- 1Remote Sensing and Assimilation, Institute of Oceanography, Universität Hamburg, CEN - Centrum für Erdsystemforschung und Nachhaltigkeit, Hamburg, Germany
- 2Met Office, Exeter, United Kingdom
- 3German Climate Computing Centre (DKRZ), Hamburg, Germany
- 4EPOC - Environnements et Paléoenvironnements Océaniques et Continentaux, Institut polytechnique de Bordeaux, Université de Bordeaux, CNRS - Centre national de la recherche scientifique, Pessac, France
- 5Max Planck Institute for Meteorology, Hamburg, Germany
- 6Geophysical Institute, University of Bergen, Bjerknes Centre for Climate Research, Bergen, Norway
- 7National Oceanic and Atmospheric Administration - NOAA/Geophysical Fluid Dynamics Laboratory, Princeton, NJ, United States
- 8University Corporation for Atmospheric Research, Boulder, CO, United States
- 9Centro Euro-Mediterraneo sui Cambiamenti Climatici, Bologna, Italy
- 10Rossby Centre, Swedish Meteorological and Hydrological Institute, Norrköping, Sweden
- 11Deutscher Wetterdienst, Offenbach, Germany
- 12The National Key Laboratory of Numerical Modeling for Atmospheric Sciences and Geophysical Fluid Dynamics, Institute of Atmospheric Physics, Chinese Academy of Sciences, Beijing, China
- 13Barcelona Supercomputing Center, Barcelona, Spain
- 14National Centre for Climate Research, Danish Meteorological Institute, Copenhagen, Denmark
- 15Canadian Centre for Climate Modelling and Analysis, Environment and Climate Change Canada, Victoria, BC, Canada
- 16Japan Agency for Marine-Earth Science and Technology, Kanagawa, Japan
- 17Atmosphere and Ocean Research Institute, University of Tokyo, Chiba, Japan
- 18Meteorological Research Institute, Japan Meteorological Agency, Ibaraki, Japan
- 19CSIRO Environment, Hobart, TAS, Australia
Due to large northward heat transport, the Atlantic meridional overturning circulation (AMOC) strongly affects the climate of various regions. Its internal variability has been shown to be predictable decades ahead within climate models, providing the hope that synchronizing ocean circulation with observations can improve decadal predictions, notably of the North Atlantic subpolar gyre (SPG). Climate predictions require a starting point which is a reconstruction of the past climate. This is usually performed with data assimilation methods that blend available observations and climate model states together. There is no unique method to derive the initial conditions. Moreover, this can be performed using full-field observations or their anomalies superimposed on the model's climatology to avoid strong drifts in predictions. How critical ocean circulation drifts are for prediction skill has not been assessed yet. We analyze this possible connection using the dataset of 12 decadal prediction systems from the World Meteorological Organization Lead Centre for Annual-to-Decadal Climate Prediction. We find a variety of initial AMOC errors within the predictions related to a dynamically imbalanced ocean states leading to strongly displaced or multiple maxima in the overturning structures. This likely results in a blend of what is known as model drift and initial shock. We identify that the AMOC initialization influences the quality of the SPG predictions. When predictions show a large initial error in their AMOC, they usually have low skill for predicting internal variability of the SPG for a time horizon of 6-10 years. Full-field initialized predictions with low AMOC drift show better SPG skill than those with a large AMOC drift. Nevertheless, while the anomaly-initialized predictions do not experience large drifts, they show low SPG skill when skill also present in historical runs is removed using a residual correlation metric. Thus, reducing initial shock and model biases for the ocean circulation in prediction systems might help to improve their prediction for the SPG beyond 5 years. Climate predictions could also benefit from quality-check procedure for assimilation/initialization because currently the research groups only reveal the problems in initialization once the set of predictions has been completed, which is an expensive effort.
1 Introduction
The Atlantic meridional overturning circulation (AMOC) transports a large amount of heat to the North Atlantic, affecting climate in the Euro-Atlantic sector. Total poleward heat transport constitutes approximately 0.4–0.6 PW (zonally accumulated heat transport over the full water column starting from the Greenland coast toward Scotland; Böning et al., 1996; Zhao et al., 2018). Previous studies have shown that the AMOC and its slow oscillation exhibit strong predictability when perturbing its initial conditions (Collins et al., 2006), and as such, is believed to be a source of predictability in decadal climate predictions (DCPs). For instance, the warming of the North Atlantic subpolar gyre (SPG) in the late 1990s has been attributed to the increased northward heat transport due to a strong AMOC (Robson et al., 2012; Williams et al., 2014; Yeager and Danabasoglu, 2014) and the subsequent cooling in the 2000s associated with the AMOC slow down (Hermanson et al., 2014). Severe winter conditions in 2010 and 2011 over northwestern Europe were associated with the interannual variability of the AMOC (Bryden et al., 2014). Understanding predictability of the AMOC under climate-change conditions is of great interest for narrowing down the uncertainty in climate-change projections (Swingedouw et al., 2022). Earlier studies of potential predictability suggest that the AMOC is predictable up to two decades ahead or even longer under certain conditions (Griffies and Bryan, 1997; Collins and Sinha, 2003; Pohlmann et al., 2004). More recent DCP studies, which compare predictions with the reconstructions of the recent past climate from the data assimilation products, show a different range of predictability for different models from 4 to 10 years (Matei et al., 2012; Persechino et al., 2013; Swingedouw et al., 2013; Polkova et al., 2014; Mignot et al., 2016; Yang et al., 2021). Due to the lack of long enough basin-wide and deep-ocean observational data, the actual prediction skill for the AMOC is difficult to assess. The best assessment that we can currently perform is against the data assimilation products, e.g., ocean reanalyses, but they poorly agree with each other (Karspeck et al., 2015; Jackson et al., 2019).
DCPs aim to provide the most accurate predictions of the near-term climate. In addition to the external forcing, they utilize the knowledge of the observed climate state. The components of the Earth System Models that are usually synchronized with the recently observed climate state are the ocean and the atmosphere (Meehl et al., 2014; Boer et al., 2016). Mostly, the studies are interested in predicting user-relevant climate indices for their further application in climate services (Marotzke et al., 2016; Smith et al., 2019; Solaraju-Murali et al., 2022). Several studies that have assessed the prediction skill for the AMOC from the DCPs reported some issues in the initialization of the AMOC (Smith et al., 2013; Kröger et al., 2017; Bilbao et al., 2021). For instance, Kröger et al. (2017) showed that errors generated during the full-field nudging procedure applied to the MPI-ESM led to artificial ocean heat transport (OHT) in the interior of the ocean. This, in turn, had an impact on the AMOC and led to large errors in surface temperature predictions in the North Atlantic region. Bilbao et al. (2021) showed that in the full-field initialized EC-Earth3 predictions, the SPG has poor skill due to an initialization shock which led to the collapse of the Labrador Sea convection and a rapid decline in the AMOC. So far, it has not been studied if AMOC initialization errors occur in other prediction systems and if they have an effect on the predictability of other climate variables as shown by Kröger et al. (2017). Moreover, there is not any study that has leveraged the multi-model ensemble to make general conclusions about the impact of initialization shock like our study aims to do. In terms of the terminology, it is worth mentioning that, theoretically, there is a distinction between initialization shock that results from an imperfect initialization method and model drift that occurs due to the existence of biases and is independent of initialization. Practically, these two types of errors are difficult to separate from each other (Mulholland et al., 2015), and sometimes the terms are used interchangeably. As we analyze the DCPs, we are interested to learn about both types of errors but in particular about those related to initialization that we hopefully can fix with more skillful initialization procedures.
Here, we examine DCPs contributing to the annually-issued World Meteorological Organization's (WMO's) Global Annual to Decadal Climate Update (Hermanson et al., 2022; www.wmolc-adcp.org). To study the effects of initialization shocks, both predictions and their initial conditions (produced with data-constrained assimilation runs) are needed. The assimilation runs are often considered as an intermediate step to produce initial conditions and usually do not receive attention in publications as much as the ocean or atmosphere reanalyses do. The quality of the assimilation runs is thus usually indirectly confirmed by the prediction skill of the DCPs. However, carrying out a full set of retrospective and future DCPs is an expensive effort (one set of hindcasts accounts for about 6,000 model years), and thus, it would be useful to identify possible initialization issues at earlier stages, which might lead to reduction of predictions' quality. Specifically, we analyze AMOC initial shocks resulting from the initialization and data assimilation procedures. The North Atlantic is the region where initialization is reported to bring most of the improvement in DCPs (Boer et al., 2013; Meehl et al., 2014). In particular, the subpolar gyre exhibits longer skill than other regions of the ocean in terms of surface temperature and ocean heat content (Polkova et al., 2019). The SPG is an important region for the deep water formation (Rhein et al., 2011). Thus, we aim to identify possible links between the AMOC initialization aspects and the prediction skill at the ocean interface in the region of the North Atlantic subpolar gyre.
2 Materials and methods
The multi-model ensemble forecasts are a part of the operational decadal predictions hosted by the WMO and coordinated by the Met Office (Hermanson et al., 2022). The decadal predictions are produced by the WMO-designated Global Producing Centres and other contributing centers. The decadal predictions include DCPP-A experiments (Decadal Climate Prediction Project Component A; Boer et al., 2016) of the CMIP6 (Climate Model Intercomparison Project Phase 6). From this data set, we analyze near-surface air temperature and the AMOC stream function. In addition to the decadal predictions, the CMIP6 historical (uninitialized) simulations for near-surface air temperature from the same prediction systems are used to distinguish the impact of external forcing from that of initialization.
The DCPs were produced with the following prediction systems: MPI-ESM-LR, MIROC6, GFDL SPEAR, CanCM4, CAFE, FGOALS-f3-L, MRI-ESM2, NorCPM1, BSC EC-Earth3, CMCC-CM2-SR5, SMHI+DMI EC-Earth3, and HadGEM3-GC31-MM (hereafter DePreSys4). Except for CanCM4 which contributed to CMIP5, all other prediction systems are contribution to CMIP6. The DCPs are ensembles of 10-year simulations, except of those from MRI-ESM2 that represent 5-year simulations. Retrospective predictions are initialized every year. The starting month differs across the prediction systems (October, November or January). We analyze the prediction skill over the common initialization period for the retrospective DCPs (hindcast period) that is 1961–2018. Further details on DCPs are presented in Table 1 and under the System Configuration Information for Global Producing Centers at www.wmolc-adcp.org.
We analyze the effect of AMOC initialization shock on the prediction skill of the North Atlantic subpolar gyre (SPG). The SPG index is calculated as the average of the near-surface temperature anomalies (w.r.t. to climatology over the period 1991–2020) over the region 45–67°N and 60–0°W (Robson et al., 2018). The choice of the climatology period follows the WMO recommendations (https://community.wmo.int/en/wmo-climatological-normals). ERA5 (Hersbach et al., 2020) and RAPID (Bryden et al., 2014) are used as the reference (observational) datasets. We apply the anomaly correlation coefficient, root mean square error, and residual correlation (Smith et al., 2019) as the verification metrics. For residual correlation, residual predicted and observed time-series are generated. For this, the uninitialized ensemble mean is removed via linear regression from the predicted and observed time-series, respectively (see for details Smith et al., 2019). The residuals represent the variability that cannot be captured by the uninitialized simulations and their correlation represents the impact of initialization. To evaluate residual correlation for the SPG index, we use the historical simulations that are available for the following models: MPI-ESM-LR (10 members), MIROC6 (33 members), GFDL SPEAR (1 member), CanCM4 (9 members), MRI-ESM2 (10 members), BSC EC-Earth3 (14 members), CMCC-CM2-SR5 (1 member), DePreSys4 (4 members), NorCPM1 (30 members), and FGOALS-f3-L (3 members). Two models, BSC EC-Earth3 and SMHI+DMI EC-Earth3, share the same historical simulations. For all the former models, in the diagnostics of residual correlation, we removed in the DCPs the external forcing signals estimated from the historical simulations stemming from the same model. For CAFE, since we do not have the corresponding historical simulations, we used the 115 members multi-model ensemble mean of the historical runs from the available models.
3 Results
3.1 Prediction skill for the SPG
We analyze predictions of the SPG index from the 12 DCP experiments (Figure 1). Overall, most DCPs predict the observed warming in the 1990s and cooling in the 2010s. Some models struggle with predicting the warming anomalies in the recent observed decade (e.g., GFDL SPEAR and NorCPM1). In addition, in the early initialization period of the 1960s–1980s, half of the prediction systems show lower SPG temperature anomalies than the ERA5 atmospheric reanalysis suggests (i.e., GFDL SPEAR, MRI-ESM2, BSC EC-Earth3, CMCC-CM2-SR5, SMHI+DMI EC-Earth3, and DePreSys4). This might have a relation to rather sparse observational records for temperature and salinity profiles that are used for initialization for that period (de Boisséson et al., 2018). Interestingly, GFDL SPEAR which only implements ocean surface initialization also shows lower SPG temperature anomalies than ERA5. Another reason could be that the DCPs overestimate warming trend. Since the climatological period for bias-correction is 1991–2020, DCPs with a too large trend would appear colder in the earlier period.
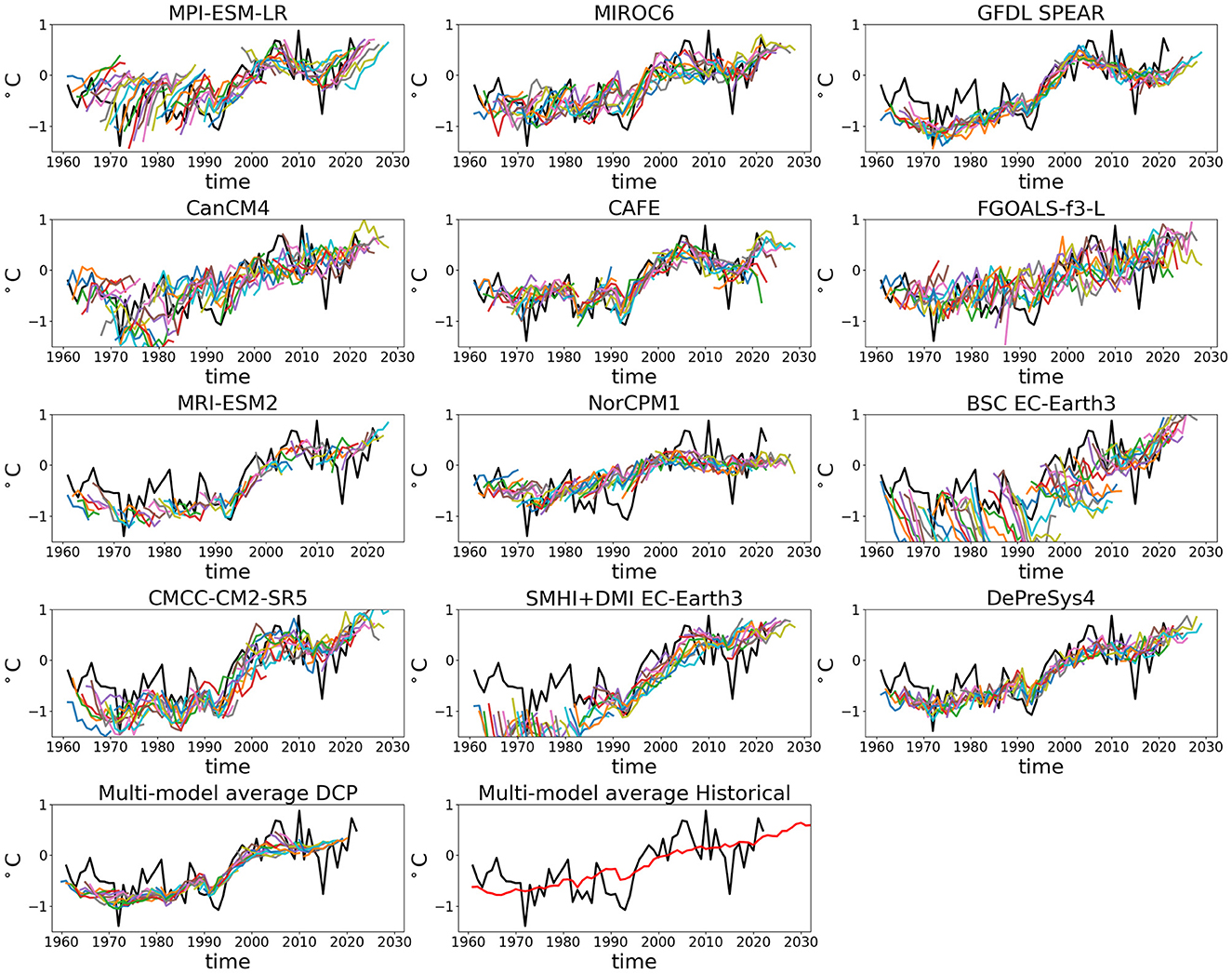
Figure 1. The time-series for the North Atlantic SPG index from ERA5 (black, all panels), the ensemble mean of the historical simulations (in red, lower panel), and the WMO initialized decadal predictions for different starting dates (in color, corresponding panel). The ensemble mean of the multi-model ensemble of all DCPs is shown in multi-color in the lower panel. The SPG index is calculated as the average of the near-surface temperature anomalies over the region 45–67°N and 60–0°W. The lead-time dependent bias (calculated with respect to 1991–2020) is removed from the hindcasts' time-series of the SPG index.
The prediction skill for the SPG index is shown in Figure 2. The models MPI-ESM-LR and CanCM4, as well as FGOALS-f3-L, show reduced SPG skill in terms of correlation, especially for the second pentad (Figure 2A). A few other models such as BSC EC-Earth3 and SMHI+DMI EC-Earth3 show enhanced root mean square errors (Figure 2B), possibly due to errors that have been associated with a non-stationary expression of the forecast drift (Bilbao et al., 2021). Overall, correlation and root mean square error for other models are consistent with each other and support long-lasting predictability for the SPG. The prediction skill from the multi-model DCP experiments, some of which are also used in our analysis, has been previously reported by Hegerl et al. (2021) for the subpolar gyre and by Delgado-Torres et al. (2022) and Kim et al. (2012) for the Atlantic Multidecadal Variability index. Similar to the results in our study, Hegerl et al. (2021) and Delgado-Torres et al. (2022) suggest low SPG skill for MPI-ESM-LR and Kim et al. (2012) suggest low Atlantic Multidecadal Variability skill for CanCM4.
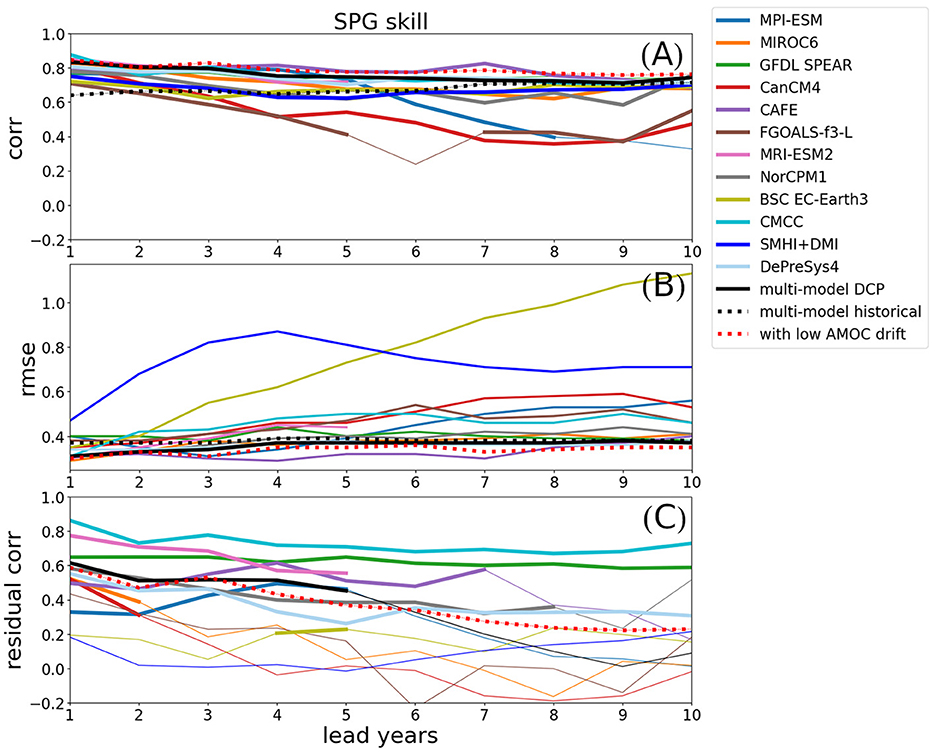
Figure 2. Prediction skill for the SPG index in terms of the anomaly correlation coefficient (corr; A), root mean square error (rmse in °C; B) and the residual correlation (residual corr; C). The skill is calculated over the period 1961–2018. The skill for the multi-model average of DCPs is in solid black and for the multi-model average of DCPs based on the four models, the choice for which will be explained at the end of Section 3 is in dashed red. The skill for the multi-model average of historical simulations is in dashed black. For correlation, the significant values are estimated with the t-test (p < 0.05; Smith et al., 2019) and significant correlation values in (A, C) are shown in bold lines.
The prediction skill for the SPG might be dominated by the externally forced signal (Borchert et al., 2021). Therefore, to assess the impact of initialization, we analyze the residual correlation (Figure 2C). The analysis of the residual correlation reveals a larger spread among DCPs from different models than that of the total correlation (Figure 2A); more DCP experiments from various models fall below the multi-model average of DCPs (Figure 2C). Overall, the residual correlation tends to be not statistically significant for the DCPs that show low skill either in terms of the root mean square error (Figure 2B) or anomaly correlation (Figure 2A).
3.2 AMOC after initialization
To analyze the prediction skill of the SPG index linked with the AMOC initialization, the initial conditions from the assimilation runs are needed. Due to the absence of these runs in the WMO dataset, we use the respective first month of each DCP as a proxy for the initial state and further examine decadal predictions from the first few months to few years after the initialization. The target here is to look for abrupt changes indicative of the potential shock from initial conditions when the system is released from observational constraints. The overturning cells in different models in the first month after initialization exhibit large differences (Figure 3). Due to different initialization months (Table 1), the AMOC cells from different models for the first lead month cannot be directly compared with each other. The analysis of the timeseries of the AMOC leadtime-dependent climatology at 25°N and 40°N shows that AMOC experiences drift of 1 to 12 Sv depending on the model and latitude (Figure 4 and Supplementary Figures 1, 2). The AMOC drift is approximately linear initially and, for some DCPs, it saturates after several years (e.g., MPI-ESM-LR, CMCC-CM2-SR5, and NorCPM1; Figure 4 and Supplementary Figures 1, 2). The AMOC drift is also not stationary, i.e., in some models, it is larger in the earlier initialization period (e.g., BSC EC-Earth3 and CAFE; Supplementary Figures 1–4) and in other models in the more recent initialization period (e.g., MPI-ESM-LR and GFDL SPEAR; Supplementary Figures 1–4).
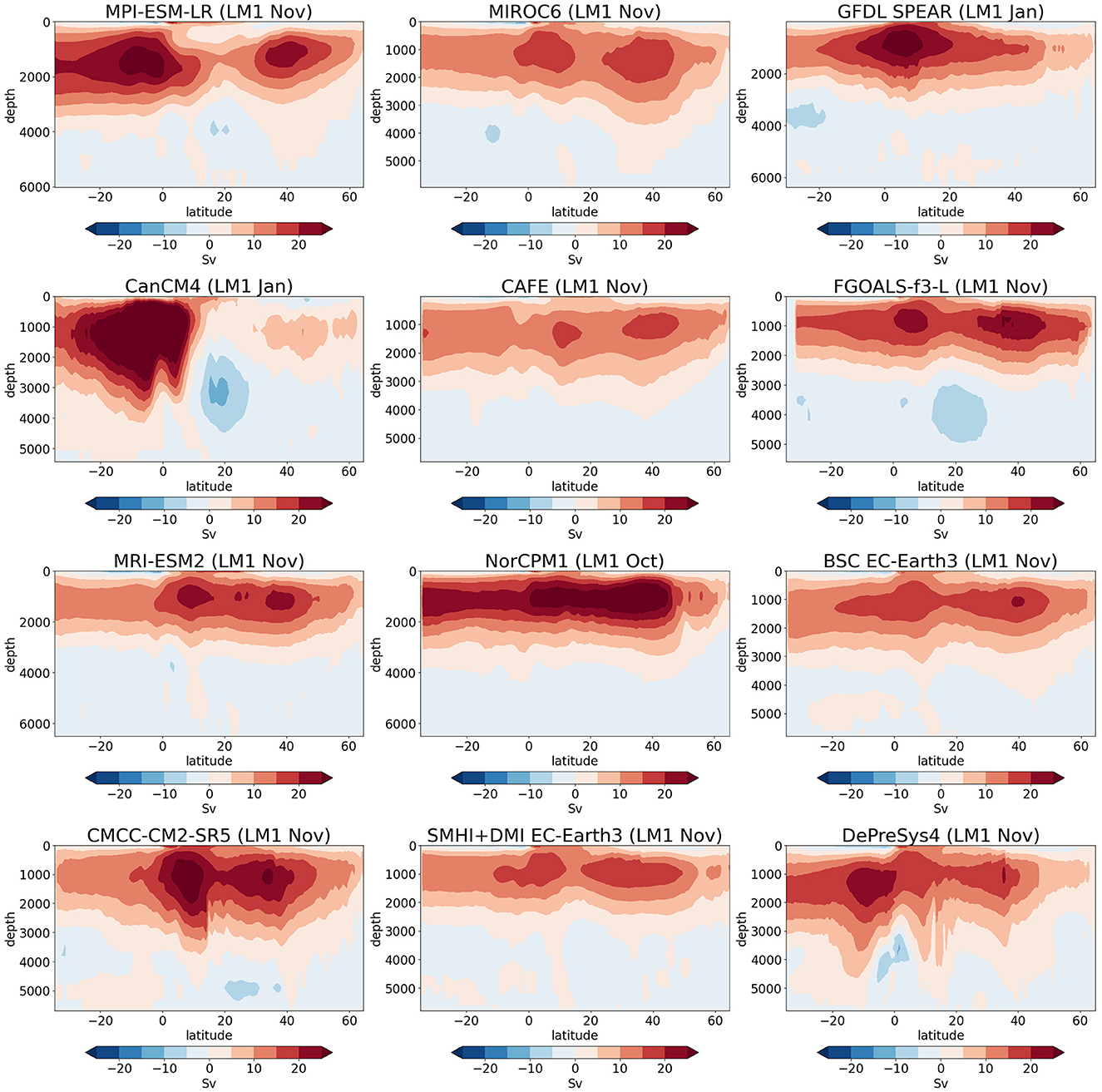
Figure 3. The AMOC cells at the first lead month after initialization (LM1) averaged over the period 1991–2020. Notably, the initialization month is different among the prediction systems: October, November, or January (see Table 1).
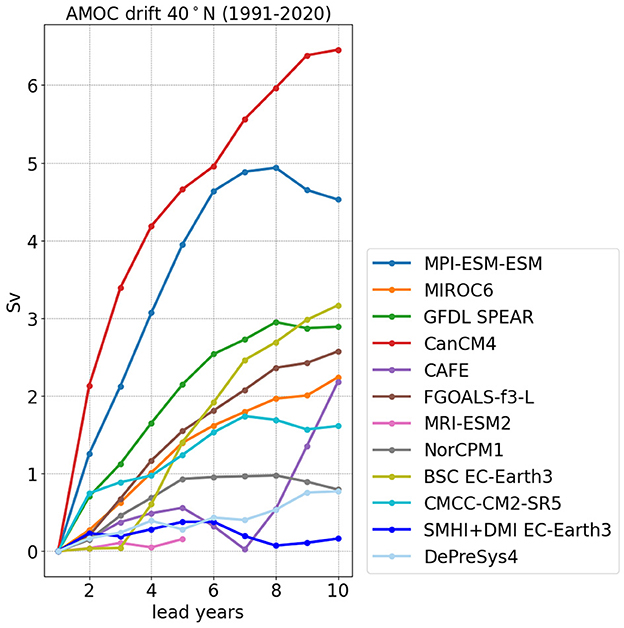
Figure 4. AMOC drift for the period 1991–2020 at 40°N and 1,000 m depth calculated as absolute difference between AMOC climatology at each lead year and AMOC climatology at the 1st lead year.
Comparing the initial AMOC cells of each DCP with that of at later lead years suggests a distorted cell structure in some of the DCPs at the initialization step (e.g., MPI-ESM-LR and CanCM4; Supplementary Figures 5–7). During integration, the upper AMOC cell in DCPs “recovers” presumably to the preferable model's state; however, depending on the severity of the initialization shock, this process might take different times in various DCPs. To visualize the AMOC drift for the whole Atlantic basin, we fit the linear regression to the leadtime-dependent climatology of AMOC (Figure 5). The climatology is calculated over the period 1991–2020, which is used to diagnose the bias for the bias-correction procedure in DCPs. If the AMOC drift is associated with the ocean heat transport toward or from the SPG gyre, it could affect the variability of the SPG, especially in the later lead years (2nd pentad) as previous studies showed that changes in the AMOC in northern high latitudes lead temperature anomaly in the subpolar gyre region by several years (Zhang and Zhang, 2015; Borchert et al., 2018). The AMOC was shown to affect the skill of the SPG in one of the early development stages of the decadal prediction system based on MPI-ESM-LR (Marotzke et al., 2016; Kröger et al., 2017, with the ocean initialization based on full-field nudging). Similarly, Robson et al. (2012) showed for the earlier version of DePreSys that ocean heat transport changes are responsible for the predictability of the SPG in the 1990s and that successful AMOC initialization was essential for skilful temperature predictions. Thus, in the following, we analyze the AMOC drift in more details and contrast it against the SPG skill in the multi-model ensemble.
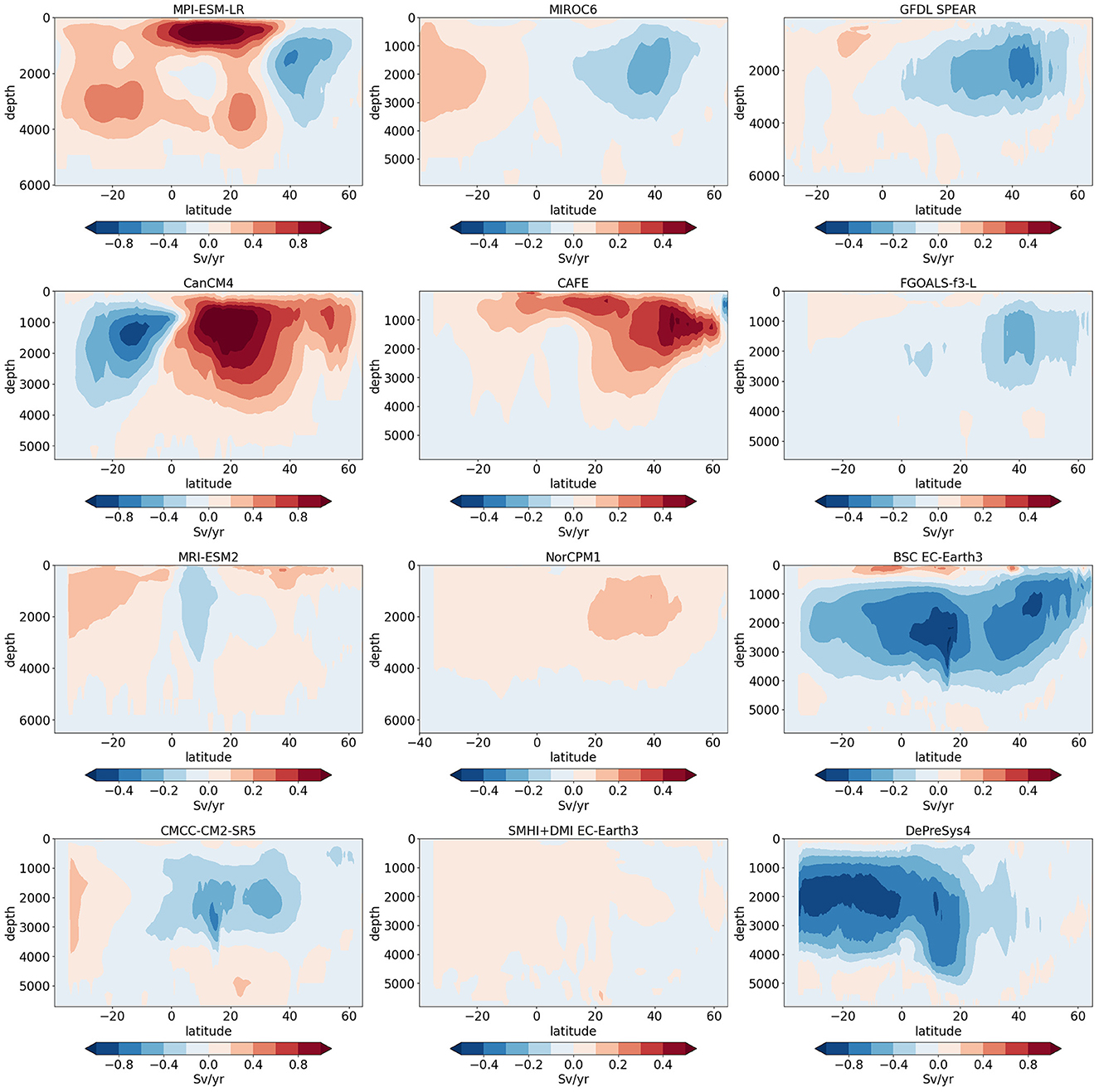
Figure 5. AMOC drift (Sv/year) calculated in terms of the slope of the linear regression fitted into 10 lead years of the leadtime-dependent climatology of AMOC, except of MRI-ESM2, for which the fit is performed over 5 lead years. The climatology is calculated over the period 1991–2020. The panels have a different range of the colorbar.
Several models show large trends in the overturning cells, pointing to the regions where models attempt to fix the AMOC structure after the initialization shock (Figure 5). For example, in CanCM4, there is more overturning in the northern hemisphere and less in the southern one. In MPI-ESM-LR, there is more overturning in 0-20°N, where there is a split of the upper overturning cell at the initialization step (Figure 3). These trends originate from initialization as the models in non-initialized experiments do not show such features; (e.g., for CanCM4 and MPI-ESM-LR; Yang and Saenko, 2012; Brune and Baehr, 2020). The DCPs with the largest drift are full-field initialized (Figure 5). Further analysis of the errors in AMOC from different DCPs are presented in Supplementary Figures 1–9. For example, comparing the AMOC cells among different models in the first month after initialization shows multiple maxima in several models, e.g., for MPI-ESM-LR and DePreSys4. However, without observing the AMOC cells from historical simulations for all models, it is difficult to assess how unusual the AMOC mean state is just after initialization and, if the AMOC in later lead years, represents the AMOC of the model attractor or if it would continue to drift further (beyond 10 lead years).
Overall, several models stand out in terms of both reduced prediction skill for the SPG in the later lead years (Figure 2) and overturning cell features (such as multiple maxima of the mean AMOC cell, AMOC drift, or AMOC root mean square error w.r.t. RAPID): MPI-ESM-LR, CanCM4, FGOALS-f3-L, BSC EC-Earth3, and SMHI+DMI EC-Earth3. A possible link between the drop of the prediction skill in the full-field initialized DCPs for their SPG surface temperatures could be the drift in the meridional ocean heat transport (OHT) induced by the assimilation procedure as reported by Kröger et al. (2017). Anomaly initialized predictions can also experience drifts, although somewhat smaller, when the anomalies, from which predictions are initialized, are not compatible with the simulated variability range. In fact, from Figures 4, 5 and Supplementary Figures 1, 2, it is evident that the anomaly-initialized DCPs also experience AMOC drift (MIROC6, FGOALS-f3-L and NorCPM1). Following the hypothesis of OHT being driven by the AMOC (Zhang and Zhang, 2015), Borchert et al. (2018) showed that in the North Atlantic region, the AMOC and the OHT are highly correlated and that OHT changes at 50°N lead to changes in the North Atlantic sea surface temperature by up to 9 years. To investigate the AMOC fingerprint, they identified the range of latitudes for the propagation of AMOC anomalies stretching between 40°N and 50°N.
3.3 Relationship between the AMOC drift and the SPG skill
Thus, as shown in Figure 6A, we contrast the SPG residual correlation for the second pentad of the DCPs (lead years 6–10) and the AMOC drift associated with initialization in the range of latitudes 40–50°N and depth 0–5,000 m. The mean AMOC drift in the larger latitudinal band 20–60°N vs. SPG residual skill is shown in Supplementary Figure 10. We also contrast the SPG skill and the AMOC root mean square error estimated with respect to the RAPID data (Figure 6B). When contrasting the AMOC drift and the SPG residual skill, three models fall into the region of low SPG residual skill and high AMOC drift: CanCM4, BSC EC-Earth3, and MPI-ESM-LR. All three models use full-field initialization in the ocean. The other four models with low SPG residual skill show small drift in the region: among them, MIROC6, SMHI+DMI EC-Earth3, FGOALS-f3-L, and NorCPM1. For the latter, the SPG residual skill is statistically significant. They are anomaly initialized in the ocean. The rest of the models are full-field initialized and show small drift at 40–50°N as well as high and significant residual correlation. This suggests that the strong AMOC drift that originates from initialization can affect the evolution of the SPG in the later lead years. However, this does not seem to be the only reason why some models lack predictability due to internal variability in the absence of the large drift. For the anomaly initialized models, this might be poor representation of the observed anomalies, with the large biases being an indicator of poor initialization. Due to the absence of long-enough observational AMOC data in the range of latitudes 40–50°N, we cannot estimate the relation between the AMOC skill in higher latitudes and the SPG skill. Instead we analyzed AMOC root mean square error at 25°N, as shown in Figure 6B. The root mean square error does not only focus on the initial shock but is a general measure of prediction errors. Similar to Figure 6A, it also points at the models CanCM4 and MPI-ESM-LR with large-scale ocean circulation errors which echo in the SPG skill. Figure 6B also highlights NorCPM1 that has a very large bias for the AMOC.
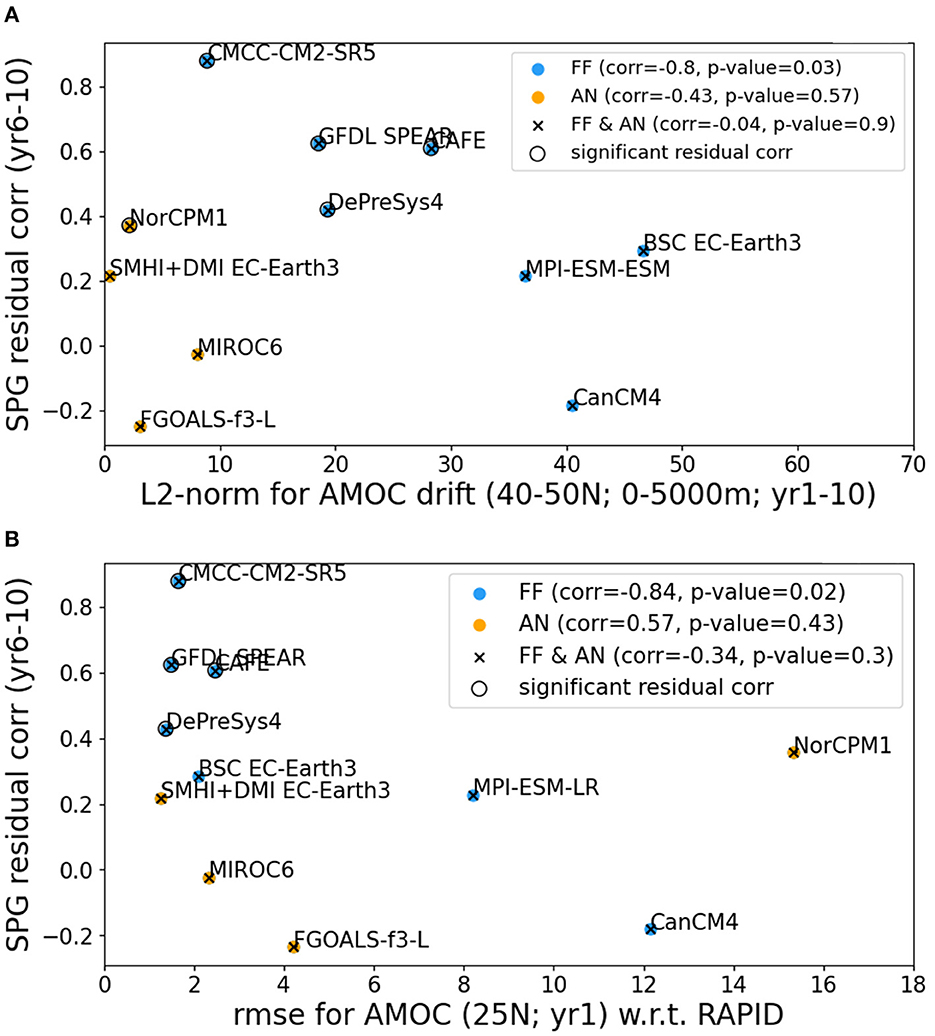
Figure 6. SPG residual skill for lead years 6–10 vs. AMOC drift (A) and vs. AMOC rmse (Sv) w.r.t. RAPID data at the first lead year (B). The significant SPG residual skill (in black circles) is estimated with the t-test, p < 0.05 (Smith et al., 2019). The AMOC drift is diagnosed as the slope of the linear regression fitted to 10 lead years of the leadtime-dependent AMOC climatology. Climatology is estimated for the period 1991–2020. Then, L2-norm of the AMOC drift is calculated for the region 40–50°N and 0–5,000 m depth as the sum of the squared AMOC drift values in each grid cell. Orange markers signify anomaly initialized DCPs, blue markers full-field initialized.
For the full-field initialized DCPs that fall into the category of low AMOC drift (CAFE, CMCC-CM2-SR5, DePreSys4, and GFDL SPEAR), we recalculated the prediction skill based on the ensemble mean constructed from these models. The subsellected multi-model mean beats the prediction skill of the full multi-model ensemble mean, especially in the last pentad of the decadal prediction years (shown in red dash in Figure 2).
Previous studies suggest the importance of SPG-AMOC coupling in the models (Sun et al., 2021). In this respect, Yang et al. (2021) found that GFDL SPEAR's predicted SPG temperatures at three different lead times (including 6–10 lead years) are highly correlated with the corresponding initial values of AMOC with correlations larger than 0.75. The initialization that updates the ocean surface and the atmosphere states with observations without breaking up this coupling could explain high skill for the SPG in this model. We analyzed correlation between AMOC at 40°N and 1,000 m depth for lead year 1 and SPG temperatures for lead years 6–10 from the WMO ensemble of DCPs. From four DCP systems (with low AMOC drift and high SPG residual skill), CMCC-CM2-SR5, GFDL SPEAR, and CAFE indeed show statistically significant correlation between the time-series of the initial AMOC at 40°N and the SPG index at later lead years (Supplementary Figure 11). This does not necessarily mean that other models, when not initialized, do not have this relationship. However, lacking or out of phase relationship particularly in the prediction mode represents yet another indicator of initialization issues.
4 Summary and discussion
While the research centers assimilate ocean and atmosphere observations from the same data, they use different models and different assimilation/initialization methods. Ocean circulation reacts sensitively to these different choices so that the initial ocean state varies across different DCPs. In our study, we dealt with the question: why do the decadal prediction systems start from so different ocean circulations but the prediction skill at the ocean surface is similar and hardly improves the skill over the historical simulations (Borchert et al., 2021)? Our results show that this may appear so at first glance because the skill at the air–sea interface is largely dominated by the externally forced response. The picture starts changing when we analyze the skill due to internal variability such that DCPs that appear to have smaller errors in initial Atlantic meridional overturning circulation have a better total skill for the subpolar gyre than a multi-model average of DCPs. Below, we summarize several key findings and recommendations for future studies:
• Multi-model and single-model predictions (e.g., CAFE and CMCC-CM2-SR5) of the SPG index are skilful on decadal time scales. Evaluations of residual variability obtained from linear regression against historical simulations suggest that the prediction skill of most of the DCPs is largely explained by the forced response, while tentative initialization of internal variability provide additional skill in the later lead years only in a few prediction systems (Figure 2). We identified systematic behavior of the initialized predictions in the 1960–80s, where most of the DCPs underestimated or overestimated temperature in the SPG region. Excluding the 1960–80s period from the analysis improved the prediction skill of some models (Supplementary Figure 12). This result calls for improving the reconstruction runs that provide the initial conditions for DCPs over 1960–80s or at least keeping in mind the possible poor performance of the DCPs in this verification period.
• The analysis of the lead-time dependent climatology of multiple DCP systems indicates individual initialization errors in the AMOC, e.g., multiple maxima in MPI-ESM-LR, strong AMOC drifts in MPI-ESM-LR, CanCM4 and BSC EC-Earth3, and large AMOC biases in NorCPM1. DCPs that initially have strong AMOC drift at 40–50°N also have generally low residual skill in the SPG for the 2nd pentad of the prediction period. The analysis indicates that the mechanism affecting the skill reduction is not local and instantaneous but rather remotely driven and lagged, presumably via changes in the ocean heat transport.
The study retains several open questions, and we would like to encourage the scientific community to contribute to the investigations since very multi-model analysis of initial shock is quite scarce, while this might constitute a crucial issue for decadal prediction systems as highlighted here. For instance, the effect of the drift is expected to be removed by the lead-time dependent bias correction. However, we observe that the lead-time dependent bias-correction does not seem to completely debias the AMOC predictions (Supplementary Figures 4, 5), which might also explain their low skill for the SPG index. On the other hand, if initialization/assimilation could indeed introduce artificial flows in the dynamically sensitive regions as suggested by Kröger et al. (2017), they might also evolve the observed anomaly contained in the initial conditions, modifying potential internal variability and creating main signal that is a drift. It thus appears necessary to further analyze the exact mechanism by which the AMOC drift and other errors could affect the SPG skill in DCPs.
The mechanism proposed by Borchert et al. (2018) about strong OHT leading to high SPG skill could be considered to understand better the relation between strong AMOC and OHT drifts and the SPG skill. For instance, models with weak AMOC mean state might be prone to having low prediction skill. Moreover, too low or too high AMOC variability could also have a negative impact on the prediction skill because the response to AMOC variability will not be appropriate. Further analysis of the assimilation runs and ocean heat content budget as in the study by Kröger et al. (2017) might be useful to trace origins of the initialization shock of the ocean circulation. We thus encourage the research groups that will contribute to future DCP and CMIP experiments along with DCPs to also plan providing initial conditions (assimilation runs) to better assess the impact of initialization shock. In addition, we encourage more institutes to contribute to WMO decadal prediction project, in order to increase the sampling for multi-model analysis, which will also improve the robustness of the results from this international initiative.
The analysis of AMOC initial shock in depth space is recommended for future studies to gain more insight into the problem from the point of view of the water mass transformation across isopycnals. The AMOC indices in depth and density space are equivalent as long as the isopycnals are relatively flat across the basin, which is the case in the tropical and subtropical regions but not in the subpolar region (50–60°N; Xu et al., 2014). In our current analysis, we relate the skill of the SPG with the heat transport between 40 and 50°N, where the relation between the two AMOC indices is still rather high.
AMOC is one of the diagnostics for the assimilation runs. In the attempt to answer the question of how good should the AMOC be in the assimilation runs, it appears reasonable to aim at representing a realistic vertical structure of the AMOC. It is necessary to further evaluate the strength and variability of AMOC on obtaining the right impact on the SPG and density dominance by temperature or salinity (Menary and Hermanson, 2018). Our study shows that DCPs with low SPG skill also have AMOC errors (strong and non-stationary drifts). In this study, we could not contrast the AMOC residual skill against the SPG residual skill mostly because the WMO dataset lacked the respective historical simulations for AMOC. The AMOC root mean square error w.r.t. RAPID points at the DCPs with both drifts and biases, suggesting value for continuing the RAPID as well as OSNAP (Overturning in the Subpolar North Atlantic Program) observing periods, to guide and improve the tuning of the underlying climate models, and the production of more realistic assimilation runs to provide initial conditions. For the 3D AMOC view, it remains difficult to judge what the good or realistic structure of the AMOC should be in each model. In this respect, the ocean reanalyses remain our best estimate so far.
Data availability statement
The data analyzed in this study is subject to the following licenses/restrictions: information about decadal predictions from the WMO dataset is available at www.wmolc-adcp.org. For access to the forecast data, send your request to d21vbGMtYWRjcCYjeDAwMDQwO21ldG9mZmljZS5nb3YudWs=. The RAPID data is available from https://rapid.ac.uk/challenge/data_download.php and the ERA5 from https://cds.climate.copernicus.eu/cdsapp#!/dataset/reanalysis-era5-single-levels?tab=form.
Author contributions
IP: Conceptualization, Formal analysis, Funding acquisition, Investigation, Project administration, Visualization, Writing – original draft. DSw: Conceptualization, Funding acquisition, Investigation, Methodology, Writing – review & editing. LH: Conceptualization, Data curation, Funding acquisition, Investigation, Methodology, Writing – review & editing. AK: Funding acquisition, Investigation, Methodology, Writing – review & editing. DSt: Funding acquisition, Investigation, Methodology, Writing – review & editing. DSm: Funding acquisition, Investigation, Methodology, Writing – review & editing. IB: Investigation, Methodology, Writing – review & editing. XY: Investigation, Methodology, Writing – review & editing. LZ: Investigation, Methodology, Writing – review & editing. DN: Investigation, Methodology, Writing – review & editing. PA: Investigation, Methodology, Writing – review & editing. MK: Investigation, Methodology, Writing – review & editing. KP: Investigation, Methodology, Writing – review & editing. HP: Investigation, Methodology, Writing – review & editing. BW: Investigation, Methodology, Writing – review & editing. RB: Investigation, Methodology, Writing – review & editing. PO: Investigation, Methodology, Writing – review & editing. SY: Investigation, Methodology, Writing – review & editing. RS-A: Investigation, Methodology, Writing – review & editing. WM: Investigation, Methodology, Writing – review & editing. TK: Investigation, Methodology, Writing – review & editing. HT: Investigation, Methodology, Writing – review & editing. YI: Investigation, Methodology, Writing – review & editing. MI: Investigation, Methodology, Writing – review & editing. RM: Investigation, Methodology, Writing – review & editing. JK: Investigation, Methodology, Writing – review & editing.
Funding
The author(s) declare financial support was received for the research, authorship, and/or publication of this article. Funding for IP was provided by the Deutsche Forschungsgemeinschaft, Project number 436413914. LH and DSm were supported by the Met Office Hadley Centre Climate Programme funded by BEIS and Defra. IB was supported by the Trond Mohn Foundation (Grant BFS2018TMT01; Bjerknes Climate Prediction Unit) and the Research Council of Norway (Grant 309562; Climate Futures). PA, DN, HP, and MK acknowledge funding from the European Union's Horizon Europe Research and Innovation Programme through the ASPECT project under grant agreement No 101081460. DSw received financial support from the French government in the framework of the University of Bordeaux's IdEx Investments for the Future program / RRI Tackling Global Change. DSw also acknowledges funding from UKRI Decadal project (MR/W009641/1). RM would like to acknowledge funding support from CSIRO DCFP (Decadal Climate Forecasting Project). HT and TK were supported by MEXT program for the advanced studies of climate change projection (SENTAN) Grant Number JPMXD0722680395.
Acknowledgments
The authors would like to thank the research centers for providing DCPs and the Met Office and the DKRZ for providing resources for our analysis. The authors also would like to thank two reviewers for providing helpful comments to improve our manuscript.
Conflict of interest
The authors declare that the research was conducted in the absence of any commercial or financial relationships that could be construed as a potential conflict of interest.
The author(s) declared that they were an editorial board member of Frontiers, at the time of submission. This had no impact on the peer review process and the final decision.
Publisher's note
All claims expressed in this article are solely those of the authors and do not necessarily represent those of their affiliated organizations, or those of the publisher, the editors and the reviewers. Any product that may be evaluated in this article, or claim that may be made by its manufacturer, is not guaranteed or endorsed by the publisher.
Supplementary material
The Supplementary Material for this article can be found online at: https://www.frontiersin.org/articles/10.3389/fclim.2023.1273770/full#supplementary-material
References
Bethke, I., Wang, Y., Counillon, F., Keenlyside, N., Kimmritz, M., Fransner, F., et al. (2021). NorCPM1 and its contribution to CMIP6 DCPP. Geosci. Model Dev. 14, 7073–7116. doi: 10.5194/gmd-14-7073-2021
Bilbao, R., Wild, S., Ortega, P., Acosta-Navarro, J., Arsouze, T., Bretonnière, P.-A., et al. (2021). Assessment of a full-field initialized decadal climate prediction system with the CMIP6 version of EC-Earth. Earth Syst. Dyn. 12, 173–196. doi: 10.5194/esd-12-173-2021
Boer, G., Kharin, V., and Merryfield, W. (2013). Decadal predictability and forecast skill. Clim. Dyn. 41, 1817–1833. doi: 10.1007/s00382-013-1705-0
Boer, G., Smith, D. M., Cassou, C., Doblas-Reyes, F., Danabasoglu, G., Kirtman, B., et al. (2016). The decadal climate prediction project (DCPP) contribution to CMIP6. Geosci. Model Dev. 9, 3751. doi: 10.5194/gmd-9-3751-2016
Böning, C. W., Bryan, F. O., Holland, W. R., and Döscher, R. (1996). Deep-water formation and meridional overturning in a high-resolution model of the North Atlantic. J. Phys. Oceanogr. 26, 1142–1164.
Borchert, L. F., Menary, M. B., Swingedouw, D., Sgubin, G., Hermanson, L., and Mignot, J. (2021). Improved decadal predictions of North Atlantic subpolar gyre SST in CMIP6. Geophys. Res. Lett. 48, e2020GL091307. doi: 10.1029/2020GL091307
Borchert, L. F., Müller, W. A., and Baehr, J. (2018). Atlantic ocean heat transport influences interannual-to-decadal surface temperature predictability in the North Atlantic region. J. Clim. 31, 6763–6782. doi: 10.1175/JCLI-D-17-0734.1
Brune, S., and Baehr, J. (2020). Preserving the coupled atmosphere–ocean feedback in initializations of decadal climate predictions. Wiley Interdiscipl. Rev. Clim. Change 11, e637. doi: 10.1002/wcc.637
Bryden, H., King, B. A., McCarthy, G. D., and McDonagh, E. (2014). Impact of a 30% reduction in Atlantic meridional overturning during 2009–2010. Ocean Sci. 10, 683–691. doi: 10.5194/os-10-683-2014
Collins, M., Botzet, M., Carril, A., Drange, H., Jouzeau, A., Latif, M., et al. (2006). Interannual to decadal climate predictability in the North Atlantic: a multimodel-ensemble study. J. Clim. 19, 1195–1203. doi: 10.1175/JCLI3654.1
Collins, M., and Sinha, B. (2003). Predictability of decadal variations in the thermohaline circulation and climate. Geophys. Res. Lett. 30, 1306. doi: 10.1029/2002GL016504
de Boisséson, E., Balmaseda, M. A., and Mayer, M. (2018). Ocean heat content variability in an ensemble of twentieth century ocean reanalyses. Clim. Dyn. 50, 3783–3798. doi: 10.1007/s00382-017-3845-0
Delgado-Torres, C., Donat, M. G., Gonzalez-Reviriego, N., Caron, L.-P., Athanasiadis, P. J., Bretonnière, P.-A., et al. (2022). Multi-model forecast quality assessment of CMIP6 decadal predictions. J. Clim. 35, 4363–4382. doi: 10.1175/JCLI-D-21-0811.1
Griffies, S. M., and Bryan, K. (1997). Predictability of North Atlantic multidecadal climate variability. Science 275, 181–184.
Hegerl, G. C., Ballinger, A. P., Booth, B. B., Borchert, L. F., Brunner, L., Donat, M. G., et al. (2021). Toward consistent observational constraints in climate predictions and projections. Front. Clim. 3, 678109. doi: 10.3389/fclim.2021.678109
Hermanson, L., Eade, R., Robinson, N. H., Dunstone, N. J., Andrews, M. B., Knight, J. R., et al. (2014). Forecast cooling of the Atlantic subpolar gyre and associated impacts. Geophys. Res. Lett. 41, 5167–5174. doi: 10.1002/2014GL060420
Hermanson, L., Smith, D., Seabrook, M., Bilbao, R., Doblas-Reyes, F., Tourigny, E., et al. (2022). WMO global annual to decadal climate update: a prediction for 2021–25. Bull. Am. Meteorol. Soc. 103, E1117–E1129. doi: 10.1175/BAMS-D-20-0311.1
Hersbach, H., Bell, B., Berrisford, P., Hirahara, S., Horányi, A., Muñoz-Sabater, J., et al. (2020). The ERA5 global reanalysis. Q. J. R. Meteorol. Soc. 146, 1999–2049. doi: 10.1002/qj.3803
Hövel, L., Brune, S., and Baehr, J. (2022). Decadal prediction of marine heatwaves in MPI-ESM. Geophys. Res. Lett. 49, e2022GL099347. doi: 10.1029/2022GL099347
Jackson, L., Dubois, C., Forget, G., Haines, K., Harrison, M., Iovino, D., et al. (2019). The mean state and variability of the North Atlantic circulation: a perspective from ocean reanalyses. J. Geophys. Res. Oceans 124, 9141–9170. doi: 10.1029/2019JC015210
Karspeck, A., Stammer, D., Köhl, A., Danabasoglu, G., Balmaseda, M., Smith, D., et al. (2015). Comparison of the Atlantic meridional overturning circulation between 1960 and 2007 in six ocean reanalysis products. Clim. Dyn. 49, 957–982. doi: 10.1007/s00382-015-2787-7
Kataoka, T., Tatebe, H., Koyama, H., Mochizuki, T., Ogochi, K., Naoe, H., et al. (2020). Seasonal to decadal predictions with MIROC6: Description and basic evaluation. J. Adv. Model. Earth Syst. 12, e2019MS002035. doi: 10.1029/2019MS002035
Kim, H.-M., Webster, P. J., and Curry, J. A. (2012). Evaluation of short-term climate change prediction in multi-model CMIP5 decadal hindcasts. Geophys. Res. Lett. 39, L10701. doi: 10.1029/2012GL051644
Kröger, J., Pohlmann, H., Sienz, F., Marotzke, J., Baehr, J., Köhl, A., et al. (2017). Full-field initialized decadal predictions with the MPI Earth System Model: an initial shock in the North Atlantic. Clim. Dyn. 51, 2593–2603. doi: 10.1007/s00382-017-4030-1
Marotzke, J., Müller, W. A., Vamborg, F. S., Becker, P., Cubasch, U., Feldmann, H., et al. (2016). MiKlip-a national research project on decadal climate prediction. Bull. Am. Meteorol. Soc. 97, 2379–2394. doi: 10.1175/BAMS-D-15-00184.1
Matei, D., Baehr, J., Jungclaus, J. H., Haak, H., Müller, W. A., and Marotzke, J. (2012). Multiyear prediction of monthly mean Atlantic meridional overturning circulation at 26.5 N. Science 335, 76–79. doi: 10.1126/science.1210299
Meehl, G. A., Goddard, L., Boer, G., Burgman, R., Branstator, G., Cassou, C., et al. (2014). Decadal climate prediction: an update from the trenches. Bull. Am. Meteorol. Soc. 95, 243–267. doi: 10.1175/BAMS-D-12-00241.1
Menary, M. B., and Hermanson, L. (2018). Limits on determining the skill of North Atlantic Ocean decadal predictions. Nat. Commun. 9, 1694. doi: 10.1038/s41467-018-04043-9
Merryfield, W. J., Lee, W.-S., Boer, G. J., Kharin, V. V., Scinocca, J. F., Flato, G. M., et al. (2013). The Canadian seasonal to interannual prediction system. Part I: Models and initialization. Monthly Weather Rev. 141, 2910–2945. doi: 10.1175/MWR-D-12-00216.1
Mignot, J., García-Serrano, J., Swingedouw, D., Germe, A., Nguyen, S., Ortega, P., et al. (2016). Decadal prediction skill in the ocean with surface nudging in the IPSL-CM5A-LR climate model. Clim. Dyn. 47, 1225–1246. doi: 10.1007/s00382-015-2898-1
Mulholland, D. P., Laloyaux, P., Haines, K., and Balmaseda, M. A. (2015). Origin and impact of initialization shocks in coupled atmosphere–ocean forecasts. Monthly Weather Rev. 143, 4631–4644. doi: 10.1175/MWR-D-15-0076.1
Nicolì, D., Bellucci, A., Ruggieri, P., Athanasiadis, P. J., Materia, S., Peano, D., et al. (2023). The Euro-Mediterranean Center on Climate Change (CMCC) decadal prediction system. Geosci. Model Dev. 16, 179–197. doi: 10.5194/gmd-16-179-2023
Persechino, A., Mignot, J., Swingedouw, D., Labetoulle, S., and Guilyardi, E. (2013). Decadal predictability of the Atlantic meridional overturning circulation and climate in the IPSL-CM5A-LR model. Clim. Dyn. 40, 2359–2380. doi: 10.1007/s00382-012-1466-1
Pohlmann, H., Botzet, M., Latif, M., Roesch, A., Wild, M., and Tschuck, P. (2004). Estimating the decadal predictability of a coupled AOGCM. J. Clim. 17, 4463–4472. doi: 10.1175/3209.1
Polkova, I., Brune, S., Kadow, C., Romanova, V., Gollan, G., Baehr, J., et al. (2019). Initialization and ensemble generation for decadal climate predictions: a comparison of different methods. J. Adv. Model. Earth Syst. 11, 149–172. doi: 10.1029/2018MS001439
Polkova, I., Köhl, A., and Stammer, D. (2014). Impact of initialization procedures on the predictive skill of a coupled ocean-atmosphere model. Clim. Dyn. 42, 3151–3169. doi: 10.1007/s00382-013-1969-4
Rhein, M., Kieke, D., Hüttl-Kabus, S., Roessler, A., Mertens, C., Meissner, R., et al. (2011). Deep water formation, the subpolar gyre, and the meridional overturning circulation in the subpolar North Atlantic. Deep Sea Res. II Top. Stud. Oceanogr. 58, 1819–1832. doi: 10.1016/j.dsr2.2010.10.061
Robson, J., Sutton, R., Lohmann, K., Smith, D., and Palmer, M. D. (2012). Causes of the rapid warming of the North Atlantic Ocean in the mid-1990s. J. Clim. 25, 4116–4134. doi: 10.1175/JCLI-D-11-00443.1
Robson, J., Sutton, R. T., Archibald, A., Cooper, F., Christensen, M., Gray, L. J., et al. (2018). Recent multivariate changes in the North Atlantic climate system, with a focus on 2005–2016. Int. J. Climatol. 38, 5050–5076. doi: 10.1002/joc.5815
Sandery, P. A., O'Kane, T. J., Kitsios, V., and Sakov, P. (2020). Climate model state estimation using variants of EnKF coupled data assimilation. Monthly Weather Rev. 148, 2411–2431. doi: 10.1175/MWR-D-18-0443.1
Smith, D. M., Eade, R., and Pohlmann, H. (2013). A comparison of full-field and anomaly initialization for seasonal to decadal climate prediction. Clim. Dyn. 41, 3325–3338. doi: 10.1007/s00382-013-1683-2
Smith, D. M., Eade, R., Scaife, A., Caron, L.-P., Danabasoglu, G., DelSole, T., et al. (2019). Robust skill of decadal climate predictions. NPJ Clim. Atmos. Sci. 2, 13. doi: 10.1038/s41612-019-0071-y
Solaraju-Murali, B., Bojovic, D., Gonzalez-Reviriego, N., Nicodemou, A., Terrado, M., Caron, L.-P., et al. (2022). How decadal predictions entered the climate services arena: an example from the agriculture sector. Clim. Serv. 27, 100303. doi: 10.1016/j.cliser.2022.100303
Sun, J., Latif, M., and Park, W. (2021). Subpolar gyre–AMOC–atmosphere interactions on multidecadal timescales in a version of the kiel climate model. J. Clim. 34, 6583–6602. doi: 10.1175/JCLI-D-20-0725.1
Swingedouw, D., Houssais, M.-N., Herbaut, C., Blaizot, A.-C., Devilliers, M., and Deshayes, J. (2022). AMOC recent and future trends: a crucial role for oceanic resolution and Greenland melting? Front. Clim. 4, 838310. doi: 10.3389/fclim.2022.838310
Swingedouw, D., Mignot, J., Labetoulle, S., Guilyardi, E., and Madec, G. (2013). Initialisation and predictability of the AMOC over the last 50 years in a climate model. Clim. Dyn. 40, 2381–2399. doi: 10.1007/s00382-012-1516-8
Tian, T., Yang, S., Karami, M. P., Massonnet, F., Kruschke, T., and Koenigk, T. (2021). Benefits of sea ice initialization for the interannual-to-decadal climate prediction skill in the Arctic in EC-Earth3. Geosci. Model Dev. 14, 4283–4305. doi: 10.5194/gmd-14-4283-2021
Williams, R. G., Roussenov, V., Smith, D., and Lozier, M. S. (2014). Decadal evolution of ocean thermal anomalies in the North Atlantic: the effects of Ekman, overturning, and horizontal transport. J. Clim. 27, 698–719. doi: 10.1175/JCLI-D-12-00234.1
Wu, B., Zhou, T., and Zheng, F. (2018). EnOI-IAU initialization scheme designed for decadal climate prediction system IAP-DecPreS. J. Adv. Model. Earth Syst. 10, 342–356. doi: 10.1002/2017MS001132
Xu, X., Chassignet, E. P., Johns, W. E., Schmitz, W. J. Jr., and Metzger, E. J. (2014). Intraseasonal to interannual variability of the atlantic meridional overturning circulation from eddy-resolving simulations and observations. J. Geophys. Res. Oceans 119, 5140–5159. doi: 10.1002/2014JC009994
Yang, D., and Saenko, O. A. (2012). Ocean heat transport and its projected change in canESM2. J. Clim. 25, 8148–8163. doi: 10.1175/JCLI-D-11-00715.1
Yang, X., Delworth, T. L., Zeng, F., Zhang, L., Cooke, W. F., Harrison, M. J., et al. (2021). On the Development of GFDL's decadal prediction system: initialization approaches and retrospective forecast assessment. J. Adv. Model. Earth Syst. 13, e2021MS002529. doi: 10.1029/2021MS002529
Yeager, S., and Danabasoglu, G. (2014). The origins of late-twentieth-century variations in the large-scale North Atlantic circulation. J. Clim. 27, 3222–3247. doi: 10.1175/JCLI-D-13-00125.1
Yukimoto, S., Kawai, H., Koshiro, T., Oshima, N., Yoshida, K., Urakawa, S., et al. (2019). The Meteorological Research Institute Earth System Model version 2.0, MRI-ESM2. 0: description and basic evaluation of the physical component. J. Meteorol. Soc. Japan Ser. II 97, 931–965. doi: 10.2151/jmsj.2019-051
Zhang, J., and Zhang, R. (2015). On the evolution of Atlantic meridional overturning circulation fingerprint and implications for decadal predictability in the North Atlantic. Geophys. Res. Lett. 42, 5419–5426. doi: 10.1002/2015GL064596
Keywords: Atlantic meridional overturning circulation, subpolar gyre, decadal predictions, prediction skill, initialization shock, initial conditions, data assimilation, internal variability
Citation: Polkova I, Swingedouw D, Hermanson L, Köhl A, Stammer D, Smith D, Kröger J, Bethke I, Yang X, Zhang L, Nicolì D, Athanasiadis PJ, Karami MP, Pankatz K, Pohlmann H, Wu B, Bilbao R, Ortega P, Yang S, Sospedra-Alfonso R, Merryfield W, Kataoka T, Tatebe H, Imada Y, Ishii M and Matear RJ (2023) Initialization shock in the ocean circulation reduces skill in decadal predictions of the North Atlantic subpolar gyre. Front. Clim. 5:1273770. doi: 10.3389/fclim.2023.1273770
Received: 07 August 2023; Accepted: 27 October 2023;
Published: 04 December 2023.
Edited by:
Shaoqing Zhang, Ocean University of China, ChinaReviewed by:
Neven Fuckar, University of St Andrews, United KingdomXiangzhou Song, Hohai University, China
Copyright © 2023 Polkova, Swingedouw, Hermanson, Köhl, Stammer, Smith, Kröger, Bethke, Yang, Zhang, Nicolì, Athanasiadis, Karami, Pankatz, Pohlmann, Wu, Bilbao, Ortega, Yang, Sospedra-Alfonso, Merryfield, Kataoka, Tatebe, Imada, Ishii and Matear. This is an open-access article distributed under the terms of the Creative Commons Attribution License (CC BY). The use, distribution or reproduction in other forums is permitted, provided the original author(s) and the copyright owner(s) are credited and that the original publication in this journal is cited, in accordance with accepted academic practice. No use, distribution or reproduction is permitted which does not comply with these terms.
*Correspondence: Iuliia Polkova, aXVsaWlhLnBvbGtvdmEmI3gwMDA0MDt1bmktaGFtYnVyZy5kZQ==; cG9sa292YSYjeDAwMDQwO2RrcnouZGU=