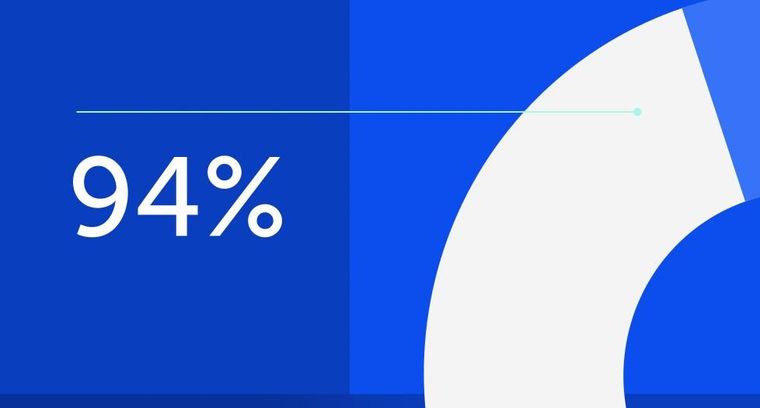
94% of researchers rate our articles as excellent or good
Learn more about the work of our research integrity team to safeguard the quality of each article we publish.
Find out more
ORIGINAL RESEARCH article
Front. Clim., 12 January 2023
Sec. Climate Mobility
Volume 4 - 2022 | https://doi.org/10.3389/fclim.2022.986088
Climate change and natural disasters might increase the vulnerability of populations in affected regions. This article investigates the link between climatic events and bilateral migration from countries in the Global South to OECD countries. A gravity model is estimated using panel data techniques and bearing in mind potential sources of heterogeneity across subsamples. The main novelty is to distinguish by climatic zones, while also considering commonly used social and economic stresses, such as income levels and incidence of conflict. The analysis is based on bilateral emigration flows from 76 developing countries to OECD countries in the last decades. The results show that increasing extreme temperatures and storms in arid and semi-arid zones act as triggers of bilateral migration from South to North and that the estimated effects are non-negligible. The results are robust to a battery of tests, including dividing the sample according to the levels of conflict and poverty.
In the twenty-first century, the sustained increase in temperature, as well as the increasing frequency of natural disasters, has become a pressing issue globally. One important area impacted by climate change is migration. The onset of extreme climatic events might increase the vulnerability of populations staying in affected regions while decreasing the availability of resources to migrate to more secure areas (Krishnamurthy, 2012; Abel et al., 2019; Borderon et al., 2019; Cattaneo et al., 2019; Wesselbaum and Aburn, 2019; Kaczan and Orgill-Meyer, 2020; Cottier et al., 2022; Piguet, 2022).
As the incidence of such natural disasters is set to increase in the upcoming years, it is of great importance to understand whether climate change by itself induces migration or rather only reinforces it by going hand in hand with increased conflict and poverty due to income losses (Homer-Dixon, 2001; Boas et al., 2022; Cottier et al., 2022). The latter highlights the observation that socioeconomic, cultural, and political factors are very important drivers of migration, and hence, climate change only acts in combination with other social, political, and economic stresses.
The main aim of this article is to exploit the heterogeneity of the effects of climatic events on international migration from the global South to the global North, distinguishing by climatic zone. The idea is that an increase in average temperature or average precipitation (slow-onset event) or the event of a drought or flood (fast-onset event) might impact differently on migration depending on whether the slow-onset or fast-onset event occurs in an arid or tropical wet (or any other) climate zone. To the best of our knowledge, such an analysis disaggregated by climatic zones has not been done in the previous literature. We also consider other potential heterogeneities of the effects depending on the poverty level and the prevalence of conflict, and therefore consider social, political, and economic contexts or stresses as well.
The previous literature has investigated the effect of climatic disasters on bilateral migrations mainly considering distinct effects by a group of income (Bennonier et al., 2019; Borderon et al., 2019), and by type of environmental events (Cattaneo et al., 2019; Wesselbaum and Aburn, 2019), covering economic stresses very broadly. As the majority of previous results were found to be highly context-dependent, it is important to further disentangle the effects according to region-specific characteristics, such as climatic zones, and according to social and political stresses, such as conflict and poverty. We contribute to the discussion by conducting such an analysis.
Our main results indicate that an increase in average temperatures is related to a rise in out-migration in the total sample and for most country groups, according to income. Having an earthquake in the previous year also goes hand in hand with more out-migration, but the same was not found for other extreme events, such as landslides, storms, fires, extreme temperatures, and floods. When it comes to the effects of climatic zones, droughts seem to reduce out-migration from arid, and semi-arid regions. In contrast, storms and wildfire spur emigration in all arid zones, whereas storms propel out-migration also in wet tropical zones. As for the role of social, political, and economic stresses, we provide evidence that a rise in extreme poverty diminishes out-migration in almost all countries. This effect is strong and is in accordance with the trapped-population arguments made in the related literature (Cattaneo and Peri, 2016; Cattaneo et al., 2019). We also find that increasing conflict together with climate change leads to rising out-migration. The type of conflict that is relevant for this outcome could be simple scarcity conflicts and/or relative deprivation conflicts (Homer-Dixon, 2001). These findings suggest that climatic events have highly context-dependent impacts on migration, and consequently addressing them requires location-based solutions.
The rest of the study is organized as follows. Section 2 revises the closely related literature, more specifically referring to studies that cover international migration flows. Section 3 presents a description of the data used in the analysis, as well as stylized facts and descriptive statistics. Section 4 discusses the model specification used as an empirical strategy while referring to the most common methods in the literature. Section 5 analyzes the estimation results, according to different subsamples and distinguishes them by climatic zones. Finally, Section 6 concludes and discusses implications for policy and future research.
The discussion on how climatic events impact migration has been ongoing for decades. Wolpert (1966), was one of the first authors to emphasize that changes in environmental stress should be accounted for when analyzing migration flows. Over the past few years, many studies with such a focus have followed, especially because of the ever-increasing attention drawn to climate change (Piguet, 2022). Some studies concentrate on internal migration (within national boundaries), whereas others focus on international migration (crossing national boundaries). Furthermore, some authors analyse the direct effects of climate change while others put the effects of climate change in a socioeconomic, social, and political context, emphasizing the indirect effects of climate change. A detailed overview of the main empirical migration studies that include climatic factors as determinants can be found in Supplementary Table A.1 in the Appendix.
Most studies considered applying some version of a gravity model, theoretically grounded on random utility maximization models characterizing agents' choices. These also control the effect of unobservable factors associated with destination countries' migration policies, origin country time-invariant characteristics, or bilateral time-invariant traits (Beine and Parsons, 2015; Cattaneo and Peri, 2016; Martínez-Zarzoso, 2020).
Recent studies have provided further insights on migration push and pull factors related to both the state and quality of institutions in sending countries, and the impact that climatic events seem to have (Hoffmann et al., 2020; Kaczan and Orgill-Meyer, 2020; Martínez-Zarzoso, 2020; Beine and Jeusette, 2021). In addition, several studies focused on asylum migration and irregular migration and its relation to climatic variations (Abel et al., 2019; Cottier and Salehyan, 2021; Schutte et al., 2021). The increasing availability of data and the development of more elaborate empirical strategies have helped to clarify previous findings on the role of different drivers of international migration, which depict heterogeneous, complex, and often context-dependent migration dynamics both within and across countries.
Contrasting with the use of more mainstream approaches of the panel data analysis, it must be noted that some interest has emerged in examining the dynamic behavior of migration as a response to slow- and fast-onset climatic factors (Cattaneo et al., 2019; Wesselbaum and Aburn, 2019). Providing further insights and considering a broader spectrum of variables and empirical strategies, Hoffmann et al. (2020), Beine and Jeusette (2021), and more recently Moore and Wesselbaum (2022) summarize the findings resulting from meta-analyses on migration. Their work on both internal and international migration considers regional heterogeneity1 and differentiates slow- and fast-onset phenomena. According to their reviews, there seems to be robust evidence of the impact of some climatic variables, such as temperature, but not for others, such as precipitation. Nonetheless, the reviews also stress that the importance of how variable measurement affects paper outcomes is not negligible, thus highlighting that finding an effect and its magnitude may greatly vary depending on the way a study builds its empirical strategy.
Examining a crucial part of any empirical strategy, the reviews conducted by Borderon et al. (2019), Cattaneo et al. (2019), and Kaczan and Orgill-Meyer (2020) allow us to explore the importance of variables other than those directly related to environmental factors that affect migration decisions locally and internationally. In addition, these studies highlight the role of the type of environmental events taking place (slow- or fast-onset), as well as the links to agricultural channels through which climatic variables may exert any influence (as well as in previous studies, such as Backhaus et al., 2015; Coniglio and Pesce, 2015). Borderon et al. (2019) note that evidence from African countries has also emphasized the relevance of environmental factors through their perceived effects, instead of their objective influence. This differentiation sheds some light on the role that individual-level psychological variables may play and their influence on other factors' effects.
Work on climate change-driven internal migration has not only pointed to the main factors motivating population displacement but also to the indirect effects that internal movements can eventually have on the international scale. Throughout several studies, it has been shown that environmentally induced migration is heavily context-dependent (Thiede et al., 2016; Borderon et al., 2019; Hoffmann et al., 2020) and often more strongly linked to short-term shocks.
Barrios et al. (2006) and Marchiori et al. (2012) set precedents in relating climatic factors' influence on migration, referring to internal and international movements, respectively. Focusing on Sub-Saharan countries, they acknowledge the effects that rainfall has on migration using several measures. Overall, rainfall level increases are associated with increased internal migration and its anomalous levels with increasing wage differences across the analyzed regions. Nonetheless, the magnitude and significance of the effects notably vary across countries.
The impact of climatic factors has also been found to depend on the local importance of agriculture given that it tends to be a vital source of income in developing countries' rural areas. Should a natural disaster severely affect agricultural incomes, it is unclear whether it would stimulate or stifle migration, since the resulting liquidity constraints could trap populations making migration less likely (Cattaneo and Peri, 2016; Bennonier et al., 2019; Borderon et al., 2019).
Koubi et al. (2016) examine slow- and fast-onset events' influence on the migration decisions of the Vietnamese with the help of provincial scale survey-based logit models. Their main findings indicate that although short-term disasters do increase the likelihood of migration, long-term environmental effects have a limited, often weakly significant effect. Moving continents and broadening the scope, Thiede et al. (2016) report similar findings for eight South American countries (focusing on rural–urban migration), highlighting the outcome heterogeneity once variables such as gender, education, and age are controlled for. Not surprisingly, the effects of climatic variables vary both in magnitude and significance when each country's geographical conditions are considered. For instance, while increased rainfall may lead to flooding in some countries and become a push factor, in others it may contribute to greater agricultural yields and discourage internal migration.
Since internal migration may have an influence on international migration, the drivers of the former have also been linked to the latter (Maurel and Tuccio, 2016; Bennonier et al., 2019; Hoffmann et al., 2020; Beine and Jeusette, 2021). A usual channel to depict climate variables' indirect effect on international migration explores the effect of rainfall and temperature on rural–urban migration, which in turn affects urban wages. The subsequent change in international wage gaps increases the likelihood and magnitude of international migration flows. In fact, Maurel and Tuccio (2016) exploit an instrumental variables strategy using decadal data for 222 countries, finding that the variation of temperature and rainfall and standard deviations of rainfall are associated with greater rural–urban migration, which in turn sparks greater international migration. Their findings fall in line with the assessment of Beine and Jeusette (2021), which highlights that the local effects of migration on urban wages drive outward international migration as wage gaps across country dyads grow. Contrasting findings have also indicated that if fast-onset shocks curtail a population's liquidity constraint, it may be the case that such constraint hinders internal migration significantly—a trapped population case—as stated by Bennonier et al. (2019) and Hoffmann et al. (2020). Nonetheless, it must be acknowledged that a common trait of these studies is the widespread use of the urbanization rate as a proxy for internal migration. Even if data availability is limited and encourages using this measure, it is likely to miss other population movements within countries, such as rural–rural, urban–urban, and urban–rural, thus underscoring any effects found, which may also relate to international migrant flows.
One way to assort these studies may follow their way of measuring migration. While some authors choose to use samples with yearly frequencies (Marchiori et al., 2012; Backhaus et al., 2015; Cai et al., 2016; Wesselbaum and Aburn, 2019; Martínez-Zarzoso, 2020; among others), others use decadal or 5-year periods (for example, Beine and Parsons, 2015; Cattaneo and Peri, 2016; Maurel and Tuccio, 2016; Bennonier et al., 2019), depending on whether their main interest lies in examining short- or long-term effects. In any case, according to recent surveys (Berlemann and Steinhardt, 2017; Borderon et al., 2019; Cattaneo et al., 2019; Beine and Jeusette, 2021), most studies focus on international migration, rather than internal migration.
An important distinction found among the revised studies concerns the way in which climatic-related events are measured. Hence, in what follows we classify studies examining climate change-induced migration into three categories, according to the main variables of interest. The first group of papers employs average temperature and rainfall levels (Barrios et al., 2006; Backhaus et al., 2015; Beine and Parsons, 2015; Coniglio and Pesce, 2015; Cai et al., 2016; Cattaneo and Peri, 2016; Thiede et al., 2016; Bennonier et al., 2019; Falco et al., 2019; Martínez-Zarzoso, 2020; Owen and Wesselbaum, 2020). The second group uses temperature and rainfall variability and anomalies (Marchiori et al., 2012; Maurel and Tuccio, 2016; Beine and Parsons, 2017; Ayansina et al., 2018; Cottier and Salehyan, 2021). Finally, a third cluster chooses to explore a set of natural disasters (Koubi et al., 2016; Berlemann and Steinhardt, 2017; Gröschl and Steinwachs, 2017; Abel et al., 2019; Wesselbaum and Aburn, 2019). Depending on the variables of interest, these studies choose to evidence the potential effects of slow- and fast-onset climatic factors on migration, which is linked to the periodicity of migration data used (5-year or decadal averages, contrasting with yearly observations).
Complementing the latter, it must be noted that the extensive use of fixed effects to control for destination (origin) country's factors that might affect migration outcome, and bilateral time-invariant factors within gravity models also mark a significant advance in empirical strategies that enable them to account for unobserved heterogeneity in some dimensions and help to identify causal effects. For instance, Beine and Parsons (2015) find no evidence of direct impacts of climate anomalies on international migration, while Cattaneo and Peri (2016) do find a positive effect for middle-income countries and a negative effect for low-income countries. Beine and Parsons (2017), in an extension of their previous work, find effects consistent with those of Cattaneo and Peri (2016), once a larger set of controls is used.
While research by economists concentrates more on the direct effects of climate change emphasizing the economic context (role of agriculture, rural–urban divide, income groups, and regions) research on climate-induced migration by political scientists focuses more on the socioeconomic and political context, and hence the indirect effect of climate change. Homer-Dixon (2001), Black et al. (2011), and Boas et al. (2019, 2022) highlight the indirect effects of climate change claiming that environmental stress by itself does not cause out-migration; it does not even cause violence and conflict but can do so if economic institutions fail and governance is weak. They conclude that the climate-induced effect of climate change on out-migration is therefore indirect.
Our study focuses on international migration (also because internal migration is hard to measure) and attempts to analyze out-migration as triggered by climate change itself and the impact of climate change in different contexts. We elaborate on the direct impact of environmental stress by looking at all sorts of climatic events (slow- and fast-onset) in different climate zones, as well as evaluating its role in the context of conflicts, poverty, and economic vulnerability.
The dataset consists of 76 countries of origin and 36 OECD-members destination countries, covering the years from 1990 to 2017, which results in 28 time periods for the panel data analysis. The dependent variable is bilateral out-migration2 from countries in the Global South to OECD destination countries, obtained from the OECD's International Migration Database (2022). It is worth noting that some of the analyses conducted consider OECD members also as origin countries (our initial sample covered 204 origin countries), as they include important sending and receiving countries, such as Mexico and Chile. The dependent variables are used in per capita terms according to the origin country's population, as depicted by the model specifications3 (see Section 4). A caveat from the OECD's IMD data is that migration inflows are based on the reported amounts of residence permits granted by the state members in a given period. As each state member might have different criteria to register migrants and to grant residence permits, there could be limitations to the data comparability among destination countries. In addition, illegal migration flows are unaccounted for by definition, which could potentially result in a slight bias in the estimations, especially if climatic factors do motivate illegal migration flows.
The main climatic variables used are annual average temperature and precipitation in the origin countries obtained from the The World Bank Group (2021).4 It is important to remark that the yearly changes in average temperatures appear to have a small variation, with 93.3% of the temperature changes ranging between −1 and 1°C in the overall sample. The same cannot be said for yearly changes in precipitation averages where 58.1% of the observed changes are within the range from −10 to 10 mm, which represents relevant yearly changes in rainfall. Nevertheless, the within-country variation in the sample is generally larger and could impact migration decisions as expected. The remaining climatic variables capture the occurrence of natural disasters or extreme weather events: droughts, earthquakes, fires, floods, landslides, storms, and extreme temperatures, and were obtained from the EM-DAT's (2022).5 The main criteria to select the list of disasters considered was based on their potential relationship with migration. We recognize that droughts, unlike other disasters, are a very complex and gradual phenomenon, and hence their inclusion in the model has to be taken with care.
We assess potential heterogeneous effects according to the predominant climatic zones,6 countries' levels of income, poverty, and conflict. We constructed sets of dummies that describe the types of the climatic zone in the countries of origin to model heterogeneous effects. The variables are based on geospatial data by the Köppen–Geiger global 1-km climate classification maps (Köppen–Geiger, 2021) and take the value of one for each climatic zone present in a country, at any level. We focus on six of the most common climatic zones observed in developing countries: semi-arid, arid, any arid (semi-arid or arid), tropical wet, tropical dry, and any tropical (wet or dry). A list of countries according to climatic zones and a map of the distribution of climatic zones at the global level are provided in Supplementary Table A.2 and Supplementary Figure A.1 in the Appendix. To distinguish the impacts across different levels of income, we use the definitions of least developed countries by the UNCTAD, of lower- and upper-middle-income countries by the World Bank, and whether countries belong to the OECD, according to membership information. To assess the effects across different levels of poverty, we constructed a “higher poverty level” variable, a dummy that takes the value of one for countries with a headcount poverty ratio (based on 1.90 USD per day poverty line) above 10%. The variable is based on data obtained from The World Bank's (2022). Regarding the effects across different levels of conflict, the “conflict” variable was created to capture countries of origin with high conflict intensity. The dummy takes the value of one for countries that belong to the highest quartile of the distribution of the four conflict indicators presented by The International Country Risk Guide (2019), that is, external and internal conflicts, ethnic tensions, and government instability.7 Table 1 presents the summary statistics for the variables used in the econometric analysis and Supplementary Table A.3 in the Appendix contains a complete description of the variables.
Our main theoretical expectations are that slow-onset climatic events, such as changes in average temperature and precipitation, should have a long-term effect on international migration. Differently, fast-onset climatic events could have a more immediate effect on displacements, especially when the corresponding phenomena are destroying the households' assets.
Concerning our prior expectations regarding the conditional effects of climatic zones, we hypothesize that the increasing frequency of climatic disasters in arid and semi-arid climatic zones could foster international migration more than in tropical climates. Whereas, the former zones are characterized by annual precipitation levels below a threshold that is close to the potential evapotranspiration, the latter is characterized by constant high temperatures and in general high annual precipitation.
To assess the impact of extreme climatic events on migration flows, we use a gravity model as an estimation technique. Gravity models rely on the idea that bilateral flows can be predicted by the size of the countries and the distance between them. The larger the size (distance), the stronger (weaker) the recipient's attractiveness to bilateral flows. Such models were originally conceptualized to identify the determinants of bilateral trade flows, but their application has been expanded ever since to a multitude of international flows, including cross-border migration (Isard, 1954; Isard and Reiner, 1966; Mayda, 2010). As described in the literature review, gravity models have been previously applied more specifically to analyze the impact of climatic variables on international migration.
Our proposed main specification builds on the approach used by Beine and Parsons (2015, 2017), Coniglio and Pesce (2015), and Gröschl and Steinwachs (2017) who use a gravity model to assess the impact of climatic variables on international migration. Similar to these studies, we apply a Poisson Pseudo-Maximum Likelihood (PPML) method to estimate the gravity model specification. One key advantage of the PPML when compared with the usual ordinary least squares (OLS) estimation methods is that it allows for the use of non-logarithmic gravity specifications, and consequently, zero values of the dependent variable can be included in the analysis. In our setting, not restricting the data to only positive values of bilateral migration avoids selection bias issues derived from accounting for only positive migration inflows. In addition, using the dependent variable in levels in the PPML model also prevents potential biases concerning Jensen's Inequality, which might hinder the interpretability of the results when the expected value of the logarithm is not equal to the logarithm of the expected value, as described by Mnasri and Nechi (2021).
However, our model specification differs from the previous studies in several ways. First, our dependent variable consists of yearly migration rates to destination countries, instead of decennial bilateral migration rates as in Gröschl and Steinwachs (2017). Second, our independent variables include an extensive set of extreme climatic events, not only rainfall and temperature as in Coniglio and Pesce (2015). Third, we limit our analysis to OECD destination countries, different from Beine and Parsons (2015, 2017). The reason for this choice is that OECD countries receive a very important part of international migration flows and recording errors are low, hence, not accounting for the remaining destinations prevents us from including unnecessary noise in the analysis. Fourth, in addition to analyzing the overall effect of climate change on migration, we explore potential heterogeneous effects according to differences in the predominant climatic zones, country-of-origin income levels, poverty incidence, and conflict presence. In particular, presenting the first study with a disaggregated analysis according to the country's climatic zones is especially important; as climate change might have different consequences depending on the region's climatic zone (Chelli et al., 2017). Finally, we use an updated dataset that covers the most recent data available on extreme climatic events. The main model specification is given by equation (1):
where the dependent variable Migrationijt consists of bilateral flows calculated as the migration flow from i to j at year t divided by the domestic population in country i, and it is explained by nine climatic variables lagged in one period (t−1). Different from other studies in the literature, we use 1-year-lagged independent variables, as we argue that the decision to migrate might be motivated by past climatic events and not come to fruition on short notice due to the time required to plan an international move. At the same time, we expect that the decision to migrate is not motivated by climatic events in a more distant past, as the urgency to move might subside over time. To assess the plausibility of these arguments, we conducted a series of preliminary lag analyses by including up to five lags of each climatic variable in the regressions.8 The results indicated that in most cases only the first-time lag (t−1) was consistently significant, apart from rare exceptions where the second or even third lags were significant, albeit at lower levels of confidence. Therefore, it seems reasonable to assume that the decision to migrate is motivated by past events but not by too distant ones.
The estimation is conducted in a panel data framework where we address unobserved heterogeneity concerns by including destination-year γjt and dyadic δij fixed effects. The destination-year γjt capture, for example, the climate factors at the destination but go much beyond as they also include other time-varying variables at the destination, such as the unemployment rate, the welfare system, border controls, and changes in immigration laws. Dyadic δij fixed effects cover things, such as distance, common border, common culture, common language, and the existence of a diaspora. We also control for heteroskedasticity and autocorrelation by using robust standard errors in the regressions that are clustered at the origin-destination level. Finally, the stochastic error term is represented by ϵijt.
It is worth noting that gravity models usually contain variables that denote the size of the countries (e.g., income and population) and the distance between them. Even though one might want to include distance or spatial proximity in the analysis and identify their impacts on out-migration,9 one has to acknowledge that doing so would bias the estimation results as other time-invariant factors would be neglected. As a side note, it is virtually impossible to account for all time-invariant dyadic factors in a regression equation. In our setting, we have omitted such variables as they are absorbed by the destination-year and dyadic fixed effects, respectively.10 Despite this fact, the main specification remains in the framework of a gravity model. Finally, we conduct additional analysis by including interaction terms in the main specification to assess the existence and importance of potential heterogeneous effects. Their inclusion is discussed in detail in the following section.
This section presents and discusses the estimation results obtained from the different specifications of the gravity model of migration considered. Although the main focus is on distinguishing by different climatic zones, we start presenting results for more traditional ways of assessing heterogeneity in the effects, that is, by income groups in Table 2 and adding extreme poverty as the control in Table 3. Next, in Table 4, results by climatic zone are shown.
Table 3. The impact of climatic events on out-migration by economic sub-samples, including extreme poverty.
Table 2 presents results for the whole sample (column 1) and by income groups. Columns 2–5 show specific results for non-OECD, least developed countries (LDC), low-middle income, and upper-middle income countries, respectively. An increase in average temperatures is related to a rise in out-migration in the total sample (column 1) and all country groups except for the upper-middle-income countries (column 5). In contrast, the fact that the variable average precipitation is not statistically significant at conventional levels indicates that we cannot find a significant, direct link between a change in average precipitation and changes in international emigration. Moreover, having an earthquake in the previous year goes hand in hand with more out-migration in almost all country groups, except for the upper-middle income countries, which supposedly have a higher budget to fight against the consequences of an earthquake and rebuild houses and infrastructure. Concerning other weather events, such as landslides, storms, fires, extreme temperatures, and floods, we do not find evidence of a systematic influence on out-migration. Tests of joint significance of climatic variables and extreme weather events indicate that both sets of variables are jointly significant in most cases.
Finally, the prevalence of droughts seems to have a systematic impact on emigration, in fact reducing out-migration. This seemingly contradictory result is justifiable. For instance, droughts could result in crop failures and famine where financial resources would be mainly allocated to ensure survival, decreasing the amount available to migrate. Therefore, an increase in poverty could be a mechanism that explains the negative effect of droughts on out-migration.11
Table 3 shows that a rise in extreme poverty diminishes out-migration in almost all countries, non-OECD countries, in LDCs, and upper-middle countries. This effect is stronger for LDC countries than for the rest (extreme poverty keeps people from emigrating) and does not get counteracted by weather events that make people leave their home country by trend. Rather, it seems that people remain stuck in their countries despite their situation. This is in accordance with the trapped population arguments made in the related literature (Cattaneo and Peri, 2016; Cattaneo et al., 2019).
Table 4 presents the results of the impact of climatic events on international migration in different climate zones. In this case, we observe that a rise in average temperature is correlated with reduced out-migration in tropical areas. This result stands in contrast to the finding in Table 2 where an increase in average temperatures goes hand in hand with more emigration and seems to be driven by the excluded climatic zones (polar, temperate, and Mediterranean). The event of droughts continues to contribute to a reduction of out-migration from arid and semi-arid regions. Again, we hypothesize that this is linked to an increase in poverty, which makes emigration less feasible. Moreover, the event of storms and wildfires spurs out-migration in all arid zones, whereas storms propel out-migration also in wet tropical zones. This finding might be linked to the destruction caused by storms.
Table 4 also shows that the impact of storms and floods is broadly similar in all climatic zones, while the impact of extreme temperature and fire events varies according to the analyzed climatic zone. At the same time, earthquakes and landslides seem to have no significant impact across the different zones. Overall, the impact of climatic events is heterogeneous and might vary across climatic zones. For instance, storms and fires foster out-migration from arid areas. However, the event of droughts has the opposite impact, it reduces out-migration by supposedly affecting income in the agricultural sector making people so poor that they cannot afford to emigrate.12
Table 5 sheds light on the role of conflict in the context of climate events. Conflict per se fuels out-migration from non-OECD and LDC countries. However, it seems to have no systematic influence in conjunction with the presence of climatic events, apart from the fact that increases in average temperature in conjunction with the prevalence of conflict reduce out-migration in LDC countries. In polar, temperate, and Mediterranean13 regions, the conflict has a non-statistically significant or very weak negative impact on out-migration. Again, the interplay of conflict and climatic events seems not to have a systematic impact on out-migration.
Figure 1 allows a more detailed look at the interplay of conflict and climatic zones. It shows that there is not much difference in out-migration reactions as to average precipitation, earthquakes, landslides, wildfires, extreme temperatures, and floods between countries in conflict and quieter countries.
Figure 1. Coefficients' plot of the climatic variables, for high and low conflict levels, by arid and tropical countries.
However, an increase in average temperatures and droughts leads to rising out-migration from arid and conflict-ridden areas and storms promote out-migration from tropical and conflict-ridden countries.
A more detailed look at the impact of weather events on out-migration is presented in Figure 2, which shows the interplay between poverty and climatic zones.
Figure 2. Coefficients' plot of the climatic variables, for high and low poverty levels, by arid and tropical countries.
Figure 2 shows that the event of earthquakes, landslides, and storms reduces out-migration from poor and arid countries (left-hand-side graph, blue color). With higher average temperatures, or the prevalence of droughts, landslides, and wildfires, it highlights reduced out-migration from tropical zones for not-so-poor countries (lower poverty levels and right-hand-side graph).
This study uses bilateral migration data to analyze the effect of climatic variables on international population displacements to OECD countries and shows that the impact of weather events on out-migration is complex. This is in accordance with the previous literature to which we add a more detailed analysis of the heterogeneity of the results depending not only on the countries' income levels and the prevalence of poverty and conflicts but also on the climatic zones that predominate in each country.
The main results indicate that higher average temperatures are related to a rise in out-migration in all country groups except for the upper-middle income countries, whereas we cannot find a significant, direct relationship between a change in average precipitation and changes in international emigration. Moreover, having earthquakes is linked to more out-migration in almost all country groups, except for the upper-middle income countries, where we argue that more financial means are available to rebuild houses and infrastructure. Concerning poverty, on average, it seems to decrease out-migration, whereas, for conflict, the opposite is the case. Moreover, climatic events impact differently out-migration depending on the climatic zones to which countries belong. For instance, an increase in average temperatures is related to increased out-migration in the overall sample but to diminished emigration from tropical countries. In the same vein, an increase in average temperatures correlates with reduced emigration when controlling for poverty.
The main policy implication of the results in this study is that the increasing frequency of climatic events and the related increasing population movements generate a pressing need to increase coordination at the international level in relation to SDG17.14 The global North and the global South have to strengthen international governance in the framework of international institutions, namely, the International Organization of Migration and the United Nations. More ambitious investments in climate mitigation and adaptation in the global South are a must and will only be feasible if the global North delivers the promised aid timely and without delay.
We leave for further research a finer assessment of the effects of climatic factors on migration using grid data at the sub-country level. In this way, the climatic zones could be identified more accurately and the heterogeneity of the effects could be examined more precisely.
The original contributions presented in the study are included in the article/Supplementary material, further inquiries can be directed to the corresponding author.
IM-Z and FN-L contributed to conception and design of the study. RDLP organized the database and performed the statistical analysis. IM-Z wrote the first draft of the manuscript. All authors contributed to manuscript revision, read, and approved the submitted version.
We acknowledge support from the Open Access Publication Funds of the University of Göttingen. IM-Z was grateful for the financial support of the Spanish Research Agency (PID2020-114646RB-C42/AEI 10.13039/501100011033).
The authors declare that the research was conducted in the absence of any commercial or financial relationships that could be construed as a potential conflict of interest.
All claims expressed in this article are solely those of the authors and do not necessarily represent those of their affiliated organizations, or those of the publisher, the editors and the reviewers. Any product that may be evaluated in this article, or claim that may be made by its manufacturer, is not guaranteed or endorsed by the publisher.
The Supplementary Material for this article can be found online at: https://www.frontiersin.org/articles/10.3389/fclim.2022.986088/full#supplementary-material
1. ^Martínez-Zarzoso (2020) considers data-driven country heterogeneity.
2. ^Return migration is not considered in this analysis even though it is an interesting aspect. Taking into account return migration would require an estimation equation with additional explanatory variables, such as emergency aid received by sending countries, government support measures, NGO support etc.
3. ^In preliminary analysis, the dependent variable has also been used in absolute and in logarithmic forms, without considerable changes in the main results. The additional regression outputs can be provided by the authors upon request.
4. ^Temperature and precipitation data are not available for 13 countries, including Bermuda, Cook Islands, Guam, Hong Kong (China), and Macau. We selected these two variables because they are used by most of the empirical studies as proxies for climate change. “CCKP offers multiple indicators, including average temperature and precipitation, which are part of the climate statistics indicators from the joint CCl/CLIVAR/JCOMM Expert Team on Climate Change Detection and Indices (ETCCDI) (see: http://etccdi.pacificclimate.org/list_27_indices.shtml)”.
5. ^This database is one of the most frequently used sources of natural disasters. The disasters considered fulfill, at a minimum, one of the following criteria: killed at least ten people, affected at least 100 people, a state of emergency was declared thereafter, emergency international assistance was called thereafter.
6. ^See Supplementary Figure A.1 in the Appendix. Please notice that although Temperate, Continental, and Polar zones were initially considered, none of them predominate in developing countries and are therefore less relevant for our analysis. Nonetheless, Supplementary Table A.7 in the Appendix presents the results considering the remaining climatic zones, where both the number of countries in each zone and the significance of climatic variables and extreme events are noticeably reduced.
7. ^In order to compare regions with higher and lower levels of conflict (as seen on Figure 1), we also constructed the dummy “non-conflict,” which is complementary to the “conflict” dummy. The same can be said for the comparison between higher and lower levels of poverty (as seen on Figure 2), where the variable “lower poverty levels” is complementary to the dummy “higher poverty levels”.
8. ^A sample of the results is provided in Supplementary Table A.4 in the Appendix.
9. ^Basically, all studies that use distance or sharing a border as explanatory variables show that being more distant is related to less out-migration. One should also take in mind that this study does not deal with internal migration which is extremely hard to measure and usually proxied by the share of urban population in total population. The drawback then is that rural-rural and urban-urban (within country) migration would not be covered.
10. ^The destination-country fixed effects only absorb the effects of the income and population variables in the destination, for this reason, in preliminary regressions, we included GDP per capita, population size, and population growth measures in the origin countries and the ratio of GDP per capita (between origin and destination). These variables were found to be consistently insignificant and omitting them from the regressions did not influence the main results.
11. ^Preliminary regressions also included various control variables, such as demographic, welfare, and economic stability indicators. However, as they were consistently not significant and did not impact the main results concerning other effects, they were omitted from the main specification. The additional regressions will be provided by the authors upon request.
12. ^It is worth noting that while the high number of interaction terms could lead to inference problems when the number of observations is low, this is not the case for our sample. Given the high number of observations, the computation of interaction the terms is still reliable and inference is possible based on sufficient numbers of observations (please refer to Table 1 for descriptive statistics).
13. ^We focus on six of the climatic zones described by Köppen–Geiger (2021). Polar, temperate, and Mediterranean are the excluded zones.
14. ^SDG17: “Strengthen the means of implementation and revitalize the global partnership for sustainable development”.
Abel, G. J., Brottrager, M., Crespo Cuaresma, J., and Muttarak, R. (2019). Climate, conflict and forced migration. Glob. Environ. Change 54, 239–249. doi: 10.1016/j.gloenvcha.2018.12.003
Ayansina, A., Maren, R., John, F. M., and Tabitha, M. (2018). Rainfall variability and drought characteristics in two agro-climatic zones: an assessment of climate change challenges in Africa. Sci. Total Environ. 630, 728–737. doi: 10.1016/j.scitotenv.2018.02.196
Backhaus, A., Martinez-Zarzoso, I., and Muris, C. (2015). Do climate variations explain bilateral migration? A gravity model analysis. IZA J. Migrat. 4, 3. doi: 10.1186/s40176-014-0026-3
Barrios, S., Bertinelli, L., and Strobl, E. (2006). Climatic change and rural–urban migration: the case of sub-Saharan Africa. J. Urban Econ. 60, 357–371. doi: 10.1016/j.jue.2006.04.005
Beine, M., and Jeusette, L. (2021). A meta-analysis of the literature on climate change and migration. J. Demogr. Econ. 87, 293–344. doi: 10.1017/dem.2019.22
Beine, M., and Parsons, C. (2015). Climatic factors as determinants of international migration. Scand. J. Econ. 117, 723–767. doi: 10.1111/sjoe.12098
Beine, M., and Parsons, C. (2017). Climatic factors as determinants of international migration: redux. CESifo Econ. Stud. 63, 386–402. doi: 10.1093/cesifo/ifx017
Bennonier, T., Millock, K., and Taraz, V. (2019). Climate Change, Migration, and Irrigation. Paris School of Economics Working Paper Series. Retrieved from https://halshs.archives-ouvertes.fr/halshs-02107098 (accessed June 10, 2022).
Berlemann, M., and Steinhardt, M. (2017). Climate change, natural disasters, and migration—a survey of the empirical evidence. CESifo Econ. Stud. 63, 353–385. doi: 10.1093/cesifo/ifx019
Black, R., Adger, W. N., Arnell, N. W., Dercon, S., Geddes, A., and Thomas, D. (2011). The effect of environmental change on human migration. Glob. Environ. Change 1 (Suppl. 1), S3–S11. doi: 10.1016/j.gloenvcha.2011.10.001
Boas, I., Farbotko, C., Adams, H., Sterly, H., Bush, S., van der Geest, K., et al. (2019). Climate migration myths. Nat. Clim. Change 9, 901–903. doi: 10.1038/s41558-019-0633-3
Boas, I., Wiegel, H., Farbotko, C., Warner, J., and Sheller, M. (2022). Climate mobilities: migration, im/mobilities and mobility regimes in a changing climate. J. Ethnic Migrat. Stud. 48, 3365–3379. doi: 10.1080/1369183X.2022.2066264
Borderon, M., Sakdapolrak, P., Muttarak, R., Kebede, E., Pagogna, R., and Sporer, E. (2019). Migration influenced by environmental change in Africa: a systematic review of empirical evidence. Demogr. Res. 41, 491–544. doi: 10.4054/DemRes.2019.41.18
Cai, R., Feng, S., Oppenheimer, M., and Pytlikova, M. (2016). Climate variability and international migration: the importance of the agricultural linkage. J. Environ. Econ. Manage. 79, 135–151. doi: 10.1016/j.jeem.2016.06.005
Cattaneo, C., Beine, M., Fröhlich, C. J., Kniveton, D., Martinez-Zarzoso, I., Mastrorillo, M., et al. (2019). Human migration in the era of climate change. Rev. Environ. Econ. Policy 13, 189–206. doi: 10.1093/reep/rez008
Cattaneo, C., and Peri, G. (2016). The migration response to increasing temperatures. J. Dev. Econ. 122, 127–146. doi: 10.1016/j.jdeveco.2016.05.004
Chelli, S., Wellstein, C., Campetella, G., Canullo, R., Tonin, R., Zerbe, S., et al. (2017). Climate change response of vegetation across climatic zones in Italy. Clim. Res. 71, 249–262. doi: 10.3354/cr01443
Coniglio, N., and Pesce, G. (2015). Climate variability and international migration: an empirical analysis. Environ. Dev. Econ. 20, 434–468. doi: 10.1017/S1355770X14000722
Cottier, F., Flahaux, M. L., Ribot, J., Seager, R., and Ssekajja, G. (2022). Framing the frame. Cause and effect in climate-related migration. World Dev. 158, 106016. doi: 10.1016/j.worlddev.2022.106016
Cottier, F., and Salehyan, I. (2021). Climate variability and irregular migration to the European Union. Glob. Environ. Change 69, 102275. doi: 10.1016/j.gloenvcha.2021.102275
EM-DAT (2022). The International Disasters Database. EM-DAT. Available online at: https://www.emdat.be/ (accessed August 9, 2022).
Falco, C., Galeotti, M., and Olper, A. (2019). Climate change and migration: is agriculture the main channel? Glob. Environ. Change 59, 101995. doi: 10.1016/j.gloenvcha.2019.101995
Gröschl, J., and Steinwachs, T. (2017). Do natural hazards cause international migration? CESifo Econ. Stud. 63, 445–480. doi: 10.1093/cesifo/ifx005
Hoffmann, R., Dimitrova, A., Muttarak, R., Crespo Cuaresma, J., and Peisker, J. (2020). A meta-analysis of country-level studies on environmental change and migration. Nat. Clim. Change 10, 904–912. doi: 10.1038/s41558-020-0898-6
Homer-Dixon, T. F. (2001). Environment, Scarcity, and Violence. Princeton, NJ: Princeton University Press.
Isard, W. (1954). Location theory and trade theory: short-run analysis. Q. J. Econ. 68, 305–320. doi: 10.2307/1884452
Isard, W., and Reiner, T. A. (1966). Regional science: retrospect and prospect. Pap. Reg. Sci. Assoc. 16, 1–16. doi: 10.1007/BF01888932
Kaczan, D., and Orgill-Meyer, J. (2020). The impact of climate change on migration: a synthesis of recent empirical insights. Clim. Change 158, 281–300. doi: 10.1007/s10584-019-02560-0
Köppen–Geiger (2021). GloH2O. Available online at: http://www.gloh2o.org/koppen/ (accessed August 9, 2022).
Koubi, V., Spilker, G., Schaffer, L., and Bernauer, T. (2016). Environmental stressors and migration: evidence from Vietnam. World Dev. 79, 197–210. doi: 10.1016/j.worlddev.2015.11.016
Krishnamurthy, P. K. (2012). Disaster-induced migration: assessing the impact of extreme weather events on livelihoods. Environ. Haz. 11, 96–111. doi: 10.1080/17477891.2011.609879
Marchiori, L., Maystadt, J.-F., and Schumacher, I. (2012). The impact of weather anomalies on migration in sub-Saharan Africa. J. Environ. Econ. Manage. 63, 355–374. doi: 10.1016/j.jeem.2012.02.001
Martínez-Zarzoso, I. (2020). Searching for grouped patterns of heterogeneity in the climate–migration link. Weather Clim. Soc. 12, 695–710. doi: 10.1175/WCAS-D-19-0116.1
Maurel, M., and Tuccio, M. (2016). Climate instability, urbanisation and international migration. J. Dev. Stud. 52, 735–752. doi: 10.1080/00220388.2015.1121240
Mayda, A. M. (2010). International migration: a panel data analysis of the determinants of bilateral flows. J. Popul. Econ. 23, 1249–1274. doi: 10.1007/s00148-009-0251-x
Mnasri, A., and Nechi, S. (2021). New nonlinear estimators of the gravity equation. Econom. Modell. 95, 192–202.
Moore, M., and Wesselbaum, D. (2022). Climatic factors as drivers of migration: a review. Environ. Dev. Sustain. doi: 10.1007/s10668-022-02191-z
OECD International Migration Database (2022). International Migration Database and Labour Market Outcomes of Immigrants. OECD. Available online at: https://www.oecd.org/els/mig/keystat.htm (accessed August 9, 2022).
Owen, P., and Wesselbaum, D. (2020). On thresholds in the climate–migration relationship. Int. Rev. Appl. Econ. 34, 400–412. doi: 10.1080/02692171.2020.1749242
Piguet, E. (2022). Linking climate change, environmental degradation, and migration: an update after 10 years. WIREs Clim. Change 13, e746. doi: 10.1002/wcc.746
Schutte, S., Vestby, J., Carling, J., and Buhaug, H. (2021). Climatic conditions are weak predictors of asylum migration. Nat. Commun. 12, 2067. doi: 10.1038/s41467-021-22255-4
The International Country Risk Guide (2019). PRS Group. The International Country Risk Guide. Available online at: https://www.prsgroup.com/explore-our-products/icrg/ (accessed August 9, 2022).
The World Bank (2022). World Development Indicators Data Bank. Available online at: https://databank.worldbank.org/source/world-development-indicators (accessed August 9, 2022).
The World Bank Group (2021). Climate Change Knowledge Portal. Washington, DC: The World Bank. Available online at: https://climateknowledgeportal.worldbank.org/ (accessed June 20, 2022).
Thiede, B., Gray, C., and Mueller, V. (2016). Climate variability and inter-provincial migration in South America, 1970-2011. Glob. Environ. Change 41, 228–240. doi: 10.1016/j.gloenvcha.2016.10.005
Wesselbaum, D., and Aburn, A. (2019). Gone with the wind: international migration. Glob. Planet. Change 178, 96–109. doi: 10.1016/j.gloplacha.2019.04.008
Keywords: natural disasters, climate change, international migration, gravity models, climatic zones, poverty, conflict
Citation: Martínez-Zarzoso I, Nowak-Lehmann F and Paschoaleto RDL (2023) Climate change, natural disasters, and international migration: A country-level analysis considering climatic zones. Front. Clim. 4:986088. doi: 10.3389/fclim.2022.986088
Received: 04 July 2022; Accepted: 23 December 2022;
Published: 12 January 2023.
Edited by:
Alex de Sherbinin, Columbia University, United StatesReviewed by:
Lucas Kluge, Potsdam Institute for Climate Impact Research (PIK), GermanyCopyright © 2023 Martínez-Zarzoso, Nowak-Lehmann and Paschoaleto. This is an open-access article distributed under the terms of the Creative Commons Attribution License (CC BY). The use, distribution or reproduction in other forums is permitted, provided the original author(s) and the copyright owner(s) are credited and that the original publication in this journal is cited, in accordance with accepted academic practice. No use, distribution or reproduction is permitted which does not comply with these terms.
*Correspondence: Inma Martínez-Zarzoso, aW1hcnRpbkB1bmktZ29ldHRpbmdlbi5kZQ==
Disclaimer: All claims expressed in this article are solely those of the authors and do not necessarily represent those of their affiliated organizations, or those of the publisher, the editors and the reviewers. Any product that may be evaluated in this article or claim that may be made by its manufacturer is not guaranteed or endorsed by the publisher.
Research integrity at Frontiers
Learn more about the work of our research integrity team to safeguard the quality of each article we publish.