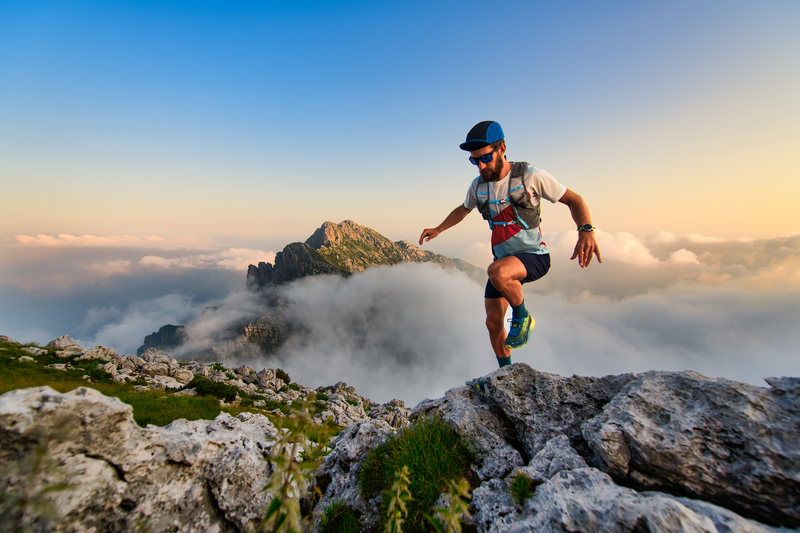
95% of researchers rate our articles as excellent or good
Learn more about the work of our research integrity team to safeguard the quality of each article we publish.
Find out more
ORIGINAL RESEARCH article
Front. Clim. , 14 July 2023
Sec. Climate and Decision Making
Volume 5 - 2023 | https://doi.org/10.3389/fclim.2023.1146402
This article is part of the Research Topic Decision Making for the Net Zero Transformation: Considerations and New Methodological Approaches View all 11 articles
In response to a growing awareness of the potential impact of climate change on financial stability, academics, financial institutions (FIs), central banks and supervisors (CB&S) have developed a suite of scenarios and analytical tools to assess forward-looking climate-related financial risks, inform macro-prudential policies, counterparty risk management and business planning decisions. Climate scenario analysis brings new challenges vs. traditional scenario analysis by FIs, particularly given the limitations, uncertainties, and trade-offs inherent in the data, models, and methods for such financial risk assessments. We argue that all scenarios are wrong, but this does not necessarily mean that they cannot be useful if used and expanded upon with full awareness of the limitations. In this paper, we analyze those limitations in the context of the specific requirements by FIs for scenario analysis and propose an approach to scenario construction and expansion to complement existing scenarios and increase their suitability for decision making for key financial use cases. Importantly, we argue that current scenarios are likely closer to the lower end of the range of plausible future risk for both physical and transition risk. This has implications for both stress testing and risk management, and business planning. We advocate for harnessing the full breadth of scenario narratives to avoid the accumulation of systemic risks and our framework provides an initial step toward this. Finally, we call for FIs, CB&S, and research institutions to work closely together to develop a more comprehensive scenario taxonomy to help navigate the implications of material financial risk under uncertainty.
JEL codes: Q51, Q43, G21, G32.
Climate scenario analysis and stress testing are widely recognized as valuable tools for private and public financial institutions (FIs), central banks and supervisors (CB&S) to assess the opportunities and risks presented by physical climate change and the transition to a low carbon economy (among others, see ACPR, 2020; CFRF, 2020; NGFS, 2020; Bank of England, 2021; Baer et al., 2022). Consequently, in recent years, these actors have developed a suite of scenarios to inform the assessment of forward-looking climate-related financial risks to inform sector-level macro-prudential policies, counterparty-level risk management and business planning decisions.
Scenario analyses are commonly used by FIs to inform risk management and business planning decisions. Multiple plausible hypotheses for the future are set up to examine the effects of a wide range of risk drivers across scenarios (BIS, 2021). This represents a what-if scenario approach that can derive conditional estimates under a given hypothesis, rather than aiming to model and predict future expected risk impacts. Traditional financial risk management, and in particular stress tests, are usually backward-looking in the form of designing tail-risk scenarios based on historical volatilities of macroeconomic and financial market data. Here, a “stress” situation is created as an exogenous shock to the system with a given likelihood of occurrence to test the resilience of FIs and to determine capital requirements (BIS, 2009). Such a scenario analysis is used to test financial institutions' portfolios prominently after the great financial crisis (GFC) and was also expanded to inform macroprudential supervision to assess financial system resilience (ESRB, 2020).
Climate scenario analysis is different to traditional approaches in several ways and this introduces new challenges. Firstly, the past is not a guide to the future and the transition and extreme climate physical events manifest through unprecedented changes. As a result, FIs and CB&S cannot derive a probability distribution from past shocks and market volatility. The risks that the transition entail are also more complex than classical financial risk, as beyond just financial system complexity, it involves socioeconomic and ecological feedbacks and unprecedented structural changes across economies which are hard to capture and may be overlooked by FIs or not yet appropriately priced in (Bolton and Kacperczyk, 2021; Eren et al., 2022). Capturing such complexity is inherently difficult and exacerbated due to increased difficulty to communicate between areas of scientific research, such as modelers of IAMs, climate scientists and financial economists (Fiedler et al., 2021). Finally, regulatory exercises are pushing FIs to consider longer timescales and so are pushed in the realm of even deeper uncertainties. But also, for CB&S that are starting to explore shorter term time horizons for regulatory setting, elevated uncertainty represents a risk appetite challenge for micro and macroprudential regulators, as acknowledged by the Bank of England's report on climate-related risks and the regulatory capital frameworks.1 Assessing forward-looking climate-related risks and opportunities hence represents a unique challenge vis-a-vis classic financial risk management.
Nevertheless, the scale of the climate challenge that lies ahead, political developments and increased scrutiny from regulators have now confronted FIs and CB&S to move away from standard risk management practices to build capabilities around innovative approaches and solutions that can capture the unprecedented shocks, interdependencies, and longer time horizons associated with climate-related financial risk (Annex 1, for example, includes a summary of the SS3-19 supervisory statement (UK), drawing out the implications for scenario analyses).
To date, organizations like the Network of Central Banks and Supervisors for Greening the Financial System (NGFS) have played a central role in developing the scenarios that many FIs are beginning to use. Other commonly used scenarios for climate risk assessment include those of the Intergovernmental Panel on Climate Change (IPCC) and the International Energy Agency (IEA).2 As a result, so-called integrated assessment models (IAMs)—augmented with macroeconomic models—have become the core tool used to produce climate-adjusted economic pathways.3 Scenarios are created by augmenting IAMs and macro models that have been proven effectively in modeling economic relationships and trends based on a variety of socio-economic assumptions. However, such an augmentation means that these models are less straight forward to interpret and increased complexity may not necessarily be transparently conveyed outside of the scientific community. Further, this may have resulted in a bias to generate climate scenarios in an integrated way that is not necessarily adequate to capture the shock-based logic used for certain financial risk use cases. Yet, available scenarios remain too simplistic to capture the true complexity of climate change and the net-zero transition. Critically, IAMs have not yet allowed to represent frictions, tipping-points and amplification dynamics beyond the smooth changes in trends that are reflected based on socio-economic and climatic optimization paths (Stern et al., 2021).
It is important to recognize that current IPCC and IAM-based scenarios were fundamentally not built for financial scenario analysis; they were built to inform climate policy. They were designed to explore the implications of different policy decisions, but not to stress test. Generally, it has been argued that there is a growing disconnect between specific scenario features and the requirements of private and public financial institutions (FIs) to inform financial decisions (Fiedler et al., 2021; Koberle et al., 2021; CGFI, 2022; Pitman et al., 2022). The needs of financial institutions around risk management are vastly different to those of policy makers for scenarios that identify the mix of actions required to reduce greenhouse gas emissions in line with temperature and emissions targets (Koberle et al., 2021). Further, many financial institutions lack the expertise to fully understand the modeling choices and assumptions underpinning these scenarios. Together this may lead to a misuse of scenarios and potentially a systematic underestimation of the risk associated with the transition. It may also lead firms to take insufficient action to manage their risks caused either by the uncertainties or a false perception of the risks.
FIs therefore face a dilemma. Given that past historic data is not good predictor for the low-carbon transition, classical financial approaches with a shock-based logic to represent tail-risk with a certain likelihood of occurrence were mostly dismissed in exploratory climate stress testing exercises (BoE, 2019; ECB, 2021). But the alternatives also have deficiencies. More generally, we observe an overarching challenge of climate scenario analysis to balance the applicability of scenarios with the required representation of complexities needed by the financial sector in the face of the unprecedented risk, urgency of the transition and planetary boundaries (Rockström et al., 2009; Chenet et al., 2019; BIS, 2020). This dilemma—if not handled appropriately—could lead to negative outcomes and the build-up of systemic risk.
The recent report by the Financial Stability Board (FSB) and the NGFS acknowledged the challenges, stating for example, that while continuous progress is made, current exercises may understate climate exposures and vulnerabilities, especially in capturing tail risks for risk management and stress testing purposes (FSB and NGFS, 2022). Assessing and managing climate risk is a new and ongoing process, with substantial learning and refinement happening continually. The NGFS is to-date on its third iteration of scenarios and with each release comes substantial refinement and improvement.4 Similarly, the Bank of England's CBES results stressed the learning nature of the exercise (Ranger et al., 2023). Yet, as reflected by recent supervisory statements (e.g., Annex 1), it is critical that firms get started in strengthening their capability in climate scenario analysis and begin to build climate dimensions into risk management and business planning. Yet, firms that responded to the PRA's consultation paper indicated that scenario analysis is one of the most challenging aspects of meeting supervisory expectations (CFRF, 2020). Further, regulatory divergence on supervisory expectations makes it difficult for firms to understand the status of emerging best practices5 or assess how they are developing against peers (Ranger et al., 2023).
In this paper, we aim to provide practitioners with guidance on how to use and augment current scenarios to aid decision making and improve risk management practices. In modeling, there is a common phrase “all models are wrong, but some are useful.” In this case, we argue that while all scenarios have limitations, this does not necessarily mean that they cannot be useful if used and expanded upon properly, in full awareness of their characteristics and inherent trade-offs. We explain those limitations in the context of the specific requirements by FIs for scenario analysis and propose an approach to complement existing scenarios and increase their suitability for decision making for key financial use cases. In other words, we first identify the needs of financial institutions and where the gaps and limitation are, and then propose some preliminary solutions, while highlighting potential for future work to address the identified gaps.
More explicitly, in the first section, we draw upon exisiting evidence and practices to provide insights into key scenario features, model characteristics that underpin scenarios, and wider scenario considerations that are important to equip financial institutions with the required knowledge on how to effectively select, use and interpret currently available scenarios. We highlight that insufficient understanding of these dimensions and associated deficiencies of currently available scenarios may lead to a misuse of scenarios and a systematic underestimation of the risk associated with the transition.
In section two, we then present a practical framework for FIs to assess and select appropriate scenarios and augment them where necessary through scenario expansion and scenario construction. It guides the user to identify potential gaps in the available range of scenarios and how to construct more disruptive, decision-relevant scenarios through an adjustment of key assumptions that allow to explore different sensitivities and better capture the range of possible pathways on how the transition may unfold. This aims to complement previous work by UNEP FI that provided a suite of short-term climate-related shock scenarios.6 We take a deep dive on three key areas to demonstrate how the framework can be used in practice: first, we show how current geopolitical developments and impacts on the energy sector may provide insights into the plausibility and likelihood of scenario assumptions. We draw out the importance to consider the possibility of a short-term disorderly transition within the energy markets if the phase out of high-emission activities is not carefully managed in parallel with the ramp-up of low emission ones. Second, we demonstrate for the Agriculture, Forest, and Other Land Use (AFOLU) sector, how an augmentation of scenario assumptions for a variety of more pessimistic mitigation potentials of emerging and developing countries could be undertaken and how this may interact with global decarbonization efforts. Third, we show how an augmentation of scenario components in the energy sector impact the financial risk outcome in climate scenario analysis, drawing upon evidence on varying levels of conservativeness around technological innovation. This is aimed to aid the user in placing where relevant scenario components sit in the probability distribution7 of the assumed impact and in terms of credibility of materialization.
In general, the framework8 aims to help balance the increasing pressure for financial firms to use scenario analysis in their business decision making processes today while capturing the full breadth of scenario narratives and granularity required by the financial sector. Finally, we call for FIs, central banks and research institutions to work more closely together to develop a more comprehensive scenario taxonomy to help navigate material financial risk under uncertainty.
The paper is structured as follows. Section 2 discusses the key obstacles to the effective use of climate scenario analysis for the financial sector. Section 3 presents the practical framework. Section 4 proposes ways forward by introducing the idea of a more comprehensive scenario taxonomy. Section 5 concludes.
The following analysis provides practical insights into key scenario features, model characteristics that underpin scenarios, and wider scenario considerations that are important to equip financial institutions with the required knowledge on how to effectively select, use and interpret currently available scenarios and increase their suitability for decision making (Table 1 provides an overview). Further, our assessment draws upon best practices and empirical evidence to highlight the relative importance of the above dimensions for different financial sector use cases. We highlight that insufficient understanding of such dimensions and associated deficiencies of scenarios may lead to a misuse of scenarios and a systematic underestimation of the risk associated with the transition. This knowledge then feeds into the framework for scenario identification and construction presented in Section 3.
Table 1. Summary of key characteristics of currently available scenarios and their different degrees of relevance for financial sector use cases.
Financial use cases are guided by the analyses of UK supervisory statements on climate change (Annex 1) as well as informed by parallel CGFI research (Ranger et al., 2023). The evidence and framework presented in this section build upon and complement classifications and taxonomies provided, among others, by Monasterolo et al. (2022) to describe the different characteristics of IAMs, or for physical risk, by Ranger et al. (2021).
We proceed as follows. First, we map out key scenario features of climate scenarios relevant to different FI needs to inform adequate scenario selection. Second, we assess underlying model characteristics that influence where current scenarios sit within the range of plausible outcomes and draw out challenges around model choices in the context of the specific requirements by FIs. Third, we explore wider scenario considerations that are relevant to frame such a climate scenario analysis. Finally, we draw out the specific challenges that FIs are confronted with when interpreting scenarios and assess the implications for the risk associated with their materialization.
Table 1 identifies six use cases of scenario analysis for FIs and CB&S, drawing from current supervisory statements, spanning aspects of risk management and business planning. It further elucidates the specifications of scenarios required, such as the time horizon, scenario pathway features and where scenarios sit in the probability distribution of materialization.9
Most importantly, each use case requires a different time horizon. Longer-term scenarios are required to help inform strategy and business planning decisions. Short- and medium-term scenarios are needed to inform risk management-related outputs such as internal or regulatory stress tests, limit setting or capital setting. Each use case also requires different scenario features which ultimately inform where the scenario sits within the probability distribution (e.g., central scenario vs. tail-risk scenario). Strategic scenarios should reflect a central scenario and reasonable expected pathways that have the potential to influence business planning, representing the highest likelihood of materialization. Whereas, stress testing requires tail-risk scenarios that allow FIs to test their resiliency to more extreme (or worst-case) plausible outcomes. This application is particularly relevant to CB&S, as well as FIs, for exploring the resiliency to the potential systemic implications of climate change for the financial system.
The specifications of these use cases, and their requirements, have implications for the suitability, interpretation, and appropriate use of scenarios. Choosing the appropriate time horizon and an initial assessment of where the scenario sits within the probability distribution for the respective use case is key to an adequate climate scenario analysis. For example, scenarios provided by organizations such as the IEA, the NGFS or the IPCC are likely to be the most appropriate for business planning applications, given the nature of the underlying models, but arguably have limitations for stress testing applications. This is because these transition scenarios show the cost-optimal mitigation pathways across different degrees and timings of policy action (IPCC, 2015), and the associated technological and behavioral changes necessary to achieve the stipulated climate target. As such, they are suitable to reflect the “smooth” transformation of the economy and can therefore inform more systemic policy decisions. For instance, informing the aggregate investment required in low-carbon technologies to transform the energy system in line with temperature goals set out in Paris Agreement.
Further, they can provide insight into what happens if sectors or governments continue on a business-as-usual path as opposed to imposing early climate action. These scenarios provide insights into the temporal effects of change and the different options we have as a society and how they may play out under reasonable expected conditions. For instance, assessing whether investment in public infrastructure within targeted sectors can reduce GDP losses and increase the penetration of renewables in the energy system.
Such scenarios can therefore act as a guide for policy makers and financial markets in setting business strategies, helping to shape market expectations and to realize the benefits from a variety of aspects of the transition. To be effective, scenario users must be able to anticipate future narratives and the range of potential outcomes that could occur given economic-socio-climatic relationships and the likely policy actions that are undertaken. This can also aid FIs to optimize strategic decisions vis-à-vis the market to benefit from and support the transition. For short- to medium-term business planning of FIs, scenarios should reflect reasonable expected scenarios that sit central in the distribution. This is to provide insight into tactical business decisions. Most of the high-level scenarios provide limited insights on the transition effects at a more granular level, such as among individual firms or institutions, that behave differently within the wider system, so will often need to be complemented with other data and analyses for a more granular assessment.
In contrast, for stress testing and broader financial risk management purposes, scenarios should reflect reasonable worst-case scenarios that sit further in the tail of the distribution (Kemp et al., 2022). This is to ensure balance sheet risks are captured under classical financial risk logic. Such scenarios need to be inherently different to a central case or best-case scenario used for longer term planning (Koberle et al., 2021). However, most of the scenarios that are being explored for risk management purposes by FIs are longer-term scenarios that do not reflect relevant transition dynamics and volatilities. For example, Figure 1 shows the relevant energy transition pathways from NGFS net-zero scenarios over the time horizon 2020–2100 (left) and when zoomed in to 2020–2030 (middle) and 2020–2040 (right). It is evident that the smooth trends depicted for a 10- and 20-year time horizon respectively represent more of a central scenario and are therefore unlikely to produce meaningful variation in financial risk outcomes when used by FIs to inform risk management and may miss a large picture of the relevant tail risk.
Figure 1. Energy-sector pathways (primary energy and electricity capacity across natural gas, oil, and coal) from the NGFS GCAM Beyond 2 Degrees scenario, zoomed in across different time horizons. Authors own calculation based on IIASA scenario explorer database. https://data.ene.iiasa.ac.at/ngfs/#/login?redirect=%2Fworkspaces.
We further explore this throughout the next subsection and argue to understand where a scenario sits within the probability distribution (e.g., tail-risk), the underlying narrative and modeling choices, as well as assumptions, need to be better understood.
We now turn to the core characteristics and assumptions of the models that underpin current scenarios that are important to consider when identifying, selecting and interpretating scenarios for both business planning and stress testing applications. Again, these core model characteristics are mapped against the needs in Table 1 to elucidate the approximate relative importance for specific financial use cases.
Different transition narratives that postulate different ways of how the transition could unfold present an important feature of models that underlie climate scenario analysis. Key assumptions on the structure of the energy system, the speed of technological progress, the extent to which socio-economic and climate constraints are reflected, as well as assumptions on institutional inertia and behavior are important in shaping the scenario narrative. Even within scenarios of one provider, such as the NGFS, different models are used to better capture the uncertainty around these assumptions by reflecting different parameter choices with significant methodological variation. Additionally, across scenario providers, e.g., the IEA vis-à-vis the NGFS, there is an even higher degree of key assumption variability—for instance, how the energy system is modeled. This is most often not transparently conveyed to the financial sector and difficult to grasp for practitioners. Such a variability, without sufficient information on what's driving it, makes it increasingly difficult for financial practitioners to judge the credibility and level of conservativeness of different parameter values and pathways. Consequently, tracing back which key differences in the variability across scenarios are driven by which assumptions and how they relate to each other is difficult to assess.
Figure 2 shows the variability of global unit cost trajectories for solar power, an important driver in IAMs to determine the speed and uptake of renewable energy to decarbonize the economy, across different scenario providers. Strikingly, the rate of change is substantially different, depending on the underlying model that was used to project such pathways. A lack of transparency around these assumptions will not allow the financial user to distinguish and classify what policy choices or complexities are driving these differences in isolation, and in turn the variation in financial risk outcomes (e.g., how energy firms are impacted across scenarios). An inadequate understanding of key assumptions is therefore highly problematic and makes interpretation and validity of results for financial practitioners increasingly difficult. Further, attaching a likelihood of materialization and judging the level of conservativness of some key assumptions is made nearly impossible (e.g., how likely are each of the scenarios against each other; what are the consequences for carbon-intensive energy firms and resulting financial risks from a faster uptake of renewables than anticipated in the IEA scenarios). This is preventing FIs from reaping the benefits that are inherent in the breadth of scenario narratives. What is more, such a variability and the surrounding uncertainty is passed on through the climate scenario modeling chain with often unclear impacts on financial risk outcomes. Interestingly, Gasparini et al. (2022) identify that such a variability of key assumptions across scenario providers (even for the same climate target narrative) leads to a significant variability in the financial risk outcomes on the counterparty level. The authors show that the financial risk for energy firms is significantly different, depending on whether technological progress and assumptions on the change in energy mix throughout the transition are taken from the NGFS or other scenario providers.
Figure 2. Projected implied rate of change of cost developments (LCOEs) in a below 2° transition scenario for solar technologies in the power sector globally across different scenario providers and models [Oxford represents technological forecasts by Way et al. (2021)]. LCOEs expressed in $/MWh at 2020 levels. Normalized starting value for visualization purposes. Authors calculation.
We further pick up how such an obstacle of scenario variability can be used to enhance the capabilities of FIs as a positive feature in the practical framework introduced in the next section.
There are also fundamental differences in relation to how transition scenarios are processed across different macroeconomic model types, which are again important to conceptualize the outcomes of a scenario analysis. For example, some general equilibrium models impose restrictions on the money supply (Pollitt and Mercure, 2017). This leads to additional investment crowding out existing investment in the transition. Within these models, the transition to a low-carbon economy is framed as diverting away from a general equilibrium, with the economic system recovering from such a deviation and bouncing back to an equilibrium (Bolton et al., 2020). This shift is associated with high economic cost in the short- to medium-term (Mercure et al., 2019). Other model approaches account for crowding in effects, and therefore new investment has wider positive effects. These models frame the transition as having a positive net economic effect (Mercure et al., 2019). The macroeconomic model choice therefore represents a significant difference in the scenario narrative that needs to be better understood when deciding between the baseline setting of scenarios according to the users' beliefs and market expectations.
The range of narratives, as a result of different modeling approaches that underlie scenarios, require FIs to improve their understanding of where in the probability distribution each scenario sits given the level of conservativeness of key assumptions whilst assessing whether this is appropriate for a given level of risk appetite. Such characteristics and the implications on the financial risk outcome need to be considered when interpreting and using these scenarios in practice. For instance, in the framework section, we demonstrate with an example, how sensitivity testing and the assessment of assumption deviations can enhance the robustness of results and test a variety of different future pathways, with varying levels of conservativeness around technological innovation, depending on the users' own beliefs around market behavior (see framework Section 3.3). It is evident throughout this subsection that the transition narrative has a high importance for both, business planning and risk management exercises.
As already identified, most scenarios are reliant on IAMs primarily developed to identify optimum policy pathways which represent smooth trends along the time horizon to reduce complexity. Further, traditional macroeconomic models are often not well-suited to capture the frictions associated with a rapid large-scale transformation to a low carbon economy and potential short-term volatilites along the transition pathway. Yet, this is especially relevant for risk management-related purposes such as risk appetite setting, stress testing and capital setting, where trajectories should reflect reasonable worst-case scenarios. Choosing scenarios that lack such key relevant characteristics are likely to underestimate the financial risk and the potential losses that could occur due to rare events, high volatilities, and frictions. For instance, as Aguais and Forest (2022) show in an empirical multi-factor credit portfolio model, credit risks are generally not driven by smooth macro-economic trends but by unexpected economic shocks that represent higher volatility and systematic deviations from average trends. Their analysis shows significantly higher climate-related credit risk in contrast to NGFS scenario approaches.
Most currently available scenarios do not adequately cover the full envelope of such possible frictions and disruptions. The transition is likely to crystallize through several discrete shock events. For instance, these might result but are not limited to a combination of a failure of adopted policy pathways to deliver the anticipated results leading to a choice to accept greater long-term climate change or apply more dramatic policy action with significant short-term financial implications. Further, rapid technological advances may abruptly shift market expectation of future policy action or climate change on financial markets (Minsky moments).10 We therefore identify the need for a wider focus on identifying and incorporating economic and financial frictions to produce disruptive scenarios that are more realistic and decision-relevant than those currently available. We discuss several cases in more detail on how such discrete shocks could materialize.
Most models assume a smooth substitution with no explicit friction or representation of non-linearities for the transition between high and low-carbon technologies in the energy sector. However, as the energy system becomes greener, its lobbying power increases and the likelihood of a carbon tax may increase. Similarly, amplification mechanisms are often not considered, such as increased investment in green technologies driving their cost down and making them more competitive with fossil fuels, which further increases green investment (Way et al., 2021). Such frictions and amplification mechanisms could induce a tipping point, where the system shifts very rapidly, causing assets to be stranded suddenly without a smooth divestment. Geographical assessments based on the resilience of specific economies to supply and demand imbalances are also important for understanding how likely different scenarios are in various regions. van de Ven and Fouquet (2017) find that the resilience of a country to energy related shocks is dependent on the fuel mix rather than economic development. Firms should also remain cognizant of how a short-term mismatch could be more impactful across geographies that lack policy regimes and the appropriate fiscal infrastructure spending capacity to react to different mismatches within the transition. It is important that different economic and socio-political contexts are understood to shape the scenario narrative and the key drivers relevant for certain jurisdictions. Alternatively, given latest developments with the war in Ukraine and the energy crisis, it may be important to reflect in scenarios a sudden change of expectations around market-driven technological progress that results in energy price spikes volatilities or increased risks that are present in the balance sheets of FIs (Mercure et al., 2018; Behnam and Litterman, 2020; van der Ploeg and Rezai, 2020; Bolton and Kacperczyk, 2021).
There is limited representation of labor frictions (other than exogenous restrictions) that might create bottlenecks when transitioning to a net-zero energy system. Labor force frictions throughout the transition are also likely to impact key economic variables. Lankhuizen et al. (2022) show that technological advancements and climate policies designed to improve energy efficiency are likely to cause labor-related bottlenecks. Fossil-fuel related jobs are also likely to disappear due to low labor market mobility. Yet, literature on labor shifts and skill shifts in relation to climate change remains scarce and contains large uncertainties.11 Additionally, these frictions could potentially restrict the speed of uptake of renewable energy and subsequently could create disruptions within the energy supply.
The assumption that it is possible to transition the economy in a frictionless way may significantly downplay the amplifying role of misaligned expectations on financial markets. For instance, Gasparini (2023), analyzing the impact of climate policy uncertainty on the valuation of fossil-fuel stocks, has found that on average brown companies are valued as if their investors believe the green transition will never happen, while green companies are valued as if their investors believe it will happen in <20 years. The two groups of investors cannot both be right, indicating that a major realignment of stock prices is likely to happen. Such frictions could again move a system very rapidly. This could cause major and sudden stress across the financial system, further slowing down the required investment to support the transition. Since a quarter of the value of global stock markets, half of the value of corporate bonds, and half of syndicated U.S. loans are from fossil fuel-related companies, the consequences of this for markets are significant. Current scenarios do not account for such behavioral frictions and feedback mechanisms between the real economy and financial markets. Note that academics such as Battiston and Monasterolo (2021) and Monasterolo et al. (2022), among others, have stressed the importance of such effects, and the NGFS is further raising awareness on such deficiencies. Operationalizing such complexities in IAMs is however an ongoing process.
Current scenarios proxy policy action with the implementation of a carbon tax. Here, well-known limitations include the insufficient geographical differentiation, complementary and distortionary effects of other forms of policies, as well as the lack of representation of misalignments between the climate commitments of jurisdictions (Mercure et al., 2019). On top of that, we argue that current scenarios are not sufficiently accounting for the time-lag between policy implementation and market reactions in the form of real-world emission reduction. In other words, models assume an instantaneous market response (Asefi-Najafabady et al., 2021), which in reality is highly unlikely and may further lead to a breach in the carbon budget, overshoot, or increased stranded assets due to delayed phase outs of infrastructure linked to carbon-intensive production processes. It is important that FIs can anticipate potential time-lags and any associated frictions relevant to their own exposure that could occur once a policy response, or discrete shock is applied to a scenario. Ideally, firms would be able to improve upon this simplification by using a dynamic model, incorporating a lag between the introduction of a carbon tax and the associated knock-on effects to other variables. These time-lags should be dependent on specific policy and become effective, as typical with standard investment projects, ranging from months to years, rather than instantaneous (Kolev et al., 2012). For example, forming views on the time required for institutions to adapt their pricing strategies, and any related consumer response associated with changing behaviors is important as demand for energy is inelastic in time-series data. Firms could also develop capabilities to recalibrate scenarios to how the impact of a carbon tax is likely to differ between sectors or geographies.12 For instance, Green (2021) provides evidence for sectoral differences due to heterogeneous elasticities, finding mixed evidence of the effectiveness and the direct relationship between carbon pricing and emissions. It is likely that incorporating emission reduction delays results in a more severe scenario where the decarbonization targets for specific time horizons are unlikely to be met. Accounting for such time-lag between policy and market response could be leveraged to reflect a more realistic picture of the likely disruptions that could materialize in the transition.
Structural challenges around modeling the complex interaction and feedback effects of climate change, the economic and financial system may lead to an underestimation of the consequences of a scenario. IAMs have been developed to capture a wide array of mechanisms that link policy decisions, the climate system, and parts of a global economy. However, several phenomena induced by climate change such as migration, crop yield shocks, and social instabilities in exposed regions, as well as feedback loops are neglected in IAMs and hence cannot be represented in climate pathways for financial exercises (Weyant, 2017; Asefi-Najafabady et al., 2021). Further, the links between climate, ecosystems and natural resources (e.g., soil, water, and forestry) which are known to be important drivers of financial risk are often excluded (Dasgupta, 2021). As Almeida et al. (2023) highlight, existing scenarios used by central banks and FIs currently do not sufficiently incorporate broader environmental risks, such as nature-related risks, in part due to methodological challenges around modeling nature-economy interactions with financial sector dependence. More broadly, IAMs remain limited in their capacity to incorporate complexities in relation to non-linearity, tipping points, and uncertainty. Rational expectation assumptions lead to individual components of the system being optimized. However, real behavior is different, as participants have limited knowledge to make appropriate choices. For instance, reflecting the behavior of fossil-fuel dependent states in supporting international climate negotiations and carbon tax policies remains irrational, with many geopolitical factors around comparative advantages driving decisions (Mercure et al., 2021). Further research has argued for the inclusion of compounding shocks alongside different factors and interdependencies that so far have been neglected (Ranger et al., 2021). Current model approaches and scenarios insufficiently capture acute physical risk shocks in models that aim to capture the climate response to assumed emission pathways (Ranger et al., 2021; Pitman et al., 2022). UNEP FI13 provides a good overview of IAMs and general limitations for financial practitioners, and academic literature is widely available (see among others, Brock and Hansen, 2017; Stern et al., 2021). Monasterolo et al. (2022) provides a good comparison of the process based IAMs used by the NGFS with alternative models.
Scenario modeling chains include various sub-models that are linked together, subsequently feeding into macro economic and lastly financial models. Simplified transmission channels and interaction effects with varying degree of granularity may result in significant information loss and an increase in the uncertainty along the modeling chain (see also Figure 4 for a representation of the various stages, including additional scenario expansion for FIs). Especially, the insufficient pass-through of extreme tail risks, cross-sectional and geographical variation ultimately results in a loss of information that would be needed by the financial sector. This can be problematic for transition risk (aimed at reflecting volatility along the transition), as well as physical risk where acute climate risks may be presented by averages, rather than extremes, which are known to be the main driver of financial risk and catastrophic socio-economic impacts (Ranger et al., 2021).
For instance, IAMs may produce sub-sectoral impacts due to a variety of regional climate policies, which are then translated in financial pathways using a macro model that lacks the sophistication to reflect sub-sectoral dynamics (e.g., NIGEM). The resulting impact and risk distribution will therefore miss relevant variation. When such impacts serve as inputs into financial models to uncover risk at the counterparty-level (e.g., to assess the transition impact on FIs balance sheet) this will not be directly possible without additional downscaling or expansion of the initial scenario pathways. What is more, such a loss of information and relevant risk variation restricts identification of comparative advantages across firms within a sector and increases the difficulty to identify the heterogeneous impact of the transition (see for instance Baer et al., 2022 for a more granular climate stress testing model, but some of the problems remain due to dependency of such approaches to macro-level scenario pathways). In the long-term, some of these problems might be addressed through the development of more sophisticated IAMs that are more tailored to the needs of the financial sector. They could include greater financial granularity in their output, but as noted earlier, this might further challenge the understanding of financial sector users. Irrespectively, it is important to highlight that combining different modeling approaches with varying degrees of granularity and complexity may understate the propagation of uncertainty and reduce accuracy through the various stages. The loss of information further exacerbates the difficulty to use scenarios.
We advocate for closer research cooperation between bottom-up modeling approaches and macro-approaches to enhance the level of granularity that such IAMs can capture. While a new generation of analytical methods exists to overcome some of these limitations, such as stock-flow consistent models, agent based-modeling or heterodox economic approaches, challenges remain. To uncover the needs of the financial sector to perform granular scenario analysis, a more sophisticated micro-level integration into existing modeling infrastructure is needed, similar to those suggested for physical risk models (Pitman et al., 2022). Overall, the insufficient pass-through of risk variation across the scenario modeling chain has a higher relevance for financial risk management use cases.
As already identified above, scenarios may not match the level of granularity needed by the financial sector and too much room is left for scenario expansion to adequately capture the full spectrum of the risk range. This confronts FIs with the challenge of achieving consistency throughout the scenario expansion with previous modeling choices and assumptions but this is difficult given the underlying assumptions are often poorly understood by the user. For an appropriate scenario expansion, it is important to understand the level of granularity and modeling complexity across the various stages in the development of a scenario, from input data to IAMs, which pass on information to macroeconomic models, which then produce macro-financial pathways that can be inputted into financial risk models. Figure 3 below provides a simple representation of the steps involved in the construction of a climate scenario for financial practitioners, including potential scenario expansions that are required to match the level of sophistication of the financial industry.
Figure 3. Key modeling chain in climate scenario analysis including scenario expansion for the financial sector. Authors' own illustration.
As noted, most IAMs and macroeconomic models do not feature a firm-level, sub-sectoral and country-specific breakdowns of climate-adjusted economic pathways. In addition to the loss of relevant risk variation, this confronts FIs with the difficult task of downscaling to reflect the level of detail needed to conduct meaningful financial risk assessments. This can be difficult in the absence of clear guidance on the scenario expansion by CB&S, as evident throughout the CBES exercise (Ranger et al., 2023). We argue that it is the modeling of relative performance between counterparties and jurisdictions that is particularly important for FIs but current modeling approaches that underlie scenarios leave much of this analysis to users to expand. What is more, the financial industry will not be interested in static views of risk from policy makers and will push back on approaches that do not match the level of sophistication of their own risk management frameworks. The relevance of such a scenario expansion will also vary across different use cases. For internal stress tests over a shorter time frame that aim to identify risk at a granular level, the expansion process is critical. In contrast, for a longer-term analysis such as strategic planning, a view on the comparative performance of individual counterparties may not be as pressing. Here, a more systemic picture of how the transition unfolds across sectors may be sufficient. What is more, models and scenarios insufficiently capture the diversity of knowledge and specific political contexts relevant across countries. For instance, using global NGFS scenarios may not be adequate to capture individual socio-political and country-specific contexts, but this may be an important feature of granular scenarios that inform policy makers locally.
Until more granular scenarios are provided by the scientific community, the financial industry will need to develop additional scenario related modeling capabilities as IAMs are unable to provide all the information financial firms require. A recent CGFI survey showed that most FIs lack the expertise and understanding and hence shift this task to third party providers (Ranger et al., 2023). This often does not build out the necessary internal capabilities and understanding required to appropriately use scenario analysis and interpret the results.
The analysis above identifies the core features of scenarios and characteristics of models that influence scenario selection, interpretation, and usability for different FI use cases. Each of these characteristics also influences the likelihood of the scenarios and are conditional on the status of policy action. Understanding where scenarios sit within the range of plausible future outcomes is essential for all use cases and has implications on intertemporal trade-off choices by FIs and CB&S. We explore this in more detail.
Little attention has been given to the probabilistic likelihood of scenarios. This is likely due to the expertise required and the inevitable degree of judgement and uncertainty prevalent within the assessment. Yet, to make practical use of scenario analysis one requires exactly such a view of the likelihood of the different potential future outcomes over the relevant time horizon. Given the complexity and lack of information provided by scenario developers, FIs and CB&S are at risk of misinterpreting where scenarios sit within the range of possible outcomes, making it difficult to interpret the results. While Lawson et al. (2023) aim to assign probabilities to scenarios to better tailor for the needs of financial and investment decision making, limitations remain across currently available scenarios. For instance, to understand what risk lies in the tail of the distribution,14 there needs to be a judgement on the likelihood of materialization across time. Most obviously, every year the transition is delayed, and emission targets are not met, the likelihood of more disruptive policy responses and potential frictions in the economic and financial system are increased. An orderly transition that limits global warming to 1.5°C may not be feasible anymore and even a disorderly scenario is shifted into the extreme tail of the probability distribution. For instance, evidence continues to show that previous central estimates may no longer be realistic. The international community is falling far short of the Paris goals, with no credible pathway to 1.5°C in place (UNEP FI, 2022). The probability of a more disorderly transition pathway is therefore significantly increasing over time (further exacerbated by macroeconomic headwinds such as global inflation and the Ukraine Crises NGFS, 2022).
On the other hand, evidence suggests that the Agriculture, Forest, and Other Land Use (AFOLU) sector, which is responsible for ca 20% of global greenhouse gas (GHG) emissions, may be represented in scenarios overly pessimistic, albeit the mitigation potential varies across key geographies (Roe et al., 2021). However, whether such mitigation potential is realized is yet to be observed. Overall, the more ambitious the assumptions are for areas of the less economically developed world the more the western financial system gets a “free” ride in terms of limiting climate change. We argue this needs to be considered more strongly when framing the scenario likelihood conditional on mitigation progress and policy action.
We observe similar effects in relation to model assumptions around the potential for removal of carbon and status of policy action and technological progress. Smith et al. (2023) find a gap between proposed carbon dioxide removal (CDR) deployment and what will be needed to meet the Paris Agreement and pursue efforts to achieve 1.5°C, placing such scenario assumptions, again, at the optimistic end. Relying on such optimistic components of scenarios could spur inaction, which in turn may increases the likelihood of the central pathway resulting in irreversible physical damage due to natural catastrophes and chronic risk increases. To avoid this damage, more disruptive policy action will be required. Overall, the status of policy action vs. scenario assumptions around mitigation progress may become increasingly decoupled as time passes by and if not regularly updated.
As highlighted above, when insufficient mitigation action shifts the probability of the scenario across time, the trade off-argument between transition costs now vs. widespread physical risk cost later becomes more relevant than a choice between different speeds of transition scenarios. Scenario analysis should therefore be interpreted through the lens of interacting transition and physical risk rather than treating these impacts independently. Importantly, economic and financial frictions should also be considered in the context of increasing uncertainty and magnitude of shocks as time progresses when the solution to climate change becomes increasingly difficult. In other words, FIs should be aware that frictions become more material when the speed of change is fast and technological, as well as socio-economic constraints are hit. For example, the possibility of a short-term disorderly transition stemming from labor-related bottlenecks within the energy sector should be considered if the ramp-down of high-emission activities is not carefully managed (McKinsey Global Institute, 2022). Simultaneously, the failure of adopted policy pathways to deliver the anticipated results could lead to an underappreciation of the intertemporal trade-off between greater long-term climate change or more dramatic policy action with significant short-term financial implications. This has further implications on the risk-build up in the economic and financial system.
The evidence presented in this section so far not only highlights the importance for considering various dimensions in the scenario selection process, the use and interpretation by financial institutions, but also support a conclusion that scenarios collectively systematically underestimate the scale of the transition and physical risks. Hence, financial institutions need to carefully examine whether current scenarios may be toward the lower bound of possible outcomes. For instance, we have demonstrated that some model characteristics, such as the omittance of relevant frictions and relevant key risk drivers, structural simplifications of IAMs, the negligence of feedback loops and tipping points and an increasingly decoupled status of policy action vs. scenario assumptions may push climate risk scenarios further into the optimistic tail of the risk distribution, leading to an underestimation of the risk.
Table 2 summarizes dimensions assessed in this section and their likely implications for the risk outcome of the scenario. Most of the identified dimensions and model characteristics suggest an underestimation of the risk, potentially giving rise to a false sense of security on how the transition may unfold. We highlighted the omittance of key risk drivers such as compounding risks, biodiversity loss and migration, that suggest we currently do not capture the full spectrum of risk. What is more, simplifications in IAMs such as the negligence of amplification mechanisms in financial markets is insufficiently reflected in current scenarios. We also discussed the potential underestimation due to lack of representation of economic and financial frictions, as well as simplified acute physical risks impacts. Further, an insufficient reflection of current policy action vs. the scenario assumptions may underestimate the risk of an increasingly disorderly transition. When mitigation progress and policy action is not sufficiently considered in climate scenario risk analysis, this may further result in the underestimation of the systemic risk build up, besides the underestimation of idiosyncratic risk for financial institutions. For instance, when firms collectively fail to transition their business strategies due to overly optimistic expectations on policy action, a steeper investment is needed in the future to adjust in a shorter timeframe to remain within a prescribed carbon budget. This will result in a systemic risk-build up, with more economic and financial costs, again increasing the severity and volatility of transition impacts even in central scenarios (Baer et al., 2021b; Way et al., 2021).
In principle, the consideration of systemic stress and risk build up has a relatively higher relevance for CB&S and confronts them with risk appetite challenges for micro and macroprudential regulators. Whilst individual financial institutions can take unilateral steps to reduce risk, regulators tasked with macroprudential oversight need to account for the aggregate decisions of financial institutions within the system. However, FIs should not ignore such important dimensions to capture the full spectrum of risk. While the transition and associated structural changes to the economic system may allow for partial individual risk hedging, as policy response become increasingly delayed and risk builds up, FIs may be affected by systemic risk even in the short term (Andersson et al., 2016).
Overall, the evidence presented in our analysis suggest that current scenarios do not adequately reflect the tail-risk or miss some risk drivers altogether. This is in line with other research, such as by Kemp et al. (2022) that show how climate scenario analysis up to date may understate the risks because it fails to consider the more extreme climate and transition outcomes. Arguably, such a systemic underestimation, while recognized by FSB and NGFS (2022), should inform upcoming climate scenario analysis exercises internationally, such as the one in the US with six major banks15 to prevent misuse and absorb the lessons from similar exercises by central banks in Europe and the limitations and wider considerations put forward in this paper.
Importantly, it should be noted that even “better” climate risk scenarios can amplify systemic risks when unintended consequences are not carefully considered. For instance, risk models can exacerbate global inequalities when flows of finance are restricted to countries based on a lack in analytical capacity or data. The lack of historical data in emerging countries may translate into greater uncertainties, higher risk estimates, and greater cost of capital needed for development, mitigation and adaptation. Therefore, the financial and public sector has an important role to distribute improvements in modeling and scenario analysis equitable across advanced and emerging economies, while advocating for its possibly unintended consequences, to help countries manage the transition which will be critical to maintaining financial stability and continued access to international capital markets. For instance, this could be achieved through making risk data freely available through initiatives such as the Global Resilience Index Initiative (GRII), while building capabilities.16
While we argue that over-reliance on currently available scenarios could lead to an underestimation of the risks, our evidence suggests that the deficiences in scenarios are not a barrier to action if they are understood, expanded upon appropriately and interpreted in full awareness of their characteristics and their inherent trade-offs. This evidence and conclusion inform our practical step-by-step framework in the next section where we identify how current scenarios can be augmented to increase their decision relevance.
Overall, our analysis so far provided practical insights into the knowledge gaps and deficiencies of scenarios. Throughout the section it also becomes evident that for different use cases specific dimensions have a relatively higher importance. For instance, scenarios for risk management and stress testing are less suitably described by a cost optimization scenario pathway that presents a smooth adjustment of the economy. The representation of economic and financial frictions is key to fulfilling the objectives of such exercises and may be insufficiently reflected in currently available scenarios. Scenario expansion to reflect risk on a granular level may have less priority for informing the long-term strategic direction of financial institutions, where the consideration of intertemporal trade-offs and risk build up may be more immediately pressing issues to reflect in scenarios. Further, for central banks and supervisors, a perspective on systemic risk may be key to identify financial stability risk, whereas individual financial institutions should focus on expanding scenarios to overcome specific challenges for counterparty-level risk assessment, in particular the insufficient granularity of current models that do not match the level needed by the financial sector, resulting in challenges and inconsistencies around the scenario expansion process. Importantly, wider considerations around where scenarios sit in terms of the level of economic stress conditional on policy action and mitigation process is critical to judge the accuracy of climate financial risk outcomes.
In the previous section we identified key scenario characteristics and wider obstacles for the effective use of climate scenario analysis. We have argued that these deficiencies may prevent an adequate use of scenarios by the financial sector if not adequately interrogated and augmented for the needs of specific financial use cases. In this section, we propose a practical step-by-step guide (see Figure 4 for overview) that aims to enhance financial practitioners understanding of the key characteristics of scenarios for a given financial sector use case, how to best augment existing scenarios to overcome identified limitations and guide users on how to sensitivity test a wider range of possible assumptions to capture the full breadth of scenario narratives, prevent risk-underestimation, and allow for the granularity required by the financial sector. The framework consists of four stages and is initially focused on risk management and stress testing applications. It aims to complement the suite of scenario guides published by organizations such as the NGFS or the Bank of International Settlements (BIS).
Figure 4. Practical framework outlining stages to understand scenario archetypes for specific use cases, expand scenarios to account for frictions and how to integrate into financial risk assessment.
More generally, our framework is an initial step toward a better systematic understanding of scenarios and their tailoring to meet the needs of financial practitioners. We argue that in the long term, the academic community has a key role to play in expanding the coverage of available scenarios so that less expansion needs to be performed by the financial sector. Until then, currently available scenarios can be augmented as an intermediate process with varying degrees of complexity. The analysis and framework proposed in this section are initial steps toward solving these issues and are aimed to assist in the learning process by improving FIs understanding and increasing the suitability of scenarios for key financial use cases in a timely manner.17
Stage I should guide the user in selecting an adequate third-party scenario based on the individual use case.
The discussion around obstacles to the effective use of climate scenario analysis put forward in Section 2 of this paper can inform such decisions. More specifically, the scenario narrative, positioning the scenario in the probability distribution of potential outcomes and the time horizon should be matched with the use case. Users should also consult other scenario guides, such as by NGFS (2020) and Koberle et al. (2021) to aid with this process. It is important to identify climate change pathways with timescales and relevant scenario characteristics that are adequate to the financial exercise and the business model of the user.18
For risk management related purposes such as climate stress testing and capital setting, users need to focus on scenarios that reflect a reasonable worst case over a short-term horizon. Choosing available scenarios that lack the key relevant scenario characteristics for this specific use case are likely skewed toward the optimistic end of the risk outcome. This would result in an underestimation of the financial consequences associated with them. It is therefore critical for the user to assess the suitability of fundamental scenario narratives and to better understand high-level scenario characteristics.
Once the characteristics of the high-level scenario are better understood and the use case clearly defined, it is important to better understand the detailed assumptions of the scenario modeling chain and to assess where individual scenario elements sit in terms of credibility of global policy action and level of conservativeness of the assumed impacts. Such a scenario deep dive informs the user on the plausibility and likelihood of the underlying assumptions present within different scenario components.
The user should clearly identify the key assumptions and associated weaknesses in the chosen third-party scenarios. Depending on the capabilities of the user and scope of the exercise, such a deep dive can be undertaken only for the scenario components that are most relevant for the user. For this, FIs should identify the key climate-related exposures within their balance sheets. The goal is to identify which assumptions and narratives FIs are most sensitive too. For instance, an asset manager may identify that a large share of her portfolio is exposed to the agricultural sector within developing and emerging markets. To then identify the scenario assumptions that are most relevant to further investigate, the user needs to understand the modeling choices and wider narrative in relation to the agricultural sector. Firms can identify key assumptions around dimensions such as the mitigation potential of land-use change, or regional carbon tax feasibility. Further investigation should then be undertaken to better understand how assumptions may be calibrated within IAMs and macro economic models in a more regional or country specific context.
Similarly, CB&S should identify areas which are exposed to systemic risk, testing key assumptions that have interdependencies which could lead to severe financial stress. Macroprudential policy should consider high-emitting exposures, identifying and understanding how structural shifts in these exposures could affect the broader economy and the financial system. All relevant scenario components and driving assumptions should be mapped. For instance, the US banking system shows a significant proportion of overall exposure to the fossil-fuel related energy system. It is therefore important to identify in the chosen scenarios the key components, assumptions and modeling choices that govern how the transition of the energy system unfolds and how FIs may hold concentrated exposures. For example, assessing unit costs pathways between high- and low-carbon power technologies, infrastructure inertia, carbon tax assumptions, or labor elasticity and substitution effects. Having a general overview of which assumptions are driving the differences within scenarios and the range or resulting outcomes is critical for the next stage to perform the necessary scenario adjustments and expansions to better suit the financial exercise use case.19 Choosing the most relevant set of scenario assumptions is also critical for lower capability stakeholders that wish to perform a more thorough stress test for a subset of their key exposure. Here, understanding the full spectrum of assumptions may simple not be possible or desired.
For the user it is now important to assess how accurate and likely the representation of assumptions is in the selected scenario. A user may question whether overall assumptions such the representation of a frictionless transition with no mismatches between energy supply and demand is likely to unfold throughout the transition by drawing upon current and historical events, as well as additional empirical evidence. For example, drawing on parallels of recent geopolitical developments and assessing evidence on energy price volatility due to supply shortages may put the instability of the energy system throughout the transition into perspective. Short-term economic pressures (e.g., Ukrainian war) or macroeconomic developments (e.g., inflation) are likely to make assumptions around capital reallocation more or less sensible in the short-medium term. For example, Figure 5 below shows the divergence of current fossil fuel price developments away from expected scenarios. According to this, the current price of fossil fuels should be discouraging demand by more than the anticipated carbon tax in the NGFS orderly 1.5 scenario. These developments are likely suggesting that increased prices in high-emitting technologies (e.g., due to a carbon tax) may not lead to the warranted demand shift as stipulated in current net-zero transition scenarios. However, the impacts of this are yet to be assessed. Nevertheless, it follows that the assumption of increased prices that will lead to an acceleration toward energy efficiency and renewable energy sources may be overly optimistic, with unclear consequences on the need for other parts of the system to decarbonize more drastically to stay within prescribed carbon budgets.
Figure 5. Current volatile price developments across key technologies and projected prices based on transition scenario by NGFS (2022). 1For realised prices, quarterly average price; for market futures, latest futures prices. 2For realised prices, Brent futures. 3For realised prices, CME natural gas physical futures. 4For realised prices, Rotterdam coal futures. 5The modelled costs show the sum of primary energy price and carbon tax projections based on average emission intensities. These are also indexed to 2020 model period, though this represents an average price level over the modelled 5-year period. Indexing the green curves to the same average value would shift the green curves down a bit.
Further, it is critical to assess the credibility of assumptions in relation to the pessimistic consequences of fiscal measures aimed at producing short-term economic relief leading to an increase in carbon emissions such as during the COVID-crisis (Hepburn et al., 2020). It needs to be assessed how such measures impact the credibility of global policy action. The transition itself will likely see periods of acceleration and deceleration and experience “shocks” from non-climate-related exogenous factors. FIs and CB&S should therefore scrutinize currently available scenarios to better reflect reality, for example, assessing scenario pathway deviations due to governments increasing fossil fuel subsidies and private finance flowing back to fossil-fuel firms, all of which is likely to set back the transition. This issue might have particular relevance in emerging economies. Overall, it is important that different economic and socio-political contexts are understood to shape the scenario narrative and the key drivers relevant for certain jurisdictions. As shown above, this can be done by individual users by drawing on additional empirical evidence and expert judgement.
Based on a more detailed understanding of the chosen scenario and identification of gaps and weaknesses, the user can now consider how good a fit the chosen scenario is and whether to use it in its current form, adjust or recalibrate subject to the sophistication of the user. Specifically, it conveys how to construct more disruptive short-term scenarios through an augmentation of key assumptions to explore different sensitivities to better capture a range of possible pathways and disruptions. Further, the user is guided to assess potential systemic interaction with other scenario components to understand the relative importance of granular recalibrations.
If the initial process performed in earlier stages identifies a mismatch between the design of the selected scenario and the use case or inadequate assumptions, then some form of recalibration should be considered. This can be done by deviating from assumptions that are inconsistent with the users' believes or are simply not suitable for the given use case. For example, assumptions that are believed to be central in the probability distribution might be altered for stress testing to construct more disruptive cases that sit further in the tail.20
We can stress the assumptions made in the pathways using a range of methodologies of varying sophistication. For instance, one may question key assumptions around the mitigation potential of different geographical regions to adjust the conservativeness of assumptions in the AFOLU sector. The AFOLU sector is responsible for around 20% of global greenhouse gas (GHG) emissions. Mitigation options for the sector therefore play a significant role in most transition pathways. The assessment provided by Roe et al. (2021) provides a valuable summary that combines many strands of published research to provide a breakdown of mitigation potential by sub-sector and geography (see Figure 6). Additionally, it provides a country-level assessment of the feasibility of AFOLU emission mitigants. The primary message in Roe et al. (2021) is that the mitigation potential from the AFOLU sector significantly exceeds that assumed by IAMs such as GCAM with the global cost-effective mitigation potential estimated at 13.8 +/– 3.1 GtCO2eq yr−1 (available up to a carbon price of $100 tCO2eq−1).
Figure 6. Country feasibility and cost-effective mitigation potential as a share of total emissions. (A) Boxplot of feasibility scores by region (B) Feasibility score (0-100) by total cost-effective mitigation potential as percent of total country emissions. Circles show relative size of total cost-effective potential in GtCO2eq yr–1 (Roe et al., 2021).
An analysis can be performed by augmenting the models assuming that AFOLU emissions follow the historic trend or remain constant over the coming decades (the recalibration can be performed at the global or regional level). Based on the GCAM 5.3 projections in NGFS, assuming global AFOLU emissions remain constant would result in around 100Gt CO2 eq. of additional emissions between 2020 and 2050 in comparison to the net zero scenario pathways. To maintain the target emissions pathway this would then require, for example, a combination of suppressed energy demand and a more rapid decarbonization of the energy system. More sophisticated methods could consider greater granularity in terms of sub-sector and geography in a way that provides a better understanding of the likelihood of the various policy decisions and associated behavioral reactions. For the foreseeable future, most of the assumption deviation will need to be performed in a qualitative fashion to inform the construction of climate-related shock scenarios for financial analysis.
Depending on a user's capability, the direct economic impact and sensitivities around the assumption deviation would then need to be derived with consideration for how this affects the overall scenario narrative and its implications for financial risk. The introduction of different degrees of, for instance, technological innovation assumptions could be tested in the user's climate scenario analysis, and the impacts on different financial risk metrics could then be assessed. For instance, the speed of technological innovation is an important driver around the deployment of renewable energy and the scale of disruption across the energy system. Some studies highlight how a faster drop in the cost of renewable energy might lead to a faster uptake of renewables in the near future (Way et al., 2021). Importantly, some energy economy models still do not properly account for these issues and have failed to realistically forecast the speed of renewable energy technology advance (Farmer et al., 2015). Figure 7 below shows the valuation changes for a set of energy firms in a climate stress test across different scenarios with varying narratives, performed by Gasparini et al. (2022) based on the TRISK model by Baer et al. (2022).
Figure 7. The figure (A, B) represent a comparison of the valuation change of a selection of power firms across scenarios following a shift from a business as usual to a net zero scenario. For (A) values are benchmarked to the NGFS Net zero 2050 scenario on the horizontal axis. 45° Diagonal line represents perfect correspondence between benchmark and other scenarios. For (B) the probability density of valuation changes is depicted across scenarios. Source: Gasparini et al. (2022).
The financial risk that stems from an energy firm is therefore widely different, depending on whether technological progress and assumptions on the change in energy mix throughout the transition are taken from the NGFS, the IEA or energy-related scenarios by Way et al. (2021). Sensitivity testing such assumption deviations to which FIs are most exposed to, can enhance the robustness of results and test a variety of different future pathways, with varying levels of conservativeness around technological innovation and different beliefs around market behavior. In other words, the user can apply similar methods to test upper and lower bounds of financial risk present in her own balance sheet, when different assumptions on the speed of the decarbonization in the energy system are applied. In the light of high uncertainty on how the transition will unfold, this provides a range of the vulnerability that the user is faced with. Additionally, the user can rely on expert judgement to pin down which part of the risk range has the highest likelihood and which one lies more in the tail. Similar approaches could be undertaken to assess the impact of the augmentation of other scenario components with varying levels of conservativeness of key assumptions (e.g., introduction of labor bottlenecks, time lags between policy and different assumptions around AFOLU mitigation potential). This can help to place where relevant scenario components sit in the probability distribution of the assumed impact and in terms of credibility of materialization.
It is important to better understand the adverse effects of the augmentation of assumptions on the systemic level and how it affects the overall scenario narrative. This can be looked at through the lens of out-of-equilibrium dynamics where the system cannot immediately recover from a shock, and return to pre-shock conditions (Farmer et al., 2015; Mercure et al., 2016) or spill-over effects to other scenario components. We propose to assess such systemic feedback across two dimensions:
• Consider the impacts of adjustments across scenario components on the economic level. For instance, an implausible representation of the transition in the energy sector without accounting for a policy time lag or technological innovation constraints may have knock-on effects on other scenario components such as the scale and speed of the transition required by the industrial and mobility sector to offset this. Further, such deviations in assumptions in the set of analyzed scenario components may have common feedback effects that could amplify each other and question the feasibility of the overall scenario itself. For instance, if in the previous stage an adjustment of key assumptions was identified to result in a failure of adopted policy pathways to deliver the anticipated change (e.g., more conservative assumptions around AFOLU mitigation potential leads to emission overshoot), it may be explored which parts of the system are likely to see more dramatic policy action across the system to maintain the original target emissions pathway (e.g., the energy system).
• Consider feedbacks between the economic and the financial-system level. A change in the expectations around policy timing, or the realization of constraints to decarbonise parts of the economic system at the required speed has the potential to influence expectations across a financial network with amplification effects that limit the availability of finance when the economic system is stressed. This is of particular importance when expanding scenarios to match financial risk models.
More generally, accounting for such systemic feedbacks can be interpreted as checks and balances to help continuously keep scenarios more realistic. Academics, FIs and CB&S should engage in information exchange and keep abreast of any developments to reveal limitations across scenario components and the status of policy vs. assumptions. This can then feedback into the overall scenario generation process to keep scenarios “honest.”
As previously outlined, most scenarios leave considerable room for expansion to not only reflect disruptions and frictions, but also to match the level of granularity needed by the financial sector. The lack of granularity of scenarios most often prevents a direct financial assessment on the counterparty level. The financial industry will need to develop additional scenario related modeling capabilities as currently available scenarios are unable to provide all the information financial firms require. Note, that Koberle et al. (2021) provides a good discussion on scenario expansion. Further, the user should aim for a complete passing through of relevant extremes and information when matching scenario pathways with micro-financial layers. While we do not propose to guide the user on how to solve these modeling challenges (as these are unique to risk model frameworks used by financial institutions), we aim to provide a high-level guidance on two potential ways forward on how such a scenario expansion process could be undertaken.
For users that performed Stage II to assess underlying assumptions of chosen scenarios to better reflect sensitivities around key risk drivers, we propose that such an improved understanding is leveraged to inform the design of exogenous financial shocks.
This follows more of a shock-based logic to risk management exercises. Understanding the scenario and different beliefs around the likelihood of key assumptions allow to frame a qualitative narrative on the severity of a financial impact. Importantly, capabilities gained throughout stage II of the framework allows the user to get accustomed to extreme shock events and therefore expand the range of hypothetical scenarios further into the tails. For instance, as we illustrated, a better understanding what's driving the speed of decarbonization in the energy system, such as assumptions around technological change, allows to design scenarios that assume a sudden expectation shift around a technological breakthrough. Similarly, shining light on potential energy price volatilities as a response to current crisis, could inform the potential magnitude of transition-related energy shocks by amplifying past market volatilities. Key here is that a closer exploration of the scenarios and the driving assumptions allows to qualitatively design scenarios that capture a broader range of plausible tail-outcomes. This helps also to ground such hypothetical shock scenarios in a narrative that one can attribute likelihoods to and shape the context of realistic extreme scenarios based on beliefs around how such an event could be potentially even more disruptive than historical events, conditional on a transition narrative. Such an approach is more closely aligned to classical stress test design and the US market-based climate stress testing approaches (see e.g., Jung et al., 2021) which are rooted in financial market modeling and are less dependent on process-based IAM scenarios. This approach however does not allow to fully integrate feedbacks and financial market amplifications. However, using scenarios and augmenting key assumptions to design qualitative shocks that are integrated into classical financial frameworks are easier and more immediately feasible. This requires less expertise and capabilities in relation to modeling and hence can serve as an appropriate first step toward further developing internal climate risk management capabilities.
For users that have capabilities to run and calibrate IAMs and macro economic modeling approaches, a more sophisticated financial analysis can be undertaken. Here, users should envision to create fully integrated links between the scenario generation and the financial models. The scenario expansion process therefore entails not only to qualitatively inform an exogenous shock but to integrate financial frictions into the climate scenario analysis modeling chain. This way, more realistic and potentially disruptive scenarios for specific use cases by the financial sector can be generated through adding more granularity in the models endogenously. This way, also systemic feedback effects within the wider model are captured. Alternatively, as an initial step, the augmentation and sensitivity testing of scenario components and associated different outcomes can also be fed into financial models without a full integration of approaches (such as a partial integration of various scenarios with different assumptions into financial risk model, undertaken by Gasparini et al., 2022). Here, as opposed to the exogenous shock creation, the user still benefits from a partial scenario integration into financial analysis to test multiple pathways around key assumptions in a quantitative fashion.
The benefit of the full scenario integration is that it could also capture endogenous policy effects and investment behavior, systemic feedback mechanisms and financial network effects, as advocated by many academics, including Battiston and Monasterolo (2021). Such an approach is highly sophisticated and not yet used as it would require integrating the role of financial complexity and financial interconnectedness more broadly in relation to the economy, however, which may be fundamental to assessing the building up of bubbles and boom-bust cycles (Farmer et al., 2021). There is growing literature around financial and economic network models to analyze the far-reaching impact of climate financial risk also on a systemic level (Stolbova et al., 2018; Battiston and Monasterolo, 2021; Cahen-Fourot et al., 2021; Roncoroni et al., 2021). It is increasingly important that such systemic models are further developed by CB&S aiming to safeguard financial stability and to inform prudential and promotional financial policies (Baer et al., 2021a). Close collaboration through participative regulatory exercises is required as the behavioral aspect of how institutions react to initial shocks, and how they shift their balance sheets is a critical driver for systemic risk.
While the framework in principle focuses on transition risk, similar steps could be undertaken for physical risk considering the specifics around acute climate risk and catastrophic modeling over the shorter time horizon. It is well-known that “top-down” approaches are likely to be flawed when applied at a granular scale, and that there are risks in employing such approaches (Ranger et al., 2021; Pitman et al., 2022). Scenario expansion with bottom-up empirical research of acute physical risk follows the same logic as the scenario expansion process to construct more disruptive short-term scenarios outlined in this framework.
Over the long-term, further investment by research institutions, FIs and CB&S is likely to deliver a next generation of models and scenarios that will help to resolve some of the caveats identified in this paper. In the near-term, we propose that these parties work together to develop a common scenario taxonomy that can help FIs to interpret and use the scenarios that exist more fully.
Today, financial practitioners are largely dependent on existing third-party climate scenarios and almost entirely dependent on third party physical climate models and IAMs. The expertise in these models, and the scenarios they produce, sit predominantly in the academic community. This is a gap that needs to be bridged. The development and application of a common scenario taxonomy will provide an effective mechanism to translate academic expertise—like that presented in this paper—into practical information to support the development of climate scenario analysis in the financial sector. This complements and further supports recommendations made by CGFI research (Ranger et al., 2023). By engaging with academic experts who contribute to the construction of climate scenarios, a comprehensive account of the probability associated with each individual scenario element, key assumptions, characteristic, and features of most widely used scenarios could be provided. This could build upon the evidence and discussion laid out in previous sections. These assessments will support the matching of available scenarios to financial sector use cases, helping to inform users about residual weaknesses that need to be addressed when interpreting results and developing new scenarios.
We propose that such a scenario taxonomy could follow the typical modeling structure of the IAMs that underpin climate scenarios (see Figure 8 for illustrative overview). On the highest level, it should consider the geographical, sectoral and sub-sectoral resolution of the climate-economic sub-systems—the energy system, land-use, the climate system, and the macro economic response. A lower level could then introduce the key assumptions within these components covering dimensions such as policy intervention, technological and investment representation, and consumer behavior. At the lowest level of the taxonomy would sit the detailed description of the granular calibration assumptions, interlinkages within the respective subcomponent, and importantly the external relationships and feedbacks with other sub-components.
Figure 8. Exploratory illustration of scenario taxonomy structure. Authors visualization with elements from NGFS; our world in data and the IEA.
The structure of the taxonomy follows closely the process of our approach to scenario adjustment, where key assumptions of sub-models are identified. At a lower level this requires specific technical expertise. Individual components should be built out and explained along a common scenario taxonomy structure and subsequently be put together in a consistent manner to allow for easy interpretation by non-experts such as financial practitioners.
A taxonomy would contribute toward mitigating the implications of wrong scenario design and misuse of scenarios. As highlighted throughout this paper, evidence suggests that climate risk assessments may underestimate the climate-related financial risks, potentially incentivizing firms to interpret the relatively benign results as upper end losses due to an inadequate focus on limitations and challenges. The adoption of a common scenario taxonomy would further support better communication between climate scenario modelers, climate scientists, engineers, and financial practitioners. This may lead to financial practitioners having a better understanding of the assumptions inherent in each climate scenario, gaining a better appreciation of where each scenario sits within the probability distribution of pathways and making more effective use of scenario analysis. The taxonomy would cover the wide range of scenario providers, the detailed individual scenario components, assumptions and the associated risk drivers and feedback dynamics.
Assessing and managing climate risk is an ongoing process, with substantial learning and refinement happening continually, which was also stressed in the Bank of England's CBES results. The NGFS is to-date on its third iteration of scenarios and with each release comes substantial refinement and improvement. Yet, climate scenario analysis brings new challenges vs. traditional scenario analysis by financial institutions, particularly given the limitations, uncertainties, and trade-offs inherent in the data, models and methods for such climate financial risk assessments. We argued that all scenarios are wrong, but this does not necessarily mean that they cannot be useful if used and expanded upon with full awareness of the characteristics and limitations. In this paper, we provided practitioners with guidance on how to elucidate such scenario deficiencies, provide evidence on where current scenarios sit within the range of plausible future outcomes to aid interpretation, and propose an approach to scenario construction and expansion for key use cases.
To this end, our analysis provided practical insights into key scenario features, model characteristics that underpin scenarios, and wider scenario considerations that are important to equip financial institutions with the required knowledge on how to effectively select, use and interpret currently available scenarios and increase their suitability for decision making. We highlight that, among others, an insufficient understanding of the transition model narrative, awareness around assumption variability and IAM model simplifications, the degree to which key risk drivers are neglected, and information loss along the climate scenario modeling chain, may lead to a misuse of scenarios, institutions avoiding the active use of scenario results or using relatively benign results as justification for inaction.21 Importantly, wider considerations around where scenarios sit in terms of the level of economic stress conditional on policy action and mitigation progress is critical to judge the accuracy of climate financial risk outcomes. Misinterpretation could also cause inefficiencies in relation to a firm's capital allocation decisions throughout the transition.
Most of the identified scenario dimensions and model characteristics suggest an underestimation of the risk, potentially giving rise to a false sense of security on how the transition may unfold. These findings, while recognized by FSB and NGFS (2022), should inform upcoming climate scenario exercises to prevent misuse and absorb the lessons from previous exercises. We advocate for closer research cooperation between academics and practitioners to enhance the level of granularity that climate risk scenarios can capture. To uncover the needs of the financial sector to preform granular scenario analysis, a more sophisticated micro-level integration into existing modeling infrastructure is needed, similar to those suggested for climatic and physical risk models (Pitman et al., 2022) and the financial industry will need to develop additional scenario related modeling capabilities. We highlight that depending on the level of sophistication of the user, also a more qualitative narrative to inform the design of exogenous financial shocks, more similar to traditional risk management, may be more immediately feasible.
These findings informed our framework that identified how current scenarios can be augmented to better capture the tail risk inherent in the transition and sensitivity test multiple plausible pathways. Our framework is an initial step toward a better systematic understanding of scenarios and their tailoring to meet the needs of financial practitioners in a timely manner. Finally, we call for FIs, CB&S, and research institutions to work closely together to develop a more comprehensive scenario taxonomy to help navigate material financial risk under uncertainty.
The original contributions presented in the study are included in the article/Supplementary material, further inquiries can be directed to the corresponding author.
MB: conceptualization, methodology, investigation, writing—original draft, and writing—review and editing. NR and RL: conceptualization, methodology, investigation, and writing—review and editing. MG: methodology, investigation, and writing—review and editing. All authors contributed to the article and approved the submitted version.
The authors would like to thank David Wilkinson and Gireesh Shrimali for their useful comments on previous drafts of this paper. We also thank two reviewers for their valuable feedback.
The authors declare that the research was conducted in the absence of any commercial or financial relationships that could be construed as a potential conflict of interest.
All claims expressed in this article are solely those of the authors and do not necessarily represent those of their affiliated organizations, or those of the publisher, the editors and the reviewers. Any product that may be evaluated in this article, or claim that may be made by its manufacturer, is not guaranteed or endorsed by the publisher.
The Supplementary Material for this article can be found online at: https://www.frontiersin.org/articles/10.3389/fclim.2023.1146402/full#supplementary-material
1. ^https://www.bankofengland.co.uk/prudential-regulation/publication/2023/report-on-climate-related-risks-and-the-regulatory-capital-frameworks
2. ^See latest climate financial risk forum guide 2022—scenario analysis in financial firms by CRFR at: https://www.fca.org.uk/publication/corporate/cfrf-guide-2022-scenario-analysis-in-financial-firms.pdf.
3. ^Arguably this trend can be traced back to the prominent use of IAMs to produce mitigation pathways, supported by the scientific community and the Intergovernmental Panel on Climate Change (IPCC).
4. ^See: https://www.ngfs.net/en/communique-de-presse/ngfs-publishes-third-vintage-climate-scenarios-forward-looking-climate-risks-assessment.
5. ^Also regulatory divergences are challenging cross-border financial firms: see: https://www.ey.com/en_gl/brexit-and-financial-services/how-regulatory-divergence-is-challenging-cross-border-financial-firms.
6. ^https://www.unepfi.org/themes/climate-change/economic-impacts-of-climate-change-exploring-short-term-climate-related-shocks-with-macroeconomic-models/
7. ^Note that the term probability distribution throughout this paper is not necessarily meant in strict probabilistic terms. It can also be expressed through expert opinions and storylines in a narrative space to define more or less likely outcomes based on expert judgement, similar to IPCC AR6 that uses storylines with linguistic uncertainties.
8. ^This framework focusses on transition risk scenarios, albeit drawing upon complementary analyses of physical risk scenario (e.g., Ranger et al., 2021) where appropriate.
9. ^For the remainder of the paper, for simplicity, we broadly differentiate into two major types of use cases (strategic/business planning and stress testing) across two types of institutions (FIs and central banks/regulators).
10. ^https://www.imf.org/en/Publications/CR/Issues/2022/04/07/United-Kingdom-Financial-Sector-Assessment-Program-Systemic-Stress-and-Climate-Related-516264
11. ^https://one.oecd.org/document/ENV/EPOC/WPIEEP(2016)18/FINAL/En/pdf, (accessed June 3, 2023).
12. ^Note that this is partially explored in the NGFS divergent net-zero scenario.
13. ^https://www.unepfi.org/wordpress/wp-content/uploads/2021/02/UNEP-FI-Pathways-to-Paris.pdf
14. ^As noted, in classical financial risk management this is done by abstracting from historic volatilities and time series, which in the case of climate change and unprecedented transition is not possible.
15. ^https://www.federalreserve.gov/publications/climate-scenario-analysis-exercise-instructions.htm
16. ^https://www.cgfi.ac.uk/global-resilience-index-initiative/
17. ^We acknowledge that there is a capability gap between advanced economies with sophisticated regulatory frameworks and those that lack resources and risk management structures to fully inject the increasing complexity around financial climate risk analysis. Frameworks such as the one presented in this paper aimed to aid the interpretation and use of existing scenarios can be particularly important in these cases.
18. ^Use-cases of climate scenario analysis: https://www.frc.org.uk/getattachment/0d28d5e8-ff89-4028-88a8-49e837db6022/FRC-Climate-Scenario-Analysis-in-Corporate-Reporting_October-2021.pdf.
For Risk Management Use Cases for climate scenario analysis, see: https://www.fca.org.uk/publication/corporate/climate-financial-risk-forum-guide-2021-risk-managment-use-cases.pdf.
19. ^Ideally, as we discuss later, such a stage would be guided by a comprehensive scenario taxonomy, that brings together expertise from different academic sources to guide financial practitioners in fully understanding each assumption and the relative importance for different use-cases.
20. ^Note, UNEP FI has created some short-term disruptive scenarios by adjusting assumptions in the NIGEM model to provide high-level trajectories of macroeconomic variables across three scenarios, including a trade war and sudden policy scenario.
21. ^“Scenarios have to be contextualized as outcomes of a set of assumptions to avoid misunderstanding or cherry-picking of information. Furthermore, pathways should be connected to an assessment of consequences to allow exploring trade-offs and synergies. This requires a basic scenario literacy of the user that the service must support.” (Auer et al., 2021).
ACPR (2020). Scenarios and Main Assumptions of the ACPR Pilot Climate Exercise. Paris: Autorité de Contrôle Prudentiel et de Résolution Direction d'études et d'analyse des risques.
Aguais, S., and Forest, L. (2022). Smooth NGFS Climate Scenarios Imply Minimal Impacts on Corporate Credit Losses. Climate Change Credit Risk Triptych Draft Working Paper. Front. Clim. 5:1127479. doi: 10.3389/fclim.2023.1127479
Almeida, E., Colesanti Senni, C., and Dunz, N. (2023). Building Blocks for Central Banks to Develop Nature Scenarios. The Inspire Sustainable Central Banking Toolbox Policy Briefing Paper, 11. London: LSE INSPIRE Network.
Andersson, M., Bolton, P., and Samama, F. (2016). Hedging climate risk. Financ. Anal. J. 72, 13–32. doi: 10.2469/faj.v72.n3.4
Asefi-Najafabady, S., Villegas-Ortiz, L., and Morgan, J. (2021). The failure of Integrated Assessment Models as a response to ‘climate emergency' and ecological breakdown: the Emperor has no clothes. Globalizations 18, 1178–1188. doi: 10.1080/14747731.2020.1853958
Auer, C., Kriegler, E., Carlsen, H., Kok, K., Pedde, S., Krey, V., et al. (2021). Climate change scenario services: from science to facilitating action. One Earth 4, 1074–1082. doi: 10.1016/j.oneear.2021.07.015
Baer, M., Caldecott, B., Kastl, J., Kleinnijenhuis, A. M., and Ranger, N. (2022). TRISK—A Climate Stress Test for Transition Risk SSRN. Available online at: https://papers.ssrn.com/sol3/papers.cfm?abstract_id=4254114 (accessed June 3, 2023).
Baer, M., Campiglio, E., and Deyris, J. (2021a). It takes two to dance: institutional dynamics and climate-related financial policies. Ecol. Econ. 190, 107210. doi: 10.1016/j.ecolecon.2021.107210
Baer, M., Kastl, J., Kleinnijenhuis, A., Thomae, J., and Caldecott, B. (2021b). The cost for the financial sector if firms delay climate action. Rep. Clim. Stress Test. Scenar. Proj. Oxf. Sustain. Finance Group Univ. Oxf. 2 Investing Initiat. 40, 95645. doi: 10.2139/ssrn.4095645
Bank of England (2021). Discussion Paper: The 2021 Biennial Exploratory Scenario on the Financial Risks From Climate Change. Discuss. Pap., 33. London.
Battiston, S., and Monasterolo, I. (2021). On the Dependence of Investor's Probability of Default on Climate Transition Scenarios, 25. SSRN. Available online at: https://papers.ssrn.com/sol3/papers.cfm?abstract_id=3743647
Behnam, C. R., and Litterman, B. (2020). Managing Climate Risk in the U.S. Financial System, 196. New York: U.S. Commodity Futures Trading Commission.
BIS (2009). Principles for Sound Stress Testing Practices and Supervision. Basel: Basel Committee on Banking Supervision; Bank for International Settlements.
BIS (2020). The Green Swan—Central Banking and Financial Stability in the Age of Climate Change. Basel.
BoE (2019). The 2021 Biennial Exploratory Scenario on the Financial Risks From Climate Change (Discussion Paper). London: Bank of England.
Bolton, P., Despres, M., Pereira da Silva, L. A., Samama, F., and Svartzman, R. (2020). The Green Swan. Central Banking and Financial Stability in the Age of Climate Change. Bank for International Settlement, Basel, Switzerland.
Bolton, P., and Kacperczyk, M. (2021). Do Investors Care about Carbon Risk? 81. USA: Journal of Financial Economics.
Brock, W. A., and Hansen, L. P. (2017). Wrestling with uncertainty in climate economic models. SSRN Electron. J. 2017, 3008833. doi: 10.2139/ssrn.3008833
Cahen-Fourot, L., Campiglio, E., Godin, A., Kemp-Benedict, E., and Trsek, S. (2021). Capital Stranding Cascades: The Impact of Decarbonisation on Productive Asset Utilisation (Research Papers No. 204). Paris: Agence Française de Développement. doi: 10.1016/j.eneco.2021.105581
CGFI (2022). Learning From the 2021/22 Climate Biennial Exploratory Scenario Exercise. London: UK CGFI, Climate Financial Risk Forum and GARP.
Chenet, H., Ryan-Collins, J., and van Lerven, F. (2019). Climate-related financial policy in a world of radical uncertainty: towards a precautionary approach. SSRN Electron. J. 2019, 3520224. doi: 10.2139/ssrn.3520224
Dasgupta, P. (2021). The Economics of Biodiversity: The Dasgupta Review: Full Report, Updated: 18 February 2021. ed H. M. Treasury. London: HM Treasury.
ECB (2021). ECB Economy-Wide Climate Stress Test: Methodology and Results. Frankfurt: Publications Office, LU.
Eren, E., Merten, F., and Verhoeven, N. (2022). Pricing of Climate Risks in Financial Markets: A Summary of the Literature. BIS Papers No 130. Basel: Bank for International Settlements.
ESRB (2020). Positively green: measuring climate change risks to financial stability. Rep. Eur. Syst. Risk Board 56.
Farmer, J. D., Hepburn, C., Mealy, P., and Teytelboym, A. (2015). A third wave in the economics of climate change. Environ. Resource. Econ. 62, 329–357. doi: 10.1007/s10640-015-9965-2
Farmer, J. D., Kleinnijenhuis, A. M., and Wetzer, T. (2021). Stress testing the financial macrocosm. SSRN Electron. J. 2021, 3913749. doi: 10.2139/ssrn.3913749
Fiedler, T., Pitman, A. J., Mackenzie, K., Wood, N., Jakob, C., and Perkins-Kirkpatrick, S. E. (2021). Business risk and the emergence of climate analytics. Nat. Clim. Change. 2021, 6. doi: 10.1038/s41558-020-00984-6
FSB and NGFS (2022). Climate Scenario Analysis by Jurisdictions: Initial Findings and Lessons, 52. Basel.
Gasparini, M. (2023). Stock Valuation and Climate Change Uncertainty: Is There a Carbon Bubble? Oxford: Insitute for New Economic Thinking.
Gasparini, M., Baer, M., and Ives, M. C. (2022). A Re-evaluation of the Financial Risks of the Net Zero Transition. SSRN. Available online at: https://papers.ssrn.com/sol3/papers.cfm?abstract_id=4254054 (accessed June 3, 2023).
Green, J. F. (2021). Does carbon pricing reduce emissions? A review of ex-post analyses. Environ. Res. Lett. 16, 43004. doi: 10.1088/1748-9326/abdae9
Hepburn, C., O'Callaghan, B., Stern, N., and Zenghelis, D. (2020). Will COVID-19 Fiscal Recovery Packages Accelerate or Retard Progress on Climate Change? Oxf. Smith Sch. Enterp. Environ. Work. Pap. No 20-02 ISSN 2732-4214 48. Oxford: Oxford's Smith School of Enterprise and the Environment.
IPCC (2015). Climate Change 2014: Migration of Climate Change. Cambridge University Press. doi: 10.1017/CBO9781107415416
Jung, H., Engle, R. F., and Berner, R. (2021). Climate stress testing. SSRN Electron. J. 2021, 3931516. doi: 10.2139/ssrn.3931516
Kemp, L., Xu, C., Depledge, J., Ebi, K. L., Gibbins, G., Kohler, T. A., et al. (2022). Climate endgame: exploring catastrophic climate change scenarios. Proc. Natl. Acad. Sci. U. S. A. 119, e2108146119. doi: 10.1073/pnas.2108146119
Koberle, A., Ganguly, G., and Ostrovnaya, A. (2021). A Guide to Building Climate-Financial Scenarios for Financial Institutions, 23. London: Imperial College London.
Kolev, A., Riess, A.-D., Zachmann, G., and Calthrop, E. (2012). “Investment and growth in the time of climate change,” in Annual Economic Conference and Publication. Luxembourg: European Investment Bank (EIB).
Lankhuizen, M., Diodato, D., Weterings, A., Ivanova, O., and Thissen, M. (2022). Identifying labour market bottlenecks in the energy transition: a combined IO-matching analysis. Econ. Syst. Res. 2022, 1–26. doi: 10.1080/09535314.2022.2048294
Lawson, J., Moss, A., Popa, A., Cairns, E., and Mackenzie, C. (2023). A Bespoke, Probabilistic Approach to Climate Scenario Analysis. London: CEPR Press Discuss. Pap. No 17944 Httpsceprorgpublicationsdp17944.
McKinsey Global Institute. (2022). The Net-Zero Transition: What it Would Cost, What it Could Bring. Available online at: https://www.mckinsey.com/capabilities/sustainability/our-insights/the-net-zero-transition-what-it-would-cost-what-it-could-bring
Mercure, J.-F., Knobloch, F., Pollitt, H., Paroussos, L., Scrieciu, S. S., and Lewney, R. (2019). Modelling innovation and the macroeconomics of low-carbon transitions: theory, perspectives and practical use. Clim. Policy 19, 1019–1037. doi: 10.1080/14693062.2019.1617665
Mercure, J.-F., Pollitt, H., Viñuales, J. E., Edwards, N. R., Holden, P. B., Chewpreecha, U., et al. (2018). Macroeconomic impact of stranded fossil fuel assets. Nat. Clim. Change 8, 588–593. doi: 10.1038/s41558-018-0182-1
Mercure, J.-F., Salas, P., Vercoulen, P., Semieniuk, G., Lam, A., Pollitt, H., et al. (2021). Reframing incentives for climate policy action. Nat. Energy 6, 1133–1143. doi: 10.1038/s41560-021-00934-2
Mercure, J. -F., Pollitt, H., Bassi, A. M., Vinuales, J. E., and Edwards, N. R. (2016). Modelling complex systems of heterogeneous agents to better design sustainability transitions policy. Glob. Environ. Chang. 37, 102–115. doi: 10.1016/j.gloenvcha.2016.02.003
Monasterolo, I., Nieto, M. J., and Schets, E. (2022). The good, the bad and the hot house world: conceptual underpinnings of the NGFS scenarios and suggestions for improvement. SSRN Electron. J. 2022, 4211384. doi: 10.2139/ssrn.4211384
NGFS (2020). NGFS Climate Scenarios for Central Banks and Supervisors. Paris: Network for Greening the Financial System.
Pitman, A. J., Fiedler, T., Ranger, N., Jakob, C., Ridder, N. N., Perkins-Kirkpatrick, S. E., et al. (2022). Acute climate risks in the financial system: examining the utility of climate model projections. Environ. Res. Clim. 2022, ac856f. doi: 10.1088/2752-5295/ac856f
Pollitt, H., and Mercure, J.-F. (2017). The role of money and the financial sector in energy-economy models used for assessing climate and energy policy. Clim. Policy 18, 1–14. doi: 10.1080/14693062.2016.1277685
Ranger, N., Clacher, I., and Bloomfield, H. (2023). Learning From the 2021/2022 Climate Biennial Exploratory Scenario (CBES) Exercise in the UK. London: UK Centre for Greening Finance and Investment (CGFI).
Ranger, N., Mahul, O., and Monasterolo, I. (2021). Assessing the Physical Climate-Related Financial Risks for Acute Climate Shocks: A Framework for Scenario Generation. Oxford: UK Centre for Greening Finance and Investment (CGFI).
Rockström, J., Steffen, W., Noone, K., Persson, Å., Chapin, F. S., Lambin, E. F., et al. (2009). A safe operating space for humanity. Nature 461, 472–475. doi: 10.1038/461472a
Roe, S., Streck, C., Beach, R., Busch, J., Chapman, M., Daioglou, V., et al. (2021). Land-based measures to mitigate climate change: potential and feasibility by country. Glob. Change Biol. 27, 6025–6058. doi: 10.1111/gcb.15873
Roncoroni, A., Battiston, S., Escobar Farfàn, L. O. L., and Martinez Jaramillo, S. (2021). Climate Risk and Financial Stability in the Network of Banks and Investment Funds (SSRN Scholarly Paper No. ID 3356459). Rochester, NY: Social Science Research Network.
Smith, S. M., Geden, O., Nemet, G. F., Gidden, M. J., Lamb, W. F., Powis, C., et al. (2023). The State of Carbon Dioxide Removal, 1st Edn. Oxford: Oxford's Smith School of Enterprise and the Environment.
Stern, N., Stiglitz, J., and Taylor, C. (2021). The Economics of Immense Risk, Urgent Action and Radical Change: Towards New Approaches to the Economics of Climate Change (No. w28472). Cambridge, MA: National Bureau of Economic Research.
Stolbova, V., Monasterolo, I., and Battiston, S. (2018). A financial macro-network approach to climate policy evaluation. Ecol. Econ. 149, 239–253. doi: 10.1016/j.ecolecon.2018.03.013
UNEP FI (2022). The Closing Window: Climate Crisis Calls for Rapid Transformation of Societies: Emissions Gap Report 2022, 65. Genéve.
van de Ven, D. J., and Fouquet, R. (2017). Historical energy price shocks and their changing effects on the economy. Energy Econ. 62, 204–216. doi: 10.1016/j.eneco.2016.12.009
van der Ploeg, F., and Rezai, A. (2020). Stranded assets in the transition to a carbon-free economy. Annu. Rev. Resour. Econ. 12, 281–298. doi: 10.1146/annurev-resource-110519-040938
Way, R., Ives, M. C., Mealy, P., and Farmer, J. D. (2021). Empirically Grounded Technology Forecasts and the Energy Transition, 23. Oxford: INET Oxford.
Keywords: financial supervision, climate change, stress testing, risk management, scenario analysis, financial risk, sustainable finance
Citation: Baer M, Gasparini M, Lancaster R and Ranger N (2023) “All scenarios are wrong, but some are useful”—Toward a framework for assessing and using current climate risk scenarios within financial decisions. Front. Clim. 5:1146402. doi: 10.3389/fclim.2023.1146402
Received: 17 January 2023; Accepted: 26 June 2023;
Published: 14 July 2023.
Edited by:
Geoff Darch, Anglian Water Services, United KingdomReviewed by:
Haoqi Qian, Fudan University, ChinaCopyright © 2023 Baer, Gasparini, Lancaster and Ranger. This is an open-access article distributed under the terms of the Creative Commons Attribution License (CC BY). The use, distribution or reproduction in other forums is permitted, provided the original author(s) and the copyright owner(s) are credited and that the original publication in this journal is cited, in accordance with accepted academic practice. No use, distribution or reproduction is permitted which does not comply with these terms.
*Correspondence: Moritz Baer, bW9yaXR6LmJhZXJAc21pdGhzY2hvb2wub3guYWMudWs=
Disclaimer: All claims expressed in this article are solely those of the authors and do not necessarily represent those of their affiliated organizations, or those of the publisher, the editors and the reviewers. Any product that may be evaluated in this article or claim that may be made by its manufacturer is not guaranteed or endorsed by the publisher.
Research integrity at Frontiers
Learn more about the work of our research integrity team to safeguard the quality of each article we publish.