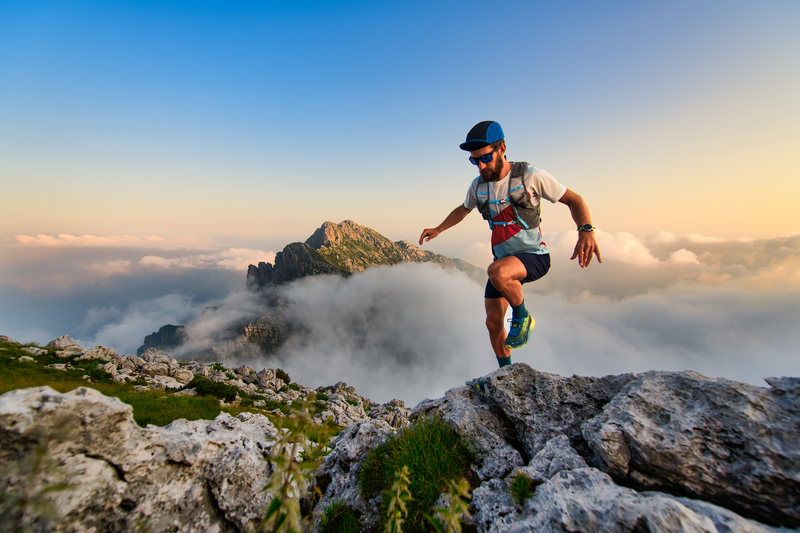
95% of researchers rate our articles as excellent or good
Learn more about the work of our research integrity team to safeguard the quality of each article we publish.
Find out more
REVIEW article
Front. Clim. , 13 June 2023
Sec. Climate and Decision Making
Volume 5 - 2023 | https://doi.org/10.3389/fclim.2023.1129378
This article is part of the Research Topic Decision Making for the Net Zero Transformation: Considerations and New Methodological Approaches View all 11 articles
Moving to a low carbon society requires pro-active decisions to transform social and physical systems and their supporting infrastructure. However, the inherent complexity of these systems leads to uncertainty in their responses to interventions, and their critical societal role means that stakes are high. Techniques for decision making under deep uncertainty (DMDU) have recently begun to be applied in the context of transformation to a low carbon society. Applying DMDU to support transformation necessitates careful attention to uncertainty in system relationships (structural uncertainty), and to actions targeting deep leverage points to transform system relationships. This paper presents outcomes of a structured literature review of 44 case studies in which DMDU is applied to infrastructure decisions. Around half of these studies are found to neglect structural uncertainty entirely, and no study explicitly considers alternative system conceptions. Three quarters of studies consider actions targeting only parameters, a shallow leverage point for system transformation. Where actions targeting deeper leverage points are included, models of system relationships are unable to represent the transformative change these interventions could effect. The lack of attention to structural uncertainty in these studies could lead to misleading results in complex and poorly understood systems. The lack of interventions targeting deep leverage points could lead to neglect of some of the most effective routes to achieving transformative change. This review recommends greater attention to deeper leverage points and structural uncertainty in applications of DMDU targeting transformative change.
Mitigating and adapting to climate change requires the transformation of a complex set of interacting social and physical systems, and the infrastructure which supports them (Boardman and Sauser, 2006; IPCC, 2014; Abson et al., 2017; Rogelj et al., 2018). Bringing about this transformation necessitates urgent decision making. However, these systems are complex, leading to a high degree of uncertainty in their response to interventions, whilst the critical role they play in the functioning of society means that there is little room for error (Pye et al., 2018; Roelich, 2020). This has led to calls to move beyond an approach to planning based on optimality in a predicted future, to one which focuses on decisions which perform well across various possible futures (Lyons and Marsden, 2019). Techniques labeled “decision making under deep uncertainty” (DMDU) have been proposed as useful to guide system transformation toward a low carbon society in this context (Gambhir et al., 2019; Groves et al., 2019; Marchau et al., 2019a). This section describes DMDU, introduces concepts of complexity and transformation as referred to in this paper, and introduces research questions around the use of DMDU techniques to catalyze the transformation of complex systems.
DMDU methods differ in terminology and detailed steps, but typically involve a process broadly in line with that schematised in Figure 1. Decisionmakers and analysts agree a set of objectives (usually represented by quantitative metrics, M), identify potential actions (sometimes referred to as “policy levers,” L) which could help achieve these objectives, and articulate of critical uncertainties (X) which may support or undermine their achievement, (sometimes considered in terms of uncertain times at which tipping points are reached) (Walker et al., 2013; Marchau et al., 2019a). One or more set(s) of system relationships (R) are defined, which map values of uncertain parameters and actions to metrics. These relationships are often embedded in a computer model. Finally, an exploration of parameter space around uncertainties with a given set of policies is performed. This process is repeated to identify a set of actions which meet objectives sufficiently well across the range of uncertainties. In some cases, longer term actions are included which are triggered only in certain circumstances, and different DMDU methods place different emphasis on the reshaping of plans in response to emerging evidence.
Figure 1. Schematic indicating the core processes involved in DMDU. After Marchau et al. (2019a).
DMDU methods have become established techniques to explore how a system could become more resilient to external threats (Marchau et al., 2019a). Amongst other applications, DMDU has been used to help protect cities from sea level rise and river flooding (Babovic et al., 2018; Sriver et al., 2018), ensure adequate supply of drinking water (Herman et al., 2014), in military operations (Lempert, 2019), and to manage supply chains under uncertain economic conditions (Kotta, 2018).
Applications of these methods to system transformation are relatively nascent. However, several recent studies have focussed on their use in supporting transitions in aspects of a system (i.e., smaller changes in constituent parts of the overall system) in support of an overall system transformation to reduce carbon emissions, particularly in a transport context. For example, Hidayatno et al. (2020) use exploratory modeling and analysis to explore policies to drive a transition from petrol to natural gas vehicles in Jakarta, Indonesia, and Lempert et al. (2020) use Robust Decision Making (RDM) to consider policies to bring about a reduction in private travel and a switch to electric vehicles in Sacramento, USA. In a broader context. Groves et al. (2019) report an application of RDM to inform a Green Climate Fund aiming to coordinate global investments to help countries transition from fossil energy technologies toward sustainable energy technologies.
However, in how they have been applied so far, it is unclear whether DMDU methods are well-suited to support decision making to enable system transformation. Bloemen et al. (2019) recognize a tendency for plans emerging from DMDU methods to focus on “incremental” or “protective” measures in the short-term, leaving firmer measures to the mid-term and system-changing interventions or transformational measures to the longer term. Bloemen et al. (2019) indicate that this may lead to “an increase in sunk costs, further increasing the threshold for switching from an incremental strategy to a transformational strategy,” whilst “due to climate change, transformational measures are inevitable in the long term.” Similar considerations led Marchau et al. (2019b) to describe “preparing a switch from incremental to transformational interventions” as one of DMDU's two most prominent challenges. Bojórquez-Tapia et al. (2022) indicate that DMDU must engage with normative and enabling approaches to substantively contribute to deliberate sustainability transformations.
There are two key areas that warrant particular attention in the use of DMDU techniques to guide system transformation and have not been well-explored so far. First, transformation is a complex process, and analysis seeking to effectively support transformative change should devote attention to the complexity of the system undergoing this transformation. Second, there is a body of literature devoted to identifying of techniques to enable system transformation. This literature is seldom referred to in literature on DMDU, but can help to inform approaches in support of transformative change.
The term “transformation” is used in different ways amongst different communities. This paper follows Feola (2015)'s definition of transformation as a major, fundamental change in the patterns, elements and interrelations of a system. The system transformation associated with moving to a low carbon society is conceptualized as having emergent characteristics associated with complex properties of the system in question, but also as having capacity for deliberate intervention to guide pace and outcomes. This overall system transformation is conceptualized as capable of being supported by smaller changes in constituent parts of the overall system, referred to as “transitions” in this paper (e.g., the transition from private to public transport). Simultaneously, we recognize that actions taken in support of wider system transformation may sometimes differ, or even be at odds with those supporting individual transitions within that system (e.g., incentivising electrification of private travel at the expense of public transport).
The concept of “complexity” is key to this definition of transformation. This too is challenging to unambiguously define, but implies an openness to multiple interpretations of system characteristics. Mitchell (2009, p. 13) offers a definition of a complex system as one that “exhibits nontrivial emergent and self-organizing behaviors.” In complex systems, there is no autonomous control over the whole system, and self-organized emergent behavior arises that cannot be predicted by understanding each of the component elements separately (Bale et al., 2015).
For systems of sufficient complexity, appropriate conceptual models describing relationships are intrinsically uncertain. This uncertainty could manifest itself in disagreement amongst stakeholders on appropriate models, but could also be collectively acknowledged by a group of stakeholders with knowledge of a complex system. In such cases, a positivist approach which aims for a single model accurately and objectively describing system relationships, is unrealistic (Lee, 1973; Sovacool et al., 2018). Further, overreliance on a single model of relationships risks allowing incumbent perspectives to dominate, which will tend to prevent transformative change (Süsser et al., 2021; Royston et al., 2022). In complex systems, an interpretivist approach, which acknowledges that different conceptions of the system of relationships are valid and explores multiple of these, is more appropriate (Funtowicz and Ravetz, 1993). Related literature on triple-loop thinking distinguishes complicated problems, for which emphasis should be placed on understanding causality within systems (double-loop thinking), with complex problems, for which emphasis should be placed on multiple interpretations of systems and on transforming systems to operate in new ways (triple-loop thinking) (Gupta, 2016; Tamarack Institute, 2020). Kwakkel et al. (2016) highlight that this can be a challenge in RDM, where a model of the system is often developed or decided upon in the initial scoping phase of the study, which is hard and expensive to revise. As such, RDM studies often effectively assume “substantial consensus among decision makers and stakeholders on the system under study” (Kwakkel et al., 2016).
Multiple conceptual models are unlikely to fully capture structural uncertainty associated with a complex system, but would represent an advance on one conceptual model. In this context, it is informative to consider examples of best practice in climate and energy systems. In climate modeling, the Coupled Model Intercomparison Project endorses 21 separate model intercomparison projects. Each of these address different aspects of the climate system using a wide variety of climate models maintained by different research groups (Eyring et al., 2016). In energy modeling, Murray et al. (2018) compare outcomes of sixteen distinct energy models to inform technology and climate policy strategies for greenhouse gas reductions in the U.S. electric power sector. These models differed in their assumptions around economic processes, interactions between timeframes, sectors, degree of foresight, technologies, and regions considered.
Comparison of large numbers of models like those of Eyring et al. (2016) and Murray et al. (2018) are resource intensive, and unlikely to be viable for decisions where stakeholders are constrained (Kwakkel et al., 2016). However, other studies represent structural uncertainty through smaller numbers of models representing diverse conceptions of a system. Pruyt and Kwakkel (2014) use three distinct system dynamics models to study radicalization in the Netherlands. Moallemi et al. (2017) model six normative contexts for transition to solar in the Indian electricity sector (government/market led, and equity/security/sustainability driven). Kwakkel et al. (2013) compare bottom-up and top-down models of global copper demand.
Central to the conceptualization of relationships to characterize a system transformation are leverage points. Leverage points represent “places within a complex system… where a small shift in one thing can produce big changes in everything” (Meadows, 1999, p. 1). Within the framework of systems thinking, Meadows (1999) develops an influential hierarchy of leverage points for system transformation. Interventions targeting deeper leverage points are generally considered more able to effect deep changes in the system (Meadows, 1999; Abson et al., 2017; Fischer and Riechers, 2019) and hence support transformation. These leverage points target different parts of the system; in order of increasing depth: altering rewards and material flows, changing processes and feedbacks, redefining goals and information flows, and changing mindsets and paradigms (schematised in Figure 2). Meadows (1999) notes that most policies target shallow leverage, while deeper leverage points are considered more challenging to access, owing to self-stabilizing tendencies within established systems. As such, explicit consideration of actions targeting deeper leverage points, and use of models capable of representing the possible impact of interventions at these deeper leverage points, are critical for decision making for transformative system change.
Figure 2. Leverage points for system transformations arranged according to their depth and system characteristics which they target. After Abson et al. (2017), Fischer and Riechers (2019) and Meadows (1999).
The common definition of deep uncertainty used by DMDU practitioners is an apt description of the situation facing decision makers seeking to catalyze system transformation to achieve a low carbon society. Lempert et al. (2003, p. 3–4) define deep uncertainty as a situation where “analysts do not know, or the parties to a decision cannot agree on, (1) the appropriate conceptual models that describe the relationships among the key driving forces that will shape the long-term future, (2) the probability distributions used to represent uncertainty about key variables and parameters in the mathematical representations of these conceptual models, and/or (3) how to value the desirability of alternative outcomes.” Following Marchau et al. (2019a), the first of these is referred to here as “structural uncertainty,” relating to how the system responds to external developments. The second is referred to as “scenario uncertainty,” relating to external developments to which the system responds.
The balance of attention between the three elements of deep uncertainty in practical applications of DMDU is unclear. The concept of “structural uncertainty” could, in principle, encompass the exploration of multiple system conceptions appropriate for complex systems. However, emphasis is often placed on uncertainty in parameter ranges rather than the structure of relationships. Further, the extent to which DMDU studies include actions which target deep leverage points for system transformation is unclear. Where actions targeting deep leverage points are included in DMDU, It is unclear whether models are of sufficient complexity to represent the transformations these actions could bring. If DMDU is to be used more extensively to guide decisions toward system transformation, a review of past DMDU studies using these methods in terms of their representation of system complexity, and their consideration of deep leverage points for transformative change, is timely. This paper presents such a review.
This paper examines the extent to which previous infrastructure DMDU case studies:
1. Represent system complexity by including multiple conceptions of system relationships (models).
2. Include actions which target deep leverage points for system transformation.
3. Include mechanisms within models of system relationships to represent the transformative potential of deep leverage points.
This is achieved through a structured literature review. Following the approach taken in a recent review of ways in which institutional, organizational, and individual context are taken into account in DMDU case studies (Bonjean Stanton and Roelich, 2021), the scope of analysis is limited to case studies focussing on the infrastructure sector. This is because of the urgent decision making required to transform infrastructure to achieve a low carbon society, and the particularly high stakes associated with infrastructure decision making (Roelich, 2020).
Selected documents are analyzed across the three guiding questions, firstly the extent to which they consider structural uncertainty. In considering the extent to which structural uncertainty is included, we initially sought to make use of the framework presented in Marchau et al. (2019a). This distinguishes uncertainty in relationships across five levels: a single deterministic model, a single stochastic model, a few alternative models, many alternative models, and an unknown system model. However, we found classifying studies on the basis of this framework impractical. The first two categories imply that the system is well-understood, and the final category precludes analysis. The majority of studies which did account for structural uncertainty included a range of conceivable values for at least one structural parameter, implying many alternative models, but ones which do not vary substantially in structure.
Instead, we developed a new framework to assess the extent to which structural uncertainty is addressed based upon where in the model structural uncertainty is represented (schematised in Figure 4). In some cases, structural uncertainty is not represented at all. At a basic level, uncertainty is represented in a parameter defining a characteristic of one or more relationships (e.g., the strength of a relationship or length of a time delay). At a deeper lever, uncertainty is considered in the functional form of relationships. Finally, at a deepest level, uncertainty is considered in terms of differing sets of key variables, and/or differing sets of connections between key variables. We equate this deepest level of uncertainty with the representation of multiple conceptions of relationships within the system, examplifioed by references in the introduction to this paper (Kwakkel et al., 2013; Pruyt and Kwakkel, 2014; Eyring et al., 2016; Moallemi et al., 2017; Murray et al., 2018).
We recognize there are limitations in this framework. In some cases parameter changes relating to structural properties of a system may transform relationships between other variables in the system, and may have a drastic influence on system behavior [see, for example, Meadows (2009, p. 51–58)]. However, we would argue that these still represent differences within one conceptual model of the system, and consider that the framework remains informative for assessing the ways in which structural uncertainty is included in considered case studies. As noted in the introduction, multiple conceptual models are unlikely to fully capture structural uncertainty associated with a complex system, but represent an advance on use of a single conceptual model.
The final two guiding questions are addressed in terms of leverage points for system transformation (see Figure 2) accessible by actions considered in studies, both in principle, and within the system models considered in those studies. Case studies are classified in terms deepest leverage point that actions in considered studies could access, and the extent to which system models considered in those studies allow this action to transform the system.
Whilst the transport sector does not represent the largest in our sample, we consider that it warrants special attention. This is due to the large scale of required transformation in this sector, the manifold societal and physical challenges associated with this (Rogelj et al., 2018), the recent emergence of DMDU studies related to transformation of this sector (Milkovits et al., 2019; Hidayatno et al., 2020; Lempert et al., 2020), and recent recommendation of DMDU methods to support ambitious transport goals in the face of “intense, large-scale, and increasingly fast-paced change” (Lempert et al., 2022). For case studies in the transport sector, diversity of motivation for studies, of model choice, and ways in which the motivation informs model choice is examined.
Documents are selected using a structured and systematic search approach in Google Scholar. The document selection process is carried out in three steps: (1) searching for documents in Google Scholar using different keyword combinations; (2) screening of returned documents; (3) collation and analysis of the results from the subset of included documents. Google Scholar is chosen here because it searches across articles, theses, books, abstracts and other academic texts returning primarily peer-reviewed documents but also non-peer-reviewed documents like projects reports, conference and working papers. This is important for this topic because many case studies applying DMDU methods are conducted by non-academic institutions and published outside of standard academic routes.
In step 1, an initial set of documents is defined through Google Scholar searches. The same combination of keywords is used in each Google Scholar search. i.e., “deep uncertainties” AND [DMDU method] AND [infrastructure sector] and “case study,” where [DMDU method] is successively Robust Decision Making, Dynamic Adaptive Planning, Dynamic Adaptive Policy Pathways, Info-Gap Decision Theory, Engineering Options Analysis, and Exploratory Modeling and [infrastructure sector] is successively water, power and energy, transport, telecommunications and waste. The first five DMDU methods are selected following those selected in Marchau et al.'s (2019a) comprehensive book on DMDU methods. “Exploratory Modeling” represents one of the foundational elements of RDM (Bankes, 1993; Lempert et al., 2003). However, in some cases this method is used to inform decision making under uncertainty outside of the RDM framework, warranting its inclusion here. The combined Google Scholar searches yield a total of 3,127 documents (including duplicates).
In step 2, documents are screened according to the five inclusion criteria in Figure 3. “Adaptation Pathways,” a predecessor of “Dynamic Adaptive Policy Pathways,” is included alongside the six DMDU methods already mentioned in search terms. The inclusion criteria “address a real word case study” is added to ensure considered studies target real world rather than hypothetical problems. The selection criterion “include analysis of candidate actions” results in the exclusion of a number Exploratory Modeling studies, which explore uncertainty without explicitly informing actions and decisions. The requirement that actions should be analyzed through a computational model is necessary to address the first and third research questions on the nature of modeled relationships.
Finally, in step 3, the selected case studies are analyzed in depth. The sector(s), DMDU method and overall focus of each study are identified, and each study is classified according to the three guiding research questions. A spreadsheet containing bibliographic details of each considered study, and summarizing findings relating to each, is included in Supplementary material.
The number of considered studies by sector is shown in Table 1. Whilst the majority of studies (34 of 44) relate to water, a significant minority (12 of 44) focus on transport. Only two studies relates to the power sector alone, and a few studies relate to two sectors (4 of 44 on transport and water, and 2 of 44 on power and water, included in both categories in Table 1). No study was identified focussing on telecommunications or waste.
Studies relating to water and power typically address resilience, whilst those relating to transport are more diverse in their objectives. Amongst studies relating to water, almost all relate to ensuring adequate water supply and/or resilience of infrastructure to increased flooding and sea level rise. Studies in the transport sector focus on fuel switching and sustainable transport policy in urban context (Milkovits et al., 2019; Hidayatno et al., 2020; Lempert et al., 2020), modal shift in inter-city transport (Hadjidemetriou et al., 2021), airport and aircraft manufacture capacity (Kwakkel et al., 2010, 2012), and sea port throughput (Halim et al., 2016). The studies focusing on power alone relate to decarbonisation of the EU power sector (Hamarat et al., 2014) and rehabilitation or replacement of a coal power plant (Bonzanigo and Kalra, 2014). The studies focusing on water and power both relate to robust investment strategies for hydropower dams (Hurford, 2016; Swanson et al., 2019). The three studies focusing water and transport all relate to resilience of transport infrastructure to flooding across geographical contexts (Rozenberg et al., 2017b; Espinet et al., 2018; Sriver et al., 2018).
The number of considered studies by DMDU method is shown in Table 2. Just over half of studies use RDM or Multi-Objective Robust Decision Making (MORDM), with a relatively wide spread of methods across other studies. The dominance of RDM and MORDM here is probably partly a result of our limiting of scope to studies using a computational model of relationships in the system. Whilst each DMDU method can be used with a quantitative model, RDM's focus on exploration of parameter space to find robust strategies makes quantitative modeling particularly central to this DMDU method.
This section examines the extent to which the selected case studies explicitly consider alternative conceptions of system relationships, and, where they do not, the extent to which structural uncertainty is considered.
None of the 44 case studies included in this paper explicitly considers alternative models of the relationships within the system. This seems surprising, given that the first unknown in the widely accepted definition of “deep uncertainty” is inability to agree on models to represent system relationships (Lempert et al., 2003; Marchau et al., 2019a).
All considered studies (44 of 44) include some form of scenario uncertainty (relating to external system developments) and around half (21 of 44) also include some form of structural uncertainty. One study makes a limited attempt to explicitly consider more than one structure of relationships (Hall and Murphy, 2012). Four studies consider more than one functional form for one or more relationships (Rozenberg et al., 2017a; Zarekarizi et al., 2020; Jaiswal et al., 2021; Ciullo et al., 2022). The remainder of studies include structural uncertainty only in terms of parameter ranges. These findings are summarized in Figure 4, and each set of studies is discussed in more detail below.
Figure 4. Scenario and structural uncertainties come in different forms. DMDU studies consider a subset of these.
The single study that considers multiple structures of relationships is limited in that each of these structures is developed in a similar manner. In a Monte Carlo approach, Hall and Murphy (2012) consider the implications of multiple hydrological models for the relationship between precipitation and water supplies. However, these models are all generated using parameter values randomly generated from the same ranges. As such, they could be seen as representing outcomes from exploring uncertain parameter within the set of relationships, rather than representing different structures of relationships associated with different understandings of a complex system.
The four studies that include different functional forms do so in contrasting ways. Considering resilience of Peru's road network to flooding events, Rozenberg et al. (2017b) use three separate functional forms for (i) the duration of disruption, and (ii) the share of traffic redirected, at different water levels. These are based upon “optimistic,” “pessimistic,” and “intermediate” expert assessments informed by historical evidence from a single highway. Considering water resource planning in Chhattisgarh, India, Jaiswal et al. (2021) use climate projections from three separate models as inputs. However, these inputs are all fed into the same series of models from that point forward, so much of the structural uncertainty is in the generation of inputs to their model, rather than within the model itself.
Considering decision making around house elevation to avoid flood damage in Pennsylvania, USA, Zarekarizi et al. (2020) use multiple probability distribution functions (PDFs) for a number of uncertain variables. This use of PDFs is atypical amongst DMDU cases, since the definition of deep uncertainty includes a condition where analysts cannot agree on an appropriate probability distribution of key variables (Lempert et al., 2003). Effectively, the PDF for the latter set of parameters is treated as an uncertainty with distinct possible forms. Zarekarizi et al. (2020) further use two functional forms to relate flood depth to property damage, based upon two curves produced by Huizinga et al. (2017). Each of these curves is presented as a central estimate in the original source and Zarekarizi et al. (2020) add 30% uniform uncertainty to each without explicit justification. Finally, Ciullo et al. (2022) consider a range of functional forms for a “fragility curve” representing the probability of a levee being breached as a function of river height. Their analysis indicates different actions gain priority depending on beliefs around the shape of this curve.
The remainder of studies consider uncertainties only in terms of parameter ranges. These are spread across diverse system characteristics including human social and economic behavior (e.g., elasticity, contact rate between agents), behavior of environmental systems (e.g., inflow and evaporation), time taken for construction and repair processes, and achievability of proposed actions. It should be noted that different parameter values for structural properties of the system can have a drastic impact on outcomes, which may be no less important than differences in functional form or overall structure of relationships. This is particularly true where those parameters relate to behavior of feedback loops driving behavior of the system (see Figure 2). However, we consider that it is not possible to explore alternative conceptions of the structure of the system (i.e., differing sets of connections between variables) through exploring parameter space alone.
This section examines the extent to which actions considered in the selected case studies can transform systems. Considered actions are divided into a range of categories, as shown in Figure 5. Most studies only include actions targeting relatively shallow leverage points. A few studies include actions which could potentially target deeper leverage points for system transformation. However, these studies use models of relationships which cannot represent the processes by which these actions could lead to a deeper transformation. Our assessment of the deepest leverage point accessible by actions considered in each study, both within the framework of the set of relationships used within that study, and in terms of deeper leverage points these actions could potentially influence in practice, are shown in Figure 6.
Figure 5. Categories of action considered in case studies (some case studies include actions in multiple categories).
Figure 6. Deepest leverage points (A) accessed by actions in each case studies, as considered in those studies, and (B) potentially accessible by actions outside of the set of relationships considered in studies.
Most studies only consider actions relating to the shallowest three leverage points for system transformation. Around three quarters of studies include actions relating to physical changes to infrastructure (building/expanding/upgrading power plants, transport links, water pumps, reservoirs, and/or processing facilities). In some cases, the timing, sequencing, location, and scale of these facilities represent parameters under exploration. In most cases, these bring about changes in material stocks and flows, the 10th shallowest leverage point (referred to as “level 10” hereafter, see Figure 2). However, in some cases, such as reservoir expansion, this is considered to access the shallower leverage point of stock size relative to flow (level 11). Almost half of studies consider changes relating to how infrastructure is operated (e.g., maintenance regimes, pumping thresholds, runway selection), relating to the shallowest leverage point of parameters (level 12). A few studies considered incentives and disincentives for particular actions (e.g., water pricing, a fee per vehicle mile traveled, and electric vehicle subsidies). There is some ambiguity in Meadows's (1999) system of leverage points around when incentives relate to parameter changes (level 12) or rule changes (level 5). However, Meadows (1999) explicitly places subsidies in the category of parameter changes, on the basis that they are designed to tweak the existing system rather than change how the system functions (by, for example, changing who has power over laws). Following Meadows (1999), incentives in case studies are considered to access the shallowest leverage point of parameter changes (level 12).
Several studies include actions which could potentially access deeper leverage points, but the model of system relationships includes no way for these actions to effect system transformation. In four cases, all focussing on water management, actions are presented framed in terms of changing rules, or better enforcing existing rules. In principle, changing rules of a system could access a relatively deep leverage point (level 5). However, these rules are confined to operation of the water system, and only considered in terms of changing parameter values associated with thresholds for particular actions. Three studies focusing on management of water supplies in a changing climate each included educational programmes to reduce water demand in diverse geographical contexts (Hall and Murphy, 2012; Kingsborough, 2016; Jaiswal et al., 2021). This relates to a change in information flows, also a relatively deep leverage point (level 6). However, in each case, this is considered only in terms of the proportional reduction in water usage it could bring about (a parameter change, level 12).
In some cases, scenario uncertainties considered in case studies could also have a drastic and transformative effect if system relationships were conceived of differently. For example, more than half of considered studies include uncertainty related to future extent and impact of climate change. Responses to such scenarios has the potential to transform mindsets and societal goals, relating to deep leverage points (Rockström et al., 2009; Intergovernmental Panel on Climate Change, 2014; Willis, 2020). However, mechanisms through which these uncertainties could cause transformative change are not included in these studies.
This section more closely examines the motivation for, and models used in, case studies in the transport sector. This sector is given special attention because it is a sector for which infrastructure transformation is particularly important for meeting sustainability goals. Transport also represents a sector in which transformation is particularly complex and challenging, owing to its entanglement with the behavior of large numbers of agents with diverse priorities, with rapidly changing technology, infrastructure, land use, and its dynamic spatial and temporal nature (Shepherd, 2014; Hollander, 2016; Intergovernmental Panel on Climate Change, 2022).
Twelve studies focus on the transport sector, with a diversity of motivations across themes of resilience, capacity management, and transitions in support of an overall transformation to a low carbon system (Table 3). Five studies relate to resilience, of road systems to flooding in Peru (Rozenberg et al., 2017b), Mozambique (Espinet et al., 2018) and the USA (Singh et al., 2020), of European ports to global economic conditions (Halim et al., 2016), and of port infrastructure to sea level rise in the USA (Sriver et al., 2018). Four studies focus on managing capacity in response to demand in diverse areas of the transport system: rail and road capacity (Legêne et al., 2020; Hadjidemetriou et al., 2021), airplane manufacturing capacity (Kotta, 2018) and airport capacity planning (Kwakkel et al., 2012). Three studies explicitly focus on transitions in one or more components of the transport system, motivated in part by reducing associated greenhouse gas emissions or air pollution. Transition studies focus on model shift from road to rail in the state of New York, USA (Milkovits et al., 2019), from petrol to natural gas vehicles in Jakarta, Indonesia (Hidayatno et al., 2020), and from private to public transport and gasoline to electric vehicles, in Sacramento, USA (Lempert et al., 2020).
A diversity of model types is used in these studies (Table 4) with substantially different characteristics. Four stage and Origin-Destination (OD) pair models both have an explicit spatial and temporal focus, with discrete journey choices between origins and destinations explicitly modeled (de Dios Ortúzar and Willumsen, 2011; Hollander, 2016). Elasticity-based models seek to represent the implications of changing costs and journey times for changing journey rates and modal shift at a macro-level, whilst agent-based models seek to model the behavior of large numbers of agents at a micro-level to produce credible behavior at the macro-level. System dynamics models emphasize the representation of key variables and dynamics influencing the behavior of the system, emphasizing feedback effects across multiple timescales and often including variables related to societal as well as physical phenomena (Shepherd, 2014; Legêne et al., 2020). Cost-benefit analyses avoid detailed modeling of physical or social processes, and instead calculate economic implications of largely exogenously defined scenarios.
There is greater diversity in model types than in motivations for studies implying that more than one model type is suitable for considering some of these questions. However, no study compares the results of more than one type of model. Subsequent paragraphs examine the considerations defining model choice amongst transport case studies.
Amongst five studies focussing on resilience, two model types are used. Three studies, which focus on resilience of road systems to flooding (Rozenberg et al., 2017b; Espinet et al., 2018), and of European ports to changing economic conditions (Halim et al., 2016) use spatially disaggregated OD pair models. In the first two studies, this model type is selected due to the importance of understanding where impacts will fall, with a view to identifying which routes are particularly important and/or vulnerable and require reinforcement or backup routes. In the second, this is associated with the finely balanced choice of routes through which goods are transported, necessitating a detailed spatial representation in the model. The final two resilience studies focus on the resilience of a single piece of infrastructure (a bridge and a port) to sea level rise, thus not requiring geographical disaggregation (Sriver et al., 2018; Singh et al., 2020). These studies uses simple cost-benefit models to evaluate appropriate reinforcement measures at different levels of sea level rise.
Amongst four studies focusing on capacity management, four different model types are used. Hadjidemetriou et al. (2021) develop an elasticity based model to calculate numbers of rail and road users under future travel time and cost scenarios. Legêne et al. (2020) develop a system dynamics model to assess road space requirements of autonomous vehicles. Kotta (2018) develops a cost-benefit model to determine a cost-effective time to build an additional aircraft hangar to accommodate rising travel demand. Finally, Kwakkel et al. (2012) develop a bespoke model to analyze airport performance under a range of external conditions, focussing on ensuring adequate capacity while assessing noise, emissions, and third party risk. This diversity of model types highlights the broad range of factors influencing travel demand, and the diversity of emphasis amongst these in different models.
Each of the three studies focussing on transitions in the transport system also uses a different type of model. Milkovits et al. (2019) develop a four stage model to calculate modal shift resulting from increasing rail and reducing road capacity, Hidayatno et al. (2020) develop an agent-based model to determine actions to drive a transition from petrol to natural gas vehicles, and finally Lempert et al. (2020) develop an elasticity based model to calculate the impact of fees per mile traveled and electric vehicle incentives in reducing private travel and GHG emissions.
Notably, whilst all three studies focussing on transitions only consider actions targeting shallow leverage points for system transformation, one study uses a model capable of assessing actions targeting deep leverage points. The agent-based model developed by Hidayatno et al. (2020) includes feedback loops relating to interaction between agents, which could allow the analysis of actions targeting deeper leverage points associated with strengthening and weakening these feedbacks (levels 7 to 9 in Figure 2), and potentially influencing information flow between these agents (relating to system design, level 6). Whilst uncertainty in feedbacks is considered in this study, actions specifically targeting deeper leverage points associated with these feedbacks are not. It is unclear how the four-stage or the elasticity-based model could be used to examine the implications of actions targeting leverage points deeper than those associated with changing material flows and parameters (levels 10 to 12).
DMDU represents a promising set of techniques for catalyzing urgently needed action to transform infrastructure to achieve sustainability goals in the context of uncertainty about the relationship between actions and outcomes. However, this review reveals limitations in applications of DMDU to support transformative decision making for infrastructure to date. These center on the breadth of models of relationships used in these studies, the extent to which considered uncertainties and actions could transform the system, and the extent to which considered models are able to represent these system changes. Addressing these issues could allow DMDU techniques to play a larger and more effective role in supporting system transformation. Based on the observations highlighted above, some recommendations are here provided for the use of models in decision making for transformative change under deep uncertainty.
Structural uncertainty is considered in about half of DMDU case studies presented here, but in most cases only through varying parameters within a single structure of relationships. The prominence of diversity of possible system conceptions in the common definition of deep uncertainty suggests that DMDU studies would benefit from including multiple models of system behavior, particularly when considering complex systems which cannot be fully described and lend themselves to diverse understandings. This omission could lead to undue confidence in results in complex and poorly understood systems. It could also risk instrumentalisation of choice of models, biasing results toward actions which are already preferred by decisionmakers, whilst appearing to take uncertainty into account.
In most case studies, considered actions target parameters and material flows, relatively shallow leverage points for bringing about system change. Where actions which could target deeper leverage points are included, models of relationships are not able to represent the process by which transformative changes might occur. If not addressed in using DMDU in decision making for transformative change, this omission risks neglecting the deepest leverage points by which system transformation could occur.
It is not clear whether these omissions reflect limitations of the DMDU methods themselves, or a broader lack of attention to these issues in the decision making context within which these methods are applied. With respect to model multiplicity, emphasis on consensus building in approaches to participatory modeling could result in a tendency to represent a system within a single model even where this is unlikely to represent the true behavior of the system (Vennix et al., 1999; Voinov et al., 2018). With respect to deeper leverage points in decision making, Li et al. (2015) highlight that socio-technical transition frameworks that address transitions are often found to be difficult to operationalize in quantitative analyses to meet policy development requirements. An additional reason for omissions could be the emergence of DMDU techniques from a focus on reducing the impact of external threats on a system (e.g., flooding or sea level rise), rather than on transformation of the system itself. The latter may require a more detailed model incorporating diverse understandings the system and how it could evolve.
Ideally, the process of transformation of systems between states should also be considered in DMDU studies directed toward transformative change. This raises a question of how computer models can be used to model processes of societal transformation. System dynamics and agent-based models are expected to be promising here, which place greater emphasis on diverse societal factors (which tend to influence deeper leverage points) than more physical models. In designing such models, priority should be given to the representation of deeper leverage points for transformation within Meadows's (1999) framework.
Halbe et al. (2015), Holtz et al. (2015), and Köhler et al. (2018) provide reviews of, and additional recommendations for, model in research focussing on system transformation. Amongst other recommendations, these reviews suggest participatory modeling, comparison of alternative system models, shared frameworks for sharing insights across disciplines, and use of structural models, which map out relationships without explicitly simulating resulting dynamics. Accessing the knowledge of diverse actors through semi-quantitative fuzzy cognitive maps also represents a promising approach (Aminpour et al., 2020). Using insights from DMDU studies in support of broader strategies for system transformation more focussed on organizations, such as transition management (Malekpour et al., 2020) vision led planning (Smeds and Jones, 2020), may also be promising approaches for accessing deeper leverage points.
The detailed analysis of the transport sector provides insights particularly relevant to transport planners, but potentially also relevant to those seeking to catalyze change in other sectors. Analysis seeking to catalyze transformation of the transport sector would benefit from incorporating multiple existing forms of model in acknowledgment of the diverse understandings of how transport systems operate (as represented by the diversity of models used in this sector). It would also benefit from an examination of the range of perspectives included in developing these models. Since transport outcomes link closely to behavior, differences in understanding between model developers and transport users could result in very different outcomes from those indicated by model-based analysis. However, the balance between properly addressing multiple interpretations of the complex transport system, acknowledging the urgency of system transformation, and operating within time and resource constraints, is far from trivial and presents a barrier to changing practice.
Where possible, transport models should be used to consider the impact of actions targeting deeper leverage points for system change (e.g., changing information flows as well as material changes). Models should be adapted and developed to represent the transformative effect of actions targeting deeper leverage points where possible, and used in conjunction with frameworks targeting deeper leverage points. Further work will seek to represent diversity of perspectives around modeling for transformative change amongst the transport community, and to work with transport organizations to embed insights into their decision making processes for transformative change.
SF conducted literature searches, analyzed literature, and wrote the first draft of the manuscript. All authors contributed to conception, design of the study, manuscript revision, read, and approved the submitted version.
The authors gratefully acknowledge funding through the UK Engineering and Physical Sciences Research Council (Grant Number EP/R007403/1).
The authors declare that the research was conducted in the absence of any commercial or financial relationships that could be construed as a potential conflict of interest.
All claims expressed in this article are solely those of the authors and do not necessarily represent those of their affiliated organizations, or those of the publisher, the editors and the reviewers. Any product that may be evaluated in this article, or claim that may be made by its manufacturer, is not guaranteed or endorsed by the publisher.
The Supplementary Material for this article can be found online at: https://www.frontiersin.org/articles/10.3389/fclim.2023.1129378/full#supplementary-material
Abson, D.J., Fischer, J., Leventon, J., Newig, J., Schomerus, T., Vilsmaier, U., et al. (2017). Leverage points for sustainability transformation. Ambio 46, 30–39. doi: 10.1007/s13280-016-0800-y
Aminpour, P., Gray, S. A., Jetter, A. J., Introne, J. E., Singer, A., and Arlinghaus, R. (2020). Wisdom of stakeholder crowds in complex social–ecological systems. Nat. Sustain. 3, 191–199. doi: 10.1038/s41893-019-0467-z
Babovic, F., Mijic, A., and Madani, K. (2018). Decision making under deep uncertainty for adapting urban drainage systems to change. Urban Water J. 15, 552–560. doi: 10.1080/1573062X.2018.1529803
Bale, C. S. E., Varga, L., and Foxon, T. J. (2015). Energy and complexity: new ways forward. Appl. Energy 138, 150–159. doi: 10.1016/j.apenergy.2014.10.057
Bankes, S. (1993). Exploratory modeling for policy analysis. Oper. Res. 41, 435–449. doi: 10.1287/opre.41.3.435
Bloemen, P. J. T. M., Hammer, F., van der Vlist, M. J., Grinwis, P., and van Alphen, J. (2019). DMDU into Practice: Adaptive Delta Management in The Netherlands, in: Decision Making under Deep Uncertainty. Cham: Springer International Publishing, 321–351. doi: 10.1007/978-3-030-05252-2_14
Boardman, J., and Sauser, B. (2006). “System of systems - the meaning of,” in Proceedings 2006 IEEE/SMC International Conference on System of Systems Engineering 2006, 118–123.
Bojórquez-Tapia, L. A., Eakin, H., Reed, P. M., Miquelajauregui, Y., Grave, I., Merino-Benítez, T., et al. (2022). Unveiling uncertainties to enhance sustainability transformations in infrastructure decision-making. Curr. Opin. Environ. Sustainabil. 55, 1–10. doi: 10.1016/j.cosust.2022.101172
Bonjean Stanton, M. C., and Roelich, K. (2021). Decision making under deep uncertainties: a review of the applicability of methods in practice. Technol. Forecast. Soc. Change 171:120939. doi: 10.1016/j.techfore.2021.120939
Bonzanigo, L., and Kalra, N. (2014). Making informed investment decisions in an uncertain world: a short demonstration. The World Bank. doi: 10.1596/1813-9450-6765
Ciullo, A., Domeneghetti, A., Kwakkel, J., De Bruijn, K., Klijn, F., and Castellarin, A. (2022). Belief-Informed Robust Decision Making (BIRDM): assessing changes in decision robustness due to changing assumptions in the distribution of deeply uncertain variables. Environ. Model. Softw. 159:105560. doi: 10.1016/j.envsoft.2022.105560
de Dios Ortúzar, J., and Willumsen, L.G. (2011). Modelling Transport, 4th Edn. Wiley. doi: 10.1002/9781119993308
Espinet, X., Rozenberg, J., Ogita, K. S. R. S., Singh Rao, K., and Ogita, S. (2018). Piloting the Use of Network Analysis and Decision-Making under Uncertainty in Transport Operations: Preparation and Appraisal of a Rural Roads Project in Mozambique Under Changing Flood Risk and Other Deep Uncertainties, Policy Research Working Papers. The World Bank. doi: 10.1596/1813-9450-8490
Eyring, V., Bony, S., Meehl, G.A., Senior, C.A., Stevens, B., Stouffer, R.J., et al. (2016). Overview of the coupled model intercomparison project phase 6 (CMIP6) experimental design and organization. Geosci. Model Dev. 9, 1937–1958. doi: 10.5194/gmd-9-1937-2016
Feola, G. (2015). Societal transformation in response to global environmental change: a review of emerging concepts. Ambio 44, 376–390. doi: 10.1007/s13280-014-0582-z
Fischer, J., and Riechers, M. (2019). A leverage points perspective on sustainability. People Nat. 1, 115–120. doi: 10.1002/pan3.13
Funtowicz, S.O., and Ravetz, J. R. (1993). Science for the post-normal age. Futures 25, 739–755. doi: 10.1016/0016-3287(93)90022-L
Gambhir, A., Cronin, C., Matsumae, E., Rogelj, J., and Workman, M. (2019). Using Futures Analysis to Develop Resilient Climate Change Mitigation Strategies. Grantham Institute Briefing Papers.
Groves, D. G., Molina-Perez, E., Bloom, E., and Fischbach, J. R. (2019). “Robust Decision Making (RDM): application to water planning and climate policy,” in Decision Making under Deep Uncertainty. Cham: Springer International Publishing, 135–163. doi: 10.1007/978-3-030-05252-2_7
Gupta, J. (2016). Climate change governance: history, future, and triple-loop learning? wiley interdisciplinary reviews: Clim. Change 7, 192–210. doi: 10.1002/wcc.388
Hadjidemetriou, G. M., Kapetas, L., and Parlikad, A. K. (2021). Flexible planning for inter-city multi-modal transport infrastructure. J. Infrastruct. Syst. 28, 1. doi: 10.1061/(ASCE)IS.1943-555X.0000664
Halbe, J., Reusser, D. E., Holtz, G., Haasnoot, M., Stosius, A., Avenhaus, W., et al. (2015). Lessons for model use in transition research: a survey and comparison with other research areas. Environ. Innov. Soc. Transit. 15, 194–210. doi: 10.1016/j.eist.2014.10.001
Halim, R. A., Kwakkel, J. H., and Tavasszy, L. A. (2016). A scenario discovery study of the impact of uncertainties in the global container transport system on European ports. Futures 81, 148–160. doi: 10.1016/j.futures.2015.09.004
Hall, J., and Murphy, C. (2012). “Adapting water supply systems in a changing climate,” in Water Supply Systems, Distribution and Environmental Effects, eds S. Quinn and V. O'Neill (Nova Science Publishers, Inc).
Hamarat, C., Kwakkel, J. H., Pruyt, E., and Loonen, E. T. (2014). An exploratory approach for adaptive policymaking by using multi-objective robust optimization. Simul. Model. Pract. Ther. 46, 25–39. doi: 10.1016/j.simpat.2014.02.008
Herman, J. D., Zeff, H. B., Reed, P. M., and Characklis, G. W. (2014). Beyond optimality: Multistakeholder robustness tradeoffs for regional water portfolio planning under deep uncertainty. Water Resour. Res. 50, 7692–7713. doi: 10.1002/2014WR015338
Hidayatno, A., Jafino, B. A., Setiawan, A. D., and Purwanto, W. W. (2020). When and why does transition fail? a model-based identification of adoption barriers and policy vulnerabilities for transition to natural gas vehicles. Energy Policy 138:111239. doi: 10.1016/j.enpol.2020.111239
Holtz, G., Alkemade, F., De Haan, F., Köhler, J., Trutnevyte, E., Luthe, T., et al. (2015). Prospects of modelling societal transitions: Position paper of an emerging community. Environ. Innov. Soc. Transit. 17, 41–58. doi: 10.1016/j.eist.2015.05.006
Huizinga, J., de Moel, H., and Szewczyk, W. (2017). Global Flood Depth-Damage Functions. Methodology and the Database With Guidelines. Joint Research Centre (JRC).
Hurford, A. P. (2016). Accounting For water-, Energy-and Food-Security Impacts in Developing Country Water Infrastructure Decision-Making Under Uncertainty. UCL (University College London).
Intergovernmental Panel on Climate Change (2014). Climate Change 2014: Working Group III: Mitigation of Climate Change. IPCC, United Nations.
Intergovernmental Panel on Climate Change (2022). Mitigation of Climate Change Summary for Policymakers (SPM). Cambridge University Press, 1–30. doi: 10.1017/CBO9780511546013.003
IPCC (2014). IPCC Fifth Assessment Synthesis Report-Climate Change 2014 Synthesis Report. IPCC Fifth Assessment Synthesis Report-Climate Change 2014, 167.
Jaiswal, R. K., Lohani, A. K., and Tiwari, H. L. (2021). A decision support system framework for strategic water resources planning and management under projected climate scenarios for a reservoir complex. J. Hydrol. 603:127051. doi: 10.1016/j.jhydrol.2021.127051
Kingsborough, A. (2016). Urban Climate Change Adaptation Pathways for Short to Long Term Decision-Making VO - RT - Thesis. University of Oxford.
Köhler, J., De Haan, F., Holtz, G., Kubeczko, K., Moallemi, E., Papachristos, G., et al. (2018). Modelling sustainability transitions: an assessment of approaches and challenges. J. Artificial. Societies Soc. Simul. 21, 8. doi: 10.18564/jasss.3629
Kotta, K. S. V. (2018). Comparing Decision Making Using Expected Utility, Robust Decision Making, and Information-Gap. Application to Capacity Expansion for Airplane Manufacturing. Iowa State University.
Kwakkel, J.H., Walker, W.E., and Haasnoot, M. (2016). Coping with the wickedness of public policy problems: approaches for decision making under deep uncertainty. J. Water Resour. Plan. Manag. 142, 1–5. doi: 10.1061/(ASCE)WR.1943-5452.0000626
Kwakkel, J. H., Auping, W. L., and Pruyt, E. (2013). Dynamic scenario discovery under deep uncertainty: the future of copper. Technol. Forecast. Soc. Change 80, 789–800. doi: 10.1016/j.techfore.2012.09.012
Kwakkel, J. H., Walker, W. E., and Marchau, V. A. W. J. (2012). Assessing the efficacy of dynamic adaptive planning of infrastructure: results from computational experiments. Environ. Plann. B Plann. Des. 39, 533–550. doi: 10.1068/b37151
Kwakkel, J. H., Walker, W. E., and Marchau, V. A. W. J. (2010). Adaptive Airport Strategic Planning. Eur. J. Transp. Infrastruct. Res. 10, 249. doi: 10.18757/ejtir.2010.10.3.2891
Lee, D. B. (1973). Requiem for large-scale models. J. Am. Plann. Assoc. 39, 163–178. doi: 10.1080/01944367308977851
Legêne, M. F., Auping, W. L., de Almeida Correia, G. H., and Arem, B. (2020). Spatial impact of automated driving in urban areas. J. Simul. 14, 295–303. doi: 10.1080/17477778.2020.1806747
Lempert, R., Syme, J., Mazur, G., Knopman, D., Ballard-Rosa, G., Lizon, K., et al. (2020). Meeting climate, mobility, and equity goals in transportation planning under wide-ranging scenarios. J. Am. Plann. Assoc. 86, 311–323. doi: 10.1080/01944363.2020.1727766
Lempert, R.J. (2019). Robust Decision Making (RDM), in: Decision Making under Deep Uncertainty. Springer, 23–51. doi: 10.1007/978-3-030-05252-2_2
Lempert, R. J., Popper, S. W., and Bankes, S. C. (2003). Shaping the next one hundred years: new methods for quantitative, long-term policy analysis. RAND. 3. doi: 10.7249/MR1626
Lempert, R. J., Popper, S. W., and Calvo Hernandez, C. (2022). Transportation Planning for Uncertain Times: A Practical Guide to Decision Making Under Deep Uncertainty for MPOs.
Li, F.G.N., Trutnevyte, E., and Strachan, N. (2015). A review of socio-technical energy transition (STET) models. Technol. Forecast. Soc. Change 100, 290–305. doi: 10.1016/j.techfore.2015.07.017
Lyons, G., and Marsden, G. (2019). Opening out and closing down: the treatment of uncertainty in transport planning's forecasting paradigm. Transportation. 48, 595–616. doi: 10.1007/s11116-019-10067-x
Malekpour, S., Walker, W.E., de Haan, F.J., Frantzeskaki, N., and Marchau, V.A.W.J. (2020). Bridging Decision Making under Deep Uncertainty (DMDU) and Transition Management (TM) to improve strategic planning for sustainable development. Environ. Sci. Policy 107, 158–167. doi: 10.1016/j.envsci.2020.03.002
Marchau, V. A. W. J., Walker, W. E., Bloemen, P. J. T. M., and Popper, S. W. (2019a). Introduction, in: Decision Making under Deep Uncertainty. Cham: Springer International Publishing, 1–20. doi: 10.1007/978-3-030-05252-2_1
Marchau, V. A. W. J., Walker, W. E., Bloemen, P. J. T. M., and Popper, S. W. (2019b). Conclusions and Outlook, in: Decision Making under Deep Uncertainty. Cham: Springer International Publishing, 393–400. 7 doi: 10.1007/978-3-030-05252-2_17
Meadows, D. (1999). Leverage Points: Places to Intervene in a System donellameadows.org/archives/leverage-points-places-to-intervene-in-a-system. Sustainability Institute 1–18.
Milkovits, M., Copperman, R., Newman, J., Lemp, J., Rossi, T., and Sun, S. (2019). Exploratory modeling and analysis for transportation: an approach and support tool - TMIP-EMAT. Transp. Res. Rec. 2673, 407–418. doi: 10.1177/0361198119844463
Moallemi, E. A., de Haan, F., Kwakkel, J., and Aye, L. (2017). Narrative-informed exploratory analysis of energy transition pathways: a case study of India's electricity sector. Energy Policy 110, 271–287. doi: 10.1016/j.enpol.2017.08.019
Murray, B. C., Bistline, J., Creason, J., Wright, E., Kanudia, A., and de la Chesnaye, F. (2018). The EMF 32 study on technology and climate policy strategies for greenhouse gas reductions in the U.S. electric power sector: an overview. Energy Econ. 73, 286–289. doi: 10.1016/j.eneco.2018.03.007
Pruyt, E., and Kwakkel, J. H. (2014). Radicalization under deep uncertainty: a multi-model exploration of activism, extremism, and terrorism. Syst. Dyn. Rev. 30, 1–28. doi: 10.1002/sdr.1510
Pye, S., Li, F. G. N., Petersen, A., Broad, O., McDowall, W., Price, J., et al. (2018). Assessing qualitative and quantitative dimensions of uncertainty in energy modelling for policy support in the United Kingdom. Energy Res. Soc. Sci. 46, 332–344. doi: 10.1016/j.erss.2018.07.028
Rockström, J., Steffen, W., Noone, K., Persson, Å., Chapin, F. S., Lambin, E. F., et al. (2009). A safe operation space for humanity. Nature 461, 472–475. doi: 10.1038/461472a
Roelich, K. (2020). What did infrastructure ever do for us? IPPR Prog. Rev. 27, 140–148. doi: 10.1111/newe.12200
Rogelj, J., Shindell, D., Jiang, K., Fifita, S., Forster, P., Ginzburg, V., et al. (2018). “Mitigation Pathways Compatible with 1.5°C in the Context of Sustainable Development,” in Global Warming of 1.5°C. An IPCC Special Report on the impacts of global warming of 1.5°C above pre-industrial levels and related global greenhouse gas emission pathw. IPCC special report Global Warming of 1.5°C, 82.
Royston, S., Foulds, C., Pasqualino, R., and Jones, A. (2022). Masters of the machinery: the politics of economic modelling within European Union energy policy. SSRN Preprint 11, 133–143. doi: 10.2139/ssrn.4212105
Rozenberg, J., Bank, W., Office, C. E., and Development, S. (2017a). PREPARING Transport for An Uncertain Climate Future : I don' t Have a Crystal Ball, But I Have a Computer.
Rozenberg, J., Briceno-Garmendia, C., Lu, X., Bonzanigo, L., and Moroz, H. (2017b). Improving the Resilience of Peru's Road Network to Climate Events, Policy Research Working Papers. The World Bank. doi: 10.1596/1813-9450-8013
Shepherd, S. P. (2014). A review of system dynamics models applied in transportation. Transportmetrica B 2, 83–105. doi: 10.1080/21680566.2014.916236
Singh, P., Ashuri, B., and Amekudzi-Kennedy, A. (2020). Application of dynamic adaptive planning and risk-adjusted decision trees to capture the value of flexibility in resilience and transportation planning. Transp. Res. Rec. 2674, 298–310. doi: 10.1177/0361198120929012
Smeds, E., and Jones, P. (2020). Developing Transition Pathways towards Sustainable Mobility in European cities Conceptual framework and practical guidance. Sustainable Urban Mobility Planning: Pathways and Links to Urban Systems (SUMP-PLUS).
Sovacool, B. K., Axsen, J., and Sorrell, S. (2018). Promoting novelty, rigor, and style in energy social science: towards codes of practice for appropriate methods and research design. Energy Res. Soc. Sci. 45, 12–42. doi: 10.1016/j.erss.2018.07.007
Sriver, R. L., Lempert, R. J., Wikman-Svahn, P., and Keller, K. (2018). Characterizing uncertain sea-level rise projections to support investment decisions, PLoS ONE, 7:e0190641. doi: 10.1371/journal.pone.0190641
Süsser, D., Ceglarz, A., Gaschnig, H., Stavrakas, V., Flamos, A., Giannakidis, G., et al. (2021). Model-based policymaking or policy-based modelling? how energy models and energy policy interact. Energy Res. Soc. Sci. 75:101984. doi: 10.1016/j.erss.2021.101984
Swanson, A. R., Sakhrani, V., and Preston, M. S. (2019). Flexible design at batoka dam: how real options analysis compares to other decision-making tools. Renew. Energy Focus 31, 1–8. doi: 10.1016/j.ref.2019.05.001
Vennix, J.A.M., Wright, J., and Prize, F. (1999). Group model-building : tackling messy problems. Syst. Dynamic. Rev. 15, 379–401.
Voinov, A., Jenni, K., Gray, S., Kolagani, N., Glynn, P. D., Bommel, P., et al. (2018). Tools and methods in participatory modeling: selecting the right tool for the job. Environ. Modell. Softw. 109, 232–255. doi: 10.1016/j.envsoft.2018.08.028
Walker, W.E., Haasnoot, M., and Kwakkel, J.H. (2013). Adapt or perish: a review of planning approaches for adaptation under deep uncertainty. Sustainabil. 5, 955–979. doi: 10.3390/su5030955
Willis, R. (2020). Too Hot To Handle? The Democratic Challenge of Climate Change. Bristol University Press. doi: 10.56687/9781529206036
Keywords: decision making under deep uncertainty, transformative change, leverage points, structural uncertainty, climate change, transport
Citation: Few S, Bonjean Stanton MC and Roelich K (2023) Decision making for transformative change: exploring model use, structural uncertainty and deep leverage points for change in decision making under deep uncertainty. Front. Clim. 5:1129378. doi: 10.3389/fclim.2023.1129378
Received: 21 December 2022; Accepted: 10 May 2023;
Published: 13 June 2023.
Edited by:
Peter Haas, University of Massachusetts Amherst, United StatesReviewed by:
Oran Young, University of California, Santa Barbara, United StatesCopyright © 2023 Few, Bonjean Stanton and Roelich. This is an open-access article distributed under the terms of the Creative Commons Attribution License (CC BY). The use, distribution or reproduction in other forums is permitted, provided the original author(s) and the copyright owner(s) are credited and that the original publication in this journal is cited, in accordance with accepted academic practice. No use, distribution or reproduction is permitted which does not comply with these terms.
*Correspondence: Sheridan Few, cy5mZXdAbGVlZHMuYWMudWs=
Disclaimer: All claims expressed in this article are solely those of the authors and do not necessarily represent those of their affiliated organizations, or those of the publisher, the editors and the reviewers. Any product that may be evaluated in this article or claim that may be made by its manufacturer is not guaranteed or endorsed by the publisher.
Research integrity at Frontiers
Learn more about the work of our research integrity team to safeguard the quality of each article we publish.