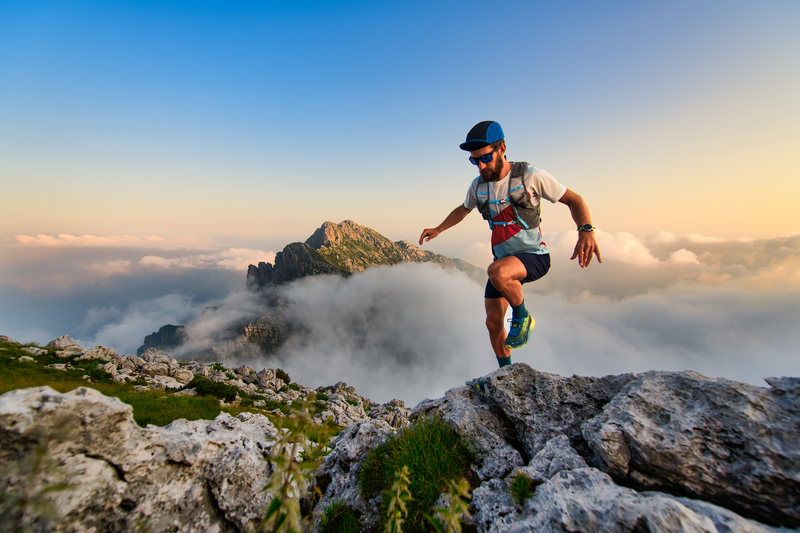
94% of researchers rate our articles as excellent or good
Learn more about the work of our research integrity team to safeguard the quality of each article we publish.
Find out more
ORIGINAL RESEARCH article
Front. Clim. , 16 January 2023
Sec. Predictions and Projections
Volume 4 - 2022 | https://doi.org/10.3389/fclim.2022.993511
This article is part of the Research Topic How Climate Predictions From Days to Decades Ahead Can Contribute to a More Sustainable Society View all 4 articles
Deciding when to plant is critical for smallholders in Africa. If they plant too early, farmers risk seedling death if the rains are not established; if they plant too late, there will not be enough rain to sustain the crop through critical development periods. In this study, we present a new decision support tool (DST) that accounts for the trade-off in the risks of early and late planting through advisories based on both short- and long-range forecasts of crop water availability. Unlike most existing operational systems, which are based solely on rainfall, the DST presented here uses ensemble forecasts of soil moisture to estimate the optimal planting date at a local scale. Evaluations using >30,000 observations of planting date and yield in Kenya, Rwanda, Uganda, Zambia and Malawi demonstrate that that planting at the optimal time would increase yield by 7–10% overall, and up to 20% for late planting farmers. The DST has been piloted by One Acre Fund for the 2019–2020, 2020–2021, and 2021–2022 seasons and there is strong demand for the service to be extended further. We conclude from the evaluations and pilots that the planting date DST has the potential to strengthen farmer decision making and hence their resilience to climate variability and change.
Global agriculture is highly vulnerable to climate variability and hence to the impacts of climate change (for example, Shi and Tao, 2014; Serdeczny et al., 2017; Bedeke, 2022). Farmers have several adaptation options open to them, including using different seed varieties, irrigation, crop diversification and adjusting the timing of cropping. All of these strategies require effective delivery of agricultural climate services (Naab et al., 2019).
Farmer surveys suggest that, in rainfed tropical annual cropping systems, adjusting cropping periods by modifying planting time is a frequently adopted strategy (Kidane et al., 2022). In part, this is because, unlike other strategies such as changing crop variety, planting at optimal time incurs minimal cost for farmers. In this context, the optimal date is the planting date that maximizes yield. Several authors have, moreover, proposed modification of planting date as a means of adapting to climate change (for example, Dharmarathna et al., 2014; Waongo et al., 2015; Dobor et al., 2016).
The impact of modifying planting date stems from the strong link between the timing of planting and yield, for a wide range of crops, including soybean (Egli and Bruening, 1992; Lee et al., 2008; Egli and Cornelius, 2009), sweet corn (Garcia et al., 2009), maize (Kombiok and Clottey, 2003), and chick pea (López-Bellido et al., 2008). Excessively early planting may result in seedling death if the rainy season is not yet established. A field survey conducted in Niger demonstrated, for example, that adhering to the local “rule of thumb” of planting after 2 days of rain places crops at risk of failure due to extended dry spells in the early stages of the monsoon season (Stern et al., 1982; Marteau et al., 2011). Excessively late planting, on the other hand, may shorten the growing season and reduce yield (Laux et al., 2008). In Burkina Faso, for example, a crop modeling study suggested that well-established rainfall-based methods of optimizing planting date result in late planting, and a reduction in yield of ~20% (Waongo et al., 2014). In general, the timing of season onset, is strongly correlated to the length of the rainy season and to total rainfall, both in West Africa and more widely (Sivakumar, 1988; Dunning et al., 2016). Therefore, sensitivity to planting date is expected to be greatest for lower rainfall years, when farmers are already highly vulnerable.
In semi-arid regions with strongly seasonal rainfall and relatively short growing seasons, the optimal planting date is broadly equivalent to the onset of the rainy season. Identification of the season onset may be considered equivalent to identification of optimal planting date. Onset may be identified in a number of ways. In Nigeria, for example, one study defined onset to have occurred when cumulative rainfall reached a spatially varying threshold (Benoit, 1977). Cumulative rainfall metrics may also distinguish between false and true rainy season onsets (Jolliffe and Sarria-dodd, 1994; Dodd and Jolliffe, 2001; Camberlin and Diop, 2003). Advanced statistical techniques (fuzzy logic) may improve the practical applicability of rainfall based criteria by ensuring that an onset date is estimated every year. Principal component analysis, moreover, allows criteria to be varied spatially (Laux et al., 2008). Practically speaking, there is clear value in being able to anticipate the rainy season, over relying on retrospective observations. Empirical forecasting models based on metrics of the African easterly jet or synoptic surface observations may provide useful information up to 2 months ahead of the onset of the rainy season in the Sahel (Omotosho, 1992; Omotosho et al., 2000).
The dependence of optimal planting date on rainfall variability has motivated the development of a number of decision support tools (DSTs), aiming to provide farmers with the climate information they require to make robust decisions on when to sow. Most of these are aimed at non-tropical regions. Reichenbach et al. (2003), for example, have developed a GIS-based crop modeling system that enables farmers in the US corn belt to relate historical climate data to growing seasons, and hence to analyze the risk of planting on a given date. Optimal planting advisories have also been shown in modeling studies to have the potential to improve crop yield in Africa. For example, integration of the aforementioned fuzzy logic algorithm (Laux et al., 2008) into the physically based crop model CropSyst (Stöckle et al., 2003) results in significant potential improvement in yield when implemented for groundnut and maize in West Africa (Laux et al., 2010).
Practical implementation of DSTs in the global south, however, presents significant challenges. In particular, the difficulty that farmers in developing countries face when accessing computer-based systems is widely recognized. An SMS-based interface to the DSSAT crop modeling system (Jones et al., 2003) has been developed for a region of the Philippines (Trogo et al., 2015). Churi et al. (2013) propose a similar technological framework but incorporating a wider range of climate and market data. The system was piloted in Tanzania, with broadly positive feedback from farmers. Both of the systems described above were developed using a participatory approach.
None of the operational systems described above advise whether farmers should or should not plant at a particular location and date. Instead, farmers are assisted in their decision making through access to multiple streams of information on biophysical and socio-economic factors. A major limitation of this approach is in assuming that farmers are able to appropriately interpret this information and translate it into action (i.e., to plant or wait). Often this is not the case, either because farmers distrust the information received or don't know how to interpret it for action. An alternative approach is to use expert judgement to collate available information into an advisory on when planting should occur. Regional and national hydrometeorological services, for instance, prepare bulletins on the onset of the rainy season, which can be used by farmers to plan planting. The example in Figure 1 is extracted from a bulletin issued by the Ghana Meteorological Agency based on seasonal forecasts.
Figure 1. Example NHMS plot of predicted rainfall season onset. The left plot maps the predicted start to the rainy season from March week 2 (MARWK2) to May week 1 (MAYWK1). The right plot displays the probability of early or late onset, compared to the climatological norm. The bulletin was issued on 9th March 2022 by the Ghana Meteorological Agency (https://www.meteo.gov.gh/gmet/download/10773/?wpdmdl=10773&refresh=62a74034638d11655128116, retrieved 13th June 2022).
In this study, we extend previous approaches to planting date decision support using a seamless system for forecasting soil moisture on both seasonal and sub-seasonal time scales. The paper is structured as follows: Section 2 details the data used and the methodology for identifying optimal planting date in an operational setting. Section 3 describes the evaluation of the system against historical data and during a series of operational pilots. Section 4 discusses and contextualizes the results.
For successful planting in regions affected by seasonal rainfall, there must be:
• Sufficient soil moisture in the upper layer of soil to support crop establishment.
• Sufficient time remaining in the rainy season to support crop development.
The general methodological approach adopted for the planting date decision support tool (DST) is to combine short term soil moisture forecasts with probabilistic assessment of local seasonal water resource satisfaction index (WRSI) (see Figure 2). The decision of when to plant is a trade-off between the risk of soil moisture deficit during the germination period, resulting in seedling death and the risk of planting too late and risking water stress at later times during the season. To reflect this trade-off the planting date DST incorporates two criteria:
Seasonal WRSI criterion: Probability that the WRSI exceeds a predefined fraction of the climatological maximum achievable WRSI is greater than a predefined value. The WRSI is calculated climatologically rather than predicted using the TAMSAT-ALERT approach because previous studies indicate that the predictability of interannual variability in WRSI on seasonal time scales is far lower than the short time scale predictability of the upper-level soil moisture (Brown et al., 2017; Boult et al., 2020). The WRSI probability criteria are fixed to 0.75 of maximum WRSI with a probability threshold of 0.5. In other words, planting may be recommended if there is a >50% probability that seasonal WRSI exceeds 75% of the climatological maximum value for the particular location/season.
Short-term upper level soil moisture criterion: Probability of the predicted upper level (top 10 cm) soil moisture exceeding a predefined threshold over a two-week period is greater than a predefined value. In this study, upper level is the top level in the soil moisture model and soil moisture is expressed as percent field capacity (PFC1). Following previous pilots of the planting date DST, the probability criteria for the soil moisture is set to 0.8.
These criteria are balanced to ensure that during the early stages of the calendar planting window, the primary decision-making criteria is upper level soil moisture, with the WRSI criteria defining the latest possible day that planting may be recommended. This is especially important during dry years, when the PFC criteria may not be met. In these cases, if the end of the WRSI window is approaching and the soil moisture criteria have not been met, farmers are advised to plant as soon as they can, bearing in mind that there is a higher than usual risk of germination failure.
The soil moisture forecasting model adopts the established TAMSAT-ALERT2 ensemble forecasting framework (Brown et al., 2017; Asfaw et al., 2018; Boult et al., 2020). TAMSAT-ALERT is a computationally light-weight system that accounts for the local historical climatology and recent variation in weather (rainfall, temperature, shortwave and longwave radiation, humidity, wind speed and pressure), local variation in soil texture and optionally the precipitation forecast.
In order to accommodate the possibility of two rainy seasons, the DST is constrained to run within predetermined large time windows for each region and season:
• Kenya and Uganda long rains: December – May.
• Malawi and Zambia rainy seasons: September – April.
• Rwanda A: August – February.
• Rwanda B: February – May.
Further details of the growing seasons can be found in Supplementary Table 2.
The soil moisture modeling approach adopted in this study is based on that used in the Joint UK Land Environment Simulator (JULES), which is based on the Met Office Surface Exchange Scheme (MOSES). The JULES/MOSES methodology is fully described in Cox et al. (1999) Best et al. (2011), and Clark et al. (2011). In order to speed up computation, and hence to allow the system to be implemented in Python over large regions, several adaptations have been made to the JULES method. Unlike JULES, the model does not include full photosynthesis or radiation schemes, resulting in differences to the way that potential evapotranspiration and stomatal conductance are derived (Boult et al., 2020).
As a result of the modifications listed above, the model requires different driving data to JULES. Specifically, rather than long and shortwave radiative fluxes, land-surface (skin) temperature is prescribed. The meteorological driving data required to run the model at the daily scale are thus: 2 m daily mean air temperature, 2 m maximum air temperature, 2 m minimum air temperature, skin temperature, surface pressure, 10 m wind speed, 2 m surface humidity and precipitation. The model is run at an hourly time step, using the JULES methods for disaggregating the daily driving data to the hourly scale.
In the model, vegetation properties are prescribed in a similar way to JULES, except that there is no capability to tile vegetation or to vary plant function types over a grid. For the purposes of this study, a maize crop was assumed to be growing for the WRSI modeling and bare soil was assumed for the soil moisture forecasts. The treatment of crops is described in the Section 2.2.3.
The model is driven with TAMSAT v3.1 rainfall data and the NCEP reanalysis (Kalnay et al., 1996; Maidment et al., 2017). The following data were used from the NCEP reanalysis: 10 m wind speed, daily 2 m minimum, maximum and mean temperature, specific humidity, sea-level pressure and land surface temperature (“skin temperature” in the NCEP dataset). All data were provided at the daily time scale and were re-gridded to a common 0.25° resolution. Both the TAMSAT and the NCEP data are complete and the only pre-processing carried out was the re-gridding of the datasets. The driving data are routinely processed within the TAMSAT system, and are publicly available from: http://gws-access.jasmin.ac.uk/public/tamsat/tamsat_alert_forcing_data/subset/Africa/0.25/yearly_files/.
Water resource satisfaction requirement index (WRSI) was calculated for maize, based on FAO parameters for growing degree days (Allen et al., 1998), and based on the ratio of actual to potential evapotranspiration inferred from the land-surface modeling results. Note that although maize is considered in this study, WRSI could be easily calculated for other crops. It would also be simple to adjust the WRSI parameters for different varieties of maize (for example a fast growing 90-day variety). This means that the decision support framework could easily be applied to other crops.
The soil moisture model used in this study was adapted for crops by allowing the leaf area index (LAI), plant height (h) and rooting depth (rd) to vary in both space and time. The variable LAI, h and rd are based on a growing degree day (GDD) model, for which the plant development stage and hence the LAI, h and rd depend on prescribed crop-specific GDD. Within a region, these stages will be reached on different dates, depending on the temperature and planting date.
The WRSI is defined as the seasonal mean percentage crop water requirement, calculated from planting to harvest, with the harvest date based on the GDD to maturity. The full method for calculating WRSI, and its relationship to soil moisture is described in Asfaw (2020) (with the description included as an appendix to Boult et al. (2020).
In this study, the TAMSAT-ALERT forecasting method described in Asfaw et al. (2018) and Boult et al. (2020) is used to produce spatially variable probabilistic forecasts of soil moisture, which are then integrated into the DST. This method is summarized as follows:
The TAMSAT-ALERT system aggregates meteorological metrics (such as precipitation) over user defined periods, which can include both the past and future. Land surface metrics, such as soil moisture, and agricultural metrics, such as crop yield, can be derived by driving impact/land surface models with the aggregated meteorological time series. Time series of meteorological variables are generated by splicing together historical data (satellite-based observations or reanalysis) with an ensemble of possible weather futures. The future weather ensemble is the weather that has occurred at the locality in question in the past.
In effect, TAMSAT-ALERT is an ensemble forecasting system, with ensemble members based on possible weather futures, derived from the climatology. It provides a quantitative answer to the question: Given the climatology, the stage of the growing season, the state of the land-surface, the weather so far in the season of interest, and the meteorological forecast, what is the likelihood of some adverse event?
Predictions of seasonal metrics are derived by statistically analyzing the ensemble. TAMSAT-ALERT can thus be used to monitor and predict any metric that can be derived from environmental time series data. It should be noted that TAMSAT-ALERT can be run without recourse to any proprietary data. In addition, TAMSAT-ALERT has the capability to incorporate the seasonal tercile rainfall forecast to weight the ensemble members to add additional forecasting ability (Asfaw et al., 2018; Boult et al., 2020).
TAMSAT-ALERT is a general framework that can incorporate any impact or land-surface model, which is driven with meteorological data. In this application, TAMSAT-ALERT will be run using the soil moisture modeling approach described in the previous sections.
Key to the success of the DST is identification of the optimal day for planting. The overarching aim is to enable farmers to plant as close as possible to the true optimal day, whilst respecting local planting practices and appetite for risk.
The DST was validated and calibrated empirically–with the aim of determining how close to the optimal planting date the recommendations were. To this end, we utilize a large agronomical dataset provided by One Acre Fund. These data include geo-located yield and planting date observations at the individual farm level for the seasons listed in Supplementary Table 1 and for the locations displayed in Figure 3. A basic quality control was undertaken to check for repeated values, missing values and infeasible locations and planting dates. The data were generally found to be of good quality.
Figure 3. Climatological water requirement satisfaction index (WRSI) calculated for variable planting dates throughout Africa. On the figure, WRSI is calculated for the planting date that results in the highest climatological WRSI (averaged over 15 years). The red circles indicate localities for which yield and planting date observations were supplied.
During the calibration and validation process, the yield achieved by farmers was compared with the difference between the recommended and observed planting dates. The yield data were gathered into bins, with each bin representing a 10-day relative difference compared to the recommended date. Yield was expressed as the median of the binned yield values. Negative differences indicate that planting occurred earlier than would have been recommended, and positive values indicate that planting occurred later than recommended. Values near zero indicate “compliant” farmers (i.e. those who planted close to the recommended date).
During the calibration, the procedure outlined above was carried out for a range of parameters, in order to determine the parameter set that resulted in recommendations closest to the true optimal planting date. The DST aims to support farmer decision making, rather than to promote radical change in behavior. As well as considering yield improvement, the calibration process therefore also took into account historical farmer behavior, in order to provide recommendations that broadly match historical local planting windows.
For soil moisture, the predefined threshold was determined through a sensitivity study, in which the PFC criteria were varied - resulting in revised estimates of optimal planting date. The number of farmers planting near each candidate optimal planting date, and the yields they achieved were compared for each country/season. In order to be consistent with established farmer practices, the choice of PFC criteria was based primarily on the number of farmers planting, with yield being a secondary criterion. Defining the threshold this way, in principle, allows the DST to be applied in cases where detailed datasets of planting date and yield are unavailable, so long as there is local knowledge on approximate historical planting dates. Figures 4, 5 show the sensitivity study results for candidate PFC criteria of 25, 50, 70, and 95% for 15-day accumulations of soil moisture (analogous analyses were carried out for 5- and 10 day accumulations). Figure 4 shows that for Kenya, Uganda, Rwanda and Zambia, a PFC of 70% results in advice that is broadly consistent with current practice – i.e., most farmers planted around the time considered optimal by the DST. Figure 5 shows that farmers who planted around the optimal time identified by the DST had higher yield than those who planted too late (see later sections for further discussion of the yield comparisons). For Malawi, in contrast, setting a criteria of PFC 70% would result in advising farmers to plant considerably later than they have done historically. Greater agreement with historical planting dates was achieved using a PFC threshold of 25%.
Figure 4. Number of farmers plotted against the difference between the actual and recommended planting time for all countries and seasons for PFC threshold criterion ranging from 25 to 95%.
Figure 5. Yield plotted against the difference between the actual and recommended planting time for all countries and seasons for PFC threshold criterion ranging from 25 to 95%.
In summary, based on these results, for Kenya, Rwanda, Uganda and Zambia, the criteria were set as follows:
• Climatological probability of a WRSI exceeding 0.75 of the maximum achievable WRSI is >0.5.
• Probability of the 15-day soil moisture exceeding 70% PFC is >0.8.
For Malawi, the criteria were set to:
• Climatological probability of a WRSI exceeding 0.4 of the maximum achievable WRSI is >0.5.
• Probability of the 15-day soil moisture exceeding 25% PFC is >0.8.
The fact that it was necessary to vary the decision support criteria is not surprising considering the variability in the climate and the fact that many farmers cultivate crops in sub-optimal conditions. Furthermore, experimental work suggests that the moisture sensitivity of root growth during the early growing season varies according to temperature, with there being more benefit to high soil moisture when temperatures are highest (Cutforth et al., 1986).
Hindcasts of upper level soil moisture were generated for all possible planting dates and for all years/seasons for which data were available (see Supplementary Table 1). These ensemble forecast were combined with the climatological WRSI forecasts, enabling the DST to be run retrospectively (i.e. in hindcast mode). The validation was carried out using the yield/planting date comparison procedure described above for individual seasons and countries. The system was judged to be working well if higher yield was achieved by farmers planting near the date that would have been recommended. The statistical significance was tested using Moody's test for the difference between medians. Because the data are noisy, the plots only include bins for which at least 25 values are available. The tables included in Supplementary Information, however, show all bins, including those with few values.
The results collated for all countries and years are displayed in Figures 6, 7. To allow for regional and interannual variation in yield, the yield is expressed relative to the yield achieved by compliant farmers for each season and country. It can be seen that the farmers in general fare better when they plant close to the recommended date. Farmers who plant earlier than recommended have slightly reduced yields, and those planting later do markedly worse. Further detail of the results is shown in Table 1, which lists median yield for each bin, along with the number of farmers, the standard deviation in yield, the level of statistical significance (P-value derived using Moody's test for the difference between medians). Note that although only bins with more than 25 data points are included in the yield/compliance plots, all data are shown in the table. In a separate analysis we controlled for other factors that might cause yield differences, and found there were no statistically significant differences in variety selection, fertilizer rate, weed management and compost use between compliant farmers and those who planted later.
Figure 6. Yield plotted against the difference between the actual and recommended planting time for all countries and seasons. Yields in all bins were significantly different to the −5 to 5 day bin.
Figure 7. Box and whiskers plot for yield difference between the median for compliant farmers (in a given season and country) and the yield achieved. The categories are: early (>10 days before recommendation); compliant (within 10 days of recommendation) and late (>10 days after the recommendation).
Table 1. Comparison between yield achieved and the difference between the observed and recommended planting dates.
Although at an aggregated level farmers on average achieve higher yield when they plant close to the optimal time, there is a lot of variability. Not all farmers who plant close to the recommended time do better than those who plant early or late. This is illustrated by box and whisker plots shown in Figure 7, which demonstrates that there is a wide range of outcomes for farmers, regardless of whether they plant early, are compliant or plant late.
A critical question for the implementation of the DST is whether it can be applied to a range of geographical, agricultural and meteorological settings. To address this, the data were analyzed for individual years and countries. The results are summarized in Figure 8 (with further plots included as Supplementary Information). As was described in the previous sections, the DST criteria were the same for all countries, except for Malawi, where farmers plant in the very earliest stages of the growing season (Supplementary Figure 10). For all the countries, planting at the optimal time recommended by the DST has benefit for yield, with significantly lower yield achieved in the late planting groups, compared to the compliant group. The results for the early planters are more mixed, reflecting the high risk/reward ratio conferred by early planting. This is more evident when the data are disaggregated by year shown. In Kenya during 2017, for example, a subset of farmers planted significantly before the recommended date and achieved a high yield. It is clear from the rainfall time series, however, that in other years farmers who planted at that time would have experienced a severe dry spell (Supplementary Figure 1). Analogously, early planters achieved high yields in Uganda during 2018, but in other years, these planters were affected by severe dry spells (Supplementary Figure 7).
Figure 8. Yield plotted against the difference between the actual and recommended planting time for individual countries–data collated from all seasons for which we have data. Red dots denote yields that are significantly different to yields within the −5 to 5 day bin.
The situation in Rwanda is different from other countries because the rainy season is divided into two growing seasons: season A and season B. Season A (August-January) is the primary growing season and season B (February-June) is a secondary, but important, season. In a sense, the planting date decision support tool has little role to play in management of season B because farmers simply plant as soon as they have harvested their season A crops (Supplementary Figure 5). However, for Season A, it is crucial that farmers plant as early as possible to benefit from a relatively short cropping period, and very few farmers plant before the recommended date (Supplementary Table 4).
Gridded soil moisture forecasts for a range of applications are run by the TAMSAT group on the Jasmin high performance computational platform (https://gws-access.jasmin.ac.uk/public/tamsat/tamsat_alert/). The forecasting model is run for all Africa, and data for farm locations is extracted by One Acre Fund. The recommendations are then forwarded by SMS to individual farmers. The driving data for the model are produced daily but in practice, running the system twice weekly has proved sufficient for operational DST applications. The DST has been piloted for One Acre Fund clients since 2019:
2019–2020: DST was run for a limited number of communities in Kenya and Zambia using an earlier version of the soil moisture forecasting technology. All advisories were produced by the TAMSAT group. The pilot sparked demand from the wider One Acre Fund client base.
2020–2021: An API was developed by One Acre Fund, enabling the DST to be run in country. The DST was run for >300k farmers in Kenya and Zambia. A trial was undertaken to test whether sending farmers an SMS prompting them to plant at the optimal time increases their likelihood of planting at the optimal time. Results from the trial suggest a potential for using SMS campaigns to influence farmers' planting dates, especially for farmers who are likely to plant after the optimal time (late planters). Specifically, 'treated' farmers were 20% more likely to plant within the optimal window and on average planted 4 days closer to the optimal date.
2021–2022: Following the 2020–2021 pilot, the service was extended to Malawi, Rwanda and Uganda with minor revisions to the calibrations and the API. The soil moisture forecasts were supplemented with additional precipitation forecast outputs (which are not formally incorporated to the API, but which are used for further interpretation by One Acre Fund).
It is notable that the DST has so far only been implemented by One Acre Fund. At early stages of development, it has made sense to focus on a single organization, to allow for testing and refinement, and to avoid the risk of users losing confidence in the system. There is, however, strong demand for this type of service (for example, Singh et al., 2018), and no technological reason why the DST cannot be scaled out more widely. The soil moisture forecasts are routinely generated for all of Africa and our intention is to place the forecasts on a public server, together with a full set of hindcasts from 2016-present, to facilitate calibration. However, although it would be technically possible to develop a subscription system that enables farmers to access the advisories directly, we are cautious about this approach. It is crucial that DST does not undermine existing farmer support systems, provided by governmental and non-governmental organizations. With this in mind, our current intention is to focus on partnering with governmental and non-governmental farmer support organizations, building their capacity to utilize the DST to enhance the services they provide.
This study has presented a new DST, aimed at supporting farmer decision making on planting date. The DST was developed in response to demand from One Acre Fund clients, who are primarily smallholder farmers in southern and eastern Africa. African smallholders are amongst the most vulnerable populations in the world to climate variability and change (for example Harvey et al., 2014). Enabling such farmers to adapt to a changing climate is thus key to improving food security in Africa. As was described in the introduction, adjustment of planting date is a key adaptation strategy to climate variability and change. This is, in part, because changes to growing season timings are projected over the next century under all emissions scenarios. Indeed, changes in seasonality of rainfall are already observed globally (Dunning et al., 2018; Wainwright et al., 2021). The capacity to cope with variability in the timings of growing seasons is thus key to building resilience to climate change. Unlike some existing systems, which use historical data to provide information on long term climate-related risk (for example Reichenbach et al., 2003), the TAMSAT-ALERT DST allows for year-to-year variation in rainy season onset. It blends the real time monitoring and forecasting approach taken for meteorological monitoring and forecasts with the risk assessments that underpin agricultural decision support.
A second feature that differentiates the TAMSAT-ALERT DST from other systems is the use of soil moisture, rather than rainfall. Soil moisture in the upper soil layers depends both on antecedent soil moisture conditions (how wet the soil is at the outset) and the flux of moisture in and out of the soil via precipitation and evapotranspiration (for example, Seneviratne et al., 2010). In other words, wet soils are less likely to dry down to critical levels during dry spells. Accounting for antecedent soil moisture thus enables a more accurate assessment of the risk of critically dry conditions in the days after planting.
Finally, previous work has demonstrated the necessity of carefully considering the delivery and context of decision support in the global south (for example, Trogo et al., 2015). Smallholders in Africa have limited access to smartphones and computers. Limited literacy in some socio-economic groups also affects capacity to interpret multiple streams of data provided within bulletins and by more sophisticated DSTs. The use of simple SMS messages has been found by One Acre Fund to be an effective way to reach farmers, including those in marginalized socio-economic groups. Perhaps more importantly, however, the TAMSAT-ALERT planting date advisories are currently provided in the context of an established and trusted system of holistic farmer support. A future challenge will be how to scale out the system more widely so that advisories can benefit all farmers–not only those served by One Acre Fund.
In summary, this paper has presented a new DST that targets a critical weather-related agricultural decision: when to plant. In addition to developing and evaluating a framework for identifying optimal planting date, we have demonstrated the implementation of the DST within a well-established agricultural support programme. Ongoing work aims to improve the skill of the DST–for example by incorporating meteorological forecasts and remotely sensed soil moisture. Even more important than this technical work, however, is developing a system that improves the resilience of smallholder farmers to climate variability and change. In this context, skillful identification of optimal planting date is only the first step. Future work will focus on robust operational solutions that can be extended at low cost throughout the global south.
The data analyzed in this study is subject to the following licenses/restrictions: Planting date and yield data available on request from One Acre Fund. Requests to access these datasets should be directed to YWxleC5zYW5hbmthQG9uZWFjcmVmdW5kLm9yZw==.
EB led the writing of the paper and the validation analysis. DA led the development of the soil moisture model. AS developed the decision support system, jointly with SA and EB. VB and RM developed the operational system. All authors contributed to the article and approved the submitted version.
EB, RM, and VB were supported by the National Center for Atmospheric Science and the Global Challenges Research Fund, via Atmospheric hazard in developing Countries: Risk assessment and Early Warning (ACREW) (NE/R000034/1) and by the Natural Environmental Research Council and the Department for Foreign International Development through the SatWIN-ALERT, and DRiSL projects (NE/R014116/1 and NE/R014272/1). VB is also supported by a NERC Knowledge Exchange Fellowship (NE/V018841/1). DA acknowledges financial support from CIMMYT TAMASA project, the Royal Society (Grant CHL\R1\180485) and the European Union's Horizon2020 Program (DOWN2EARTH, Grant 869550).
AS and SA were employed by One Acre Fund.
The remaining authors declare that the research was conducted in the absence of any commercial or financial relationships that could be construed as a potential conflict of interest.
All claims expressed in this article are solely those of the authors and do not necessarily represent those of their affiliated organizations, or those of the publisher, the editors and the reviewers. Any product that may be evaluated in this article, or claim that may be made by its manufacturer, is not guaranteed or endorsed by the publisher.
The Supplementary Material for this article can be found online at: https://www.frontiersin.org/articles/10.3389/fclim.2022.993511/full#supplementary-material
1. ^
Where θ is the soil moisture, θfc is the soil moisture at field capacity, where field capacity is 80% of soil saturation.
2. ^Tropical Applications of Meteorology using SATellite-based data (TAMSAT) AgricuLtural Early waRning system (ALERT).
Allen, R. G., Pereira, L. S., Raes, D., and Smith, M. (1998). FAO irrigation and drainage paper No. 56. Rome Food Agric.e Org. United Nations 56, e156.
Asfaw, D., Black, E., Brown, M., Nicklin, K. J., Otu-Larbi, F., Pinnington, E., et al. (2018). TAMSAT-ALERT v1: a new framework for agricultural decision support. Geosci. Model Dev. 11, 2353–2371. doi: 10.5194/gmd-11-2353-2018
Asfaw, D. T. (2020). Combining multiple streams of environmental data into a decision support tool for maize based systems in Sub-Saharan Africa (Unpublished doctoral thesis). University of Reading, Reading, United Kingdom.
Bedeke, S. B. (2022). Climate change vulnerability and adaptation of crop producers in sub-Saharan Africa: a review on concepts, approaches and methods. Environ. Dev. Sustain. 1–35. doi: 10.1007/s10668-022-02118-8
Benoit, P. (1977). The start of the growing season in Northern Nigeria. Agric.Meteorol. 18, 91–99. doi: 10.1016/0002-1571(77)90042-5
Best, M. J., Pryor, M., Clark, D. B., Rooney, G. G., Essery, R., Ménard, C. B., et al. (2011). The Joint UK Land Environment Simulator (JULES), model description–Part 1: energy and water fluxes. Geosci. Model Dev. 4, 677–699. doi: 10.5194/gmd-4-677-2011
Boult, V. L., Asfaw, D. T., Young, M., Maidment, R., Mwangi, E., Ambani, M., et al. (2020). Evaluation and validation of TAMSAT-ALERT soil moisture and WRSI for use in drought anticipatory action. Meteorol. Appl. 27, e1959. doi: 10.1002/met.1959
Brown, M., Black, E., Asfaw, D., and Otu-Larbi, F. (2017). Monitoring drought in Ghana using TAMSAT-ALERT: a new decision support system. Weather 72, 201–205. doi: 10.1002/wea.3033
Camberlin, P., and Diop, M. (2003). Application of daily rainfall principal component analysis to the assessment of the rainy season characteristics in Senegal. Clim. Res. 23, 159–169. doi: 10.3354/cr023159
Churi, A. J., Mlozi, M. R., Mahoo, H., Tumbo, S. D., and Casmir, R. (2013). A decision support system for enhancing crop productivity of smallholder farmers in semi-arid agriculture. Int. J. Inf. 3.
Clark, D. B., Mercado, L. M., Sitch, S., Jones, C. D., Gedney, N., Best, M. J., et al. (2011). The Joint UK Land Environment Simulator (JULES), model description–Part 2: carbon fluxes and vegetation dynamics. Geosci. Model Dev. 4, 701–722. doi: 10.5194/gmd-4-701-2011
Cox, P. M., Betts, R. A., Bunton, C. B., Essery, R. L. H., Rowntree, P. R., and Smith, J. (1999). The impact of new land surface physics on the GCM simulation of climate and climate sensitivity. Clim. Dyn. 15, 183–203. doi: 10.1007/s003820050276
Cutforth, H. W., Shaykewich, C. F., and Cho, C. M. (1986). Effect of soil water and temperature on corn (Zea mays L.) root growth during emergence. Can. J. Soil Sci. 66, 51–58. doi: 10.4141/cjss86-006
Dharmarathna, W. R. S. S., Herath, S., and Weerakoon, S. B. (2014). Changing the planting date as a climate change adaptation strategy for rice production in Kurunegala district, Sri Lanka. Sustain. Sci. 9, 103–111. doi: 10.1007/s11625-012-0192-2
Dobor, L., Barcza, Z., Hlásny, T., Árendás, T., Spitkó, T., and Fodor, N. (2016). Crop planting date matters: Estimation methods and effect on future yields. Agric. For. Meteorol. 223, 103–115. doi: 10.1016/j.agrformet.2016.03.023
Dodd, D. E., and Jolliffe, I. T. (2001). Early detection of the start of the wet season in semiarid tropical climates of western Africa. Int. J. Climatol. 21, 1251–1262 doi: 10.1002/joc.640
Dunning, C.M., Black, E.C., and Allan, R.P. (2016). The onset and cessation of seasonal rainfall over Africa. J. Geophys. Res. Atmos. 121, 11–405. doi: 10.1002/2016JD025428
Dunning, C. M., Black, E., and Allan, R. P. (2018). Later wet seasons with more intense rainfall over Africa under future climate change. J. Clim. 31, 9719–9738. doi: 10.1175/JCLI-D-18-0102.1
Egli, D. B., and Bruening, W. (1992). Planting date and soybean yield: evaluation of environmental effects with a crop simulation model: SOYGRO. Agric. For. Meteorol. 62, 19–29. doi: 10.1016/0168-1923(92)90003-M
Egli, D. B., and Cornelius, P. L. (2009). A regional analysis of the response of soybean yield to planting date. Agron. J. 101, 330–335. doi: 10.2134/agronj2008.0148
Garcia, A. G., Guerra, L. C., and Hoogenboom, G. (2009). Impact of planting date and hybrid on early growth of sweet corn. Agron. J. 101, 193–200. doi: 10.2134/agronj2007.0393
Harvey, C. A., Rakotobe, Z. L., Rao, N.S., Dave, R., Razafimahatratra, H., Rabarijohn, R. H., et al. (2014). Extreme vulnerability of smallholder farmers to agricultural risks and climate change in Madagascar. Philos. Trans. R. Soc. B 369, 20130089. doi: 10.1098/rstb.2013.0089
Hersbach, H., Bell, B., Berrisford, P., Hirahara, S., Horányi, A., Muñoz-Sabater, J., et al. (2020). The ERA5 global reanalysis. Q. J. R. Meteorol. Soc. 146, 1999–2049. doi: 10.1002/qj.3803
Jolliffe, I. T., and Sarria-dodd, D. E. (1994). Early detection of the start of the wet season in tropical climates. Int. J. Climatol. 14, 71–76. doi: 10.1002/joc.3370140106
Jones, J. W., Hoogenboom, G., Porter, C. H., Boote, K. J., Batchelor, W. D., Hunt, L. A., et al. (2003). The DSSAT cropping system model. Eur. J. Agron. 18, 235–265. doi: 10.1016/S1161-0301(02)00107-7
Kalnay, E., Kanamitsu, M., Kistler, R., Collins, W., Deaven, D., Gandin, L., et al. (1996). The NCEP/NCAR 40-year reanalysis project. Bull. Am. Meteorol. Soc. 77, 437–472.
Kidane, R., Wanner, T., Nursey-Bray, M., Masud-All-Kamal, M., and Atampugre, G. (2022). The role of climatic and non-climatic factors in smallholder farmers' adaptation responses: insights from rural Ethiopia. Sustainability 14, 5715. doi: 10.3390/su14095715
Kombiok, J. M., and Clottey, V. A. (2003). Maize yield and soil N as affected by date of planting mucuna in maize-mucuna intercropping in Ghana. Trop. Agric. 80, 77–82.
Laux, P., Jäckel, G., Tingem, R. M., and Kunstmann, H. (2010). Impact of climate change on agricultural productivity under rainfed conditions in Cameroon—A method to improve attainable crop yields by planting date adaptations. Agric. For. Meteorol. 150, 1258–1271. doi: 10.1016/j.agrformet.2010.05.008
Laux, P., Kunstmann, H., and Bárdossy, A. (2008). Predicting the regional onset of the rainy season in West Africa. Int. J. Climatol. 28, 329–342. doi: 10.1002/joc.1542
Lee, C. D., Egli, D. B., and TeKrony, D. M. (2008). Soybean response to plant population at early and late planting dates in the Mid-South. Agron. J. 100, 971–976. doi: 10.2134/agronj2007.0210
López-Bellido, F. J., López-Bellido, R. J., Khalil, S. K., and López-Bellido, L. (2008). Effect of planting date on winter kabuli chickpea growth and yield under rainfed Mediterranean conditions. Agron. J. 100, 957–964. doi: 10.2134/agronj2007.0274
Maidment, R. I., Grimes, D., Black, E., Tarnavsky, E., Young, M., Greatrex, H., et al. (2017). A new, long-term daily satellite-based rainfall dataset for operational monitoring in Africa. Sci. data 4, 1–19. doi: 10.1038/sdata.2017.82
Marteau, R., Sultan, B., Moron, V., Alhassane, A., Baron, C., and Traoré, S. B. (2011). The onset of the rainy season and farmers' sowing strategy for pearl millet cultivation in Southwest Niger. Agric. For. Meteorol. 151, 1356–1369. doi: 10.1016/j.agrformet.2011.05.018
Naab, F. Z., Abubakari, Z., and Ahmed, A. (2019). The role of climate services in agricultural productivity in Ghana: the perspectives of farmers and institutions. Clim. Serv. 13, 24–32. doi: 10.1016/j.cliser.2019.01.007
Omotosho, J. B. (1992). Long-range prediction of the onset and end of the rainy season in the West African Sahel. Int. J. Climatol. 12, 369–382. doi: 10.1002/joc.3370120405
Omotosho, J. B., Balogun, A. A., and Ogunjobi, K. (2000). Predicting monthly and seasonal rainfall, onset and cessation of the rainy season in West Africa using only surface data. Int. J. Climatol. 20, 865–880. Available online at: https://doi.org/10.1002/1097-0088(20000630)20:8 <865::AID-JOC505>3.0.CO;2-R
Reichenbach, S. E., Waltman, W. J., Jindal, G., Nelson, L. A., Meyer, S. J., Peake, J. S., et al. (2003). The Planting Date Calculator: A Decision-Support Tool for Agriculture. Computer Science and Engineering Department, University of Nebraska—Lincoln: Lincoln, NE, USA.
Seneviratne, S. I., Corti, T., Davin, E. L., Hirschi, M., Jaeger, E. B., Lehner, I., et al. (2010). Investigating soil moisture–climate interactions in a changing climate: a review. Earth Sci. Rev. 99, 125–161. doi: 10.1016/j.earscirev.2010.02.004
Serdeczny, O., Adams, S., Baarsch, F., Coumou, D., Robinson, A., Hare, W., et al. (2017). Climate change impacts in Sub-Saharan Africa: from physical changes to their social repercussions. Reg. Environ. Change 17, 1585–1600. doi: 10.1007/s10113-015-0910-2
Shi, W., and Tao, F. (2014). Vulnerability of African maize yield to climate change and variability during 1961–2010. Food Secur. 6, 471–481. doi: 10.1007/s12571-014-0370-4
Singh, C., Daron, J., Bazaz, A., Ziervogel, G., Spear, D., Krishnaswamy, J., et al. (2018). The utility of weather and climate information for adaptation decision-making: current uses and future prospects in Africa and India. Clim. Dev. 10, 389–405. doi: 10.1080/17565529.2017.1318744
Sivakumar, M. V. K. (1988). Predicting rainy season potential from the onset of rains in Southern Sahelian and Sudanian climatic zones of West Africa. Agric. For. Meteorol. 42, 295–305. doi: 10.1016/0168-1923(88)90039-1
Stern, R. D., Dennett, M. D., and Dale, I. C. (1982). Analysing daily rainfall measurements to give agronomically useful results. I. Direct methods. Exp. Agric. 18, 223–236. doi: 10.1017/S001447970001379X
Stöckle, C. O., Donatelli, M., and Nelson, R. (2003). CropSyst, a cropping systems simulation model. Eur. J. Agron. 18, 289–307. doi: 10.1016/S1161-0301(02)00109-0
Trogo, R., Ebardaloza, J. B., Sabido, D. J., Bagtasa, G., Tongson, E., and Balderama, O. (2015), “SMS-based smarter agriculture decision support system for yellow corn farmers in Isabela,” in 2015 IEEE Canada International Humanitarian Technology Conference (IHTC2015). IEEE. 1–4.
Wainwright, C.M., Black, E., and Allan, R.P. (2021). Future changes in wet and dry season characteristics in CMIP5 and CMIP6 simulations. J. Hydrometeorol. 22, 2339–2357. doi: 10.1175/JHM-D-21-0017.1
Waongo, M., Laux, P., and Kunstmann, H. (2015). Adaptation to climate change: The impacts of optimized planting dates on attainable maize yields under rainfed conditions in Burkina Faso. Agric. For. Meteorol. 205, 23–39. doi: 10.1016/j.agrformet.2015.02.006
Keywords: Africa, climate, soil moisture, planting date, decision support tool, rainfall
Citation: Black E, Asfaw DT, Sananka A, Aston S, Boult VL and Maidment RI (2023) Application of TAMSAT-ALERT soil moisture forecasts for planting date decision support in Africa. Front. Clim. 4:993511. doi: 10.3389/fclim.2022.993511
Received: 13 July 2022; Accepted: 19 December 2022;
Published: 16 January 2023.
Edited by:
Silje Lund Sørland, Norwegian Research Institute (NORCE), NorwayReviewed by:
Byomkesh Talukder, York University, CanadaCopyright © 2023 Black, Asfaw, Sananka, Aston, Boult and Maidment. This is an open-access article distributed under the terms of the Creative Commons Attribution License (CC BY). The use, distribution or reproduction in other forums is permitted, provided the original author(s) and the copyright owner(s) are credited and that the original publication in this journal is cited, in accordance with accepted academic practice. No use, distribution or reproduction is permitted which does not comply with these terms.
*Correspondence: Emily Black, ZS5jLmwuYmxhY2tAcmVhZGluZy5hYy51aw==
Disclaimer: All claims expressed in this article are solely those of the authors and do not necessarily represent those of their affiliated organizations, or those of the publisher, the editors and the reviewers. Any product that may be evaluated in this article or claim that may be made by its manufacturer is not guaranteed or endorsed by the publisher.
Research integrity at Frontiers
Learn more about the work of our research integrity team to safeguard the quality of each article we publish.