- Centre for Atmospheric Sciences, Indian Institute of Technology Delhi, New Delhi, India
Ninety climate models, from four consortiums—CMIP5, CMIP6, NEX-GDDP, and CORDEX—are evaluated for the simulation of seasonal temperature and precipitation over India, and subsequently, using the best ones, their future projections are made for the country. NEX-GDDP is found to be the best performer for the simulation of surface air temperature for all the four seasons. For the simulation of precipitation, CMIP6 performs the best in DJF and MAM seasons, while NEX-GDDP performs the best in JJAS and ON seasons. The selected models suggest that temperature will increase over the entire Indian landmass, relatively more over the north-western part of the country. Furthermore, the rate of warming will be more in winter than in summer. The models also suggest that precipitation will increase over central eastern and north-eastern India in the monsoon season, and over peninsular India during post-monsoon months.
Introduction
Climate change is the greatest threat facing humanity. The unabated and unchecked rise in global temperature, mainly due to human influence, will render severe and lethal extremes in the form of blazing heatwaves, frequent and pervasive droughts, record floodings, intense storms, and among others (Hirabayashi et al., 2013; Lee and Marotzke, 2021; IPCC, 2022). India, where the indication of an impending climate change is already apparent and is expected to get even worse in an ever-warming world, is among the most vulnerable regions where a large population depends on climate sensitive sectors like agriculture, fisheries, animal husbandry, human health, tourism etc (Krishnan et al., 2020). Given the grave vulnerability of the region to climate change's consequences, it is highly imperative to have credible records of future changes to aid effective adaptation and mitigation efforts.
Global climate models (GCMs) are essential for understanding various climatic behaviors and providing future climate predictions. Since the inception of climate modeling, several improvements have been incorporated progressively, which have enhanced our understanding of the existing climate over time and provided more reliable future predictions. However, despite huge advances, GCMs are often faced with difficulties when it comes to simulating the local and regional climatic features and as a result hinders the regional impact assessments (Mishra et al., 2018a; Rana et al., 2020) imperative for formulating informed adaptation and mitigation efforts. Several studies showed an unsatisfactory performance of global models over India (Mishra et al., 2018a; Jain et al., 2019a), especially for the precipitation, and reported the presence of large biases in their simulations. These biases in global models are often attributed to poorly resolved physical processes, coarser resolutions, oversimplified parameterization of complex climate-relevant processes (Wei and Qiao, 2017; Mishra et al., 2018a), among others.
To overcome this difficulty and to make impact assessments at local and regional scales more promising, one of the approaches is the downscaling of output from global models to generate a higher resolution picture. There are two downscaling methods such as statistical and dynamical downscaling. Dynamical downscaling uses regional models often driven by global model output to generate region-specific climate information (Giorgi et al., 2009), whereas statistical downscaling uses statistical techniques to produce high resolution climate information. One of the international efforts to obtain dynamically downscaled products is the Coordinated Regional Climate Downscaling Experiment (CORDEX, Giorgi et al., 2009), which provides finer-scale regional projections. The CORDEX datasets showed promising improvement in simulating several climatic features for many countries (Endris et al., 2013), but for India, no significant improvements are exhibited, although efforts have been made by several authors (Mishra et al., 2018b; Jain et al., 2019a). Previous regional climate modeling studies showed useful results at the levels of homogenous zones and cities (Dash et al., 2013, 2015). Based on some previous studies, one can infer that some of the dynamically downscaled climate products are useful, even at the city level (Dash et al., 2013, 2015; Pattnayak et al., 2013). However, there are questions about the robustness of those results. Keeping this in view, the single model studies or multi-model mean (MMM) from a single set of experiments such as CIMP5 and CMIP6 (Menon et al., 2013; Sharmila et al., 2015; Moon and Ha, 2020) are not adequate to be used to provide reliable future projections, particularly at the regional scales. Therefore, taking the MMM based on models from different consortiums, such as CMIP5, CMIP6, CORDEX, and NEX-GDDP, is one solution, which not only facilitates intercomparison but also facilitates quantification of uncertainties in a way to produce reliable projections. Considering the heterogeneity of the Indian climate, it is very essential to examine the state-of-the-art downscaled climate products for their societal use at the regional and local levels. Similarly, several statistical downscaling methods have been used to generate high-resolution features. One such product includes the National Aeronautics and Space Administration (NASA) Earth Exchange Global Daily Downscaled Projections (NEX-GDDP, Thrasher et al., 2012). The dataset has been featured in several studies and reported to have alleviated major biases in temperature and precipitation simulations, including over India (Jain et al., 2019b; Sahany et al., 2019). More details of these datasets are given in the Data and Methodology section.
Overall, each dataset has its own advantages and disadvantages; hence, while generating future projections, it is imperative to assess the capacity of different datasets to reproduce the observed features. This study is one such work in that direction. The objectives of this study are (i) to evaluate the performance of CMIP6, CMIP5, NEX-GDDP, and CORDEX in simulating the seasonal surface air temperature and precipitation over India with respect to observations during four different seasons, namely, December to February (DJF), March to May (MAM), June to September (JJAS), and October and November (ON), and to select the most suitable product that shows better performance, among others, in simulating surface air temperature and precipitation during each of the seasons, and (ii) to generate future projections for each of the seasons from the selected models under the most aggressive emission scenarios.
Data and methodology
The India Meteorological Department (IMD) provides gridded high-resolution daily temperature and precipitation data for regional climate applications. In this study, daily observations of surface air temperature (at 1.0° × 1.0° resolution) and precipitation (at 0.25° × 0.25° resolution) from the IMD are used for the period 1975–2005 (Srivastava et al., 2009; Pai et al., 2014). This study analyzes the daily and monthly mean surface air temperatures and precipitation outputs from 10 CORDEX South Asia models from 1975 to 2005 (http://cccr.tropmet.res.in/cordex/files/downloads.jsp). Monthly data on surface air temperature and precipitation from historical simulations of 21 selected Coupled Model Intercomparison Project, Phase 5 (CMIP5) models are analyzed for 1975–2005. CMIP5 (Taylor et al., 2012) datasets are available from the ESGF (https://esgf-index1.ceda.ac.uk/search/cmip5-ceda). Similarly, the monthly data from historical simulations of selected 38 CMIP6 models (Eyring et al., 2016) are used for 1975–2005. The new future societal development pathways, known as shared socioeconomic pathways (SSPs), the high-forcing scenario SSP5-8.5 (2015–2099), are used in this study (O'Neill et al., 2016). The CMIP6 datasets are available from the ESGF (https://esgf-node.llnl.gov/search/cmip6).
The National Aeronautics and Space Administration (NASA) Earth Exchange Global Daily Downscaled Projections (NEX-GDDP) constitute a set of global, high-resolution, bias-corrected data that has been statistically downscaled from the CMIP5 model outputs. The bias correction is done by utilizing the global climate data from the Global Meteorological Forcing Dataset (GMFD) provided by the Terrestrial Hydrology Research Group at Princeton University (Sheffield et al., 2006) using the bias-corrected spatial disaggregation (BCSD) method (Wood et al., 2002, 2004; Thrasher et al., 2012). The CMIP5 model data are first compared with the GMFD data for the period 1950–2005 using quantile mapping, and the corrections obtained are used to adjust the future projections. The NEX-GDDP daily data from the same 21 CMIP5 models are used for 1975–2005 (historical) and 2015–2099 (RCP8.5 scenario; Thrasher et al., 2012). We also used (Tables 1, 2) the CORDEX (10 models), CMIP5 (21 models, the same models as NEX-GDDP), CMIP6 (38 models), and NEX-GDDP (21 models) to compute the Multi-model ensemble means (MMMs). The surface air temperatures from IMD, CORDEX, CMIP5, and CMIP6 are re-gridded to NEX-GDDP (at 0.25° × 0.25°) resolution for comparison. The statistical significance of Pearson's correlation is determined by a two-tailed Student's t-test that is used to test the null hypothesis. The trends in temperature and precipitation are calculated using the Theil–Sen estimator (Theil, 1950; Sen, 1968), and the significance is estimated using the Mann–Kendall test (Mann, 1945; Kendall, 1975).
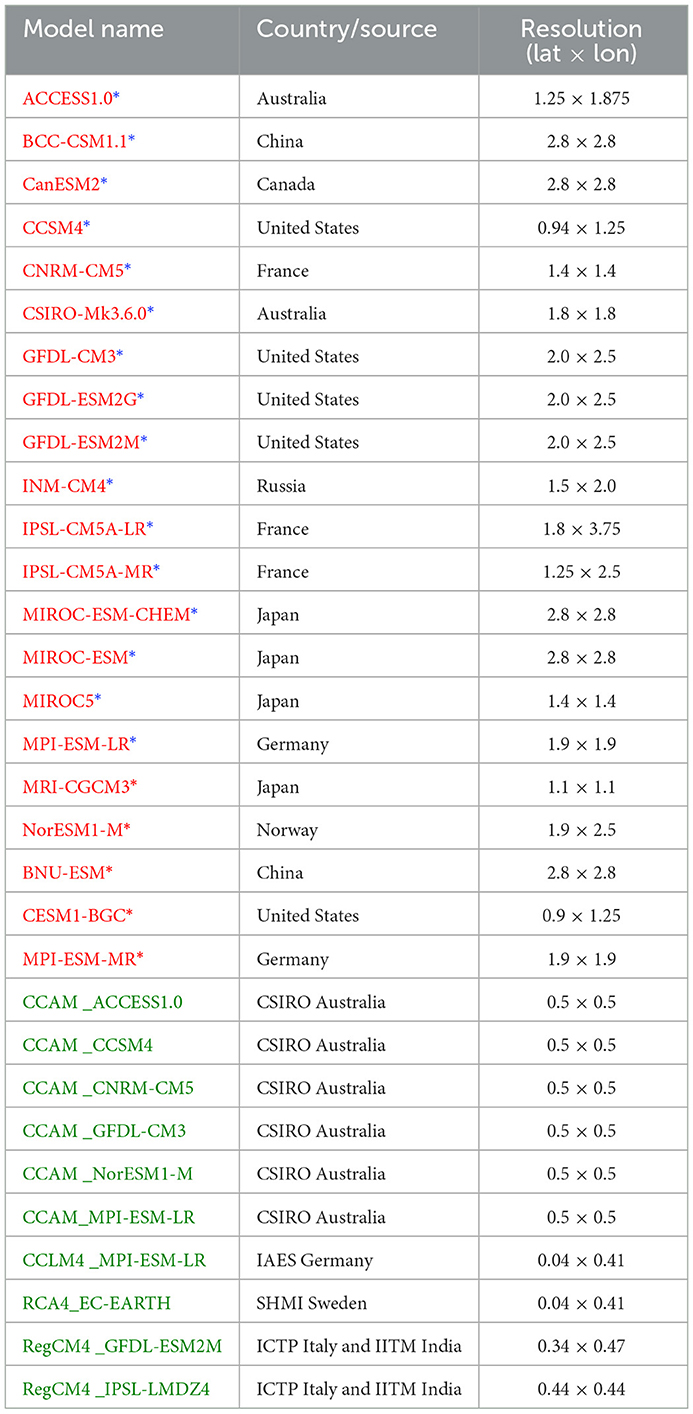
Table 1. List of CMIP5 (red color), NEX-GDDP (blue asterisk and blue color), and CORDEX (green color) models used in this study.
Results
The performance of models in simulating area-averaged surface air temperature (hereafter temperature) and precipitation over the four seasons, their root mean square error (RMSE) and pattern correlation coefficient (PCC) values, is calculated with respect to observations from 1975 to 2005 (Figure 1).
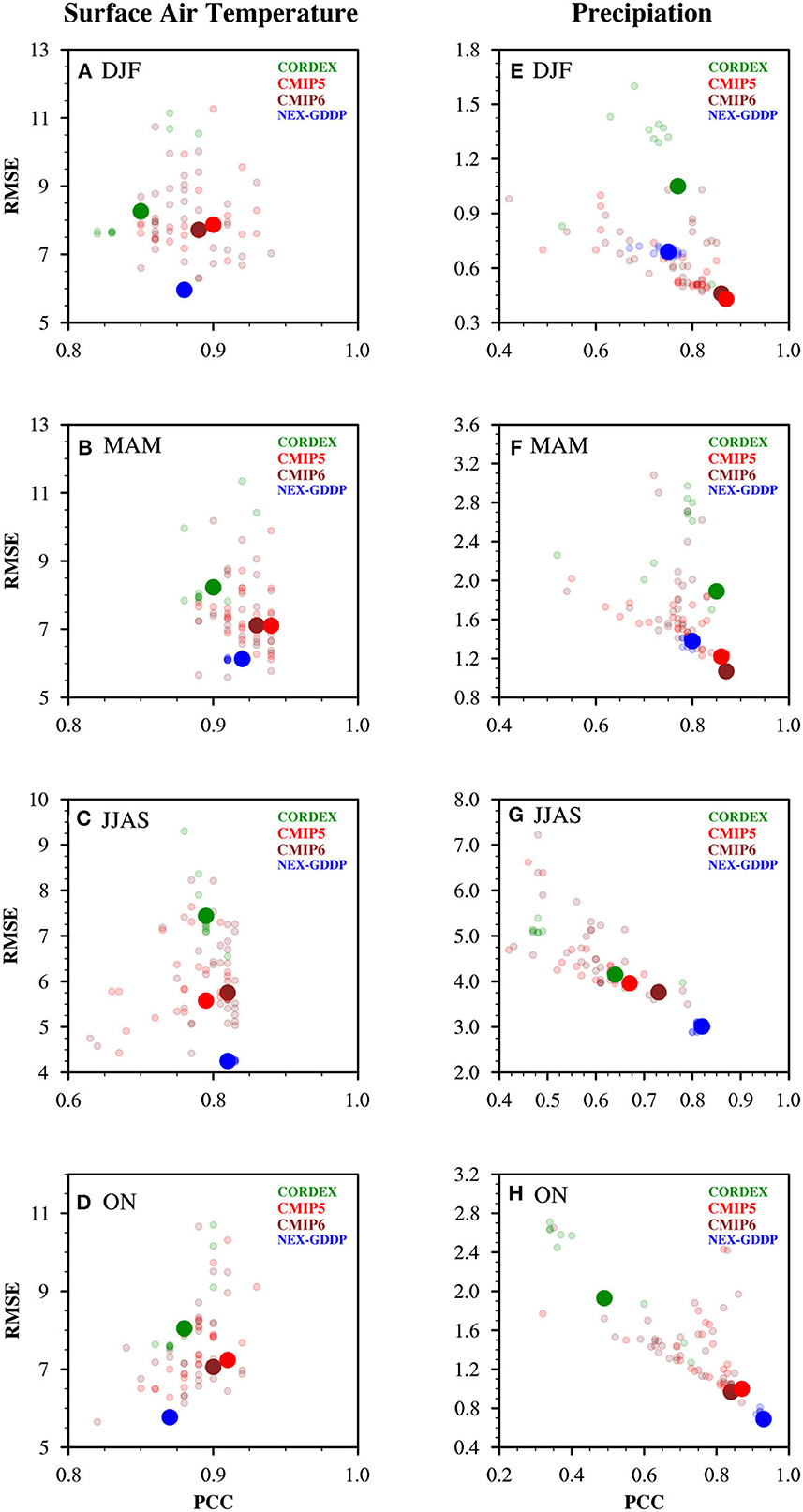
Figure 1. Root mean square error (RMSE) and pattern correlation coefficient (PCC) values of area-averaged mean surface air temperature (A–D) and precipitation (E–G) with respect to IMD observations for the period 1975–2005 over India for DJF (A, E), MAM (B, F), JJAS (C, G), and ON (D, H). The green, red, brown, and blue colors represent the CORDEX, CMIP5, CMIP6, and NEX-GDDP models, respectively. Smaller dots represent individual models and the larger dots represent MMM.
For temperature (Figures 1A–D), CORDEX MMM shows higher RMSE values, whereas NEX-GDDP MMM shows lower values for all the four seasons (DJF, MAM, JJAS, and ON). CMIP5 and CMIP6 MMM do not exhibit much difference and have comparable RMSE and PCC values during all the four seasons. These figures show that the NEX-GDPP (hereafter NEX) model shows little spread, whereas the CORDEX, CMIP5, and CMIP6 models exhibit a considerable spread in their RMSE and PCC values. CMIP5 MMM exhibits higher PCC values for all the seasons except for JJAS, during which NEX shows a higher PCC. Higher PCC values of CMIP5 MMM in DJF (0.9), MAM (0.94), and ON (0.91) are comparable to that of NEX (0.88 for DJF, 0.92 for MAM, and 0.87 for ON). NEX is found to be the best performer for the simulation of temperature for all the four seasons. Therefore, reasonably large PCC and lower RMSE values together make NEX a better option among others in simulating temperature and thus can be used to generate credible future projections.
For precipitation (Figures 1E–H), CMIP5 and CMIP6 exhibit higher and comparable PCC and RMSE values during DJF and MAM seasons; however, given the sizeable amount of improvement incorporated in CMIP6 (Volodin and Gritsun, 2018; Tatebe et al., 2019; Wu et al., 2019; Bi et al., 2020; Boucher et al., 2020) and several studies reporting the improved skill of CMIP6 over India (Gusain et al., 2020; Choudhury et al., 2022), we thereby found that CMIP6 is a better option for both seasons. During JJAS and ON, it is clear that NEX outperforms the other MMMs in terms of PCC and RMSE values, providing it with a better choice for generating future projections during these two seasons.
After selecting suitable MMMs for each of the seasons in simulating the historical mean temperature and precipitation, we go ahead to present the future projections over India. Figure 2 presents the spatial pattern of trends in temperature and precipitation during all the four seasons for the period 2015–2099 under the RCP8.5 emission scenario for CMIP5 products and SSP5-8.5 for CMIP6 products. Stippling denotes trends significant at 99% level.
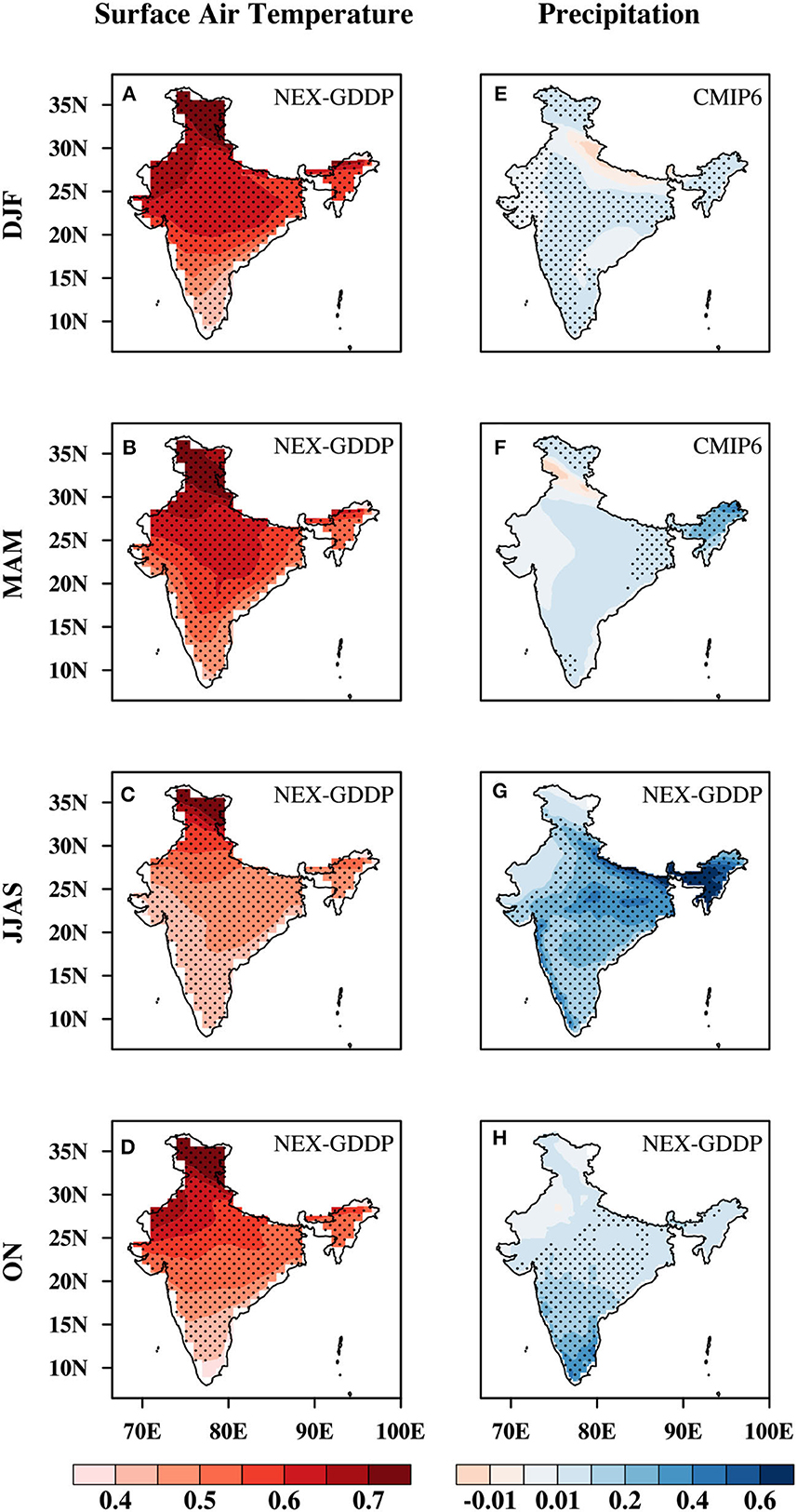
Figure 2. Spatial pattern of future trends in surface air temperature (A–D) and precipitation (E–H) for the period 2015–2099 for DJF (A, E), MAM (B, F), JJAS (C, G), and ON (D, H). The text inside the box indicates the MMM used to generate the trends. Stippling shows trends significant at the 99% confidence level. The emission scenarios used for NEX-GDDP and CMIP6 are RCP8.5 and SSP5-8.5, respectively.
The temperature trends for 2015–2099 (RCP8.5 scenario) using the NEX data are shown in Figures 2A–D. A pervasive warming trend is shown during all the four seasons, increasing progressively in the higher latitudes. The maximum warming is noted over the north and northwestern part of India during all the seasons. It can be seen that the maximum warming trends over the northern Himalayas (>0.7°C decade−1) and minimum warming trends (0.4–0.55°C decade−1) over the peninsular India during all seasons. During DJF and MAM, relatively maximum warming trends (>0.6°C decade−1) are observed over the northwest and parts of central northeast regions, with a moderate warming rate (0.55–0.65°C decade−1). During JJAS, relatively minimum warming trends are observed compared to other seasons, with a higher magnitude of trends over the northern Himalayas (>0.7°C decade−1), moderate over north-western, central northeast, and parts of Indian peninsular regions (0.4–0.6°C decade−1). During ON, the maximum rate of warming is seen over the northern Himalayas and parts of north-western regions (>0.6°C decade−1), moderate warming over remaining parts of the north-western region, central northeast regions (0.5–0.6°C decade−1), and a minimum rate over the peninsular region (<0.5°C decade−1).
The future trends in precipitation under SSP5-8.5 and RCP8.5 scenarios are shown in Figures 2E–H; for DJF and MAM, we have selected CMIP6 as a better candidate (justified aforementioned), whereas for JJAS and ON, NEX MMM is selected for credible future projections. The monsoon precipitation is expected to increase significantly almost over the entire Indian landmass except for parts of north-western regions and parts of the northern Himalayas, whereas, for other seasons, a significant increase in precipitation is expected over the limited parts of India. A uniformly increasing precipitation trend (0.1 mm day−1 decade−1) over most parts of the country during DJF is also noted, accompanied by a significantly increasing trend over the northeast (0.1–0.3 mm day−1 decade−1) during MAM. During JJAS, the north-eastern region exhibits a higher rate of increase (>0.5 mm day−1 decade−1), central northeast regions and Western Ghats a moderate rate of increase (0.3–0.5 mm day−1 decade−1), and peninsular regions a lower rate of increase (0.1–0.2 mm day−1 decade−1). During ON, significantly increasing trends are observed over the peninsular region (0.3–0.5 mm day−1 decade−1) and parts of west central and central northeast regions (0.2–0.3 mm day−1 decade−1). The remaining regions show weak insignificantly increasing trends.
Figure 3 presents the time series of weighted area averaged temperature and precipitation projections during the four seasons from their corresponding suitable MMMs for the period 2015–2099. The solid lines indicate the time series from MMMs, and the shading represents the intermodel standard deviation.
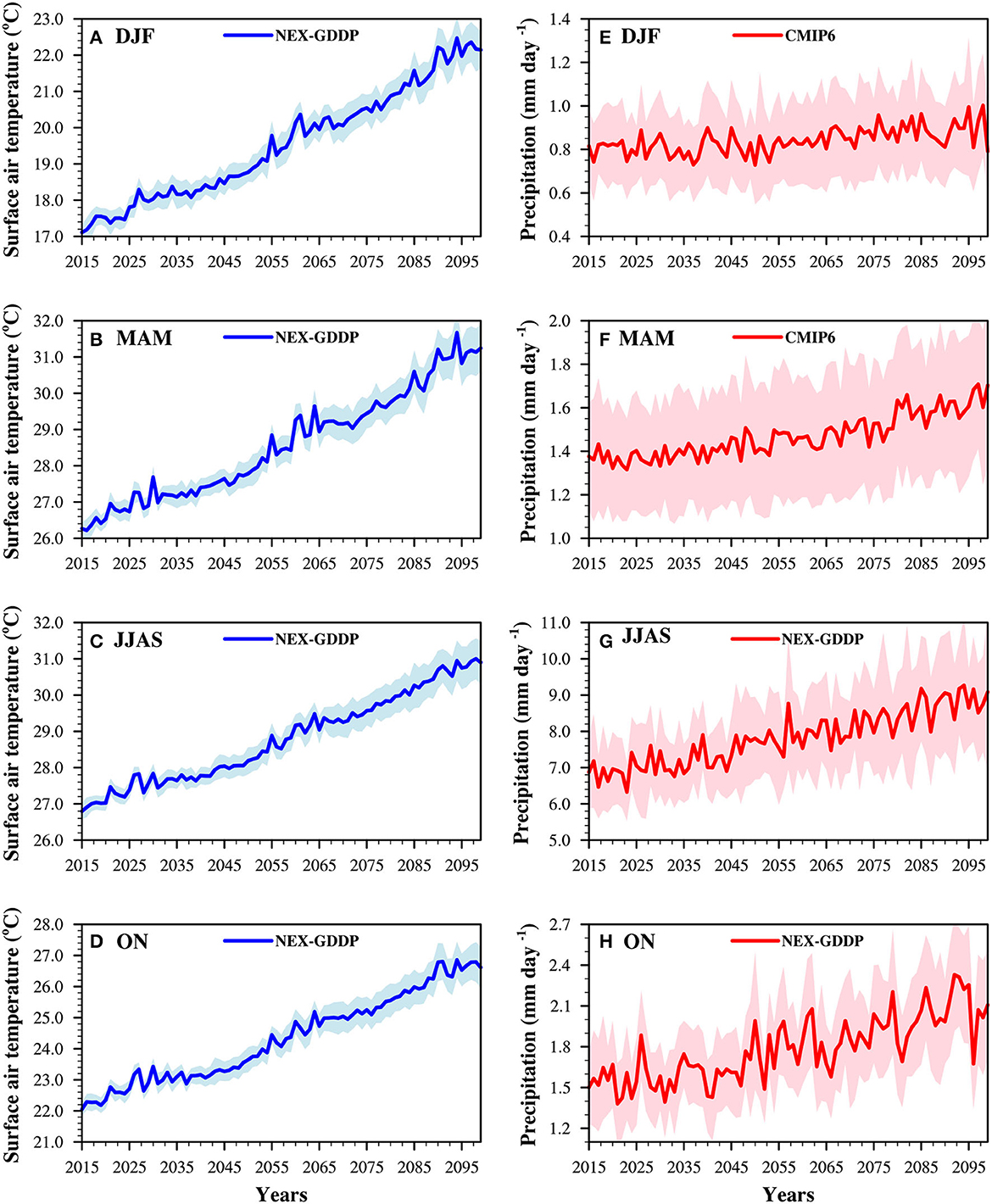
Figure 3. Time series of surface air temperature (A–D) and precipitation (E–H) for the period 2015-−2099 for DJF (A, E), MAM (B, F), JJAS (C, G), and ON (D, H). The text inside the box indicates the MMM used to generate the time series. The solid lines show the MMM and the shadings show the intermodel standard deviation. The emission scenarios used for NEX-GDDP and CMIP6 are RCP8.5 and SSP5-8.5, respectively.
Temperature (Figures 3A–D) will steadily increase in the future during all seasons. The rate of warming is by and large the same during all the seasons except for JJAS which exhibits a relatively lower rate. The warming level at end of the twenty-first century is ~5°C during all the seasons except for monsoon season, which shows a relatively lower warming (4°C).
Unlike temperature, precipitation exhibits a higher intermodal standard deviation (Figures 3E–H), hence a higher uncertainty in the projected values. Precipitation increases during all seasons, projecting a higher precipitation by the end of the twenty-first century. During DJF and MAM, CMIP6 projects an increase in precipitation (0.2–0.3 mm day−1); however, during DJF, the rate of increase in precipitation is relatively low. Among all the seasons, JJAS exhibits a higher increase by ~2 mm day−1. During ON, a large interannual variability can be seen, indicating that the region would encounter more erratic rainfall during that season. The projected increase for that season would be ~0.5 mm day−1 by the end of the century.
Discussion and conclusions
The performance of major modeling consortiums (CMIP5, CMIP6, NEX-GDDP, and CORDEX) to represent surface air temperature and precipitation across the Indian landmass over four seasons (DJF, MAM, JJAS, and ON) has been examined. Following that, the products that best represent temperature and precipitation for each season were selected, and future projections were created using the models selected. We find that for temperature, the NEX-GDDP showed a better agreement with the observations in simulating the historical (1975–2005) temperatures during all the four seasons. For precipitation, CMIP6 had the best performance during DJF and MAM, but during JJAS and ON, NEX-GDDP showed far better agreement with the observations.
We find that the Indian subcontinent will experience widespread warming with stronger warming over the higher latitudes. Maximum warming will be over the northwestern parts of the country, whilst the peninsular region will experience relatively lesser warming. Among all seasons, the monsoon season will witness the least warming. Similarly, future precipitation projections reveal that monsoon precipitation is expected to increase significantly over most parts of the Indian landmass.
We find that the temperature will increase steadily in future during all the seasons, with maximum warming of ~4–5°C by the end of the current century. Similarly, future precipitation projections show an increasing trend during all four seasons, with the monsoon season experiencing the maximum increase in precipitation by ~2 mm day−1. Rising mean temperatures may lead to an increase in high-temperature extremes in future and an increase in both mean and variability of precipitation might have a strong influence on changes in high-precipitation extremes. These findings would be useful for future planning.
Data availability statement
This study uses the data from CMIP5 and CMIP6 models available at the Earth System Grid Federation (ESGF; https://esgf-index1.ceda.ac.uk/search). The NEX-GDDP data can be found at this link (https://ds.nccs.nasa.gov/thredds/catalog/bypass/NEX-GDDP/catalog.html). The CORDEX South Asia models data can be found at this link (http://cccr.tropmet.res.in/cordex/files/downloads.jsp). The IMD data can be found at this link (http://www.imd.gov.in/).
Author contributions
PS and SM conceived the idea and designed the work. PS processed the data and created the figures. PS and NK performed the analysis and wrote the manuscript with the inputs from all the co-authors. All authors contributed to the article and approved the submitted version.
Funding
The research is partly supported by the DST Center of Excellence in Climate Modeling at IIT Delhi and the YES Foundation.
Acknowledgments
We thank the modeling groups for making their data available through CMIP5, CMIP6, NEX-GDDP, and CORDEX. We thank the IMD for providing data. Figures are created using NCAR/NCL software version 6.4.0.
Conflict of interest
The authors declare that the research was conducted in the absence of any commercial or financial relationships that could be construed as a potential conflict of interest.
Publisher's note
All claims expressed in this article are solely those of the authors and do not necessarily represent those of their affiliated organizations, or those of the publisher, the editors and the reviewers. Any product that may be evaluated in this article, or claim that may be made by its manufacturer, is not guaranteed or endorsed by the publisher.
References
Bi, D., Dix, M., Marsland, S., O'farrell, S., Sullivan, A., Bodman, R., et al. (2020). Configuration and spin-up of ACCESS-CM2, the new generation Australian community climate and earth system simulator coupled model. J. Southern Hemisph. Earth Syst. Sci. 70, 225–251. doi: 10.1071/ES19040
Boucher, O., Servonnat, J., Albright, A. L., Aumont, O., Balkanski, Y., Bastrikov, V., et al. (2020). Presentation and evaluation of the IPSL-CM6A-LR climate model. J. Adv. Model. Earth Syst. 12, e2019MS002010. doi: 10.1029/2019MS002010
Choudhury, B. A., Rajesh, P. V., Zahan, Y., and Goswami, B. N. (2022). Evolution of the Indian summer monsoon rainfall simulations from CMIP3 to CMIP6 models. Clim. Dyn. 58, 2637–2662. doi: 10.1007/s00382-021-06023-0
Dash, S. K., Mamgain, A., Pattnayak, K. C., and Giorgi, F. (2013). Spatial and temporal variations in Indian summer monsoon rainfall and temperature: An analysis based on RegCM3 simulations. Pure Appl. Geophys. 170, 655–674. doi: 10.1007/s00024-012-0567-4
Dash, S. K., Pattnayak, K. C., Panda, S. K., Vaddi, D., and Mamgain, A. (2015). Impact of domain size on the simulation of Indian summer monsoon in RegCM4 using mixed convection scheme and driven by HadGEM2. Clim. Dyn. 44, 961–975. doi: 10.1007/s00382-014-2420-1
Endris, H. S., Omondi, P., Jain, S., Lennard, C., Hewitson, B., Chang'a, L., et al. (2013). Assessment of the performance of CORDEX regional climate models in simulating East African rainfall. J. Clim. 26, 8453–8475. doi: 10.1175/JCLI-D-12-00708.1
Eyring, V., Bony, S., Meehl, G. A., Senior, C. A., Stevens, B., Stouffer, R. J., et al. (2016). Overview of the Coupled Model Intercomparison Project Phase 6 (CMIP6) experimental design and organization. Geoscientific Model Dev. 9, 1937–1958. doi: 10.5194/gmd-9-1937-2016
Giorgi, F., Jones, C., and Asrar, G. R. (2009). Addressing climate information needs at the regional level: The CORDEX framework. World Meteorol. Org. Bullet. 58, 175.
Gusain, A., Ghosh, S., and Karmakar, S. (2020). Added value of CMIP6 over CMIP5 models in simulating Indian summer monsoon rainfall. Atmos. Res. 232, 104680. doi: 10.1016/j.atmosres.2019.104680
Hirabayashi, Y., Mahendran, R., Koirala, S., Konoshima, L., Yamazaki, D., Watanabe, S., et al. (2013). Global flood risk under climate change. Nat. Clim. Change 3, 816–821. doi: 10.1038/nclimate1911
IPCC (2022). “Climate change 2022: Impacts, adaptation, and vulnerability,” in Contribution of Working Group II to the Sixth Assessment Report of the Intergovernmental Panel on Climate Change, eds H.-O. Pörtner, D. C. Roberts, M. Tignor, E. S. Poloczanska, K. Mintenbeck, A. Alegría, et al. (Cambridge; New York, NY: Cambridge University Press), 3,056.
Jain, S., Salunke, P., Mishra, S. K., and Sahany, S. (2019a). Performance of CMIP5 models in the simulation of Indian summer monsoon. Theoret. Appl. Climatol. 137, 1429–1447. doi: 10.1007/s00704-018-2674-3
Jain, S., Salunke, P., Mishra, S. K., Sahany, S., and Choudhary, N. (2019b). Advantage of NEX-GDDP over CMIP5 and CORDEX data: Indian summer monsoon. Atmos. Res. 228, 152–160. doi: 10.1016/j.atmosres.2019.05.026
Krishnan, R., Sanjay, J., Gnanaseelan, C., Mujumdar, M., Kulkarni, A., and Chakraborty, S. (2020). Assessment of Climate Change Over the Indian Region: A Report of the Ministry of Earth Sciences (MOES), Government of India (Berlin: Springer Nature), 226. doi: 10.1007/978-981-15-4327-2
Lee, J. Y., and Marotzke, J. (2021). Climate Change 2021: The Physical Science Basis. (Cambridge: Cambridge University Press), 553–672.
Menon, A., Levermann, A., and Schewe, J. (2013). Enhanced future variability during India's rainy season. GRL 40, 3242–3247. doi: 10.1002/grl.50583
Mishra, S. K., Sahany, S., and Salunke, P. (2018a). CMIP5 vs. CORDEX over the Indian region: how much do we benefit from dynamical downscaling? Theoret. Appl. Climatol. 133, 1133–1141. doi: 10.1007/s00704-017-2237-z
Mishra, S. K., Sahany, S., Salunke, P., Kang, I. S., and Jain, S. (2018b). Fidelity of CMIP5 multi-model mean in assessing Indian monsoon simulations. NPJ Clim. Atmos. Sci. 1, 1–8. doi: 10.1038/s41612-018-0049-1
Moon, S., and Ha, K. J. (2020). Future changes in monsoon duration and precipitation using CMIP6. NPJ Clim. Atmos. Sci. 3, 1–7. doi: 10.1038/s41612-020-00151-w
O'Neill, B. C., Tebaldi, C., Vuuren, D. P. V., Eyring, V., Friedlingstein, P., Hurtt, G., et al. (2016). The scenario model intercomparison project (ScenarioMIP) for CMIP6. Geoscientific Model Dev. 9, 3461–3482. doi: 10.5194/gmd-9-3461-2016
Pai, D. S., Sridhar, L., Rajeevan, M., Sreejith, O. P., Satbhai, N. S., and Mukhopadhyay, B. (2014). Development of a new high spatial resolution (0.25 × 0.25) long period (1901–2010) daily gridded rainfall data set over India and its comparison with existing data sets over the region. Mausam 65, 1–18. doi: 10.54302/mausam.v65i1.851
Pattnayak, K. C., Panda, S. K., and Dash, S. K. (2013). Comparative study of regional rainfall characteristics simulated by RegCM3 and recorded by IMD. Glob. Planet Change 106, 111–122. doi: 10.1016/j.gloplacha.2013.03.006
Rana, A., Nikulin, G., Kjellström, E., Strandberg, G., Kupiainen, M., Hansson, U., et al. (2020). Contrasting regional and global climate simulations over South Asia. Clim. Dyn. 54, 2883–2901. doi: 10.1007/s00382-020-05146-0
Sahany, S., Mishra, S. K., and Salunke, P. (2019). Historical simulations and climate change projections over India by NCAR CCSM4: CMIP5 vs. NEX-GDDP. Theoret. Appl. Climatol. 135, 1423–1433. doi: 10.1007/s00704-018-2455-z
Sen, P. K. (1968). Estimates of the regression coefficient based on Kendall's tau. J. Am. Stat. Assoc. 63, 1379–1389. doi: 10.1080/01621459.1968.10480934
Sharmila, S., Joseph, S., Sahai, A. K., Abhilash, S., and Chattopadhyay, R. (2015). Future projection of Indian summer monsoon variability under climate change scenario: an assessment from CMIP5 climate models. Glob. Planet Change 124, 62–78. doi: 10.1016/j.gloplacha.2014.11.004
Sheffield, J., Goteti, G., and Wood, E. F. (2006). Development of a 50-year high-resolution global dataset of meteorological forcings for land surface modeling. J. Clim. 19, 3088–3111. doi: 10.1175/JCLI3790.1
Srivastava, A. K., Rajeevan, M., and Kshirsagar, S. R. (2009). Development of a high resolution daily gridded temperature data set (1969–2005) for the Indian region. Atmos. Sci. Lett. 10, 249–254. doi: 10.1002/asl.232
Tatebe, H., Ogura, T., Nitta, T., Komuro, Y., Ogochi, K., Takemura, T., et al. (2019). Description and basic evaluation of simulated mean state, internal variability, and climate sensitivity in MIROC6. Geoscientific Model Dev. 12, 2727–2765. doi: 10.5194/gmd-12-2727-2019
Taylor, K. E., Stouffer, R. J., and Meehl, G. A. (2012). An overview of CMIP5 and the experiment design. Bullet. Am. Meteorol. Soc. 93, 485–498. doi: 10.1175/BAMS-D-11-00094.1
Theil, H. (1950). “A rank-invariant method of linear and polynomial regression analysis,” in Nederlandse Akademie van Wetenschappen, Series A (Amsterdam: Statistical Department of the Mathematisch Centrum), 386–392.
Thrasher, B., Maurer, E. P., McKellar, C., and Duffy, P. B. (2012). Bias correcting climate model simulated daily temperature extremes with quantile mapping. Hydrol. Earth Syst. Sci. 16, 3309–3314. doi: 10.5194/hess-16-3309-2012
Volodin, E., and Gritsun, A. (2018). Simulation of observed climate changes in 1850–2014 with climate model INM-CM5. Earth Syst. Dyn. 9, 1235–1242. doi: 10.5194/esd-9-1235-2018
Wei, M., and Qiao, F. (2017). Attribution analysis for the failure of CMIP5 climate models to simulate the recent global warming hiatus. Sci. China Earth Sci. 60, 397–408. doi: 10.1007/s11430-015-5465-y
Wood, A. W., Leung, L. R., Sridhar, V., and Lettenmaier, D. P. (2004). Hydrologic implications of dynamical and statistical approaches to downscaling climate model outputs. Clim. Change 62, 189–216. doi: 10.1023/B:CLIM.0000013685.99609.9e
Wood, A. W., Maurer, E. P., Kumar, A., and Lettenmaier, D. P. (2002). Long-range experimental hydrologic forecasting for the eastern United States. J. Geophys. Res. 107, ACL-6. doi: 10.1029/2001JD000659
Keywords: Indian land, climate change, multi-model mean, seasonal temperature and precipitation, future trends
Citation: Salunke P, Keshri NP, Mishra SK and Dash SK (2023) Future projections of seasonal temperature and precipitation for India. Front. Clim. 5:1069994. doi: 10.3389/fclim.2023.1069994
Received: 14 October 2022; Accepted: 20 January 2023;
Published: 24 February 2023.
Edited by:
Swadhin Kumar Behera, Japan Agency for Marine-Earth Science and Technology (JAMSTEC), JapanReviewed by:
Satyaban B. Ratna, University of East Anglia, United KingdomRaju Attada, Indian Institute of Science Education and Research Mohali, India
Copyright © 2023 Salunke, Keshri, Mishra and Dash. This is an open-access article distributed under the terms of the Creative Commons Attribution License (CC BY). The use, distribution or reproduction in other forums is permitted, provided the original author(s) and the copyright owner(s) are credited and that the original publication in this journal is cited, in accordance with accepted academic practice. No use, distribution or reproduction is permitted which does not comply with these terms.
*Correspondence: Saroj Kanta Mishra, skm@iitd.ac.in