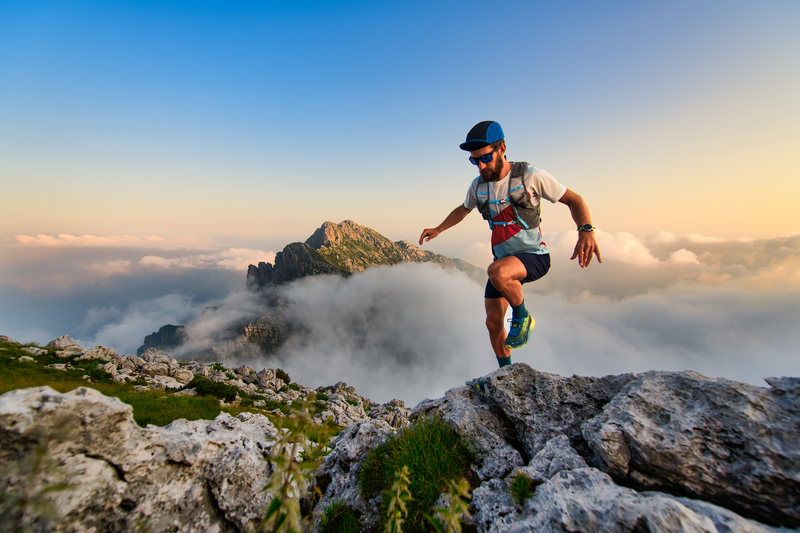
95% of researchers rate our articles as excellent or good
Learn more about the work of our research integrity team to safeguard the quality of each article we publish.
Find out more
ORIGINAL RESEARCH article
Front. Clim. , 24 April 2023
Sec. Climate Risk Management
Volume 5 - 2023 | https://doi.org/10.3389/fclim.2023.1100600
This article is part of the Research Topic Climatic Compound and Cascading Extremes in a Changing Climate: Variability, Predictability, and Causality View all 4 articles
Physical and societal risks across the natural, managed, and built environments are becoming increasingly complex, multi-faceted, and compounding. Such risks stem from socio-economic and environmental stresses that co-evolve and force tipping points and instabilities. Robust decision-making necessitates extensive analyses and model assessments for insights toward solutions. However, these exercises are consumptive in terms of computational and investigative resources. In practical terms, such exercises cannot be performed extensively—but selectively in terms of priority and scale. Therefore, an efficient analysis platform is needed through which the variety of multi-systems/sector observational and simulated data can be readily incorporated, combined, diagnosed, visualized, and in doing so, identifies “hotspots” of salient compounding threats. In view of this, we have constructed a “triage-based” visualization and data-sharing platform—the System for the Triage of Risks from Environmental and Socio-Economic Stressors (STRESS)—that brings together data across socio-environmental systems, economics, demographics, health, biodiversity, and infrastructure. Through the STRESS website, users can display risk indices that result from weighted combinations of risk metrics they can select. Currently, these risk metrics include land-, water-, and energy systems, biodiversity, as well as demographics, environmental equity, and transportation networks. We highlight the utility of the STRESS platform through several demonstrative analyses over the United States from the national to county level. The STRESS is an open-science tool and available to the community-at-large. We will continue to develop it with an open, accessible, and interactive approach, including academics, researchers, industry, and the general public.
Climate and our natural as well as managed environments are changing. Society is growing and its aging infrastructure to provide reliable energy, water, and transportation systems is increasingly strained and challenged by needed expansion, upgrades, and modernization. Further, the global economy, energy systems, and supply chains are faced with the challenge of transformational changes at a global scale. Simply put, the world is facing increasing and interwoven physical and transitional risks. In order to confront these challenges, the scientific community, stakeholders, and citizen scientists must view the world as complex, interwoven networks that co-evolve and are increasingly inter-connected. Multi-Sector Dynamics (MSD, Figure 1) explores interactions and interdependencies among human and natural systems and how these systems co-evolve in response to short-term shocks and long-term influences and stresses (e.g., Reed et al., 2022). For example, economic growth might put a pressure on public services and squeeze out low-income households (e.g., Frank, 2009), carbon policies can lead to different distributional impacts on households (e.g., García-Muros et al., 2022), and failed economies lead to widespread poverty, unemployment, tax base decline, and transform demographic geographies (e.g., Hochstenbach and Musterd, 2018). Under a changing global environment, weather may become more extreme, leading to increasing extreme events, such as droughts and floods (e.g., Stott, 2016). Efforts to curb emissions will affect air pollution, but can also exert unintended and unequal stress on communities (e.g., Kiesecker et al., 2019). Similarly, water pollution, or efforts to reduce it, while having clear health benefits can have unequal social and economic impacts (e.g., Mueller and Gasteyer, 2021). As the world economy changes, perhaps turning away from fossil fuels to toward other energy resources, resource use could change drastically leading to regional increases and declines in related industries. A growing global population that is becoming wealthier will put greater pressure on energy, land, and water resources.
Figure 1. Schematic representation of the multi-sector dynamic framework that recognizes the co-evolving nature of socio-economic and physical systems. These systems must be considered as connected to gain insights into the various landscapes of vulnerabilities, compounding risks, and environmental instabilities and inequalities.
In this vein, among the major challenges that Federal and State agencies face in addressing and identifying these problems lies in the difficulty of combining data to: assess hazards, impacts, stressors, exposures, vulnerabilities, and inequities; quantify overall risk; and set priorities. Currently, much of the needed data is disaggregated across multiple agencies, universities, and research groups, and presented at different geographical scales in varying formats. Finding and accessing all these data are also a challenge in itself. More importantly, combining these data and creating metrics to address “overall risk” is unintuitive. Recent studies have introduced and formalized a conceptual basis of “risk” across various scientific disciplines (e.g., Cappelli et al., 2021; Reed et al., 2022) primarily on climate change factors (e.g., Otto et al., 2017). On a more granular level, communities around the world will face multiple risk factors over the coming decades that do not evolve from climate change alone (e.g., Moss et al., 2016; Pescaroli and Alexander, 2018; Aven and Zio, 2021). In addition, the vulnerability, exposure, and resilience of communities—their ability to successfully cope with risk factors—varies. Poorer communities have fewer financial resources on which to draw, and minority communities often do not receive the attention or aid of other communities. Communities with many elderlies or children have a larger proportion of more dependent persons. The overall health of a community and access to health care also results in varying ability to cope with stressors, such as exposure to heat or pollution. Gathering data and projections necessary to understand environmental change and a community's vulnerabilities is itself a significant undertaking. Recruiting experts, evaluating available data and projections, and determining vulnerable infrastructure, populations, and economic activity even at a very low level can require hundreds of thousands of dollars, if not many millions.
With these considerations in mind, an efficient, low-cost, screening-level assessment platform that can identify “hot-spots” of co-existing, co-evolving situations and inform where resources and efforts can be most appropriately deployed would be valuable. This type of platform could be used to prioritize efforts in vulnerable regions to increase resilience against the identified potential compounding stresses. Overall, we refer to this as a “risk-triage” approach, with “triage” aligning with its definitional context of “...assigning of priority order to projects on the basis of where funds and other resources can be best used, are most needed, or are most likely to achieve success” (Merriam Webster Dictionary, 2021). Under these considerations, we have developed a System for the Triage of Risks from Environmental and Socio-Economic Stressors (STRESS) platform (URL—mst.mit.edu). The STRESS platform's motivating goals are to: characterize the extent that various risk factors (i.e., threats, hazards, impacts, stressors, exposures, vulnerabilities, and inequities) co-exist; compile metrics that quantify these risk factors in flexible and combinable fashion; identify how the aggregate risk landscape changes when individual risk factors are combined; and provide any user of the platform the ability explore various risk-factor combinations of their choosing. From this screening-level assessment, “hotspots” of risk can be identified that point to deeper diagnoses of risks at a more granular and detailed level, and these steps should lead to action to improve resilience. Identifying the most vulnerable region provides an opportunity to intervene before there are serious effects.
This paper describes a collection of data, constructed metrics, and an interactive, visualization platform to enable citizens, scientists, stakeholders, businesses, communities, state and Federal governments to identify particularly vulnerable regions, populations, infrastructure, and resources. In Section 2, we discuss conceptual considerations that motivate the risk-triage platform development, the supporting data collection, as well as a description of the subset of data used for the combinatory metrics and website that implements the conceptual model. To highlight the current capabilities of the platform, Section 3 provides selected examples and demonstrative analyses over the United States from the national to county level. In Section 4, we provide a summary and closing remarks describe our continued efforts to expand the platform's presented capabilities.
Stakeholders, legislators, decision-makers, as well as the community-at-large face increasingly complex exposures and compounding effects from co-evolving environmental, economic, and societal pressures. A number of online data, visualization, and analysis platforms are currently available to the community-at-large for exploring a wide range of climate, social, and environmental hazards (e.g., Pickard et al., 2015; Temper et al., 2015; Iturbide et al., 2020; U.S. EPA, 2020, 2022; Zuzak et al., 2021; NOAA NCEI, 2022). All these platforms carry unique perspectives and strengths, yet what they lack is the ability to combine and weight user-selected metrics to quantitatively assess compounding hazards. The extent of data that is accessible to visualize and quantify these pressures and stresses is substantial. Yet as noted, flexible and user-specified combinatory diagnostics such as these would provide valuable perspectives and identify salient priorities and vulnerabilities for investment and action. To use additional examples as conceptual motivation, imagine a situation in which a particular region of interest (e.g., nation, state, or county) is prone to high flood risk. Any flood risk metric by itself would identify areas at high risk, however, infrastructure, demographics, and poverty could make some areas less able to withstand a flood and less resilient to recover than others. However, compiling and combining all the necessary data to make a quantitative assessment with all these combined factors (and potentially weighted according to a decision-maker's judgement) is not readily available. Similarly, communities might use such a tool identify their vulnerabilities with respect to an array of stressors and their combinations. A platform that can combine compounding factors that identify the most risk-prone areas is valuable and insightful as a triage-response assessment. In more general terms, we can conceptualize this data-fusion need (Figure 2) to set the stage for our triage framework. Generally speaking, we can view a situation where there is an extensive ecosystem of data that covers a wide range of metrics that describe the states of various systems. However, these data come in various formats, configurations, units, and spatiotemporal attributes (i.e., span of data, resolution, and averaging to name a few), and in order for these metrics to be combined, one would need to collect, inspect, and “normalize” the data in such a way as to justify their combinatory use (center of Figure 2). Then, in order to provide an intuitive, exploratory, and interactive capability, the platform should allow the user to select and design their own combinations, visualize the aggregate metrics (for more rapid inspection), and download the resultant data fields for further analysis.
Figure 2. Conceptual framework of the risk-triage approach. The supporting web platform consists of a metadata collection obtained from various repositories and sources whose data differ in attributes, formats, content, units, granularities, etc. (i.e., Appendix A). From this ecosystem of data, a subset of the data is chosen that span across the multi-sector dynamics (MSD) purview (e.g., Reed et al., 2022). These data are further refined and normalized into a set of metrics that can be used for combinatory analyses. The platform is designed to be flexible and interactive—such that the user can select their own combination and weights (conceptually depicted by the line thicknesses) of these metrics. The result is an aggregate metric where the user can download the resulting set of data and map image created by the platform (URL: mst.mit.edu).
We believe that under the conception of a “risk-triage” capability, a visualization and data-sharing platform should include metrics of stressors, risks, vulnerabilities, and resilience across socio-economic, climate, water, land, energy, demographic, and infrastructure sectors, and that any metric should characterize the conditions and resources needed to cope with both gradual and sudden stressors. For example, the tool should encompass a gradual event such as economic decline from the fading of a communities' dominant industry, or a sudden, extreme event like a large flood. The platform likewise needs to be flexible: it must encompass and have the ability to quantitatively combine and visualize different types of metrics. The tool should include metrics that indicate current and the potential of future stresses. However, it cannot be expected to provide precise predictors of what and when something will happen, but rather, the tool can convey a quantitative description of multiple factors that can be viewed individually and in any desired combination—so that the impact of compounding effects can be assessed under current conditions and future pathways. These futures could be a result of plausible (and uncertain) trajectories of climate, weather extremes, socio-economic growth, land cover/use, land and ecosystem productivity, water resources, and air quality to name a few.
As depicted in our conceptual design of the platform (Figure 2). Our initial data mining efforts have focused on gathering a larger and supporting collection of data that (currently) span the following broad risk-factor categories: climate, water, energy, land, economics, demographics, infrastructure, and environmental opinions. There are numerous data bases available from various Federal, non-profit, and open-science research institutions that provide a wide range of social, economic, and environmental variables (e.g., Pickard et al., 2015; Temper et al., 2015; Iturbide et al., 2020; U.S. EPA, 2020, 2022; Zuzak et al., 2021; NOAA NCEI, 2022). Appendix A provides a more complete description of the various data portals, platforms, and repositories from which we have extracted data. The initial focus of our data collection has been over the United States at the county-level scale. Our collection of data currently includes over 100 variables that quantify a variety of fluxes, flows, states, and conditions across various landscapes of socio-environmental sectors, demographics, and public opinion. We have and will continue to collect publicly available data from the community-at-large. Therefore, it is important to note that our data collection is ongoing, and we will continue to expand and refine this initial collection, and subsequent papers will provide these details. Nevertheless, given our conceptual design described in the previous section, we have relied on these data to provide a “one-stop” collection of data (i.e., as depicted as the left end of Figure 2). Any of these data can be viewed on the platform and also downloaded such that a user can apply these data for additional analyses (and demonstrated in Section 3).
However, in view of our conceptual approach and methodology to create a combinatory set of data (center of Figure 2), we note that all these data are not provided in a consistent form such that they can be selectively combined. Therefore, we must further refine these data to synthesize a collection of metrics that allow for flexible, aggregate diagnostics. The method by which this is done is detailed in the next section. To perform this transformation for all the data we have collected may ultimately be desirable, but for this initial assessment is untenable and unwarranted. In order to assess and highlight the insights gained thru this approach, we have selected a subset of our larger data collection to serve as the basis for the combinatory risk metrics analysis and visualization. Conceivably, any variable from the risk-factor categories (described above) can be represented and selected. We have selected variables from the following risk-factor categories: water; land; climate; economy; energy; health; and demographics. From these, we have further refined and constructed 17 variables for use as combinatory risk metrics—and have focused on the contemporary conditions. Thus, within this subset of data we have utilized only the data that represents the most recent (or “current”) conditions. These details and the collection of data we have used for this demonstration of combinatory metrics is described in Table 1. It is important to emphasize that this collection of combinatory metrics (center of Figure 2) will continue to be expanded under the STRESS platform's goal to provide a comprehensive assessment of compounding and co-evolving risks.
Table 1. Description and data-source information for the risk metrics that have been constructed for the combinatory analyses.
A primary consideration for the combinatory metrics that allows for construction of an aggregate metric is that each of the metrics described above carries inconsistent units, and therefore in their constructed form, any combinatory, comparative, and/or prioritizing diagnostics are untenable. To provide a capacity for these metrics to be combined quantitatively that assures numerical consistency, there are two possible procedures that could be exercised. The first option would be to numerically recast all these metrics under a common-scale categorization of “risk” (e.g., Messer et al., 2014; Strzepek et al., 2021). The advantage of this approach is that it preserves the absolute nature of risk, and when combining all the metrics of interest, the resultant metric would highlight all areas “at risk” that have compounded accordingly. The difficulty of this approach is that the rescaling of the raw values according to a common risk scale, and combined under user-specified configurations, would be prone to subjective judgement and lead to distortions of one or more metrics over others. In addition, the process by which each variable is cast into a generic risk scale would be exhaustive and require extensive, expert judgement. A second option, which we have adopted for our initial platform development, is to normalize the metric by ranking their values across any pooled sample of the data. There are a number of options by which to normalize and rank a pooled set of raw data values (e.g., Strzepek et al., 2021). Considering the intent of the STRESS platform to provide a prioritization of risk, we have taken a percentile ranking approach. This also aligns with our “triage” methodology, which is to identify high-priority regions. As such, for any selected pooling of data (in this case, county-level data pooled across the United States), the following conversion is made. Given the raw value of the data, Vr, we construct a percentile ranking value, Vp, c, for each county (c) value using the expression:
The minimum (min) and maximum (max) values are obtained from the pooled county-level data collection across the United States or for a particular state (as determined by the user). The values of Vp ranges from 0 to 100 and is unitless, with a value of 0 indicating that the corresponding region has the lowest risk relative to all the other regions across the pooled data, and a value of 100 indicating the highest relative risk.
As previously noted, the intent of the STRESS platform is to provide the capability to perform user-specified, combinatory analyses and visualization. The use of composite metrics and analyses has been documented and used extensively to explore the complexity and inter-dependencies of various environmental issues (e.g., Saisana et al., 2005; Greco et al., 2019). The main issues are the choice weighting and aggregation methods across metrics, indicators, and/or variates that are of interest. Among the two more widely used approaches to determining weights (e.g., Sharpe and Andrews, 2012), “explicit” methods consist of evaluating surveys of responses from expert judgement using a Budget Allocation Process or an Analytic Hierarchy Process. The alternative and more objective approaches evaluate the relative importance of indicators based on data compression analyses. Various statistical methods such as Principal Component Analysis (PCA) or Factor Analysis (FA) can be applied for this purpose (e.g., Ram, 1982). These methods place a higher weight on “orthogonal” modes of variability that describe the maximum portion of aggregate variance, and thus a subset of metrics can have more importance in the final weighting. More recently approaches based on artificial intelligence models have been used for this purpose (e.g., Paulvannan Kanmani et al., 2020; Jiménez-Fernández et al., 2022). However, considering the intent of our platform to provide a flexible, user-inspired tool to explore multiple combinations of physical and transition risks, the debatable issue to the approaches above is that the portion of explained variance does not necessarily directly correspond to the highest value or importance of any metric, and an exhaustive set of expert judgements that span all possible combinations of our collected metrics is elusive. Given this, a final option is to give the user the choice of weights and aggregate the resultant metric accordingly. A wide variety of aggregation methods are used to combine indicators once their relative importance has been chosen. The most common is linear aggregation, though more complex non-compensatory methods are also possible (e.g., Greco et al., 2019). We have initially adopted a linear aggregation, and therefore at the user's discretion, any combination of these normalized risk metrics can be combined, at various selected weights—such that the resulting aggregate risk metric Va based on a total of N normalized metrics combined, described by:
Where the assigned weight, Wi, for each individual normalized risk metric (Vp, i) selected by the user is a constant value (from 0.1 to 1) across all counties. From this procedure, the STRESS platform also constructs a distribution of the resultant data that is displayed. The resultant combinatory metrics offer an intuitive and collective ability to identify regions most in need of attention. As previously mentioned, the intent of the platform is to provide the user with the flexibility to choose or consider a range of combinations and weights. We allow users to view and download a single metric or a selection of aggregate metrics given their focus and interests. In this way, a user of the platform could be able to identify, for example, the “top-10” riskiest locations/regions with the most vulnerable populations, resources, and infrastructure across all potential stressors, allowing for specific risks and weights within those categories. Or one could create a more focused assessment, for example, of infrastructure most at risk from extreme flooding with highly vulnerable populations. In the section that follows, we provide examples of how this procedure can be used to assess a variety of combinatory, socio-environmental assessments, research, and outreach applications.
As previously discussed, in the context of “triage”, the STRESS platform is intended to provide a rapid assessment, allowing reasonable prioritization, on the basis that an efficient “hotspot” analysis is more effective than exhaustive, extensive deep-dive assessments that are time-and-resource consuming. In fact, the intent of the “risk-triage” approach is to identify more specific targets for these deep-dive assessments. A user can choose various combinations to assess the co-existence of multiple risk factors—and to be able to gauge the “severity” in such a way that provides a quantitative basis for identifying “hotspots” of risk. Our approach to construct these combinatory risk metrics is flexible and extensible by design. In the sections that follow, we demonstrate this flexibility through a number of visualization and tabular examples.
One visual example of the STRESS platform's capabilities is the ability to efficiently indicate where environmental risks compound (Figure 3). In this demonstration, the current risks to land disturbances, water availability and quality, as well as exposure to poor air quality can be displayed individually (top panels of Figure 3A), and prominent hotspot regions that face higher levels of each of these separate risks can be discerned (orange/red shaded areas). In viewing these as separate mappings, it is not readily apparent to what degree the more severe areas of these individual environmental risks co-exist and potentially compound. Through the ability of the STRESS platform to combine all four metrics (Figure 3B—all with equal weighting), the landscape of the relative compounding risks is more clearly discernable, with the more prominent visible “hotspots” located across California, the upper and lower Mississippi basin, the Ohio River basin, Texas, the Southeast as well as Mid-Atlantic states.
Figure 3. Shown is an example of the combinatory metric analysis provided by the risk-triage framework. In the top panel (A), the maps show the results for each metric of exposure to air particulates, water stress, water quality, and land disturbance. In the bottom panel (B), the result of the four metrics, combined with equal weighting, is shown. In all panels, the risk metric is unitless and is the result of the normalization procedure (described in Section 2.2). Shades of orange and red indicate the counties with the highest relative risk, while shades of blue indicate the lowest relative degree of risk.
These hotspot areas may all be exacerbated by human-forced changes in climate, extreme events, land use, as well as water and energy demands. Future electricity demand is driven by socio-economic factors (e.g., GDP growth, population growth, technology costs and resulting electricity prices) as well environmental factors, particularly temperature, as driven by climate change, which drives demand for heating and cooling (e.g., Auffhammer et al., 2017; Van Ruijven et al., 2019). The highest density and connections of highest-voltage transmission lines are particularly concentrated in the hotspots of the compounding air-land-water risks across the Central U.S. (orange overlay lines in Figure 4A denote Level 3 transmission lines ≥ 345 kV). Concurrently, regions of the nation with the largest portion of employment in the fossil fuel industry, along with high levels of poverty and unemployment flank the lower portions of the Mississippi River (Figure 4B). National and global actions to reduce greenhouse emissions could limit risks to land, water, and air quality in the upper basin, but at the same time impacts on the fossil fuel industry could have significant employment impacts in the lower Basin where poverty and unemployment are already disproportionate. Further, climate-related extremes (e.g., droughts, floods, and events that damage transportation infrastructure) can adversely affect the flow of goods along the country's major river route (Figure 4B, the heavy blue line along the Mississippi river). Such disruptions would impact upstream and downstream regions in multiple respects that include energy supplies, agricultural products and inputs, as well as manufactured and raw materials, and all these would have follow-on impacts in other parts of the transportation network, industrial sectors, agriculture and land use, as well as the energy sector. All of these highlight the potential locations of and connections between contrasting regional effects of a low-carbon energy transition.
Figure 4. Maps produced from the STRESS platform illustrate the “hotspot” visualization and analysis capability of the user-defined, use-inspired interface. Panel (A) shows the result of quantitatively combining the current level of risks in water availability and quality, land disturbance, and exposure to poor air quality. Orange and red shades indicate “hotspot” areas of these three risks co-existing to the highest degree relative to all U.S. counties. Also shown is the graphical overlay of high-voltage transmission lines (green ≥ 230 kV and orange ≥ 345 kV) across the nation's energy grid. Panel (B) shows the result of combining risk metrics that convey the current levels of employment in fossil energy as well as demographic metrics of poverty, unemployment, and non-white population. Also shown as an overlay (blue lines) is the extent of major riverine and marine “highways” with the thickness of the lines depicting the relative total value of goods transported (coal, petro, food, chemical, manufacturing, and raw materials).
The co-existence of these interconnected risks in the example above provides motivation, location, and guidance for deeper-dive studies on human-natural system interactions; grid resiliency; and transportation infrastructure. To study these in more detail requires models of greater sophistication and large computational expense—but the presented triaging analyses identifies areas for targeted study, and in doing so reduces the need for exhaustive model simulations and analyses. There are, of course, many other combinations and overlays of compounding risks that can be visualized and explored with the triage platform that highlight other regions and multi-sector linkages, and these can guide model configurations, tested hypotheses, and experimental frameworks for a variety of detailed use case studies. Our ongoing efforts will continue to build upon our collection of historical and contemporary variables and expand upon our list of combinatory risk metrics in support of these screening-level multi-sector assessments.
As mentioned, many combinations of the risk metrics can be readily explored through the STRESS platform and provide more quantitative insights as to the areas of concern. For example, climate change, population growth, demographics, poverty, economic activity, and low-carbon energy transformation are but some of the major factors that can affect water demands. Looking at the current landscape of the combined risk of water stress and quality (Figure 5A), the risk is widespread and therefore it is difficult to distinguish exactly which areas of highest priority and/or concern would be. In considering only water stress and quality risks—most of the western U.S., the upper and mid-Mississippi regions, New England, the Mid Atlantic, and parts of the Southeast all convey a scattered map with locations of higher stress. The county-level results can be aggregated at the state level and ranked according to various factors in order to gain quantitative prioritization. For example, a “top 5 list” of the highest-at-risk states can be constructed by counting only the counties that are contained in the top 10% of the nationally pooled distributions (shown at the side of the maps in Figure 5) and summing those for every state according to either: total number of counties, percentage of counties in the state, or total population of those counties (Table 2—and tabulated from data downloaded from the constructed aggregate metrics on the platform). Based on any of these three categories, California stands out as the most salient state at risk with the highest or 2nd highest ranking. Other states that clearly stand out at higher risk are Illinois and Texas (with two top-five rankings). For the remainder of the results, the choice of the ranking criterion can have an important impact to a state's ranking. For example, over two-thirds of Delaware's counties experience combined water risk, placing it at the top of the percentage-of-counties ranking—but due to its lower total population as well as small total number of counties, it is at a distinct disadvantage to be top ranked in those ranking categories. Similarly, Nebraska having a high number of total counties gives the potential for a higher total number of counties under risk, and as such, it places 2nd in that category. However, its low total population and high total number of counties put it at a disadvantage to place in those ranking categories.
Figure 5. As in Figure 3, but highlighting the contrast between combinatory landscapes of: (A) water stress and water quality risks; and (B) water stress, water quality, and population below the poverty level.
Table 2. Summary of results from the combinatory risk metric combining water stress and water quality.
There are other important sensitivities to these highest-at-risk rankings. For example, the prioritization of a state to receive attention and assistance to mitigate water risks might also include a consideration of the extent of poverty. With this consideration in mind, we combine the water stress, water quality, and extent of poverty risk metrics, all with equal weighting. The results (Figure 5B) reveal important shifts in the overall landscape of risk as well as to the highest-at-risk state rankings (Table 3) discussed above. Many of the regions across the northern U.S. at higher risk from only water stress and quality are buffered, and most of the highest at-risk counties are in states across the southern half of the United States. This impact is also clearly seen in the top-five ranked state results. With the addition of poverty into the risk assessment, Illinois drops out of all the top rankings considered, in addition to Connecticut, Delaware, and Montana. The most notable addition to the highest rankings is New Mexico, making into all three of the highest-ranking lists. Other additions contributing to this southern shift of states within the top rankings include Georgia, Kentucky, and West Virginia. One exception to this overall shift is Oregon, in which one-third of its counties at water stress and poverty risk place it just within the top ranking of that category (but absent in the water-only risk results). Nevertheless, there are two states that stand out as the highest ranked across both the combined risk metrics: California and Texas.
Table 3. As in Table 2—but for the combinatory risk metric of water stress, water quality, and population below the poverty level.
Like water stress, resiliency to flooding is not simply a case of the risk of flooding itself and its consequences which damages property and contaminates water supplies, but also the ability of communities to rebuild and repair damage, which can be hindered by poverty (e.g., Hallegatte et al., 2018; McDermott, 2022) and may also carry important equity dimensions. We can examine all these facets through a successive combinatory analysis provided by the STRESS framework. To first order, we find that as the risk metric incorporates poverty and equity (i.e., non-white population) successively, the hotspots (Figure 6) as well as the top-ranked states (Table 4) move predominantly toward the southern half of the United States. Most notably, the highest ranked states in the Northeast, in terms of the physical risk of flooding, are diminished when poverty and non-white population factors are incorporated. Conversely, there is a prominent clustering and high ranking from all the risk factors combined (i.e., flood, water quality, poverty, and non-white population) in the Carolinas and surrounding states (West Virginia and Georgia). Irrespective of these notable shifts in the landscapes of risk—West Virginia, North Carolina, and Kentucky consistently rank high and experience all these combinations of risk across the largest portion of their counties.
Figure 6. As in Figure 3, but highlighting the contrast between combinatory landscapes of: (A) aggregate flood risk and water quality risk; (B) aggregate flood risk, water quality risk, and population below the poverty level; and (C) aggregate flood risk, water quality risk, population below the poverty level, and non-white population.
Table 4. Summary of results from the combinatory risk metrics considering: (1) flood and water quality; (2) flooding, water quality, and poverty; and (3) flooding, water quality, poverty, and nonwhite population.
The U.S. is a major producer and exporter of food and agricultural products (e.g., USDA, 2021; FAO, 2022). Cropland in the U.S. is exposed to several risks, among which is water stress, but can vary considerably by region. An initial visualization (Figure 7) and tabulation of the cropland area data (Table 5), which is readily downloadable from the STRESS platform, indicates that among the top-10 states that contain the highest amount of cropland area—all but one (Montana) contain or flank the sharp east-west gradient of Climate Moisture Index (CMI, see Appendix A.3 for further details) that delineates regions of precipitation excess (CMI > 0) or deficit (CMI < 0). For the case of when a county achieves a negative CMI value, this would be indicative of a salient transition into a “water stress” situation, in that irrigation will likely be required to sustain water demand for rainfed crops. We tighten this convention by assigning a threshold for a county under “water stress” when its CMI drops below −0.1 (and therefore so is the cropland within it). From this criterion, the decadal-scale shifts in CMI across the timespan of the data (between the 1980–1999 and 2000–2019 averaging periods) reveal that in all but one state (North Dakota), the total area of cropland within the top-10 cropland area states experiencing water stress increases (Table 5). The largest changes, in terms of absolute and relative change, occur in Kansas (over 1.5 M additional acres, a 6% increase), Iowa (over 2.1 M additional acres, an 8% increase), and Nebraska (over 1.1 M additional acres, a 6% increase). Another notable change is the emergence of Illinois from initially none to over 293,000 acres of farmland located in counties experiencing water stress (by our definition). Overall, over the past four decades (1980–2019) 5.5 million acres of additional farmland across the top-10 cropland-area states have been exposed to water stress.
Figure 7. (A) Map displays cropland area (acres) across U.S. counties. Gray shaded areas denote missing data and/or no cropland recorded for county. Map results based on data retrieved from the USDA National Agricultural Statistics Service (NASS). See text for further information. In panel (B), the map display Climate Moisture Index (CMI- unitless) multiplied by a factor of 10 across U.S. counties averaged for two 20-year time periods (left panels 1980–1999 and right panels 2000–2019) based on the meteorological driver data from the three reanalyses data sets compiled for the platform (top panels NARR, middle panels ERA5, and bottom panels MERRA2). See text for further information regarding the calculation of CMI as well as the reanalyses data.
Table 5. Summary of results from combinatory metrics considering: (1) cropland area (acres); (2) area of cropland experiencing “water stress” (acres) for the 1980–1999 period (i.e., left panels in Figure 7); and (3) area of cropland under water stress (acres) for the 2000–2019 period (i.e., right panels in Figure 7).
While these recent trends in water stress locate formidable risks across the country's largest cropland areas, in other areas, several risks may co-exist and reinforce each other (such as land conditions and water stress), threatening yields and increasing the chances of potential negative impacts in agricultural income and local livelihoods—especially in areas of poverty. The triage platform allows an efficient assessment of major cropland areas subject to multiple risks at the National and State levels, as also as identifying if these places are already subject to social challenges, high poverty levels or unemployment rates. Figure 8 highlights these combinations. While panels a, b, and c show the distribution of water stress, populations below poverty level and highly erodible land taken individually, panels d, e, and f combine each two of these variables. These combinations reduce the frequency of higher risk areas, mainly in the Eastern side of the country, although several “hot spots” arise. As example, water stress and highly erodible land (panel e) reinforce each other at central areas along the borders of New Mexico with Texas and Colorado as well as between Kansas and Nebraska. Combining population below poverty level to these (panel g), and comparing it with cropland area (panel h), allows to identify the highest risk areas for cropland production due to water stress and erosion and how they overlap with higher poverty levels, highlighted in panels g and h. Among the most salient areas with these multi-dimension risks include counties within California, Texas, New Mexico, Montana, and Washington.
Figure 8. Maps indicating U.S. conditions of: (A) water stress, (B) population below poverty line, (C) highly erodible land, as well as combinations of those in panels (D–G); and (H) cropland areas.
We can use the triage platform to provide insights about both transition and physical risks. In terms of transition risk, we can look at employment in fossil fuels (Figure 9A), which suggests potential risk of economic hardship if the country moves away from fossil fuels and toward low-carbon alternatives. We see that Texas, Louisiana, Oklahoma, Kansas, Wyoming, North Dakota and West Virginia stand out as having high shares of people employed in jobs related to fossil fuels. We can then combine this metric with data on the population below the poverty level (shown in Figure 9B). Areas that already have a high poverty rate and have the potential for significant job loss with a transition away from fossil fuel use are particularly vulnerable to economic distress (Figure 9C). The triage platforms can identify individual counties where this combined risk is particularly high. These areas would be good candidates for targeted job retraining programs or green jobs development to help ameliorate the transition risks.
Figure 9. Maps indicating transition and physical risks related to fossil fuels: (A) employment in fossil fuels (transition risk); (B) population below poverty level; (C) combined fossil employment and poverty (transition risk); (D) flood risk; (E) combined fossil employment and flood risk (physical risk); and (F) combined fossil employment, flood risk and poverty (both transition and physical risk).
Looking at employment in fossil fuels also tells us where the most fossil assets are located. Combining that information with flood risk (shown in Figure 9D), we can identify fossil assets at risk of flooding. The resulting combined map (Figure 9E) indicates that areas of West Virginia and western Pennsylvania have high risks of physical damage to fossil assets due to flooding. Areas to the east and west of the lower Mississippi River, as well as pockets along the Gulf Coast, also have high physical risks. Fossil assets along the Gulf Coast face additional physical risks due to sea level rise, hurricanes and storm surge. Areas identified as facing high physical risks should be further investigated to consider investments in protective measures and/or relocation. Combining poverty with these aspects of transition and physical risk related to fossil fuels (Figure 9F) reduce the aggregate relative risk in counties across northern areas of the contiguous United States.
The following case study applies the risk-triage approach from a local (town/county) perspective. Background: The owner of a company is being offered incentives to move their food processing business to a town in Vandalia, IL which is in Fayette County, IL. They are looking to locate in an area with access to reliable infrastructure (including energy and transportation), minimal flood/drought risk, and a decent economy to maintain and support their employees. They may also be interested in expanding into growing some of their own produce and would like to know if the area is conducive to that activity. The STRESS platform can help evaluate these concerns by reviewing different landscapes of socio-economic, health, and environmental risk.
Looking at Fayette, IL over the water stress dataset from 2010 to 2015, there doesn't appear to be any indications of significant water stress (Figure 10). Some of the surrounding counties may experience significant water stress (Figure 10a). There is light to moderate risk of drought when looking through the various years and models of the hydrologic drought index (not shown). The climate moisture index is around 0 for this area (not shown) which means it is not an area of extreme heat or cold on average. There is a concern when looking at the 100-year flood as the results show that this area is under high risk (Figure 10e).
Figure 10. Maps display: (a) the average water stress (unitless) from 2010 to 2015 for the state of Illinois and the result for the county of Fayette, IL is highlighted to note that it has an average water stress of 0; (b) the per capita personal income across Illinois (based on the 2019 Census data collected). Fayette, IL is on the lower end of personal income with a value of $40 k/person; and (c) the level of water quality across Illinois. Fayette, IL has some concerns regarding water quality with a ranking of 0.8 out of two where two is the worst ranking; (d) the percentage of population below the poverty level across Illinois. Fayette, IL has a moderately high percentage (16%) of people below poverty level relative to other counties; (e) the 100-year flood risk for Illinois, and depicting the high flood risk value of eight out of nine for Fayette, IL, and (f); flood map that includes overlays of major highway (thin gray lines), railways (thick gray line); as well as waterways (blue lines); and (g) flood map that includes overlay of electrical transmission lines at 345 kV, and indicating that these transmission lines run through Fayette, IL.
We can define reliable transportation infrastructure as access to major highways and waterways that are in proximity and not located in areas prone to extreme events that would degrade the quality and reliability of the infrastructure. We can also look at the surrounding areas to evaluate if there is redundancy in the infrastructure. The location of Fayette, IL has prime access to major transportation infrastructure including road, rail, and nearby major waterway. There are some redundancies in the highways as it appears to have access from north to south and east to west. Both highways and rail run through the county (Figure 10f). The Mississippi River is near allowing freight transport of food. While there is low concern regarding droughts in this area, there is a significant flood risk which could impact the transportation infrastructure. In addition to transportation infrastructure, the STRESS platform provides information on the energy infrastructure. Figure 10g shows major electrical transmission lines run through Fayette, IL (>345 kV).
The per capita personal income from 2018 was ~$30 k/person (Figure 10b). This is on the lower end of personal income. When looking at the different areas of employment, it appears that ~6% of employed people are in the category of agriculture, forestry, fishing and hunting while 18% are employed by healthcare and social assistance. The STRESS platform also provides information on population and unemployment. Fayette, IL has ~4% unemployment rate which is lower than the national average (not shown). Unfortunately, 16% of the population in this county is below the poverty level which is higher than the national average (Figure 10d). The population under 18 is 21% and over 65 is 18%, which places it within an average population age distribution relative to the national values.
Figure 10c shows Fayette, IL with poor water quality (0.8) but low to negative irrigation deficit which shows that water availability shouldn't be a concern. Although, as mentioned previously, there is significant concern of flooding. It is noted that there is no critical habitat of concern in Fayette, IL.
If we ranked all the metrics as listed below, we find that the overall risk given our prioritized concerns is about at 57/100, which we would consider a moderate risk. In comparison with the state of IL, this is about average or a bit lower than average risk (Figure 11). The resultant aggregate metric was obtained through the following combinatory weights:
• Maximum: Water Stress, Water Quality, Flood Risk, Temperature Stress, Poverty Level, and Unemployment
• Medium: Highly Erodible Cropland, Land Disturbance
• Minimum: Employment in Fossil Fuels, Population under 18, Population over 65, and Non-white Population.
Figure 11. Map depicting the overall risk that results from combining all factors from Figs. and weighing them according to the business owner's main concerns. Fayette, IL has a moderate overall risk.
In conclusion, after evaluating Fayette, Il with the STRESS platform, the company determined that while there is significant risk of flooding and a poor economy, the overall risk given the company's prioritized concerns is average. It should also be noted that the overall combined metrics did not include the enormous benefit of access to reliable transportation infrastructure (road, rail, and waterways). The risk of flooding in terms of the manufacturing plant can be minimized by locating the building outside the immediate flood zones. While poor economy was listed as a risk, the company likes to view it as an opportunity to help contribute to improving the situation by adding to employment and stimulating the local economy. The opportunity to look at growing crops appears to have some potential given the high level of agriculture already in the area, but also some concerns with water quality, land disturbance and flood potential. Investment into growing their own crops would need to be investigated further.
We have described and demonstrated a System for the Triage of Risks from Environmental and Socio-Economic Stressors (STRESS) platform that is designed to serve as visualization tool for multi-sector, combinatory risk analysis and data download. STRESS's motivating goals are to: characterize the extent that various risk factors (i.e., threats, hazards, impacts, stressors, exposures, vulnerabilities, and inequities) co-exist; compile metrics that quantify these risk factors in flexible and combinable fashion; identify how the aggregate risk landscape changes when individual risk factors are combined; and provide any user of the platform the ability explore various risk-factor combinations of their choosing. Overall, the objectives by which the STRESS platform has been and continues to be developed are (1) describe “hotspots” and prioritize further action and deeper inspection of risk or threats rather than just report data, and (2) strive for comprehensiveness, as data prepared by various agencies and institutes each have a relatively narrowed auspice (3) create normalized risk metrics that allow for user-defined, combinatory analyses across the wide range of risk factors that span human and environmental systems. The risk-triage platform is intended to be a tool in and of itself and is publicly available. As we continue to develop our platform across more of these landscapes, it can be used to motivate and guide additional analysis and deeper dives by the research community. The platform has also generated broader interest and support from various stakeholders to incorporate and integrate other landscapes of hazards and risks such as: biodiversity, human health, and systemic racism. The platform has been designed to readily incorporate additional data and model results as well as support an open-science research community. Our source code is on GitHub (github.com/cypressf/climate-risk-map). You can view discussion of technical planning and discussion on our GitHub issues link (github.com/cypressf/mit-climate-data-viz/issues).
We present several illustrative examples that highlight features and capabilities of the STRESS platform. We show that in terms of the combined air-energy-land-water risks, the most prominent “hotspots” are located across California, the upper and lower Mississippi basin, the Ohio River basin, Texas, the Southeast as well as Mid-Atlantic states. Concurrently, we find regions of the nation with the largest portion of employment in the fossil fuel industry, along with high levels of poverty and unemployment flank the lower portions of the Mississippi River. National and global actions to reduce greenhouse emissions could limit risks to land, water, and air quality in the upper basin, but at the same time impacts on the fossil fuel industry could have significant employment impacts in the lower Basin where poverty and unemployment are already disproportionate. Overall, these highlight the potential locations of and connections between contrasting regional effects of any low-carbon energy transition strategy.
Another inspection with the STRESS platform that considers state-to-state rankings of severe water stress shows regions that are robust (i.e., California, Illinois and Texas) but also sensitive to the choice of criterion upon which to base a ranking of water risk (Delaware and Nebraska). Further considerations that include socio-demographic conditions, such as poverty, can have a substantial impact on these rankings. The combination of poverty and water-stress into the risk ranking results in a notable shift to southern states that rank among the highest (New Mexico, Georgia, Kentucky, and West Virginia). A similar impact is seen when combining flood risk with poverty and ethnicity (i.e., non-white population), with a southerly shift to “hotspots” of combined risk that incorporate the socio-demographic dimensions. Further echoing these water issues, when we alternatively combine these metrics with the extent of cultivated lands, we find that over the past few decades, at least an additional 5.5 million acres of farmland are in counties exposed to increased water stress, with Iowa, Kansas, and Nebraska the top three states. However, this landscape changes considerably when combined with risks in land erosion and poverty, and the “hotspots” move to counties within California, Texas, New Mexico, Montana, and Washington. We further illustrate the platform's ability to explore multiple facets of risk through a focus on transition risks. We show that there is a large region across the south-central U.S. and Appalachia that experiences relatively high levels of fossil fuel employment and poverty—and underscores a transition risk to low-carbon energy proliferation.
These examples of co-existence of interconnected risks provide guidance and motivation for deeper-dive use case studies on human-natural system interactions; grid resiliency; and transportation infrastructure. In another demonstration, we also highlight the ability of the platform to scan multiple sectors and overlays and provide a combinatory risk inspection at a county level. The result provided a multi-dimension assessment of risk for a (hypothetical) company who had interest in expanding their business within a particular county (Fayette, IL). In this vein, we have developed the platform to be flexible such that various metrics can be augmented or changed in response to feedback or requests from the research community and more importantly, government and private stakeholders as well as citizen scientists. Such feedback may also point to areas where new data or prediction capabilities are needed.
In view of these aspects to our STRESS platform, as part of our ongoing efforts we will continue to build upon our collection of historical and contemporary variables and expand upon our list of combinatory risk metrics. There are many other overlays and different combinations of risk factors, not discussed in this study, that can be explored with the triage platform that highlights other regions or different use cases. Subsequent studies will expand upon this assessment, and we will seek opportunities to partner and complement these efforts to create as comprehensive and inclusive a repository for data-driven science in multisector dynamics. Thus far, our STRESS platform has been focused on historical and contemporary landscapes of data and combinatory metrics. Another key development task will be to expand the STRESS platform to provide a comprehensive assessment of future projections that incorporates uncertainty as well as historical variability and trends. In doing so, we expect these advances to provide insights that identify: the extent that trajectories from current, co-existing risks can compound and intensify; and the extent to which new and unprecedented risks can emerge as “hotspots” for potential tipping points. Given the ability of the STRESS platform to explore many different combinations of these emerging risks, we also expect the insights that we obtain can help prioritize and identify locations for deeper-dive studies that can assess specific actions and adaptations that are needed to reduce, remove, or reverse risks; as well as the extent to which insights gained from one region/location could be applicable to other locations facing similar risks.
The datasets presented in this study can be found in online repositories. The names of the repository/repositories and accession number(s) can be found in the article/Supplementary material.
All authors listed have made a substantial, direct, and intellectual contribution to the work and approved it for publication.
The inception and continued development of the STRESS platform coverage of the United States is supported by the Department of Energy's Multi-Sector Dynamics program (DE-FOA-0001968). In addition, the expansion of the platform's capabilities to health-equity impacts is supported by Biogen Incorporated. The authors also thank further support from sponsor membership of the MIT Joint Program on the Science and Policy of Global Change (JPSPGC). The STRESS platform is maintained on the svante high-performance computing cluster, a facility located at the MIT's Massachusetts Green High Performance Computing Center and jointly supported by: the MIT JPSPGC; Department of Earth, Atmosphere, and Planetary Sciences; Department of Civil and Environmental Engineering; the Institute for Data, Systems, and Society; and the Center for Global Change Science.
The authors declare that the research was conducted in the absence of any commercial or financial relationships that could be construed as a potential conflict of interest.
All claims expressed in this article are solely those of the authors and do not necessarily represent those of their affiliated organizations, or those of the publisher, the editors and the reviewers. Any product that may be evaluated in this article, or claim that may be made by its manufacturer, is not guaranteed or endorsed by the publisher.
The Supplementary Material for this article can be found online at: https://www.frontiersin.org/articles/10.3389/fclim.2023.1100600/full#supplementary-material
Auffhammer, M., Baylis, P., and Hausman, C. (2017). Climate change is projected to have severe impacts on the frequency and intensity of peak electricity demand across the United States. PNAS 114, 1886–1891. doi: 10.1073/pnas.1613193114
Aven, T., and Zio, E. (2021). Globalization and global risk: how risk analysis needs to be enhanced to be effective in confronting current threats. Reliability Eng. Syst. Saf. 205, 107270. doi: 10.1016/j.ress.2020.107270
Cappelli, F. V., Costantini, V., and Consoli, D. (2021). The trap of climate change-induced “natural” disasters and inequality. Global Environ. Change 70, 102329. doi: 10.1016/j.gloenvcha.2021.102329
CIESIN (2018). Gridded Population of the World, Version 4 (GPWv4): Population Density Adjusted to Match 2015 Revision UN WPP Country Totals, Revision 11. Palisades, NY: NASA Socioeconomic Data and Applications Center (SEDAC); Center for International Earth Science Information Network - Columbia, University.
Di, Q., Amini, H., Shi, L., Kloog, I., Silvern, R., Kelly, J., et al. (2019). An ensemble-based model of PM2.5 concentration across the contiguous united states with high spatiotemporal resolution. Environ. Int. 130, 104909. doi: 10.1016/j.envint.2019.104909
Di, Q., Wei, Y., Shtein, A., Hultquist, C., Xing, X., Amini, H., et al. (2021). Daily and Annual PM2.5 Concentrations for the Contiguous United States, 1-km Grids, v1 (2000 - 2016). Palisades, NY: NASA Socioeconomic Data and Applications Center (SEDAC).
FAO (2022). Trade of Agricultural Commodities. 2000–2020. FAOSTAT Analytical Brief Series No. 44. Rome: FAO.
Frank, M. W. (2009). Inequality and growth in the united states: evidence from a new state-level panel of income inequality measures. Econ. Inq. 47, 55–68. doi: 10.1111/j.1465-7295.2008.00122.x
García-Muros, X., Morris, J., and Paltsev, S. (2022). Toward a just energy transition: a distributional analysis of low-carbon policies in the USA. Energy Econ. 105, 105769. doi: 10.1016/j.eneco.2021.105769
Greco, S., Ishizaka, A., Tasiou, M., and Torrisi, G. (2019). On the methodological framework of composite indices: a review of the issues of weighting, aggregation, and robustness. Soc. Indic. Res. 141, 61–94. doi: 10.1007/s11205-017-1832-9
Hallegatte, S., Vogt-Schilb, A., Rozenberg, J., Bangalore, M., and Beaudet, C. (2018). From poverty to disaster and back: a review of the literature. Econ. Dis. Clim. Change 4, 223–247. doi: 10.1007/s41885-020-00060-5
Hochstenbach, C., and Musterd, S. (2018). Gentrification and the suburbanization of poverty: changing urban geographies through boom and bust periods. Urban Geogr. 39, 26–53. doi: 10.1080/02723638.2016.1276718
Iturbide, M., Gutiérrez, J., Alves, L., Bedia, J., Cerezo-Mota, R., Cimadevilla, E., et al. (2020). An update of IPCC climate reference regions for subcontinental analysis of climate model data: definition and aggregated datasets. Earth Syst. Sci. Data 12, 2959–2970. doi: 10.5194/essd-12-2959-2020
Jiménez-Fernández, E., Sánchez, A., and Pérez, E. S. (2022). Unsupervised machine learning approach for building composite indicators with fuzzy metric. Exp. Syst. Appl. 200, 116927. doi: 10.1016/j.eswa.2022.116927
Kiesecker, J., Baruch-Mordo, S., Kennedy, C. M., Oakleaf, J. R., et al. (2019). Hitting the target but missing the mark: unintended environmental consequences of the Paris climate agreement. Front. Environ. Sci. 7, 151. doi: 10.3389/fenvs.2019.00151
McDermott, T. K. J. (2022). Global exposure to flood risk and poverty. Nat. Commun. 13, 3529. doi: 10.1038/s41467-022-30725-6
Merriam Webster Dictionary. (2021). “triage” Merriam-Webster.com. Available online at: https://www.merriam-webster.com (accessed February 12, 2023).
Messer, L. C., Jagai, J. S., Rappazzo, K. M., and Lobdell, D. T. (2014). Construction of an environmental quality index for public health research. Environ. Health 13, 39. doi: 10.1186/1476-069X-13-39
Moss, R., Fisher-Vanden, K., Delgado, A., Backhaus, S., Barrett, C., Bhaduri, B., et al. (2016). Understanding Dynamics and Resilience in Complex Interdependent Systems. U.S. Global Change Research Program Interagency Group on Integrative Modeling. Available online at: https://climatemodeling.science.energy.gov/publications/understanding-dynamics-and-resilience-complex-interdependent-systems
Mueller, J.T., and Gasteyer, S. (2021). The widespread and unjust drinking water and clean water crisis in the United States. Nat. Commun. 12, 3544. doi: 10.1038/s41467-021-23898-z
NOAA NCEI (2022). U.S. Billion-Dollar Weather and Climate Disasters. National Centers for Environmental Information. Available online at: https://www.ncei.noaa.gov/access/billions/
Otto, I.M., Reckien, D., Reyer, C.P.O, Marcus, R., LeMasson, V., Jones, L., Norton, A., et al. (2017). Social vulnerability to climate change: a review of concepts and evidence. Reg. Environ. Change 17, 1651–1662. doi: 10.1007/s10113-017-1105-9
Paulvannan Kanmani, A, Obringer, R., Rachunok, B., and Nateghi, R. (2020). assessing global environmental sustainability via an unsupervised clustering framework. Sustainability 12, 563. doi: 10.3390/su12020563
Pescaroli, G., and Alexander, D. (2018). Understanding compound, interconnected, interacting, and cascading risks: a holistic framework. Risk Anal. 38, 2245–2257. doi: 10.1111/risa.13128
Pickard, B.R., Daniel, J., Mehaffey, M., Jackson, L. E., and Neale, A. (2015). EnviroAtlas: a new geospatial tool to foster ecosystem services science and resource management. Ecosyst. Serv. 14, 45–55. doi: 10.1016/j.ecoser.2015.04.005
Ram, R. (1982): Composite indices of physical quality of life, basic needs fulfilment, and income: a principal component representation. J. Dev. Econ. 11, 227–247. doi: 10.1016/0304-3878(82)90005-0.
Reed, P.M., Hadjimichael, A., Moss, R. H., Brelsford, C., Burleyson, C. D., Cohen, S., et al. (2022). Multisector dynamics: advancing the science of complex adaptive human-Earth systems. Earths Fut. 10, e2021EF002621. doi: 10.1029/2021EF002621
Saisana, M., Saltelli, A., and Tarantola, S. (2005). Uncertainty and sensitivity analysis techniques as tools for the quality assessment of composite indicators. J. R. Stat. Soc. Ser. A 168, 307–323. doi: 10.1111/j.1467-985X.2005.00350.x
Scott, J. H., Gilbertson-Day, J. W., Moran, C., Dillon, G. K., Short, K. C., and Vogler, K. C. (2020). Wildfire Risk to Communities: Spatial datasets of landscape-wide wildfire risk components for the United States. Fort Collins, CO: Forest Service Research Data Archive.
Sharpe, A., and Andrews, B. (2012). As Assessment of Weighting Methodologies for Composite Indicators: The Case of the Index of Economic Well-being. CSLS Research Report No. 2012-10, 49.
Stott, P. (2016). How climate change affects extreme weather events. Science 352, 1517–1518. doi: 10.1126/science.aaf7271
Strzepek, K., Schlosser, C. A., and Goudreau, J. (2021). Hydroclimatic Analysis of Climate Change Risks to Global Corporate Assets in Support of Deep-Dive Valuation. Joint Program Report Series Report 350. p. 16. Available online at: http://globalchange.mit.edu/publication/17593
Temper, L., del Bene, D., and Martinez-Alier, J. (2015). Mapping the frontiers of front lines of global environmental justice: the EJAtlas. J. Polit. Ecol. 22, 255–278. doi: 10.2458/v22i1.21108
U.S. EPA (2020). Environmental Quality Index. Technical Report (2006-2010). Washington, DC: U.S. Environmental Protection Agency, EPA/600/R-20/367. Available online at: https://cfpub.epa.gov/ncea/risk/recordisplay.cfm?deid=350545 (accessed June 5, 2022).
U.S. EPA (2022). EJSCREEN Environmental Justice Mapping and Screening Tool, EJSCREEN Technical Documentation. Washington, DC: U.S. Environmental Protection Agency Office of Policy, 115. Available online at: https://www.epa.gov/system/files/documents/2023-01/EJScreen%20Technical%20Documentation%20October%202022.pdf
USDA (2021). United States Agricultural Export Yearbook. USDA Foreign Agricultural Service, 74. Available online at: https://www.fas.usda.gov/sites/default/files/2022-04/Yearbook-2021-Final.pdf
Van Ruijven, B., Sue Wing, I., and De Cian, E. (2019). Amplification of future energy demand growth due to climate change. Nat. Commun. 10, 2762. doi: 10.1038/s41467-019-10399-3
Zuzak, C., Goodenough, E., Stanton, C., Mowrer, M., Ranalli, N., Kealey, D., et al. (2021). National Risk Index Technical Documentation. Washington, DC: Federal Emergency Management Agency. Available online at: https://www.fema.gov/sites/default/files/documents/fema_national-risk-index_technical-documentation.pdf (accessed June 5, 2022).
Keywords: environmental, socio-economic, compounding risks, visualization platform, equity, triage, screening tools
Citation: Schlosser CA, Frankenfeld C, Eastham S, Gao X, Gurgel A, McCluskey A, Morris J, Orzach S, Rouge K, Paltsev S and Reilly J (2023) Assessing compounding risks across multiple systems and sectors: a socio-environmental systems risk-triage approach. Front. Clim. 5:1100600. doi: 10.3389/fclim.2023.1100600
Received: 28 November 2022; Accepted: 13 March 2023;
Published: 24 April 2023.
Edited by:
Scott Bremer, University of Bergen, NorwayReviewed by:
Byomkesh Talukder, York University, CanadaCopyright © 2023 Schlosser, Frankenfeld, Eastham, Gao, Gurgel, McCluskey, Morris, Orzach, Rouge, Paltsev and Reilly. This is an open-access article distributed under the terms of the Creative Commons Attribution License (CC BY). The use, distribution or reproduction in other forums is permitted, provided the original author(s) and the copyright owner(s) are credited and that the original publication in this journal is cited, in accordance with accepted academic practice. No use, distribution or reproduction is permitted which does not comply with these terms.
*Correspondence: C. Adam Schlosser, Y2FzY2hAbWl0LmVkdQ==
Disclaimer: All claims expressed in this article are solely those of the authors and do not necessarily represent those of their affiliated organizations, or those of the publisher, the editors and the reviewers. Any product that may be evaluated in this article or claim that may be made by its manufacturer is not guaranteed or endorsed by the publisher.
Research integrity at Frontiers
Learn more about the work of our research integrity team to safeguard the quality of each article we publish.