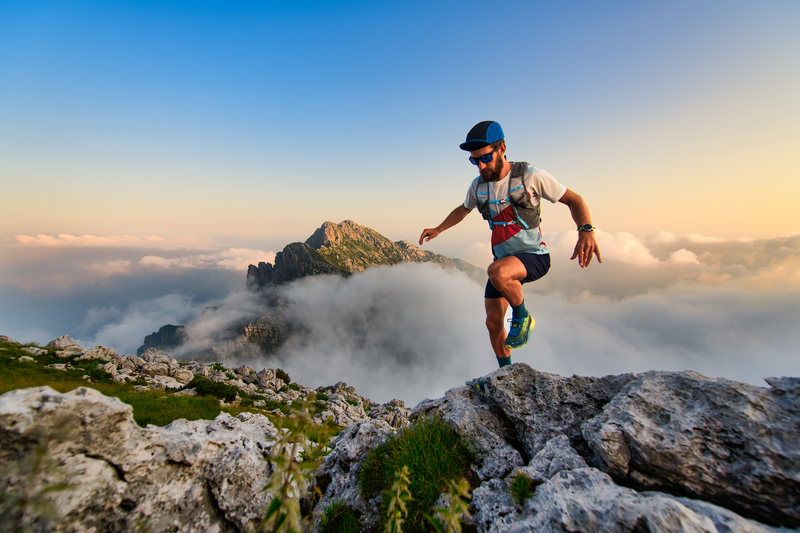
94% of researchers rate our articles as excellent or good
Learn more about the work of our research integrity team to safeguard the quality of each article we publish.
Find out more
REVIEW article
Front. Clim. , 30 November 2022
Sec. Climate Adaptation
Volume 4 - 2022 | https://doi.org/10.3389/fclim.2022.938975
Systematic tools and approaches for measuring climate change adaptation at multiple scales of spatial resolution are lacking, limiting measurement of progress toward the adaptation goals of the Paris Agreement. In particular, there is a lack of adaptation measurement or tracking systems that are coherent (measuring adaptation itself), comparable (allowing comparisons across geographies and systems), and comprehensive (are supported by the necessary data). In addition, most adaptation measurement efforts lack an appropriate counterfactual baseline to assess the effectiveness of adaptation-related interventions. To address this, we are developing a “Biomass Climate Adaptation Index” (Biomass CAI) for agricultural systems, where climate adaptation progress across multiple scales can be measured by satellite remote sensing. The Biomass CAI can be used at global, national, landscape and farm-level to remotely monitor agri-biomass productivity associated with adaptation interventions, and to facilitate more tailored “precision adaptation”. The Biomass CAI places focus on decision-support for end-users to ensure that the most effective climate change adaptation investments and interventions can be made in agricultural and food systems.
Unless greenhouse gas (GHG) emissions are curbed significantly within decadal timeframes to follow low emissions scenarios and allow us to remain within 1.5°C by mid century, our social and ecological systems will experience more frequent and intense climate change impacts throughout the rest of this century and beyond (IPCC, 2018, 2021). Such climate change impacts will include temperature increases, sea level rise, changes to precipitation patterns, and an increased prevalence and intensity of extreme weather shocks (IPCC, 2018, 2021). The productivity of many agricultural systems is expected to be negatively impacted by climate change impacts (Mbow et al., 2019), potentially reducing yields of major staple crops by 3–12% by 2050, and by 11–25% by 2100 (Wing et al., 2021). Without effective adaptation measures at farm and landscape levels, climate change will negatively impact livelihoods and food security dependant on farming (Morton, 2007; Wheeler and Von Braun, 2013; Lipper et al., 2014; Mbow et al., 2019; Wing et al., 2021). To strengthen the resilience of rural and agricultural communities at risk of climate impacts, a wide ranging portfolio of “Climate-Smart Agriculture” (CSA) practices are being deployed and scaled (Lipper et al., 2014; Rosenstock et al., 2019; FAO, 2013). Adaptation progress is critical to meeting the Paris Agreement goals, with financing entities pledging investments (The World Bank Group, 2021) and over 131 parties now prioritizing adaptation of agricultural systems in their nationally determined contributions (NDCs) (Strohmaier et al., 2016).
Conceptualization of climate change adaptation has juxtaposed incremental vs. transformational adaptation in agricultural systems (Howden et al., 2010; Kates et al., 2012; Park et al., 2012; Rickards and Howden, 2012; Rippke et al., 2016; Vermeulen et al., 2018). Incremental adaptation refers to small changes made to an existing farming system to mitigate the impacts of climate shocks, such as changing planting times or varieties in accordance with weather projections (Howden et al., 2010; Kates et al., 2012; Rickards and Howden, 2012). Fitting with the concept that systems can migrate to different states with differing levels of resilience (Holling, 1973; Allen et al., 2019), agricultural systems can undergo transformational adaptation by fundamentally changing their system state when climate change threatens the system's existence (Howden et al., 2010; Kates et al., 2012; Rickards and Howden, 2012).
It is considered that transformational adaptation may be more appropriate than incremental adaptation for agricultural systems that face intense climate change projections (Howden et al., 2010; Kates et al., 2012; Park et al., 2012; Rickards and Howden, 2012; Rippke et al., 2016; Vermeulen et al., 2018), with investment in incremental adaptation being criticized for delaying the implementation of transformational adaptations (Rickards and Howden, 2012). However, transformational adaptation efforts have significant inertia and path dependency effects to overcome, are often disorderly in practice (Vermeulen et al., 2018) and involve significant risk and barriers (Howden et al., 2010; Kates et al., 2012; Park et al., 2012; Rickards and Howden, 2012; Vermeulen et al., 2018). Both incremental and transformational adaptation processes require more robust planning processes with the involvement of multiple stakeholders (Howden et al., 2010; Kates et al., 2012; Park et al., 2012; Vermeulen et al., 2018), financial support (Kates et al., 2012; Rickards and Howden, 2012; Vermeulen et al., 2018), and better monitoring and decision-support tools to identify gaps and develop an “evidence base” for decision-making (Kates et al., 2012; Park et al., 2012; Rickards and Howden, 2012; Rippke et al., 2016; Vermeulen et al., 2018).
The resilience of social-ecological systems has been extensively investigated, where early definitions refer to resilience as “the ability of a system to absorb changes of state variables, driving variables, and parameters, and still persist” (Holling, 1973). This conceptualization of resilience recognizes that resilient systems can be unstable, shifting to a new state in different “basins of attraction” to promote resilience (Holling, 1973; Allen et al., 2019). The idea that systems can dramatically change state in a manner that leads to improved resilience is referred to as “transformation” (Walker et al., 2004). Frameworks have been devised to help understand resilience, however many complicate the definition further by introducing conflicting ideas (Allen et al., 2019). For example, some approaches focus on the stability of systems in terms of their “robustness, resistance and recovery”, insinuating that systems must remain in or return to their original state (Allen et al., 2019; Grafton et al., 2019). However, conflicting arguments should be considered together to move forward toward improved decision-making for resilience building (Allen et al., 2019). This is critically important when considering the threat of climate change, which could involuntarily push systems beyond an equilibrium threshold into a state that is maladapted to climate change impacts (Folke et al., 2010). For instance, some crops in regions of Sub-Saharan Africa could see thresholds crossed before 2100, beyond which their cultivation would not be feasible in the regions affected (Rippke et al., 2016). There remains an ongoing need for transformation of small scale systems (e.g., agricultural systems), to facilitate wider scale Earth resilience (Folke et al., 2010).
Although they are frequently juxtaposed, incremental and transformational adaptation do not necessarily need to be viewed as entirely separate pathways (Kates et al., 2012; Park et al., 2012; Rickards and Howden, 2012; Vermeulen et al., 2018). Incremental and transformational adaptation can be considered as components of a broader “Adaptation Action Cycle” (Park et al., 2012), where they occur in connected cycles across four key stages: identification of the problem and development of goals, creation of an adaptation plan, implementation of the adaptation, and monitoring and evaluation (Park et al., 2012). After monitoring and evaluation (indicated by the arrows in Figure 1), a system has the opportunity to switch from incremental to transformational adaptation, or vice versa (Park et al., 2012). In this context, “adaptation tracking systems” are critically important to monitor trends in adaptation progress through time and space, which is fundamental for (a) assessing the performance of adaptation interventions and evaluating how successful different adaptation investments are, (b) recognizing priorities, and (c) directing attention and investments toward these priority areas (Ford et al., 2013; Ford and Berrang-Ford, 2016). If evidence shows that a transformation is required, a system can enter a “preparatory phase” of decision-making (Rippke et al., 2016).
Figure 1. Schematic of transformational and incremental adaptation in relation to the benefit gained from the adaptation and the risks involved. Redrawn from Howden et al. (2010) and Rickards and Howden (2012), taking the “Adaptation Action Cycle” (Park et al., 2012) into consideration. The black arrows represent information provided by monitoring and evaluation tracking systems, allowing a transition between adaptation states (Park et al., 2012).
Adaptation tracking systems have to date gained limited attention within the UNFCCC process (Ford et al., 2015). Indeed, there are no adaptation tracking systems that are currently universally accepted or deployed by the global adaptation community (Adaptation Committee, 2021). An urgent need for the development of systematic tracking procedures and methodologies that can assess global progress toward adaptation goals is recognized (Ford et al., 2013, 2015; Ford and Berrang-Ford, 2016; Berrang-Ford et al., 2019; Adaptation Committee, 2021). Investigations into methodologies to track adaptation are increasing, driven partly by the climate financing community calling for evidence behind the outcomes of adaptation investments (Ford et al., 2013; Ford and Berrang-Ford, 2016; Jacobs and Al-Azar, 2019), and the demand for national reporting and transparency to meet the Paris Agreement's global goal on adaptation (Ford et al., 2015; UNFCCC, 2015; Ramasamy, 2017; Rosenstock et al., 2017; Berrang-Ford et al., 2019; Jacobs and Al-Azar, 2019).
Adaptation tracking systems should be “consistent, coherent, comparable and comprehensive”—referred to as the “4Cs” (Ford and Berrang-Ford, 2016). Although the “4Cs” framework is largely applied to national level reporting for adaptation in all sectors, the “4Cs” are also important to consider for any tracking methodology. Firstly, a tracking system must have a “consistent” definition of adaptation (Ford and Berrang-Ford, 2016). The UNFCCC and the IPCC define adaptation as an “adjustment in natural or human systems in response to actual or expected climatic stimuli or their effects, which moderates harm or exploits beneficial opportunities” (Agard et al., 2014; UNFCCC, 2021). In the context of the agriculture sector, the broad concept of adaptation is now well understood. For example, adaptation interventions under the banner of “Climate-Smart Agriculture” are considered as particular actions that increase productivity and resilience to climate shocks (FAO, 2013; Lipper et al., 2014; Rosenstock et al., 2019). “Coherency” refers to the ability of an adaptation tracking system to appropriately measure a successful adaptation, rather than for example, the quantity of adaptation interventions implemented (Ford and Berrang-Ford, 2016). A tracking system must also be “comparable” to enable assessment between different areas and across different time periods, involving metrics that are transparent and easily collected throughout time to analyse adaptation progress (Ford and Berrang-Ford, 2016). “Comprehensive” tracking systems composed of good quality and abundant data can facilitate comparability (Ford and Berrang-Ford, 2016).
Developing a comparable and comprehensive adaptation tracking system faces difficulties due to methodological and empirical challenges associated with data collection (Ford et al., 2015; Ford and Berrang-Ford, 2016; Adaptation Committee, 2021). For example, there are a lack of accepted indicators that can be applied universally, compared to mitigation which can be measured universally using greenhouse gas concentrations (Brooks et al., 2011; Ford et al., 2015; Ramasamy, 2017; Jacobs and Al-Azar, 2019; Adaptation Committee, 2021). It is also time consuming and expensive to collect data on multi- and wide- scales (FAO, 2013; Ramasamy, 2017; FAO and UNDP, 2019; Jacobs and Al-Azar, 2019), i.e., data collected using surveys which can take months to gather and process, and can cause a delay in obtaining findings that may be required quickly for further adaptation planning (FAO and UNDP, 2019). A further challenge noted by many in the adaptation tracking sphere is the difficulty in devising a “counterfactual baseline” to compare adaptation progress to, especially as baselines shift with climate change (Brooks et al., 2011; FAO, 2013; Ford et al., 2013, 2015; Dinshaw et al., 2014; Ford and Berrang-Ford, 2016; Ramasamy, 2017; FAO and UNDP, 2019; Jacobs and Al-Azar, 2019). A tracking system that is “coherent, standardized and relevant” is required, while also being inexpensive and accurate (Rosenstock et al., 2017).
To develop next-generation adaptation tracking systems, there is an opportunity to establish new methods of data collection building on technological innovation (Ford et al., 2016; Rosenstock et al., 2017; FAO and UNDP, 2019). In particular, remote sensing technology presents a major opportunity to improve climate change adaptation tracking systems (Ford et al., 2016; Rosenstock et al., 2017; FAO and UNDP, 2019; Schiavon et al., 2021), with the potential to track a range of indicators at high spatial and temporal resolution (Rosenstock et al., 2017). Indeed, the IPCC have acknowledged the key role of remote sensing for monitoring land based systems (IPCC, 2020). Satellite remote sensing (SRS) demonstrates the ability to consistently monitor agriculture systems across all geospatial locations globally, providing data that can generate more impartial and reliable evidence to direct decision-making (Atzberger, 2013; Yang et al., 2013). Remote sensing tools are consequently becoming more commonplace within the agriculture sector, such as the Copernicus programme in the European Union which is being used for tracking progress and issuing payments to farmers under the Common Agricultural Policy (European Commision., 2018; Schiavon et al., 2021). In the context of biomass (natural or agricultural), vegetation indices are commonly used metrics derived from SRS, such as the Normalized Difference Vegetation Index.
The Normalized Difference Vegetation Index (NDVI) is a popular vegetation index used to monitor the productivity (Pettorelli et al., 2005) and therefore the “greenness” of vegetation (Reed et al., 1994). As chlorophyll within photosynthetic organisms absorbs red light (between 0.6 and 0.7 μm) rather than near infrared light (between 0.75 and 1.35 μm), a “contrast” is created (Myneni et al., 1995; Pettorelli et al., 2005; Dinan et al., 2015). Using SRS, the reflectance of red and near infrared light and the contrast between these can be calculated using the NDVI equation (Equation 1), with values falling between −1 and 1 (Huete et al., 1994; Reed et al., 1994; Myneni et al., 1995; Pettorelli et al., 2005; Dinan et al., 2015).
Equation 1:
A range of metrics, such as maximum NDVI and cumulative NDVI, can be derived from an NDVI time-series spanning a vegetation growing season, indicating temporal phenological changes (Pettorelli et al., 2005). NDVI provides an indication of photosynthetic activity (Tucker, 1979; Asrar et al., 1984; Huete et al., 1994; Reed et al., 1994; Myneni et al., 1995; Pettorelli et al., 2005; Dinan et al., 2015), and has therefore been extensively applied to the agricultural sector to monitor crop productivity and yield (Moriondo et al., 2007; Huang et al., 2013; Lopresti et al., 2015), in addition to identification and monitoring of crop systems and management zones on a wide scale (Vrieling et al., 2011; Damian et al., 2020; Lebrini et al., 2020). NDVI has further been used to estimate net primary productivity of vegetation (Vrieling et al., 2011; Anchang et al., 2019), and to track climate change impacts on vegetation (Liu et al., 2015; Piedallu et al., 2019).
Remote sensing is also being investigated as a method to monitor the resilience of ecosystems (Ndungu et al., 2019; Jones et al., 2021). For example, NDVI has been used to measure the resilience of ecosystems following exposure to shocks such as a drought (Washington-Allen et al., 2008; Ndungu et al., 2019; Von Keyserlingk et al., 2021). However, limited research has to date been conducted to assess the use of NDVI to track climate change adaptations in the agriculture sector. Recently, the potential of MODIS satellite derived NDVI as a tool for monitoring adaptations has been demonstrated focusing on sites in Burkina Faso (Nyamekye et al., 2021) and Kenya (Ndungu et al., 2019). Using the RESTREND method (Evans and Geerken, 2004; Ibrahim et al., 2015), it is possible to monitor adaptation interventions compared to a counterfactual (Nyamekye et al., 2021). For this method, NDVI is predicted using linear regression modeling with precipitation or soil moisture data, which is then subtracted from observed NDVI to show land degradation independent of climatic influence (Evans and Geerken, 2004; Ibrahim et al., 2015).
Climatic factors, evapotranspiration, water quality, and topography all have an impact on crop growth (Jia et al., 2020). NDVI can be used to examine crop growth and its relationship with various factors to reveal the important factors for intervention and tracking climate adaptation (Phan et al., 2021; Shen and Evans, 2021; Yadav and Geli, 2021; Rigden et al., 2022). For instance, in China, precipitation was found to be the leading cause of agricultural failure over other factors (Peng et al., 2008), whereas Lamchin et al. (2018) found temperature to be the most influential factor in vegetation growth in the Asia region. Agricultural yields, such as of tea in Vietnam (Phan et al., 2021), and corn, sorghum, alfalfa, and wheat in New Mexico, USA (Yadav and Geli, 2021) have been predicted by calculating the deviation of historical mean NDVI to the current and assessing the correlation with water stress, extreme weather events, and soil moisture. From time series NDVI (Rigden et al., 2022) and (Wei et al., 2015) have depicted the cropping calendar and explored climatic variability's impact on crop yield (Rigden et al., 2022). Wei et al. (2015) define onset and end of growth as the dates when the reconstructed NDVI time-series curve increases and decreases to 20% of the overall level, respectively, and the peak of growth is defined as the date when the reconstructed NDVI time-series curve reaches the maximum. This capability endorses NDVI for developing biomass-based climate adaptation index and provides quasi-real-time information for different farming systems. Such research serves as a basis for our current “Tracking Adaptation Progress in Agricultural Systems” (TAPAS) program, which is investigating the use of SRS and deep learning derived NDVI to track agri-biomass resilience fostered by climate change adaptation interventions. Although a multitude of interdisciplinary indicators are essential for tracking adaptation as highlighted in Table 1, an SRS based NDVI monitoring system of agri-biomass provides a straightforward and ex-situ method of tracking adaptation interventions in agricultural systems to aid decision-making (Ndungu et al., 2019; Nyamekye et al., 2021).
Table 1. Selection of adaptation tracking indicators from the TAAS framework (Ramasamy, 2017).
The availability of simultaneous high spatiotemporal resolution remote sensing data is highly desirable for more effectively monitoring and predicting vegetation growth (Ning et al., 2015; Bento et al., 2020; Maselli et al., 2020; Kloos et al., 2021; Measho et al., 2021; Wang et al., 2021). It is now becoming easier to improve the spatiotemporal resolution of remote sensing data using machine learning to enhance vegetation monitoring and prediction capacity (Ferchichi et al., 2022). For instance, Mishra and Shahi (2021) have applied two deep learning (DL) models, convolutional neural networks (CNNs) and long-short-term memory (LSTM), and one more traditional machine learning model (i.e., random forest -RF) for spatiotemporal data fusion of Landsat 8 and Sentinel-2 NDVI datasets for predicting vegetation growth in China. Htitiou et al. (2021) have also used deep learning-based Very Deep Super-Resolution (VDSR) for spatiotemporal data fusion of NDVI retrieved from Sentinel-2 and Landsat 8 images for crop monitoring. Their study indicated that VDSR performed better than the enhanced spatial and temporal adaptive reflectance fusion model (ESTARFM) and the flexible spatiotemporal data fusion (FSDAF) spatiotemporal image fusion algorithms in terms of producing the least blurred images and predictions of NDVI values. To produce a high spatial resolution NDVI dataset for investigating vegetation dynamics in heterogeneous landscapes, Liao et al. (2016) proposed the NDVI-Bayesian Spatiotemporal Fusion Model (NDVI-BSFM), which integrates the Moderate Resolution Imaging Spectroradiometer (MODIS) and Landsat 8 NDVI.
Various machine learning (ML) algorithms are presented for predicting vegetation conditions using NDVI data. For instance, Huang et al. (2017) proposed the use of Multiple Linear Regression (MLR), Artificial Neural Network (ANN), and Support Vector Machine (SVM) models to improve NDVI prediction. Meanwhile, for predicting NDVI from non-stationary big remote sensing time series long short-term memory (LSTM) neural networks have been proposed by Reddy and Prasad (2018) and Rhif et al. (2020) and conventional LSTM (ConvLSTM) for crop forecasting by Ahmad et al. (2020b). The Elman recurrent neural network model (ERNN) has been used for short-term NDVI index forecasting (Stepchenko and Chizhov, 2015). Machine learning model-based extreme gradient boosting method has been used to predict vegetation growth represented by NDVI throughout the growing season from 2001 to 2018 in China (Li et al., 2021). By assessing NDVI, leaf area index (LAI) and normalized difference water index (NDWI) derived from Landsat 8 surface reflectance, grape yield estimations were made using artificial neural network (ANN) based machine learning and regression analysis (Arab et al., 2021).
Vegetation indices (NDVI and EVI) extracted from the 2001 to 2018 MODIS dataset have also been used to forecast their values in 2019 using Vector Regression, Random Forest (RF), and Linear and Polynomial Regression (Roy, 2021). For predicting maize yield from land surface temperature (LST) and NDVI in Pakistan, Ahmad et al. (2020a) applied the K-Nearest Neighbor clustering machine learning model. In predicting drought impacts on crop yield Mann et al. (2019) used a machine learning-based random forest model that takes NDVI, precipitation, and evaporation as indicators. Despite the availability of numerous deep machine learning models, their prediction accuracy may vary greatly when used in biomass-based adaptation indexes. For instance, CNN and RF display good performance in vegetation growth predictions from NDVI (Ayhan et al., 2020; Li et al., 2021; Mishra and Shahi, 2021; Ferchichi et al., 2022). The performance of machine learning models can be evaluated through a range of approaches, including Root Mean Square Error (RMSE), coefficient of determinates (R2), Pearson correlation (R), and structural similarity (SSIM), which have been used by Rhif et al. (2020), Ahmad et al. (2020b), Arab et al. (2021), Htitiou et al. (2021), Mishra and Shahi (2021), and Roy (2021). Htitiou et al. (2021) use NDVI values extracted from spatial transects created across the study site to compare the performance of Very Deep Super-Resolution (VDSR) against the enhanced spatial and temporal adaptive reflectance fusion model (ESTARFM) and the flexible spatiotemporal data fusion (FSDAF) method in producing high resolution NDVI time series datasets. Based on the random point (RPO) sample construction method, Li et al. (2022) have investigated the prediction capacity of four machine learning approaches, (backpropagation neural network, decision tree, RF, and support vector machine), to predict the quality of cultivated land, where RF was found to be the most accurate.
The accuracy and performance of combined feature engineering forecasting model (SF-CNN) and CNN for forecasting NDVI have been assessed using the root mean square error (RMSE), mean absolute percentage error (MAPE), Nash-Sutcliffe coefficient (NSE), and mean absolute error (MAE) statistics indicators (Cui et al., 2020). Furthermore, to improve the performance of machine learning prediction accuracy ensemble algorithms have been incorporated into a variety of applications including crop yield prediction and forest structure and biomass estimation (Zhang et al., 2022a). Ensemble machine learning has been used to improve vegetation and cropland classification accuracy (Aguilar et al., 2018; Drobnjak et al., 2022). For above ground biomass estimation a stacking ensemble algorithm has been used (Zhang et al., 2022b). Zhang et al. (2022a) have identified bagging, boosting, and stacking as widely used ensemble techniques. The use of ensemble machine learning algorithms in biomass-based adaptation index development will improve the performance of the index. Evaluation indices, such as Lin's Concordance Correlation Coefficient (LCCC), can further improve prediction accuracy. Zhao et al. (2022) uses ensemble modeling, averaging models using Granger–Ramanathan averaging (GRA) and LCCC, to improve prediction accuracy. They found that, though both methods improved prediction accuracy, GRA was better performing.
To meet the need for an integrative tracking system for measuring agricultural adaptation across multiple scales, within the TAPAS program we are developing a Biomass Climate Adaptation Index (Biomass CAI) as a versatile tracking tool based on SRS data to assist monitoring and decision-making for agricultural resilience. For a given area of adaptation, the Biomass CAI metric uses inputs of both observed NDVI directly from satellites, and predicted NDVI from deep learning algorithms. As the deep learning algorithms predict NDVI accounting for shifts in climatic perturbations, this represents the “counterfactual” situation that would occur if the adaptation intervention was not implemented. By subtracting the deep learning predicted NDVI from observed NDVI, our Biomass CAI can calculate a “score” for any given geospatial location, showing the deviation observed NDVI measurements make from the counterfactual baseline. The Biomass CAI presents scores over time in a time-series, giving the end-user a quantitative indication of the agri-biomass productivity and therefore the comparative “success” of the adaptation intervention over time. Figure 2A provides a schematic representation of a Biomass CAI time-series. Using SRS to collect NDVI data for the Biomass CAI complements the comparability and comprehensiveness components of the “4Cs” (Ford and Berrang-Ford, 2016) due to the high spatial and temporal resolution of satellites such as MODIS (Dinan et al., 2015), Sentinel-2 (Drusch et al., 2012) and Landsat (Wulder et al., 2019); and the capabilities of deep learning AI algorithms to generate predicted data.
Figure 2. (A) Schematic showing theoretical time-series trajectories of the Biomass CAI. Positive Biomass CAI values in the “Zone of Adaptation” reflect larger observed NDVI values than predicted NDVI values, meaning the adaptation has improved agri-biomass (crop) productivity. Observed NDVI values less than predicted NDVI values will give a negative Biomass CAI “score” in the “Zone of Degradation”, meaning the adaptation intervention has not improved agri-biomass productivity and a transformational shift could be considered; (B) End-users at Global/national scale (Conde and Lonsdale, 2005; FAO, 2013; Ford et al., 2013, 2015; Ford and Berrang-Ford, 2016; Berrang-Ford et al., 2019), regional/landscape scale (Conde and Lonsdale, 2005; Galarraga et al., 2011; FAO, 2013) and local cale (Conde and Lonsdale, 2005; FAO, 2013; Sherman and Ford, 2014) of the TAPAS Biomass CAI at corresponding spatial resolutions with appropriate satellites outlined. Sentinel-2 (Drusch et al., 2012) and Landsat (Wulder et al., 2019) appropriate satellites for local level analysis, while MODIS (Dinan et al., 2015) is an appropriate satellite for analysis at landscape, national and global resolutions.
Imageries for crop monitoring can currently be obtained from a variety of sources, including remote sensing (RS) by satellite, aerial, and unmanned aerial vehicles (UAVs) that collect data across a range of spatial, temporal, and spectral resolutions (Yao et al., 2017). Phan et al. (2021) use 1 km spatial resolution NDVI derived from MODIS for yield prediction and assessing lag time between growing season and climatic variables for a homogeneous farming system such as tea farming. Similar data have also been used by Rigden et al. (2022) to explore the trend of drought impacts on rice, cassava, maize, and sweet potato yield in Madagascar. The impact of climate change on farming systems in Sub-Saharan Africa has also been tracked using an 8 km resolution AVHRR-NDVI (Vrieling et al., 2011). A sequence of Landsat 30-meter resolution NDVI has been used by Shen and Evans (2021) for estimating wheat yields in fields.
However, for detecting crop health patterns and making appropriate interventions such as fertilizer or pesticides, multispectral and fine spatial resolution data is required. Yao et al. (2017), for example, were able to estimate wheat leaf area index (LAI) effectively with UAVs narrowband multispectral image (400–800 nm spectral regions, and 10 cm resolution) under varying growth conditions during five critical growth stages, and provide potential technical support for nitrogen fertilization optimization. Satellite data with a wider spectral band and multispectral imagery can help differentiate crop characteristics (i.e., leaves, area) at a stand level (Gnädinger and Schmidhalter, 2017; Jin et al., 2017; Varela et al., 2018), estimate crop yield (Fernandez-Ordonez and Soria-Ruiz, 2017; Yadav and Geli, 2021; Rigden et al., 2022); assess crop health such as pest pressure patterns that cannot be detected by thermal imagery (Khanal et al., 2017), examine soil moisture (Hassan-Esfahani et al., 2017), and crop water stress (Maselli et al., 2020). To overcome the spatial and spectral variations between remote sensing data for developing the CAI, it is important to recognize that satellite data are more likely to be influenced by several factors, including farming system, crop type, growing state, management objectives, and data availability.
To ensure relevance to end-users and multicriteria decision-making, our proposed Biomass CAI incorporates concepts of resilience. The application of a “resilience lens” to monitoring tools can provide more resilience-oriented outputs and outcomes (Douxchamps et al., 2017). The Biomass CAI encompasses the idea that resilient systems are dynamic (Holling, 1973; Allen et al., 2019) and can shift between incremental and transformational adaptation following monitoring and evaluation in the cycle of adaptation (Park et al., 2012). In this respect, if values remain in the “Zone of Degradation”, it can be inferred that the adaptation has not been successful and that transformation could be considered and planned as part of the “preparatory” phase for transformation (Rippke et al., 2016) and “transformative governance” (Chaffin et al., 2016). Such “pro-active” adaptation planning in anticipation of climate change shocks is crucial to avoid agricultural systems shifting involuntarily into a new maladapted state due to climate change shocks (Folke et al., 2010; Kates et al., 2012; Park et al., 2012; Vermeulen et al., 2018).
Throughout the development of the Biomass CAI, identification of potential barriers and solutions is important, as outlined in Table 2. “Attribution” is a barrier faced by most adaptation tracking systems, where the cause of success or failure is difficult to attribute directly to an adaptation initiative (Brooks et al., 2011; FAO, 2013; Ford et al., 2013; Dinshaw et al., 2014; Ford and Berrang-Ford, 2016; Ramasamy, 2017; Berrang-Ford et al., 2019; FAO and UNDP, 2019; Jacobs and Al-Azar, 2019; Adaptation Committee, 2021). Despite this, adaptation tracking systems with robust and flexible counterfactual scenarios (Brooks et al., 2011; Dinshaw et al., 2014), combined with large spatial and temporal datasets (Ford et al., 2013) can provide a strong evidence base to attribute a particular adaptation initiative as the source of success or failure. The flexibility of counterfactual baselines must focus on the wider environment to help overcome attribution difficulties, accounting for changes in both climate and economic state (Brooks et al., 2011; Dinshaw et al., 2014). The Biomass CAI can incorporate deep learning derived counterfactual baselines accounting for weather patterns, combined with detailed spatial and temporal datasets due to the nature of SRS. However, the integration of socioeconomic data is also key to overcoming this challenge of attribution (Brooks et al., 2011; Dinshaw et al., 2014). This is important because, if a score decreases on the Biomass CAI, this could reflect the failure of an adaptation to improve resilience to climate shocks, or it could alternatively reflect an increase in the cost of inputs (e.g., fertilizer, seeds, irrigation) meaning farmers have less inputs overall. Despite this, as any application of the Biomass CAI will focus on a particular geospatial location combined with counterfactual data from the same time period, end-users can have the flexibility to integrate the Biomass CAI into their broader assessments of the effectiveness of the adaptation intervention (s) at their locations of interest. The barrier of attribution will be a focus during the ongoing research and development of the Biomass CAI.
Table 2. Summary of characteristics needed for the Biomass CAI, barriers, and approaches to overcome such barriers.
The TAPAS Biomass CAI allows for use of satellite derived NDVI data appropriate for different resolutions (scales) and questions (Figure 2B). We acknowledge a range of remote sensing data sources will be required for different agricultural systems, different crops, and different geospatial locations; requiring further research to enable accurate tracking and inclusivity by TAPAS. For instance, in perennial systems such as coffee, an extreme rainfall event may destroy flowers of coffee trees meaning production would decline, but high NDVI values would be recorded due to canopy growth. For rice-based systems, techniques such as Synthetic Aperture Radar (SAR) have been used to monitor and map rice productivity (Nelson et al., 2014; Setiyono et al., 2018), an option that TAPAS is exploring. Even where appropriate satellites and data sources are used, one of the major limitations of remote sensing as a means of data collection is that data needs to be processed into a format that can be understood and analyzed by end-users (Jacobs and Al-Azar, 2019; Ndungu et al., 2019). End-users must also be enabled to routinely use the Biomass CAI for their geo-locations of interest, requiring user friendly interfaces (Jacobs and Al-Azar, 2019; Ndungu et al., 2019), training manuals to guide use and interpretation (FAO and UNDP, 2019) and/or collaboration with the TAPAS program to facilitate knowledge transfer, adoption and scaling (FAO, 2013; Ndungu et al., 2019). The TAPAS Biomass CAI platform is being developed with a graphical user interface (GUI) via mobile phone and low-bandwidth internet access, essential to bridging the “digital divide” represented by lack of access to bandwidth (Hilbert, 2016). Many regions of Africa, South America and Asia additionally have limited capacity to implement SRS based monitoring systems through direct use of satellite data (Romijn et al., 2012), where a more centralized “country-led” (FAO, 2013) Biomass CAI platform for analysis of any geospatial location within these regions may be more useful and sustainable, also minimizing expense (Romijn et al., 2012).
Importantly, NDVI measures are “blind” to many other important metrics of adaptation (including socio-economic metrics), meaning the Biomass CAI cannot be used as a solitary tracking mechanism for adaptation monitoring. The Biomass CAI will be most powerful when used with other multi-criteria indicators measuring adaptation processes and outcomes in frameworks such as TAAS (Ramasamy, 2017). For example, it is important that the Biomass CAI is complemented by “gender sensitive indicators” to assess the impact an adaptation intervention has on equality and female empowerment, in addition to crop productivity (FAO, 2013; Ramasamy, 2017; FAO and UNDP, 2019). The Biomass CAI represents a quantitative and universally scalable indicator for adaptation tracking that can be integrated with additional qualitative and quantitative data of relevance to the geospatial location subject to adaptation intervention(s). Such a case-by-case approach using the Biomass CAI as a reference “anchor” can allow for improved inter-comparability between adaptation intervention(s) at different locations, thereby improving understanding of the underlying processes behind “successful” adaptation. Although the Biomass CAI cannot directly address the time and expense required to collect data for other indicators (FAO, 2013; Ramasamy, 2017; FAO and UNDP, 2019; Jacobs and Al-Azar, 2019); the TAPAS program is exploring integration of crowdsourcing approaches into the Biomass CAI interface, to simultaneously assess crop productivity and gather participatory socio-economic data from local stakeholders to feed into larger indicator frameworks (Ford et al., 2016; Rosenstock et al., 2017; FAO and UNDP, 2019).
The Biomass CAI requires careful selection of satellites due to varying spatial and temporal resolutions. Figure 2B provides an overview of the satellites the TAPAS program is exploring for integration into our Biomass CAI platform for use at different scales, by different stakeholders and for different systems. Satellite data from satellites such as MODIS (Dinan et al., 2015) are being integrated into the Biomass CAI platform to track NDVI at global, national and landscape-level. MODIS has a minimum spatial resolution of 250 meters, and is advantageous as it can calculate a 16 day NDVI composite to minimize interference from aspects such as clouds (Dinan et al., 2015). To measure adaptation progress at local resolution on smallholder farms with a typical size of two hectares (141 x 141 meters) or less (Lowder et al., 2016), high spatial resolution satellites are needed to infer NDVI and enhance “pixel purity” without influence from other features such as non-agricultural vegetation, roads and buildings (Duveiller and Defourny, 2010). Landsat (Wulder et al., 2019) and Sentinel- 2 (Drusch et al., 2012) would be appropriate satellites for this purpose, with spatial resolutions of 30 meters (Wulder et al., 2019) and 10–60 meters (Drusch et al., 2012), respectively.
Time-series biomass maps and current crop yield estimates generated using SRS and NDVI have the potential to help various level stakeholders by providing information on how yield varies over time and space and optimizing crop management (Yao et al., 2017; Shen and Evans, 2021). Furthermore, it contributes to advanced crop and environmental analytical tools that assist farmers in implementing the appropriate management practices at the appropriate rates, times, and locations, hence, meeting both economic and environmental targets (Khanal et al., 2017). The use of RS and NDVI has the potential to improve the agricultural system by allowing stakeholders to conveniently and cost-effectively collect, visualize, and evaluate crop status and associated factors at various stages of production, as well as address problems quickly (Jung et al., 2021; Xu, 2021). Integration by the TAPAS program of multiple satellites to the Biomass CAI platform will help recognize and mitigate any bias and uncertainties arising from different satellite sensors and algorithms (Yang et al., 2013). The TAPAS project aims to identify the satellites most applicable for different spatial resolutions during the development of the Biomass CAI.
National and global-level stakeholders such as the Parties (governments) to the United Nations Framework Convention on Climate Change (UNFCCC), climate and agri-financing entities, ministries, private sector companies and NGOs are central to the development of country-wide adaptation policies, plans and investments in the agriculture sector (Conde and Lonsdale, 2005; FAO, 2013; Ford et al., 2013, 2015; Ford and Berrang-Ford, 2016; Berrang-Ford et al., 2019). Enabling decision-making by such stakeholders is a fundamental utility of the Biomass CAI. The UNFCCC's signatory parties enacted the Paris Agreement in 2015, representing a major step forward in tackling climate change with both mitigation and adaptation recognized as key goals (UNFCCC, 2015). Indeed, Article 7 of the Paris Agreement indicates the need for monitoring and evaluation systems to facilitate national reporting to the UNFCCC in the form of National Adaptation Plans (NAPs) and Nationally Determined Contributions (NDCs) (UNFCCC, 2015). NAPs aim to build on short term National Adaptation Programmes of Action (NAPAs) by planning for longer term climate impacts, acknowledging that transformational adaptation may be necessary (UNFCCC, 2012). The Biomass CAI can be integrated into monitoring and evaluation frameworks in NAPs and NDCs, tracking the progress of climate change adaptation interventions, identifying areas where transformational adaptation needs are arising, and providing the UNFCCC process with a universally comparable “anchored” metric for measuring adaptation progress regarding the resilience of the photosynthetic biomass that humanity is dependent upon. The Biomass CAI can also be used by the climate investment community (e.g., Adaptation Fund, Green Climate Fund, Development Banks, etc.) alongside existing project specific monitoring frameworks (Jacobs and Al-Azar, 2019) to allow more appropriate targeting of adaptation investments (Ford et al., 2013). Subsequently, the Biomass CAI will help foster transparent communication between donors and recipients (Dinshaw et al., 2014). There is potential for synergies with the IFAD Adaptation Framework Tool, where the Biomass CAI could augment the evidence base for both national adaptation planning and climate investments (IFAD, 2021).
Landscape-level stakeholders such as regional governments, private sector companies, NGOs and financing entities (Conde and Lonsdale, 2005; Galarraga et al., 2011; FAO, 2013) are important actors involved in the implementation of adaptation plans and programmes as they have more interaction with people than national stakeholders (Galarraga et al., 2011). This level of governance can enable the development of policies informed by local needs, such as better allocation and management of resources at a landscape level (Rama Rao et al., 2022). Targets set by central government, such as in National Adaptation Plans (NAPs), can be developed by local government to take into account the specific socioeconomic, political, and environmental factors of a particular landscape (Ji et al., 2022). As farms in an agro-ecosystem are often connected (Veldkamp et al., 2001), discussion has focused on “Climate-Smart Landscapes” to ultimately align goals and create synergies between adaptation, mitigation and food security (Scherr et al., 2012). There is a need for tracking systems at the landscape scale to monitor these synergies and to foster the development of “Climate-Smart Landscapes” over time (Scherr et al., 2012). By tracking the spatial progress of adaptation in a particular region or landscape, the Biomass CAI can aid regional-level stakeholders to identify and evaluate areas where adaptation interventions are underperforming or subject to diminishing resilience to climatic shocks. Landscape-level stakeholders, such as local government, can often be in a better position to understand the local factors which may be affecting the performance of adaptation interventions. Based on a robust use of Biomass CAI results with other indicators, revised landscape-level plans can be adjusted according to the specific needs of a region. If the Biomass CAI indicates a significant decrease in a region or values remain in the “Zone of Degradation”, the stakeholders can move forward with an “evidence base” that can aid the development and implementation of more tailored transformational adaptation interventions (Kates et al., 2012; Park et al., 2012; Rickards and Howden, 2012; Chaffin et al., 2016; Rippke et al., 2016; Vermeulen et al., 2018).
Farm-level stakeholders such as farm households, farmers organizations and value chain actors are vital stakeholders that can work from the “bottom up” as part of a participatory approach to develop, implement and sustain adaptation projects (Conde and Lonsdale, 2005; FAO, 2013; Sherman and Ford, 2014). Indeed, indigenous stakeholders have been noted as key actors in the development and use of existing remote sensing decision support tools (Ndungu et al., 2019). Differences in farm sizes, exposures to climate stresses and access to adaptive capacity all contribute to the challenge of effective measurement of adaptation interventions at farm-level. As it can measure across different geographic scales (including across farm-scales), the Biomass CAI can be used by farm-level stakeholders for more specific “precision adaptation” than regional and national stakeholders. We define precision adaptation as “climate change adaptation interventions which are spatially and temporally targeted to have the most impact on climate change adaptation for any biophysical and/or socio-economic system with a defined geospatial boundary”. We consider that such “precision adaptation” will be most powerful when focused on 10–60 meter spatial resolution units (Drusch et al., 2012; Wulder et al., 2019) across the geospatial footprint of each farm. Biomass CAI data can be used to classify individual farms according to their resilience to climate change, and hence their value locally and within agri-value chains. The integration of farmers (including women and young people) in adaptation planning and implementation processes is particularly important, with discussions at the COP23 Koronivia Joint Work on Agriculture (KJWA) calling for the integration of such local stakeholders into adaptation discussion, planning and monitoring (FAO, 2019). Using ubiquitous mobile phone technology, farmers can also be enabled as key Biomass CAI end-users to show where certain adaptations are performing best or worst on their farms, to build capacity and knowledge for planning both incremental and transformational adaptations (Kates et al., 2012; Park et al., 2012; Rickards and Howden, 2012; Chaffin et al., 2016; Rippke et al., 2016; Vermeulen et al., 2018).
Broadening the Biomass CAI end-users beyond the agriculture sector can also support wider adaptation and conservation interventions (e.g., biodiversity, ocean and ecosystem services conservation). Natural vegetation and marine ecosystems are two of the most important carbon sinks for effective mitigation (IPCC, 2018). Due to the capability of SRS based vegetation indices to measure biomass of different ecosystems, the Biomass CAI can be adapted to monitor biomass productivity and conservation in forests (Zhu and Liu, 2015) and marine photosynthetic organisms [i.e., macroalgae (Garcia et al., 2013) and microphytobenthos (Daggers et al., 2018)]. For example, many plant and tree species will need to migrate with climate change to avoid a “migration lag” that can ultimately lead to their extinction (Corlett and Westcott, 2013). In anticipation of such ecological state shifts, there are proposals that “assisted migration” can be implemented (Corlett and Westcott, 2013; Williams and Dumroese, 2013), where monitoring is a critical aspect of tracking plant movements and assisted migration efforts (Corlett and Westcott, 2013; Williams and Dumroese, 2013). Indeed, SRS is suggested as an important tool for biodiversity monitoring (Pereira et al., 2013) with major potential for the integration of the Biomass CAI into biodiversity conservation initiatives and decision-making at multiple scales.
Tracking climate change adaptation is imperative for end-users in the agriculture sector to monitor adaptation progress and recognize priorities (Ford et al., 2013; Ford and Berrang-Ford, 2016) to inform evidence-based planning and investment regarding incremental and transformational adaptation (Kates et al., 2012; Park et al., 2012; Rickards and Howden, 2012; Chaffin et al., 2016; Rippke et al., 2016; Vermeulen et al., 2018) in response to climate projections that could decimate crop yields and reduce food security (Morton, 2007; Wheeler and Von Braun, 2013; Lipper et al., 2014; Mbow et al., 2019; Wing et al., 2021). Although different adaptation tracking approaches are challenged to secure agreement (and adoption) by multiple stakeholders regarding their validity and effectiveness (Adaptation Committee, 2021), a recent report by the UNFCCC's Adaptation Committee highlights the need for flexible tracking mechanisms and frameworks that can adapt to more innovative approaches to data collection (Adaptation Committee, 2021). The Biomass CAI we are developing presents a revolutionary and innovative approach for tracking biomass productivity in locations undergoing adaptation, utilizing remote sensing capabilities to deliver worldwide monitoring at farm, landscape, national and global-levels and encompassing a deep learning predicted counterfactual baseline. The Biomass CAI can support agricultural communities in sustaining resilience through appropriate incremental or transformational adaptations in anticipation of adverse climate change projections, linking to crop yield and economic data where available. Working with high-resolution satellites (Drusch et al., 2012; Wulder et al., 2019), the Biomass CAI can also facilitate “precision adaptation”, allowing end-users to channel context-specific adaptation interventions to particular areas. The potential for the Biomass CAI is evident and development of the Biomass CAI is currently underway in our TAPAS program. We anticipate that future research and partnerships will be needed to address knowledge gaps regarding appropriate RS data sources for different crops, growth periods and geospatial locations; the barrier of attribution (Brooks et al., 2011; FAO, 2013; Ford et al., 2013; Dinshaw et al., 2014; Ford and Berrang-Ford, 2016; Ramasamy, 2017; Berrang-Ford et al., 2019; FAO and UNDP, 2019; Jacobs and Al-Azar, 2019; Adaptation Committee, 2021), the “digital divide” (Romijn et al., 2012; Hilbert, 2016), and the integration of multidisciplinary indicator frameworks (Brooks et al., 2011; Ramasamy, 2017; Jacobs and Al-Azar, 2019) to ultimately provide a comprehensive overview of adaptation interventions that deliver resilience outcomes.
The original contributions presented in the study are included in the article/supplementary material, further inquiries can be directed to the corresponding author.
The concept of a Biomass CAI was originated by AJ and developed by CS, AJ, AG, AL, and LR during the Concept and Seed Phase of the Science Foundation Ireland–Department of Foreign Affairs and Trade (Ireland) funded Tracking Adaptation Progress in Agriculture (TAPAS) project (SFI Grant No: 19/FIP/AI/7515). Under supervision of CS and PM, AF developed a range of drafts of the manuscript, which were revised by CS and PM. Additional contributions to the manuscript were made by the TAPAS team members/authors. The manuscript was finalized by CS prior to submission. All authors contributed to the article and approved the submitted version.
This study was funded by CIAT Bioversity Alliance Bioversity International - CIAT and Science Foundation Ireland.
CS, AG, and AJ acknowledge funding from Science Foundation Ireland—Department of Foreign Affairs and Trade (Ireland) for the Tracking Adaptation Progress in Agriculture (TAPAS) project (SFI Grant No: 19/FIP/AI/7515). This paper arose from a collaborative research project between the Master's degree program in Climate Change, Agriculture and Food Security of the University of Galway, and the TAPAS project. Part of this work was carried out under Real Time Monitoring of Food Systems Work Package of the Digital Innovation and Transformation Initiative with the financial support of the CGIAR Systems Transformation Research Program | Digital Innovation and Transformation Initiative under the project Tracking Climate Adaptation Progress in Agriculture. The Initiative Digital Innovation and Transformation is carried out with support from CGIAR Trust Fund Donors and through bilateral funding agreements. We would like to thank all funders who supported this research through their contributions to the CGIAR Trust Fund.
The authors declare that the research was conducted in the absence of any commercial or financial relationships that could be construed as a potential conflict of interest.
All claims expressed in this article are solely those of the authors and do not necessarily represent those of their affiliated organizations, or those of the publisher, the editors and the reviewers. Any product that may be evaluated in this article, or claim that may be made by its manufacturer, is not guaranteed or endorsed by the publisher.
Adaptation Committee (2021). Considering approaches to reviewing the overall progress made in achieving the global goal on adaptation. Bonn: AC/2021/TP/GGA, UNFCCC.
Agard, J., Schipper, L. F., Birkmass, J., Campos, M., Dubex, C., Nojiri, Y., et al. (2014). “Annex II: Glossary”. IPCC.
Aguilar, R., Zurita-Milla, R., Izquierdo-Verdiguier, E., and De By, R. (2018). A cloud-based multi-temporal ensemble classifier to map smallholder farming systems. Remote Sens. 10, 729. doi: 10.3390/rs10050729
Ahmad, I., Singh, A., Fahad, M., and Waqas, M. M. (2020a). Remote Sens.-based framework to predict and assess the interannual variability of maize yields in Pakistan using Landsat imagery. Comput. Electron. Agric. 178, 105732. doi: 10.1016/j.compag.2020.105732
Ahmad, R., Yang, B., Ettlin, G., Berger, A., and Rodríguez-Bocca, P. (2020b). A machine-learning based ConvLSTM architecture for NDVI forecasting. Int. Trans. Oper. Res. doi: 10.1111/itor.12887
Allen, C. R., Angeler, D. G., Chaffin, B. C., Twidwell, D., and Garmestani, A. (2019). Resilience reconciled. Nat. Sustain. 2, 898–900. doi: 10.1038/s41893-019-0401-4
Anchang, J. Y., Prihodko, L., Kaptu,é, A. T., Ross, C. W., Ji, W., Kumar, S. S., et al. (2019). Trends in Woody and Herbaceous Vegetation in the Savannas of West Africa. Remote Sens. 11, 576. doi: 10.3390/rs11050576
Arab, S. T., Noguchi, R., Matsushita, S., and Ahamed, T. (2021). Prediction of grape yields from time-series vegetation indices using satellite remote sens and a machine-learning approach. Remote Sens. Applicat: Society Env. 22, 100485. doi: 10.1016/j.rsase.2021.100485
Asrar, G. Q., Fuchs, M., Kanemasu, E. T., and Hatfield, J. L. (1984). Estimating absorbed photosynthetic radiation and leaf area index from spectral reflectance in wheat1. Agron. J. 76, 300–306. doi: 10.2134/agronj1984.00021962007600020029x
Atzberger, C. (2013). Advances in remote sens of agriculture: context description, existing operational monitoring systems and major information needs. Remote Sens. 5, 949–981. doi: 10.3390/rs5020949
Ayhan, B., Kwan, C., Budavari, B., Kwan, L., Lu, Y., Perez, D., et al. (2020). Vegetation detection using deep learning and conventional methods. Remote Sens. 12, 2502. doi: 10.3390/rs12152502
Bento, V. A., Gouveia, C. M., Dacamara, C. C., Libonati, R., and Trigo, I. F. (2020). The roles of NDVI and land surface temperature when using the vegetation health index over dry regions. Glob. Planet. Change 190. doi: 10.1016/j.gloplacha.2020.103198
Berrang-Ford, L., Biesbroek, R., Ford, J. D., Lesnikowski, A., Tanabe, A., Wang, F. M., et al. (2019). Tracking global climate change adaptation among governments. Nat. Clim. Chang. 9, 440–449. doi: 10.1038/s41558-019-0490-0
Brooks, N., Anderson, S., Ayers, J., Burton, I., and Tellam, I. (2011). “Tracking adaptation and measuring development”, in IIED Climate Change Working Paper No. 1. IIED (London: IIED).
Chaffin, B. C., Garmestani, A. S., Gunderson, L. H., Benson, M. H., Angeler, D. G., Arnold, C. A., et al. (2016). Transformative environmental governance. Annu. Rev. Environ. Resour. 41, 399–423. doi: 10.1146/annurev-environ-110615-085817
Conde, C., and Lonsdale, K. (2005). “Engaging stakeholders in the adaptation process,” in Adaptation Policy Frameworks for Climate Change. (Cambridge and New York: Cambridge University Press) p. 47–66.
Corlett, R. T., and Westcott, D. A. (2013). Will plant movements keep up with climate change? Trends Ecol. Evol. 28, 482–488. doi: 10.1016/j.tree.2013.04.003
Cui, C., Zhang, W., Hong, Z., and Meng, L. (2020). Forecasting NDVI in multiple complex areas using neural network techniques combined feature engineering. Int. J. Digit. Earth. 13, 1733–1749. doi: 10.1080/17538947.2020.1808718
Daggers, T. D., Kromkamp, J. C., Herman, P. M., and Van Der Wal, D. (2018). A model to assess microphytobenthic primary production in tidal systems using satellite remote sens. Remote Sens. Environ. 211, 129–145. doi: 10.1016/j.rse.2018.03.037
Damian, J. M., Pias, O. H. D. C., Cherubin, M. R., Fonseca, A. Z. D., Fornari, E. Z., and Santi, A. L. (2020). Applying the NDVI from satellite images in delimiting management zones for annual crops. Sci. Agric. 77. doi: 10.1590/1678-992x-2018-0055
Dinan, K., Barreto Munoz, A., Solano, R., and Huete, A. (2015). “MODIS Vegetation Index User's Guide (MOD13 Series)”. Arizona: The University of Arizona.
Dinshaw, A., Fisher, S., Mcgray, H., Rai, N., and Schaar, J. (2014). “Monitoring and evaluation of climate change adaptation: methodological approaches”, in OECD Environment Working Papers (Paris: OECD).
Douxchamps, S., Debevec, L., Giordano, M., and Barron, J. (2017). Monitoring and evaluation of climate resilience for agricultural development–a review of currently available tools. World Dev. Perspect. 5, 10–23. doi: 10.1016/j.wdp.2017.02.001
Drobnjak, S., Stojanovi,ć, M., Djordjevi,ć, D., Bakra,č, S., Jovanovi,ć, J., and Djordjevi,ć, A. (2022). Testing a new ensemble vegetation classification method based on deep learning and machine learning methods using aerial photogrammetric images. Front. Environ. Sci. Eng. 10. doi: 10.3389/fenvs.2022.896158
Drusch, M., Del Bello, U., Carlier, S., Colin, O., Fernandez, V., Gascon, F., et al. (2012). Sentinel-2: ESA's optical high-resolution mission for GMES operational services. Remote Sens. Environ. 120, 25–36. doi: 10.1016/j.rse.2011.11.026
Duveiller, G., and Defourny, P. (2010). A conceptual framework to define the spatial resolution requirements for agricultural monitoring using remote sens. Remote Sens. Environ. 114, 2637–2650. doi: 10.1016/j.rse.2010.06.001
European Commision. (2018). “Commission Implementing Regulation (EU) 2018/746 of 18 May 2018 amending Implementing Regulation (EU) No 809/2014 as regards modification of single applications and payment claims and checks”. European Commission (Brussels: EC).
Evans, J., and Geerken, R. (2004). Discrimination between climate and human-induced dryland degradation. J. Arid Environ. 57, 535–554. doi: 10.1016/S0140-1963(03)00121-6
FAO (2013). “Climate-Smart Agriculture Sourcebook”. Rome (Rome: Food and Agricultural Organization of the United Nations).
FAO (2019). Koronivia Joint Work on Agriuclture: Analysis of submissions on topics 2(b) and 2(c). Rome: Food and Agricultural Organization of the United Nations.
FAO and UNDP. (2019). Strengthening monitoring and evaluation for adaptation planning in agriculture sectors. Rome.
Ferchichi, A., Abbes, A. B., Barra, V., and Farah, I. R. (2022). Forecasting vegetation indices from spatio-temporal remotely sensed data using deep learning-based approaches: a systematic literature review. Ecol. Inform. 68, 101552. doi: 10.1016/j.ecoinf.2022.101552
Fernandez-Ordonez, Y. M., and Soria-Ruiz, J. (2017). “Maize crop yield estimation with Remote Sens. and empirical models”, in 2017 IEEE International Geoscience and Remote Sens. Symposium (IGARSS) (New York, NY: IEEE). doi: 10.1109/IGARSS.2017.8127638
Folke, C., Carpenter, S. R., Walker, B., Scheffer, M., Chapin, T., and Rockström, J. (2010). Resilience thinking: integrating resilience, adaptability and transformability. Ecol. Soc. 15, 20. doi: 10.5751/ES-03610-150420
Ford, J., Berrang-Ford, L., Biesbroek, R., Araos, M., Austin, S., and Lesnikowski, A. (2015). Adaptation tracking for a post-2015 climate agreement. Nat. Clim. Chang. 5, 967–969. doi: 10.1038/nclimate2744
Ford, J. D., and Berrang-Ford, L. (2016). The 4Cs of adaptation tracking: consistency, comparability, comprehensiveness, coherency. Mitig. Adapt. Strateg. Glob. Chang. 21, 839–859. doi: 10.1007/s11027-014-9627-7
Ford, J. D., Berrang-Ford, L., Lesnikowski, A., Barrera, M., and Heymann, S. J. (2013). How to track adaptation to climate change: a typology of approaches for national-level application. Ecol. Soc. 18, 40. doi: 10.5751/ES-05732-180340
Ford, J. D., Tilleard, S. E., Berrang-Ford, L., Araos, M., Biesbroek, R., Lesnikowski, A. C., et al. (2016). Opinion: Big data has big potential for applications to climate change adaptation. Proc. Nat. Acad. Sci. 113, 10729–10732. doi: 10.1073/pnas.1614023113
Galarraga, I., Gonzalez-Eguino, M., and Markandya, A. (2011). The role of regional governments in climate change policy. Environ. Policy Gov. 21, 164–182. doi: 10.1002/eet.572
Garcia, R. A., Fearns, P., Keesing, J. K., and Liu, D. (2013). Quantification of floating macroalgae blooms using the scaled algae index. J. Geophys. Res. 118, 26–42. doi: 10.1029/2012JC008292
Gnädinger, F., and Schmidhalter, U. (2017). Digital counts of maize plants by unmanned aerial vehicles (UAVs). Remote Sens. 9, 544. doi: 10.3390/rs9060544
Grafton, R. Q., Doyen, L., Bén,é, C., Borgomeo, E., Brooks, K., Chu, L., et al. (2019). Realizing resilience for decision-making. Nat. Sustain 2, 907–913. doi: 10.1038/s41893-019-0376-1
Hassan-Esfahani, L., Torres-Rua, A., Jensen, A., and Mckee, M. (2017). Spatial root zone soil water content estimation in agricultural lands using bayesian-based artificial neural networks and high- resolution visual, NIR, and thermal imagery. Irrig. Drain. Syst. 66, 273–288. doi: 10.1002/ird.2098
Hilbert, M. (2016). The bad news is that the digital access divide is here to stay: Domestically installed bandwidths among 172 countries for 1986–2014. Telecomm. Policy 40, 567–581. doi: 10.1016/j.telpol.2016.01.006
Holling, C. S. (1973). Resilience and stability of ecological systems. Annu. Rev. Ecol. Syst. 4, 1–23. doi: 10.1146/annurev.es.04.110173.000245
Howden, S., Crimp, S., and Nelson, R. (2010). “Australian agriculture in a climate of change”, in: Managing climate change: papers from the Greenhouse 2009 conference: Commonwealth Scientific and Industrial Research Organization (Canberra: CSIRO), p. 101–111.
Htitiou, A., Boudhar, A., and Benabdelouahab, T. (2021). Deep learning-based spatiotemporal fusion approach for producing high-resolution NDVI time-series datasets. Can. J. Remote Sens. 47, 182–197. doi: 10.1080/07038992.2020.1865141
Huang, J., Wang, X., Li, X., Tian, H., and Pan, Z. (2013). Remotely sensed rice yield prediction using multi-temporal NDVI data derived from NOAA's-AVHRR. PLoS ONE. 8, e70816. doi: 10.1371/journal.pone.0070816
Huang, S., Ming, B., Huang, Q., Leng, G., and Hou, B. (2017). A case study on a combination NDVI forecasting model based on the entropy weight method. Water Resour. Manag. 31, 3667–3681. doi: 10.1007/s11269-017-1692-8
Huete, A., Justice, C., and Liu, H. (1994). Development of vegetation and soil indices for MODIS-EOS. Remote Sens. Environ. 49, 224–234. doi: 10.1016/0034-4257(94)90018-3
Ibrahim, Y. Z., Balzter, H., Kaduk, J., and Tucker, C. J. (2015). Land degradation assessment using residual trend analysis of GIMMS NDVI3g, soil moisture and rainfall in Sub-Saharan West Africa from 1982 to 2012. Remote Sens. 7, 5471–5494. doi: 10.3390/rs70505471
IPCC (2018). “Global Warming of 1.5°C. An IPCC Special Report on the impacts of global warming of 1.5°C above pre-industrial levels and related global greenhouse gas emission pathways, in the context of strengthening the global response to the threat of climate change, sustainable development, and efforts to eradicate poverty”, Masson-Delmotte, V., Zhai, P., P?Rtner, H-O., Roberts, D., Skea, J., Shukla, P. R., et al. (eds).
IPCC (2020). Climate Change and Land: An IPCC Special Report on climate change, desertification, land degradation, sustainable land management, food security, and greenhouse gas fluxes in terrestrial ecosystems.
IPCC (2021). “Climate Change 2021: The Physical Science Basis. Contribution of Working Group I to the Sixth Assessment Report of the Intergovernmental Panel on Climate Change”, (eds.) Masson-Delmotte, V., Zhai, P., Pirani, A., Connors, S., Péan, L. C., Berger, S., et al. (eds). Cambridge University Press.
Jacobs, H., and Al-Azar, R. (2019). “Dare to Understand and Measure (DaTUM). A literature review of Monitoring and Evaluation (M&E) frameworks for Climate-Smart Agriculture”. Rome: FAO.
Ji, Q., Managi, S., and Zhang, D. (2022). Introduction to the special feature on managing climate risks for a sustainable future: adaptation strategies and resilience-building. Sustain. Sci. 17, 1717–1721. doi: 10.1007/s11625-022-01229-5
Jia, L., Li, Z. B., Xu, G. C., Ren, Z. P., Li, P., Cheng, Y. T., et al. (2020). Dynamic change of vegetation and its response to climate and topographic factors in the Xijiang River basin, China. Environ. Sci. Pollut. Res. Int. 27, 11637–11648. doi: 10.1007/s11356-020-07692-w
Jin, X., Liu, S., Baret, F., Hemerl,é, M., and Comar, A. (2017). Estimates of plant density of wheat crops at emergence from very low altitude UAV imagery. Remote Sens. Environ. 198, 105–114. doi: 10.1016/j.rse.2017.06.007
Jones, L., Constas, M. A., Matthews, N., and Verkaart, S. (2021). Advancing resilience measurement. Nat. Sustain. 4, 288–289. doi: 10.1038/s41893-020-00642-x
Jung, J., Maeda, M., Chang, A., Bhandari, M., Ashapure, A., and Landivar-Bowles, J. (2021). The potential of Remote Sens. and artificial intelligence as tools to improve the resilience of agriculture production systems. Curr. Opin. Biotechnol. 70, 15–22. doi: 10.1016/j.copbio.2020.09.003
Kates, R. W., Travis, W. R., and Wilbanks, T. J. (2012). Transformational adaptation when incremental adaptations to climate change are insufficient. Proc. Nat. Acad. Sci. 109, 7156–7161. doi: 10.1073/pnas.1115521109
Khanal, S., Fulton, J., and Shearer, S. (2017). An overview of current and potential applications of thermal remote sens in precision agriculture. Comput. Electron. Agric. 139, 22–32. doi: 10.1016/j.compag.2017.05.001
Kloos, S., Yuan, Y., Castelli, M., and Menzel, A. (2021). Agricultural drought detection with MODIS based vegetation health indices in southeast Germany. Remote Sens. 13. doi: 10.3390/rs13193907
Lamchin, M., Lee, W. K., Jeon, S. W., Wang, S. W., Lim, C. H., Song, C., et al. (2018). Long-term trend of and correlation between vegetation greenness and climate variables in Asia based on satellite data. MethodsX. 5, 803–807. doi: 10.1016/j.mex.2018.07.006
Lebrini, Y., Boudhar, A., Htitiou, A., Hadria, R., Lionboui, H., Bounoua, L., et al. (2020). Remote monitoring of agricultural systems using NDVI time series and machine learning methods: a tool for an adaptive agricultural policy. Arabian J. Geosci. 13, 1–14. doi: 10.1007/s12517-020-05789-7
Li, C., Wang, J., Ge, L., Zhou, Y., and Zhou, S. (2022). Optimization of sample construction based on NDVI for cultivated land quality prediction. Int. J. Environ. Res. Public Health 19, 7781. doi: 10.3390/ijerph19137781
Li, X., Yuan, W., and Dong, W. (2021). A machine learning method for predicting vegetation indices in China. Remote Sens. 13, 1147. doi: 10.3390/rs13061147
Liao, L., Song, J., Wang, J., Xiao, Z., and Wang, J. (2016). Bayesian method for building frequent landsat-like NDVI datasets by integrating MODIS and landsat NDVI. Remote Sens. 8, 452. doi: 10.3390/rs8060452
Lipper, L., Thornton, P., Campbell, B. M., Baedeker, T., Braimoh, A., Bwalya, M., et al. (2014). Climate-smart agriculture for food security. Nat. Clim. Chang. 4, 1068–1072. doi: 10.1038/nclimate2437
Liu, Y., Li, Y., Li, S., and Motesharrei, S. (2015). Spatial and temporal patterns of global NDVI trends: correlations with climate and human factors. Remote Sens. 7, 13233–13250. doi: 10.3390/rs71013233
Lopresti, M. F., Di Bella, C. M., and Degioanni, A. J. (2015). Relationship between MODIS-NDVI data and wheat yield: a case study in Northern Buenos Aires province, Argentina. Inf. Process. Agric. 2, 73–84. doi: 10.1016/j.inpa.2015.06.001
Lowder, S. K., Skoet, J., and Raney, T. (2016). The number, size, and distribution of farms, smallholder farms, and family farms worldwide. World Dev. 87, 16–29. doi: 10.1016/j.worlddev.2015.10.041
Mann, M. L., Warner, J. M., and Malik, A. S. (2019). Predicting high-magnitude, low-frequency crop losses using machine learning: an application to cereal crops in Ethiopia. Clim. Change 154, 211–227. doi: 10.1007/s10584-019-02432-7
Maselli, F., Chiesi, M., Angeli, L., Fibbi, L., Rapi, B., Romani, M., et al. (2020). An improved NDVI-based method to predict actual evapotranspiration of irrigated grasses and crops. Agri. Water Manag. 233, 106077. doi: 10.1016/j.agwat.2020.106077
Mbow, C. C, Rosenzweig, L. G., Barioni, T. G., Benton, M., Herrero, M., et al. (2019). “Food Security. In: Climate Change and Land: an IPCC special report on climate change, desertification, land degradation, sustainable land management, food security, and greenhouse gas fluxes in terrestrial ecosystems”, Shukla, J. S., Calvo Buendia, E., Masson-Delmotte, V., Pörtner, H. O., Roberts, D. C., Zhai, P., et al. IPCC.
Measho, S., Chen, B., Pellikka, P., Guo, L., Zhang, H., Cai, D., et al. (2021). Assessment of vegetation dynamics and ecosystem resilience in the context of climate change and drought in the horn of Africa. Remote Sens. 13. doi: 10.3390/rs13091668
Mishra, B., and Shahi, T. B. (2021). Deep learning-based framework for spatiotemporal data fusion: an instance of Landsat 8 and Sentinel 2 NDVI. J. Appl. Remote Sens. 15. doi: 10.1117/1.JRS.15.034520
Moriondo, M., Maselli, F., and Bindi, M. (2007). A simple model of regional wheat yield based on NDVI data. Eur. J. Agron. 26, 266–274. doi: 10.1016/j.eja.2006.10.007
Morton, J. F. (2007). The impact of climate change on smallholder and subsistence agriculture. Proc. Nat. Acad. Sci. 104, 19680–19685. doi: 10.1073/pnas.0701855104
Myneni, R. B., Hall, F. G., Sellers, P. J., and Marshak, A. L. (1995). The interpretation of spectral vegetation indexes. IEEE Transact. Geosci. Remote Sens. 33, 481–486. doi: 10.1109/TGRS.1995.8746029
Ndungu, L., Oware, M., Omondi, S., Wahome, A., Mugo, R., and Adams, E. (2019). Application of MODIS NDVI for monitoring Kenyan rangelands through a web based decision support tool. Front. Env. Sci. 7, 187. doi: 10.3389/fenvs.2019.00187
Nelson, A., Setiyono, T., Rala, A. B., Quicho, E. D., Raviz, J. V., Abonete, P. J., et al. (2014). Towards an operational SAR-based rice monitoring system in Asia: examples from 13 demonstration sites across Asia in the RIICE project. Remote Sens. 6, 10773–10812. doi: 10.3390/rs61110773
Ning, T., Liu, W., Lin, W., and Song, X. (2015). NDVI variation and its responses to climate change on the northern loess plateau of China from 1998 to 2012. Adv. Meteorol. 2015, 1–10. doi: 10.1155/2015/725427
Nyamekye, C., Schönbrodt-Stitt, S., Amekudzi, L. K., Zoungrana, B. J. B., and Thiel, M. (2021). Usage of MODIS NDVI to evaluate the effect of soil and water conservation measures on vegetation in Burkina Faso. Land Degradat. Dev. 32, 7–19. doi: 10.1002/ldr.3654
Park, S. E., Marshall, N. A., Jakku, E., Dowd, A. M., Howden, S. M., Mendham, E., et al. (2012). Informing adaptation responses to climate change through theories of transformation. Global Env. Change. 22, 115–126. doi: 10.1016/j.gloenvcha.2011.10.003
Peng, D. L., Huang, J. F., Cai, C. X., Deng, R., and Xu, J. F. (2008). Assessing the response of seasonal variation of net primary productivity to climate using Remote Sens. data and geographic information system techniques in Xinjiang. J. Integr. Plant Biol. 50, 1580–1588. doi: 10.1111/j.1744-7909.2008.00696.x
Pereira, H. M., Ferrier, S., Walters, M., Geller, G. N., Jongman, R., Scholes, R. J., et al. (2013). Essential biodiversity variables. Science. 339, 277–278. doi: 10.1126/science.1229931
Pettorelli, N., Vik, J. O., Mysterud, A., Gaillard, J.-M., Tucker, C. J., and Stenseth, N. C. (2005). Using the satellite-derived NDVI to assess ecological responses to environmental change. Trends Ecol. Evol. 20, 503–510. doi: 10.1016/j.tree.2005.05.011
Phan, P., Chen, N., Xu, L., Dao, D. M., and Dang, D. (2021). NDVI variation and yield prediction in growing season: a case study with tea in Tanuyen Vietnam. Atmosphere. 12, 962. doi: 10.3390/atmos12080962
Piedallu, C., Chéret, V., Denux, J.-P., Perez, V., Azcona, J. S., Seynave, I., et al. (2019). Soil and climate differently impact NDVI patterns according to the season and the stand type. Sci. Total Env. 651, 2874–2885. doi: 10.1016/j.scitotenv.2018.10.052
Rama Rao, C. A., Raju, B. M. K., Samuel, J., Rao, A. V. M. S., Kumar, R. N., Srinivasa Rao, M., et al. (2022). Impact of climate change on productivity of food crops: a sub-national level assessment for India. Environ. Res. Commun. doi: 10.1088/2515-7620/ac8b68
Ramasamy, S. (2017). “Tracking adaptation in agricultural sectors: climate change adaptation indicators”. Food and Agriculture Organisation of the United Nations (Rome: FAO).
Reddy, D. S., and Prasad, P. R. C. (2018). Prediction of vegetation dynamics using NDVI time series data and LSTM. Model. Earth Syst. Environ. 4, 409–419. doi: 10.1007/s40808-018-0431-3
Reed, B. C., Brown, J. F., Van Der Zee, D., Loveland, T. R., Merchant, J. W., and Ohlen, D. O. (1994). Measuring phenological variability from satellite imagery. J. Vegetat. Sci. 5, 703–714. doi: 10.2307/3235884
Rhif, M., Ben Abbes, A., Martinez, B., and Farah, I. R. (2020). A deep learning approach for forecasting non-stationary big remote sens. time series. Arabian J. Geosci. 13, 561–571. doi: 10.1007/s12517-020-06140-w
Rickards, L., and Howden, S. M. (2012). Transformational adaptation: agriculture and climate change. Crop Pasture Sci. 63, 240–250. doi: 10.1071/CP11172
Rigden, A. J., Golden, C., and Huybers, P. (2022). Retrospective predictions of rice and other crop production in madagascar using soil moisture and an NDVI-based calendar from 2010–2017. Remote Sens. 14, 1223. doi: 10.3390/rs14051223
Rippke, U., Ramirez-Villegas, J., Jarvis, A., Vermeulen, S. J., Parker, L., Mer, F., et al. (2016). Timescales of transformational climate change adaptation in sub-Saharan African agriculture. Nat. Clim. Chang. 6, 605–609. doi: 10.1038/nclimate2947
Romijn, E., Herold, M., Kooistra, L., Murdiyarso, D., and Verchot, L. (2012). Assessing capacities of non-Annex I countries for national forest monitoring in the context of REDD+. Environ. Sci. Policy 19, 33–48. doi: 10.1016/j.envsci.2012.01.005
Rosenstock, T. S., Lamanna, C., Chesterman, S., Hammond, J., Kadiyala, S., Luedeling, E., et al. (2017). When less is more: Innovations for tracking progress toward global targets. Curr. Opinion Env. Sustainab. 26–27, 54–61. doi: 10.1016/j.cosust.2017.02.010
Rosenstock, T. S., Lamanna, C., Namoi, N., Arslan, A., and Richards, M. (2019). “What is the evidence base for climate-smart agriculture in East and Southern Africa? A systematic map,” in The Climate-Smart Agriculture Papers, eds T. Rosenstock, A. Nowak, and E. Girvetz (Cham: Springer). doi: 10.1007/978-3-319-92798-5_12
Roy, B. (2021). Optimum machine learning algorithm selection for forecasting vegetation indices: MODIS NDVI and EVI. Remote Sens. Applications: Society and Environment. 23, 100582. doi: 10.1016/j.rsase.2021.100582
Scherr, S. J., Shames, S., and Friedman, R. (2012). From climate-smart agriculture to climate-smart landscapes. Agriculture Food Security. 1, 1–15. doi: 10.1186/2048-7010-1-12
Schiavon, E., Taramelli, A., Tornato, A., and Pierangeli, F. (2021). Monitoring environmental and climate goals for European agriculture: user perspectives on the optimization of the Copernicus evolution offer. J. Environ. Manage. 296, 113121. doi: 10.1016/j.jenvman.2021.113121
Setiyono, T. D., Quicho, E. D., Gatti, L., Campos-Taberner, M., Busetto, L., Collivignarelli, F., et al. (2018). Spatial rice yield estimation based on MODIS and Sentinel-1 SAR data and ORYZA crop growth model. Remote Sens. 10, 293. doi: 10.3390/rs10020293
Shen, J., and Evans, F. H. (2021). The potential of landsat NDVI sequences to explain wheat yield variation in fields in Western Australia. Remote Sens. 13, 2202. doi: 10.3390/rs13112202
Sherman, M. H., and Ford, J. (2014). Stakeholder engagement in adaptation interventions: an evaluation of projects in developing nations. Climate Policy. 14, 417–441. doi: 10.1080/14693062.2014.859501
Stepchenko, A., and Chizhov, J. (2015). NDVI short-term forecasting using recurrent neural networks. Int. J. Sci. Environ. Technol. 3. doi: 10.17770/etr2015vol3.167
Strohmaier, R., Rioux, J., Seggel, A., Meybeck, A., Bernoux, M., Salvatore, M., et al. (2016). “The agriculture sectors in the Intended Nationally Determined Contributions: analysis”, in: Environment and Natural Resources Management Working Paper 62.
The World Bank Group (2021). “Climate Change Action Plan 2021-2025, Supporting Green, Resilient and Inclusive Development”. Washington DC.
Tucker, C. J. (1979). Red and photographic infrared linear combinations for monitoring vegetation. Remote Sens. Environ. 8, 127–150. doi: 10.1016/0034-4257(79)90013-0
UNFCCC (2012). “National Adaptation Plans - Technical guidelines for the national adaptation plan process”. Least Developed Countries Expert Group.
Varela, S., Dhodda, P., Hsu, W., Prasad, P. V., Assefa, Y., Peralta, N., et al. (2018). Early-season stand count determination in corn via integration of imagery from unmanned aerial systems (UAS) and supervised learning techniques. Remote Sens. 10, 343. doi: 10.3390/rs10020343
Veldkamp, A., Kok, K., De Koning, G., Schoorl, J., Sonneveld, M., and Verburg, P. H. (2001). Multi-scale system approaches in agronomic research at the landscape level. Soil Tillage Res. 58, 129–140. doi: 10.1016/S0167-1987(00)00163-X
Vermeulen, S. J., Dinesh, D., Howden, S. M., Cramer, L., and Thornton, P. K. (2018). Transformation in practice: a review of empirical cases of transformational adaptation in agriculture under climate change. Front. Sustainable Food Syst. 2, 65. doi: 10.3389/fsufs.2018.00065
Von Keyserlingk, J., De Hoop, M., Mayor, A., Dekker, S., Rietkerk, M., and Förster, S. (2021). Resilience of vegetation to drought: Studying the effect of grazing in a Mediterranean rangeland using satellite time series. Remote Sens. Environ. 255, 112270. doi: 10.1016/j.rse.2020.112270
Vrieling, A., De Beurs, K. M., and Brown, M. E. (2011). Variability of African farming systems from phenological analysis of NDVI time series. Clim. Change 109, 455–477. doi: 10.1007/s10584-011-0049-1
Walker, B., Holling, C. S., Carpenter, S. R., and Kinzig, A. (2004). Resilience, adaptability and transformability in social–ecological systems. Ecol. Soc. 9. doi: 10.5751/ES-00650-090205
Wang, H., Li, Z., Cao, L., Feng, R., and Pan, Y. (2021). Response of NDVI of natural vegetation to climate changes and drought in China. Land. 10, 966. doi: 10.3390/land10090966
Washington-Allen, R. A., Ramsey, R., West, N. E., and Norton, B. E. (2008). Quantification of the ecological resilience of drylands using digital remote sens. Ecol. Soc. 13. doi: 10.5751/ES-02489-130133
Wei, W., Wu, W., Li, Z., Yang, P., and Zhou, Q. (2015). Selecting the optimal NDVI time-series reconstruction technique for crop phenology detection. Intelligent Automat. Soft Comput. 22, 237–247. doi: 10.1080/10798587.2015.1095482
Wheeler, T., and Von Braun, J. (2013). Climate change impacts on global food security. Science. 341, 508–13. doi: 10.1126/science.1239402
Williams, M. I., and Dumroese, R. K. (2013). Preparing for climate change: forestry and assisted migration. J. Forest. 111, 287–297. doi: 10.5849/jof.13-016
Wing, I. S., De Cian, E., and Mistry, M. N. (2021). Global vulnerability of crop yields to climate change. J. Environ. Econ. Manage. 109, 102462. doi: 10.1016/j.jeem.2021.102462
Wulder, M. A., Loveland, T. R., Roy, D. P., Crawford, C. J., Masek, J. G., Woodcock, C. E., et al. (2019). Current status of Landsat program, science, and applications. Remote Sens. Environ. 225, 127–147. doi: 10.1016/j.rse.2019.02.015
Xu, D. (2021). RETRACTED ARTICLE: Agricultural climate change based on remote sens image and emergency material supply management of agriculture, rural areas and farmers. Arabian J. Geosci. 14, 2076. doi: 10.1007/s12517-021-07221-0
Yadav, K., and Geli, H. M. E. (2021). Prediction of crop yield for new mexico based on climate and remote sens data for the 1920–2019 period. Land. 10, 1389. doi: 10.3390/land10121389
Yang, J., Gong, P., Fu, R., Zhang, M., Chen, J., Liang, S., et al. (2013). The role of satellite Remote Sens. in climate change studies. Nat. Clim. Chang. 3, 875–883. doi: 10.1038/nclimate1908
Yao, X., Wang, N., Liu, Y., Cheng, T., Tian, Y., Chen, Q., et al. (2017). Estimation of wheat LAI at middle to high levels using unmanned aerial vehicle narrowband multispectral imagery. Remote Sens. 9, 1304. doi: 10.3390/rs9121304
Zhang, Y., Liu, J., and Shen, W. (2022a). A review of ensemble learning algorithms used in remote sens. applications. Applied Sci. 12. doi: 10.3390/app12178654
Zhang, Y., Ma, J., Liang, S., Li, X., and Liu, J. (2022b). A stacking ensemble algorithm for improving the biases of forest aboveground biomass estimations from multiple remotely sensed datasets. GIScience Remote Sens. 59, 234–249. doi: 10.1080/15481603.2021.2023842
Zhao, D., Wang, J., Zhao, X., and Triantafilis, J. (2022). Clay content mapping and uncertainty estimation using weighted model averaging. Catena. 209, 105791. doi: 10.1016/j.catena.2021.105791
Keywords: climate change, agriculture, resilience, adaptation, remote sensing, artificial intelligence, machine learning
Citation: Ferguson A, Murray C, Mesfin Tessema Y, McKeown PC, Reymondin L, Loboguerrero AM, Talsma T, Allen B, Jarvis A, Golden A and Spillane C (2022) Can remote sensing enable a Biomass Climate Adaptation Index for agricultural systems? Front. Clim. 4:938975. doi: 10.3389/fclim.2022.938975
Received: 08 May 2022; Accepted: 11 October 2022;
Published: 30 November 2022.
Edited by:
Bao-Jie He, Chongqing University, ChinaReviewed by:
Dongxue Zhao, The University of Queensland, AustraliaCopyright © 2022 Ferguson, Murray, Mesfin Tessema, McKeown, Reymondin, Loboguerrero, Talsma, Allen, Jarvis, Golden and Spillane. This is an open-access article distributed under the terms of the Creative Commons Attribution License (CC BY). The use, distribution or reproduction in other forums is permitted, provided the original author(s) and the copyright owner(s) are credited and that the original publication in this journal is cited, in accordance with accepted academic practice. No use, distribution or reproduction is permitted which does not comply with these terms.
*Correspondence: Charles Spillane, Y2hhcmxlcy5zcGlsbGFuZUBudWlnYWx3YXkuaWU=
Disclaimer: All claims expressed in this article are solely those of the authors and do not necessarily represent those of their affiliated organizations, or those of the publisher, the editors and the reviewers. Any product that may be evaluated in this article or claim that may be made by its manufacturer is not guaranteed or endorsed by the publisher.
Research integrity at Frontiers
Learn more about the work of our research integrity team to safeguard the quality of each article we publish.