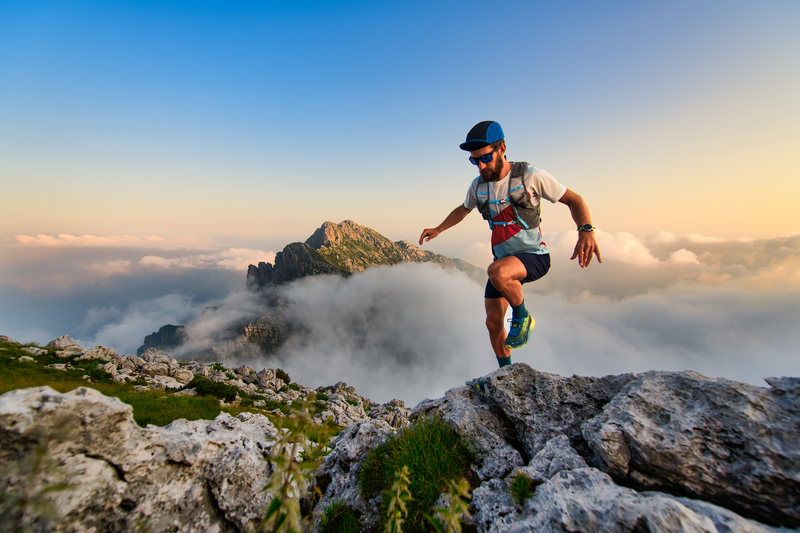
95% of researchers rate our articles as excellent or good
Learn more about the work of our research integrity team to safeguard the quality of each article we publish.
Find out more
REVIEW article
Front. Clim. , 23 August 2022
Sec. Climate Services
Volume 4 - 2022 | https://doi.org/10.3389/fclim.2022.908661
This article is part of the Research Topic Insights in Climate Services: 2021 View all 8 articles
We describe an innovative forecast presentation that aims to overcome obstacles to using seasonal climate forecasts for decision making, trace factors that influenced how seasonal forecast conventions have evolved, and describe a workshop process for training and supporting farmers in sub-Saharan Africa to use probabilistic seasonal forecasts. Mainstreaming seasonal climate forecasts through Regional Climate Outlook Forums (RCOFs) was an important milestone in the development of climate services. Most RCOFs and National Meteorological Services (NMS) adopted a subjective process to arrive at a consensus among different sources of prediction, and express the forecast as probabilities that rainfall in the upcoming season will fall in “below-normal,” “normal” or “above-normal” historical tercile categories. The Flexible Forecast is an online presentation that rectifies the main criticisms of the tercile convention by presenting downscaled forecasts as full probability distributions in probability-of-exceedance format along with the historical climate distribution. A map view provides seasonal forecast quantities, anomalies, or probabilities of experiencing above or below a user-selected threshold in amount or percentile, at the spatial resolution of the underlying gridded data (typically 4 to 5 km). We discuss factors that contributed to the persistence of the tercile convention, and milestones that paved the way to adopting seasonal forecast methods and formats that better align with user needs. The experience of adopting the new flexible forecast presentation regionally and at a national level in Eastern Africa illustrates the challenges and how they can be overcome. We also describe a seasonal forecast training and planning workshop process that has been piloted with smallholder farmers in several African countries. Beginning with participants' collective memory of past seasonal climate variations, the process leads them incrementally to understand the forecast presented in probability-of-exceedance format, and apply it to their seasonal planning decisions.
The development of forecasting at a seasonal lead time created new opportunities to manage risk in climate-sensitive sectors, and contributed significantly to the eventual development of climate services. Operational seasonal climate forecasts are available to decision makers from global and regional climate centers and many National Meteorological Services (NMS), through online portals, bulletins, and in-person forums. Since their inception in 1997, a network of Regional Climate Outlook Forums (RCOFs) across the developing world has been a focal point of international efforts to support the production of seasonal climate forecasts, and their use by climate-sensitive sectors (Buizer et al., 2016; WMO, 2016). Most global, regional and national seasonal forecast providers present the forecasts as maps showing the probability that total rainfall or average temperature in the upcoming season will fall in “below-normal,” “normal” or “above-normal” tercile categories (Min et al., 2009; Barnston and Tippett, 2014). This format has the advantage of showing probabilistic information about the forecast in a single map. However, it is quite constraining for decision makers, as it hides a great deal of information that seasonal forecast systems provide and that decision makers need for planning and risk management decisions.
This paper describes a forecast presentation that aims to address many of the known needs of decision makers operating at different levels, and overcomes longstanding obstacles to using seasonal climate forecasts for local decision making. We trace developments that initially impeded then later enabled adoption of improved seasonal forecast methods and new ways to present forecast information, and describe our experience training and supporting smallholder farmers in sub-Saharan Africa to interpret and use downscaled seasonal forecasts expressed as a shifted probability distribution.
Regional Climate Outlook Forums (RCOFs), initiated in 1996 in Southern Africa, helped mainstream the routine use of seasonal forecasts across the developing world, and bolstered the foundation for climate services (Hansen et al., 2011; Hewitt et al., 2020). Many RCOFs and most NMS follow conventions that the first RCOFs adopted, including using a subjective process to arrive at a consensus among different forecasts. These consensus seasonal forecasts are presented as maps of tercile probabilities that are homogeneous over large areas, without any information about the spatial and interannual variability of the underlying local climate. NMS are charged with downscaling and tailoring the forecasts to user needs. In many countries, the probabilistic information is often collapsed into a deterministic forecast of the most probable 1 or 2 tercile categories (e.g., “rainfall will be normal to above-normal”) before it reaches rural populations and the general public.
The initiation of RCOFs coincided with a strong and highly-publicized 1997/98 El Niño event. These events led to a surge of awareness within climate-sensitive sectors and the general public; and interest, research and investment in supporting applications of seasonal forecasts, particularly for agriculture, across the developing world (Hansen et al., 2011; Vaughan et al., 2014). Experience with farmers and other local decision makers, across countries and contexts, quickly revealed several weaknesses that limited the usability of forecasts available through RCOFs and NMS for local agricultural decision-making. The most widely reported criticism of the resulting forecast products is that they do not directly provide information about expected climate conditions at the local scale at which most climate-sensitive decision are made (O'Brien et al., 2000; Jochec et al., 2001; Ingram et al., 2002; Patt and Gwata, 2002; Podestá et al., 2002; Vogel and O'Brien, 2006). Although analyses of local historical data could be used to infer local historical rainfall ranges from forecast tercile probabilities, forecast probabilities derived at a regional scale are not necessarily reliable at a local scale (e.g., Gong et al., 2003), and may not provide additional information about local climate conditions that are important for decisions. Second, forecast probabilities are associated with thresholds that are defined by tercile boundaries (i.e., 67th and 33rd percentiles of the distribution) and not by needs of decision makers. In agriculture, relevant thresholds are context-specific, defined by factors such as crop water and growing season length requirements. Malaria control planning in Southern Africa is concerned about the probability for precipitation to fall into the climatological 25th percentile tails (Thomson et al., 2006). Disaster risk managers are concerned with relatively infrequent extreme events, such as the probability for rainfall to exceed the 90th percentile. Third, forecast categories are difficult to interpret. The need to process both directional shifts from the “normal” tercile, and probability shifts from the 33% climatological tercile probability (e.g., “increased probability of below-normal rainfall”) imposes a level of complexity that often leads to misinterpretation. Research with farmers in Australia found that they have difficulty distinguishing between the probability of experiencing a forecast category and the direction of the event from “normal” (median in this study), and have particular trouble when changes in the probability (decreased from climatology) and the event (exceeding the median) are in opposite directions (Coventry and Dalgleish, 2014). These forecast categories are often misinterpreted as something other than historic terciles (O'Brien et al., 2000; Patt and Schrag, 2003; Klopper et al., 2006; Pennesi, 2007). For example, communal farmers in Zimbabwe considered most years as “below normal” and very few as “normal” (Patt and Schrag, 2003), and Namibian farmers interpreted “normal” in a manner that included unusually high rainfall years (O'Brien et al., 2000). Furthermore, in some countries the NMS interprets probabilities differently (e.g., in Ethiopia as percentage of land area falling in the given tercile). Fourth, acting appropriately on forecast information requires understanding the degree of accuracy, or conversely the uncertainty, of the forecast (Ziervogel and Calder, 2003; Suarez and Patt, 2004). Although probabilistic tercile forecasts account for forecast uncertainty, the format leaves ambiguity about the accuracy of the forecast system, which can in turn lead to inappropriate management responses (Nicholls and Kestin, 1998; Orlove and Tosteson, 1999; Hammer et al., 2001; Changnon, 2002). Finally, farmers need information beyond average conditions during the growing season, such as timing of the season start and end, and risk of damaging dry spells or other extremes (O'Brien et al., 2000; Phillips and McIntyre, 2000; Ingram et al., 2002; Klopper et al., 2006).
The Flexible Forecast Maproom is an online seasonal forecast presentation that aims to address many of the known needs of decision makers. It was first developed at the IRI as an alternative way to present the IRI's operational global seasonal forecasts (Blumenthal et al., 2014), and later adapted and extended to several collaborating Regional Climate Centers (RCCs) and NMS building on the IRI's ENACTS initiative (Nsengiyumva et al., 2021). What makes the forecasts flexible, relative to the tercile convention, is the ability to present information derived from the full probability distribution of the forecast along with historical climatological conditions for a specified location.
The Flexible Forecast Maproom opens with a map view, and allows users to access additional information for any selected location at the spatial resolution of the forecast. For decision makers who work at an aggregate scale, the opening map view provides forecast probabilities of experiencing above or below a user-specified threshold or percentile of the climatological distribution, and in some implementations, forecast quantities or anomalies in terms of physical units (temperature in °C, or precipitation in mm) (Figure 1). Selected forecast statistics are shown at the spatial resolution of the underlying gridded data. By selecting an individual grid cell location, decision makers who work at a local scale can access locally downscaled seasonal forecast, expressed as full probability distributions in probability-of-exceedance (POE) and probability density function formats, along with the historical climatological distributions (Figure 2).
Figure 1. Map view of Flexible Forecast Maproom from Senegal, showing forecast probability that July-September 2022 precipitation will exceed the 50th percentile, issued April 2022. Source: https://iridl.ldeo.columbia.edu/maproom/ACToday/Senegal/SeasRainFcst.html?Set-Language=en&field=exceeding (accessed June 22, 2022).
Figure 2. Pixel view of Flexible Forecast Maproom from Senegal, showing July-September 2022 precipitation forecast for a 4-km pixel location in Kaolack District, issued April 2022. Source: https://iridl.ldeo.columbia.edu/maproom/ACToday/Senegal/SeasRainFcst.html?Set-Language=en®ion=bb%3A-15.88125%3A13.55625%3A-15.84375%3A13.59375%3Abb&field=exceeding&plotrange2=1000 (accessed June 22, 2022).
The general process for producing a forecast distribution for a given location involves first statistically downscaling large-scale predictors for each hindcast year onto observed historical data, then using hindcast residuals to derive the probability distribution around the expected value of the current downscaled forecast. The Senegal forecast shown in Figures 1, 2 was produced by downscaling General Circulation Models (GCM) rainfall fields onto gridded historical rainfall data for 1993–2016, using canonical correlation analysis (Mason et al., 2019; Acharya et al., 2021). The predictor was rainfall from an ensemble of three General Circulation Models (GCM), CanSIPS v.1, COLA-RSMAS-CCSM4 and NCEP-CFS v.2 from the North American Multimodel Ensemble project (Kirtman et al., 2014), over the domain 5–2°N and 5–25°W. The predictand was July-September rainfall total on a ~4 km grid across Senegal, generated by merging quality-controlled daily rain gauge data with estimates from satellite thermal infrared remote sensing (Dinku et al., 2022). While the linear downscaling method used is expected to provide the best possible estimates of the forecast distribution at each location, random variability and any instances of inhomogeneity in the sample of historical observations can lead to discontinuities between adjacent locations.
The Flexible Forecast addresses several common criticisms of the tercile convention (Hansen et al., 2011, 2019) (Table 1). Downscaling the forecast onto local climate data, and presenting the climatological distribution alongside the forecast distribution, in physical units (e.g., mm rainfall), provides all of the information needed to anticipate upcoming seasonal climate conditions at the local scale of decision-making. Presenting the full forecast distribution provides forecast quantities or probabilities associated with any decision-relevant threshold, and avoids misinterpretations that are common to categorical probabilistic forecasts. The ability to compare the shapes and position of the forecast and climatological distributions conveys the degree of uncertainty of the forecast relative to climatology, and hence the skill of the forecast, more clearly than tercile probability shifts. Assuming hindcast residuals are used to calibrate a forecast distribution, the narrower the forecast distribution, the greater the accuracy of the forecast system.
Table 1. Solutions to constraints to usability of tercile seasonal forecast presentation [adapted from Hansen et al. (2019)].
One of the features that makes the new presentation flexible is the ability to represent a range of predictands in a common format. Prototype Flexible Forecast products have been developed for onset and duration of the rainfed agricultural season, and for crop water requirements satisfaction index based on a daily soil water balance in Rwanda, and for coffee yields in Guatemala (Pons et al., 2021). Although these variables could also be presented in the tercile format, the objective forecast and statistical downscaling methods that make the Flexible Forecast possible are also prerequisites to generating seasonal forecasts of many useful derived seasonal climate and impact variables.
The use of POE graphs to communicate seasonal forecasts and climatological time series as probability distributions is not new. Forecasts based on the full probability distribution have been promoted as a complement or alternative to categorical probability formats for the USA (Barnston et al., 2000; Hartmann et al., 2002; National Academy of Sciences, 2006), and were adopted alongside the tercile convention by NOAA's Climate Prediction Center in the 1990s (Barnston et al., 2000). Within agriculture, POE graphs had been widely used in weather-driven agricultural modeling applications agricultural decision support tools by the mid-2000s, particularly in Australia and the USA (e.g., Moore et al., 1997; Paz et al., 2007; Breuer et al., 2008; Carberry et al., 2009; Hochman et al., 2009). Yet the tercile convention, and other probabilistic categorical formats (e.g., probability of exceeding median) have dominated forecast information generated by global, regional and national climate institutions.
The persistence of the tercile convention, despite widespread criticism, can be explained in part by the inertia of the RCOF process, belief that seasonal forecasts were meaningful only over large areas, national data gaps and NMS policies that limited the use of historical observations to evaluate and interpret forecasts at a local scale, and engagement processes that limited the influence of users. Through most of their 25-year history, RCOFs tended to perpetuate the status quo by implicitly endorsing the tercile convention as best practice, and by providing member country NMS with regional consensus forecasts in a form that limited options for responding to additional decision maker needs. As the RCOFs succeeded in raising awareness and mainstreaming seasonal forecasts across the developing world, the conventions they used to communicate them naturally shaped public understanding of seasonal forecasts.
While much of the early research on the applications of seasonal forecasts focused on farmers, some researchers argued against supporting farm level applications based on belief that useful skill was possible only at an aggregate scale (e.g., Broad and Agrawala, 2000; Johnston et al., 2004; Ziervogel, 2004; Ziervogel and Downing, 2004), until accumulating experience (e.g., Gong et al., 2003; Moron et al., 2006; Robertson et al., 2009) showed that downscaling to individual stations resulted in only modest loss of skill. Although gaps in meteorological observations and restrictive NMS data policies did not hinder the forecast process of international climate modeling centers or the subjective consensus process that early RCOFs adopted, these barriers to accessing historical observations shifted the challenge of interpreting and evaluating forecasts relative to local climatology from forecast providers to users, and may have delayed evaluations of forecast skill at a local scale.
The RCOFs were initially established to raise the credibility of the forecasts available from NMS by building their capacity and by reconciling multiple and sometimes conflicting forecast sources (Patt et al., 2007). The role of the international, regional and national climate institutions that led the RCOFs included convening users from climate-sensitive sectors, disseminating forecast information, and educating users. Although the RCOF process did improve awareness, credibility and use of seasonal forecasts, the process that gave users little influence over the design of the climate information products and little ownership of the process adversely impacted their salience and legitimacy (Basher et al., 2001; Cash and Buizer, 2005; Cash et al., 2006; Vogel and O'Brien, 2006; Hansen et al., 2007; Patt et al., 2007). The climate services community has since recognized the weaknesses of such a supply-driven approach, and endorsed the need to engage users in co-producing services (Kirchhoff et al., 2015; Buizer et al., 2016; Bednarek et al., 2018). Still, two decades later an analysis based on RCOF literature and interviews of participants in the WMO Global RCOF Review workshop (September, 2017), concluded that “user engagement within the RCOFs is currently framed quite narrowly, and, in practice, the role of users is often constrained to downstream involvement (i.e., after the forecast has been produced)” (Daly and Dessai, 2018).
Developments that helped enable the growing adoption of alternatives to the tercile convention include: gradual improvements in seasonal prediction practice and skill, adoption of multivariate statistical downscaling tools, expanding adoption of data merging to fill historical NMS data gaps, technical advances in objective multi-model seasonal forecast downscaling methods, a WMO recommendation to adopt objective seasonal forecast methods; and successful experience training and supporting decision makers to understand and use forecasts presented as full probability distributions.
The earliest RCOFs based subjective consensus forecasts on a mix of dynamic and statistical forecast systems. During the subsequent 2–1/2 decades, improvements in GCMs, ocean temperature forecasts, muti-model ensemble methods and post-processing have led to improvements in predictive skill and hence user confidence in seasonal forecasts (Khan et al., 2017; Johnson et al., 2019; Lin et al., 2020; Becker et al., 2022).
Most RCOFs adopted the use of a small set of multivariate statistical tools, particularly the Climate Predictability Tool (CPT, Mason et al., 2019), to correct spatial biases, calibrate forecasts, derive forecast probability distribution from hindcast residuals, and assess forecast skill. In many cases, RCOFs used consistent sets of objective downscaling methods to process forecasts from different sources, then applied a subjective process to arrive at a final consensus among the different sources. Supported by RCOF training, NMS in many countries developed the capacity to use these tools, yet their use to tailor downscaled seasonal forecasts to the needs of farmers and other local decision makers was limited to custom products and pilot activities with users at station locations with complete historical records. The development of merging methods to fill data gaps overcame the final technical obstacle to produce localized forecast information across a country on an operational basis. The IRI's ENACTS (Enhancing National Climate Services) initiative has supported more than a dozen NMS to fill spatial and temporal gaps in their station records by merging quality-controlled station records with proxy satellite remote sensing (for rainfall) and reanalysis (for temperature) data, producing long-term (beginning in 1981), high-resolution (~4 km) gridded historical data sets (Dinku et al., 2022). Because NMS steward much more data than are available to external organizations, these national data sets are expected to be of higher quality than similar global products (Dinku et al., 2014, 2018, 2022).
Although the availability of statistical downscaling tools and data merging methods addressed the main technical hurdles to addressing tercile forecast usability constraints, these tools initially required selecting a single seasonal prediction model and therefore conflicted with the established practice of basing operational forecasts on a consensus among multiple forecast models. In 2017, WMO began to recommend that RCOFs globally adopt seasonal forecast procedures that are objective, traceable and reproducible, and that quantify forecast quality (WMO, 2020). In response, IRI introduced a next generation (NexGen) seasonal forecasts system that enables forecasters to select the best dynamical models for the region or country of interest through a process-based evaluation, and automates the generation and verification of tailored multi-model, statistically calibrated predictions (Acharya et al., 2021). CPT was modified to combine and downscale multiple predictor fields gridded historical climate data, and linked to a Python interface that automates the forecast production process. These developments address the main sources of reluctance to adopting objective forecast methods and addressing the limitations of the tercile convention.
Through three overlapping projects supported by the U.S. and UK governments, we supported simultaneous efforts by the regional climate center, ICPAC (IGAD Climate Prediction and Applications Center), and by Rwanda's NMS, Meteo Rwanda, to implement improved objective seasonal forecasting methods. The introduction of flexible presentation of seasonal forecast in East Africa started at Meteo Rwanda in 2017. Meteo Rwanda's Flexible Forecast Maproom was based on available global seasonal forecast model output, downscaled onto their ENACTS 4-km gridded dataset using CPT. The Flexible Forecast Maproom was introduced to the IGAD Climate Predication and Application Center (ICPAC) around the same time, leveraging the tools developed for Rwanda. ICPAC, based in Nairobi, provides a range of services and online information products to strengthen the resilience of 11 member countries in the Greater Horn of Africa (GHA) region, and organizes the Greater Horn of Africa Climate Outlook Forum (GHACOF) three times a year.
Years of repeated stakeholder interactions, beginning with a side meeting at the 44th meeting in August 2016, were required for GHACOF to fully accept the new forecast presentation. Although ICPAC staff, and user communities we interacted with at these events, responded favorably to the flexible forecasts, ICPAC and the NMS in the region were slow to adopt the new presentation. Interactions between ICPAC and member country NMS impeded change at both levels. The NMS looked to ICPAC and GHACOF to define best practice, and resisted deviating from their established conventions. On the other hand, among the NMS Directors, who constitute the board that governs ICPAC, some initially resisted efforts to change GHACOF conventions. In our interactions at a 44th GHACOF (August 2016) side event, and in project collaborations in Rwanda and Ethiopia, some NMS directors expressed reluctance to abandon the established tercile convention that was familiar to them and their users, and concern that downscaled forecasts should be should be developed by NMS at the national rather than by ICPAC at the regional level. The main technical hurdle was that ICPAC and NMS still used a subjective consensus among multiple forecasts to derive their official forecasts, and objective forecasts produced by downscaling with CPT were not consistent with the official forecasts.
This started changing because of three main developments. First, ICPAC adopted fully objective seasonal forecast methods in response to WMO's recommendation. Second, IRI introduced a next generation (“NextGen”) seasonal forecasting system that generalized CPT to use multiple dynamical model outputs as predictors. This made it possible to use the same set of predictors for official forecasts—still following the tercile convention—and downscaled forecasts in the Flexible Forecast format. The adoption of NexGen by ICPAC, following initial implementation by Rwanda and Ethiopia, created a favorable environment for adopting the flexible forecast presentation in the region, as countries look to ICAPC for guidance, and ICPAC plays a major role in strengthening member countries' NMS. Finally, participants at the 48th GHACOF (February 2018) agreed to introduce objective, downscaled seasonal forecasts, in parallel with the established consensus forecasts, and over 2–4 years evaluate which forecast products best serve the GHACOF user community (Kipkogei et al., 2018).
While farmers routinely make critical farming and livelihood decisions in the face of climate variability and imperfect information, some training is needed to understanding any new probabilistic information format. Workshops that combine training and participatory planning have been shown to increase farmers' use of probabilistic climate information and resulting benefits in Zimbabwe (Patt et al., 2005), Burkina Faso (Roncoli et al., 2008), Kenya (Rao et al., 2015), Senegal (Ouedraogo et al., 2021) and Rwanda (Birachi et al., 2020). Several participatory communication processes have been designed to help farmers and other local decision makers understand and use seasonal forecasts in the conventional tercile format. Participatory Scenario Planning (PSP), developed by CARE International, convenes local governments and other stakeholders to incorporate seasonal forecasts into their planning (Ambani et al., 2018). PSP accounts for the uncertainty of seasonal forecasts by discussing options that would be appropriate for each of the forecast tercile categories. Participatory Integrated Climate Services for Agriculture (PICSA), developed by the University of Reading, combines location-specific climate information with participatory activities including resource mapping, activity calendars and budgeting activities to support farmers to improve crop, livestock and livelihood decisions (Clarkson et al., 2022). It emphasizes using historical analyses to adapt the farming and livelihood system to the local climate, and includes a seasonal forecast workshop process based on tercile forecasts.
Recognizing the limitations of the tercile format, the IRI developed and piloted a participatory workshop process in 2004 to help farmers understand and act on probabilistic seasonal forecasts in the POE format that would later be incorporated into the Flexible Forecast Maproom. Although the new format was not yet routinely available at the time, we considered that building capacity in the user community to understand, use and demand improved climate information would better serve farmers in the long term than building capacity to make use of products that were widely available but poorly aligned with their needs (Hansen et al., 2019).
The workshop process was based on a few premises. Farmers have a good understanding of climate variability and factor the resulting uncertainty into their management decisions, but they understand climate uncertainty in terms of their memory of past climate variations and impacts rather than in statistical terms. Although farmers, out of necessity, know how to factor climatic uncertainty into their decisions, they are subject to common cognitive biases and decision errors associated with probabilistic information (Tversky and Kahneman, 1974, 1985)—some of which work against effective use of climate information (Nicholls and Kestin, 1998; Stern and Easterling, 1999). We assumed that participating farmers have a basic level of numeracy, but made no assumptions about their literacy or prior ability to read graphs.
The training component of the workshop follows a step-by step process that starts with farmers' memory of climate variability, and leads them to interpret the new graphical forecast format and apply it to their seasonal planning decisions. Using collective experience and graphs of historical climate variability to introduce the new POE forecast format reduces cognitive challenges to processing probabilistic forecast information and applying it to management decisions, through several mechanisms. First, information about climate variability is presented as natural frequencies associated with past variability (e.g., “growing season rainfall exceeded 600 mm in 10 of the past 30 years”) before it explained as equivalent probabilities of future conditions (e.g., “the probability that rainfall will exceed 600 mm in the next growing season is 33%”). Research shows that expressing probabilities as equivalent natural frequencies largely eliminates widespread biases in interpreting probabilities (Gigerenzer and Hoffrage, 1995; Cosmides and Tooby, 1996). Second, activities that connect abstract statistical information to personal and vicarious experience reduce cognitive challenges to acting appropriately on probabilistic information by connecting the mind's analytical and experiential processing systems (Marx et al., 2007)1. “Analytical processing” refers to the system our minds use to process complex information, including probabilistic information that is expressed in statistical terms such as a probability distribution or the probability of experiencing an outcome within a particular category. “Experiential processing” refers to the system our minds use to process information that is obtained through repeated experience. Even when people are trained to interpret probabilistic information accurately, experiential processing tends to dominate decision making because it is connected to strong emotion (Epstein, 1994; Evans, 2008). Research suggests that participatory activities that relate statistical information to experience, and hence integrate the analytical and processing modes, can strengthen ability to incorporate probabilistic information into decision making and reduce common biases (Epstein, 1994; Hansen et al., 2004; Weber et al., 2004; Leron and Hazzan, 2006; Marx et al., 2007).
Like other participatory climate communication processes, the workshop incorporates group discussion to take advantage of farmer-to-farmer social learning. Farmers in any community are heterogeneous. Those who quickly understand the new climate information products and their implication for farm management decisions help their peers learn. It also seeks to build confidence in the forecast and the institution that provides it. The workshop aims to demystify forecasts produced by the NMS by presenting it with data that describe their local climate, discussing (in simplified terms) the process of producing probabilistic forecasts, and showing the accuracy and uncertainty of the forecast in transparent terms. We believe this builds confidence in using the forecast, by shifting the object of trust from the forecast provider to the data and the process.
There are six steps to the seasonal forecast training, presentation and planning workshop (Table 2). The workshop process has a training component (steps 1–4) and a shorter forecast presentation and planning component (steps 56).
Step 1, purpose and key concepts, involves explaining the purpose and roadmap of the workshop, and agreeing on the meaning of key concepts including: weather, climate, variability, frequency, uncertainty, probability, and forecast. Since the workshop will use these concepts in ways that might be new to the community, either because their language does not have an equivalent word, or the closest word has a different meaning within the community, it is important to agree on what words best represent each concept, and on the meaning in the context of climate information. If time is available, recognizing and discussing the community's traditional climate indicators can help demonstrate respect and foster trust.
Step 2, from memory to variability, is designed to help farmers relate time series graphs to their collective experience. The process starts with eliciting participants' collective memory of growing season rainfall (i.e., “dry,” “medium,” “wet”), and resulting agricultural performance (i.e., “poor,” “medium,” “good”) for the five most recent years. Measured seasonal rainfall totals are then presented and discussed. Involving participants in constructing a time series graph, to scale, for the set of years they discussed fosters understanding and confidence, particularly where participants are unfamiliar with graphs. Once they are comfortable with the format, computer-generated graphs with the full set of available historical data are presented and discussed.
Step 3, from variability to probability, introduces the probability of exceedance (POE) graph and participants them to interpret it. However, it is first presented in frequency terms, as a way to describe historical variability. Involving participants in sorting recent growing season rainfall totals onto a blank POE graph, with frequency rather than probability on the vertical axis, helps them understand the new format and recognize its relationship with the time series graph. Once they are comfortable with the format, the facilitator replaces relative frequency with probability on the vertical axis, and explains that the relative frequency of experiencing particular climate conditions in the past is an estimate of the probability that they will be experienced in the future. Once participants are comfortable with the graph format and its interpretation, they practice reading the probability of experiencing rainfall above, and then below, some threshold that they agree would be relevant to farm decisions.
Step 4, forecasts shift probabilities, presents a forecast as new information that shifts the historical (i.e., climatological) probability distribution, and equips participants to interpret a forecast in POE format. In a location where farmers are familiar with El Niña and/or La Niña, the shifted distribution can be obtained by showing a POE graph for El Niño alongside the climatological distribution, and the corresponding time series graph with El Niño years highlighted. A simplified explanation of what meteorologists mean when they refer to El Niño (i.e., warmer than normal eastern equatorial Pacific Ocean temperatures), and how it can influence climate conditions in other parts of the world, can help build confidence. The alternative is to ask participants to agree on a familiar location with a wetter (dryer) climate, discus how its climate would be represented by a POE curve to the right (left) of their location, and lead participants to discuss what it would be like to operate their farm in the climate of the other location. Once participants demonstrate a qualitative understanding of what a forecast shift in the probability distribution means, they practice reading probabilities of experiencing rainfall above and below the agreed seasonal rainfall total thresholds from Step 3, for a forecast from a previous year.
Steps 1–4 equip farmers to trust and interpret the seasonal forecast. In steps 5–6, the focus shifts from training to planning for the upcoming season. When the forecast is presented, concepts presented in steps 3–4 will be applied to interpret the actual forecast.
The current seasonal forecast, current climate conditions, and potentially other information that might impact farmers' plans for the upcoming season are presented in Step 5. If the current forecast includes additional seasonal variables (e.g., season onset and cessation dates, probabilities of dry spells, growing degree-days), these forecasts can be presented and discussed. The forecast presentation leads into Step 6, farm planning, when farmers discuss and decide what seasonal management decisions, if any, they will change in response to the forecast. Group discussion allows participants to learn from other farmers, and get feedback on their ideas. While agricultural extension personnel and other professionals present may provide information about management options and answer questions, the process aims to support farmers' decision making.
The full forecast training, communication and planning workshop is needed only the first time farmers are exposed to the Flexible Forecast format. It might be desirable to conduct the training (steps 1–4) during a slow part of the agricultural calendar when farmers and facilitators have more time available, and hold a shorter workshop to present the actual forecast and adjust plans for the upcoming season (steps 5–6). The size of a workshop should generally be limited to about 25–30 farmers so all individuals can participate in discussion and participatory activities, and the facilitator can gauge when participants understand the concepts.
The process can be adapted to farmers with differing education and literacy levels. For example, where literacy rates are low, pictures could be used to identify graph components and characterize farmers' perceptions of recent years, and more discussion and repetition will likely be required. Where participants are unfamiliar with percentages, probabilities could be expressed as number of years out of ten. The participatory activities deriving time series and POE graphs could probably be eliminated and overall time reduced where farmers are already familiar with graphs. While our pilot training workshops took 12–14 h over two days, it may be possible to reduce them to a single day by eliminating or reducing participatory activities generating graphs, if the majority of farmers are literate and comfortable with graphs.
We first developed the training process in 2003 as a self-guided tutorial in the form of PowerPoint slides, and tested it with 13 farmers in southern Florida. Answers to questions built into the tutorial and a subsequent questionnaire indicated that the participants found the tutorial useful and understandable, interpreted the climatological and forecast distribution graphs correctly in most cases, and expressed greater willingness to modify their farming practices in response to an El Nino or La Nina forecast as a result (Hansen et al., 2004). With encouraging results from the initial study, we adapted the logic as a workshop protocol, and piloted it with groups of farmers in two locations in Kenya in 2004, in Senegal (Ndiaye et al., 2013) and Kenya (Njiru et al., 2015) in 2011, and as part of a training of trainers workshop in Tanzania in 2013 (Hansen, 2015).
For each of these pilot workshops, research teams manually downscaled seasonal forecasts and generated and printed the historical and forecast graphs, based on local station records. In Rwanda, the Flexible Forecast presentation and elements of the seasonal forecast workshop process were incorporated into the PICSA approach. The experience demonstrated that Flexible forecast Maprooms in combination with historical analysis Maprooms enable participatory processes to scale to more than 110,000 farmers, by allowing agricultural extension personnel or other trained facilitators to access, download and print the graphs used for farmer workshops, for any grid cell location in the country, without placing additional burdens on NMS staff (Hansen et al., 2021).
Reporting from workshop breakout groups and one quantitative evaluation provide evidence of the effectiveness of the workshop process. Each of the pilot workshops included breakout groups that discussed the interpretation of hypothetical forecasts and their implications for management. In all cases, breakout group reports to plenary demonstrated that participating farmers were able to interpret forecasts presented as shifted POE graphs, and identify appropriate farm management responses. Participants typically identified management responses that were consistent with responses that local agricultural experts had suggested, but that included options that the experts had not considered. The Kenya 2011 workshops were part of a small (n = 117 farmers) randomized study that assessed the effectiveness of the training workshops, and workshops in which forecast-based management advisories were disseminated, alone and in combination, relative to control villages that did not participate in forecast-related workshops (Rao et al., 2015). The forecast showed a weak probability shift toward higher rainfall for the upcoming October-December growing season. The results indicate that both forecast training and forecast-based management advisories contribute to farmer decision making and livelihoods, with some differences in the type of benefit. Farmers who were provided forecast training or advisories, alone or in combination, on average reduced cropped areas, invested in more intensive crop management, achieved higher cereal yields, and placed higher subjective economic value on the service expressed as willingness-to-pay, relative to farmers from control villages. Between the two types of intervention, subjective value was more strongly associated with the training workshop, and changes in management and cereal yields were more strongly associated with the advisory workshop. Heterogeneity among sampled villages and small sample size raise the possibility that differences were due to factors other than the forecast communication interventions.
Constraints to the usability of seasonal forecasts for local decision making in the developing world have been recognized and studied in depth since RCOFs were initiated in 1997. Many of these constraints reflect the conventions that were adopted for producing and communicating forecast information, confounded by unavailability of local historical data needed to interpret forecasts, rather than limitations that are inherent to seasonal climate prediction. The situation has begun to change as a few countries have adopted objective forecast methods and a Flexible Forecast online presentation. The slow process of adopting improved forecast methods and new information products reflects a tension between user demand and supply-side forces that favor the status quo. Now that major technical and institutional impediments have largely been addressed, we anticipate that more countries, regional centers and RCOFs will soon expand the range of options and formats by which their users can access seasonal forecast information—building on the principles embodied in the Flexible Forecast Maproom whether or not they adopt the Maprooms described in this paper.
Smallholder farmers are among the most vulnerable populations to the impact of a variable and changing climate, and hence the focus of much of the effort to support the application of seasonal forecasts. These efforts reflect a tension between efforts to simplify forecast information based on assumed limitations of smallholder farmers on the one hand, and on the other hand efforts to build farmers' capacity to access, understand and use the climate information that best aligns with their decisions. We have taken the latter approach, seeking to support farmers to understand and use information in the Flexible Forecast format. Although we have not yet had opportunity to rigorously assess the effectiveness of our participatory process relative to other formats and communication strategies, the available evidence supports the expectation that farmers can take advantage of the rich information available in the Flexible Forecast presentation, with modest training and support.
JH led development of the paper and overall framing, coordinated, and integrated coauthor input. TD drafted text on experience in Eastern Africa and the ENACTS approach. AR contributed text on seasonal forecasting methods and the IRI global flexible forecast. RC, ST, and SM provided text to improve clarity and completeness and supporting references, throughout the manuscript. All authors contributed to the article and approved the submitted version.
We acknowledge funding from the International Development Association (IDA) of the World Bank to the Accelerating Impact of CGIAR Climate Research for Africa (AICCRA) project. Accelerating Impacts of CGIAR Climate Research for Africa (AICCRA) is a project that helps deliver a climate-smart African future driven by science and innovation in agriculture. It is led by the Alliance of Bioversity International and CIAT, and supported by a grant from the International Development Association (IDA) of the World Bank. Explore AICCRA's work at aiccra.cgiar.org.
We gratefully acknowledge guest editors Shraddhanand Shukla, Celso Von Randow, Elena Tarnavsky, and Chris Funk for leading the Insights in Climate Services: 2021 special topic collection. Asher Siebert produced the Senegal forecast illustrated in Figures 1, 2. The paper draws significantly on experience and lessons from climate service projects: (a) Rwanda Climate Services for Agriculture, (b) Climate Services for Africa—both supported by the United States Agency for International Development (USAID), (c) WISER Support to ICPAC, supported by the UK Government's Foreign, Commonwealth and Development Office (FCDO) as part of the Weather and Climate Services for Africa (WISER) program, and (d) Adapting Agriculture to Climate Today for Tomorrow (ACToday), supported by Columbia World Projects, Columbia University in the City of New York.
The authors declare that the research was conducted in the absence of any commercial or financial relationships that could be construed as a potential conflict of interest.
All claims expressed in this article are solely those of the authors and do not necessarily represent those of their affiliated organizations, or those of the publisher, the editors and the reviewers. Any product that may be evaluated in this article, or claim that may be made by its manufacturer, is not guaranteed or endorsed by the publisher.
1. ^Terminology varies in the cognitive psychology literature. The distinction between analytical and experiential processing (Epstein, 1994; Marx et al., 2007) is roughly equivalent to System 1 and System 2 thinking (Stanovich and West, 2000; Evans, 2003), intuition and reasoning (Kahneman, 2003), and more recently, Type 1 and Type 2 processing (Evans and Stanovich, 2013).
Acharya, N., Ehsan, M. A., Admasu, A., Teshome, A., and Hall, K. J. C. (2021). On the next generation (NextGen) seasonal prediction system to enhance climate services over Ethiopia. Clim. Serv. 24, 100272. doi: 10.1016/j.cliser.2021.100272
Ambani, M., Shikuku, P., Maina, J. W., and Percy, F. (2018). Practical guide to PSP: Participatory Scenario Planning Using Seasonal Forecasts. Geneva: CARE International. Available online at: https://careclimatechange.org/wp-content/uploads/2019/06/Practical-guide-to-PSP-web.pdf (accessed August 9, 2022).
Barnston, A. G., He, Y., and Unger, D. A. (2000). A forecast product that maximizes utility for state-of-the-art seasonal climate prediction. Bull. Am. Meteorologic. Soc. 81, 1271–1280.
Barnston, A. G., and Tippett, M. K. (2014). Climate information, outlooks, and understanding–where does the IRI stand? Earth Perspect. 1, 20. doi: 10.1186/2194-6434-1-20
Basher, R., Clark, C., Dilley, M., and Harrison, M. (2001). “Coping with climate: a way forward,” in Summary and Proposals for Action, ed R. Basher et al. Palisades, New York, NY: International Research Institute for Climate Prediction.
Becker, E. J., Kirtman, B. P., L'Heureux, M., Muñoz, Á. G., and Pegion, K. (2022). A decade of the North American Multimodel Ensemble (NMME): research, application, and future directions. Bull. Am. Meteorologic. Soc. 103, E973–E995. doi: 10.1175/BAMS-D-20-0327.1
Bednarek, A. T., Wyborn, C., Cvitanovic, C., Meyer, R., Colvin, R. M., Addison, P. F. E., et al. (2018). Boundary spanning at the science–policy interface: the practitioners' perspectives. Sustain. Sci. 13, 1175–1183. doi: 10.1007/s11625-018-0550-9
Birachi, E., Hansen, J. W., Radeny, M., Mutua, M., Mbugua, M. W., Munyangeri, Y., et al (2020). Rwanda Climate Services for Agriculture: Evaluation of farmers' awareness, use and impacts. Working Paper. CGIAR Research Program on Climate Change, Agriculture and Food Security. Available online at: https://cgspace.cgiar.org/handle/10568/108052 (Accessed December 14, 2020).
Blumenthal, M. B., Bell, M., del Corral, J., Cousin, R., and Khomyakov, I. (2014). IRI Data Library: enhancing accessibility of climate knowledge. Earth Perspectiv. 1, 19. doi: 10.1186/2194-6434-1-19
Breuer, N. E., Cabrera, V. E., Ingram, K. T., Broad, K., and Hildebrand, P. E. (2008). AgClimate: a case study in participatory decision support system development. Climatic Change 87, 385–403. doi: 10.1007/s10584-007-9323-7
Broad, K., and Agrawala, S. (2000). The Ethiopia food crisis–uses and limits of climate forecasts. Science 289, 1693–1694. doi: 10.1126/science.289.5485.1693
Buizer, J., Jacobs, K., and Cash, D. (2016). Making short-term climate forecasts useful: linking science and action. Proceed. Nat. Acad. Sci. 113, 4597–4602. doi: 10.1073/pnas.0900518107
Carberry, P. S., Hochman, Z., Hunt, J. R., Dalgliesh, N. P., McCown, R. L., Whish, J. P. M., et al. (2009). Re-inventing model-based decision support with Australian dryland farmers. 3. Relevance of APSIM to commercial crops. Crop Pasture Sci. 60, 1044–1056. doi: 10.1071/CP09052
Cash, D., and Buizer, J. (2005). Knowledge-action systems for seasonal to interannual climate forecasting: summary of a workshop report to the Roundtable on Science and Technology for Sustainability, Policy and Global Affairs. Washington, DC.
Cash, D. W., Borck, J. C., and Patt, A. G. (2006). Countering the loading-dock approach to linking science and decision making: Comparative analysis of El Niño/Southern Oscillation (ENSO) forecasting systems. Sci. Technol. Hum. Values 31, 465–494. doi: 10.1177/0162243906287547
Changnon, S. A. (2002). Impacts of the Midwestern drought forecasts of 2000. J. Appl. Meteorol. 41, 1042–1052.
Clarkson, G., Dorward, P., Poskitt, S., Stern, R. D., Nyirongo, D., Fara, K., et al. (2022). Stimulating small-scale farmer innovation and adaptation with Participatory Integrated Climate Services for Agriculture (PICSA): Lessons from successful implementation in Africa, Latin America, the Caribbean and South Asia. Clim. Serv. 26, 100298. doi: 10.1016/j.cliser.2022.100298
Cosmides, L., and Tooby, J. (1996). Are humans good intuitive statisticians after all? rethinking some conclusions from the literature on judgment under uncertainty. Cognition 58, 1–73. doi: 10.1016/0010-0277(95)00664-8
Coventry, W. L., and Dalgleish, L. I. (2014). Farmers' accuracy interpreting seasonal climate forecast probability. Int. J. Climatol. 34, 2097–2107. doi: 10.1002/joc.3825
Daly, M., and Dessai, S. (2018). Examining the goals of the regional climate outlook forums: what role for user engagement?, Weath. Clim. Soc. 10, 693–708. doi: 10.1175/WCAS-D-18-0015.1
Dinku, T., Faniriantsoa, R., Cousin, R., Khomyakov, I., Vadillo, A., Hansen, J. W., et al. (2022). ENACTS: advancing climate services across africa. Front. Clim. 3, 763.
Dinku, T., Hailemariam, K., Maidment, R., Tarnavsky, E., and Connor, S. (2014). Combined use of satellite estimates and rain gauge observations to generate high-quality historical rainfall time series over Ethiopia. Int. J. Climatol. 34, 2489–2504. doi: 10.1002/joc.3855
Dinku, T., Thomson, M. C., Cousin, R., del Corral, J., Ceccato, P., Hansen, J., et al. (2018). Enhancing National Climate Services (ENACTS) for development in Africa. Clim. Develop. 10, 664–672. doi: 10.1080/17565529.2017.1405784
Epstein, S. (1994). Integration of the cognitive and the psychodynamic unconscious. Am. Psychol. 49, 709–724. doi: 10.1037/0003-066X.49.8.709
Evans, J. S. B. T. (2003). In two minds: dual-process accounts of reasoning. Trends Cogn. Sci. 7, 454–459. doi: 10.1016/j.tics.2003.08.012
Evans, J. S. B. T. (2008). Dual-processing accounts of reasoning, judgment, and social cognition. Ann. Rev. Psychol. 59, 255–278. doi: 10.1146/annurev.psych.59.103006.093629
Evans, J. S. B. T., and Stanovich, K. E. (2013). Dual-process theories of higher cognition: advancing the debate. Perspectiv. Psychologic. Sci. 8, 223–241. doi: 10.1177/1745691612460685
Gigerenzer, G., and Hoffrage, U. (1995). How to improve Bayesian reasoning without instruction: frequency formats. Psychologic. Rev. 102, 684–704. doi: 10.1037/0033-295X.102.4.684
Gong, X., Barnston, A. G., and Ward, M. N. (2003). The effect of spatial aggregation on the skill of seasonal precipitation forecasts. J. Clim. 16, 3059–3071. doi: 10.1175/1520-0442(2003)016andlt;3059:TEOSAOandgt;2.0.CO;2
Hammer, G. L., Hansen, J. W., Phillips, J. G., Mjelde, J. W., Hill, H., Love, A., et al. (2001). Advances in application of climate prediction in agriculture. Agricult. Syst. 70, 515–553. doi: 10.1016/S0308-521X(01)00058-0
Hansen, J. (2015). Training workshop on communicating weather and climate information with farmers, Same, Tanzania, September 2013. CCAFS Workshop Report. Copenhagen: CGIAR Research Program on Climate Change, Agriculture and Food Security (CCAFS). Available online at: https://cgspace.cgiar.org/handle/10568/68883 (accessed March 28, 2022).
Hansen, J., Kagabo, D., Clarkson, G., Furlow, J., and Fiondella, F. (2021). Climate Services for Agriculture: Empowering Farmers to Manage Risk and Adapt to a Changing Climate in Rwanda (Final Project Report). Report. Wageningen: CGIAR Research Program on Climate Change, Agriculture and Food Security. Available online at: https://cgspace.cgiar.org/handle/10568/115240 (accessed June 14, 2022).
Hansen, J., Marx, S., and Weber, E. U. (2004). The role of climate perceptions, expectations, and forecasts in farmer decision making: The Argentine Pampas and South Florida, IRI Technical Report. 04–01. Palisades, New York.
Hansen, J. W., Baethgen, W., Osgood, D., Ceccato, P., and Ngugi, R. K. (2007). Innovations in climate risk management: protecting and building rural livelihoods in a variable and changing climate. J. Semi-Arid Tropic. Agricult. Res. 4, 1–38. doi: 10.7916/D8ZW1S4C
Hansen, J. W., Mason, S. J., Sun, L., and Tall, A. (2011). Review of seasonal climate forecasting for agriculture in sub-Saharan Africa. Experiment. Agricult. 47, 205–240. doi: 10.1017/S0014479710000876
Hansen, J. W., Vaughan, C., Kagabo, D. M., Dinku, T., Carr, E. R., Körner, J., et al. (2019). Climate services can support african farmers' context-specific adaptation needs at scale. Front. Sustain. Food Syst. 3, 21. doi: 10.3389/fsufs.2019.00021
Hartmann, H. C., Bales, R., and Sorooshian, S. (2002). Weather, climate, and hydrologic forecasting for the US Southwest: a survey. Clim. Res. 21, 239–258. doi: 10.3354/cr021239
Hewitt, C. D., Allis, E., Mason, S. J., Muth, M., Pulwarty, R., Shumake-Guillemot, J., et al. (2020). Making society climate resilient: international progress under the global framework for climate services. Bull. Am. Meteorologic. Soc. 101, E237–E252. doi: 10.1175/BAMS-D-18-0211.1
Hochman, Z., Rees, H., van Carberry, P. S., Hunt, J. R., McCown, R. L., Gartmann, A., et al. (2009). Re-inventing model-based decision support with Australian dryland farmers. 4. Yield Prophet® helps farmers monitor and manage crops in a variable climate. Crop Pasture Sci. 60, 1057–1070. doi: 10.1071/CP09020
Ingram, K. T., Roncoli, M. C., and Kirshen, P. H. (2002). Opportunities and constraints for farmers of west Africa to use seasonal precipitation forecasts with Burkina Faso as a case study. Agricult. Syst. 74, 331–349. doi: 10.1016/S0308-521X(02)00044-6
Jochec, K. G., Mjelde, J. W., Lee, A. C., and Conner, R. J. (2001). Use of seasonal climate forecasts in rangeland-based livestock operations in West Texas. J. Appl. Meteorol. 40, 1629–1639. doi: 10.1175/1520-0450(2001)040andlt;1629:UOSCFIandgt;2.0.CO;2
Johnson, S. J., Stockdale, T. N., Ferranti, L., Balmaseda, M. A., Molteni, F., Magnusson, L., et al. (2019). SEAS5: the new ECMWF seasonal forecast system. Geoscientific Model Develop. 12, 1087–1117. doi: 10.5194/gmd-12-1087-2019
Johnston, P. A., Archer, E. R. M., Vogel, C. H., Bezuidenhout, C. N., Tennant, W. J., and Kuschke, R. (2004). Review of seasonal forecasting in South Africa: producer to end-user. Climate Res. 28, 67–82. doi: 10.3354/cr028067
Kahneman, D. (2003). A perspective on judgment and choice: mapping bounded rationality. Am. Psychol. 58, 697–720. doi: 10.1037/0003-066X.58.9.697
Khan, M. Z. K., Sharma, A., and Mehrotra, R. (2017). Global seasonal precipitation forecasts using improved sea surface temperature predictions. J. Geophysic. Res. Atmosphere. 122, 4773–4785. doi: 10.1002/2016JD025953
Kipkogei, O., Siebert, A., Jordan, P., Cousin, R., Jama, A., and Hansen, J. W. (2018). Advancing the use of gridded, online climate information for risk management in the Horn of Africa. Report. Wageningen: CGIAR Research Program on Climate Change, Agriculture and Food Security, p. 22. Available online at: https://cgspace.cgiar.org/handle/10568/92093 (accessed July 5, 2022).
Kirchhoff, C. J., Esselman, R., and Brown, D. (2015). Boundary organizations to boundary chains: prospects for advancing climate science application. Clim. Risk Manage. 9, 20–29. doi: 10.1016/j.crm.2015.04.001
Kirtman, B. P., Min, D., Infanti, J. M., Kinter, J. L., Paolino, D. A., Zhang, Q., et al. (2014). The North American multimodel ensemble: phase-1 seasonal-to-interannual prediction; phase-2 toward developing intraseasonal prediction. Bull. Am. Meteorologic. Soc. 95, 585–601. doi: 10.1175/BAMS-D-12-00050.1
Klopper, E., Vogel, C. H., and Landman, W. A. (2006). Seasonal climate forecasts—Potential agricultural-risk management tools?. Climatic Change 76, 73–90. doi: 10.1007/s10584-005-9019-9
Leron, U., and Hazzan, O. (2006). The rationality debate: application of cognitive psychology to mathematics education. Educ. Stud. Mathematic. 62, 105–126. doi: 10.1007/s10649-006-4833-1
Lin, H., Merryfield, W. J., Muncaster, R., Smith, G. C., Markovic, M., Dupont, F., et al. (2020). The Canadian seasonal to interannual prediction system Version 2 (CanSIPSv2). Weather Forecast. 35, 1317–1343. doi: 10.1175/WAF-D-19-0259.1
Marx, S. M., Weber, E. U., Orlove, B. S., Leiserowitz, A., Krantz, D. H., Roncoli, C., et al. (2007). Communication and mental processes: experiential and analytic processing of uncertain climate information. Global Environ. Change, 17, 47–58. doi: 10.1016/j.gloenvcha.2006.10.004
Mason, S. J., Tippett, M. K., Song, L., and Muñoz, Á. G. (2019). Climate Predictability Tool version 16.2.3.
Min, Y.-M., Kryjov, V. N., and Park, C.-K. (2009). A probabilistic multimodel ensemble approach to seasonal prediction. Weather Forecast. 24, 812–828. doi: 10.1175/2008WAF2222140.1
Moore, A. D., Donnelly, J. R., and Freer, M. (1997). GRAZPLAN: Decision support systems for Australian grazing enterprises. III. Pasture growth and soil moisture submodels, and the GrassGro DSS. Agricult. Syst. 55, 535–582. doi: 10.1016/S0308-521X(97)00023-1
Moron, V., Robertson, A. W., and Ward, M. N. (2006). Seasonal predictability and spatial coherence of rainfall characteristics in the tropical setting of Senegal. Monthly Weather Rev. 134, 3248–3262. doi: 10.1175/MWR3252.1
National Academy of Sciences (2006). Completing the Forecast: Characterizing and Communicating Uncertainty for Better Decisions Using Weather and Climate Forecasts, National Research Council Committee on Estimating and Communicating Uncertainty in Weather and Climate Forecasts. Washington, DC: National Academies Press.
Ndiaye, O., Moussa, A. S., Seck, M., Zougmor,é, R. B., and Hansen, J. (2013). Communicating Seasonal Forecasts to Farmers in Kaffrine, Senegal for Better Agricultural Management. Available online at: https://cgspace.cgiar.org/handle/10568/27888 (accessed March 28, 2022).
Nicholls, N., and Kestin, T. (1998). Communicating climate. Climatic Change 40, 417–420. doi: 10.1023/A:1005483401062
Njiru, E. N., Ariithi, C. K. K., Rao, K. P. C., Mutinda, D., and Hansen, J. W. (2015). Farmer training workshops on understanding probabilistic seasonal forecasts: Wote, Kenya, 14-16 and 20-23 September 2011. CCAFS Workshop Report. Copenhagen: CGIAR Research Program on Climate Change, Agriculture and Food Security (CCAFS). Available online at: https://cgspace.cgiar.org/handle/10568/68859 (accessed March 28, 2022).
Nsengiyumva, G., Dinku, T., Cousin, R., Khomyakov, I., Vadillo, A., Faniriantsoa, R., et al. (2021). Transforming access to and use of climate information products derived from remote sensing and in situ observations. Remote Sens. 13, 4721. doi: 10.3390/rs13224721
O'Brien, K., Sygna, L., Otto Naess, L., Kingamkono, R., Hochobeb, B., Næss, L. O., et al. (2000). Is information enough? User responses to seasonal climate forecasts in Southern Africa. Oslo.
Orlove, B. S., and Tosteson, J. L. (1999). The Application of Seasonal to Interannual Climate Forecasts Based on El Niño—Southern Oscillation (ENSO) Events: Australia, Brazil, Ethiopia, Peru, and Zimbabwe. Available online at: https://escholarship.org/uc/item/4b88q4mj (accessed April 22, 2019).
Ouedraogo, I., Diouf, N. S., Ablouka, G., Zougmor,é, R. B., and Whitbread, A. (2021). Utility and triggers in uptake of agricultural weather and climate information services in Senegal, West Africa. Atmosphere 12, 1515. doi: 10.3390/atmos12111515
Patt, A., and Gwata, C. (2002). Effective seasonal climate forecast applications: Examining constraints for subsistence farmers in Zimbabwe. Global Environmental Change 12, 185–195. doi: 10.1016/S0959-3780(02)00013-4
Patt, A., Suarez, P., and Gwata, C. (2005). Effects of seasonal climate forecasts and participatory workshops among subsistence farmers in Zimbabwe. Proceed. Nat. Acad. Sci. USA 102, 12623–12628. doi: 10.1073/pnas.0506125102
Patt, A. G., Ogallo, L., and Hellmuth, M. (2007). Learning from 10 years of climate outlook forums in Africa. Science 318, 49–50. doi: 10.1126/science.1147909
Patt, A. G., and Schrag, D. P. (2003). Using specific language to describe risk and probability. Climatic Change 61, 17–30. doi: 10.1023/A:1026314523443
Paz, J. O., Fraisse, C. W., Hatch, L. U., Garcia y Garcia, A., Guerra, L. C., Uryasev, O., et al. (2007). Development of an ENSO-based irrigation decision support tool for peanut production in the southeastern US. Comput. Electron. Agricult. 55, 28–35. doi: 10.1016/j.compag.2006.11.003
Pennesi, K. (2007). Improving forecast communication: Linguistic and cultural considerations. Bull. Am. Meteorologic. Soc. 88, 1033–1044. doi: 10.1175/BAMS-88-7-1033
Phillips, J., and McIntyre, B. (2000). ENSO and interannual rainfall variability in Uganda: Implications for agricultural management. Int. J. Climatol. 20, 171–182.
Podestá, G., Letson, D., Messina, C., Royce, F., Ferreyra, R. A. A., Jones, J., et al. (2002). Use of ENSO-related climate information in agricultural decision making in Argentina: A pilot experience. Agricult. Syst. 74, 371–392. doi: 10.1016/S0308-521X(02)00046-X
Pons, D., Muñoz, Á. G., Meléndez, L. M., Chocooj, M., Gómez, R., Chourio, X., et al. (2021). A coffee yield next-generation forecast system for rain-fed plantations: the case of the Samalá Watershed in Guatemala. Weather and Forecast. 36, 2021–2038. doi: 10.1175/WAF-D-20-0133.1
Rao, K. P. C., Hansen, J. W., Njiru, E., Ndegwa, W., and Oyoo, A. (2015). Impacts of seasonal climate communication strategies on farm management and livelihoods in Wote, Kenya. Working Paper. Available online at: https://cgspace.cgiar.org/handle/10568/68832 (accessed December 14, 2020).
Robertson, A. W., Moron, V., and Swarinoto, Y. (2009). Seasonal predictability of daily rainfall statistics over Indramayu district, Indonesia. Int. J. Climatol. 29, 1449–1462. doi: 10.1002/joc.1816
Roncoli, C., Jost, C., Kirshen, P., Sanon, M., Ingram, K. T., Woodin, M., et al. (2008). From accessing to assessing forecasts: an end-to-end study of participatory climate forecast dissemination in Burkina Faso (West Africa). Climatic Change 92, 433. doi: 10.1007/s10584-008-9445-6
Stanovich, K. E., and West, R. F. (2000). Individual differences in reasoning: implications for the rationality debate?. Behav. Brain Sci. 23, 645–665. doi: 10.1017/S0140525X00003435
Stern, P. C., and Easterling, W. E. (1999). Making Climate Forecasts Matter. Washington, DC: National Academy Press. doi: 10.17226/6370
Suarez, P., and Patt, A. G. (2004). Cognition, caution, and credibility: the risks of climate forecast application. Risk Decis. Policy 9, 75–89. doi: 10.1080/14664530490429968
Thomson, M. C., Doblas-Reyes, F. J., Mason, S. J., Hagedorn, R., Connor, S. J., Phindela, T., et al. (2006). Malaria early warnings based on seasonal climate forecasts from multi-model ensembles. Nature 439, 576–579. doi: 10.1038/nature04503
Tversky, A., and Kahneman, D. (1974). Judgment under uncertainty: Heuristics and biases: Biases in judgments reveal some heuristics of thinking under uncertainty. Science 185, 1124–1131. doi: 10.1126/science.185.4157.112
Tversky, A., and Kahneman, D. (1985). “The framing of decisions and the psychology of choice,” in Behavioral Decision Making, ed. G. Wright (Boston, MA: Springer). 25–41. doi: 10.1007/978-1-4613-2391-4_2
Vaughan, L. F., Furlow, J., Higgins, W., Nierenberg, C., and Pulwarty, R. (2014). US Investments in International Climate Research and Applications: reflections on contributions to interdisciplinary climate science and services, development, and adaptation. Earth Perspectiv. 1, 23. doi: 10.1186/2194-6434-1-23
Vogel, C., and O'Brien, K. (2006). Who can eat information? examining the effectiveness of seasonal climate forecasts and regional climate-risk management strategies. Clim. Res. 33, 111–122. doi: 10.3354/cr033111
Weber, E. U., Shafir, S., and Blais, A.-R. (2004). Predicting risk sensitivity in humans and lower animals: risk as variance or coefficient of variation. Psychologic. Rev. 111, 430–445. doi: 10.1037/0033-295X.111.2.430
WMO (2016). Regional Climate Outlook Forums. Geneva: World Meteorological Organization, p. 52. Available online at: https://library.wmo.int/doc_num.php?explnum_id=3191 (accessed August 9, 2022).
WMO (2020). Guidance on Operational Practices for Objective Seasonal Forecasting. Geneva: World Meteorological Organization (WMO-No. 1246). Available online at: https://library.wmo.int/doc_num.php?explnum_id=11073 (accessed March 30, 2022).
Ziervogel, G. (2004). Targeting seasonal climate forecasts for integration into household level decisions: the case of smallholder farmers in Lesotho. Geographic. J. 170, 6–21. doi: 10.1111/j.0016-7398.2004.05002.x
Ziervogel, G., and Calder, R. (2003). Climate variability and rural livelihoods: assessing the impact of seasonal climate forecasts in Lesotho. Area 35, 403–417. doi: 10.1111/j.0004-0894.2003.00190.x
Keywords: seasonal forecast, farmers, national meteorological services, participatory, Maproom, ENACTS, East Africa
Citation: Hansen JW, Dinku T, Robertson AW, Cousin R, Trzaska S and Mason SJ (2022) Flexible forecast presentation overcomes longstanding obstacles to using probabilistic seasonal forecasts. Front. Clim. 4:908661. doi: 10.3389/fclim.2022.908661
Received: 30 March 2022; Accepted: 27 July 2022;
Published: 23 August 2022.
Edited by:
Shraddhanand Shukla, University of California, Santa Barbara, United StatesReviewed by:
Tamuka Magadzire, University of California, Santa Barbara, United StatesCopyright © 2022 Hansen, Dinku, Robertson, Cousin, Trzaska and Mason. This is an open-access article distributed under the terms of the Creative Commons Attribution License (CC BY). The use, distribution or reproduction in other forums is permitted, provided the original author(s) and the copyright owner(s) are credited and that the original publication in this journal is cited, in accordance with accepted academic practice. No use, distribution or reproduction is permitted which does not comply with these terms.
*Correspondence: James W. Hansen, amhhbnNlbkBpcmkuY29sdW1iaWEuZWR1
Disclaimer: All claims expressed in this article are solely those of the authors and do not necessarily represent those of their affiliated organizations, or those of the publisher, the editors and the reviewers. Any product that may be evaluated in this article or claim that may be made by its manufacturer is not guaranteed or endorsed by the publisher.
Research integrity at Frontiers
Learn more about the work of our research integrity team to safeguard the quality of each article we publish.