- 1IGAD Climate Prediction and Application Center, Nairobi, Kenya
- 2Kenya Meteorological Department, Nairobi, Kenya
- 3Norwegian Computing Center, Oslo, Norway
- 4Department of Geography, University of Sussex, Brighton, United Kingdom
- 5Met Office, Exeter, United Kingdom
- 6School of Earth and Environment, University of Leeds, Leeds, United Kingdom
- 7Norwegian Research Centre, Bergen, Norway
- 8Department of Earth and Climate Sciences, University of Nairobi, Nairobi, Kenya
- 9Tanzania Meteorological Authority, Dar es Salaam, Tanzania
- 10Uganda National Meteorological Authority, Kampala, Uganda
- 11Rwanda Meteorological Agency, Kigali, Rwanda
- 12Agence Nationale de la Météorologie de Djibouti, Djibouti, Djibouti
- 13Ethiopia Meteorological Institute, Addis Ababa, Ethiopia
- 14Institut Géographique du Burundi, Bujumbura, Burundi
- 15Ministry of Energy and Water Resources, Mogadishu, Somalia
- 16National Oceanic and Atmospheric Administration, CPC, Washington, DC, United States
The Greater Horn of Africa is prone to extreme climatic conditions, thus, making climate services increasingly important in supporting decision-making processes across a range of climate sensitive sectors. This study aims to provide a comprehensive review of the recent advances, gaps and challenges in the provision of climate services over the region, for each of the components of the Global Framework for Climate Services. The study explores various milestones that have been achieved toward climate service delivery. The achievements include improvement of station network coverage, and enhancing the capacity of member states to utilize various tools in data analysis and generate routine climate products. The advancement in science, and availability of High-Performance Computing has made it possible for forecast information to be provided from nowcasting to seasonal timescales. Moreover, operationalizing of the objective forecasting method for monthly and seasonal forecasts has made it possible to translate tercile forecasts for applications models. Additionally, innovative approaches to user engagement through co-production, communication channels, user-friendly interfaces, and dissemination of climate information have also been developed. Despite the significant progress that has been made in the provision of climate services, there are still many challenges and gaps that need to be overcome in order to ensure that these services are effectively meeting the needs of users. The research of the science underpinning climate variability, capacity building and stakeholder engagement, as well as improved data management and quality control processes are some of the gaps that exist over the region. Additionally, communication and dissemination of climate information, including timely warnings and risk communication, require improvement to reach diverse user groups effectively. Addressing these challenges will require strengthened partnerships, increased investment in capacity building, enhanced collaboration between the climate information producers and stakeholders, and the development of user-friendly climate products. Bridging these gaps will foster greater resilience to climate-related hazards and disasters in the Greater Horn of Africa and support sustainable development in the region.
1 Introduction
The economies and livelihoods of the Greater Horn of Africa (GHA) region are heavily reliant on climate-sensitive sectors such as agriculture, tourism, forestry, and water resources, making it one of the most vulnerable regions to the effects of climate change and variability (Kinyangi et al., 2009; Kogo et al., 2021; Bedasa and Bedemo, 2022). To effectively make decisions in these sectors and to increase the region's resilience to climate-related hazards, it is essential to provide timely, reliable, and actionable climate information. Most parts of the region are arid and semiarid making the populations vulnerable to changes in rainfall. For example, in the arid and semiarid regions, depressed rainfall usually leads to shortage of pasture, and limited water availability (Kebede and Taye, 2021; Mdemu, 2021; Lelenguyah et al., 2023). The three major rainy seasons in the area are the Long rains (from March to May, MAM, Figure 1A), the Short rains (from October to December, OND, Figure 1C), over the equatorial parts of the region and northern Tanzania, and the rains from June to September (JJAS, Figure 1B) over the northern parts of the region. The rainfall patterns in the region are influenced by several factors, including the north-south migration of the Inter-Tropical Convergence Zone, the Indian Ocean Dipole, and the El Nino Southern Oscillation (ENSO).
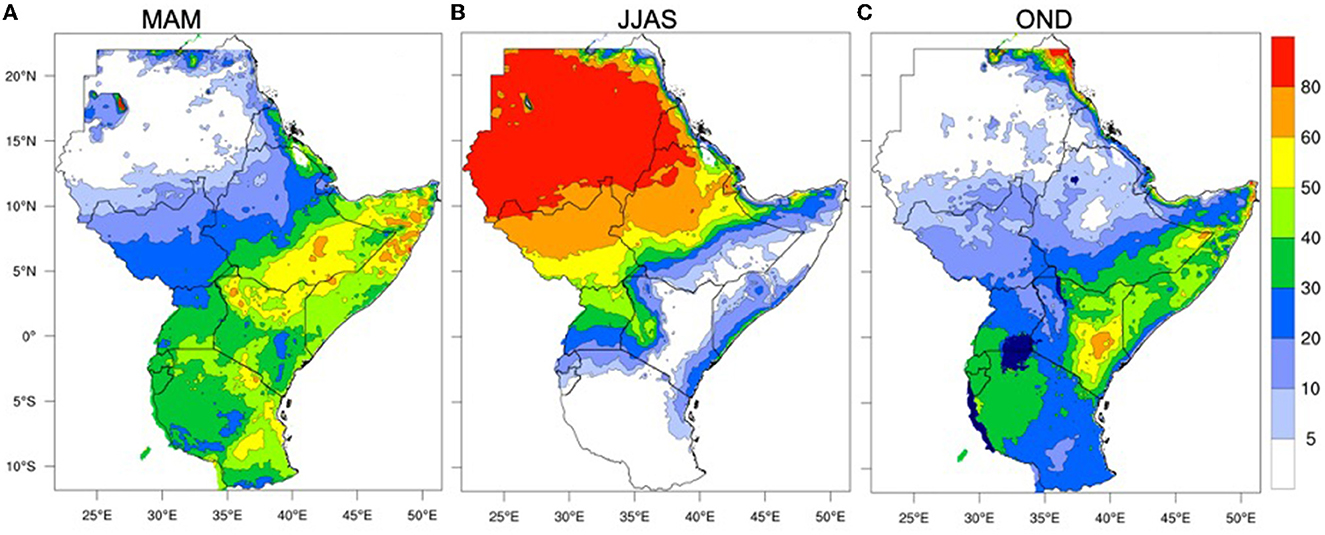
Figure 1. Seasonal percentage contribution to total annual rainfall over the Greater Horn of Africa for the (A) March–May (B) June–September and (C) October–December seasons.
The GHA region continues to face shocks of weather and climate extremes. Over the past decades, the region has experienced numerous flooding and drought events (Bizimana and Schilling, 2010; Funk, 2012; Mwangi et al., 2014; Nicholson, 2014; Kilavi et al., 2018; Kikwasi and Mbuya, 2019; MacLeod et al., 2021a; Wainwright et al., 2021a,b; Kiptum et al., 2023). In recent years, several severe droughts have occurred over the region including the 2010/2011 drought over the Horn of Africa which affected more than 12 million people and resulted in significant loss of lives (Dutra et al., 2013; Funk et al., 2014; Mwangi et al., 2014). The 2016/2017 drought also left several millions of people facing food and water shortages (Funk et al., 2018). The 2020-2022 drought left approximately 48 million people in the region facing food and water shortages and over 11 million livestock dead (ICPAC, 2023). In addition to the drought conditions, the region has experienced numerous flooding events in the past decade including the MAM 2018, OND 2019, MAM 2020, and OND 2023 events (Kilavi et al., 2018; OCHA, 2018; Reliefweb, 2019, 2023; Chang'a et al., 2020; Wainwright et al., 2021a,b). The flooding events during OND 2019 also led to conducive conditions for the breeding of the desert locusts which subsequently led to the outbreak of desert locusts infestation that destroyed large rangeland areas (Salih et al., 2020), and exacerbated the forage shortage during the 2020 to 2022 drought event (Reliefweb, 2022; FarmAfrica, 2023).
The Early Warnings For All Initiative (EW4All) that aims at ensuring that lives and livelihoods are protected from extreme climate hazards by 2027, was formally initiated by the UN Secretary-General in November 2022 at the COP27 meeting in Sharm El-Sheikh, Egypt [(World Meteorological Organisation (WMO), 2022a)]. To achieve this and increase the region's resilience to these extreme events necessitates a rigorous stock-take on the climate services offered over the GHA. Climate services refer to the production, translation, transfer, and use of climate information and knowledge to support decision-making processes across different sectors and levels of society (Vaughan and Dessai, 2014). They are designed to help individuals, businesses, and governments make informed decisions in the face of changing weather and climate patterns. Climate services are essential for enabling communities and countries to manage risks, increase resilience, and capitalize on opportunities in a changing climate (World Meteorological Organisation, 2011). Managing climate risk is ensured by having access to the best decision-relevant climate information and having the skills to use it (Dinku, 2019).
To ensure efficient implementation of climate services, the World Meteorological Organization established the Global Framework for Climate Services (GFCS). The GFCS consists of five components which are (i) user interface platforms, (ii) Climate Services Information System, (iii) Observations and Monitoring, (iv) Research, Modeling and Prediction, and (v) Capacity Development (Hewitt et al., 2012). This study aims to provide a comprehensive review of the recent advances, gaps and challenges in the provision of climate services over the Greater Horn of Africa in each of the components of the GFCS. The study will highlight key developments in data collection and analysis, climate modeling, as well as the use of innovative tools and approaches to enhance the accessibility and usability of climate information. Additionally, the study will discuss the challenges and opportunities facing the region in the provision of climate services and provide recommendations for future research and action.
2 Advances in implementation of the components for Global Framework for Climate Services
2.1 User interface platform
Various user interface platforms (UIPs) exist over the region and these include, The Greater Horn of Africa Climate Outlook Forum (GHACOF, Ogallo et al., 2008), National Outlook Forums (Kenya Metorological Department, 2022; Teshome and Demissie, 2022; Anticipation Hub, 2023) and sub-national climate Outlook forums also known as Participatory Scenario Planning workshops [conducted in most counties in Kenya, and some regions in Somalia, Uganda, and Ethiopia, Ambani et al., 2021; World Meteorological Organisation (WMO), 2022b]. These platforms provide a leading role in creating opportunity for dialogue between countries, sectors, academia, users and producers. In addition, a sector specific UIP has been established that focuses on Agriculture and Food security; the Food Security and Nutrition Working Group (Gudoshava et al., 2022a). Furthermore, digital platforms such as the East Africa Hazards Watch allow for users to obtain area specific climate information. Further information on the East Africa Hazards watch will be discussed in Section 2.2.3.
2.2 Climate services information system
This section summarizes the advances that have been made in provision of the climate services through forecast provision, coproduction of climate services and forecast dissemination. It outlines the different products that are being provided, tools that have aided in the provision of the products, various coproduction methods and the different media channels for information dissemination.
2.2.1 Forecast generation
In forecast generation the study focuses on the advances from nowcasting timescales to seasonal timescales that have occurred at both national and regional level.
2.2.1.1 Nowcasting
Nowcasting is defined as “forecasting with local detail, by any method, over a period from the present to 6 h ahead, including a detailed description of the present weather” (World Meteorological Organisation, 2017). The availability of nowcasting forecast products has enhanced the accuracy, and timely generation of weather warnings to save lives and property (Roberts et al., 2021a,b; Youds et al., 2021). Notably, fishers, boat operators, and communities on Lake Victoria are able to access customized forecasts and warnings that advise their decisions to venture into the lake thereby significantly reducing fatalities on the lake (Roberts et al., 2021b).
Countries within the GHA are making strides toward operationalizing nowcasting beyond aviation forecasts. For instance, Uganda1 and Rwanda2 issue 6-h forecasts of precipitation, winds and temperature for various regions. In addition, Rwanda provides tracks of storms reaching to 1 h and wind shear based on the Doppler weather radar products using Thunderstorm Initiation, Tracking, and Analysis (TITAN) software to support disaster risk reduction in addition to aviation operations.
Furthermore, the FASTA app3 provides forecasted storm rainfall information for the next 2 h over East Africa (Figure 2a). FASTA utilizes Convective Rainfall Rate (CRR) and Rapidly Developing Thunderstorms (RDT) products from EUMETSAT's NoWCasting Satellite Application Facility (NWC SAF). The app builds on research carried out assessing prediction skill of nowcasting products over Eastern Africa and is freely available to Kenyan users (FASTA, 2023). A Nowcasting Catalog4 is also available through which meteorologists can access products (Figure 2b). Hill et al. (2020) demonstrated that the nowcasting products have skill at lead times of at least 1.5 h.
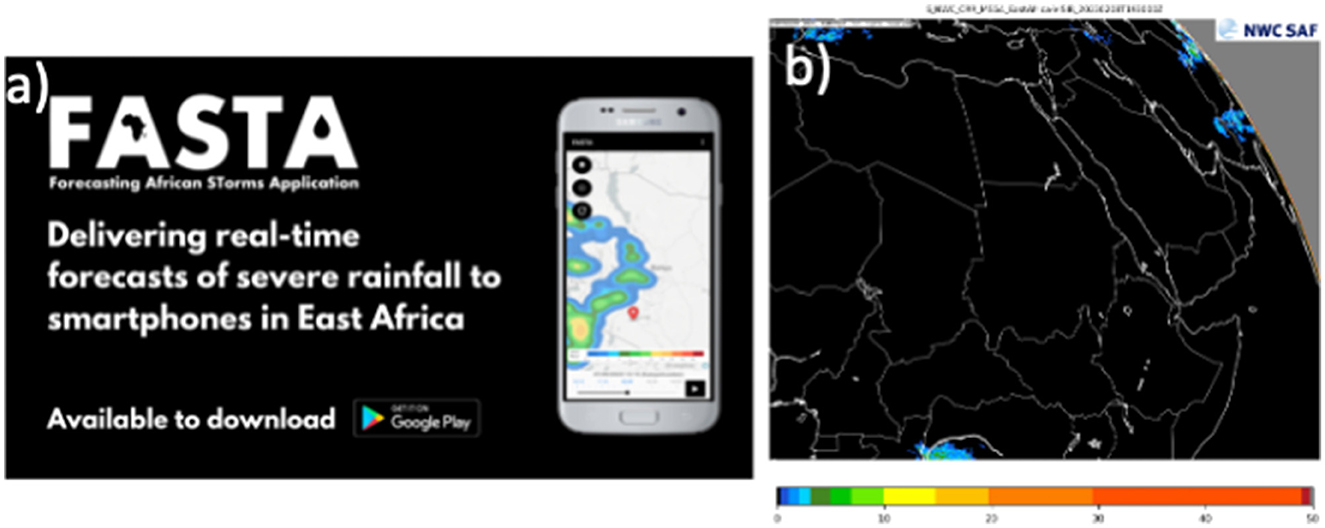
Figure 2. (a) FASTA app that is utilized by stakeholders to obtain real time weather information (b) NWCSAF products extracted from the Global Challenges Research Fund African SWIFT website (https://science.ncas.ac.uk/swift/resources/view/13470190).
2.2.1.2 Short range forecasts
Short range forecasts refer to weather predictions that are beyond 12 h and up to 72 h (Doblas-Reyes et al., 2003). National Meteorological and Hydrological Services (NMHSs) within the GHA region routinely issue short range weather forecasts using a wide array of tools (Lungo et al., 2020; MacLeod et al., 2021b). These include observed data from manned and automatic stations, satellite data, radiosonde data, radar data that are used in operational forecasting to validate the numerical weather prediction products from Meteo-France model of Action de Recherche Petite Echelle Grande Echelle (ARPEGE), the European Center for Medium Range Weather Forecasts (ECMWF), National Oceanic and Atmospheric Administration (NOAA) Climate Prediction Center, Meteoblue, United Kingdom Met Office (UKMO), South Africa Weather Services (SAWS), Regional Specialized Meteorological Center La Réunion-Tropical Cyclone Center among others (e.g., Lungo et al., 2020; Cafaro et al., 2021; MacLeod et al., 2021b).
Access to high resolution outputs from for example the UKMO Tropical Africa model has enabled targeted warnings to the public for example the Kenya Meteorological Department issues out warning information of heavy rainfall over informal settlements in Nairobi.5 In addition to heavy rainfall warnings NHMSs also issue advisories and warnings for strong winds, and large waves (MacLeod et al., 2021b).
During times when there is a threat of landfall from a tropical cyclone over the region, the regional climate center over the GHA (IGAD Climate Prediction and Applications Center [ICPAC]) provides the cyclone tracks on daily basis and issues warnings if there is imminent disaster (Mwanthi et al., 2019). Figure 3 is an example of the forecast information that is issued for early warning.
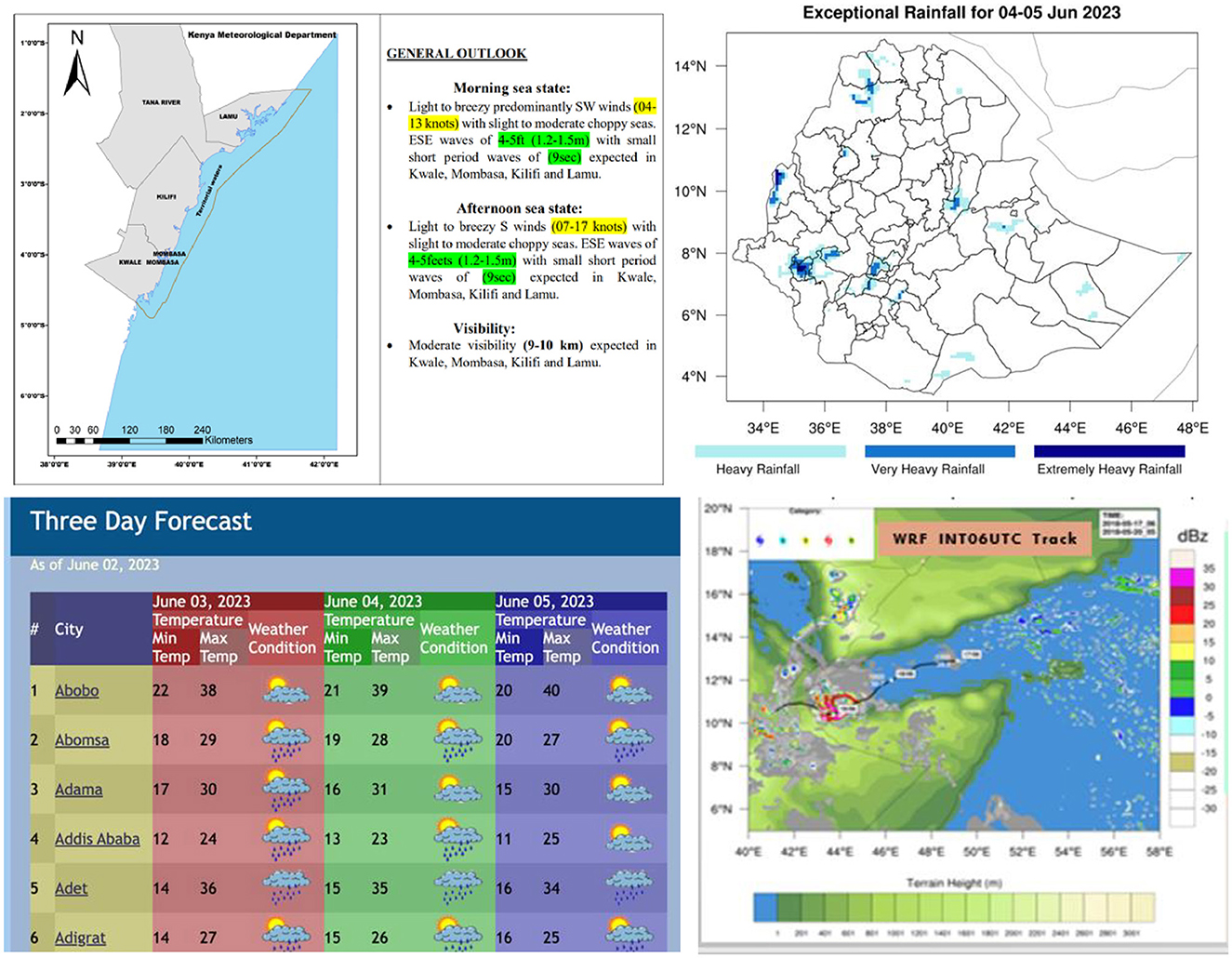
Figure 3. Example of Short-range forecast products that are currently disseminated by the National Meteorological and Hydrological services and the cyclone tracks that are produced by ICPAC.
2.2.1.3 Medium range forecasts
Medium range forecasts extend from about 3 to 10 days in advance (Doblas-Reyes et al., 2003). ICPAC and the majority of the NMHSs in the GHA region downscale the forecast information dynamically to local scale by utilizing the Weather and Research Forecasting model (WRF, Skamarock et al., 2008; Case et al., 2014; Opijah et al., 2014; Lungo et al., 2020) and routinely issue out these forecasts. The capability to generate medium-range forecasts with optimal physics combinations customized for the region and at high resolution has been facilitated by the availability of High-Performance Computing systems at both regional and national levels (AFDB, 2020; WISER, 2021).
At the regional level, 10 km resolution simulations are produced at weekly timescales, while at the national level (e.g., Kenya, Rwanda, Ethiopia) the convective permitting resolution of 4 km is utilized. In tropical Africa the main contribution to daily rainfall comes from deep convective systems (Fink et al., 2017), thus, the use of the convective permitting resolution has the potential to improve the rainfall forecast (Clark et al., 2016). In addition to the weekly forecasts, 5- and 10-day forecasts are also issued by the NMHS over the region. The results of these simulations include unusually heavy rain, total amount of rain, anomalies, and mean temperature variations. Further, NHMSs produce agrometeorological, hydrometeorological, biometeorological and other specialized forecasts and bulletins that are tailored to users' needs. Example weekly products are shown in Figure 4 at the regional level.
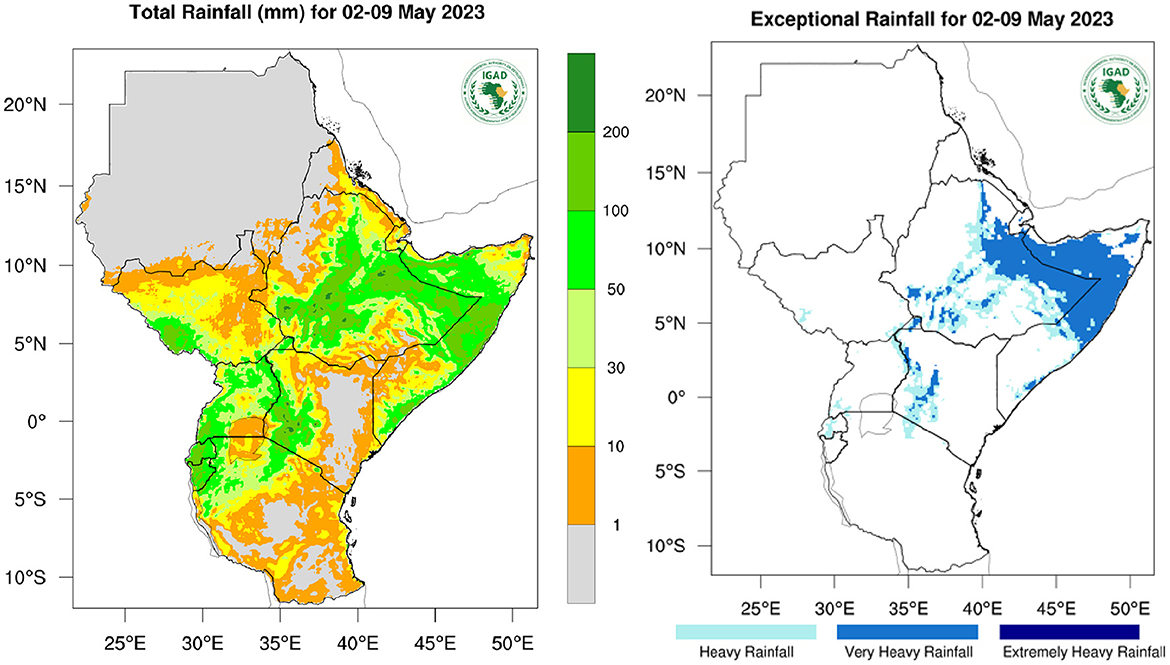
Figure 4. Example of the weekly WRF outputs that are produced at regional level. The example shows the total rainfall and exceptional rainfall during a particular week. Exceptional rainfall is calculated by comparing the forecast rainfall and the WRF hindcast rainfall for that particular week.
2.2.1.4 Sub-seasonal forecasts
Sub-seasonal forecasts, from 2 weeks to a season, bridge the gap between weather and seasonal forecasting (Vitart et al., 2012, 2017; Robertson et al., 2015). The sub-seasonal forecast exhibit skill over the GHA region (de Andrade et al., 2021; Endris et al., 2021; MacLeod et al., 2021a) hence their potential to be used for decision making in various sectors (White et al., 2017, 2021). In the agriculture and food security sector it was reported that the sub-seasonal forecast enhances the tactical decision making such as application of pesticides, weeding and irrigation time periods (Gudoshava et al., 2022a). Furthermore, sub-seasonal forecast products are also utilized by Kenya Meteorological Department (KMD) to improve their seasonal rainfall onset prediction (MacLeod et al., 2021b).
ICPAC and the NMHS over the region routinely provide monthly forecasts utilizing datasets derived from long-range seasonal forecasts. However, from 2019 to 2022 ICPAC and KMD were granted access to the real-time outputs of operational sub-seasonal prediction systems as part of the real-time pilot phase of S2S project (Hirons et al., 2021, 2023; Gudoshava et al., 2022a). During the real-time initiative period, forecasts were issued on weekly or biweekly timescales and provided information with a temporal resolution of the order of a week, describing average temperatures, rainfall, soil moisture, wind speed and direction, and probabilities of exceeding predefined rainfall thresholds. In this period, collaboration among operational centers, research institutions, and stakeholders resulted in the co-development of a variety of products tailored to specific user groups, including hydrology, energy, disaster risk reduction, and food security (Hirons et al., 2021; Gudoshava et al., 2022a; Visman et al., 2022). Figure 5 shows the example products that were co-produced during the S2S real time initiative.
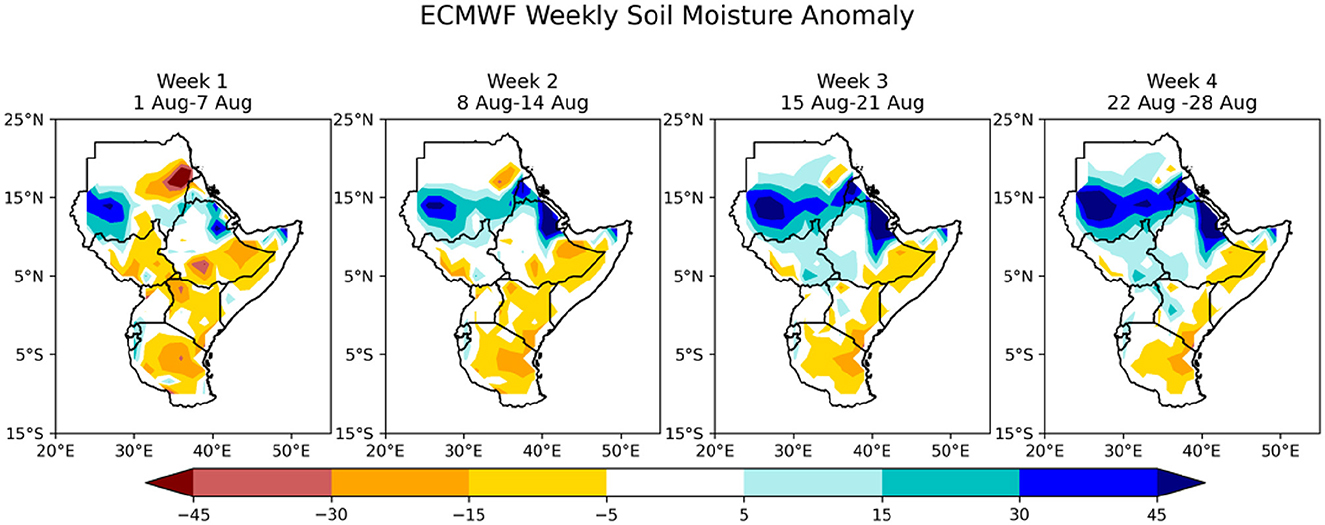
Figure 5. Sub-seasonal forecasts example of soil moisture that was issued out at biweekly timescales to the Food Security and Nutrition Working Group. The soil moisture datasets were obtained from ECMWF S2S forecasting system. The hindcast period in the ECMWF system is the past 20 years for example a forecast for 2022 will utilize a hindcast period of 2001 to 2021.
2.2.1.5 Seasonal forecasts
Seasonal forecasting is defined as predictions of average conditions for a particular period (e.g., 3-month period, 4 month period). The forecasts are updated monthly and largely rely on slowly changing parts of the climate system. In 2017, the WMO recommended that its designated Regional Climate Centers adopt objective seasonal forecasting methods that are based primarily on model outputs and are reproducible, traceable, and verifiable [(World Meteorological Organisation (WMO), 2020)], unlike the widely used semi-subjective methods. Prior to June to September 2019 season, ICPAC had progressively adopted a wide range of methodologies to produce objective seasonal rainfall and temperature forecast. While these methods are objective, blending of various outputs into a single consensus forecast was achieved using a semi-subjective process. The resulting forecast (and its accuracy) thus relied on the opinions and experience of the experts available during the consensus, thus making these forecasts difficult to reproduce. These subjective methods have since been replaced by an objective method based on the statistical downscaling of outputs of dynamical prediction models.
The forecast is constructed from the outputs of up to nine seasonal prediction systems each processed with three statistical calibration methods; the Canonical Correlation Analysis (CCA), logistic and linear regression. Table 1 is a summary of the forecast models that are currently utilized for objective forecasting over the region by ICPAC.
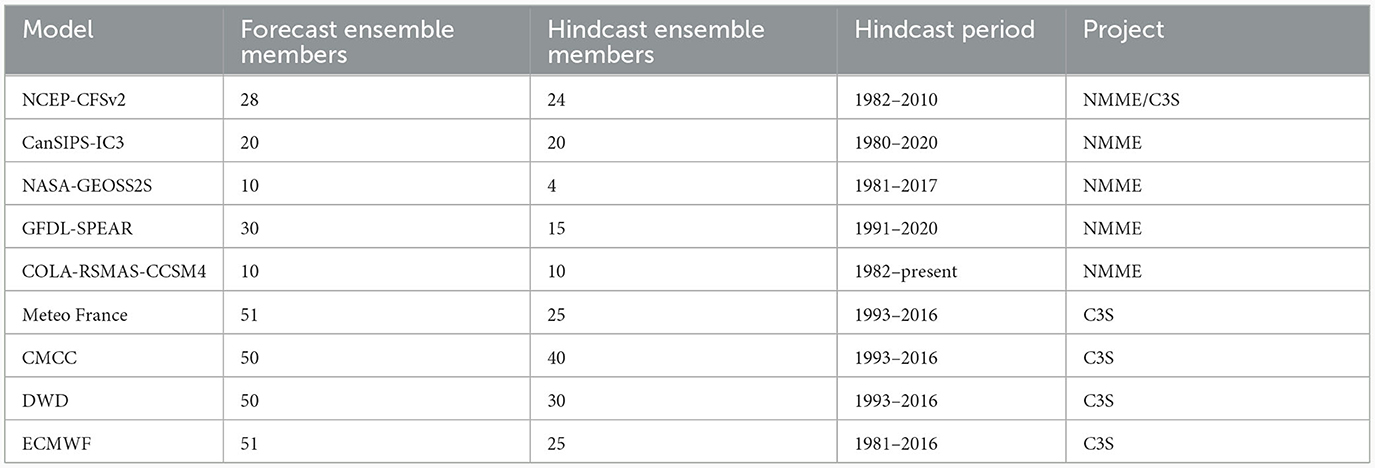
Table 1. Summary of the operational Global Climate models currently utilized at ICPAC and the project each model is participating in.
The outputs from the three different techniques and the nine models are then averaged to obtain the consolidated objective forecast. An example of the seasonal forecast produced by the technique is shown in Figure 6. In comparison with the consensus forecast, the objective forecast shows a full range of the possible probabilities in each tercile category.
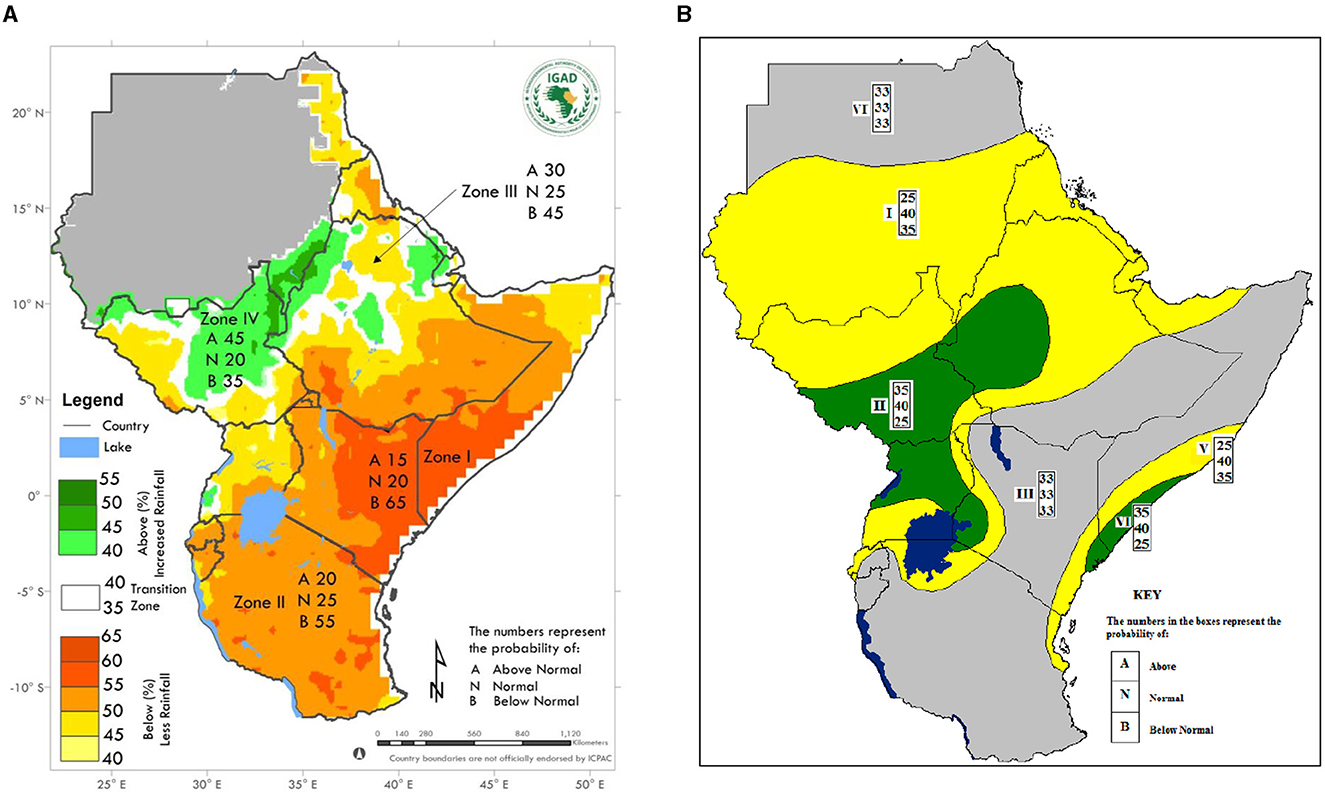
Figure 6. Example of the objective forecast issued (A) vs. the previously produced consensus forecast (B). The objective forecast contains the full probability distribution legend of the three different tercile categories while the consensus forecast does not show the full probability distribution.
In addition to the seasonal probabilistic rainfall and temperature outputs that were initially issued in the Climate Outlook Forums, intra-seasonal forecasts, probabilities of exceedance, and the standardized precipitation index are also issued. The intra-seasonal forecasts include rainfall onset, maximum wet and dry spells length, and the timing of these (Figure 7).
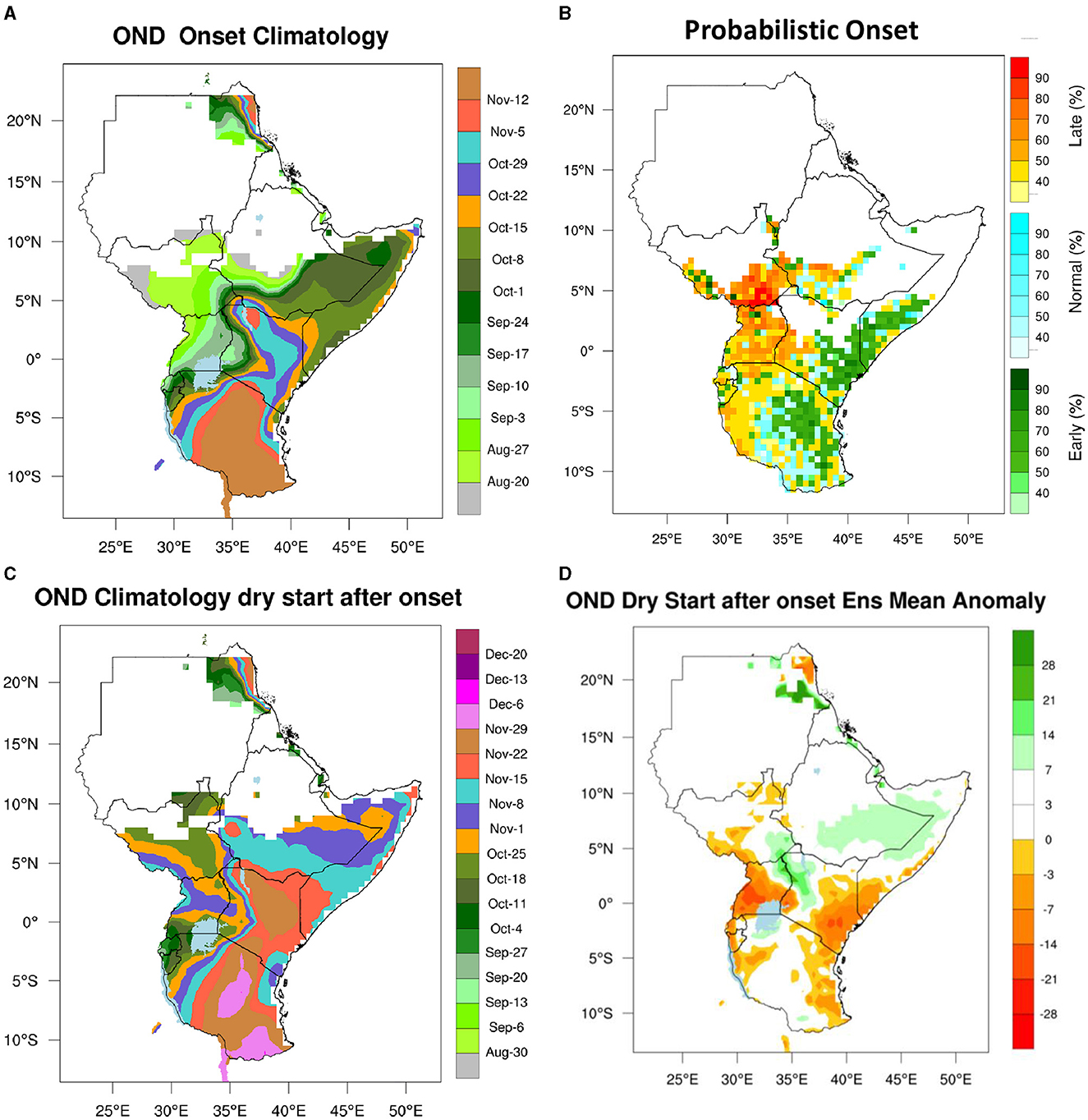
Figure 7. Example of Intraseasonal characteristics products that are produced before the start of the major rainfall seasons over the GHA region (A) onset, (B) probabilistic onset, (C) maximum dryspell, and (D) anomaly of maximum dry spell. Products are disseminated during the Greater Horn of Africa Climate Outlook Forum and also available from the ICPAC website.
One major advantage of utilizing the objective method is that the output datasets can be used in application models such as crop modeling, hydrology, and forage forecasts. Currently, the outputs from the seasonal forecasts are translated into seasonal water forecasts for a drainage basin (MacLeod et al., 2023). These pilot operational experimental water forecasts would soon become part of products disseminated to regional stakeholders at GHACOF. The methodology applied translates objective tercile climate probabilities into impact-relevant water balance forecasts at high spatial resolution in an efficient, transparent, and flexible way.
2.2.1.6 Evaluation tools
It is essential to continuously improve the forecasting system and monitor the performance of the real-time forecasts. Such evaluation is of key importance for identifying strengths and weaknesses of different prediction systems. It is also required for recalibration of dynamical forecasts and for parameter estimation of statistical forecast systems. ICPAC has developed an in-region R tool to evaluate the performance of the objective seasonal forecasts. The tool is based on the R verification library.
In addition to this R in-region tool, a publicaly available R package has been developed and is currently being utilized by ICPAC and NMHS over the GHA to evaluate the seasonal objective forecasts (Heinrich-Mertsching et al., 2023a). The SeaVal package can be easily adapted to country level or regional level outside the Greater Horn of Africa. It allows calculation and visualization of all WMO-recommended evaluation metrics (Mason, 2018) and for each model in the multi-model objective ensemble forecast. Figure 8 shows examples of evaluation metrics calculated by SeaVal. The shown plots are directly generated by the software. For this example, metrics were calculated from August-initialized forecasts by ECMWFs SEAS5 system, for November and December precipitation.
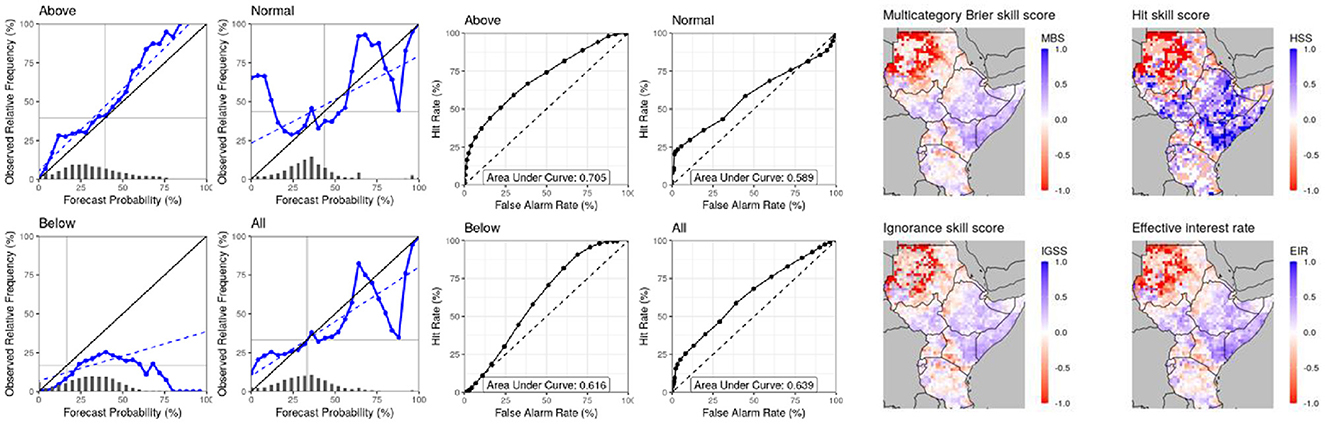
Figure 8. Examples plots of several evaluation metrics, generated by SeaVal. From left to right we have reliability diagrams, ROC-curves, and spatial maps of the Multi-category Brier Skill Score, Hit, Ignorance and Effective Interest rate skills.
In practice, forecasts are evaluated by comparing their past predictions to observational datasets. This involves several subtasks, such as loading past observations and matching forecasts and observations on different grids. The SeaVal package provides software for all of these steps, aiming to simplify evaluation as far as possible. In particular, it provides a direct interface to monthly means precipitation data (CHIRPS, Funk et al., 2015), which is one of the most used datasets sets of reference observations for the GHA region.
The package is being developed with accessibility in mind. It has been installed on ICPACs HPC and can moreover easily be installed on any computer. This allows the regional NMHSs to access the software directly. In November 2022, members of the NMHSs were trained to utilize SeaVal. The package does not require deep knowledge of the R programming language. The software is continuously improved and new features are added regularly.
2.2.2 Coproduction of climate services
Co-production of climate services refers to a collaborative approach to developing and using climate information and services, where stakeholders from various sectors work together to create and implement climate solutions (Carter et al., 2019; ICPAC, 2021). A decade ago, most climate products were produced with limited interaction with climate information users. However, in recent years user engagement has been key in the development of climate services, supporting interpretation and increasing the utility of climate information in the decision-making process (Hirons et al., 2021; ICPAC, 2021; Gudoshava et al., 2022a). Co-production has led to the provision of new climate products over the region such as probability of exceedance, soil moisture anomalies, extreme rainfall at weekly timescales, standardized precipitation index, vegetation condition index, and intra-seasonal characteristics including maximum dry and wet spells and their timing.
Different co-production techniques have been utilized for the different user groups in order to fully engage with the climate information users. The testbed approach, which serves as a forum to generate sound interaction between stakeholders (e.g., forecasters, academics and climate information users) was utilized to co-produce climate services at both national and regional level for the energy, water, and agriculture and food security sectors (Hirons et al., 2021). The Participatory Impact Pathway Analysis (PIPA) method brings together climate information producers, users and other stakeholders and make explicit the users objectives and needs. The method has been utilized at the country level for example in Kenya it was utilized to understand the reasons for the limited use of forecasts and co-produce tailored products for the National drought early warning system (Mwangi Visman, 2020). Numerous activities over the region have also utilized the Participatory Integrated Climate Services for Agriculture (PICSA)] at local level to inform decision making at farm level [(World Meteorological Organisation (WMO), 2022b)]. Tall et al. (2014), showed that a face-to-face discussion with the farmers can help in breaking down the complex climate information into simple terms that can increase the climate information uptake. This method was adopted at subnational level where Participatory Scenario Planning (PSP) has been utilized in the provision of climate services [Ambani et al., 2019, 2021; World Meteorological Organisation (WMO), 2022b]. During the PSP, there is provision of presentation and integration of the Indigenous knowledge in the seasonal forecasts. In many instances, there is alignment between the forecasts derived from indigenous knowledge and those provided by the NMHS (Liang, 2017). This facilitates a smooth consensus-building process and scenario development by the communities. The integration of the Indigenous knowledge in the seasonal forecasts at sub-national level has enhanced sense of ownership of the forecast by farmers/pastoralists (Muriithi et al., 2018). In cases where there is disagreement the forecasts from the meteorological department are given preference. Indigenous forecasters often rely on indicators such as animal intestines, bird migration, and celestial observations (Liang, 2017).
2.2.3 Communication channels and feedback
Rigby et al. (2022) reported that from the interviews carried out over parts of the Greater Horn of Africa region participants elaborated that information should be understandable and usable for the intended recipient, and the method of dissemination should be tailored. Over the past years, the region has invested in tailoring the dissemination methods. Digital platforms are increasingly being utilized in climate services to provide access to climate data, tools, and information to a wider audience. In addition to the physical engagements that are utilized in forecast dissemination, social media, radio, TV, emails, short message services, and website are also utilized. Figure 9 shows the number of users accessing the ICPAC website6 worldwide and the webpages that are frequently visited for the period 1 August 2021 to 31 July 2023.
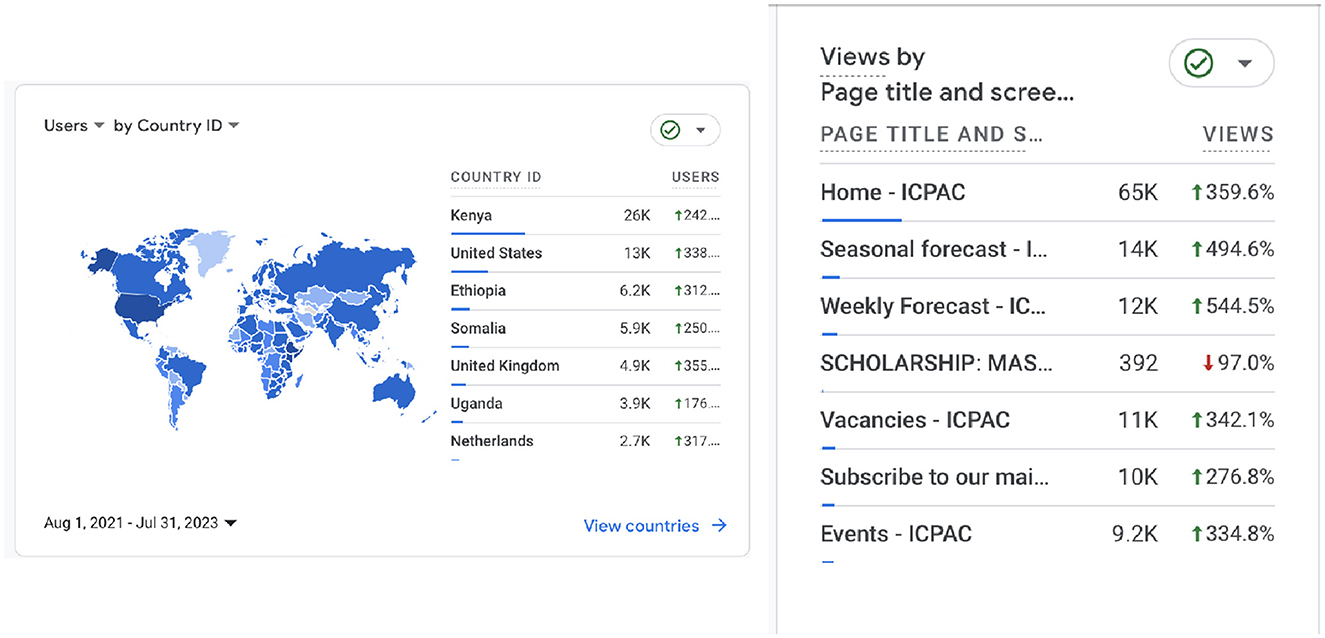
Figure 9. Summary of users that access the ICPAC website by geographical locations and the pages that the users visit. The time period for this analysis is 1 August 2021 to 31 July 2023.
ICPAC has also developed the East Africa hazards watch,7 a platform where stakeholders can interact with the data and get impact-based forecasts for their specific locations (Figure 10). The online platform can generate reports for users on specific regions and how many people are likely to be impacted. Additionally, a user can obtain information on the major hazards, over the region for example, chance of extreme rainfall, drought and food insecurity over the region.
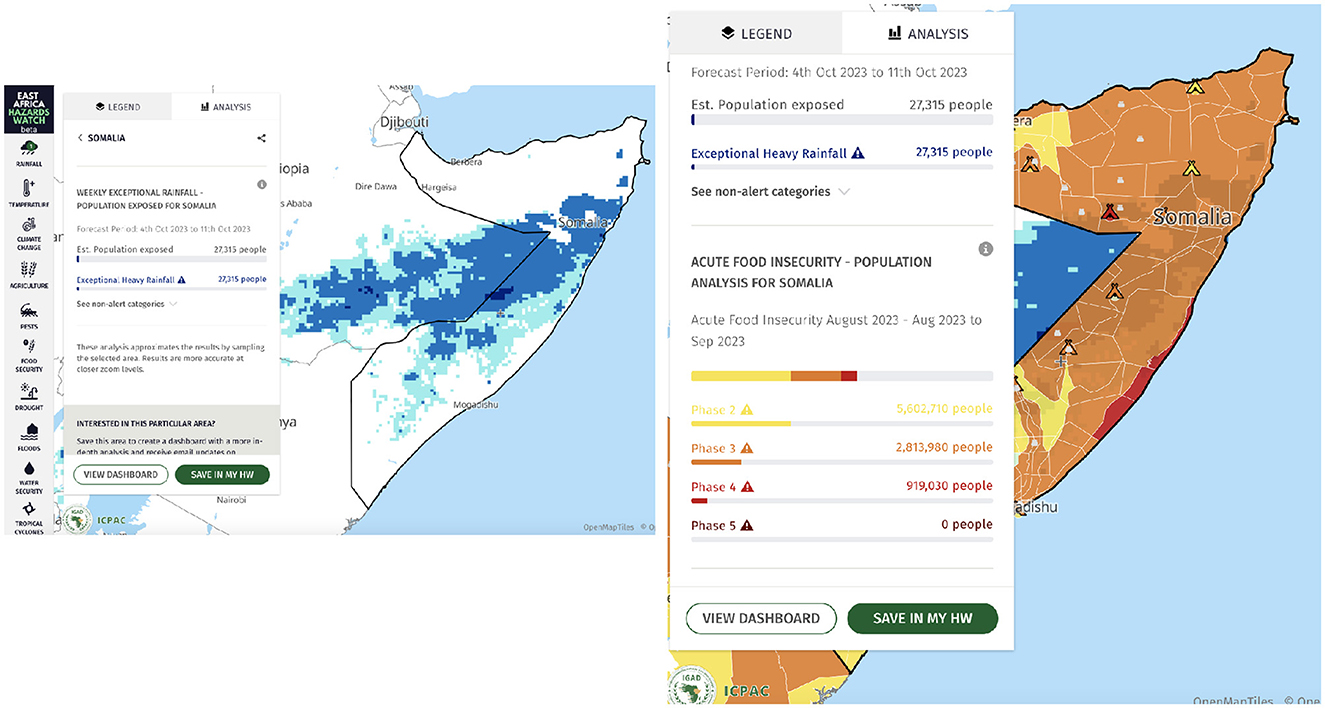
Figure 10. Extract of the information that can be obtained from the East Africa Hazards Watch (https://eahazardswatch.icpac.net/map/ea/).
In addition, ICPAC and the NMHS utilize maprooms.8 Maprooms is a suite of free, location and sector-specific online analyses and visualizations tool of climate data which supports decision-making and adaptation in various climate sensitive sectors.
Numerous countries have established initiatives to disseminate the climate information, including the mobile phone SMS database system, FarmSMS over Tanzania (Kijazi et al., 2021), PlantVillage, in Kenya that's provides both text and television-based advisories, Radio learning Clubs in Rwanda (Funk et al., 2023), and Ethiopian Digital AgroClimate Advisory Platform (Seid et al., 2020). Additionally, for local users' co-delivery of climate information and a feedback channel has been developed through Seasonal Media Action Plan (SMAP), a platform where the media communicates the climate information directly through local radio stations and are able to interact with users (callers) and get feedback on the usefulness of the climate information [(World Meteorological Organisation (WMO), 2022b)]. One major advantage of this method is that the information is communicated in the native language, thus, reducing the confusion that can arise from language differences.
2.3 Observations and monitoring
The GHA is one of the regions that have sparse observational weather stations (Dinku et al., 2014; Kondela et al., 2015; Nsabagwa et al., 2016; Dinku, 2019; Mathew, 2022; Tzachor et al., 2023). Since data is key in weather and climate analysis, several interventions have been initiated with an aim of improving climate data services. Firstly, most countries have worked toward bridging the gap in the number of observing stations through the adoption of Automatic Weather Stations (AWS), 3-D printed automated weather stations, installation of radars, utilization of remote sensing data, and high-resolution reanalysis (Shanko, 2015; Waniha et al., 2019; Muita et al., 2021; Dinku et al., 2022a). Secondly, consultations between NMHSs and ICPAC have been conducted to understand the gaps in maintaining and collecting observational data from the different member states. Through these consultations, a Memorandum of Understanding has been signed, and this is expected to enhance data sharing in the region (CLIMSA Data Managers report, 2021). Thirdly, the management and archiving of weather/climate data has received a major boost since the introduction of the non-proprietary version of climate database management system promoted by the World Meteorological Organization (WMO) known as Climsoft.9
More important is the capacity to use the available data to generate routine products and provide services to end users. In this regard, several tools have been developed with the aim of creating efficient workflows from data management and archiving to generation of climate products (Dinh, 2022). Some examples include; (i) the Climate Data Tools (CDT) which has improved the generation of blended data products (satellite and station datasets), quality control procedures, and the generation of climatological products (Dinku et al., 2022b); and (ii) AWS Data Tools (ADT) which is an online tool for efficiently managing AWS network especially from vendors who use different databases (Faniriantsoa and Dinku, 2022). All these interventions have been felt at both national and regional levels.
2.4 Research, modeling, and prediction
In recent years significant strides have been made in climate research across Eastern Africa, with particular focus on skills assessment (e.g., Kipkogei et al., 2017; Kilavi et al., 2018; de Andrade et al., 2021; Endris et al., 2021), customization and evaluation of the Weather Research and forecasting model (e.g., Pohl et al., 2011; Argent et al., 2015; Otieno et al., 2020; Anyah et al., 2022; Nooni et al., 2022), convective permitting simulations (e.g., Woodhams et al., 2018; Finney et al., 2019; Misiani et al., 2020; Cafaro et al., 2021) and understanding the climate drivers and how their representation in models can affect the accuracy (e.g., Funk et al., 2019; Gudoshava and Semazzi, 2019; Wainwright et al., 2019, 2021a,b; Kolstad et al., 2021; Lyon and DeWitt, 2012; Nicholson, 2014; Vellinga and Milton, 2018; Gudoshava et al., 2022b; Liu et al., 2022; Maybee et al., 2023; Mwanthi et al., 2023; Palmer et al., 2023). With the increase in ensemble members from Global Climate Centers and the need to run high resolution models, there is need for methods of selecting ensemble members. Heinrich-Mertsching et al. (2023b) showed that sub-selection of ensemble members leads to systematically higher skill than random sub-selection. The economic impact of extreme weather has increased over the past decades and necessitates the production of forecasts that includes information on what the weather will do i.e., impact-based forecasts [(World Meteorological Organisation (WMO), 2021)]. A number of studies in the region have also focused on the impact-based forecasts (e.g., Boult et al., 2020; Mwangi et al., 2021; Busker et al., 2023; Mitheu et al., 2023a), however the production of operational impact-based forecasts is still limited over the region.
2.5 Capacity development
A number of capacity building initiatives have been conducted over the region (Dinh, 2022; Grossi et al., 2022; Kiplagat, 2022). Capacity building workshops on use of data processing tools such as CDT have been completed (Faniriantsoa, 2017; Dinh, 2022; Faniriantsoa and Grossi, 2022). A python version of the Climate Predictability Tool, International Research Institute for Climate Society (IRI), was recently developed and training with this tool has been conducted at both regional and national level (Grossi et al., 2021, 2022; Seid et al., 2021). Additionally, the tools to generate the objective forecast products have been extended to the National Meteorological and Hydrological Services (NMHSs) over Eastern Africa by the provision of access to the data and scripts on the ICPAC High performance computing (HPC) system. Furthermore, training on objective forecasting has been conducted during the capacity-building workshops that precede the Greater Horn of Africa Climate Outlook Forums and the annual Foundational training workshop (Kiplagat, 2022; Lore and Lines, 2022). Additionally, new infrastructure has been established for prediction purposes. ICPAC received HPC systems that currently is also utilized by the member states in dynamical downscaling and also postprocessing of the model outputs (AFDB, 2020; United Kingdom Met Office, 2020; WISER, 2021). The dissemination of weather and climatic information requires the use of media; thus efforts have been done over the region to train journalists on the climate terms and breaking down the information to make it understandable (Jelagat, 2023). A Massive Open Online Course has been established and journalists are able to take the course for free.10 The online courses are available in English, French, and Arabic.
3 Challenges/gaps, and way forward in provision of climate services
In this section the study highlights the Challenges/Gaps in the provision of climate services at both regional and national level. Furthermore, the section outlines several potential methods/ways forward.
3.1 User interface platforms
Although there has been progress in the establishment of user interface platforms, there is need for establishment of sector specific UIP at both regional and national level as recommended by WMO (Pathak and Lúcio, 2018; Dupar and Weingärtner, 2021; Kijazi et al., 2021). There is need to routinely collect feedback from users about the usefulness and effectiveness of climate information products and services, and then redesign information products and services (Lennard et al., 2018). Building upon the existing Technical Advisory Committees (TAC) for example the Disaster Risk management TAC over Eastern Africa rather than forming new pathways can quicken the process of formation of UIP at sector level.
3.2 Climate services information system
3.2.1 Generation of forecast information and availability of tools
Currently a limited number of NMHS over the region have the FASTA app developed over their countries with only the Kenya Meteorological Department utilizing the app. Hence there is need for this app to be utilized in other countries. Additionally, a number of NMHS are not aware of the existence of the nowcasting catalog that is currently being utilized by KMD. Hence, a need of raising of awareness on software and tools available for nowcasting. Capacity building of the use of the app and interpretation of the products in the catalog also needs to be conducted.
The development of impact-based forecast requires the availability of the socio-economic datasets so as to develop the different thresholds, however these datasets are limited over the region (Mitheu et al., 2023b). To improve the availability and quality of socio-economic data in the region sustained investment in data collection and analysis, as well as greater collaboration between governments, international organizations, and other stakeholders will be required. With improved data, it will be possible to develop more accurate and effective impact-based forecasts that can help to reduce the risks and impacts of natural disasters in the region. A single dashboard that will host the different datasets will make it easier to access the data and develop the IBF.
Even though routine skills verification of the seasonal forecasts is conducted, there is limited verification of the forecasts for the nowcasting to sub-seasonal timescales. Thus, easily adaptable tools for skill verification for these timescales need to be developed over the region.
3.2.2 User engagement and co-production
User engagement is essential for increased uptake and ownership of the forecast products; however, the co-production is resource-intensive and funding is required in order for the process to continue (Hirons et al., 2021). During the GCRF African SWIFT project (Parker et al., 2021), not enough resources were allocated to the co-production thereby leading to limited engagements with the users. The prototype products that were produced did not have enough feedback for further enhancement. Gudoshava et al. (2022a), reported that the users who are not involved in the co-production process have difficulties in interpreting the forecast products. It is, therefore, essential to develop digital tools that can be used in the upscaling of the coproduction process and also invest in more co-production processes.
3.3 Observations and monitoring
Climate services are based on consistent climate data of high quality. Although a lot has been achieved over the recent past, serious gaps within the climate data management process could stagnate the positive trend already realized. One key gap is related to building the capacity of NMHSs both infrastructurally and in staff skills (Mwesigwa et al., 2015; Lennard et al., 2018; Waruru, 2018; Nsabagwa et al., 2019). Additionally, in most countries over the GHA region the management and maintenance plan/operational procedures for weather and climate observations is relatively weak (Shanko, 2015; Nsabagwa et al., 2016; Aura et al., 2019). For this to be sustainable, governments should provide financial support, through special budgetary allocations, to ensure sustainability of the stations as most synoptic stations are currently not functional (Aura et al., 2019; Mathew, 2022). NMHSs should also position themselves strategically to take advantage of global initiatives which promise to bring change to weather/climate observations such as the Systematic Observations Financing Facility (SOFF) an initiative by WMO and the Alliance for Hydromet Development [(World Meteorological Organisation (WMO), 2020)].
3.4 Research, modeling, and prediction
During the Long rains (March–May) season, the seasonal forecast skill is generally lower (Dutra et al., 2013; Walker et al., 2019) than the other two seasons over the GHA that is the Short Rains (October–December) and the June to September season. The low skill in the Long rains has the potential to erode the public trust thereby reducing the uptake of the early warning information, reduce preparedness to the extreme events, and misallocation of resources by the government and humanitarian organizations. To tackle this issue, further research needs to be conducted to enhance the accuracy of Long rains forecasts. This could involve the integration of new statistical approaches, ensemble member selection (Heinrich-Mertsching et al., 2023b), ongoing research on exploring the utilization of machine learning techniques (Deman et al., 2022) and hybrid methods that take into consideration the different forecasting approaches.
3.5 Capacity development
There is limited technical expertise in the region for data processing and climate modeling from the National Meteorological and Hydrological Agencies (Lennard et al., 2018). Figure 11 illustrates the skills expertise of the forecasters that were trained at ICPAC. It is evident that most of the forecasters have limited skills in most programming languages especially the analysis software such as R and Python. A fair number of participants have also indicated the need for further training on tools that are utilized to generate the seasonal forecast. To address this challenge, efforts are underway to build the capacity in climate modeling related fields over the region. These include in-country trainings, the 2-week foundational training, 1 week training before the GHACOF, 2–3 months attachments of forecasters at ICPAC and also the monthly forecast generation training meetings. In addition to these interventions training manual and online portals can be developed that can help the forecasters in developing the skills required to produce the forecasts at all timescales.
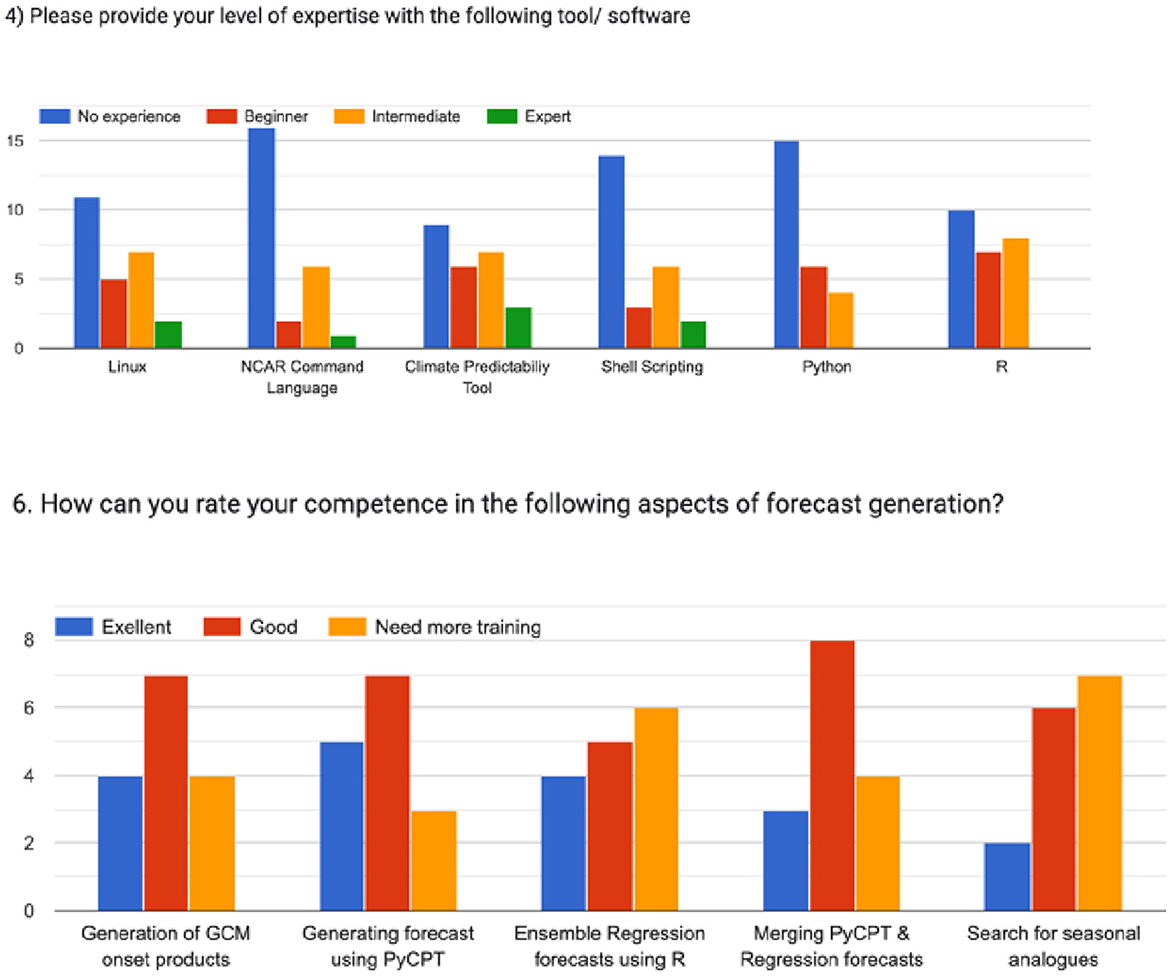
Figure 11. Extract of the survey outputs on the skills of forecasters from the Member states over the Greater Horn of Africa on use of tools/software and ability to generate the forecasts.
High-performance computing facilities are limited over the region which limits the resolution and complexity of models that can be run (Kijazi et al., 2021; Heinrich-Mertsching et al., 2023b). Considering that the region has complex topography and large inland water bodies it is essential to run the models and resolutions that can resolve these local effects (Argent et al., 2015; Ntwali et al., 2016; Kerandi et al., 2017). The coarse resolution simulations can result in lower accuracy and precision in the forecasts (Kerandi et al., 2017). In addition to the limited HPC systems there is also inadequate storage facilities for the simulations that are produced. Investment in a High-performance computing systems, collaboration with institutions that have advanced high computing systems and utilization of the cloud computing systems could likely alleviate the computational and storage problems currently faced.
While progress has been made in enhancing the communication of climate information, particularly through journalist training for seasonal forecasting, there remains a notable gap in interpreting this information. The existing training efforts have predominantly focused on seasonal forecasts, with limited attention given to more frequent forecasts, ranging from nowcasting to sub-seasonal forecasts. Therefore, there is a crucial necessity for additional specialized training programs across all timescales. The aim of these initiatives should be equipping journalists with the skills and knowledge essential for accurate climate reporting. Moreover, there is a persistent challenge in the interpretation of probabilistic forecasts, as highlighted by user feedback (Gudoshava et al., 2022a). Consequently, further engagement with stakeholders through co-production and media channels is imperative to address these interpretation difficulties.
4 Conclusion
Advances in climate services through forecast generation, co-production of climate services, capacity building, and communication of climate information has been enhanced the Greater Horn of Africa. The human capacity to produce forecasts has been enhanced through various training activities targeted at forecasters from the different NMHS. Access to the HPC located at ICPAC, by the member states forecasters has allowed for ease of scale-up of the objective forecasts and sustainability of production of these forecasts operationally. The sustainability of some products has been achieved through various strategies at both regional and national levels.
Despite the advancements, several challenges, and gaps persist. Capacity gaps in data collection, analysis, and interpretation pose significant challenges to the provision of accurate and timely climate services. Limited availability and accessibility of high-quality socioeconomic data hinder the development of effective impact-based forecasts. Additionally, the communication and dissemination of climate information to end-users remains a challenge, particularly in reaching vulnerable communities with low access to information.
Addressing these challenges requires collaborative efforts between meteorological agencies, researchers, policymakers, and other stakeholders. Investments in capacity building, particularly in data collection, forecast development, and analysis, are essential to enhance the accuracy and reliability of climate services. Strengthening partnerships and knowledge exchange between stakeholders can facilitate the co-production of climate information that meets the specific needs of different sectors and communities. Improving communication channels and enhancing accessibility to climate information will enhance the effectiveness and impact of climate services, particularly for vulnerable populations. By addressing these issues, the region will be better equipped to deal with the effects of climate variability and change. Decision-makers and stakeholders will also have the knowledge they need to take early action and reduce any potential negative impacts brought on by extreme weather events or long-term climate trends. Therefore, active participation in initiatives such as the Sendai Framework for Disaster Risk Reduction, Systematic Observations Financing Facility, Early Warnings for All, and other programs dedicated to enhancing early warning systems is crucial for the region.
Author contributions
MG: Conceptualization, Writing – original draft, Methodology. GO: Conceptualization, Writing – original draft. EK: Conceptualization, Writing – original draft. HM: Conceptualization, Writing – original draft. JO: Writing – review & editing, Resources. CH-M: Resources, Writing – review & editing. CW: Resources, Writing – review & editing. HE: Resources, Writing – review & editing. AM: Resources, Writing – review & editing. MK: Writing – review & editing. EM: Writing – review & editing, Conceptualization. AC: Writing – review & editing. DP: Conceptualization, Writing – review & editing. JM: Writing – review & editing, Conceptualization. PM: Resources, Writing – review & editing. POO: Writing – review & editing. PO: Writing – review & editing, Resources. DA: Resources, Writing – review & editing. AK: Writing – review & editing. IM: Resources, Writing – review & editing. PA: Writing – review & editing, Resources. HH: Writing – review & editing, Resources. MW: Writing – review & editing, Resources. BA: Writing – review & editing, Resources. EK: Resources, Writing – review & editing. MS: Resources, Writing – review & editing. TB: Writing – review & editing. MT: Writing – review & editing, Conceptualization. ZS: Funding acquisition, Supervision, Writing – review & editing. ZA: Funding acquisition, Supervision, Writing – review & editing. GA: Funding acquisition, Supervision, Writing – review & editing.
Funding
The author(s) declare financial support was received for the research, authorship, and/or publication of this article. The research was supported by funding from the European Union's Horizon 2020 programs through the Co-production of Climate Services for East Africa (CONFER) and DOWN2EARTH projects (grants 869730 and 869550) and the Intra-ACP Climate Services and Related Applications CLIMSA project (grant number ACP/FED/038-833). The Intra-ACP Climate Services and Related Applications project paid for the article publishing charges.
Conflict of interest
JO and MK employed by Kenya Meteorological Department. CH-M was employed by Norwegian Computing Center. AC was employed by Met Office. DP was employed by Norwegian Research Centre. DA and AK were employed by Tanzania Meteorological Authority. IM was employed by Uganda National Meteorological Authority. PA was employed by Rwanda Meteorological Agency. YH and SW were employed by Agence Nationale de la météorologie de Djibouti. BA was employed by Ethiopia Meteorological Institute. EK was employed by Institut Géographique du Burundi. ZS was employed by National Oceanic and Atmospheric Administration.
The remaining authors declare that the research was conducted in the absence of any commercial or financial relationships that could be construed as a potential conflict of interest.
Publisher's note
All claims expressed in this article are solely those of the authors and do not necessarily represent those of their affiliated organizations, or those of the publisher, the editors and the reviewers. Any product that may be evaluated in this article, or claim that may be made by its manufacturer, is not guaranteed or endorsed by the publisher.
Footnotes
1. ^https://unma.go.ug/weather/six-hourly-updates
2. ^https://www.meteorwanda.gov.rw/index.php?id=55
4. ^https://science.ncas.ac.uk/swift/
5. ^https://africanswift.org/short-term-forecasting-kenya/
7. ^https://eahazardswatch.icpac.net/map/ea/
8. ^http://digilib.icpac.net/maproom/index.html
10. ^https://bbcmediaactionilearn.org/course/view.php?id=242
References
AFDB (2020). Available online at: https://www.afdb.org/en/topics-and-sectors/initiatives-partnerships/climate-for-development-in-africa-climdev-africa-initiative (accessed December 16, 2023).
Ambani, M., Gbetibouo, G. A., and Percy, F. (2021). “Multi-stakeholder dialogue to co-design anticipatory adaptation: lessons from participatory scenario planning in Africa,” in Handbook of Climate Change Management: Research, Leadership, Transformation, eds W. Leal Filho, J. Luetz, and D. Ayal (Cham: Springer), 1–25. doi: 10.1007/978-3-030-22759-3_111-1
Ambani, M., Shikuku, P., Percy, F., and Maina, J. (2019). “Practical guide to participatory scenario planning,” in CARE Climate Change. Available online at: https://careclimatechange.org/practical-guide-to-participatory-scenario-planning-seasonal-climate-information-for-resilient-decision-making/ (accessed January 4, 2024).
Anticipation Hub (2023). Forecasting the June to September season at Somalia's Climate Outlook Forum. Available online at: https://www.anticipation-hub.org/news/forecasting-the-june-to-september-season-at-somalias-climate-outlook-forum (accessed January 18, 2024).
Anyah, R., Xia, S., and Semazzi, F. (2022). Influence of water table dynamics on spatial and temporal patterns of hydroclimate extremes over Lake Victoria Basin, East Africa: comparison of wet and dry years. Int. J. Climatol. 42, 7878–7898. doi: 10.1002/joc.7682
Argent, R., Sun, X., Semazzi, F., Xie, L., and Liu, B. (2015). The development of a customization framework for the WRF Model over the Lake Victoria basin, Eastern Africa on seasonal timescales. Adv. Meteorol. 2015:653473. doi: 10.1155/2015/653473
Aura, S., Muita, R., Oloo, P., Muchemi, D., Kucera, P., Mwangi, S., et al. (2019). Intercomparison of TAHMO, 3DPAWS Automatic Weather Stations and KMD's Dagoretti Corner, Nairobi Synoptic Weather Station Data, UK Met Office. Available online at: https://www.metoffice.gov.uk/binaries/content/assets/metofficegovuk/pdf/business/international/wiser/wiser0170_highway_obs_data_intercomparison.pdf
Bedasa, Y., and Bedemo, A. (2022). The effect of climate change on food insecurity in the Horn of Africa. GeoJournal 1–11. doi: 10.1007/s10708-022-10733-1
Bizimana, J. P., and Schilling, M. (2010). “Geo-information technology for infrastructural flood risk analysis in unplanned settlements: a case study of informal settlement flood risk in the Nyabugogo flood plain, Kigali City, Rwanda,” in Geospatial Techniques in Urban Hazard and Disaster Analysis, eds P. Showalter, and Y. Lu (Dordrecht: Springer), 99–124. doi: 10.1007/978-90-481-2238-7_6
Boult, V. L., Asfaw, D. T., Young, M., Maidment, R., Mwangi, E., Ambani, M., et al. (2020). Evaluation and validation of TAMSAT-ALERT soil moisture and WRSI for use in drought anticipatory action. Meteorol. Appl. 27:e1959. doi: 10.1002/met.1959
Busker, T., de Moel, H., van den Hurk, B., and Aerts, J. C. (2023). Impact-based seasonal rainfall forecasting to trigger early action for droughts. Sci. Total Environ. 898:165506. doi: 10.1016/j.scitotenv.2023.165506
Cafaro, C., Woodhams, B. J., Stein, T. H., Birch, C. E., Webster, S., Bain, C. L., et al. (2021). Do convection-permitting ensembles lead to more skillful short-range probabilistic rainfall forecasts over tropical East Africa? Weather Forecast. 36, 697–716. doi: 10.1175/WAF-D-20-0172.1
Carter, S., Steynor, A., Waagsaether, K., Vincent, K., and Visman, E. (2019). “Co-production of 858 African weather and climate services,” in Manual, Cape Town: SouthSouthNorth.859. Available online at: https://futureclimateafrica.org/coproduction-manual (accessed December 16, 2021).
Case, J. L., Mungai, J., Sakwa, V., Kabuchanga, E., Zavodsky, B. T., Limaye, A. S., et al. (2014). “Toward improved land surface initialization in support of regional WRF forecasts at the Kenya Meteorological Service (KMS),” in Conference on Numerical Weather Prediction (No. M14-3465). Available online at: https://ntrs.nasa.gov/citations/20140010112
Chang'a, L., Kijazi, A., Mafuru, K., Kondowe, A., Osima, S., Mtongori, H., et al. (2020). Assessment of the evolution and socio-economic impacts of extreme rainfall events in October 2019 over the East Africa. Atmos. Clim. Sci. 10, 319–338. doi: 10.4236/acs.2020.103018
Clark, P., Roberts, N., Lean, H., Ballard, S. P., and Charlton-Perez, C. (2016). Convection-permitting models: a step-change in rainfall forecasting. Meteor. Appl. 23, 165–181. doi: 10.1002/met.1538
de Andrade, F. M., Young, M. P., MacLeod, D., Hirons, L. C., Woolnough, S. J., Black, E., et al. (2021). Subseasonal precipitation prediction for Africa: forecast evaluation and sources of predictability. Weather Forecast. 36, 265–284. doi: 10.1175/WAF-D-20-0054.1
Deman, V., Koppa, A., Waegeman, W., MacLeod, D. A., Bliss Singer, M., Miralles, D., et al. (2022). Seasonal prediction of Horn of Africa long rains using machine learning: the pitfalls of preselecting correlated predictors. Fronti. Water 4:1053020. doi: 10.3389/frwa.2022.1053020
Dinh, D. (2022). Advanced Climate Data Tool (CDT) Training and Automatic Weather Station Data Tool (ADT) Part II for Kenya Meteorological Department (KMD). Available online at: https://cgspace.cgiar.org/items/a0f54098-38e7-413a-949a-f9e58122cac8
Dinku, T. (2019). “Challenges with availability and quality of climate data in Africa,” in Extreme Hydrology and Climate Variability (Elsevier), 71–80.
Dinku, T., Faniriantsoa, R., Cousin, R., Khomyakov, I., Vadillo, A., Hansen, J. W., et al. (2022a). ENACTS: advancing climate services across Africa. Front. Clim. 3:176. doi: 10.3389/fclim.2021.787683
Dinku, T., Faniriantsoa, R., Islam, S., and Nsengiyumva, G. (2022b). The climate data tool: enhancing climate services across Africa. Front. Clim. 185:787519. doi: 10.3389/fclim.2021.787519
Dinku, T., Hailemariam, K., Maidment, R., Tarnavsky, E., and Connor, S. (2014). Combined use of satellite estimates and rain gauge observations to generate high-quality historical rainfall time series over Ethiopia. Int. J. Climatol. 34, 2489–2504. doi: 10.1002/joc.3855
Doblas-Reyes, F., Garcia, A., Hansen, J., Mariani, L., Nain, A., Ramesh, K., et al. (2003). “Weather and climate forecasts for agriculture,” in Guide to Agricultural, Meteorological Practices, eds H. P. Das and K. Stigter (Geneva: World Meteorological Organisation), 57.
Dupar, M., and Weingärtner, L. (2021). Investing for Sustainable Climate Services: Insights from African experience. ODI Report. Available online at: https://www.econstor.eu/handle/10419/251137
Dutra, E., Magnusson, L., Wetterhall, F., Cloke, H. L., Balsamo, G., Boussetta, S., et al. (2013). The 2010–2011 drought in the Horn of Africa in ECMWF reanalysis and seasonal forecast products. Int. J. Climatol. 33, 1720–1729. doi: 10.1002/joc.3545
Endris, H. S., Hirons, L., Segele, Z. T., Gudoshava, M., Woolnough, S., Artan, G. A., et al. (2021). Evaluation of the skill of monthly precipitation forecasts from global prediction systems over the Greater Horn of Africa. Weather Forecast. 36, 1275–1298. doi: 10.1175/WAF-D-20-0177.1
Faniriantsoa, R. (2017). Training on IRI Climate Data Tools and developing a method for integrating climate data in Kigali, Rwanda. CCAFS Workshop Report. Wageningen: CGIAR Research Program on Climate Change, Agriculture and Food Security (CCAFS).
Faniriantsoa, R., and Dinku, T. (2022). ADT: The automatic weather station data tool. Front. Clim. 156:933543. doi: 10.3389/fclim.2022.933543
Faniriantsoa, R., and Grossi, A. (2022). Advanced Training on Climate Data Tools (CDT) and AWS Data Tools (ADT): Installation and Training for the Ethiopian National Meteorological Agency (NMA). Available online at: https://hdl.handle.net/10568/119761 (accessed January 21, 2024).
FarmAfrica (2023). Available online at: https://www.farmafrica.org/what-we-do-1/climate-crisis-drought-in-eastern-africa (accessed September 14, 2023).
FASTA (2023). Bringing Near Real-time Storm Information to Africa to Save Lives and Protect Livelihoods. FASTA. Available online at: https://fastaweather.com/ (accessed June 7, 2023).
Fink, A. H., Engel, T., Ermert, V., van der Linden, R., Schneidewind, M., Redl, R., et al. (2017). “Mean climate and seasonal cycle,” in Meteorology of Tropical West Africa: The Forecasters' Handbook, eds D. J. Parker, and M. Diop-Kane (Hoboken, NJ: John Wiley and Sons), 139.
Finney, D. L., Marsham, J. H., Jackson, L. S., Kendon, E. J., Rowell, D. P., Boorman, P. M., et al. (2019). Implications of improved representation of convection for the East Africa water budget using a convection-permitting model. J. Clim. 32, 2109–2129. doi: 10.1175/JCLI-D-18-0387.1
Funk, C., Harrison, L., Segele, Z., Rosenstock, T., Steward, P., Anderson, C. L., et al. (2023). Tailored forecasts can predict extreme climate informing proactive interventions in East Africa. Earths Future 11:e2023EF003524. doi: 10.1029/2023EF003524
Funk, C., Harrison, L., Shukla, S., Pomposi, C., Galu, G., Korecha, D., et al. (2018). Examining the role of unusually warm Indo-Pacific sea-surface temperatures in recent African droughts. Q. J. R. Meteorol. Soc. 144, 360–383. doi: 10.1002/qj.3266
Funk, C., Hoell, A., Shukla, S., Blade, I., Liebmann, B., Roberts, J. B., et al. (2014). Predicting East African spring droughts using Pacific and Indian Ocean sea surface temperature indices. Hydrol. Earth Syst. Sci. 18, 4965–4978. doi: 10.5194/hess-18-4965-2014
Funk, C., Pedreros, D., Nicholson, S., Hoell, A., Korecha, D., Galu, G., et al. (2019). Examining the potential contributions of extreme “Western V” sea surface temperatures to the 2017 March–June East African Drought. Bull. Am. Meteorol. Soc. 100, S55–S60. doi: 10.1175/BAMS-D-18-0108.1
Funk, C., Peterson, P., Landsfeld, M., Pedreros, D., Verdin, J., Shukla, S., et al. (2015). The climate hazards infrared precipitation with stations—a new environmental record for monitoring extremes. Sci. Data 2, 1–21. doi: 10.1038/sdata.2015.66
Funk, C. C. (2012). Exceptional warming in the western Pacific-Indian Ocean warm pool has contributed to more frequent droughts in eastern Africa. Bull. Am. Meteorol. Soc. 93, 1049–1051. doi: 10.1175/BAMS-D-12-00021.1
Grossi, A., Dinku, T., Faniriantsoa, R., Robertson, A., and Singh, B. (2021). Regional Training for East and Southern Africa. AICCRA Workshop Report. Wageningen: CGIAR Research Program on Climate Change, Agriculture and Food Security (CCAFS).
Grossi, A., Robertson, A., Muñoz, A., Singh, B., Dinku, T., Demissie, T., et al. (2022). East and Southern Africa Regional Training On the Improved NextGen Seasonal Forecasting Approach (PyCPT 2). Available online at: https://cgspace.cgiar.org/items/342216b0-aa2c-4d6b-aedc-fe94079f21fc
Gudoshava, M., and Semazzi, F. H. (2019). Customization and validation of a regional climate model using satellite data over East Africa. Atmosphere 10, 317. doi: 10.3390/atmos10060317
Gudoshava, M., Wainwright, C., Hirons, L., Endris, H. S., Segele, Z. T., Woolnough, S., et al. (2022b). Atmospheric and oceanic conditions associated with early and late onset for Eastern Africa short rains. Int. J. Climatol. 42, 6562–6578. doi: 10.1002/joc.7627
Gudoshava, M., Wanzala, M., Thompson, E., Mwesigwa, J., Endris, H. S., Segele, Z., et al. (2022a). Application of real time S2S forecasts over Eastern Africa in the co-production of climate services. Clim. Serv. 27:100319. doi: 10.1016/j.cliser.2022.100319
Heinrich-Mertsching, C., Cunen, C., and Scheuerer, M. (2023a). Available online at: https://cran.r-project.org/web/packages/SeaVal/index.html (accessed December 10, 2023).
Heinrich-Mertsching, C., Sørland, S. L., Gudoshava, M., Koech, E., Bahaga, T. K., and Sobolowski, S. P. (2023b). Subselection of seasonal ensemble precipitation predictions for East Africa. Q. J. R. Meteorol. Soc. 149, 2634–2653. doi: 10.1002/qj.4525
Hewitt, C., Mason, S., and Walland, D. (2012). The global framework for climate services. Nat. Clim. Change 2, 831–832. doi: 10.1038/nclimate1745
Hill, P. G., Stein, T. H., Roberts, A. J., Fletcher, J. K., Marsham, J. H., and Groves, J. (2020). How skilful are nowcasting satellite applications facility products for tropical Africa? Meteorol. Appl. 27, e1966. doi: 10.1002/met.1966
Hirons, L., Thompson, E., Dione, C., Indasi, V. S., Kilavi, M., Nkiaka, E., et al. (2021). Using co-production to improve the appropriate use of sub-seasonal forecasts in Africa. Clim. Serv. 23, 100246. doi: 10.1016/j.cliser.2021.100246
Hirons, L., Wainwright, C. M., Nying'uro, P., Quaye, D., Ashong, J., Kiptum, C., et al. (2023). Experiences of co-producing sub-seasonal forecast products for agricultural application in Kenya and Ghana. Weather 78, 148–153. doi: 10.1002/wea.4381
ICPAC (2021). ICPAC Guide for Engagement in Coproduing Climate Services. Available online at: https://www.icpac.net/media/documents/A_Guide_for_Engagement_in_Co-Producing_Climate_Services_.pdf (accessed January 21, 2024).
ICPAC (2023). FSNWG Food Security and Nutrition Update. Available online at: https://www.icpac.net/fsnwg/january-2023-update/ (accessed December 19, 2023).
Jelagat (2023). Strengthening Communication of Weather and Climate Information in Eastern Africa. Available online at: https://icpac.medium.com/strengthening-communication-of-weather-and-climate-information-in-eastern-africa-6aff011ef8ee
Kebede, S., and Taye, M. T. (2021). “Groundwater scarcity and management in the arid areas in East Africa,” in Global Groundwater, eds A. Mukherjee, B. R. Scanlon, and? A. Aureli (Amsterdam: Elsevier), 177–186. doi: 10.1016/B978-0-12-818172-0.00013-X
Kenya Metorological Department (2022). 5th National Climate Outlook Forum (NCOF_OND, 2022). Available online at: https://meteo.go.ke/media-center/gallery/national-climate-outlook-forum-ncof-workshop-held-nakuru-21st-22nd-february
Kerandi, N. M., Laux, P., Arnault, J., and Kunstmann, H. (2017). Performance of the WRF model to simulate the seasonal and interannual variability of hydrometeorological variables in East Africa: a case study for the Tana River basin in Kenya. Theor. Appl. Climatol. 130, 401–418. doi: 10.1007/s00704-016-1890-y
Kijazi, A., Chang'a, L., Merchades, M., IYonah, I., Msemo, H., Levira, P., et al. (2021). Implementation of the Global Framework for Climate Services Adaptation Programme in Africa. World Meteorological Organization.
Kikwasi, G., and Mbuya, E. (2019). Vulnerability analysis of building structures to floods: The case of flooding informal settlements in Dar es salaam, Tanzania. Int. J. Build. Pathol. Adapt. 37, 629–656. doi: 10.1108/IJBPA-07-2018-0056
Kilavi, M., MacLeod, D., Ambani, M., Robbins, J., Dankers, R., Graham, R., et al. (2018). Extreme rainfall and flooding over central Kenya including Nairobi city during the long-rains season 2018: causes, predictability, and potential for early warning and actions. Atmosphere 9:472. doi: 10.3390/atmos9120472
Kinyangi, J., Herrero, M. T., Omolo, A., Steeg, J. V. D., and Thornton, P. K. (2009). Scoping Study on Vulnerability to Climate Change and Climate Variability in the Greater Horn of Africa: Mapping Impacts and Adaptive Capacity. Available online at: https://cgspace.cgiar.org/server/api/core/bitstreams/152b24ec-e594-4778-b0a0-5d57edb7e008/content
Kipkogei, O., Mwanthi, A. M., Mwesigwa, J. B., Atheru, Z. K. K., Wanzala, M. A., Artan, G., et al. (2017). Improved seasonal prediction of rainfall over East Africa for application in agriculture: statistical downscaling of CFSv2 and GFDL-FLOR. J. Appl. Meteorol. Climatol. 56, 3229–3243. doi: 10.1175/JAMC-D-16-0365.1
Kiplagat (2022). Available online at: https://confer-h2020.eu/2022/12/confer-s-annual-foundational-climate-prediction-workshop/ (accessed September 14, 2023).
Kiptum, A., Mwangi, E., Otieno, G., Njogu, A., Kilavi, M., Mwai, Z., et al. (2023). Advancing operational flood forecasting, early warning and risk management with new emerging science: Gaps, opportunities and barriers in Kenya. J. Flood Risk Manag. e12884. doi: 10.1111/jfr3.12884
Kogo, B. K., Kumar, L., and Koech, R. (2021). Climate change and variability in Kenya: a review of impacts on agriculture and food security. Environ. Dev. Sustain. 23, 23–43. doi: 10.1007/s10668-020-00589-1
Kolstad, E. W., Macleod, D., and Demissie, T. D. (2021). Drivers of subseasonal forecast errors of the East African short rains. Geophys. Res. Lett. 48:e2021GL.093292. doi: 10.1029/2021GL093292
Kondela, E., Nungu, A., and Pehrson, B. (2015). “Status of existing weather observation station network in Tanzania and the possibility to automate and densify it,” in AFRICON 2015 (Addis Ababa: IEEE), 1–5. doi: 10.1109/AFRCON.2015.7331954
Lelenguyah, G. L., Nyangito, M. M., Wasonga, O. V., and Bett, R. C. (2023). Seasonal distribution of livestock in Samburu county, northern Kenya: a response to variable pasture and water in semi-arid rangelands. Afr. J. Range Forage Sci. 1–14. doi: 10.2989/10220119.2023.2210611
Lennard, C., Steynor, A., Kloppers, K., Tadross, M., Dlamini, L., Madajewicz, M., et al. (2018). NMHS Capacity Development Assessment Report. 2018, USAID-supported Assessing Sustainability and Effectiveness of Climate Information Services in Africa Project. Washington, DC.
Liang, S. (2017). Incorporating Indigenous Knowledge in the Local Government's Early Warning System: A Case Study from Baringo County, Kenya. Lund: Lund University Libraries. Available online at: https://lup.lub.lu.se/luur/download?func=downloadFile&recordOId=8922488&fileOId=8923513 (accessed December 19, 2023).
Liu, Y., Liu, Y., Wang, W., Fan, X., and Cui, W. (2022). Soil moisture droughts in East Africa: Spatiotemporal patterns and climate drivers. J. Hydrol. Reg. Stud. 40:101013. doi: 10.1016/j.ejrh.2022.101013
Lore and Lines (2022). Building Resilience Across East Africa through the Production and Communication of Seasonal Forecasts, ICPAC Medium Available online at: https://icpac.medium.com/building-resilience-across-east-africa-through-the-production-and-communication-of-seasonal-11525bff90f7 (accessed May 5, 2023).
Lungo, A., Kim, S., Jiang, M., Cho, G., and Kim, Y. (2020). Sensitivity study of WRF simulations over tanzania for extreme events during wet and dry seasons. Atmosphere 11:459. doi: 10.3390/atmos11050459
Lyon, B., and DeWitt, D. G. (2012). A recent and abrupt decline in the East African long rains. Geophys. Res. Lett. 39, L02702. doi: 10.1029/2011GL050337
MacLeod, D., Kilavi, M., Mwangi, E., Ambani, M., Osunga, M., Robbins, J., et al. (2021b). Are Kenya Meteorological Department heavy rainfall advisories useful for forecast-based early action and early preparedness for flooding? Nat. Hazards Earth Syst. Sci. 21, 261–277. doi: 10.5194/nhess-21-261-2021
MacLeod, D., Quichimbo, E. A., Michaelides, K., Asfaw, D. T., Rosolem, R., Cuthbert, M. O., et al. (2023). Translating seasonal climate forecasts into water balance forecasts for decision making. PLOS Clim. 2:e0000138. doi: 10.1371/journal.pclm.0000138
MacLeod, D. A., Dankers, R., Graham, R., Guigma, K., Jenkins, L., Todd, M. C., et al. (2021a). Drivers and subseasonal predictability of heavy rainfall in equatorial East Africa and relationship with flood risk. J. Hydrometeorol. 22, 887–903. doi: 10.1175/JHM-D-20-0211.1
Mason, S. J. (2018). Guidance on Verification of Operational Seasonal Forecasts. Report, WMO-No. 1220. Available online at: https://library.wmo.int/idurl/4/56227 (accessed January 20, 2024).
Mathew, G. (2022). Development of Integrated Sensor Network for Agro-meteorological Data Collection in Tanzania [Doctoral dissertation]. Arusha: NM-AIST.
Maybee, B., Ward, N., Hirons, L. C., and Marsham, J. H. (2023). Importance of Madden–Julian oscillation phase to the interannual variability of East African rainfall. Atmos. Sci. Lett. 24:e1148. doi: 10.1002/asl.1148
Mdemu, M. V. (2021). Community's vulnerability to drought-driven water scarcity and food insecurity in central and northern semi-arid areas of Tanzania. Front. Clim. 3:737655 doi: 10.3389/fclim.2021.737655
Misiani, H. O., Finney, D. L., Segele, Z. T., Marsham, J. H., Tadege, A., Artan, G., et al. (2020). Circulation patterns associated with current and future rainfall over Ethiopia and South Sudan from a convection-permitting model. Atmosphere 11:1352. doi: 10.3390/atmos11121352
Mitheu, F., Stephens, E., Petty, C., Ficchì, A., Tarnavsky, E., Cornforth, R., et al. (2023a). Impact-based Flood early warning for rural livelihoods in Uganda. Weather Clim. Soc. 15:3. doi: 10.1175/WCAS-D-22-0089.1
Mitheu, F., Tarnavsky, E., Ficchì, A., Stephens, E., Cornforth, R., Petty, C., et al. (2023b). The utility of impact data in flood forecast verification for anticipatory actions: case studies from Uganda and Kenya. J. Flood Risk Manag. e12911. doi: 10.1111/jfr3.12911
Muita, R., Kucera, P., Aura, S., Muchemi, D., Gikungu, D., Mwangi, S., et al. (2021). Towards increasing data availability for meteorological services: inter-comparison of meteorological data from a synoptic weather station and two automatic weather stations in Kenya. Am. J. Clim. Change 10, 300–316. doi: 10.4236/ajcc.2021.103014
Muriithi, G. M., Olago, D. O., Ouma, G. O., and Oriaso, S. O. (2018). Reliability of indigenous traditional knowledge and conventional weather forecastsin the face of climate change and variability in baringo county, Kenya. Int. J. Recent Sci. Res. 9, 28136–28141. doi: 10.24327/ijrsr.2018.0907.2401
Mwangi, E., Taylor, O., Todd, M. C., Visman, E., Kniveton, D., Kilavi, M., et al. (2021). Mainstreaming forecast based action into national disaster risk management systems: experience from drought risk management in Kenya. Clim. Dev. 14, 741–756. doi: 10.1080/17565529.2021.1984194
Mwangi, E., Wetterhall, F., Dutra, E., Di Giuseppe, F., and Pappenberger, F. (2014). Forecasting droughts in East Africa. Hydrol. Earth Syst. Sci. 18, 611–620. doi: 10.5194/hess-18-611-2014
Mwangi and Visman (2020). Available online at: https://reliefweb.int/sites/reliefweb.int/files/resources/Technical%20Paper_%20Drought%20forecast%20based%20action_%20The%20ForPac%20approach_final.pdf (accessed May 11, 2023).
Mwanthi, A., Salih, A., Segele, Z., and Artan, G. (2019). “Application of vortex-tracking WRF in forecasting tropical cyclones; case of tropical cyclone sagar 16-20th May 2018,” in Geophysical Research Abstracts (Vol. 21).
Mwanthi, A. M., Mutemi, J. N., Dyer, E., James, R., Opijah, F. J., Webb, T., et al. (2023). Representation of land–atmosphere coupling processes over Africa in coupled model intercomparison project Phase 6. Clim. Dyn. 1–13. doi: 10.1007/s00382-023-06710-0
Mwesigwa, A., Musoke, M. G. N., Semwanga, A. G., Sansa-Otim, J., Nabukenya, J., Basalirwa, C. P. K., et al. (2015). Report on National Survey Assessing the Status of Weather Records' Storage and Access in Selected Weather Stations in Uganda. Available online at: https://wimea-ict.net/downloads/reports/RC2-%20Weather%20Records%20Survey%20Report%20June%202015.pdf (accessed January 19, 2024).
Nicholson, S. E. (2014). A detailed look at the recent drought situation in the Greater Horn of Africa. J. Arid Environ. 103, 71–79. doi: 10.1016/j.jaridenv.2013.12.003
Nooni, I. K., Tan, G., Hongming, Y., Saidou Chaibou, A. A., Habtemicheal, B. A., Gnitou, G. T., et al. (2022). Assessing the performance of WRF Model in simulating heavy precipitation events over East Africa using satellite-based precipitation product. Remote Sens. 14:1964. doi: 10.3390/rs14091964
Nsabagwa, M., Byamukama, M., Kondela, E., and Otim, J. S. (2019). Towards a robust and affordable automatic weather station. Dev. Eng. 4:100040. doi: 10.1016/j.deveng.2018.100040
Nsabagwa, M., Byamukama, M., Otim, J. S., and Okou, R. (2016). “Network densification strategies for automatic weather stations: challenges and opportunities for Uganda,” in 2016 IST-Africa Week Conference (Durban: IEEE), 1–8. doi: 10.1109/ISTAFRICA.2016.7530633
Ntwali, D., Ogwang, B., and Ongoma, V. (2016). The impacts of topography on spatial and temporal rainfall distribution over Rwanda based on WRF model. Atmos. Clim. Sci. 6, 145–157. doi: 10.4236/acs.2016.62013
OCHA (2018). Flash Update 6: Floods in Kenya 7th June 2018. Tech. rep. UN Office for the Coordination of Humanitarian Affairs. Available online at: https://perma.cc/B47A-HSYF (accessed January 11, 2021).
Ogallo, L., Bessemoulin, P., Ceron, J. P., Mason, S. J., and Connor, S. J. (2008). Adapting to climate variability and change: the Climate Outlook Forum process. WMO Bull. 57.
Opijah, F., Mutemi, J., and Ogallo, L. (2014). Application of the EMS-WRF model in dekadal rainfall prediction over the GHA region. Afr. J. Phys. Sci. 1, 18–24.
Otieno, G., Mutemi, J. N., Opijah, F. J., Ogallo, L. A., and Omondi, M. H. (2020). The sensitivity of rainfall characteristics to cumulus parameterization schemes from a WRF model. Part I: a case study over East Africa during wet years. Pure Appl. Geophys. 177, 1095–1110. doi: 10.1007/s00024-019-02293-2
Palmer, P. I., Wainwright, C. M., Dong, B., Maidment, R. I., Wheeler, K. G., Gedney, N., et al. (2023). Drivers and impacts of Eastern African rainfall variability. Nat. Rev. Earth Environ. 4, 254–270. doi: 10.1038/s43017-023-00397-x
Parker, D. J., Blyth, A. M., Woolnough, S. J., Dougill, A. J., Bain, C. L., de Coning, E., et al. (2021). The African SWIFT project: growing science capability to bring about a revolution in weather prediction. Bull. Am. Meteorol. Soc. 103, 349–369. doi: 10.1175/BAMS-D-20-0047.1
Pathak, J., and Lúcio, F. D. F. (2018). “The global framework for climate services adaptation programme in Africa,” in Resilience (Amsterdam: Elsevier), 217–226. doi: 10.1016/B978-0-12-811891-7.00017-7
Pohl, B., Crétat, J., and Camberlin, P. (2011). Testing WRF capability in simulating the atmospheric water cycle over Equatorial East Africa. Clim. Dyn. 37, 1357–1379. doi: 10.1007/s00382-011-1024-2
Reliefweb (2019). Kenya: Floods and Landslides – Oct 2019, Tech. rep., reliefweb. Available online at: https://perma.cc/8C4Q-HTQQ (accessed January 11, 2023).
Reliefweb (2022). Available online at: https://reliefweb.int/report/ethiopia/eastern-africa-region-regional-food-security-nutrition-update-2022-first-quarter-may (accessed July 12, 2023).
Reliefweb (2023). Available online at: https://reliefweb.int/report/ethiopia/deadly-climate-induced-flooding-displaces-nearly-16-million-people-across-horn-africa (accessed December 10, 2023).
Rigby, J. M., Yohannis, M. A., Preist, C., Singer, M. B., Waema, T. M., Wausi, A. N., et al. (2022). Climate services for the Greater Horn of Africa: interviews exploring practitioner perspectives from Kenya and beyond. Clim. Dev. 15, 188–200. doi: 10.1080/17565529.2022.2074350
Roberts, A. J., Fletcher, J. K., Groves, J., Marsham, J. H., Parker, D. J., Blyth, A. M., et al. (2021a). Nowcasting for Africa: advances, potential and value. Weather 77, 250–256. doi: 10.1002/wea.3936
Roberts, R. D., Goodman, S. J., Wilson, J. W., Watkiss, P., Powell, R., Petersen, R. A., et al. (2021b). Taking the HIGHWAY to save lives on lake Victoria. Bull. Am. Meteorol. Soc. 103, 1–65. doi: 10.1175/BAMS-D-20-0290.1
Robertson, A. W., Kumar, A., Peña, M., and Vitart, F. (2015). Improving and promoting subseasonal to seasonal prediction. Bull. Am. Meteorol. Soc. 96, ES49–ES53. doi: 10.1175/BAMS-D-14-00139.1
Salih, A. A., Baraibar, M., Mwangi, K. K., and Artan, G. (2020). Climate change and locust outbreak in East Africa. Nat. Clim. Chang. 10, 584–585. doi: 10.1038/s41558-020-0835-8
Seid, J., Demissie, T., Tesfaye, K., Tamene, L., and Solomon, D. (2020). Ethiopian Digital Agroclimate Advisory Platform (EDACaP) Technical Working Document| Brief Version. Available online at: https://hdl.handle.net/10568/109663 (accessed August 5, 2023).
Seid, J., Teshome, A., and Demissie, T. (2021). “Python Climate Predictability Tool (PyCPT) training for improved seasonal climate prediction over Ethiopia,” in CCAFS Workshop Report. Addis Ababa: CGIAR Research Program on Climate Change, Agriculture and Food Security (CCAFS).
Shanko, D. (2015). Use of Automatic Weather Stations in Ethiopia. National Meteorological Agency (NMA) report. Addis Ababa.
Skamarock, W. C., Klemp, J. B., Dudhia, J., Gill, D. O., Barker, D. M., Duda, M. G., et al. (2008). A description of the advanced research WRF version 3. NCAR Tech. Note 475, 113.
Tall, A., Hansen, J., Jay, A., Campbell, B. M., Kinyangi, J., Aggarwal, P. K., et al. (2014). Scaling up Climate Services for Farmers: Mission Possible. Learning from Good Practice in Africa and South Asia. CCAFS Report. Available online at: https://hdl.handle.net/10568/42445 (accessed September 13, 2023).
Teshome, A., and Demissie, T. (2022). National Climate Outlook Forum Kiremt 2022 Impact Assessment and Bega 2022/23 Climate Impact Outlook. Workshop Report. Accelerating Impacts of CGIAR Climate Research for Africa (AICCRA). Available online at: https://hdl.handle.net/10568/126924 (accessed August 12).
Tzachor, A., Richards, C. E., Gudoshava, M., Nying'uro, P., Misiani, H., Ongoma, J. G., et al. (2023). How to reduce Africa's undue exposure to climate risks. Nature 620, 488–491. doi: 10.1038/d41586-023-02557-x
United Kingdom Met Office (2020). Available online at: https://www.gov.uk/government/news/uk-supercomputer-to-combat-africas-worst-locust-outbreak-in-decades (accessed December 15, 2023).
Vaughan, C., and Dessai, S. (2014). Climate services for society: origins, institutional arrangements, and design elements for an evaluation framework. Wiley Interdiscip. Rev. Clim. Change 5, 587–603. doi: 10.1002/wcc.290
Vellinga, M., and Milton, S. F. (2018). Drivers of interannual variability of the East A frican “Long R ains”. Q. J. R. Meteorol. Soc. 144, 861–876. doi: 10.1002/qj.3263
Visman, E., Hirons, L., Todd, M., Mwangi, E., Dione, C., Gudoshava, M., et al. (2022). Institutionalising Co-production of Weather and Climate Services: Learning From the African SWIFT and ForPAc Projects. WhiteRose. doi: 10.48785/100/99
Vitart, F., Ardilouze, C., Bonet, A., Brookshaw, A., Chen, M., Codorean, C., et al. (2017). The subseasonal to seasonal (S2S) prediction project database. Bull. Am. Meteorol. Soc. 98, 163–173. doi: 10.1175/BAMS-D-16-0017.1
Vitart, F., Robertson, A. W., and Anderson, D. L. (2012). Subseasonal to Seasonal Prediction Project: Bridging the gap between weather and climate. Bull. World Meteorol. Organ. 61, 23.
Wainwright, C. M., Finney, D. L., Kilavi, M., Black, E., and Marsham, J. H. (2021a). Extreme rainfall in East Africa, October 2019–January 2020 and context under future climate change. Weather 76, 26–31. doi: 10.1002/wea.3824
Wainwright, C. M., Marsham, J. H., Keane, R. J., Rowell, D. P., Finney, D. L., Black, E., et al. (2019). ‘Eastern African Paradox' rainfall decline due to shorter not less intense Long Rains. NPJ Clim. Atmos. Sci. 2, 34. doi: 10.1038/s41612-019-0091-7
Wainwright, C. M., Marsham, J. H., Rowell, D. P., Finney, D. L., and Black, E. (2021b). Future changes in seasonality in East Africa from regional simulations with explicit and parameterized convection. J. Clim. 34, 1367–1385. doi: 10.1175/JCLI-D-20-0450.1
Walker, D. P., Birch, C. E., Marsham, J. H., Scaife, A. A., and Graham, R. J. (2019). Skill of dynamical and GHACOF consensus seasonal forecasts of East African rainfall. Clim. Dyn. 53, 4911–4935. doi: 10.1007/s00382-019-04835-9
Waniha, P. F., Roberts, R. D., Wilson, J. W., Kijazi, A., and Katole, B. (2019). Dual-polarization radar observations of deep convection over Lake Victoria basin in East Africa. Atmosphere 10, 706. doi: 10.3390/atmos10110706
Waruru (2018). 54% of Africa's Surface Weather Stations can't Capture Data Properly. Available online at: https://www.downtoearth.org.in/news/climate-change/54-of-africa-s-surface-weather-stations-71-of-its-upper-air-weather-stations-unable-to-capture-accurate-data-60685 (accessed May 13, 2023).
White, C. J., Carlsen, H., Robertson, A. W., Klein, R. J., Lazo, J. K., Kumar, A., et al. (2017). Potential applications of subseasonal-to-seasonal (S2S) predictions. Meteorol. Appl. 24, 315–325. doi: 10.1002/met.1654
White, C. J., Domeisen, D. I., Acharya, N., Adefisan, E. A., Anderson, M. L., Aura, S., et al. (2021). Advances in the application and utility of subseasonal-to-seasonal predictions. Bull. Am. Meteorol. Soc. 1–57. doi: 10.5194/egusphere-egu22-6186
WISER (2021). Available online at: https://www.metoffice.gov.uk/binaries/content/assets/metofficegovuk/pdf/business/international/wiser/w2sip-project-summary-final.pdf (accessed December 15, 2023).
Woodhams, B. J., Birch, C. E., Marsham, J. H., Bain, C. L., Roberts, N. M., Boyd, D. F., et al. (2018). What is the added value of a convection-permitting model for forecasting extreme rainfall over tropical East Africa? Monthly Weather Rev. 146, 2757–2780. doi: 10.1175/MWR-D-17-0396.1
World Meteorological Organisation (WMO) (2020). Guidance on Operational Practices for Objective Seasonal Forecasting. Available online at: https://library.wmo.int/viewer/57090?medianame=1246_en_#page=1andviewer=pictureando=andn=0andq= (accessed February 17, 2023).
World Meteorological Organisation (WMO) (2021). WMO Guidelines on Multi-hazard Impact-based Forecast and Warning Services Part II: Putting Multi-Hazard IBFWS into Practice. Available online at: https://library.wmo.int/records/item/57739-wmo-guidelines-on-multi-hazard-impact-based-forecast-and-warning-services?language_id=13andback=andoffset (accessed July 17, 2023).
World Meteorological Organisation (WMO) (2022a). Available online at: https://wmo.int/media/news/early-warnings-all-action-plan-unveiled-cop27 (accessed December 10, 2023).
World Meteorological Organisation (WMO) (2022b). Enhancing Climate Advisories for Resilience in East Africa. Available online at: https://public.wmo.int/en/enhancing-climate-advisories-resilience-east-africa (accessed June 10, 2023).
World Meteorological Organisation WMO. (2011). Climate Knowledge for Action: A Global Framework for Climate Services and Empowering the Most Vulnerable. Available online at: https://library.wmo.int/viewer/35862?medianame=1065_en_#page=1andviewer=pictureando=downloadandn=0andq= (accessed February 5, 2023).
World Meteorological Organisation WMO. (2017). Guidelines for Nowcasting Techniques. Geneva: WMO. Available online at: https://library.wmo.int/doc_num.php?explnum_id=3795 (accessed February 10, 2023).
Keywords: Greater Horn of Africa, co-production, capacity development, observations and monitoring, forecast production, Global Framework for Climate Services (GFCS)
Citation: Gudoshava M, Otieno G, Koech E, Misiani H, Ongoma JG, Heinrich-Mertsching C, Wachana C, Endris HS, Mwanthi A, Kilavi M, Mwangi E, Colman A, Parker D, Mutemi JN, Machio P, Omay PO, Ombai P, Anande D, Kondowe A, Mugume I, Ayabagabo P, Houssein HY, Waiss MS, Abeshu B, Kayoya E, Sharawe MN, Bahaga T, Todd M, Segele Z, Atheru Z and Artan G (2024) Advances, gaps and way forward in provision of climate services over the Greater Horn of Africa. Front. Clim. 6:1307535. doi: 10.3389/fclim.2024.1307535
Received: 04 October 2023; Accepted: 12 January 2024;
Published: 30 January 2024.
Edited by:
Solomon H. Gebrechorkos, University of Oxford, United KingdomReviewed by:
Neil Hart, University of Oxford, United KingdomJoshua Ngaina, Food and Agriculture Organization of the United Nations, Italy
Copyright © 2024 Gudoshava, Otieno, Koech, Misiani, Ongoma, Heinrich-Mertsching, Wachana, Endris, Mwanthi, Kilavi, Mwangi, Colman, Parker, Mutemi, Machio, Omay, Ombai, Anande, Kondowe, Mugume, Ayabagabo, Houssein, Waiss, Abeshu, Kayoya, Sharawe, Bahaga, Todd, Segele, Atheru and Artan. This is an open-access article distributed under the terms of the Creative Commons Attribution License (CC BY). The use, distribution or reproduction in other forums is permitted, provided the original author(s) and the copyright owner(s) are credited and that the original publication in this journal is cited, in accordance with accepted academic practice. No use, distribution or reproduction is permitted which does not comply with these terms.
*Correspondence: Masilin Gudoshava, masilin.gudoshava@igad.int