetter austral summer (DJF), while in the drier austral winter (JJA), both enhanced intensity and frequency, along with shorter dry spells, contribute to overall projected precipitation increase. This could exacerbate flood and landslide risks throughout the year in this densely populated region, potentially causing significant impacts, and notably during the dry season, traditionally less prone to such hazards.”
Future wetter conditions have been suggested before for SEB, based on simulations of the Eta regional model driven by HadCM3 (Marengo et al., 2012), and are supported by the CMIP5 simulations of HadGEM2-ES RCP8.5 (see for example Reboita et al., 2022; Figure 4). However, recent studies using the Eta2 model at higher resolutions (20 km and 5 km) found a marked decrease in precipitation over SEB and the large metropolitan regions within it during the rainy season (the austral summer, DJF) (Chou et al., 2014; Mourão et al., 2016; Lyra et al., 2018), and associated this decrease to reduction in the frequency of the SACZ and its future activity (Chou et al., 2014). It has already been noted by Reboita et al. (2022) that while CMIP5 GCMs project wetter conditions over southeast Brazil, the Eta model projects drying.
Despite the general reduction in total precipitation, Lyra et al. (2018) show a small increase in precipitation during the drier, austral winter (JJA) in parts of the region and note an increase the magnitude of heavy rainfall over some areas in this region and in the number of extreme rainfall events. The authors also note that the precipitation associated with the SACZ in DJF over the historical period was underestimated by the model.
An increase in intensity and extreme precipitation was also found by Jeferson de Medeiros et al. (2022) for Earth System models (ESMs) from CMIP3, CMIP5 and CMIP6. As the heavily populated states of São Paulo and Rio de Janeiro are located near the transition between wetter and drier domains, and their future climate is highly sensitive to future changes of the SACZ, further investigation is required to determine the future precipitation properties in these geographically and socially complex regions.
The future drying over the EAMZ (and during the drier season over WAMZ) is accompanied by increased seasonality in the future climate and longer dry spells. The WAMZ, where precipitation is currently spread throughout the year, is projected to have more seasonal contrasts in rainfall and a more defined drier season during the austral winter (JASO) with longer dry spells. An increase in seasonality over most parts of South America in CMIP6 models was found by Almazroui et al. (2021) and by Ritchie et al. (2022) who show that the projected temperature seasonal cycle amplitude the Amazon has already increased in the last three decades and projected to increase further in the future. An increase in the length of dry spells over the Amazon has also been shown by recent studies of Reboita et al. (2022), Jeferson de Medeiros et al. (2022; for CMIP6 models), and da Silva et al. (2023), and supports the finding of Alves et al. (2021), showing more frequent future drier intervals but also wetter wet periods in Brazil on timescales from daily to seasonal. A more pronounced dry season between Jul. to Oct. could lead to greater water resources variability in Amazonia and to water scarcity with risk of seasonal drought in the region and potential impact on the evolution of natural vegetation and is consistent with the projections of Beck et al. (2018), showing a future contraction of the tropical rainforest (Af) over Amazonia based on CMIP5 models RCP8.5 projections.
We found a clear signal of increasing future intensity and extreme precipitation rates, over regions of both wetter and drier future in both models. Areas with projected increased mean precipitation (e.g., SEB and WAMZ during the wet season) show increased intensities (SEB: Figures 8C,F, 10E; WAMZ: Figure 10C), while precipitation frequency remains relatively stable or slightly decreases. This suggests that increased precipitation intensity, rather than frequency, is the primary driver of higher mean precipitation in these regions. Conversely, areas with projected decreased mean precipitation (e.g., EAMZ and WAMZ during the dry season) show either stable or slightly enhanced precipitation intensities (EAMZ: Figures 10A,B; WAMZ: Figure 10C), indicating that reduced precipitation frequency is the primary factor contributing to the drying trend.
Precipitation becomes less frequent over most parts of Brazil (apart from SEB in the dry season), and for Amazonia a drier future will be characterised by less frequent but higher intensity rainfall events. These findings are consistent with Jeferson de Medeiros et al. (2022) who suggested that the occurrence of heavy rainfall will be more severe in the future and concentrated in fewer events.
A similar enhanced contribution from heavy precipitation over areas of both future wetting and drying was noted for Africa by Berthou et al. (2019b). However, whereas results of the CP4-A show a consistently greater intensification of 3-hourly precipitation in the CPRCM than in the driving GCM, over South America, we found smaller and less consistent differences between the models that vary by sub-region. For example, in the WAMZ and SEB the GCM shows a greater intensification (in percent change), although the intensity of precipitation (in absolute values) is still higher in the CPRCM. Also, the values of future extreme precipitation at the high end of the distribution in the GCM can be higher than in the CPRCM (Figure 11), a feature that has not been identified before and requires further analysis.
The signal of change in the EAMZ (reduction in mean, and frequency, longer dry spells and an increase in intensity and extremes) is stronger in the CPRCM and in SEB both models respond in a similar way (and magnitude) to the future climate forcing.
We note that the results presented here are from a single CPRCM model simulation, driven by high end (RCP8.5) scenario and therefore cannot reflect the range of possible future projections. Additionally, in order to comprehensively evaluate the impacts of the projected changes presented in this manuscript, it is essential to quantify them at the subregional level using the CPRCM and GCM outputs, and employing metrics directly relevant to end-users. However, the improvement in the CPRCM precipitation characteristics over the driving GCM and better resemblance to observations, as presented in Halladay et al. (2023) give us greater confidence in these climate projections and we wish to see the CPRCM projections, combined with wider range of climate information from state-of-the-art models, being used to inform impact studies in the water resources, agriculture, ecosystems, and urban sectors in the region.
Ongoing and future work is focused on understanding the robustness of the CPRCM projections, through a detailed assessment of the possible mechanisms and their dynamic interpretation. To be able to provide reliable projections of future hazards like floods, landslides, and droughts in SEB, and to understand changes to rainfall characteristics over the Amazon for example, we need a more detailed investigation into the dynamic and the processes that govern them. This includes a better understanding of the models’ biases regarding the South America monsoon features (onset, peak and length), and its components (e.g., the position and intensity of the SACZ and its associated cloud bands, and the characteristics of the moisture transport from the Amazon to SEB by the LLJ). Another area of future work is an assessment of the response of the natural vegetation to the drying conditions and of a possibility of Amazon dieback based on our findings of a more distinct dry season in the WAMZ during Aug.-Oct., as well as looking into projected deforestation scenarios over the Amazon rainforest and their effects on local precipitation and the southward moisture transport. These aims require Earth system models with dynamic vegetation, and preferably an ensemble of CPRCMs simulations to account for the uncertainty in the future projections.
Exploring further the results of this CPRCM and comparing it to other CPRCM studies for this region holds immense promise for advancing our understanding of regional climate and weather patterns. Refining CPRCMs to simulate more complex regional climate phenomena, such as the interactions between land, atmosphere, and ocean at high resolutions, could provide valuable insights into local storm dynamics. Furthermore, coupling CPMs with other Earth system components could contribute to a more comprehensive understanding of regional climate change impacts and contribute to more reliable future projections.
The raw data supporting the conclusions of this article will be made available by the authors, without undue reservation.
RK: Conceptualization, Data curation, Formal analysis, Investigation, Methodology, Software, Validation, Visualization, Writing – original draft, Writing – review & editing. KH: Conceptualization, Data curation, Methodology, Software, Validation, Writing – review & editing. LA: Conceptualization, Methodology, Writing – review & editing. RC: Conceptualization, Methodology, Writing – review & editing. AH: Conceptualization, Methodology, Visualization, Writing – review & editing.
The author(s) declare that financial support was received for the research, authorship, and/or publication of this article. All authors were supported by the Newton Fund through the Met Office Climate Science for Service Partnership Brazil (CSSP Brazil). Lincoln Alves was supported by São Paulo Research Foundation – FAPESP (grant no. 2022/08622-0). Open Access funding enabled and organized by CSSP Brazil.
We thank Nick Savage, Simon Tucker, Carwyn Pelley, Ben Johnson, and Giorgia Fosser for technical assistance and to Marcia Zilli for fruitful discussions that greatly improved the manuscript.
The authors declare that the research was conducted in the absence of any commercial or financial relationships that could be construed as a potential conflict of interest.
The author(s) declared that they were an editorial board member of Frontiers, at the time of submission. This had no impact on the peer review process and the final decision.
All claims expressed in this article are solely those of the authors and do not necessarily represent those of their affiliated organizations, or those of the publisher, the editors and the reviewers. Any product that may be evaluated in this article, or claim that may be made by its manufacturer, is not guaranteed or endorsed by the publisher.
1. ^Met Office Hadley Centre global Environmental model version 2 with Earth System components.
2. ^All the RCMs and GCMs mentioned here are using parameterised convection.
Almazroui, M., Ashfaq, M., Islam, M. N., Rashid, I. U., Kamil, S., Abid, M. A., et al. (2021). Assessment of CMIP6 performance and projected temperature and precipitation changes over South America. Earth Syst. Environ. 5, 155–183. doi: 10.1007/s41748-021-00233-6
Alves, L. M., Chadwick, R., Moise, A., Brown, J., and Marengo, J. A. (2021). Assessment of rainfall variability and future change in Brazil across multiple timescales. Int. J. Climatol. 41, E1875–E1888. doi: 10.1002/joc.6818
Ambrizzi, T., Reboita, M. S., da Rocha, R. P., and Llopart, M. (2019). The state of the art and fundamental aspects of regional climate modeling in South America. Ann. N. Y. Acad. Sci. 1436, 98–120. doi: 10.1111/nyas.13932
Avila-Diaz, A., Benezoli, V., Justino, F., Torres, R., and Wilson, A. (2020). Assessing current and future trends of climate extremes across Brazil based on reanalyses and earth system model projections. Clim. Dyn. 55, 1403–1426. doi: 10.1007/S00382-020-05333-Z
Baker, J. C. A., Garcia-Carreras, L., Buermann, W., Castilho De Souza, D., Marsham, J. H., Kubota, P. Y., et al. (2021). Robust Amazon precipitation projections in climate models that capture realistic land–atmosphere interactions. Environ. Res. Lett. 16:074002. doi: 10.1088/1748-9326/ABFB2E
Barichivich, J., Gloor, E., Peylin, P., Brienen, R. J. W., Schöngart, J., Espinoza, J. C., et al. (2018). Recent intensification of Amazon flooding extremes driven by strengthened Walker circulation. Sci. Adv. 4:eaat8785. doi: 10.1126/sciadv.aat8785
Beck, H. E., Zimmermann, N. E., McVicar, T. R., Vergopolan, N., Berg, A., and Wood, E. F. (2018). Present and future Köppen-Geiger climate classification maps at 1-km resolution. Sci. Data 5, 1–12. doi: 10.1038/sdata.2018.214
Bennett, A. C., Rodrigues de Sousa, T., Monteagudo-Mendoza, A., Esquivel-Muelbert, A., Morandi, P. S., Coelho de Souza, F., et al. (2023). Sensitivity of south American tropical forests to an extreme climate anomaly. Nat. Clim. Chang. 13, 967–974. doi: 10.1038/s41558-023-01776-4
Berthou, S., Kendon, E. J., Chan, S. C., Ban, N., Leutwyler, D., Schär, C., et al. (2020). Pan-European climate at convection-permitting scale: a model intercomparison study. Clim. Dyn. 55, 35–59. doi: 10.1007/s00382-018-4114-6
Berthou, S., Kendon, E. J., Rowell, D. P., Roberts, M. J., Tucker, S., and Stratton, R. A. (2019a). Larger future intensification of rainfall in the west African Sahel in a convection-permitting model. Geophys. Res. Lett. 46, 13299–13307. doi: 10.1029/2019GL083544
Berthou, S., Rowell, D. P., Kendon, E. J., Roberts, M. J., Stratton, R. A., Crook, J. A., et al. (2019b). Improved climatological precipitation characteristics over West Africa at convection-permitting scales. Clim. Dyn. 53, 1991–2011. doi: 10.1007/s00382-019-04759-4
Boulton, C. A., Lenton, T. M., and Boers, N. (2022). Pronounced loss of Amazon rainforest resilience since the early 2000s. Nat. Clim. Chang. 12, 271–278. doi: 10.1038/s41558-022-01287-8
Bush, M., Allen, T., Bain, C., Boutle, I., Edwards, J., Finnenkoetter, A., et al. (2020). The first met Office unified model–JULES regional atmosphere and land configuration, RAL1. Geosci. Model Dev. 13, 1999–2029. doi: 10.5194/gmd-13-1999-2020
Castellanos, E. J., Lemos, M. F., Astigarraga, L., Chacón, N., Cuvi, N., Huggel, C., et al. (2022). “Chapter 12: central and South America,” in Climate change 2022: Impacts, adaptation and vulnerability – working group II contribution to the sixth assessment report of the intergovernmental panel on climate change. Cambridge University Press, 1689–1816.
Chou, S. C., Lyra, A., Mourão, C., Dereczynski, C., Pilotto, I., Gomes, J., et al. (2014). Assessment of climate change over South America under RCP 4.5 and 8.5 downscaling scenarios. Am. J. Clim. Chang. 3, 512–527. doi: 10.4236/ajcc.2014.35043
da Fonseca Aguiar, L., and Cataldi, M. (2021). Social and environmental vulnerability in Southeast Brazil associated with the South Atlantic convergence zone. Nat. Hazards 109, 2423–2437. doi: 10.1007/s11069-021-04926-z
da Silva, M. L., de Oliveira, C. P., Esilva, C. M. S., and de Araújo, J. M. (2023). Analysis of climate extremes indices in tropical South America through the RegCM4.7. Int. J. Climatol. 43, 4506–4531. doi: 10.1002/joc.8100
Debortoli, N. S., Camarinha, P. I. M., Marengo, J. A., and Rodrigues, R. R. (2017). An index of Brazil’s vulnerability to expected increases in natural flash flooding and landslide disasters in the context of climate change. Nat. Hazards 86, 557–582. doi: 10.1007/s11069-016-2705-2
Dee, D. P., Uppala, S. M., Simmons, A. J., Berrisford, P., Poli, P., Kobayashi, S., et al. (2011). The ERA-interim reanalysis: configuration and performance of the data assimilation system. Q. J. R. Meteorol. Soc. 137, 553–597. doi: 10.1002/qj.828
Dominguez, F., Rasmussen, R., Liu, C., Ikeda, K., Prein, A., Varble, A., et al. (2024). Advancing south American water and climate science through multidecadal convection-permitting modeling. Bull. Am. Meteorol. Soc. 105, E32–E44. doi: 10.1175/BAMS-D-22-0226.1
ESA (2017). Land Cover CCI Product User Guide Version 2. Tech. Rep. [WWW Document]. Available at: maps.elie.ucl.ac.be/CCI/viewer/download/ESACCI-LC-Ph2-PUGv2_2.0.pdf
Garcia, B., Libonati, R., and Nunes, A. (2018). Extreme drought events over the Amazon Basin: the perspective from the reconstruction of south American Hydroclimate. Water 10:1594. doi: 10.3390/w10111594
Gatti, L. V., Basso, L. S., Miller, J. B., Gloor, M., Gatti Domingues, L., Cassol, H. L. G., et al. (2021). Amazonia as a carbon source linked to deforestation and climate change. Nature 595, 388–393. doi: 10.1038/s41586-021-03629-6
Gimeno, L., Vázquez, M., Eiras-Barca, J., Sorí, R., Stojanovic, M., Algarra, I., et al. (2020). Recent progress on the sources of continental precipitation as revealed by moisture transport analysis. Earth Sci. Rev. 201:103070. doi: 10.1016/j.earscirev.2019.103070
Halladay, K., Kahana, R., Johnson, B., Still, C., Fosser, G., and Alves, L. (2023). Convection-permitting climate simulations for South America with the met Office unified model. Clim. Dyn. 61, 5247–5269. doi: 10.1007/s00382-023-06853-0
Hartley, A. J., MacBean, N., Georgievski, G., and Bontemps, S. (2017). Uncertainty in plant functional type distributions and its impact on land surface models. Remote Sens. Environ. 203, 71–89. doi: 10.1016/J.RSE.2017.07.037
Hirota, M., Flores, B. M., Betts, R., Borma, L. S., Esquivel-Muelbert, A., Jakovac, C., et al. (2021). “Chapter 24: resilience of the Amazon forest to global changes: assessing the risk of tipping points” in Amazon assessment report 2021 (UN Sustainable Development Solutions Network (SDSN)).
Huffman, G. J., Bolvin, D. T., Nelkin, E. J., Wolff, D. B., Adler, R. F., Gu, G., et al. (2007). The TRMM multisatellite precipitation analysis (TMPA): quasi-global, multiyear, combined-sensor precipitation estimates at fine scales. J. Hydrometeorol. 8, 38–55. doi: 10.1175/JHM560.1
Intergovernmental Panel on Climate Change (IPCC) (2022). “Impacts of 1.5°C global warming on natural and human systems” in Global warming of 1.5°C (Cambridge University Press), 175–312.
Intergovernmental Panel on Climate Change (IPCC) (2023). “Technical summary” in Climate change 2021 – the physical science basis (Cambridge University Press), 35–144.
Jeferson de Medeiros, F., Prestrelo de Oliveira, C., and Avila-Diaz, A. (2022). Evaluation of extreme precipitation climate indices and their projected changes for Brazil: from CMIP3 to CMIP6. Weather Clim. Extrem. 38:100511. doi: 10.1016/J.WACE.2022.100511
Jones, C., and Carvalho, L. M. V. (2002). Active and break phases in the south American monsoon system. J. Clim. 15, 905–914. doi: 10.1175/1520-0442(2002)015<0905:AABPIT>2.0.CO;2
Kendon, E. J., Prein, A. F., Senior, C. A., and Stirling, A. (2021). Challenges and outlook for convection-permitting climate modelling. Philos. Trans. R. Soc. A Math. Phys. Eng. Sci. 379:20190547. doi: 10.1098/rsta.2019.0547
Kendon, E. J., Stratton, R. A., Tucker, S., Marsham, J. H., Berthou, S., Rowell, D. P., et al. (2019). Enhanced future changes in wet and dry extremes over Africa at convection-permitting scale. Nat. Commun. 10:1794. doi: 10.1038/s41467-019-09776-9
Klingaman, N. P., Martin, G. M., and Moise, A. (2017). ASoP (v1.0): a set of methods for analyzing scales of precipitation in general circulation models. Geosci. Model Dev. 10, 57–83. doi: 10.5194/GMD-10-57-2017
Kodama, C., Ohno, T., Seiki, T., Yashiro, H., Noda, A. T., Nakano, M., et al. (2021). The nonhydrostatic ICosahedral atmospheric model for CMIP6 HighResMIP simulations (NICAM16-S): experimental design, model description, and impacts of model updates. Geosci. Model Dev. 14, 795–820. doi: 10.5194/gmd-14-795-2021
Lapola, D. M., Pinho, P., Barlow, J., Aragão, L. E. O. C., Berenguer, E., Carmenta, R., et al. (2023). The drivers and impacts of Amazon forest degradation. Science 379:eabp8622. doi: 10.1126/science.abp8622
Lucas-Picher, P., Argüeso, D., Brisson, E., Tramblay, Y., Berg, P., Lemonsu, A., et al. (2021). Convection-permitting modeling with regional climate models: latest developments and next steps. Wiley Interdiscip. Rev. Clim. Chang. 12:e731. doi: 10.1002/WCC.731
Lyra, A., Tavares, P., Chou, S. C., Sueiro, G., Dereczynski, C., Sondermann, M., et al. (2018). Climate change projections over three metropolitan regions in Southeast Brazil using the non-hydrostatic eta regional climate model at 5-km resolution. Theor. Appl. Climatol. 132, 663–682. doi: 10.1007/s00704-017-2067-z
Marengo, J. A., Camarinha, P. I., Alves, L. M., Diniz, F., and Betts, R. A. (2021a). Extreme rainfall and hydro-geo-meteorological disaster risk in 1.5, 2.0, and 4.0°C global warming scenarios: an analysis for Brazil. Front. Clim. 3:610433. doi: 10.3389/fclim.2021.610433
Marengo, J. A., Chou, S. C., Kay, G., Alves, L. M., Pesquero, J. F., Soares, W. R., et al. (2012). Development of regional future climate change scenarios in South America using the eta CPTEC/HadCM3 climate change projections: climatology and regional analyses for the Amazon, São Francisco and the Paraná River basins. Clim. Dyn. 38, 1829–1848. doi: 10.1007/s00382-011-1155-5
Marengo, J. A., Espinoza, J.-C., Fu, R., Jimenez Muñoz, J. C., Muniz Alves, L., Ribeiro da Rocha, H., et al. (2021b). “Chapter 22: long-term variability, extremes, and changes in temperature and hydro meteorology” in Amazon assessment report 2021 (UN Sustainable Development Solutions Network (SDSN)).
Marengo, J. A., Galdos, M. V., Challinor, A., Cunha, A. P., Marin, F. R., Vianna, M. D. S., et al. (2022). Drought in Northeast Brazil: a review of agricultural and policy adaptation options for food security. Clim. Resil. Sustain. 1:e17. doi: 10.1002/cli2.17
Marengo, J. A., Seluchi, M. E., Cunha, A. P., Cuartas, L. A., Goncalves, D., Sperling, V. B., et al. (2023). Heavy rainfall associated with floods in southeastern Brazil in November–December 2021. Nat. Hazards 116, 3617–3644. doi: 10.1007/s11069-023-05827-z
Marengo, J. A., Torres, R. R., and Alves, L. M. (2017). Drought in Northeast Brazil—past, present, and future. Theor. Appl. Climatol. 129, 1189–1200. doi: 10.1007/s00704-016-1840-8
Miller, J. D., Vischel, T., Fowe, T., Panthou, G., Wilcox, C., Taylor, C. M., et al. (2022). A modelling-chain linking climate science and decision-makers for future urban flood management in West Africa. Reg. Environ. Chang. 22, 1–15. doi: 10.1007/S10113-022-01943-X/FIGURES/5
Mourão, C., Chou, S. C., and Marengo, J. (2016). Downscaling climate projections over La Plata Basin. Atmos. Clim. Sci. 6, 1–12. doi: 10.4236/acs.2016.61001
Ortega, G., Arias, P. A., Villegas, J. C., Marquet, P. A., and Nobre, P. (2021). Present-day and future climate over central and South America according to CMIP5/CMIP6 models. Int. J. Climatol. 41, 6713–6735. doi: 10.1002/joc.7221
Parry, I. M., Ritchie, P. D. L., and Cox, P. M. (2022). Evidence of localised Amazon rainforest dieback in CMIP6 models. Earth Syst. Dynam. 13, 1667–1675. doi: 10.5194/ESD-13-1667-2022
Parsons, L. A. (2020). Implications of CMIP6 projected drying trends for 21st century Amazonian drought risk. Earth’s Future 8:e2020EF001608. doi: 10.1029/2020EF001608
Powell, R. L., Yoo, E.-H., and Still, C. J. (2012). Vegetation and soil carbon-13 isoscapes for South America: integrating remote sensing and ecosystem isotope measurements. Ecosphere 3, 1–25. doi: 10.1890/ES12-00162.1
Prein, A. F., Gobiet, A., Suklitsch, M., Truhetz, H., Awan, N. K., Keuler, K., et al. (2013). Added value of convection permitting seasonal simulations. Clim. Dyn. 41, 2655–2677. doi: 10.1007/s00382-013-1744-6
Prein, A. F., Langhans, W., Fosser, G., Ferrone, A., Ban, N., Goergen, K., et al. (2015). A review on regional convection-permitting climate modeling: demonstrations, prospects, and challenges. Rev. Geophys. 53, 323–361. doi: 10.1002/2014RG000475
Reboita, M. S., Kuki, C. A. C., Marrafon, V. H., de Souza, C. A., Ferreira, G. W. S., Teodoro, T., et al. (2022). South America climate change revealed through climate indices projected by GCMs and eta-RCM ensembles. Clim. Dyn. 58, 459–485. doi: 10.1007/s00382-021-05918-2
Rehbein, A., and Ambrizzi, T. (2023). Mesoscale convective systems over the Amazon basin in a changing climate under global warming. Clim. Dyn. 61, 1815–1827. doi: 10.1007/s00382-022-06657-8
Reynolds, R. W., Smith, T. M., Liu, C., Chelton, D. B., Casey, K. S., and Schlax, M. G. (2007). Daily high-resolution-blended analyses for sea surface temperature. J. Clim. 20, 5473–5496. doi: 10.1175/2007JCLI1824.1
Ritchie, P. D. L., Parry, I., Clarke, J. J., Huntingford, C., and Cox, P. M. (2022). Increases in the temperature seasonal cycle indicate long-term drying trends in Amazonia. Commun. Earth Environ. 3:199. doi: 10.1038/s43247-022-00528-0
Rowell, D. P., and Berthou, S. (2023). Fine-scale climate projections: what additional fixed spatial detail is provided by a convection-permitting model? J. Clim. 36, 1229–1246. doi: 10.1175/JCLI-D-22-0009.1
Ruv Lemes, M., Sampaio, G., Fisch, G., Alves, L. M., Maksic, J., Guatura, M., et al. (2023). Impacts of atmospheric CO2 increase and Amazon deforestation on the regional climate: a water budget modelling study. Int. J. Climatol. 43, 1497–1513. doi: 10.1002/joc.7929
Senior, C. A., Marsham, J. H., Berthou, S., Burgin, L. E., Folwell, S. S., Kendon, E. J., et al. (2021). Convection-permitting regional climate change simulations for understanding future climate and informing decision-making in Africa. Bull. Am. Meteorol. Soc. 102, E1206–E1223. doi: 10.1175/BAMS-D-20-0020.1
Skansi, M. D. L. M., Brunet, M., Sigró, J., Aguilar, E., Arevalo Groening, J. A., Bentancur, O. J., et al. (2013). Warming and wetting signals emerging from analysis of changes in climate extreme indices over South America. Glob. Planet. Chang. 100, 295–307. doi: 10.1016/j.gloplacha.2012.11.004
Smith, C., Baker, J. C. A., and Spracklen, D. V. (2023). Tropical deforestation causes large reductions in observed precipitation. Nature 615, 270–275. doi: 10.1038/s41586-022-05690-1
Stratton, R. A., Senior, C. A., Vosper, S. B., Folwell, S. S., Boutle, I. A., Earnshaw, P. D., et al. (2018). A Pan-African convection-permitting regional climate simulation with the met office unified model: CP4-Africa. J. Clim. 31, 3485–3508. doi: 10.1175/JCLI-D-17-0503.1
Vieira, R. M. D. S. P., Tomasella, J., Barbosa, A. A., Martins, M. A., Rodriguez, D. A., Rezende, F. S. D., et al. (2021). Desertification risk assessment in Northeast Brazil: current trends and future scenarios. Land Degrad. Dev. 32, 224–240. doi: 10.1002/ldr.3681
Wagner, F. H., Favrichon, S., Dalagnol, R., Hirye, M. C., Mullissa, A., and Saatchi, S. (2024) “Amazon’s 2023 drought: Sentinel-1 reveals extreme Rio Negro River contraction”. arXiv. Available at: http://arxiv.org/abs/2401.16393 (accessed April 3, 2024).
Walsh, R. P. D., and Lawler, D. M. (1981). Rainfall seasonality: description, spatial patterns and change through time. Weather 36, 201–208. doi: 10.1002/j.1477-8696.1981.tb05400.x
Warszawski, L., Friend, A., Ostberg, S., Frieler, K., Lucht, W., Schaphoff, S., et al. (2013). A multi-model analysis of risk of ecosystem shifts under climate change. Environ. Res. Lett. 8:044018. doi: 10.1088/1748-9326/8/4/044018
Zilli, M. T., Hart, N. C. G., Coelho, C. A. S., Chadwick, R., de Souza, D. C., Kubota, P. Y., et al. (2023). Characteristics of tropical–extratropical cloud bands over tropical and subtropical South America simulated by BAM-1.2 and HadGEM3-GC3.1. Q. J. R. Meteorol. Soc. 149, 1498–1519. doi: 10.1002/qj.4470
Keywords: climate change, convection-permitting model, precipitation projections, future changes, South America, Brazil
Citation: Kahana R, Halladay K, Alves LM, Chadwick R and Hartley AJ (2024) Future precipitation projections for Brazil and tropical South America from a convection-permitting climate simulation. Front. Clim. 6:1419704. doi: 10.3389/fclim.2024.1419704
Received: 18 April 2024; Accepted: 27 August 2024;
Published: 01 November 2024.
Edited by:
Charles Jones, University of California, Santa Barbara, United StatesReviewed by:
Laura Harrison, University of California, Santa Barbara, United StatesCopyright © 2024 Kahana, Halladay, Alves, Chadwick and Hartley. This is an open-access article distributed under the terms of the Creative Commons Attribution License (CC BY). The use, distribution or reproduction in other forums is permitted, provided the original author(s) and the copyright owner(s) are credited and that the original publication in this journal is cited, in accordance with accepted academic practice. No use, distribution or reproduction is permitted which does not comply with these terms.
*Correspondence: Ron Kahana, cm9uLmthaGFuYUBtZXRvZmZpY2UuZ292LnVr
Disclaimer: All claims expressed in this article are solely those of the authors and do not necessarily represent those of their affiliated organizations, or those of the publisher, the editors and the reviewers. Any product that may be evaluated in this article or claim that may be made by its manufacturer is not guaranteed or endorsed by the publisher.
Research integrity at Frontiers
Learn more about the work of our research integrity team to safeguard the quality of each article we publish.