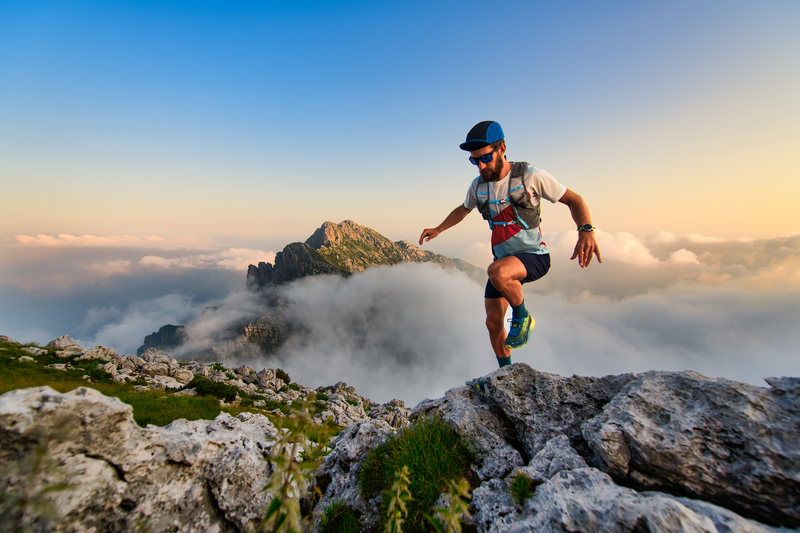
95% of researchers rate our articles as excellent or good
Learn more about the work of our research integrity team to safeguard the quality of each article we publish.
Find out more
ORIGINAL RESEARCH article
Front. Clim. , 23 May 2022
Sec. Climate Services
Volume 4 - 2022 | https://doi.org/10.3389/fclim.2022.852824
This article is part of the Research Topic Past, Present and Future Drought Risk and Impact Assessments View all 4 articles
Dry conditions occurred over São Paulo state (southeastern Brazil) from February to July 2018, causing the driest semester in 35 years. Socioeconomic impacts included a record number of fire spots, most adverse conditions to pollutant dispersion in 3 years and the winter's lowest water reservoirs stored volume in 17 years. This paper discusses climate drivers to the onset and persistence of the dry conditions, with special attention to the intraseasonal forcing. Barotropic atmospheric circulations forced by the intraseasonal Pacific-South America teleconnection pattern, embedded in the lower frequency setup of the Pacific Decadal Oscillation and the Atlantic Multidecadal Oscillation, were identified as main large-scale forcings to reduce precipitation. Drought evolution was modulated by other intraseasonal drivers such as the Madden Julian, Antarctic and 10–30 days Oscillations. A break in the 6-month dry condition, in March 2018, highlighted the important role of such oscillations in determining precipitation anomalies over SP. Results show that intraseasonal phenomena and their interactions control drought characteristics such as magnitude, persistence and spatial distribution within a setup determined by lower-frequency oscillations. The intraseasonal timescale seems to be key and must be considered for a complete description and understanding of the complex drought evolution process in São Paulo.
Drought is a natural phenomenon that occurs whenever water availability is significantly below normal levels over a long period and the existing demand cannot be met (Redmond, 2002). It is considered a natural climatological disaster as it becomes severe and extensive in highly populated areas (Cunha et al., 2019). Prolonged periods of drought reflect negatively over hydrologic reservoirs which may cause losses to agriculture and livestock, risks to human lives (e.g., waterborne diseases such as dehydration, contaminated and polluted water intake, etc.) and the environment (e.g., fires).
One of the most important and challenging features of the drought phenomenon is that its impacts escalate with time, leading to different categories (Wilhite and Glantz, 1985). Thus, several indices have been developed to identify drought and to assess their severity, such as the Palmer Drought Severity Index (Palmer, 1965) and the Standardized Precipitation Index (SPI) (McKee et al., 1993). The World Meteorological Organization (WMO) recommends the use of SPI for operative monitoring purposes (Hayes et al., 2011) because SPI computation is based solely on precipitation at different timescales (i.e., accumulated precipitation over given time spans) to monitor drought conditions affecting systems with different resilience times. Below average precipitation in the timescale of 1 month characterizes meteorological drought. If this condition persists for 2–3 months, soil moisture decreases significantly, leading to agricultural drought. Further consecutive months of meteorological drought will develop hydrological drought, where streamflows and reservoir levels are impacted by water shortage (WMO - World Meteorological Organization, 2012).
Over South America, documented main drivers of drought include teleconnection patterns established with the Pacific, Atlantic and Indian basins, atmospheric blockings and decreasing of precipitating synoptic systems (Otto et al., 2015; Seth et al., 2015; Nobre et al., 2016; Rodrigues and Woollings, 2017; Shimizu et al., 2017; Reboita et al., 2021). In the last two decades, dry conditions have been impacting Brazil (Drumond et al., 2021) with important episodes in the Amazon (Marengo et al., 2008; Coelho et al., 2012), Northeast and Southeast regions (Coelho et al., 2016a; Finke et al., 2020).
One of the most important economic regions of South America is the state of São Paulo (SP), which is located in the southeastern part of the continent between ~19.9◦S-25◦S and 50.5◦W-47.9◦W. It has a population of more than 46.6 million inhabitants (IBGE, 2021), nearly 22% of the total Brazilian population, and is a major business and agricultural Brazilian pole. The precipitation regime and water availability in this state is of crucial importance for population and economic activities.
SP is located in subtropical latitudes, so its climate is determined by both tropical and extratropical atmospheric forcing. The mean annual precipitation, distributed in well-defined rainy warm season and dry cold season, is driven by the south-american monsoon system (Zhou and Lau, 1998; Raia and Cavalcanti, 2008). During the year, the passage of cold fronts also influences rainfall, by favoring convergence zones that bring widespread rain during the summer and by causing localized storms in winter. Rainfall variability in SP is affected by the large scale low-frequency teleconnection patterns El Niño—Southern Oscillation (ENSO), Pacific Decadal Oscillation (PDO) and Atlantic Multidecadal Oscillation (AMO), with below (above)-normal precipitation tending to occur within the cold (warm) phases of ENSO/PDO and warm (cold) phase of AMO (Vásquez et al., 2018; Reboita et al., 2021). Embedded in these large-scale conditions, intraseasonal forcings are also important rainfall modulators in the region. The Pacific-South America (PSA) modes (Mo and Paegle, 2001) and the Antarctic Oscillation (AAO—Thompson and Wallace, 2000; Reboita et al., 2009) are two climate patterns that act on a wide range of timescales, including the intraseasonal range (Mo and Higgins, 1998; Pohl et al., 2010). Along with the Madden-Julian Oscillation (MJO—Madden and Julian, 1994) and higher-frequency oscillations (Gonzalez and Vera, 2014), these patterns are important regulators of SP rainfall. PSA's influence is very diverse, even depending on the convective trigger location, so it is not straightforward to define which signal of the pattern enhances or decreases precipitation. As for the other oscillations, rainfall over SP tends to decrease in the positive phase of AAO (Vasconcellos et al., 2019; Reboita et al., 2021) and in the phases 4, 5 and 7 of the MJO (Alvarez et al., 2016; Giovannettone et al., 2020). South Atlantic SST is also linked to SP rainfall in intraseasonal timescale, with the positive phase of the South Atlantic Dipole Index (Nnamchi et al., 2011) corresponding to dry conditions over SP in summer and autumn (Bombardi et al., 2014; Reboita et al., 2021).
In the first semester of 2018, monthly dry conditions over SP escalated to the driest autumn-winter in 35 years. Though climate patterns leading to SP dry conditions in summer are extensively documented (e.g., Seth et al., 2015; Bier et al., 2021; Abatan et al., 2022, among many others), studies focusing on autumn and winter are less numerous. Summer droughts' socioeconomic impacts are related to water reservoirs refilling, while impacts in autumn and winter are mainly related to fires and poor air quality.
The 2018 dry autumn-winter also contributed to the worsening of the extreme hydrological drought that persisted until 2021 (Naumann et al., 2021) and was the Central Brazil most intense case in decades (Getirana et al., 2021). There are numerous references discussing the conditions from 2019 onward (e.g., Grimm et al., 2020; Gomes et al., 2021; Marengo et al., 2021), but to the authors' best knowledge there are no studies addressing climate drivers of the dry conditions at the very onset of this important event, and few studies address the role of intraseasonal oscillations in drought. In this context, the objective of this study is to discuss climatological drivers and the development of rainfall deficit in SP during the first half of the year 2018, with a special focus on the intraseasonal timescale. Section Impacts of the 2018 Drought in SP illustrates socioeconomic impacts of such conditions; Section Data and Methodology shows the data and methodologies applied in this study; section Results presents the results of climate analyses, and Section Discussions and Conclusions brings some discussions and conclusions.
The Queimadas program from the Instituto Nacional de Pesquisas Espaciais (INPE, Brazilian National Institute of Space Research; INPE, 2021) showed a total of 3,019 active fire spots in the state of SP during 2018. Even though this number is lower than the average for the period 1999–2020, July/2018 registered the highest record since the beginning of their monitoring (84% above the climatological mean). Several articles from local newspapers pointed out fires in different municipalities in SP state, mainly depicting the number of fire spots, the spread of fires over populated areas, damages in forests—threatening several animal species—and crops (especially sugarcane fields) and the poor air quality due to the fire smoke, leading to low visibility and respiratory diseases (INPE, 2021).
Despite all damages registered by the media, only a few civil defense municipalities officially reported the disasters to the federal government; following the Sistema Integrado de Informações sobre Desastres (S2iD, Integrated Disaster Information System; S2ID, 2021), in August/2018 the municipality of Taciba registered the drought as a disaster, where the whole population were somehow affected and the private agricultural sector had an expressive monetary loss (S2ID, 2021). In addition, the municipality of Rio Claro also reported disasters related to fires in May, July and August. The environmental company from SP state (Companhia Ambiental do Estado de São Paulo; CETESB, 2019) described the period between May and September as the most adverse for dispersion of primary pollutants in the SP state and that the 2018 winter was considered the most adverse when compared with the last 3 years. In the Metropolitan Region of São Paulo, most of the automatic stations registered a slight increase (compared to 2017) in the mean concentrations of the fine particulate matter 2.5 (PM2.5), which is extremely harmful to the respiratory system (Arbex et al., 2012).
With dry conditions persisting from 2017 to 2018, SP and southeastern Brazil faced a severe water crisis. The water shortage in reservoirs built up from the previous hydrological drought event of 2013–2014.
Figure 1 shows the useful volume (percentage of the water volume between the minimum pool level and the full capacity of the reservoir) stored in the Southeast/Midwest water subsystem, that includes the state of SP, in the last two decades. The drought of 2000–2001 lowered the subsystem's level drastically (Jardini et al., 2002; Filgueiras and Silva, 2003), but the rainy period of 2005–2014 brought the reservoirs back to their normal state. Severe dry conditions returned in 2014, decreasing the stored volume to 15.8% by the end of that year. The clear contrast between reservoir volumes in the first and second decades of the 2000's may be in part linked to the interannual variability of the AAO; this discussion is presented in Section Discussions and Conclusions. Rigid water use control, gradual return of rain in 2016 and near average rainfall in 2017 were not sufficient to bring the useful volume of the subsystem back to the levels it had during most of the previous decade (Figure 1). Thus, it reached January/2018 with 31.1%, a very low volume for the peak of austral summer. After the dry rainy season, the level was at 34.2% in July/2018—the lowest level for July in 17 years (ONS, 2022).
Figure 1. Monthly stored useful volume in the Brazilian Southeast/Midwest subsystem between 2000 and 2021. In the lower panel, the time series for the year 2018 (indicated by the black bars in the upper panel). Source: ONS (2022).
Though reaching such low reservoir levels, the 2018 water scarcity was not as harmful to the general population as during the 2013–2015 drought event. It was because the state government created a program of rational water use and adapted the water system by connecting reservoirs (Braga and Kelman, 2020). These actions highlight the importance of governmental mitigation plans during water shortage periods.
Monthly mean precipitation for the period 1979–2020 was obtained from the Climate Research Unit (CRU) TS4.05 dataset (Harris et al., 2020) with horizontal resolution of 0.5◦ latitude and longitude. Air temperature and horizontal wind components at pressure levels, 500-hPa geopotential height, mean sea level pressure (MSLP) and outgoing longwave radiation (OLR) were obtained from NCEP/NCAR monthly datasets (Liebmann and Smith, 1996; Kanamitsu et al., 2002) with horizontal resolution of 2.5◦ x 2.5◦. Sea surface temperature (SST) anomalies were calculated using the 5th version of the NOAA Extended Reconstructed SST dataset (ERSSTv5; Huang et al., 2017).
For SPI and most of the anomalies computed throughout this work, the climatological reference period used is 1981–2010, as recommended by WMO - World Meteorological Organization (2017). Different reference periods (due to the use of available products or methodology needings) are informed and, when suitable, justified.
Regarding the climate indices, some of them are calculated using climatological periods that do not match the 1981–2010 period. It occurred because diverse datasets were used in this study, to acknowledge climate data publicly and readily available to the community. It is a way to show that these derived products can be assembled to help describe the climate state during drought events, as long as all datasets are reliable and all climatologies are drawn from approximately similar periods.
Figure 2 shows the SP state location in Brazil and South America, along with the area used for SPI calculations in this study, indicated by a red rectangle (53.2◦W-44◦W and 25.2◦S-19.8◦S). SPI was calculated from the areal average of mean monthly precipitation to represent droughts in a broad area, avoiding localized precipitation anomalies resulting from smaller scale processes. Correlation between SPI obtained from areal average and from weather stations is moderate to high, as shown by Gozzo et al. (2019), indicating that SPI areal average may be a good representation of the drought conditions over diverse locations in SP.
Figure 2. State of São Paulo (southeastern Brazil) shaded in orange and area over which the average precipitation and SPI were calculated (53.2◦W-44◦W and 25.2◦S-19.8◦S).
To compute SPI it is necessary to accumulate precipitation over monthly periods (1, 2, 3 months and so on) for a sufficiently long time (discussions on the “sufficient” length can be found in Wu et al., 2005). A statistical cumulative distribution function (CDF) is fitted to the time series of accumulated precipitation for each investigated monthly period. This CDF is then used to transform the accumulated precipitation values into standardized values (the SPI values) using a normal distribution having zero mean and unit variance by matching the percentiles of the two distributions. For this study, the CDF of the Gamma distribution and the 1981–2010 period are used for computing the SPI values for different timescales (SPI-1, SPI-6 and SPI-12 for accumulations over 1, 6, and 12 months, respectively). Further information on the SPI calculation, including equations, references and a more detailed description of the process' steps can be found in Lloyd-Hughes and Saunders (2002). For timescales larger than 1 month, the SPI month corresponds to the last month of the accumulated period (for example, the July SPI-6 represents the rainfall deviation for the February-March-April-May-June-July period).
SPI is calculated from the time series of mean average precipitation inside the red rectangle in Figure 3A, and from this value the dry condition is categorized in mild, moderate, severe and extreme, according to Table 1 from McKee et al. (1993) and WMO - World Meteorological Organization (2012). The intensity of the dry period is defined as the lowest SPI value registered during the event (Spinoni et al., 2014).
Figure 3. (A) Boxplot (box-and-whiskers plot) of areal average monthly precipitation (mm) constructed using climatological data in the period 1981–2010 and observations from CRU dataset for 2018; (B) SPI time series using 1981–2010 reference period, from 2012 to 2020 (timescales 1) and from 1979 to 2020 (timescales 6 and 12). Purple lines mark the year 2018 in the time series.
Blocking/cyclones/cold fronts frequency anomalies, intraseasonal/SST/stream function anomalies and wave activity flux (WAF) were calculated to discuss atmospheric patterns linked to the 2018 dry conditions identified via SPI.
Atmospheric blocking events are identified using the Tibaldi and Molteni (1990) index, adapted to the Southern Hemisphere (Tibaldi et al., 1994), based on the difference in 500-hPa geopotential height between two latitudes persisting for at least 3 days. Anomalies are presented as the percentage difference of blocked days from analyzed months with respect to the long term monthly climatology.
Extratropical cyclones anomalies (the difference in the number of cyclones by square degrees with respect to the climatology) are obtained using a tracking numerical scheme based on Murray and Simmonds (1991). Firstly, the cyclones are identified in the MSLP field from NCEP reanalysis with 6 h-frequency. Then, the climatology and anomalies are computed. Details on the cyclone density track product presented in this study can be found in Reboita and Marrafon (2021).
Cold fronts counting is performed using an objective criteria based on temperature decrease, MSLP increase and southward to northward shift of meridional wind. In each grid point, cold fronts are identified when these three changes occur simultaneously from 1 day to the next one (Pampuch and Ambrizzi, 2016).
The recursive Butterworth filter (Roberts and Roberts, 1978) with a cutoff period of 20–90 days is applied to daily OLR data in order to evaluate the intraseasonal signal of convection over SP. It is commonly employed in climate and hydro-climate analyses (Pichard et al., 2017; Afargan-Gerstman and Domeisen, 2020; Bhattacharya and Coats, 2020). To compute the anomalies, this filter needs to calculate its climatology using a period that contains the studied years—in our case, 2018—so it could not use the 1981–2010 period. Thus, the period 1981–2019 was used as the climate normal for our analyses.
Atmospheric teleconnections are assessed by stream function fields at upper and lower troposphere (200 and 850 hPa), calculated through a Poisson equation using the horizontal wind (Hawkins and Rosenthal, 1965). This quantity represents the rotational component of horizontal wind, with negative (positive) anomalies associated with anticyclonic (cyclonic) circulation anomalies in the Southern Hemisphere. With these maps, it is possible to identify patterns resembling the Pacific-South America—PSA Rossby wave trains (Mo and Paegle, 2001; O'Kane et al., 2017). The circulation patterns associated with these teleconnections are presented in Supplementary Figure 1, to facilitate interpretations in Section Results. To infer where stationary Rossby wave trains are emitted and/or absorbed, the upper-level wave activity flux (WAF) divergence field is calculated following Takaya and Nakamura (2001). Divergence/convergence of WAF vectors shows regions of Rossby wave emission/absorption. Table 2 shows a summary of atmospheric circulation analyses, with the used variables and climatological reference periods, and references to the computation methodology.
The relationship between dry conditions in SP and large-scale climate patterns was assessed through the use of climate indices. The Oceanic Niño Index (ONI), Southern Oscillation Index (SOI), AAO, PDO and AMO indices were obtained from the National Oceanic and Atmospheric Administration (NOAA), where further information about their computation can be found. The South Atlantic Subtropical Dipole Index (SASDI) was calculated following Morioka et al. (2011) and the PSA index was calculated as described in Souza and Reboita (2021). Table 3 shows the data and reference period used for the indices computation, and links to time series and detailed information on their methodology.
Characteristics of the 2018 drought over SP and its contextualization within the last decades are determined using precipitation data and the SPI time series on 1, 6, and 12 months timescales (denoted as SPI-1, SPI-6, SPI-12), suited to represent meteorological, agricultural and hydrological droughts, respectively (WMO - World Meteorological Organization, 2012).
The boxplot of monthly precipitation (Figure 3A) shows that accumulation for February 2018 (131.6 mm) was considerably lower than the climatological 1981–2010 mean (194.6 mm) and below the 25th percentile of the distribution. This also occurred in April, May and June 2018 (with accumulations of 42.4, 36.5, and 24.5 mm, respectively), and December 2018 (174.9 mm, and climatological mean of 219.8 mm). By the thresholds of SPI-1 (upper panel of Figure 3B), dry conditions were moderate in February, May and December (SPI-1 = −1.42, −1.46, and −1.33, respectively) and extreme in April (SPI-1 = −2.06). Given that the driest months were in summer (February and December) and mainly in autumn (April and May), this year had a quite different development in comparison to 2014, 2015, and 2017, when dry conditions were more severe during summer, with subsequent months near normal (Coelho et al., 2016b). In 2018, a not so dry rainy season was followed by a very dry autumn. This study will focus on these dry conditions from February to July, with the moderately wet break in March (Figure 3B). This break in dry conditions is an interesting feature of this 6-month drought, as it was not originated from just few sparse strong rain events, but from a month with persistent and widespread precipitation in central and eastern SP (as illustrated by Supplementary Figure 2)—or in other words, a month where climate drivers must have been somewhat different from the rest of the period.
We will not address conditions between November/2018-January/2019, since they were already discussed by do Nascimento Silva et al. (2020)—readers interested in this period are referred to this work.
Calculations of the spatial distribution of SPI-1 illustrate how the drought signal changes over the region along the period. February drought was more spatially localized, limited to the eastern portion of SP (Figure 4A). In March, central and eastern SP had above-average precipitation (SPI > 0), and the dry signal was restricted to the northwestern portion of the state (Figure 4B). Dry conditions intensified and spread spatially in April, when severe conditions occurred over the central and eastern SP and extreme drought was registered in western SP, along with northern Paraná, southern Mato Grosso do Sul and most of Paraguai (Figure 4C). Drought ceased to affect Bolivia and Paraguay in May, at the time when conditions also weakened in SP (Figure 4D). In June (Figure 4E), mild/moderate conditions prevailed over SP, while drought intensified over northern Argentina. In July, most of SP experienced mild conditions, with the westernmost area having a signal of extreme drought, linked to a drier signal affecting the western Brazil and eastern Bolivia (Figure 4F).
Figure 4. Spatial distribution of negative SPI-1 in (A) February, (B) March, (C) April, (D) May, (E) June, and (F) July, 2018. South America countries and SP political borders are drawn in the map, and the blue rectangle represents the area over which SPI is averaged.
Persistent monthly dry conditions shown in Figure 4 resulted in an extreme drought in the February-July period (July SPI-6 = −2.15), the most intense 6-month drought registered in SP since 1984.
The remarkable SP dry conditions registered in the first semester of 2018 arose from a combination of several climate forcings at different temporal and spatial scales, such as the teleconnections in Table 4 and intraseasonal oscillations. This section describes the intraseasonal and synoptic forcings with special attention to their differences from February (moderate dry) to March (mild wet) to April (extreme dry). These months illustrate how climate patterns act to rapidly vary drought intensity and spatial distribution.
Table 4. Southern Oscillation Index (SOI), South Atlantic Subtropical Dipole Index (SASDI) and Antarctic Oscillation (AAO), Pacific Decadal Oscillation (PDO), Atlantic Multidecadal Oscillation (AMO), Pacific-South America 1 and 2 (PSA1/PSA2) index values during 2018.
In February 2018, a negative phase of PSA2 was configured (Table 4), leading to an anomalous barotropic cyclonic circulation over Uruguay and Southern Brazil (Figure 5A). The ONI index was near −1, i.e., a La Niña event was occurring (Supplementary Table 1).
Figure 5. (A–F) 200 hPa (shaded) and 850 hPa (contours) streamfunction (10−7 m2 s−1) anomalies with respect to 1981–2010; (G–L) wind speed (shaded, m s−1) and wave activity flux (vectors, m2 s−2) at 200 hPa, for February to July 2018.
The positive SST anomaly on the western Pacific (Figure 6A), between Indonesia and Japan, strengthened the upper-level jet and produced a strong Rossby wave source, triggering a well-defined stationary wavetrain over North America and a weaker one toward South America (Figures 5A,G). Large scale disturbances can propagate from one hemisphere to another when a “westerly duct” is configured in the equatorial region (Webster and Holton, 1982), as was the case in that month (figure not shown). This Northern Hemisphere wavetrain propagating through the equatorial “window” (Li et al., 2019) toward South America intensified the PSA2-induced cyclonic anomaly near the Brazilian coast.
Figure 6. (A–F) Sea surface temperature (K) and (G–L) blocking frequency (% of days) anomalies with respect to the 1981–2010 climatology, for February to July 2018.
PSA2 turned neutral in March, while PSA1 reached the negative phase (Table 4). SST anomalies still show colder waters in the Equatorial Pacific (Figure 6B) in the decaying phase of a La Niña event (Supplementary Table 1). This condition triggers a strong barotropic wavetrain associated with negative PSA1, but diversion and absorption occurring in the Pacific basin (Figure 5H) disturbed the wave-like appearance in the stream function anomaly field (Figure 5B). In spite of that, the figure clearly shows a barotropic anticyclone acting over SP, the expected configuration for negative PSA1. This circulation may have played a role in maintaining drier conditions to the north of the state in March (Figure 4B), but it did not prevent above-average precipitation in central and eastern SP (Supplementary Figure 2a) that led to a break in dry conditions. The PSA1 negative phase persisted in April (Table 4), and in this month the wave pattern can be seen propagating through a well-defined WAF originating near 160◦W-30◦S (Figure 5I). Negative PSA1 is usually established during La Niña, when there is suppressed (enhanced) convection over equatorial Pacific (near 20◦S on eastern Pacific) at the meridian 160◦W, as shown by Mo and Higgins (1998) and Mo and Paegle (2001). This scenario was observed in April (Supplementary Table 1 and Figure 5C). By this month, the La Niña-induced PSA1 led to dry conditions over southeastern Brazil in agreement with other studies (Paegle and Mo, 2002; Gozzo et al., 2021; Santos et al., 2021). From May to July, PSA1 was neutral. Regarding the PSA2, its positive phase in April (Table 4) forced an anticyclonic anomaly over the South Atlantic that intensified dry conditions over SP. PSA2 was still positive in May (Figure 5D) and turned neutral in June and July (Figures 5E,F). From May to July, the WAF analysis indicate that PSA2 was not linked to an Equatorial Pacific teleconnection, being instead a manifestation of the internal atmospheric dynamics (Figures 5J,K,L). This is consistent with the weakening of SST anomalies in the ENSO region of the Pacific basin in these months (Figures 6D,E,F). Following the neutral PSA, dry conditions over SP weakened (Figure 4).
February was characterized by strong suppression of convection over SP in the 20–90 days timescale (Figure 7G), resulting in a broad positive OLR area in southern Brazil (Figure 7A). This condition is associated with the MJO in phase 7 during summer (Alvarez et al., 2016). In March, a totally different intraseasonal setup takes place: a strong convection-induced signal is present in South Brazil (including SP), and convective suppression occurs in Central Brazil and the Southeastern coast (Figures 7B,H). This may be linked to a Rossby wave-like 10–30 days period oscillation (Gonzalez and Vera, 2014). The convective signal observed in March 2018 bear striking resemblance to the EOF pattern of OLR presented by those authors, with a strong negative area over South Brazil near the boundaries with Argentina and Paraguay, and a positive area northwest-southeast oriented from Tocantins to Espirito Santo Brazilian states (Figures 1B, 7H and 7-day 0 from Gonzalez and Vera, 2014). In April, intraseasonal enhanced convection returns to Central Brazil (Figures 7C,I), associated with MJO phases 1 and 2 effects during autumn (Alvarez et al., 2016). Though MJO do not influence SP directly, subsidence in compensation of the Central Brazil convection was likely important to increase dry conditions over the state during the month.
Figure 7. (A–F) OLR (W m−2) and (G–L) 20–90 days filtered OLR (W m−2) anomalies with respect to the 1981–2010 (1981–2019) climatology for OLR (filtered OLR), for February to July 2018.
In May MJO did not impact rainfall over SP (with a more localized signal of suppressed convection, Figures 7D,J), but in June the Oscillation brought enhanced convection over the state (Figures 7E,K). Nevertheless, this situation did not bring abundant rainfall, as it occurred within the dry season when there is a small amount of moisture available in the region. In July, MJO in phases 4 and 5 forced a substantial area of suppressed convection over SP (Figure 7L). This condition strengthened dry conditions in western SP and in the regions along the borders of Paraguay and Bolivia and Brazil (Figure 4F). Anomalies of filtered OLR did not occur over SP in this month (Figure 7F), indicating that though the rainfall was below normal, cloudiness was near normal in the month.
The AAO index in February was positive (Table 4). This phase tends to decrease cyclone density in mid-latitudes near the eastern coast of Argentina (Reboita et al., 2015), and to decrease frontogenesis frequency between 50◦S and 30◦S over the South Atlantic (Reboita et al., 2009). Both effects were observed during the month (Figures 8A,G), reducing the number of cold and stationary fronts that, during summer, may couple with the monsoon circulation to cause widespread and persistent rainfall over SP.
Figure 8. (A–F) cyclones track density (10−3 cyclones degree lat−2) and (G–L) surface front (fronts month−1) anomalies with respect to the 1981–2010 climatology, for February to July 2018. Source: http://www.grec.iag.usp.br/data/index_USA.php
However, the effects of AAO on cyclones and fronts was absent during March, when the Oscillation went neutral (Table 4). With increased cyclogenetic (Figure 8B) and frontogenetic (Figure 8H) activities at the eastern coast of Argentina and southern Brazil, and a weaker South Atlantic Subtropical High (SASH) during the month (Supplementary Figure 4a), more transients displaced toward SP, favoring precipitation at eastern and central regions of the state. In April, AAO turned negative, maintaining above-average frontogenetic activity over Argentina (Figure 8C), but the negative PSA1-induced anticyclonic anomaly in subtropical South Atlantic displaced the strengthened SASH westward (Supplementary Figure 4b), preventing significant impacts of the fronts over SP. From May to July, AAO turned neutral. Cyclogenesis frequency was below normal in June (Figure 8E) and above normal in July (Figure 8F) in latitudes near the state of SP. Enhanced frontogenetic activity contributed to increased front passages in SP in May, June and July (Figures 8J,K,L), but they did not revert the dry conditions, since frontal systems are not expected to bring widespread precipitation during winter.
Over the South Atlantic, a negative South Atlantic Dipole (SAD) phase is configured in February, as indicated by the SASDI index value of −1.29 (Table 4). The cyclonic (anticyclonic) circulation anomaly over southeastern South America (South Atlantic) during negative SAD and neutral ENSO is present (Supplementary Figures 3a,g) but displaced to the east with respect to the composites of Bombardi et al. (2014). The difference in positioning may come from the fact that February/2018 was not ENSO neutral, but within a La Niña event. The cyclonic anomaly in this month, driven by the PSA2 and intensified by an interhemispheric wave propagation, displaced the South Atlantic Convergence Zone (SACZ) northward of its climatological position (Figure 7A), decreasing available moisture and precipitation in SP. This SACZ displacement is shown by Bier et al. (2021) to be an important feature of summer dry periods in SP.
In March, SASDI became even more negative (Table 4) and low-level circulation was similar to February (Supplementary Figures 3b,h). The persistence of similar SAD conditions in both months contrasted with their inverse precipitation anomalies over SP, suggesting that SAD was not relevant to cause a wetter March.
During April and May, warmer SST in the extratropical South Atlantic, due to the presence of the anticyclonic circulation anomaly of the PSA2 (Supplementary Figures 3c,d,i,j), maintained the negative SAD (SASDI = −1.14 and −0.77, respectively—Table 4), and the index went neutral in June and July, along with the decreased dry conditions.
In the equatorial Atlantic, a cold SST anomaly near 10◦N (Figure 6C) shifted the Intertropical Convergence Zone (ITCZ) to the south of its climatological position in April, near north and northeastern Brazil (Figure 7C). The strengthening of the ITCZ over this region tends to suppress convection in SP (Souza and Cavalcanti, 2009; Hohenegger and Jakob, 2020). Linear Pearson correlation of OLR anomaly between the ITCZ (during austral autumn, between 50◦W-10◦E e 5◦N-9◦N) and the SP state (red rectangle in Figure 1) regions for the period 1981–2010 resulted in a correlation coefficient of −0.33. Though low, it is statistically significant at the 95% level according to a t-test, reinforcing the hypothesis that a stronger ZCIT over northern Brazil may inhibit convection over SP. This forcing persisted in May, and in June and July, the correlation is still negative (although lower in magnitude) and statistically significant at the 5% level, but it may not have influenced the 2018 winter as the ITCZ did not lay anomalously southward during these months (Figures 8C–E).
In the synoptic timescale, atmospheric blocking persistence upstream of South America hampers the displacement of precipitating systems from the Pacific basin toward south and southeastern Brazil. Accordingly, the blocking frequency over eastern South Pacific was above average in February (Figure 6G). This may have been a dynamical response to the WAF convergence (Takaya and Nakamura, 2001) near the Chilean coast (Figure 5G).
Similar condition occurred in March (Figures 5H, 6H). This above-average blocking may have played a part in maintaining the dry signal in northern SP during the month, while other forcings acted to increase precipitation over the central state (Figure 4B). In April, a strong blocking over northern Argentina and southern Brazil (Figure 6I) canceled the effect of increased high-latitude frontogenesis over SP (Figure 8I). From May onward, the blocking effect on Pacific transients lost importance, since it was either weaker (Figure 6J) or positioned over central Brazil (Figures 6K,L).
The 2018 drought climate drivers at intraseasonal timescales, discussed in the previous subsection, are superimposed on an atmospheric circulation variability of lower frequency. This section briefly addresses the conditions of PDO and AMO, two low-frequency climate oscillations known to influence the SP rainfall climate. Their impact occurs by changing the magnitude of horizontal moisture transport from equatorial regions toward southeastern South America (e.g., Silva et al., 2011; Jones and Carvalho, 2018; Reboita et al., 2021). But more importantly, these teleconnections act to enhance downward vertical motion over SP.
Kayano et al. (2019) show that the aforementioned teleconnection also occurs via a PSA wavetrain: during autumn, cold PDO and warm AMO (CPDO/WAMO) conditions favor a wavetrain pattern that tends to intensify the SASH. PDO and AMO signals were relatively weak during 2018 (neither reached the 1 standard-deviation magnitude in the considered months—Table 4), but they were still in their cold and warm phases, respectively. Indeed, in April/2018, the barotropic anticyclonic center of the PSA wavetrain over South Atlantic strengthened the SASH (Figure 5C) and displaced it toward the continent (Supplementary Figure 4), reinforcing subsidence in the state of SP. Besides PSA, CPDO/WAMO also enhances downward motion over SP by modulating the Hadley circulation cell (Kayano et al., 2019). In autumn, it promotes a more vigorous upward motion in the ITCZ region and reinforces anomalous subsidence in the subtropics (as seen in April and May—Figures 8C,D).
During the first semester of 2018, SP experienced the driest 6-month period in more than three decades, occurring from February to July, with a break in March. As it developed in the autumn-winter period, its main societal impacts were the fires and poor air quality, besides maintaining low levels at water reservoirs. Climate drivers acting on the onset and development of the dry conditions were addressed in this study, through the SPI computation and reanalysis data.
Dry conditions in February/2018 were driven by the PSA2 pattern, the positive phase of AAO, a convection-suppressing phase of the MJO and increased atmospheric blockings over the Southeastern Pacific. Above-average rainfall was registered in March, in the central and south regions, due to intraseasonal induced convection and increased frequency of frontal systems (decurring from the intraseasonal AAO turning from positive to neutral). In April, dry conditions returned with greater intensity. The joint influence of PSA1/PSA2 patterns, SASH strengthened by the cold PDO and warm AMO phases, and compensating subsidence from the ITCZ (displaced southward of its climatological position), led to more widespread and intense dry conditions than in February. Dryness persisted until July, weakening along with the weakening of the main drivers.
Figure 9 shows the setup for dry conditions in February (Figure 9A) and April (Figure 9B). In both months, intraseasonal PSA and MJO signals were the most important drivers. A distinction between summer and autumn is seen in the role of SACZ in the former, and SASH and ITCZ in the latter.
Figure 9. Schematic representation of the main climate drivers for the (A) February 2018 and (B) April 2018 dry conditions.
The intraseasonal AAO also contributed to this scenario, enhancing the drought in February, during the positive phase, and weakening it in the following months. The precipitation pattern change between February and March was in part due to the AAO turning from positive to neutral, illustrating the importance of this oscillation to characterize monthly dry conditions over SP. But the role of AAO in SP drought seems not limited to its intraseasonal variability. The Oscillation also presents an interannual variability that, albeit much smaller than the intraseasonal one (Pohl and Camberlin, 2014), may contribute to longer wet and dry periods over SP. An example can be seen on annual mean water reservoir levels in the last two decades (Figure 10A), where the levels (thus precipitation volumes) were higher during the period 2003–2013, and lower during 2014–2020. The relationships between reservoir levels and ENSO, PDO and AMO were inconclusive, since the correlation coefficient for ENSO was very low and the phases of PDO and AMO did not match the observed precipitation behavior—PDO was mostly negative in the first decade of the period, and AMO remained positive throughout the whole period. However, correlation with the annual AAO was evident.
Figure 10. Annual mean of (A) stored useful volume in the Brazilian Southeast/Midwest subsystem between 2000 and 2021 (Source: ONS, 2022) and (B) Antarctic Oscillation index. The black (red) vertical lines indicate the period of 2003–2013 (2014–2021) and the horizontal dashed line in (B) indicates the zero value of the index.
At the interannual timescale, it is clear that the period 2003–2013 was characterized by more frequent and intense negative episodes of AAO, while in the drier period of 2014–2021, AAO was predominantly positive (Figure 10B). The link between the two time series can be inferred even at the annual timescale, for example from 2000 to 2003 and from 2008 to 2011—when the annual AAO is positive (negative), the useful volume of the reservoirs is lower (higher). The Spearman correlation coefficient of −0.47 from 2000 to 2021, significant at the 99% level, corroborates this relation. This succinct description points to an interesting possible link between the interannual AAO variability and precipitation over SP, and further studies are suggested.
By comparing the 2018 conditions with two recent important droughts in southeastern Brazil, in years 2001 and 2014, we conclude that dry conditions occurred for the most part due to anomalous upper- and lower-level tropospheric circulations within a semi-stationary Rossby wave train propagating from the Pacific basin toward South America. But in each of these 3 years, the resultant circulation anomalies over Brazil were different. In February 2018, a strong cyclonic barotropic circulation anomaly occurred southward of SP, while an anticyclonic anomaly was present over Southeast Brazil in summer/autumn 2001 (Drumond and Ambrizzi, 2005) and in Southwestern South Atlantic in 2014 (Coelho et al., 2016a). This difference in circulation patterns arose from the different source regions of the Rossby waves: in 2018 they originated over the Pacific in longitudes around 180◦W and over the Northern Hemisphere, but in 2014 their source was more to the east of the southern Pacific basin, between 150◦W and 110◦W (Coelho et al., 2016a), and in 2001 the wavetrain was triggered by persistent convection associated with anomalously warm SST in Indonesia and northern Australia, around 130◦E (Cavalcanti and Kousky, 2004; Drumond and Ambrizzi, 2005).
These comparisons highlight that though southeastern Brazil dry events are triggered by the PSA mechanism, differences in the wave may result in distinct impacts—while the 2001 and 2014 circulation patterns led to more dryness in Central and northern Southeast regions of Brazil (Drumond and Ambrizzi, 2005; Coelho et al., 2016b), the 2018 wave trains led to a stronger drought in the southern Southeast, including SP, Paraguay, northern Argentina and part of Southern Brazil (Figure 4).
Distinct configurations of the PSA pattern also impact the South Atlantic SST configuration. So, different Atlantic SST patterns near Southeastern Brazil are linked to different positioning of the drought conditions, as discussed by Bier et al. (2021). A semi-stationary barotropic anomalous anticyclone near the coast tends to locate the dryness more to the north during summer (over the states of Minas Gerais and Espirito Santo, and not so much over SP), and it is associated with positive SST anomalies in the South Atlantic, near SP, due to radiative warming and calm winds (Rodrigues et al., 2019). Dry conditions more to the south (i.e., with more impact over SP) are linked to a wide mid-tropospheric ridge and a northward displacement of SACZ, and an opposite condition in the Atlantic: a negative SST anomaly at the latitudes of SP (Pampuch et al., 2016; Bier et al., 2021). The PSA wavetrain in summer led in 2001 and 2014 to a SST pattern associated with drought impacts more to the north, while in 2018, the wavetrain forced an SST characteristic of dryness more to the south.
In short, the main conclusions of this work may be summarized in three points:
1) Intraseasonal oscillations (PSA, MJO, 10-30 days, AAO) are very important to the maintenance of dry conditions in SP, controlling drought characteristics such as magnitude, persistence and spatial distribution within a larger-scale low-frequency setup determined by other oscillations such as ENSO, PDO and AMO;
2) PSA modes are likely the main drivers of the drought in SP, with their ways of influence and affected regions depending on particular conditions of each period;
3) The AAO influence on precipitation over SP seems to extend from the intraseasonal to the interannual timescales.
A last comment should be made about the issue of climate indices. We applied here values made available by different research groups, and then climatological reference periods are not standardized. But since in the present study the indices were used only to support the characterization of the climate state during dry months (and not to a deep analysis of low-frequency variability modes), we consider that these periods' discrepancies should not affect our discussions. It could be considered a side result of this study that readily available indices may be put together to characterize climate events, even with slight differences in the climatological period. However, it may not always be the case, and we do not deny that this discrepancy could produce results with important differences in other occasions. Thus, we make a suggestion for the uniformization of the reference periods among the research groups in meteorological centers, if possible.
Results from this investigation contribute to improve knowledge about drought drivers and manifestation, since the 2018 dry conditions occurred in seasons not frequently addressed in the literature (autumn-winter) and the intraseasonal drivers were of utmost importance not only for the onset of dry conditions, but to their evolution. The comparison with climate drivers in previous recent dry events shows that though some atmospheric forcings are common to most droughts, it is difficult to establish a unique conceptual model of such a complex phenomenon. Thus, predictions of drought intensity, duration and impacted area remain a major scientific and practical challenge. Considering that drought frequency has been increasing in SP and tends to be even more common in a future climate change scenario (IPCC, 2021), a better scientific understanding of the phenomenon, efficient mitigation strategies for dry periods and the adoption of environmental protection policies are more than urgent.
Publicly available datasets were analyzed in this study. Data can be found in the following links: http://www.grec.iag.usp.br/data/index_USA.php; https://psl.noaa.gov/data/gridded/data.ncep.reanalysis2.html; https://www.ecmwf.int/en/forecasts/datasets/reanalysis-datasets/era5; https://meteorologia.unifei.edu.br/teleconexoes/; https://psl.noaa.gov/data/climateindices/list/.
LG and AD conceived this article. LP, NC, CK, RP, and PB worked on drought impacts. LG, AD, and MR developed the methodological design. LG, AD, LP, and RP performed the Precipitation Index analyses. AB, CBC, HP, AT, HG, MC, and PB provided maps and analyses of climatological drivers. LG wrote the text, with the aid of NC, AD, MC, CK, and MR. TA, CASC, and RR revised the text and made suggestions for improving the manuscript. All authors contributed to the article and approved the submitted version.
The authors declare that the research was conducted in the absence of any commercial or financial relationships that could be construed as a potential conflict of interest.
All claims expressed in this article are solely those of the authors and do not necessarily represent those of their affiliated organizations, or those of the publisher, the editors and the reviewers. Any product that may be evaluated in this article, or claim that may be made by its manufacturer, is not guaranteed or endorsed by the publisher.
Authors thank the reviewers and the meteorological centers that provided data applied in the study. Thanks for the financial support provided by FAPESP, FAPEMIG, CNPq, and CAPES. The authors acknowledge the Coordenação de Aperfeiçoamento de Pessoal de Nível Superior (CAPES) Finance Code 001. AD acknowledges the financial support from the Brazilian National Council for Scientific and Technological Development - CNPq (100186/2021-1). LP acknowledges the financial support from CNPq (426530/2018-7). TA was supported by the National Institute of Science and Technology for Climate Change Phase 2 under CNPq Grant 465501/2014-1, FAPESP Grants 2014/50848-9 and 2017/09659-6. TA also specifically acknowledges the support of CNPq under grants 304298/2014-0 and 301397/2019-8.
The Supplementary Material for this article can be found online at: https://www.frontiersin.org/articles/10.3389/fclim.2022.852824/full#supplementary-material
Abatan, A. A., Tett, S. F. B., Dong, B., Cunningham, C., Rudorff, C. M., Klingaman, N. P., et al. (2022). Drivers and physical processes of drought events over the state of São Paulo, Brazil. Clim Dyn. 1–15. doi: 10.1007/s00382-021-06091-2
Afargan-Gerstman, H., and Domeisen, D. I. V. (2020). Pacific modulation of the North Atlantic storm track response to sudden stratospheric warming events. Geophys. Res. Lett. 47, e2019GL085007. doi: 10.1029/2019GL085007
Alvarez, M. S., Vera, C. S., Kiladis, G. N., and Liebmann, B. (2016). Influence of the Madden Julian Oscillation on precipitation and surface air temperature in South America. Clim. Dyn. 46, 245–262. doi: 10.1007/s00382-015-2581-6
Arbex, M. A., Santos, U. D. P., Martins, L. C., Saldiva, P. H. N., Pereira, L. A. A., and Braga, A. L. F. (2012). Air pollution and the respiratory system. J. Bras. Pneumol. 38, 643–655. doi: 10.1590/S1806-37132012000500015
Bhattacharya, T., and Coats, S. (2020). Atlantic-Pacific gradients drive last millennium hydroclimate variability in Mesoamerica. Geophys. Res. Lett. 47, e2020GL088061. doi: 10.1029/2020GL088061
Bier, A. A., Ferraz, S. E. T., and Ambrizzi, T. (2021). Summer dry events on synoptic and intraseasonal timescales in the Southeast Region of Brazil. Atmósfera. doi: 10.20937/ATM.53025
Bombardi, R. J., Carvalho, L. M., Jones, C., and Reboita, M. S. (2014). Precipitation over eastern South America and the South Atlantic Sea surface temperature during neutral ENSO periods. Clim. Dyn. 42, 1553–1568. doi: 10.1007/s00382-013-1832-7
Braga, B., and Kelman, J. (2020). Facing the challenge of extreme climate: the case of Metropolitan São Paulo. Int. J. Water Resour. Dev. 36, 278–291. doi: 10.1080/07900627.2019.1698412
Cavalcanti, I. F. A., and Kousky, V. E. (2004). Drought in Brazil during Summer and Fall 2001 and associated atmospheric circulation features. Rev. Climanálise 2, 1–10. Available online at: http://climanalise.cptec.inpe.br/~rclimanl/revista/pdf/criseing.pdf
CETESB (2019). Qualidade do ar no estado de São Paulo 2018. Available online at: https://cetesb.sp.gov.br/ar/wp-content/uploads/sites/28/2019/07/Relat%C3%B3rio-de-Qualidade-do-Ar-2018.pdf (accessed December 16, 2021).
Coelho, C. A. S., Cardoso, D. H. F., and Firpo, M. A. F. (2016b). Precipitation diagnostics of an exceptionally dry event in São Paulo, Brazil. Theoret. Appl. Meteorol. 125, 769–784. doi: 10.1007/s00704-015-1540-9
Coelho, C. A. S., Cavalcanti, I. A. F., Costa, S. M. S., Freitas, S. R., Ito, E. R., Luz, G., et al. (2012). Climate diagnostics of three major drought events in the Amazon and illustrations of their seasonal precipitation predictions. Meteorol. Applic. 19, 237–255. doi: 10.1002/met.1324
Coelho, C. A. S., De Oliveira, C. P., Ambrizzi, T., Reboita, M. S., Carpenedo, C. B., Campos, J. L. P. S, et al. (2016a). The 2014 southeast Brazil austral summer drought: regional scale mechanisms and teleconnections. Clim. Dyn. 46, 11–12. doi: 10.1007/s00382-015-2800-1
Cunha, A. P., Marengo, J. A., Alvala, R. C., Deusdara-Leal, K. R., Cuartas, L. A., Seluchi, M., et al. (2019). Secas e seus Impactos no Brasil 2018. N.1, Janeiro 2019. CEMADEN, Sao Jose dos Campos, SP. 19p.
do Nascimento Silva, P., Capucin, B. C., Silva, B. A., and Reboita, M. S. (2020). Características anômalas do verão de 2019. Rev. Bras. Clim. 27, 612–634. doi: 10.5380/abclima.v27i0.69492
Drumond, A., and Ambrizzi, T. (2005). The role of SST on the South American atmospheric circulation during January, February and March 2001. Clim. Dyn. 24, 781–791. doi: 10.1007/s00382-004-0472-3
Drumond, A., Stojanovic, M., Nieto, R., Gimeno, L., Liberato, M. L., Pauliquevis, T., et al. (2021). Dry and wet climate periods over Eastern South America: identification and characterization through the SPEI index. Atmosphere 12, 155. doi: 10.3390/atmos12020155
Filgueiras, A., and Silva, T. M. V. (2003). Wind energy in Brazil - present and future. Renew. Sust. Energy Rev. 7, 439–451. doi: 10.1016/S1364-0321(03)00068-6
Finke, K., Jiménez-Esteve, B., Taschetto, A. S., Ummenhofer, C. C., Bumke, K., and Domeisen, D. I. V. (2020). Revisiting remote drivers of the 2014 drought in South-Eastern Brazil. Clim. Dyn. 55, 3197–3211. doi: 10.1007/s00382-020-05442-9
Getirana, A., Libonati, R., and Cataldi, M. (2021). Brazil is in water crisis—it needs a drought plan. Nature. 600, 218–220. doi: 10.1038/d41586-021-03625-w
Giovannettone, J., Paredes-Trejo, F., Barbosa, H., dos Santos, C. A., and Kumar, T. L. (2020). Characterization of links between hydro-climate indices and long-term precipitation in Brazil using correlation analysis. Int. J. Climatol. 40, 5527–5541. doi: 10.1002/joc.6533
Gomes, M. S., de Albuquerque Cavalcanti, I. F., and Müller, G. V. (2021). 2019/2020 drought impacts on South America and atmospheric and oceanic influences. Weather Clim. Extremes 100404. doi: 10.1016/j.wace.2021.100404
Gonzalez, P. L., and Vera, C. S. (2014). Summer precipitation variability over South America on long and short intraseasonal timescales. Clim. Dyn. 43, 1993–2007. doi: 10.1007/s00382-013-2023-2
Gozzo, L. F., Palma, D. S., Custodio, M. S., and Machado, J. P. (2019). Climatology and trend of severe drought events in the state of São Paulo, Brazil, during the 20th century. Atmosphere 10, 190. doi: 10.3390/atmos10040190
Gozzo, L. F., Palma, D. S., de Souza Custódio, M., and Drumond, A. (2021). Padrões climatológicos associados a eventos de seca no leste do estado de São Paulo. Rev. Bras. Clim. 28, 321–341. doi: 10.5380/rbclima.v28i0.76268
Grimm, A. M., Almeida, A. S., Beneti, C. A. A., and Leite, E. A. (2020). The combined effect of climate oscillations in producing extremes: the 2020 drought in southern Brazil. RBRH 25, 1–12. doi: 10.1590/2318-0331.252020200116
Harris, I., Osborn, T. J., Jones, P., and Lister, D. (2020). Version 4 of the CRU TS monthly high-resolution gridded multivariate climate dataset. Sci Data 7 109, 1–18. doi: 10.1038/s41597-020-0453-3
Hawkins, H. F., and Rosenthal, S. L. (1965). On the computation of stream functions from the wind field. Monthly Weather Review 93, 245–252.
Hayes, M., Svodoba, M., Wall, N., and Widhalm, M. (2011). The Lincoln declaration on drought indices: universal meteorological drought index recommended. Bull. Am. Meteorol. Soc. 92, 485–488. doi: 10.1175/2010BAMS3103.1
Hersbach, H., Bell, B., Berrisford, P., Hirahara, S., Horányi, A., Muñoz-Sabater, J., et al. (2020). The ERA5 global reanalysis. Quart. J. R. Meteorol. Soc. 146, 1999–2049. doi: 10.1002/qj.3803
Hohenegger, C., and Jakob, C. (2020). A relationship between ITCZ organization and subtropical humidity. Geophys. Res. Lett. 47, e2020GL088515. doi: 10.1029/2020GL088515
Huang, B., Thorne, P. W., Banzon, V. F., Boyer, T., Chepurin, G., Jay, H., et al. (2017). NOAA Extended Reconstructed Sea Surface Temperature (ERSST), Version 5. NOAA National Centers for Environmental Information. doi: 10.7289/V5T72FNM
IBGE (2021). Avaialble online at: https://www.ibge.gov.br/apps/populacao/projecao/index.html?utm_source=portalandutm_medium=popclockandutm_campaign=novo_popclock (accessed December 06, 2021).
INPE (2021). Queimadas. Avaialble online at: https://queimadas.dgi.inpe.br/queimadas/portal-static/estatisticas_estados/; https://queimadas.dgi.inpe.br/~rqueimadas/namidia/ (accessed November 01, 2021).
IPCC (2021). AR6 Climate Change 2021: The Physical Science Basis. Avaialble online at: https://www.ipcc.ch/report/ar6/wg1/#FullReport (accessed November 29, 2021).
Jardini, J. A., Ramos, D. S., Martini, J. S. C., Reis, L. B., and Tahan, C. M. A. (2002). Brazilian energy crisis. IEEE Power Eng. Rev. 22, 21–24. doi: 10.1109/MPER.2002.994845
Jones, C., and Carvalho, L. M. V. (2018). The influence of the Atlantic Multidecadal Oscillation on the eastern Andes low-level jet and precipitation in South America. npj Clim. Atmos. Sci. 1, 40. doi: 10.1038/s41612-018-0050-8
Kanamitsu, M., Ebisuzaki, W., Woollen, J., Yang, S. K., Hnilo, J. J., Fiorino, M., et al. (2002). Ncep–doe amip-ii reanalysis (r-2). Bull. Am. Met. Soc. 83, 1631–1644. doi: 10.1175/BAMS-83-11-1631
Kaplan A. Cane M. Kushnir Y. Clement A. Blumenthal M. and Rajagopalan, B. (1998). Analyses of global sea surface temperature 1856-1991. J. Geophys. Res. 103, 567–589. doi: 10.1029/97JC01736
Kayano, M. T., Andreoli, R. V., and Souza, R. A. F. D. (2019). El Niño–southern oscillation related teleconnections over South America under distinct Atlantic multidecadal oscillation and Pacific Interdecadal oscillation backgrounds: La Niña. Int. J. Climatol. 39, 1359–1372. doi: 10.1002/joc.5886
Li, Y., Feng, J., Li, J., and Hu, A. (2019). Equatorial windows and barriers for stationary Rossby wave propagation. J. Clim. 32, 6117–6135. doi: 10.1175/JCLI-D-18-0722.1
Liebmann, B., and Smith, C. A. (1996). Description of a complete (interpolated) outgoing longwave radiation dataset. Bull. Am. Meteorol. Soc. 77, 1275–1277.
Lloyd-Hughes, B., and Saunders, M. A. (2002). A drought climatology for Europe. Int. J. Climatol. 22, 1571–1592. doi: 10.1002/joc.846
Madden, R. A., and Julian, P. R. (1994). Observations of the 40–50-day tropical oscillation — a review. Mon. Weather Rev. 122, 814–837.
Marengo, J. A., Cunha, A. P., Cuartas, L. A., Deusdará Leal, K. R., Broedel, E., Seluchi, M. E., et al. (2021). Extreme drought in the Brazilian Pantanal in 2019–2020: characterization, causes, and impacts. Front. Water 3, 13. doi: 10.3389/frwa.2021.639204
Marengo, J. A., Nobre, C. A., Tomasella, J., Oyama, M. D., De Oliveira, G. S., De Oliveira, R., et al. (2008). The drought of Amazonia in 2005. J. Clim. 21, 495–516. doi: 10.1175/2007JCLI1600.1
McKee, T. B., Doesken, N. J., and Kleist, J. (1993). “The relationship of drought frequency and duration to time scales,” in Proceedings of the Eighth Conference on Applied Climatology (Boston, MA), 179–184. Available online at: https://www.droughtmanagement.info/literature/AMS_Relationship_Drought_Frequency_Duration_Time_Scales_1993.pdf (accessed November 09, 2021).
Mo, K. C., and Higgins, R. W. (1998). The Pacific–South American modes and tropical convection during the Southern Hemisphere winter. Monthly Weather Rev. 126, 1581–1596.
Mo, K. C., and Paegle, J. N. (2001). The Pacific–South American modes and their downstream effects. Int. J. Climatol. 21, 1211–1229. doi: 10.1002/joc.685
Morioka, Y., Tozuka, T., and Yamagata, T. (2011). On the growth and decay of the subtropical dipole mode in the South Atlantic. J. Clim. 24, 5538–5554. doi: 10.1175/2011JCLI4010.1
Murray, R. J., and Simmonds, I. (1991). A numerical scheme for tracking cyclone centres from digital data. Austral. Meteorol. Magazine 39, 155–166.
Naumann, G., Podesta, G., Marengo, J., Luterbacher, J., Bavera, D., Arias Muñoz, C., et al. (2021). The 2019-2021 extreme drought episode in La Plata Basin, EUR 30833 EN. Publications Office of the European Union, Luxembourg.
Nnamchi, H. C., Li, J., and Anyadike, R. N. C. (2011). Does a dipole mode really exist in the South Atlantic Ocean? J. Geophys. Res. Atmospheres 116, D15104.1–15. doi: 10.1029/2010JD015579
Nobre, C., Marengo, J., Seluchi, M., Cuartas, L., and Alves, L. (2016). Some characteristics and impacts of the drought and water crisis in Southeastern Brazil during 2014 and 2015. J. Water Resour. Protect. 8, 252–262. doi: 10.4236/jwarp.2016.82022
O'Kane, T. J., Monselesan, D. P., and Risbey, J. S. (2017). A multiscale reexamination of the Pacific–South American pattern. Monthly Weather Rev. 145, 379–402. doi: 10.1175/MWR-D-16-0291.1
ONS (2022). Histórico da Operação. Energia armazenada. Avaialble online at: http://www.ons.org.br/Paginas/resultados-da-operacao/historico-da-operacao/energia_armazenada.aspx. (accessed March 01, 2022).
Otto, F. E. L., Coelho, C. A. S., King, A., de Perez, E. C., Wada, Y., van Oldenborgh, G. J., et al. (2015). Factors other than climate change, main drivers of 2014/15 water shortage in south east Brazil. Bull. Am. Met. Soc. 96, 51–56. doi: 10.1175/BAMS-D-15-00120.1
Paegle, J. N., and Mo, K. C. (2002). Linkages between summer rainfall variability over South America and sea surface temperature anomalies. J. Clim. 15, 1389–1407. doi: 10.1175/1520-0442(2002)015<1389:LBSRVO>2.0.CO;2
Palmer, W.C (1965). Meteorological Drought, ed R. M. White. Washington, DC: U. S. Weather Bureau. Available online at: https://www.ncdc.noaa.gov/temp-and-precip/drought/docs/palmer.pdf (accessed November 09, 2021).
Pampuch, L. A., and Ambrizzi, T. (2016). Sistemas Frontais sobre a América do Sul Parte II: monitoramento Mensal em dados da Reanálise I do NCEP/NCAR. Ciênciae Natura 38, 105–110. doi: 10.5902/2179460X19811
Pampuch, L. A., Drumond, A., Gimeno, L., and Ambrizzi, T. (2016). Anomalous patterns of SST and moisture sources in the South Atlantic Ocean associated with dry events in southeastern Brazil. Int. J. Climatol. 36, 4913–4928. doi: 10.1002/joc.4679
Pichard, G.;, G., Arnaud-Fassetta, V., and Moron Roucaute, E. (2017). Hydroclimatology of the Lower Rhône Valley: historical flood reconstruction (AD 1300–2000) based on documentary and instrumental sources. Hydrol. Scie. J. 62, 11, 1772–1795. doi: 10.1080/02626667.2017.1349314
Pohl, B., and Camberlin, P. (2014). A typology for intraseasonal oscillations. Int. J. Climatol., 34, 430–445. doi: 10.1002/joc.3696
Pohl, B., Fauchereau, N., Reason, C. J. C., and Rouault, M. (2010). Relationships between the Antarctic Oscillation, the Madden–Julian oscillation, and ENSO, and consequences for rainfall analysis. J. Clim. 23, 238–254. doi: 10.1175/2009JCLI2443.1
Raia, A., and Cavalcanti, I. F. A. (2008). The life cycle of the South American monsoon system. J. Clim. 21, 6227–6246. doi: 10.1175/2008JCLI2249.1
Reboita, M. S., Ambrizzi, T., Crespo, N. M., Dutra, L. M. M., Ferreira, G. W. D. S., Rehbein, A., et al. (2021). Impacts of teleconnection patterns on South America climate. Ann. N. Y. Acad. Sci. 1504, 116–153. doi: 10.1111/nyas.14592
Reboita, M. S., Ambrizzi, T., and Rocha, R. P. D. (2009). Relationship between the southern annular mode and southern hemisphere atmospheric systems. Rev. Bras. de Met. 24, 48–55. doi: 10.1590/S0102-77862009000100005
Reboita, M. S., Da Rocha, R. P., Ambrizzi, T., and Gouveia, C. D. (2015). Trend and teleconnection patterns in the climatology of extratropical cyclones over the Southern Hemisphere. Clim. Dyn. 45, 1929–1944. doi: 10.1007/s00382-014-2447-3
Reboita, M. S., and Marrafon, V. H. (2021). Ciclones Extratropicais: o que são, climatologia e impactos no Brasil. Terræ Didatica 17, 1–13, e021032. doi: 10.20396/td.v17i00.8666028
Redmond, K. T (2002). The depiction of drought: a commentary. Bull. Amer. Meteor. Soc. 83, 1143–1147.doi: 10.1175/1520-0477(2002)083andlt;1143:TDODACandgt;2.3.CO;2
Roberts, J., and Roberts, T. D. (1978). Use of the Butterworth low-pass filter for oceanographic data. J. Geophys. Res. Oceans 83, 5510–5514. doi: 10.1029/JC083iC11p05510
Rodrigues, R. R., Taschetto, A. S., Gupta, A. S., and Foltz, G. R. (2019). Common cause for severe droughts in South America and marine heatwaves in the South Atlantic. Nat. Geosci. 12, 620–626. doi: 10.1038/s.41561-019-0393-8
Rodrigues, R. R., and Woollings, T. (2017). Impact of atmospheric blocking on South America in Austral summer. J. Clim. 30, 1821–1837 doi: 10.1175/JCLI-D-16-0493.1
S2ID (2021). Integrated Disaster Information System (S2ID). Avaialble online at: https://s2id.mi.gov.br/ (accessed October 17, 2021).
Santos, E. B., de Freitas, E. D., Rafee, S. A. A., Fujita, T., Rudke, A. P., Martins, L. D., et al. (2021). Spatio-temporal variability of wet and drought events in the Paraná River basin—Brazil and its association with the El Niño—Southern oscillation phenomenon. Int. J. Climatol. 41, 4879–4897. doi: 10.1002/joc.7104
Seth, A., Fernandes, K., and Camargo, S. J. (2015). Two summers of São Paulo drought: origins in the western tropical Pacific. Geophys. Res. Lett. 42, 10–816. doi: 10.1002/2015GL066314
Shimizu, M. H., Ambrizzi, T., and Liebmann, B. (2017). Extreme precipitation events and their relationship with ENSO and MJO phases over northern South America. Int. J. Climatol. 37, 2977–2989. doi: 10.1002/joc.4893
Silva, G. A. M., Drumond, A., and Ambrizzi, T. (2011). The impact of El Niño on South American summer climate during different phases of the Pacific Decadal Oscillation. Theor Appl Climatol. 106, 307–319. doi: 10.1007/s00704-011-0427-7
Souza, C., and Reboita, M. S. (2021). Ferramenta para o Monitoramento dos Padrões de Teleconexão na América do Sul. Terræ Didatica 17, 1–13. doi: 10.20396/td.v17i00.8663474
Souza, P., and Cavalcanti, I. F. A. (2009). Atmospheric centres of action associated with the Atlantic ITCZ position. Int. J. Climatol. 29, 2091–2105. doi: 10.1002/joc.1823
Spinoni, J., Naumann, G., Carrao, H., Barbosa, P., and Vogt, J. (2014). World drought frequency, duration, and severity for 1951–2010. Int. J. Climatol. 34, 2792–2804. doi: 10.1002/joc.3875
Takaya, K., and Nakamura, H. (2001). A formulation of a phase-independent wave-activity flux for stationary and migratory quasigeostrophic eddies on a zonally varying basic flow. J. Atmos. Sci. 58, 608–627. doi: 10.1175/1520-0469(2001)058andlt;0608:AFOAPIandgt;2.0.CO;2
Thompson, D. W., and Wallace, J. M. (2000). Annular modes in the extratropical circulation. Part I: month-to-month variability. J. Clim. 13, 1000–1016. doi: 10.1175/1520-0442(2000)013andlt;1000:AMITECandgt;2.0.CO;2
Tibaldi, S., and Molteni, F. (1990). On the operational predictability of blocking. Tellus A 42, 343–365. doi: 10.3402/tellusa.v42i3.11882
Tibaldi, S., Tosi, E., Navarra, A., and Pedulli, L. (1994). Northern and Southern Hemisphere variability of blocking frequency and predictability. Mon. Weather Rev. 122, 1971–2003. doi: 10.1175/1520-0493(1994)122andlt;1971:NASHSVandgt;2.0.CO;2
Vasconcellos, F. C., Pizzochero, R. M., and Cavalcanti, I. F. A. (2019). Month-to-month impacts of southern annular mode over south america climate. Anuário Do Instituto De Geociências - UFRJ. 42, 783–792. doi: 10.11137/2019_1_783_792
Vásquez, P. I. L., de Araujo, L. M. N., Molion, L. C. B., de Araujo Abdalad, M., Moreira, D. M., et al. (2018). Historical analysis of interannual rainfall variability and trends in southeastern Brazil based on observational and remotely sensed data. Clim. Dyn. 50, 801–824. doi: 10.1007/s00382-017-3642-9
Webster, P. J., and Holton, J. R. (1982). Cross-equatorial response to middle latitude forcing in a zonally varying basic state. J. Atmos. Sci. 39, 722–7332.
Wilhite, D. A., and Glantz, M. H. (1985). Understanding the drought phenomenon: the role of definitions. Water Int. 10, 111–120. doi: 10.1080/02508068508686328
WMO - World Meteorological Organization (2012). Standardized Precipitation Index User Guide (M. Svoboda, M. Hayes and D. Wood). (WMO-No. 1090). Geneva.
WMO - World Meteorological Organization (2017). WMO Guidelines on the Calculation of Climate Normals (WMO-No. 1203). Geneva.
Wu, H., Hayes, M. J., Wilhite, D. A., and Svoboda, M. D. (2005). The effect of the length of record on the standardized precipitation index calculation. Int. J. Climatol. 25, 505–520. doi: 10.1002/joc.1142
Keywords: drought, SPI, São Paulo, intraseasonal oscillations, teleconnection
Citation: Gozzo LF, Drumond A, Pampuch LA, Ambrizzi T, Crespo NM, Reboita MS, Bier AA, Carpenedo CB, Bueno PG, Pinheiro HR, Custodio MdS, Kuki CAC, Tomaziello ACN, Gomes HB, da Rocha RP, Coelho CAS and Pimentel RdM (2022) Intraseasonal Drivers of the 2018 Drought Over São Paulo, Brazil. Front. Clim. 4:852824. doi: 10.3389/fclim.2022.852824
Received: 11 January 2022; Accepted: 12 April 2022;
Published: 23 May 2022.
Edited by:
Michael C. Kruk, National Oceanic and Atmospheric Administration (NOAA), United StatesReviewed by:
María Cleofé Valverde, Federal University of ABC, BrazilCopyright © 2022 Gozzo, Drumond, Pampuch, Ambrizzi, Crespo, Reboita, Bier, Carpenedo, Bueno, Pinheiro, Custodio, Kuki, Tomaziello, Gomes, da Rocha, Coelho and Pimentel. This is an open-access article distributed under the terms of the Creative Commons Attribution License (CC BY). The use, distribution or reproduction in other forums is permitted, provided the original author(s) and the copyright owner(s) are credited and that the original publication in this journal is cited, in accordance with accepted academic practice. No use, distribution or reproduction is permitted which does not comply with these terms.
*Correspondence: Luiz Felippe Gozzo, bHVpei5nb3p6b0B1bmVzcC5icg==
Disclaimer: All claims expressed in this article are solely those of the authors and do not necessarily represent those of their affiliated organizations, or those of the publisher, the editors and the reviewers. Any product that may be evaluated in this article or claim that may be made by its manufacturer is not guaranteed or endorsed by the publisher.
Research integrity at Frontiers
Learn more about the work of our research integrity team to safeguard the quality of each article we publish.