- 1Carbon Solutions, Saint Paul, MN, United States
- 2Department of Chemical and Biological Engineering, Princeton University, Princeton, NJ, United States
- 3National Renewable Energy Laboratory, Golden, CO, United States
- 4Department of Chemical and Petroleum Engineering, University of Calgary, Calgary, AB, Canada
Introduction: Liquid-solvent direct air capture (DAC) is a prominent approach for carbon dioxide removal but knowing where to site these systems is challenging because it requires considering a multitude of interrelated geospatial factors. Two of the most pressing factors are: (1) how should DAC be powered to provide the greatest net removal of CO2 and (2) how does weather impact its performance?.
Methods: To investigate these questions, this study develops a process-level model of a liquid-solvent DAC system and couples it to a 20-year dataset of temperature and humidity conditions at a ~9km resolution across the contiguous US.
Results and discussion: We find that the amount of CO2 sequestered could be 30% to 50% greater than the amount of CO2 removed from the atmosphere if natural gas is burned on site to power DAC, but that the optimal way to power DAC is independent of capture rate (i.e., weather), depending solely on the upstream GHG intensity of electricity and natural gas. Regardless of how it is powered, air temperature and humidity conditions can change the performance of DAC by up to ~3x and can also vary substantially across weather years. Across the continuous US, we find that southern states (e.g., Gulf Coast) are preferrable locations for a variety of reasons, including higher and less variable air temperature and relative humidity. Lastly, we also find the performance of liquid-solvent DAC calculated with monthly means is within 2% of the estimated performance calculated with hourly data for more than a third of the country, including in the states with weather most favorable for liquid-solvent DAC.
1 Introduction
There are two primary reasons why carbon dioxide removal (CDR) technologies may be essential components of future economies. First, unless global CO2 emissions peak by 2025 or earlier, achieving a 1.5°C or 2°C climate warming goal will require removing CO2 that was previously emitted from the atmosphere (IPCC, 2022). For reference, the growth of global energy-related CO2 emissions was positive (i.e., 0.9%), reaching a new high of over 36.8 GtCO2 in 2022 (IEA, 2023). As such, and supported by recent carbon budget estimates, the window for limiting global warming to 1.5°C without CDR is rapidly closing (Friedlingstein et al., 2022). Second, prior work has also demonstrated it may be less expensive to transition to net-zero carbon economies instead of absolute zero carbon economies due to some technologies, processes, or sectors that are “hard to decarbonize” (Davis et al., 2018). In this role, CDR would be necessary to offset these residual “hard to decarbonize” emissions (Larson et al., 2020; EPRI and GTI Energy, 2022).
There are multiple technologies and approaches for CDR and across them, direct air capture (DAC) is one of the most prominent. DAC is a system built for the sole purpose of CDR and has been referred to as a “backstop technology” because as much DAC as needed could, in theory, be deployed (National Academies of Sciences, 2019; Fuhrman et al., 2023). When coupled with geologic CO2 storage, DAC provides CDR through multiple steps: atmospheric CO2 is absorbed or adsorbed into a solvent or solid material, electricity and heat are used to detach the captured CO2 and compress it, and then that CO2 is sequestered to permanently isolate it from the atmosphere. Depending on the cost and availability of other CDR approaches, DAC deployment sufficient to provide gigatonne-levels of CDR may be required by 2050 (Fuhrman et al., 2023).
There are many challenges to scaling DAC to the gigatonne level and, among them, is knowing where to best deploy the technology. Siting DAC is difficult because it requires simultaneously answering a multitude of interrelated geospatial questions relating to a variety of factors including CO2 storage, energy, weather, environmental impacts (e.g., water consumption), cost, environmental justice, and community acceptance. This study is focused on two of these questions: (1) what energy source(s) provide the greatest net removal of CO2?; and (2) in what locations (in the contiguous United States) are weather conditions most conducive to favorable DAC performance?
DAC is very energy intensive. For example, given the deployment levels of DAC required to achieve climate targets and current energy efficiencies, prior work has found DAC alone in 2100 may require as much as half the total global energy consumed in 2016 (Realmonte et al., 2019). But identifying where this energy should come from in a decarbonized future is difficult. For one, different energy sources have different GHG intensities, and this can impact the overall performance of DAC (Hanna et al., 2021; Terlouw et al., 2021). Further, when integrated into the energy system, prior work has shown that, given its high cost, it is optimal for DAC to provide as much CDR as possible, thus be paired with energy sources that are continuously available (Breyer et al., 2020; Bistline and Blanford, 2021; Hanna et al., 2021). These two difficulties drive the energy challenges related to siting DAC because energy sources that (a) have low carbon footprints and (b) are available continuously are not geospatially ubiquitous. When the other factors, such as cost or land-use availability of these resources are also considered, the challenge grows even more difficult.
There are a wide variety approaches for DAC that are being developed and in this study, we build on our prior work that focuses on liquid-solvent DAC (An et al., 2022). Liquid-solvent DAC is sometimes referred to as “high-temperature DAC” because the process requires 900°C heat to liberate CO2, that was captured from the atmosphere, from the liquid solvent (Keith et al., 2018). Given the high temperature of this thermal load, natural gas is typically considered for the energy source but recent work has also considered liquid-solvent DAC heated with electric resistance heating (Pett-Ridge et al., 2023). The electrical requirements of liquid-solvent DAC could be met from the electricity grid (or off-grid renewables) or with an on-site natural gas turbine with CO2 capture. Electricity is used by nearly all the units that comprise the DAC system and the primary electric loads are for the CO2 compressor, the air separation unit, and the fans moving air across the air contactor.
In addition to energy, the performance of liquid-solvent DAC is also influenced by ambient air temperature and relatively humidity, as demonstrated in our prior work that found liquid-solvent DAC performance increased with increasing humidity and temperature (An et al., 2022). As such, the optimal locations for deploying DAC will also be influenced by the weather, which substantially increases the complexity of siting DAC given the geospatial complexities of weather. Despite this fact, we are aware of only two studies investigating the geospatial ramifications of weather to DAC performance. The first, Sendi et al. (2022) uses hourly temperature and humidity data for 5 years (2016–2020) and focuses on solid-sorbent (a.k.a., “low-temperature” DAC). The second, Pett-Ridge et al. (2023) studies liquid-solvent DAC systems and uses daily temperature and humidity averages, forecasted by a variety of models in 2050, to inform a county-level resolution analysis.
In this paper, we estimate the performance of liquid-solvent DAC across the contiguous United States at a fine-resolution both geospatially and temporally. This is novel for multiple reasons. First, we use hourly temperature and relative humidity dataset across twenty years (2000–2019) at a ~ 9 km resolution, which totals approximately 12 billion datapoints. To our knowledge, this is the highest resolution dataset yet used to study DAC performance. Further, as this large dataset requires both computational resources and expertise to use, we also investigate, for the first time, when and if using monthly averages of the weather data is an appropriate approximation.
Additionally, our study is also novel in that we improve the process-level modeling from our prior work (An et al., 2022) in multiple ways to further investigate how liquid-solvent DAC could be powered. In our prior work, we did not explicitly model the scenario where electricity demand was met by the grid and instead used the results from the on-site natural gas turbine scenario to approximate the electric loads and amount of natural gas required for the calciner. As the CO2 flow through the system decreases when the electricity demand is powered by the grid, this reduces the quantity of natural gas required in the calciner and the quantity of electricity consumed by CO2 compression. In this study, we relax this simplification from our prior work by explicitly modeling the scenario in which electricity demand is met with the grid.
2 Methods and materials
At a high-level, our methodology consists of two tasks:
1. We built a model of a generic 1 MtCO2/yr. solvent-based DAC system using the Institute for the Design of Advanced Energy Systems (IDAES) framework. Using this model, we simulated the performance of DAC under three different modes of operation across a wide range of CO2 capture rates. Section 2.1 describes this portion of our methods work in more detail.
2. Using a response model derived from our prior work (An et al., 2022) and the IDAES results, we estimated the geospatial performance of the generic 1 MtCO2/yr. system using temperature and relative humidity data. Section 2.2 describes this portion of our methods in more detail.
2.1 Process-level model of liquid solvent DAC
Our IDAES model extends the simulations completed in our prior work (An et al., 2022) and is grounded in the work done by Keith et al. (2018). As shown in Figure 1, the system consists of four primary units: the air contactor, the pellet reactor, the calciner, and the steam slaker. In the air contactor, the CO2 in the air contacts a solution of potassium hydroxide, where it reacts to form potassium carbonate. In the pellet reactor, the potassium carbonate reacts with calcium hydroxide to form calcium carbonate, which precipitates out of solution. The calcium carbonate is washed and goes to the calciner, where high heat drives off the CO2, producing calcium oxide. The calcium oxide then enters the steam slaker, where calcium hydroxide is regenerated. Section 1 of the Supplementary material provides a more detailed discussion of the assumptions for the IDAES model.
2.1.1 Modes of operation
The energy requirements of a liquid-solvent direct air capture system take two forms (Keith et al., 2018): the heat required to achieve 900°C in the calciner, and the electricity required to power all process units. These energy requirements can be met by three different combinations of natural gas and electricity, as outlined in Figure 2 and listed below:
1. Natural gas is burned on site to provide both the heat in the calciner and to power a combustion turbine that provides electricity. In this study, we refer to this mode as “all natural gas.”
2. Natural gas is burned on site to provide the heat in the calciner but the grid is used for all electricity requirements. In this study, we refer to this mode as “mixed.”
3. An electric calciner is used and all electricity demand is met with the grid. In this study, we refer to this mode as “all grid.” To our knowledge, the technical feasibility of an electric calciner for a 1 MtCO2/yr. DAC system has not yet been assessed in the peer-reviewed literature, but we include it in this study because it has been considered in prior life cycle and techno-economic assessments (Liu et al., 2020; McQueen et al., 2021).
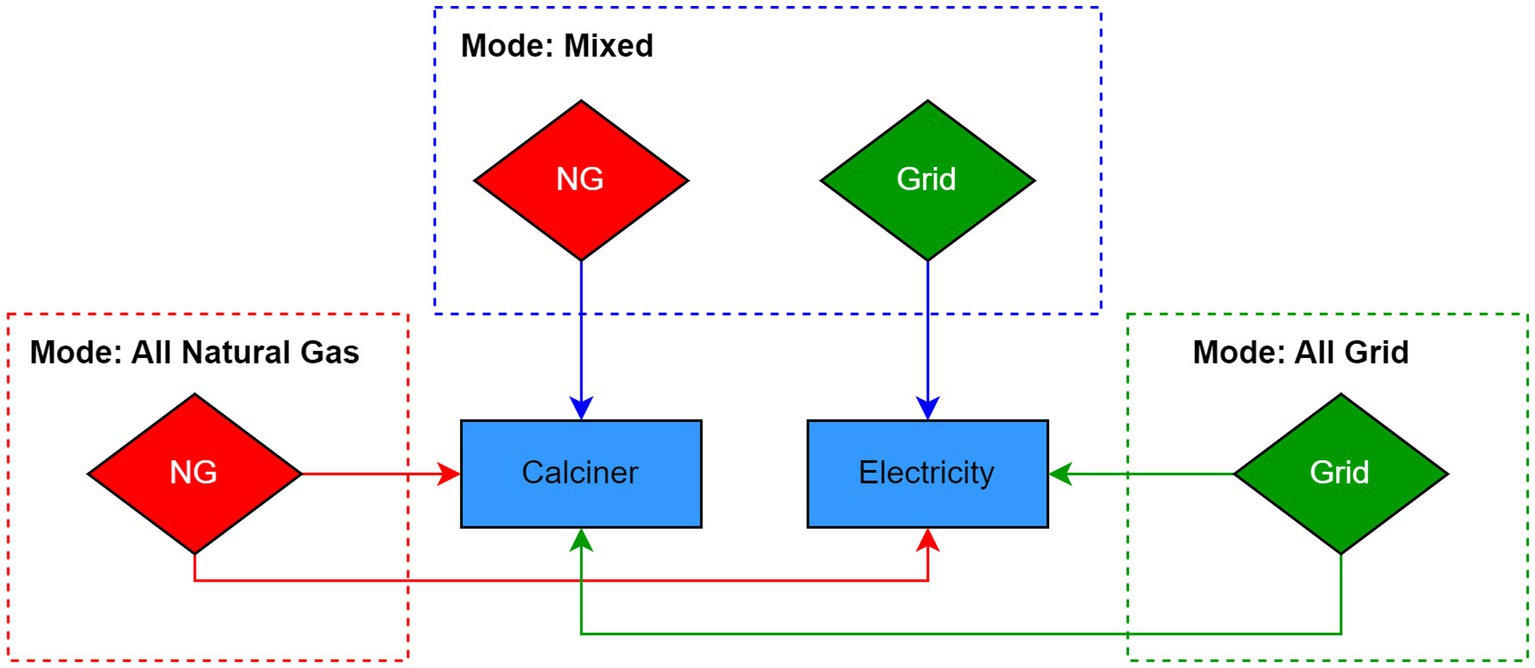
Figure 2. Summary of different modes for energy sources. More detailed process diagrams for each of these modes are available in Section 1.2 of the Supplementary material.
Except for the “all grid” mode, the total amount of CO2 that must be sequestered by DAC will be greater than the total amount of CO2 captured from the atmosphere for two primary reasons. First, additional CO2 is generated and captured on site from the natural gas combustion in the calciner and/or turbine. In the “all natural gas” mode, for example, 90% of the CO2 generated from natural gas in the combustion turbine is captured in a CO2 absorber, which is mixed with the CO2 captured from the atmosphere. The remaining 10% of the CO2 enters the air contactor along with the CO2 from the atmosphere. The CO2 generated from the natural gas in the calciner is inherently mixed with the CO2 driven off from CaCO3, and thus all CO2 generated in the calciner is captured. Second, this increase in CO2 flow through the system also increases the electricity demand of some components of the DAC process, which in turn requires more natural gas combustion. The largest example of this is the CO2 compressor, which requires substantially more power as more CO2 is captured, thus requiring more natural gas combustion on site to provide that power. Our IDEAS simulations fully captures these feedback loops.
2.1.2 CO2 Removal ratio as a function of GHG intensity of energy
We use the IDAES model to find the preferred energy mode of operation that provides the greatest net removal of CO2 over the use phase of the DAC system. This portion of our study allows us to answer, for example, when “all grid” mode is preferable to the “all natural gas” mode. As the net removal of CO2 depends on the upstream greenhouse gas (GHG) intensity of natural gas and electricity, we calculate and use a “CO2 removal ratio” to identify preferred modes of operation across different CO2 capture rates. The CO2 removal ratio metric, R, is calculated with Eq. 1:
Where Q is the quantity of GHGs emitted by the energy sources (i.e., electricity, natural gas) upstream of the DAC system, G is the quantity of CO2 that is generated and released (i.e., the small portion that is not captured) on site from natural gas combustion, and A is the quantity of CO2 captured from the atmosphere by the DAC system. Q is a function of the GHG intensity of electricity and natural gas, and in this study, we estimate the upstream CO2e emissions using a range of assumed GHG intensities: 0 to 70 gCO2e/MJ for natural gas and 0 to 1,000 gCO2e/kWh for electricity. We vary the GHG intensities over ranges (instead of just a case study example of a single set of GHG intensities, for example) because the GHG intensities both vary geospatially across the United States. Further, it is likely that they will also change in the future as more clean energy is deployed on the grid and upstream leakage of natural gas is reduced. As such, these ranges of upstream GHG intensity of natural gas and electricity were chosen to encompass the full range of values observed for each energy source in the United States, down to zero. These values include the full life cycle emissions of generating natural gas and electricity as calculated by GREET (ANL, 2023). Further, the quantity of CO2 captured, A, includes only the CO2 captured from the atmosphere and not the additional CO2 captured from on-site natural gas combustion. We also estimate the “net sequestration” which is the quantity of CO2 captured from the atmosphere (not including additional CO2 captured from on-site natural gas combustion), less the sum of the quantity of CO2e emitted by the energy sources upstream of the DAC facility and the quantity of CO2 that is generated and released on site from natural gas combustion.
2.2 Weather data
In this study, we used hourly temperature and relative humidity data for the years 2000 through 2019 from the ERA5-Land dataset (Munoz Sabater, 2019), post-processed by the European Centre for Medium-Range Weather Forecasts. ERA5-Land provides a consistent view of the variability in meteorological variables over several decades at ~9 km horizontal resolution. We selected a reanalysis dataset because it combines model data with observations into a complete and consistent framework representing the physics of the climate system, including climate decades into the past. Across the temporal and spatial boundaries of this study, this dataset included about 12 billion datapoints. Section 2.2.1 describes how this dataset was processed and Section 2.2.2 describes how we applied it to estimate DAC performance. Additional analyses that contextualize and explore the weather data are presented through a time series, exploratory factor analysis, and a sensitivity analysis in Sections 3.1 to 3.3 of the Supplementary material.
2.2.1 Data processing
The ERA5-Land climate reanalysis dataset provided hourly temperature and dewpoint temperature data for the Contiguous United States (CONUS) covering the 20-year period, 2000–2019. ERA5-Land data were processed in Google Earth Engine (Gorelick et al., 2017), involving filtering and masking to extract the relevant temperature and dewpoint temperature values. As relative humidity was not directly available in the ERA5 dataset, we calculate it using Tetens formula (Tetens, 1930), which is derived from the Clausius-Clapeyron equation. This approach ensured consistency in the calculation of relative humidity across the entire study area. Section 2.3 of the Supplementary material provides more information on the application of geospatial data in Google Earth Engine.
To facilitate an evaluation of the impact of time sampling on estimated DAC sequestration, monthly means of temperature and relative humidity were calculated from the hourly values. This resulted in the creation of companion monthly time series of temperature and relative humidity.
2.2.2 Applying weather data to estimate DAC performance
Both hourly and monthly temperature and relative humidity values were filtered to exclude all hours (or months) when the temperature fell outside the range of 0°C to 40°C. This temperature range is based on our prior work (An et al., 2022), and represents the operation range of the reaction–diffusion process within the DAC system. If the temperature is too low (i.e., about −2°C), the liquid-solvent will freeze, thus halting the operation of the air contactors within the DAC facility. While there is nothing preventing the DAC system from running above 40°C, published data on the kinetics of CO2 absorption into the solvent is only available up to this temperature (An et al., 2022).
To estimate the capture rate variability across the 20-year period and for each location within CONUS, we employed a multi-step approach. First, we developed regression equations using results from our previous work (An et al., 2022) and the IDAES simulations. These equations enabled us to calculate the CO2 capture rate for each grid point and time step. These equations relate capture rate to temperature and relative humidity parameters and are provided in Section 2.1 of the Supplementary material.
Next, we utilized the capture rate values alongside the formulas presented in Section 2 of the Supplementary material to determine the CO2 mass flow rate in metric tons per hour (or month). As seen in Section 2.2 of the Supplementary material, these formulas are regressed from the IDAES simulations, and account for how the mass and energy flows and operating parameters change based on the capture rate and energy modes. Finally, to gain a broader perspective on annual performance variations, we aggregated the hourly (monthly) mass flow rates to annual scales for each location. This multi-step approach, encompassing regressed equations from prior work and IDAES simulations that include detailed system-specific calculations, enabled us to capture the intricate interplay between temperature and humidity conditions, technology characteristics, and resulting CO2 capture performance across the diverse geographical landscape of the Contiguous US.
2.2.3 DAC performance metric: quantity of CO2 sequestered
For this portion of our analysis, we present the geospatial performance of the 1 MtCO2/yr DAC system using one metric: the quantity of CO2 sequestered. We use the term “sequestered” to differentiate from the term “captured” because this metric (i.e., CO2 sequestered) is defined as the sum of the quantity of CO2 captured from the atmosphere and the amount of CO2 captured from any on-site natural gas combustion. Unlike our process-level results, the CO2 sequestered does not account for the upstream GHG intensity of the energy because the upstream GHG intensities of natural gas and electricity change geospatially. Further, the total CO2 sequestered is not affected by the small quantity of CO2 that is generated and released (i.e., not captured) on site from natural gas combustion. As a result of using CO2 sequestration as the performance metric (and not the net sequestration), we ensure that all geospatial and temporal differences in DAC performance are driven entirely by differences in weather and not a combination of weather and the upstream GHG intensity of energy. In other words, the total CO2 sequestration is the quantity of CO2 that must be permanently isolated from the atmosphere downstream of the DAC facility. As such, for this study, more favorable weather conditions result in higher CO2 sequestration, thus higher DAC performance.
3 Results
We first provide the process-level results of our IDAES simulations (Section 3.1) before discussing the performance of DAC as a function of weather (Section 3.2) and the sensitivity of DAC performance to the time-sampling of the weather data (Section 3.3). Different metrics are used across these results sections. As described in Section 2.1.2, we use the process-level modeling results (Section 3.1) to gain insight into how liquid-solvent DAC should be powered to provide the greatest net-removal of CO2. This portion of our study thus incorporates the upstream GHG intensity of the energy sources. But as described in Section 2.2.3, the weather results (Sections 3.2 and 3.3) do not include the GHG intensity of the energy sources to ensure that all geospatial and temporal differences in DAC performance are driven entirely by differences in weather. Before presenting our results, we provide a summary reference of the definitions of these metrics:
• CO2 capture rate is the percent of CO2 captured from the atmosphere at the air contactor, which changes because of changes in air temperature and relative humidity.
• The CO2 removal ratio is defined with Eq. 1.
• The net sequestration is the quantity of CO2 captured from the atmosphere (not including additional CO2 captured from on-site natural gas combustion), less the sum of the quantity of CO2e emitted by the energy sources upstream of the DAC facility and the quantity of CO2 that is generated and released on site from natural gas combustion.
• CO2 sequestered is the quantity of CO2 that must be permanently isolated from the atmosphere, downstream of the DAC facility. For example, if the DAC facility is using geologic CO2 storage for sequestration, this would be the quantity of CO2 injected into the subsurface. This includes both the CO2 captured from the atmosphere and from on-site natural gas combustion.
3.1 DAC process-level modeling results
Table 1 shows the amount of CO2 sequestered for every tonne of CO2 captured from the atmosphere for the three different energy modes modeled in IDAES. The total amount of CO2 sequestered is greater than the amount captured from the atmosphere when natural gas is burned on site for two reasons, described in Section 2.1.1. As shown in Table 1, when both these reasons are accounted for in our IDAES model, the additional amount of CO2 can account for upwards of ~50% of the total amount of CO2 captured if natural gas is burned on-site to provide all process heat and power requirements.
Figure 3 shows the maximum CO2 removal ratio and corresponding net sequestration rate as a function of the upstream GHG intensity of both natural gas (y-axis) and electricity (x-axis) for two different air contactor capture rates: 50% in A) and 90% in B). Please see Section 2.1.2 for a definition of the CO2 removal ratio and net sequestration.
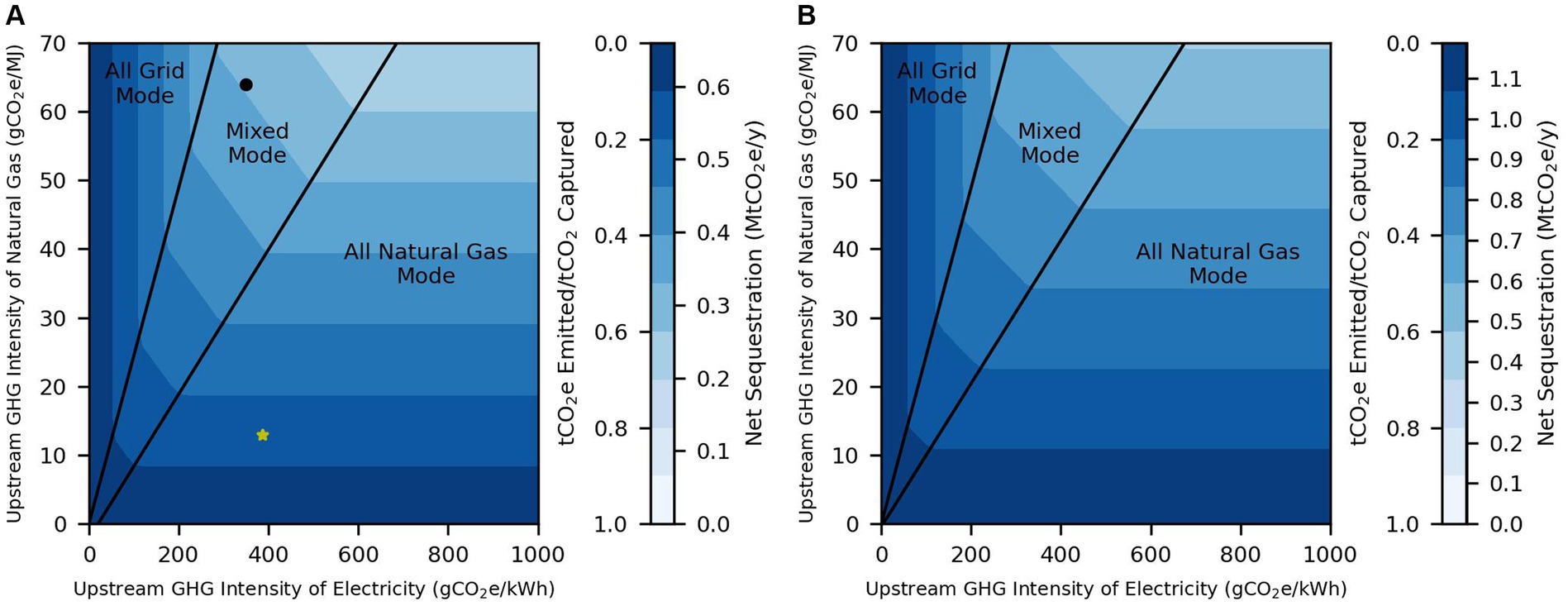
Figure 3. Maximum CO2 removal ratio as a function of the upstream GHG intensity of natural gas and electricity for (A) 50% capture rate; (B) 90% capture rate. The solid black lines indicate the boundary of where each energy mode results in the lowest emissions based on the upstream GHG intensity of electricity and natural gas. The two points in (A) are added to give a reference of current upstream GHG intensity combinations: the black dot shows the Permian basin and Western Electricity Coordinating Council (WECC) average (350 gCO2e/kWh and 64 gCO2e/MJ) as an approximation for New Mexico (Chen et al., 2022); and the yellow star shows the average in the USA (387 gCO2e/kWh and 13 gCO2e/MJ), based on data from the Greenhouse gases, Regulated Emissions, and Energy use in Technologies (GREET) Model (ANL, 2023).
As shown on the right side of both subplots of Figure 3, when the GHG intensity of electricity is high enough, the “all natural gas” mode is preferred because the upstream GHG intensity from electricity is greater than the upstream GHG intensity of natural gas. As the upstream GHG intensity of electricity emissions decreases (i.e., moving to the left in the subplots), the “mixed mode” becomes preferred, and then at very low upstream GHG intensity of electricity, the “all grid” mode becomes preferred. As seen with the two reference points in Figure 3, there are locations in the United States in which the “mixed mode” and “all natural gas mode” would result in the maximum CO2 removal ratio. For example, on average in the USA (yellow star), the “all natural gas mode” results in the greatest CO2 removal ratio, and is thus would be the preferred energy mode. In New Mexico for comparison (black dot), the “mixed mode” is preferred because the GHG intensity of electricity decreases and the GHG intensity of natural gas increases relative to the USA average. And, if GHG intensity of electricity decreases enough in New Mexico (i.e., below ~300 gCO2e/kWh), all else equal (i.e., moving the black dot to the left), the “all grid mode” would become preferred.
Figure 3 also shows that the net CO2 sequestration increases with increasing capture rate. For example, the dark blue color indicates a net CO2 sequestration greater than 0.6 MtCO2/yr. in Figure 3A and a net CO2 sequestration greater than 1.1 MtCO2/yr. in Figure 3B. This difference between these subplots occurs because less CO2 is captured from the atmosphere when the annual CO2 capture rate is 50%, as in A), compared to 90%, as in B). Despite this difference, the boundary line where different operational modes are preferable changes very minimally as a function of CO2 capture rate. As such, Figure 3 demonstrates that the optimal mode of operation is independent of the CO2 capture rate (i.e., weather conditions) and is thus only a function of the upstream GHG intensities of both natural gas and electricity. This finding is consistent with our prior work and can be seen even more clearly in Section 1.4 of the Supplementary material.
3.2 DAC performance as a function of weather
Figure 4 shows the average total CO2 sequestered across all 20 years of weather data, demonstrating the rich complexity of differences in performance of high-temperature DAC across the contiguous United States. In other words, Figure 4 shows how much CO2 would need to be sequestered if the same 1 MtCO2/yr. liquid-solvent DAC facility was constructed across the United States. As stated in Section 2.2.2, this does not account for the upstream GHG intensity of natural gas or electricity so that all geospatial differences observed can be entirely contributed to changes in weather.
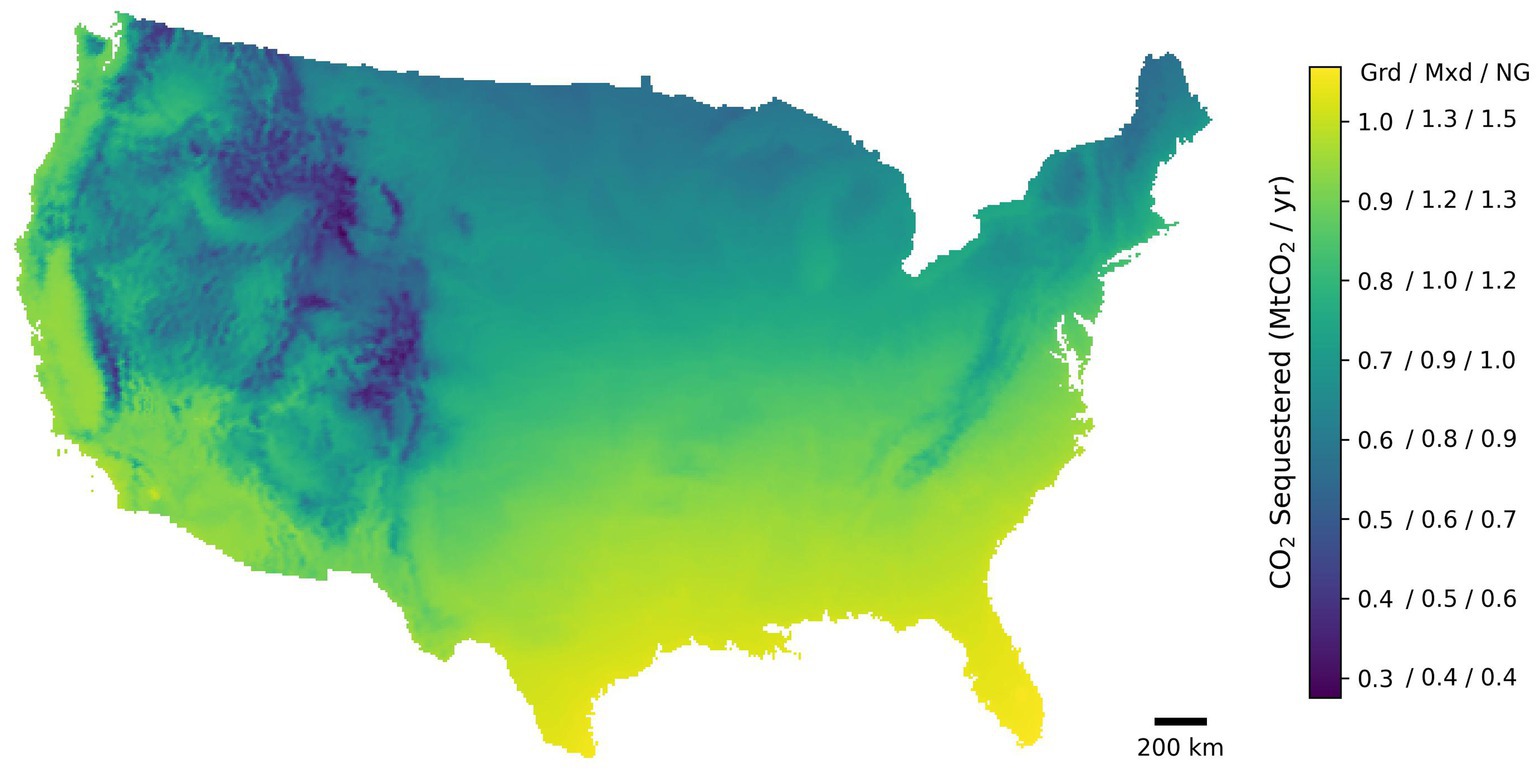
Figure 4. Total CO2 sequestration averaged across all 20 years of weather data. The color bar shows the differences between the three energy mode scenarios (grid, mixed, natural gas). As previously discussed in Table 1, the three energy mode options yield different quantities of total CO2 sequestered due to on-site natural gas combustion.
Figure 4 shows the clear impact of latitude and, as expected, the potential for CO2 sequestration decreases with increasing latitude. The map also shows the dwindling effect that elevation (cold and dry) has on liquid-solvent DAC performance, exemplified by the complex terrain of the US Mountain West and Great Basin. Interestingly, it also demonstrates that the West Coast has relatively high DAC potential within about 200 km of the ocean and in the far south of the Southwestern US. Overall, these geospatial trends are driven predominantly by the sensitivity of the liquid solvent DAC process to temperature and humidity. As further discussed in Section 3.2 of the Supplementary material, about 76% of the variability is driven by temperature and the remaining 24% is driven by relative humidity. Additionally, the results of the sensitivity analysis in Section 3.3 of the Supplementary material show that achieving a 10% change in CO2 capture rate efficiency requires a 62% change in relative humidity, but only an 11°C change in air temperature. These findings are consistent with our prior work that found the capture rate was more sensitive to temperature than relative humidity (An et al., 2022).
Figure 5 further demonstrates two additional advantages of southern latitudes compared to northern latitudes. The first advantage of southern latitudes compared to northern latitudes can also be seen by the broadening of the 1-sigma range in the mean (the grey band and area) when moving from south to north. That is, the interannual variability in CO2 sequestration is smaller in the south than the north. For example, across the average year of weather, the CO2 sequestration at Miami’s latitude would only fall by as little as 0.01 Mt. year over year, from its typical 1.05 to 1.04 MtCO2/yr1 (in “all grid” mode). Conversely at a higher latitude locations like Minneapolis sequestration can decrease by about 0.1 Mt., from 0.6 MtCO2/yr. to 0.5 MtCO2/yr. (in “all grid” mode) based solely on interannual weather variability.
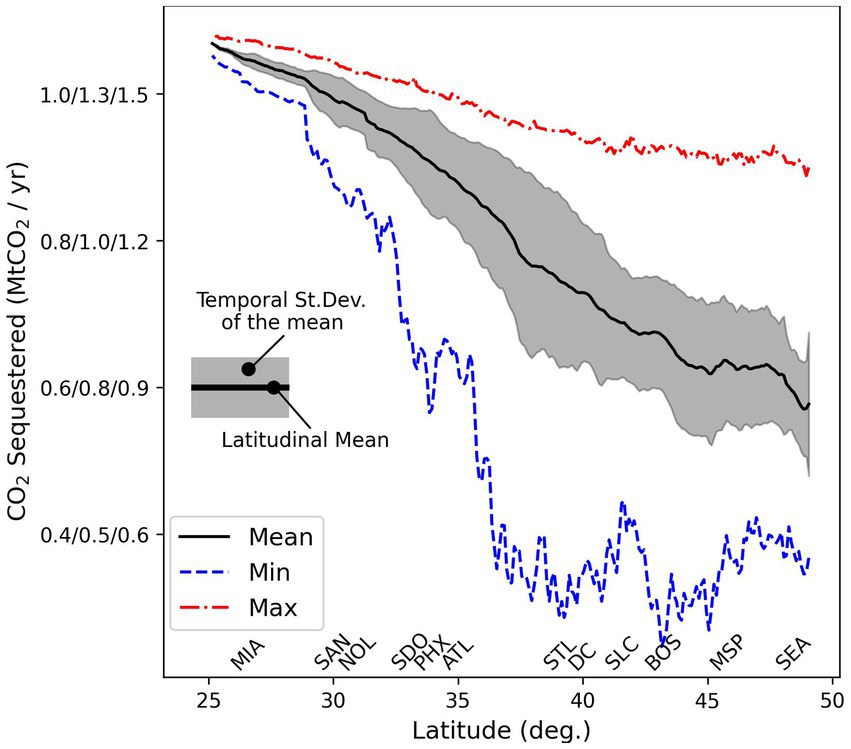
Figure 5. Variation in CO2 sequestration by latitude averaged across all 20 years of weather data. This figure shows the mean trend in CO2 sequestration across the contiguous United States and its temporal variability. The min and max values for each latitudinal band are also shown. Reference cities are listed on the horizontal axis: Miami (MIA), San Antonio (SAN), New Orleans (NOL), San Diego (SDO), Phoenix (PHX), Atlanta (ATL), Saint Louis (STL), Washington DC (DC), Salt Lake City (SLC), Boston (BOS), Minneapolis (MSP), and Seattle (SEA).
The second advantage of southern latitudes shown in Figure 5 is that the asymmetry of the minimum and maximum lines relative to the mean grows with increasing latitudes. The asymmetry is that the line of minimum CO2 sequestration deviates on average about 30% below the central lines, whereas the line of maximum sequestration deviates from the mean by only 20% on average. While the broadening of this asymmetrical range at latitudes below New Orleans are clearly driven by latitudinal differences in land surface area, north of there, the broadening asymmetry reveals a latitude-based bias with stronger minimum value outliers at higher latitudes. In addition to underscoring the favorability of lower latitudes for higher CO2 sequestration potential, it also highlights important considerations for regional DAC assessments.
Figure 6 illustrates the distribution of annual DAC sequestration data, encompassing a 20-year period from 2000 through 2019. This plot consists of 20 lines, each representing the distribution of all hourly DAC sequestration values throughout CONUS for that year.
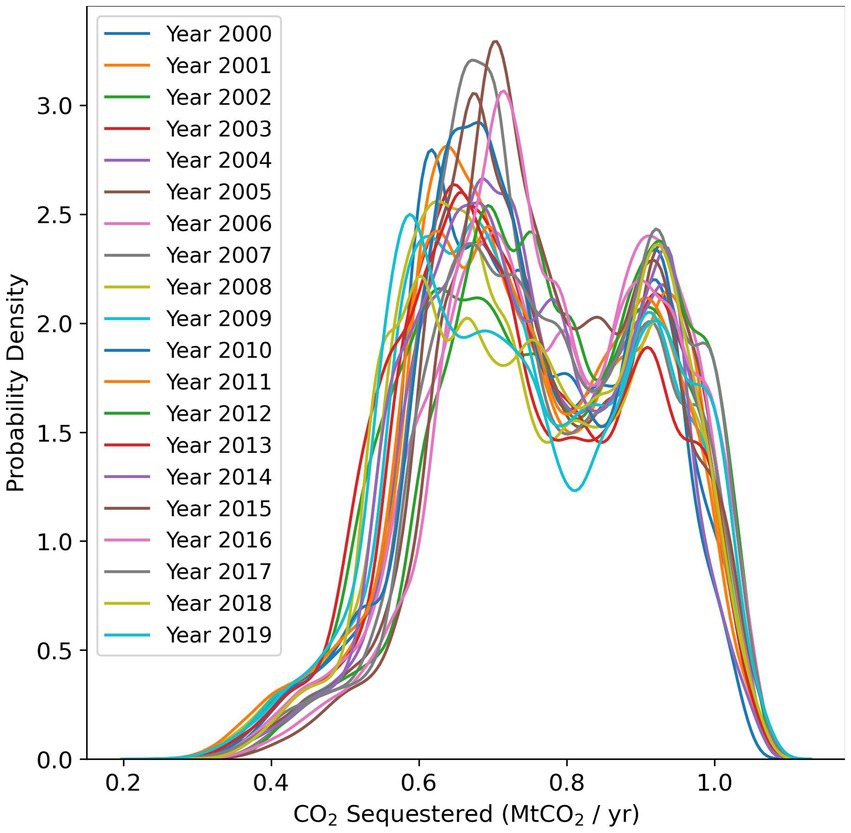
Figure 6. Probability density of annual CO2 sequestration for different weather years. For ease of presentation, the CO2 sequestration values shown on the x-axis assume “all grid” mode operation. As seen in the color bar of Figure 4 or the y-axis of Figure 5, the values shown on the x-axis would simply be proportionally larger if either of the other two modes were plotted instead.
As shown in Figure 6, the distribution of DAC sequestration across the contiguous United States follows a bimodal pattern in most years with peaks at 0.6 MtCO2/yr. and 0.9 MtCO2/yr. While the latitudinal data shown previously in Figure 5 suggests some difference among years, when viewed through Figure 6, those differences between years become more apparent. The shape of individual years is emphasized, and it is apparent that a notable amount of variability exists in potential sequestration from year to year. For example, no 2 years exhibit the same sequestration curve, which suggests the performance in 1 year will be different than in another, depending on the geospatial location.
Figure 6 suggests that while the performance of DAC will change each year, the consistency of the performance is higher in locations with the highest sequestration potential (i.e., > 0.8 MtCO2/yr). For example, the spread among years is more pronounced on the left compared to the right. In other words, Figure 6 demonstrates a fourth advantage of lower latitudes: there is less variability in performance across weather years.
Table 2 presents a view of the average change in DAC performance due to interannual fluctuations in weather conditions across space and time. Across weather years, the sequestration of the average location in the Contiguous US fluctuates between 0.72 MtCO2/yr. in the lowest performing years to 0.80 MtCO2/yr. in the highest performing years, resulting in an interannual variability range of approximately 11.5%. Additionally, the location exhibiting the lowest average sequestration rate (over 20 years) stands at 0.28 MtCO2/yr., and the highest is 1.07 MtCO2/yr., emphasizing the significant geographical disparities in DAC performance across geography.
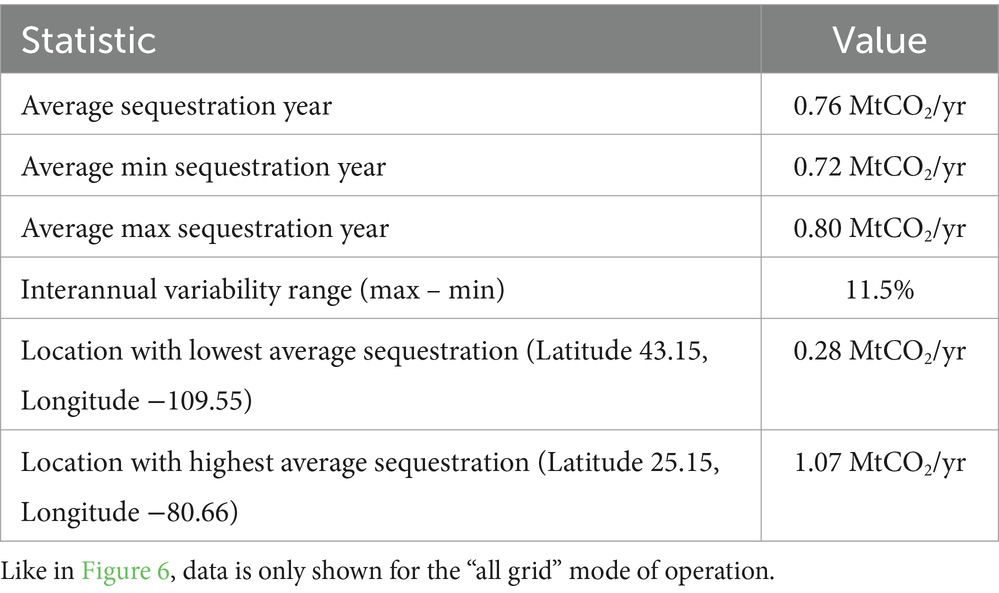
Table 2. Summary statistics indicating the range over which DAC performance varies in response to year-to-year fluctuations in weather.
3.3 Sensitivity of DAC performance to the temporal granularity of weather data
Figure 7 illustrates the potential for CO2 sequestration by DAC, based on average annual totals across all 20 years of weather data. Figure 7A is the same as Figure 4 except that it categorizes the removal potential into different classes, from high to low for improved visual comparison. The color bar of each subplot shows the sequestration potential across all three modes of operation.
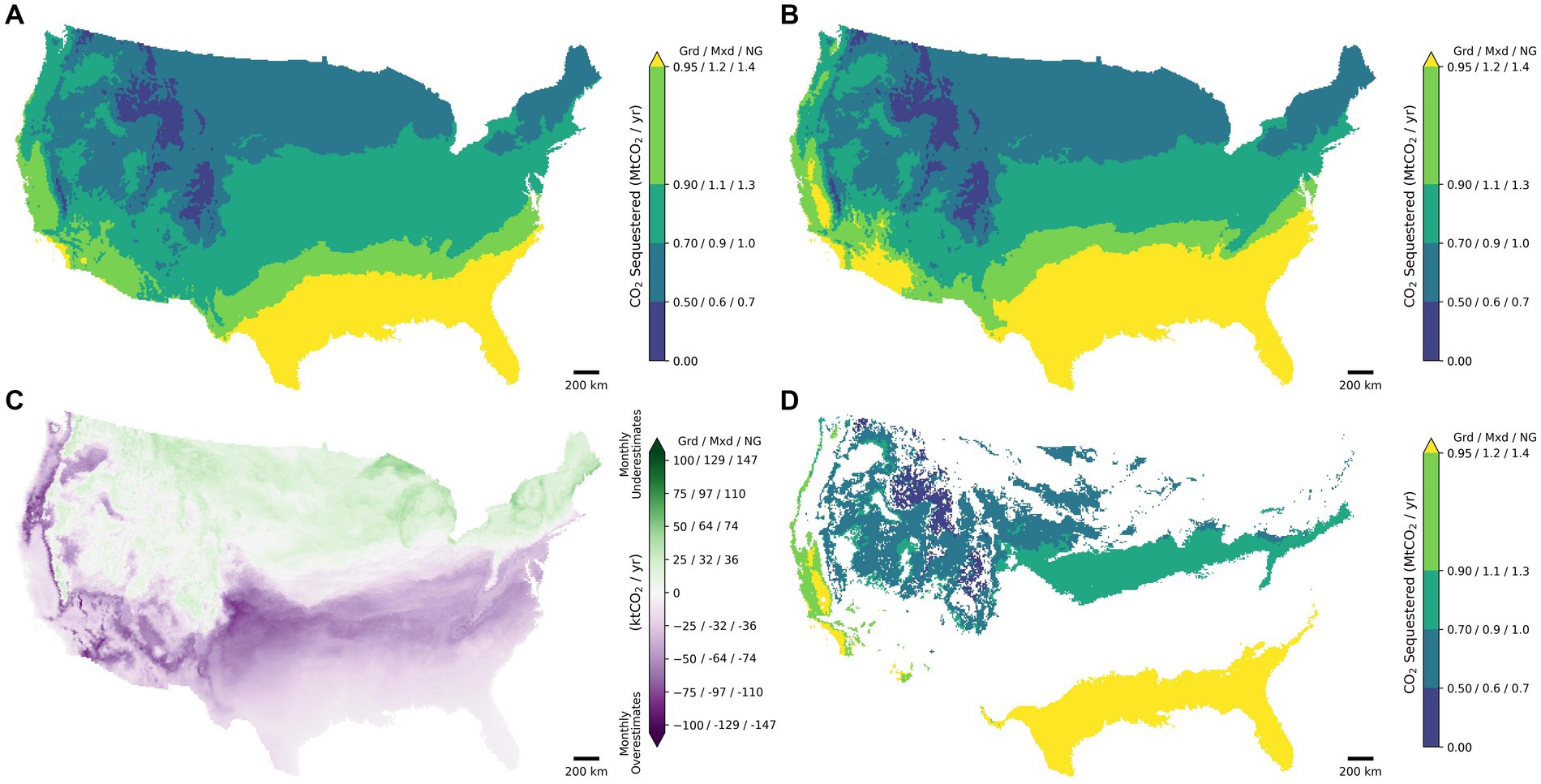
Figure 7. Differences in potential CO2 sequestration due to temporal granularity of weather data: (A) CO2 sequestration calculated with hourly weather data; (B) CO2 sequestration calculated with monthly mean weather data; (C) Residual (hourly – monthly) in CO2 sequestration, i.e., the difference between hourly and monthly mean weather input; and (D) Locations for which the hourly and monthly CO2 sequestration is less than 2% different.
Figure 7A illustrates that the highest sequestration potential is concentrated along the southern Gulf-Atlantic zone but that this high sequestration zone does not extend further west beyond Southeastern Texas. Even though high temperatures are common to the climate normal in the US Southwest, high humidity is not. In other words, while the temperature has a larger impact on the performance of DAC compared to humidity (as previously discussed and further presented in Section 3.2 of the Supplementary material), temperature alone is an inadequate predictor of CO2 sequestration potential.
Figure 7A also shows that much of the contiguous United States falls into the moderate sequestration potential category of 0.7 to 0.9 MtCO2/yr. (“all grid” mode values). Representing nearly all of the Western, Midwestern, and Northeastern US, this zone covers a wide range of landscapes, land-use types and climate conditions. Note however, as mentioned in Figures 5, 6, that the regularity in sequestration decreases with increasing latitude, which has implications for year-to-year predictability in sequestration totals in this zone. Despite some of the highest and most predictable temperatures, only restricted parts of the Southwestern US including parts of Southern California fall into the moderately high yield category (0.9–0.95 MtCO2/yr). This effect is due to low humidity conditions, which limit CO2 removal due to excessive evaporation that limit the flux of CO2 into solution at the air contactor.
Collectively, the subplots in Figure 7 show that CO2 sequestration tends to be slightly overestimated throughout locations in warm to mild climates if it is computed from monthly means, as exemplified by the broader high sequestration (yellow) zone in Figure 7B. This overestimation by monthly weather data is attributable to the data filtering process. Because liquid-solvent DAC cannot operate at sub-zero temperatures, those data points are excluded from both data series. No month in the Southern US averaged less than 0°C, therefore all monthly data are used. However, in that same region there are locations where the hourly temperature does fall below 0°C and are thus excluded from the total, which lowers the sequestration amount relative to the method computed from the monthly average, thus giving rise to the bias in DAC performance when estimated from monthly weather data.
The bias in CO2 sequestration when computed from monthly values is visualized in Figure 7C, which maps where monthly data tend to either over- or underestimate CO2 sequestration potential. Overall, sequestration computed from monthly weather data is more likely to be overestimated than underestimated. The mismatch (hourly – monthly) ranges from −12.2% (monthly overestimates) to +8.3% (monthly underestimates) and is on average − 1.1%. The mismatch is generally small but can lead to substantial over/underestimation of CO2 sequestration over time (e.g., if the DAC facility is operating for a decade or more). The spatial variability of mismatch also suggests that the choice of location will also dictate the temporal granularity of the weather data that is used to project sequestration potential.
Regionally, the most pronounced mismatch is in the mid latitudes, visualized in dark purple in Figure 7C. This is somewhat an artefact of the choice of month (as opposed to year) as a sampling unit for comparison. This high mismatch band occurs at these mid-latitudes because it is far enough north that some winter months begin to be filtered due to low temperatures, which eliminates entire months in the monthly data, whereas in the hourly data, only majority fractions of the month are filtered, and the remainder still adds to the total sequestration, thus giving rise to mismatch error.
Figure 7D is the same as Figure 7B except that it is limited to displaying locations where the disparity between monthly and hourly data is 2% or less. Thus, this map highlights areas where using monthly weather data with lower temporal resolution is a reasonably accurate approach. Interestingly, though not unexpected, the locations where monthly weather data are the best approximation of hourly are those in the high sequestration zone. This can be contributed to the overall consistency of warm weather in the high sequestration zone, as previously discussed.
Table 3 classifies the contiguous US by the temporal mismatch between hourly and monthly values and shows that DAC sequestration based on monthly values are accurate to within 2% error for 39% of the land area in the Contiguous US.
4 Discussion of results
Overall, our results demonstrate the critical importance of considering weather when siting DAC. For example, we find that the total range in temperature and humidity conditions throughout the Contiguous US can result in a ~ 3x difference in DAC performance (Table 2; Figure 4). As the purpose of DAC, and thus the underlying business model for DAC companies, is to provide CDR, our study demonstrates that the importance of weather cannot be understated. While the decisions focusing on where to locate DAC are driven by a variety of interrelated geospatial factors, our results suggest that future DAC site developers should consider weather early in the siting process. Or at a minimum, exclude areas from considering in which the weather is likely to be unfavorable.
Our results are also different compared to Pett-Ridge et al. (2023), who found western Texas and northern Alaksa to be more favorable for liquid-solvent DAC compared to the Gulf Coast. There are two primary reasons for this discrepancy. First, Pett-Ridge et al. (2023) considered the quantity of technically recoverable natural gas within their analysis, which resulted in concluding that regions with large natural gas reserves were most favorable to liquid solvent DAC (i.e., West Texas, northern Alaska). Second, Pett-Ridge et al. (2023) used daily averages of forecasted data for 2050, averaged across variety of datasets from different climate models. As a result of this averaging of modeled weather, the temporal variability in their data is more muted compared to the data used in this study. Further, the forecasted weather data used in Pett-Ridge et al. (2023) is also generally warmer than that used in this study, and thus generally more favorable to liquid-solvent DAC performance compared to this study.
In addition to demonstrating the importance of considering weather, our study also had multiple implications for energy decisions related to liquid-solvent DAC siting. First, we find that the preferred mode of operation is very sensitive to the upstream GHG intensities of electricity and natural gas. While our results demonstrate it is possible to make energy decisions based on these two factors alone, it is much harder to accurately quantify the upstream GHG intensity of natural gas compared to electricity. As such, DAC decision makers may benefit from performing rigorous uncertainty analyses if initial results suggest the “mixed” or “all natural gas” modes are preferred. Additionally, if either modes that burn natural gas on site are preferred, decision makers should also be aware that this design decision may require constructing upwards of ~30% to ~50% more CO2 compression, transportation, and storage infrastructure compared to the “all grid” mode. Even in the Gulf Coast, which is one of the most favorable locations for geologic CO2 storage in the country (Ogland-Hand et al., 2023), needing 30 to 50% more CO2 transportation and storage infrastructure will likely have substantial cost, feasibility, and timeline ramifications to deploying and operating the DAC facility.
There are caveats to our findings. For example, our findings are not intended to universally apply to regions with different weather patterns or geographic characteristics outside the contiguous United States. Additional caveats that were beyond the scope of this study but could be areas of focus for future work are listed below:
• We consider a generic 1 MtCO2/yr. liquid-solvent DAC facility and do not consider any feedback in its design to the weather conditions. Our process-level model was developed based on the information presented by Keith et al. (2018) and has not been validated against real-world performance (e.g., measured by Carbon Engineering) or other DAC system frameworks. As such, it is possible that the performance of DAC could be improved above what is shown here by optimizing the process-level design for the weather in a specific location or as future process-level design improvements are made to the liquid-solvent DAC system.
• We do not consider cost trade-offs in this analysis. For example, it is likely that “all grid” or “mixed mode” systems will have a higher cost than an “all natural gas” systems, which could also influence which energy mode is preferred.
• We do not consider the GHG emissions resulting from the construction of the DAC facility, CO2 transportation infrastructure, or CO2 storage infrastructure. These emissions could be considered in future work that further examines which energy mode is preferred (Madhu et al., 2021).
• We only consider temperature and relatively humidity, but additional weather parameters can also substantially influence the performance of DAC. For example, surface air pressure changes the partial pressure of CO2 and can consequently constrain CO2 capture efficiency. As the variability of air pressure, often associated with elevation changes, is substantial across the United States, this weather parameter in particular will be important to consider in future work. While the study relies on fine-resolution spatial and temporal weather data (the horizontal resolution of ERA5 Land hourly reanalysis data is ~9 km), not all processes and feedbacks in the physical weather system are completely represented by the underlying weather model (e.g., sub-grid-scale variability and local extreme events, vegetation dynamics). This limitation constrains the generalizability of our findings.
• Our study only used weather data from prior years, but weather patterns will change because of climate change. Changes in long-term weather trends might affect the extrapolation of these results into the future. For example, rising temperatures and precipitation would lead to better DAC performance. In contrast, rising temperatures but drier conditions, or colder temperatures but increased humidity may increase, or decrease, performance. As such, the impact of climate change on DAC performance is likely to vary regionally.
• Our prior work found that the water consumption of liquid-solvent DAC also changes as a function of air temperature and relative humidity (An et al., 2022), but we did not incorporate water consumption into this analysis. Based on our prior work and others [e.g., Pett-Ridge et al. (2023)], removing gigatonnes of CO2 with liquid-solvent DAC may require billions of tonnes of fresh water. Further, some locations that this analysis suggests are most favorable to liquid-solvent DAC deployment may also face water shortages in the future [e.g., Florida (NRDC, 2010)]. As such, it is possible that locations with favorable CO2 removal performance may be poor candidates for DAC deployment due to unfavorable water consumption.
5 Conclusion
In this study we created a process-level model of a liquid-solvent DAC system in IDAES and coupled it to a fine-resolution spatial and temporal dataset of temperature and humidity conditions to explore the impact of ambient weather on DAC performance. We found that:
1. The amount of CO2 sequestered can be far greater than the amount of CO2 removed from the atmosphere if natural gas is used on site to power DAC. For example, when weather conditions yield a 75% capture rate, 1.48 tCO2 are sequestered for every tonne of CO2 removed from the atmosphere in the “all natural gas” mode (Table 1).
2. The preferred energy mode of operation (i.e., “all grid,” “all natural gas,” “mixed”) for liquid-solvent DAC facilities is independent of air contactor capture rate and depends solely on the upstream GHG intensities of electricity and natural gas. As such, the decision of how to power liquid-solvent DAC could be made without considering weather (Figure 3).
3. Temperature and humidity conditions exert substantial influence over the performance of liquid-solvent DAC. Across the Contiguous US, the ambient weather conditions of a location can translate to a 3x difference in CO2 sequestration (Table 2; Figure 4). For example, in “all grid” mode the average DAC performance varies over the Contiguous US from 0.3 to 1.1 MtCO2/yr. While both temperature and relative humidity drive changes in DAC performance, temperature has about 3 times the influence compared to relative humidity (Supplementary Table S4).
4. The performance of liquid-solvent DAC can vary substantially across weather years, depending on location. In most years, the variability performance across weather years follows a bimodal pattern (Table 2; Figure 6).
5. Southern tier states in the US are preferrable for liquid-solvent DAC systems for multiple reasons. In southern latitudes: the average CO2 sequestration potential is higher on average across weather years (Figure 4); the minimum sequestration potential is higher on average (Figure 5); and there is less variability in total sequestration potential across weather years (Figure 6).
6. The estimated performance of liquid-solvent DAC calculated with monthly means is within 2% of the estimated performance calculated with hourly data for more than a third of the country (Table 3). Notably, the regions of the country that are most favorable to liquid-solvent DAC (i.e., the Southeastern US) can be approximated with monthly means because these locations have the most consistently warm temperature and relatively humidity conditions across time (Figure 7). But, depending on the desired accuracy, approximately one-tenth to one-half of the US experiences weather conditions too variable to be reliably represented by coarse time-sampled weather data.
Data availability statement
The original contributions presented in the study are included in the article/Supplementary material, further inquiries can be directed to the corresponding author.
Author contributions
B-GB: Data curation, Formal analysis, Investigation, Methodology, Software, Validation, Visualization, Writing – original draft. CG: Data curation, Formal analysis, Investigation, Methodology, Software, Validation, Writing – original draft. KA: Resources, Writing – review & editing. SM: Resources, Writing – review & editing. RM: Resources, Supervision, Writing – review & editing. JO-H: Conceptualization, Funding acquisition, Methodology, Project administration, Supervision, Visualization, Writing – original draft.
Funding
The author(s) declare that financial support was received for the research, authorship, and/or publication of this article. This material is based upon work supported by the U.S. Department of Energy, Small Business Innovations Research program under Award DE-SC0022486. The content is solely the responsibility of the authors and does not necessarily represent the official views of the Department of Energy. The work of SM was supported in part by funding from the Canada First Research Excellence Fund.
Acknowledgments
The authors would like to thank Jeffrey A. Bennett for assistance with technical vocabulary related to life cycle assessment.
Conflict of interest
B-GB, CG, RM, and JO-H were employed by Carbon Solutions.
The remaining authors declare that the research was conducted in the absence of any commercial or financial relationships that could be construed as a potential conflict of interest.
Publisher’s note
All claims expressed in this article are solely those of the authors and do not necessarily represent those of their affiliated organizations, or those of the publisher, the editors and the reviewers. Any product that may be evaluated in this article, or claim that may be made by its manufacturer, is not guaranteed or endorsed by the publisher.
Supplementary material
The Supplementary material for this article can be found online at: https://www.frontiersin.org/articles/10.3389/fclim.2024.1394728/full#supplementary-material
References
An, K., Farooqui, A., and McCoy, S. T. (2022). The impact of climate on solvent-based direct air capture systems. Appl. Energy 325:119895. doi: 10.1016/j.apenergy.2022.119895
ANL (2023) Greenhouse gases, regulated emissions, and energy use in technologies model. Available at: https://greet.anl.gov/
Bistline, J. E. T., and Blanford, G. J. (2021). Impact of carbon dioxide removal technologies on deep Decarbonization of the electric power sector. Nat. Commun. 12:3732. doi: 10.1038/s41467-021-23554-6
Breyer, C., Fasihi, M., and Aghahosseini, A. (2020). Carbon dioxide direct air capture for effective climate change mitigation based on renewable electricity: a new type of energy system sector coupling. Mitig. Adapt. Strateg. Glob. Chang. 25, 43–65. doi: 10.1007/s11027-019-9847-y
Chen, Y., Sherwin, E. D., Berman, E. S. F., Jones, B. B., Gordon, M. P., Wetherley, E. B., et al. (2022). Quantifying regional methane emissions in the New Mexico Permian Basin with a comprehensive aerial survey. Environ. Sci. Technol. 56, 4317–4323. doi: 10.1021/acs.est.1c06458
Davis, S. J., Lewis, N. S., Shaner, M., Aggarwal, S., Arent, D., Azevedo, I. L., et al. (2018). Net-zero emissions energy systems. Science 360:eaas9793. doi: 10.1126/science.aas9793
EPRI and GTI Energy (2022) Net-Zero 2050: U.S. Economy-Wide Deep Decarbonization Scenario Analysis. Available at: https://lcri-netzero.epri.com/
Friedlingstein, P., O'Sullivan, M., Jones, M. W., Andrew, R. M., Gregor, L., Hauck, J., et al. (2022). Global carbon budget 2022. Earth Syst. Sci. Data 14, 4811–4900. doi: 10.5194/essd-14-4811-2022
Fuhrman, J., Bergero, C., Weber, M., Monteith, S., Wang, F. M., Clarens, A. F., et al. (2023). Diverse carbon dioxide removal approaches could reduce impacts on the energy-water-land system. Nat. Clim. Chang. 13, 341–350. doi: 10.1038/s41558-023-01604-9
Gorelick, N., Hancher, M., Dixon, M., Ilyushchenko, S., Thau, D., and Moore, R. (2017). Google earth engine: planetary-scale geospatial analysis for everyone. Remote Sens. Environ. 202, 18–27. doi: 10.1016/j.rse.2017.06.031
Hanna, R., Abdulla, A., Xu, Y., and Victor, D. G. (2021). Emergency deployment of direct air capture as a response to the climate crisis. Nat. Commun. 12, 1–13. doi: 10.1038/s41467-020-20437-0
IEA (2023) CO2 emissions in 2022. Available at: https://www.iea.org/reports/co2-emissions-in-2022
IPCC (2022) Climate change 2022- mitigation of climate change-summary for policymakers (SPM). Available at: https://www.ipcc.ch/report/ar6/wg3/downloads/report/IPCC_AR6_WGIII_SPM.pdf
Keith, D. W., Holmes, G., St. Angelo, D., and Heidel, K. (2018). A process for capturing CO2 from the atmosphere. Joule 2, 1573–1594. doi: 10.1016/j.joule.2018.05.006
Larson, E., Greig, C., Jenkins, J., Mayfield, E., Pascale, A., Zhang, C., et al. (2020) Net-zero America: Potential pathways, infrastructure, and impacts. Available at: https://netzeroamerica.princeton.edu/the-report
Liu, C. M., Sandhu, N. K., McCoy, S. T., and Bergerson, J. A. (2020). A life cycle assessment of greenhouse gas emissions from direct air capture and Fischer-Tropsch fuel production. Sustain. Energy Fuels 4, 3129–3142. doi: 10.1039/c9se00479c
Madhu, K., Pauliuk, S., Dhathri, S., and Creutzig, F. (2021). “Understanding Environmental Trade-offs and Resource Demand of Direct Air Capture Technologies Through Comparative Life-cycle Assessment,” Nature Energy. doi: 10.1038/s41560-021-00922-6
McQueen, N., Desmond, M. J., Socolow, R. H., Psarras, P., and Wilcox, J. (2021). Natural gas vs. Electricity for Solvent-Based Direct air Capture. Front. Clim. 2:618644. doi: 10.3389/fclim.2020.618644
Munoz Sabater, J. (2019). “ERA5-land monthly averaged data from 1981 to present” in Copernicus climate change service (C3S) climate data store (CDS). Available at: https://doi.org/10.24381/cds.68d2bb30
National Academies of Sciences, Engineering, and Medicine. (2019) Negative Emissions Technologies and Reliable Sequestration: A Research Agenda. Washington, DC: The National Academies Press.
NRDC (2010). Climate change, water, and risk: Current water demands are not sustainable : Water Facts Available at: https://www.nrdc.org/sites/default/files/WaterRisk.pdf.
Ogland-Hand, J., Cox, K. J., Adams, B. M., Bennett, J. A., Johnson, P. J., Middleton, E. J., et al. (2023). How to net-zero America: Nationwide cost and capacity estimates for geologic CO2 storage. Eng. Arch. doi: 10.31224/3293
Pett-Ridge, J., Kuebbing, S., Mayer, A. C., Hovorka, S., Pilorgé, H., Baker, S. E., et al. (2023) Roads to removal: options for carbon dioxide removal in the United States. Available at: https://roads2removal.org/resources/
Realmonte, G., Drouet, L., Gambhir, A., Glynn, J., Hawkes, A., Köberle, A. C., et al. (2019). An inter-model assessment of the role of direct air capture in deep mitigation pathways. Nat. Commun. 10, 3277–3212. doi: 10.1038/s41467-019-10842-5
Sendi, M., Bui, M., Mac Dowell, N., and Fennell, P. (2022). Geospatial analysis of regional climate impacts to accelerate cost-efficient direct air capture deployment. One Earth 5, 1153–1164. doi: 10.1016/j.oneear.2022.09.003
Terlouw, T., Treyer, K., Bauer, C., and Mazzotti, M. (2021). Life cycle assessment of direct air carbon capture and storage with low-carbon energy sources. Environ. Sci. Technol. 55, 11397–11411. doi: 10.1021/acs.est.1c03263
Keywords: direct air capture, climate, carbon footprint, IDAES, GIS
Citation: Brooks B-G, Geissler CH, An K, McCoy ST, Middleton RS and Ogland-Hand JD (2024) The performance of solvent-based direct air capture across geospatial and temporal climate regimes. Front. Clim. 6:1394728. doi: 10.3389/fclim.2024.1394728
Edited by:
Mijndert Van Der Spek, Heriot-Watt University, United KingdomReviewed by:
Till Strunge, Technical University of Berlin, GermanyPaola Alejandra Saenz Cavazos, Imperial College London, United Kingdom
Copyright © 2024 Brooks, Geissler, An, McCoy, Middleton and Ogland-Hand. This is an open-access article distributed under the terms of the Creative Commons Attribution License (CC BY). The use, distribution or reproduction in other forums is permitted, provided the original author(s) and the copyright owner(s) are credited and that the original publication in this journal is cited, in accordance with accepted academic practice. No use, distribution or reproduction is permitted which does not comply with these terms.
*Correspondence: Jonathan D. Ogland-Hand, am9uYXRoYW4ub2dsYW5kLWhhbmRAY2FyYm9uc29sdXRpb25zbGxjLmNvbQ==