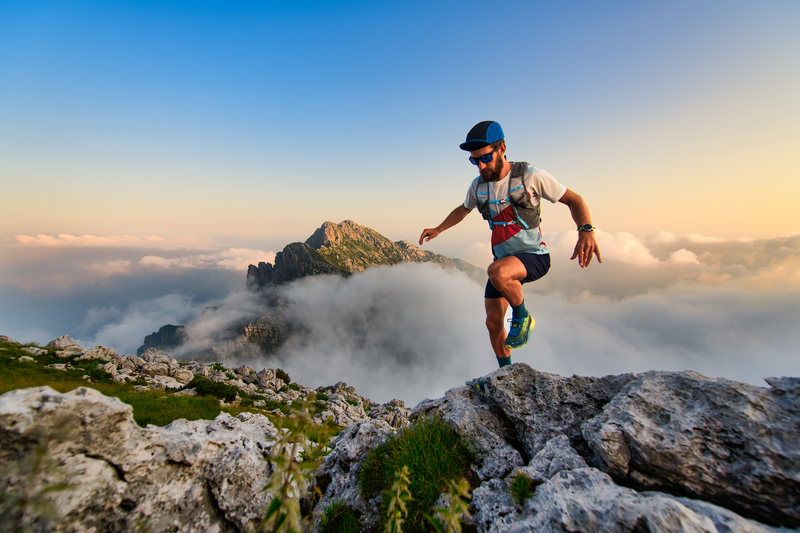
94% of researchers rate our articles as excellent or good
Learn more about the work of our research integrity team to safeguard the quality of each article we publish.
Find out more
POLICY AND PRACTICE REVIEWS article
Front. Clim. , 28 March 2022
Sec. Carbon Dioxide Removal
Volume 4 - 2022 | https://doi.org/10.3389/fclim.2022.841907
This article is part of the Research Topic Harmonizing Life Cycle Analysis (LCA) and Techno-Economic Analysis (TEA) guidelines: A Common Framework for consistent conduct and transparent reporting of carbon dioxide removal and CCU Technology Appraisal View all 7 articles
The mitigation of climate change requires research, development, and deployment of new technologies that are not only economically viable but also environmentally benign. Systematic and continuous technology assessment from early technology maturity onwards allows assessment practitioners to identify economic and environmental characteristics. With this information, decision-makers can focus time and resources on the most promising technologies. A broad toolset for technology assessment exists—stretching from the well-established life cycle assessment (LCA) methodology to more loosely defined techno-economic analysis (TEA) methods and the increasingly popular principles of technology maturity assessment such as the concept of technology readiness levels (TRL). However, current technology assessment practice faces various challenges at early stages, resulting in a potential mismatch of study results and stakeholders' needs and an escalation of assessment effort. In this practice review, we outline current challenges in the interplay of LCA, TEA, and TRL and present best practices for assessing early-stage climate change mitigation technologies in the field of carbon capture and utilization (CCU). The findings help practitioners systematically identify the TRL of a technology and adapt technology assessment methodologies accordingly. We highlight the methodological challenges for practitioners when adapting the goal and scope, identifying benchmark technologies, creating a comprehensive inventory, comparing early stage to commercial stage, ensuring clarity of recommendations for decision-making under high uncertainty, and streamlining conventional LCA and TEA assessment approaches and provide actionable recommendations. Overall, this work contributes to identifying promising technologies faster and more systematically, accelerating the development of new technologies for climate change mitigation and beyond.
The mitigation of climate change requires the development of a variety of innovative, cost-efficient, and environmentally benign technologies—among them a great variety of emerging technologies that employ carbon dioxide capture and utilization (CCU) (Hepburn et al., 2019). CCU is a concept where CO2 from emitters or air serves as a feedstock and is further converted to products and services. Emissions from CCU products and services may be used for another production iteration. Therefore, CCU can turn a conventional linear economy (“extract, convert, emit”) into a circular economy (“capture, convert, and capture again”) (see Figure 1). CCU offers three key advantages: economic value creation, greenhouse gas emissions reduction, and replacement of fossil or biogenic feedstocks. CCU technologies can produce chemicals, materials, and fuels, such as CCU methanol, CCU concrete, or CCU jet fuel. The potential of CCU is estimated to be several billion tons of CO2 use per year in the following decades; however, today, most CCU technologies are still under research and development and have not yet reached a commercial scale (Artz et al., 2018; Zimmermann, 2021).
Figure 1. A schematic overview of the carbon capture and utilization (CCU) cycle. Products and services (right) release carbon emissions during their lifecycle. Through CCU, these emissions can be captured and utilized in various conversion processes to provide products and services once again, closing the cycle. Reprinted with permission from Zimmermann et al. (2017).
Many decisions are being made during the research and development of clean technologies in general and CCU technologies in particular, impacting the technology's environmental footprint and economic performance. Conducting a systematic and consistent assessment helps researchers, engineers, managers, and funders to identify key performance indicators, compare technologies in development to incumbent technologies, understand the feasibility potential, and finally make and monitor decisions that reduce environmental impacts and improve performance; systematic and consistent assessments help to focus time and resources on the most promising pathways (Kleinekorte et al., 2020; Zimmermann et al., 2020). Such assessments provide strategic arguments for academic papers, project proposals, and reports and enable stage-gate evaluations for industrial technology development and deployment processes (Wender et al., 2014; Cremonese et al., 2020). Conducting technology assessments is especially crucial at the early stages of a technology when decisions tend to have substantial implications. These implications pass onto the subsequent development stages, and reversing them later in the process becomes very costly (Ruiz-Mercado et al., 2012).
A broad toolset for technology assessments exists, the most prominent being Life Cycle Assessment (LCA) and Techno-Economic Assessment (TEA). LCA evaluates potential environmental impacts throughout the technology life cycle from raw material extraction to final disposal (ISO, 2006a, Amd1: 2020; Rebitzer et al., 2004; ILCD1). TEA, in contrast, evaluates the technical and economic performance of a technology, typically focusing on the life cycle stages of production. The International Organization for Standardization (ISO) has standardized the LCA methodology. It consists of four phases: (1) The Goal and scope phase formulates the research question for the study and defines the necessary level of detail, reflecting the needs of decision-making, e.g., during R&D. The scope describes the conditions and assumptions under which the results of the study are valid (Zimmermann et al., 2020), e.g., by defining the investigated processes (system boundaries) and the basis on which processes are compared to each other (functional unit). (2) The inventory analysis phase collects or calculates mass and energy balances for all unit processes in scope. (3) The Life Cycle Impact Assessment (LCIA) phase “translates” the inventory data to environmental impacts by associating the flows from and to the environment (elementary flows) with specific characterization factors for each environmental impact category (e.g., climate change). (4) The interpretation phase ensures that the goal and scope of the study are met and evaluates the results to derive robust conclusions and potential recommendations. Finally, an assessment report presents the results of an LCA, clearly stating the goal and scope under which the results are valid. The described LCA phases can be applied to TEA as well (Zimmermann et al., 2020).
While LCA and TEA can provide critical insights early on in development, early-stage assessments suffer from (1) unknowns in product's performance in the specific application, (2) open questions in scale-up, (3) limitations in data availability, and (4) high data uncertainty compared to matured or existing commercial technologies (Hetherington et al., 2014). The results of early and later stage LCAs and TEAs can vary—studies of a technology at an early stage might conclude a different performance compared to studies of the same technology repeated at a later, more mature stage (Parvatker and Eckelman, 2019). Typically, more and more efficiency measures such as material recycling streams and heat integration are implemented during the development of a process technology. For example, a technology might use a different processing method (e.g., batch vs. continuous) at a laboratory-scale version compared to its more mature, commercial-scale version. Therefore, LCA and TEA can serve as tools to support decision-making dependent on the technology maturity (e.g., as screening tools to reduce the remaining technology options).
The most popular concept currently used to describe the maturity of a technology is the framework of “technology readiness levels” (TRLs). The TRL framework is a systematic and qualitative scaling method that evaluates the maturity of a technology based on nine levels of technology readiness. The levels start at TRL 1 “basic principles observed and reported” and end at TRL 9 “Actual system operated over the full range of expected mission conditions.” For a general TRL description and a TRL description for the chemical industry (see Table 1) (US Department of Energy, 2011b; European Commission, 2014b). Beyond academic literature, this framework is also quite prevalent in industrial technology development and deployment processes. Whether companies use it directly, use it with slight modifications, or have developed an in-house method, the core concept typically remains similar. Furthermore, the TRL concept is increasingly used by funding agencies facilitating technology screening and focus of funding within a specific range of TRL or maturity levels (European Association of Research Technology Organisations, 2014).
Table 1. Technology readiness levels—general and chemical industry TRL definitions (US Department of Energy, 2011a; Buchner et al., 2019).
It is also important to note that TRL identification does not guarantee that a technology meets expectations in an application; TRL is solely a measure of maturity. While the technology readiness levels might suggest that a technology's development state can be easily summarized and communicated in just one number, the identification of a TRL remains challenging as the description of TRL criteria are often generic, requiring individual interpretation and making an objective identification of TRL difficult (Buchner et al., 2019).
Thus, current technology assessment guidelines and practices face various open questions at early stages, increasing case-specific solutions, a lack of comparability between studies, the risk of missing key characteristics, and an escalation of assessment effort. This work outlines existing challenges, first in technology maturity assessment and second in early-stage LCA and TEA, and presents corresponding best practices and actionable recommendations. With this contribution, we aim to extend guidance for early-stage LCA and TEA practice and improve decision-making in the research and development of climate change mitigation technologies, in particular for the emerging field of CCU technologies. Thus, the present work continues an ongoing discussion on technology assessment for CCU initiated by the Global CO2 Initiative (Sick et al., 2020), based on findings of an expert workshop and best practices from academic, governmental, and industrial literature and practice.
Scientific papers, research and development (R&D) proposals, and management meetings use technology maturity as an indicator to provide the audience with a quick understanding of how far technology development has progressed. Besides communicating the development state to others, a sounder analysis and understanding of a technology's maturity also help practitioners choose suitable LCA and TEA methods, reducing effort and increasing the robustness of further technology assessment. Often, practitioners identify technology maturity by experience only, which can lead to an unintended subjective judgment, or perhaps in the worst case, even tailored to the intended result, as compared to a systematic, repeatable, and consistent assessment of the technology's state that is strongly preferred.
To ensure that a maturity assessment is as systematic, repeatable, and consistent as possible, practitioners can follow a three-step approach to identify a technology's readiness level, also valid for CCU (US Department of Energy, 2011a; European Commission, 2014a): In the first step, practitioners identify the elements of a technology. A technology can consist of multiple elements. Practitioners can define technology elements at a scale of their choice—from an individual piece of equipment (e.g., a CO2 absorber) to an overall plant (e.g., an integrated CCU fuels plant). In the second step, practitioners identify the TRL of each technology element individually, potentially encountering a range of questions (see paragraph below). In the third step, practitioners develop a technology maturation plan, listing actions to bring the technology elements to the desired TRL.
To facilitate technology maturity assessment in general and for CCU in particular, we have collected five critical questions for the identification of a TRL for a technology element and prepared a set of answers, summarizing earlier work (Buchner et al., 2019; Zimmermann et al., 2020; Zimmermann, 2021):
1) Where are the beginning and end of the TRL scale?
We see the beginning of the TRL scale when an idea for a technology is created, i.e., when researchers apply scientific and engineering principles to one or multiple processing steps (e.g., idea for a novel CO2 capture mechanism). Similarly, we see the end of the TRL scale when the technology is used in a commercial operation, meaning that a company, or any form of organization, operates a device or plant in the intended environment (e.g., first of a kind CO2 capture plant for commercial operation).
2) Are technology readiness levels phases or milestones?
While both perspectives exist in the literature, treating TRL as phases can lead to ambiguous descriptions, such as “early TRL Y” or “late TRL X,” which leaves room for interpretation. To improve clarity, we recommend adopting the milestones perspective and describing any technology as having “reached TRL Z.”
3) What type of scale should be used for TRL?
For TRL, we do not recommend using a ratio scale, as is typical for mass, and distance—but instead an ordinal scale, allowing the comparison of lower, higher, or equal ranks. Such an ordinal TRL scale allows identifying a minimum and maximum TRL but does not foresee calculating an average (arithmetic mean) TRL. Using an average TRL is discouraged as the TRL numbers represent categories, not intervals. For example, if a CCU fuels technology has four elements with CO2 capture at TRL 4, fuel synthesis step A at TRL 5, fuel synthesis step B at TRL 6, and fuel upgrading at TRL 7, the minimum TRL of the system is TRL 4. The first three questions are represented in Figure 2, where the knowledge about a technology (green) is sketched as increasing over time, while TRL is represented as ordinal-scale milestones. The milestones are reached when the knowledge has advanced to fulfill the requirements for the next TRL (black).
Figure 2. The schematic relationship between technology knowledge, TRL, assessment uncertainty, and remaining technology options. As a result of ongoing R&D, technology knowledge advances, and the next TRL or stage-gate is reached. TEA, and LCA support decision-making at each TRL or stage-gate, filtering out unpromising technology options, decreasing remaining technology options, and reducing assessment uncertainty.
4) What are the requirements to achieve a TRL, and how should they be measured?
The requirements for a TRL can vary from one technology domain to another. For example, the production scale of fuels is several orders of magnitude larger than the production scale of pharmaceuticals. We suggest using categories or indicators with pre-defined and descriptive values typical for the related maturity in a field and documenting their proof with tangible deliverables to make the assessment more objective. For example, for a CCU chemicals technology, practitioners could identify “kinetic data” as typical data for TRL 3, “pilot-plant scale” as typical production scale in TRL 7, or “production facility” as typical work location for TRL 9. The indicator values should be industry-specific to increase clarity—in the example, they are tailored to the chemical industry. Furthermore, practitioners should use multiple indicators such as reported data, production scale, work location, involved stakeholders to make TRL identification representative and objective.
5) How can practitioners derive the TRL of an overall system from TRLs of individual elements?
The recommended approach first evaluates the maturity of each technology element individually. It second derives the TRL of the overall system from the lowest TRL among its elements (e.g., the CCU fuel example from Question 3—the overall TRL equals the system's minimum, TRL 4). This process points out the elements with the lowest TRL, which become the bottleneck or limiting factors for the overall systems development process.
Following a maturity assessment of a CCU technology, practitioners typically continue conducting LCAs and TEAs. In the early stages, the knowledge about the future technology is meager, and many options for the final technology setup are not defined yet. For example, if researchers have not yet determined the catalyst concept for CO2 conversion, engineers have difficulty specifying reaction conditions and downstream operations. With advancing knowledge about a technology, TRL increases (see the previous chapter). Figure 2 depicts how unpromising technology options are filtered out at each stage-gate or TRL. As a result, remaining technology options decrease, and assessment uncertainty shrinks. The following steps of R&D can then focus on the remaining technology options.
As visualized in Figure 2, crucial data inputs, commonly available for conventional LCAs and TEAs at the commercial level, are missing or highly uncertain at the research level. Assessments at an early stage aim to support research and funding decisions. The involved stakeholders demand requirements different from assessments at the commercial level (e.g., the identification of bottlenecks for process improvements) (Kleinekorte et al., 2020). Both missing information and special requirements bring additional challenges when conducting LCAs and TEAs at early stages. In the following, we derive six significant challenges and collect best practices for CCU from discussions in current academic, industrial, and governmental literature and practice.
Commercial-stage assessments typically provide quantified insights to environmental impact and economic performance questions for a technology in a specific setting. However, studies for early-stage technologies often fail to answer these questions at the same level of detail, as many options for the technology remain yet to be defined, and large parts of the specific settings are still unknown.
When defining the goal and scope of early-stage studies, LCA and TEA practitioners must manage the stakeholders' expectations and consider this knowledge gap. To manage stakeholder expectations, practitioners need to adapt the goals for early-stage studies not to conventional TRL 9 or commercial operations but for the most pressing questions at the current early development stage. The available data determines whether and in what detail a question can be explored (e.g., what yields are required to compete with incumbent technologies costs or which process unit is the key contributor to environmental impact). For example, a stage-gate process, which reduces the number of technology options at each gate, requires the adaptation of goals to support decision-making at each stage. Typical stage-gate decisions for a chemical CCU technology might be selecting catalysts at TRL 3, choosing equipment types at TRL 4, or narrowing the range of operating conditions at TRL 5.
In addition, practitioners need to define functional units, reference flows, and system boundaries. Functional units serve as the relative basis for the comparison and shall reflect all functions of the CCU process. Reference flows measure the outputs from processes required to fulfill the function expressed by the functional unit (ISO, 2006a, Amd1: 2020). The system boundaries specify the processes within the assessment's scope, which are fulfilling the function (Zimmermann et al., 2020). The goal and scope should be defined iteratively between practitioners and stakeholders (ISO, 2006b; European Commission - Joint Research Center, 2010; Zimmermann et al., 2020; Sylvia et al., 2021, Amd2: 2020, 2006, Amd1: 2020). As best practice for this iterative process, Bergerson et al. provide a list of questions to discuss with stakeholders during goal and scope definition, helping manage expectations (Bergerson et al., 2020).
With increasing maturity and data availability, practitioners can also increase the goal's level of detail. While the screening of various reactions at low TRL requires a simplification of assumptions (e.g., only considering the stoichiometry of the primary reaction with complete conversion and perfect selectivity), the comparison of options for equipment types at medium TRL requires specific models for each type and expected operation conditions (Roh et al., 2020; Zimmermann et al., 2021). Thus, LCAs and TEAs can be conducted several times during the R&D process—for example, at each stage-gate, the goal and scope can be refined, asking more detailed questions and providing more specific evaluations at each stage. An assessment at the next higher TRL is a new iteration and can build on the previous results. For example, while the research question of an assessment of a CO2 capture technology at a low TRL may focus on the general feasibility and potential, it becomes more detailed at a mid TRL, such as specifying availability, costs, and environmental impacts of different CO2 sources, and even further detailed at a high TRL, for example, discussing the equipment alternatives for one specific capture process.
Typically, research questions aim to identify a technologies' relative benefits and drawbacks. This identification requires comparing the assessed technology to a reference or benchmark technology, and practitioners need to select the benchmark technology according to the function and market of the assessed technology to ensure comparability.
One complication for early-stage assessments arises when the potential benchmark technologies are also under development. It is paramount to have information on the final function and the related reference flow, markets, and customer segments of the assessed technology and the benchmark for selecting a future benchmark technology. However, such data is subject to change, not yet defined in detail, or missing entirely at early development stages. For example, CO2-based methanol can serve as a chemical intermediate, combustion fuel, or medium for electricity storage and release; potential benchmark technologies for fuels in the shipping market could be hydrogen, ammonia, methane, bio-oils, or also electric propulsion. Another complication for early-stage assessments arises from co-product use, as recycling and disposal strategies may deviate between the assessed technology and a benchmark. For example, producing e-crude from CO2 by Fischer-Tropsch synthesis provides several products, including fuel gas, fuel oils, and waxes, which can each replace products in different industries and thus potentially each require the selection of a separate benchmark.
At the start of each assessment iteration, identifying and updating the function and market is highly encouraged, as this information is one of the cornerstones for conducting an assessment (ISO, 2006a, Amd1: 2020); if not or only rudimentary available, we recommend setting up a joint identification process with the commissioners and stakeholders of the study (Sylvia et al., 2021). When selecting the benchmark, three considerations are key:
First, the benchmark should reflect the goal of the low TRL study. One typical goal at low TRLs is replacing an existing fossil technology with a CCU technology. In this case, the existing technology represents the so-called “marginal technology” and, as such, serves as the benchmark technology. However, besides the assessed CCU technology, there might be additional and better-performing technology alternatives. Ensuring that the assessment captures the competitiveness of the CCU technology to both existing and future technology alternatives, we recommend including benchmarks reflecting the marginal and future technology. Therefore, practitioners should encourage stakeholders to explore which technology options might exist and how they could be included in the comparison. For example, a methanol company plans to replace a conventional steam methane reforming process with a production based on CO2 from industrial flue gas. In this case, fossil methane production serves as benchmark technology, but other CCU or bio-based methanol production pathways could also be of interest for the comparison. Another typical goal at low TRLs is to identify the best-performing unit operations. The unit operations should be compared to each other, including all related impacts or costs. For example, an R&D team needs to decide between a separation via distillation vs. a separation via extraction. The selection of a unit process has implications for the overall process that the R&D team needs to consider—the extraction, for example, requires the separation and recycling of the solvent.
Second, practitioners must ensure that both processes fulfill the same function(s) as defined by the functional unit at each study iteration. If the products of the benchmark technology and the assessed CCU technology are chemically identical (e.g., CO2-based methanol and fossil methane-based methanol), they will serve the same function, and the system boundary can be limited to cradle-to-gate (Kleinekorte et al., 2020; Zimmermann et al., 2020). In this case, product quality (e.g., purity) must be the same for both technologies.
Third, the benchmark should reflect the technology expected to be replaced when the novel CCU technology enters the market. Skone et al. recently developed recommendations to enable a harmonized selection of benchmarks at low TRL and suggest using the best-in-class technology based on greenhouse gas emissions (Skone et al., 2019).
If practitioners identify multiple functions or markets, we suggest a prioritization based on stakeholder engagement, or conducting a series of assessments, showing the pros and cons for each function or market separately as described by Wender et al. (Wender et al., 2014). If practitioners identify multiple functional units, we suggest prioritizing or conducting a series of assessments, which helps evaluate the sensitivity toward those methodological choices and interpret the results (Mendoza Beltran et al., 2020). Last, while it may be apparent to LCA and TEA practitioners that the lacking information at early maturity limits the evaluation, this limitation needs to be communicated clearly to stakeholders, especially in the interpretation and discussion sections.
A central challenge when assessing early-stage technologies is that, in many cases, data necessary for creating an inventory or calculating impacts, costs, or revenues is missing. Reasons for missing data can be manifold. Typical examples are critical reaction and process data are not yet measured or modeled, technical performance has not yet been demonstrated in the specific function, environmental impact data are not yet characterized in standard LCA databases, or costs and revenue data are outside of budget reach. Practitioners can estimate missing inventory, impact, cost, and revenue data for all maturity stages to overcome the data gaps (Parvatker and Eckelman, 2019).
Each assessment iteration should start with a round of stakeholder engagement. Establishing, updating, and validating the block flow diagram during the stakeholder engagement is critical. Practitioners can use this information to estimate mass and energy balances data and build the inventory (Tsoy et al., 2020). At TRL 2, the reaction's stoichiometry and enthalpy, the theoretical foundation for any mass and energy balance, provide the first data points. At TRL 3, practitioners can enrich the mass and energy balance with available information such as yield and reaction conditions (Althaus et al., 2007; Meys et al., 2020; Roh et al., 2020; Zimmermann et al., 2021). At TRL 4 and higher, practitioners can estimate missing data points from expert interviews or common industry values, such as a standard purge rate or typical energy consumption of a specific separation process, as information about the planned unit operations becomes available (Piccinno et al., 2016).
Practitioners can use the calculated mass and energy flows for both LCA and TEA—chemical engineering textbooks provide typical values and heuristics (Perry et al., 1997; Towler and Sinnott, 2008). If found significant, practitioners can estimate missing data, employing heuristics, expert interviews, machine learning tools, common industry values, or values from similar markets (Zimmermann and Schomäcker, 2017; Roh et al., 2020; Zimmermann et al., 2020; Sayyed Ahmad et al., 2022).
Process simulation can be applied if sufficient information and technical expertise are available. Process simulation does require the specification of a process flow diagram, but this can also be hypothetical at early stages. However, practitioners must make many assumptions, for which they must involve subject matter experts. The R&D community used process simulation already for a large variety of CCU technologies, such as the individual conversions of CO2 to CO (van Bavel et al., 2020), methanol (Pérez-Fortes et al., 2016b; Blumberg et al., 2017), formic acid (Pérez-Fortes et al., 2016a), DME (Michailos et al., 2019; Ostovari et al., 2020) or carbonates (Ostovari et al., 2020) and the combination of individual conversion steps to value chains for chemicals (Kätelhön et al., 2019; Roh et al., 2019; Lee et al., 2021), and plastics (Meys et al., 2021).
During the impact assessment phase, characterization factors help to quantify environmental impacts. As CCU technologies might use or produce novel materials (e.g., CO2-based organic polymers, filling materials, or concrete), those materials might not be well-characterized. In case of lacking impact assessment methods or characterization factors for novel materials, practitioners can follow chapter 6.7.4 of the ILCD handbook (European Commission - Joint Research Center, 2010). First, practitioners should evaluate the relevance of missing characterization factors assuming a conservative value or realistic worst-case value (e.g., based on expert knowledge or scientific literature). Practitioners can disregard the missing characterization factor if applying a characterization factor to the related elementary flow does not lead to relevant changes in an impact category. Nevertheless, the ILCD handbook recommends reporting missing characterization factors.
However, if applying a characterization factor leads to relevant changes, the contribution of the elementary flow is significant. In this case, the ILCD handbook recommends finding a more accurate and precise value for the missing characterization factor (European Commission - Joint Research Center, 2010). Molecular structure-based impact prediction or machine learning models can provide estimates of characterization factors (Roy et al., 2015; Dearden, 2016). Nevertheless, deriving characterization factors at a high quality is unrealistic for conventional LCA project timeframe and budget, as this requires deep expertise in life cycle impact assessment (LCIA) and specialized knowledge and data in the relevant chemical and environmental science issues. In practice, practitioners, and stakeholders must accept the limitations arising from a missing characterization factor and report them and their implications in the interpretation section.
Last, practitioners need to clarify whether and how the assessment can address the research question if data is missing. If the missing data makes answering a research question impossible, stakeholders and practitioners need to adapt it to the available data or pause the study until research and development have generated the missing data.
Not only data availability is a central challenge, but also the representativeness of available data. Data measured or calculated at low TRLs does not likely represent the final process accurately, as changes of unit operations, equipment, and the inclusion of efficiency measures such as heat integration or material recycling are common steps in research and development. But how can practitioners compare early-stage technologies with commercial-stage technologies representatively?
The conventional approach for comparing across different TRLs entails forecasting a technology's characteristics at an early stage to a commercial stage and comparing the predicted features to the incumbent or benchmark technologies (Tsoy et al., 2020). This approach is further called a “full-scope” assessment.
A second possible approach for comparing representatively across different TRLs is taking the reverse perspective from forecasting: “backcasting.” With backcasting, we mean simplifying the characteristics of a commercial-stage incumbent or benchmark technology to data typically available at an early stage and then comparing them to the ones of the early-stage technology in focus. As this approach uses technology maturity as a scope, it is further called a “maturity-scope” assessment (Buchner et al., 2018; Zimmermann et al., 2021).
For maturity-scope assessments that backcast commercial-stage data to early stages, shortcut methods provide suitable toolsets (Roh et al., 2020; Tsoy et al., 2020; Zimmermann et al., 2021). Shortcut methods differ from conventional, “full-scope” methods taking the shortcut of limiting scopes and leaving out intricate details, allowing simple comparisons. Shortcut methods are versatile when data availability is limited, such as at low maturity, or for reducing assessment effort, such as when comparing many alternatives (Zimmermann et al., 2021). When using maturity-scope assessments, practitioners should first estimate the best-case performance to check whether environmental impact reduction or economic benefits are possible at all. Practitioners could then add scenarios estimating a realistic case evaluating the robustness of comparisons, and investigating if a unit process may have a relevant contribution to the overall process.
Practitioners can also combine the two described forecasting and backcasting approaches: the commercial-stage data is backcasted, and early-stage technology's data forecasted but only to the next maturity stage. This hybrid approach allows for an evaluation of the R&D process by providing information on the technology's maximum potential and room for improvement (Zimmermann et al., 2021). For example, the assessment of a CCU technology producing Dimethyl Carbonate finds an energy efficiency of 72% at TRL 2; after further development of that technology to TRL 4, a new assessment iteration finds an energy efficiency of 51% (Zimmermann et al., 2021). While a slight decrease of efficiency from including material and energy losses to the assessment scope is to be expected when moving from a theoretical concept to a preliminary process design, such substantial decrease shows that additional factors contribute to the efficiency losses, which the R&D team needs explore in a subsequent design iteration.
Assessments at early stages have broader probability distributions and larger error bars than assessments at late stages, making recommendations in assessments at early stages much less specific and clear (Mendoza Beltran et al., 2018). Uncertainty itself is propagated from data, models, and choices to LCA and TEA results and can therefore not be avoided; the key is to derive meaningful recommendations also under uncertainty. Wynne defined four types of uncertainty: “risk”—known system parameters and known probabilities, “uncertainty”—known system parameters and unknown probability distributions, “ignorance”—unknown system parameters and unknown probability distribution, and “indeterminacy”—future development is inherently undetermined (Wynne, 1992). At high maturity, information on inventories, impact categories, characterization factors, and costs are generally available; ignorance and indeterminacy are typically low. Practitioners can analyze and quantify risk and uncertainty using conventional approaches, such as Monte-Carlo simulations, focusing on the “known unknowns.”
At low maturity, conventional approaches reach their limits, as “unknown unknowns” largely contribute to uncertainty (van der Giesen et al., 2020). For example, the timeline until market entry at early stages is still long, and the future market conditions are unknown. To deal with ignorance and indeterminacy, practitioners can consider multiple plausible futures scenarios, and involve a panel of stakeholders with most likely opposing views (van der Giesen et al., 2020). Practitioners can limit the impacts of uncertainty and increase the clarity of recommendations already during the goal and scope definition: The goal and scope of the study could be limited to identify promising options or deselect unpromising options. This way, practitioners can derive meaningful recommendations based on relative comparisons or best-case assumptions, reducing the amount of data required (Ravikumar et al., 2021a). Heijungs provides a more detailed discussion on uncertainty assessment for comparative decision-making (Heijungs, 2021).
Practitioners can further increase the clarity of recommendations by analyzing the uncertainty factors' impact on the final results and their correlations with sensitivity analysis. Ideally, practitioners exclude uncertainty factors with minimal impact on results or correlated factors affecting the assessed technology's and benchmark's results in the same way (Heijungs et al., 2019). Available methods include an anticipatory decision-driven approach (Ravikumar et al., 2018), stochastic multi-attribute analysis, and global or moment-independent sensitivity analysis (Borgonovo et al., 2012; Cucurachi et al., 2016; Ravikumar et al., 2021b).
Lastly, practitioners need to check whether the uncertainty in the results allows answering the research questions or if the goal and scope need to be adapted or the study interrupted until the uncertainty is reduced by research and development.
Required resources and times may depend on the purpose of the technology assessment. Data quality requirements are not critical when stakeholders request ex-ante comparative LCAs and TEAs for screening technology pathways. Such a study could be completed relatively quickly at a lower level of detail based on the “backcasting” approach described in 3.4 or existing publicly available studies with some effort in terms of harmonization. In contrast, practitioners need to apply a robust and comprehensive methodology and high-quality data for prescriptive LCA and TEA, as they generally require some level of certification.
When LCA and TEA are required for funding or developing new policies, technology developers and agencies often wait until the end of the development stage to engage with assessment experts, with the expectation to confirm the advantages of economic and environmental impacts with a high level of confidence. Data inventory collection and curing are time and resource-intensive, and conducting comprehensive LCAs and TEAs is, therefore, a time-consuming process. At low TRLs, the availability of primary data is limited, and gathering data would take a long time. Since assessment time is critical to support decision-making in the R&D process, LCA and TEA practitioners should consider trade-offs between the level of detail and the required analysis time to provide support in a timely fashion.
Simplified LCA and TEA (“shortcut”) methods (also see 3.4) can provide an effective and efficient solution for technology assessment at low TRLs (Beemsterboer et al., 2020). Streamlined approaches can be helpful to screen technology options or assess low TRL technologies. For example, the “Life-cycle Screening of Emerging Technologies” method (LiSET) developed by Hung et al. provides an LCA method that allows practitioners to extend the assessment scope from screening systematically and streamlined LCA to full LCA (Hung et al., 2020; Moni et al., 2020). Finally, a close collaboration between LCA and TEA practitioners, the R&D team, and further stakeholders can reduce the number of assessment iterations and overall assessment effort.
Overall, we identified six challenges in current assessment guidelines and practice of early-stage technologies: meeting stakeholders' needs, defining functions and benchmarks, dealing with data availability, comparing across TRL, making recommendations under high uncertainty, and coping with limited assessment resources. Figure 3 provides an overview of these challenges and summarizes the best practices identified in this manuscript. Figure 3 highlights assessment attributes impacted by these best practices (“significance”). The significance attributes help define the value and rigor of an assessment and include:
• Alignment and utility: the usefulness of the assessment to those who commissioned and requested it or who may be impacted by it
• Context and comparison: the ability to clearly understand the function of a technology being evaluated and the technology's performance relative to benchmarks and other options
• Assumptions: the thorough reporting of all “assumed truths” required for the assessment and any methodologies deployed to reach those “assumed truths”
• Transparency and trust: the reproducibility of the assessment, the communication of the complete results (including unknowns), and the justification of methodologies employed.
Figure 3. Summary of the identified challenges, corresponding best practices, and significance in LCA and TEA practice for assessment of early-stage technologies.
The mitigation of climate change requires new technologies and systems that are economically feasible and environmentally benign. While their impact and performance need to be evaluated from the early stages, technology assessment faces various challenges. In this work, we have identified six challenges and collected best practices for technology readiness identification and early-stage LCA and TEA. Our discussion includes adapting the goal and scope definition, identifying benchmark technologies, creating comprehensive inventories, comparing different maturity stages, ensuring clarity of recommendations under high uncertainty, and streamlining conventional LCA and TEA.
The described challenges and best practices help LCA and TEA practitioners to improve their assessments in the four areas of alignment and utility, context and comparisons, assumptions, and transparency (see Figure 3). Further, the findings should help increase general awareness of the specific challenges and existing best practices for early-stage technology assessment—with a central element being the close interaction between assessment practitioners and their stakeholders. The results further enable practitioners to systematically identify the TRL of a technology and adapt technology assessment methodology accordingly. In addition, the findings inform the ongoing development and refinement of technology assessment guidelines.
Overall, this work contributes to identifying promising technologies faster and more systematically, accelerating the development of new technologies for climate change mitigation and beyond. Future work needs to derive practical approaches guiding practitioners in early-stage technology assessment, reducing the number of case-specific approaches by:
• Guiding hands-on study design, especially in goal and scope definition, systematically including the needs of stakeholders
• Facilitating collection and estimation of data by providing data sources and estimation methods relevant at each TRL
• Supporting practitioners in the interpretation of results, especially in deriving recommendations under high uncertainty
• Offering time-efficient project management approaches
As the development of technologies, the assessment of technologies is an ever-evolving area that requires constant adaption and refinement of its approaches. This continuous evolution lives from a frequent exchange of best practices, publication of studies that identify unpromising technologies, open sharing of broad sets of data, and lively discussions on assessment methodologies of the participating practitioner community.
AZ and TL: conceptualization. AZ, TL, and SM: investigation and writing—original draft. AZ, TL, SM, JS, FB, and AB: writing—review and editing. TL, AZ, and JS: visualization. JS, FB, and AB: supervision. All authors contributed to the article and approved the submitted version.
AZ: Netzero.Partners, Berlin. TL: EIT Climate-KIC (Co-funded by the European Union), Global CO2 Initiative. SM: The US Department of Energy National Energy Technology Laboratory (NETL) Contract Number DE-FE0025912. JS: The US Department of Energy National Renewable Energy Laboratory (NREL) Contract Number DE-AC36-08GO28308, and the US Department of Energy Office of Energy Efficiency and Renewable Energy Bioenergy Technologies Office (BETO). FB: National Research Council of Canada. This work was authored in part by the National Renewable Energy Laboratory, operated by Alliance for Sustainable Energy, LLC, for the US Department of Energy (DOE) under Contract No. DE-AC36-08GO28308. Funding provided by the US Department of Energy Office of Energy Efficiency and Renewable Energy Bioenergy Technologies Office. The US Government retains and the publisher, by accepting the article for publication, acknowledges that the US Government retains a nonexclusive, paid-up, irrevocable, worldwide license to publish or reproduce the published form of this work, or allow others to do so, for US Government purposes.
The views expressed in the article do not necessarily represent the views of the DOE or the US Government. This project was funded by the United States Department of Energy, National Energy Technology Laboratory, in part, through a site support contract. Neither the United States Government nor any agency thereof, nor any of their employees, nor the support contractor, nor any of their employees, makes any warranty, express or implied, or assumes any legal liability or responsibility for the accuracy, completeness, or usefulness of any information, apparatus, product, or process disclosed, or represents that its use would not infringe privately owned rights. Reference herein to any specific commercial product, process, or service by trade name, trademark, manufacturer, or otherwise does not necessarily constitute or imply its endorsement, recommendation, or favoring by the United States Government or any agency thereof. The views and opinions of authors expressed herein do not necessarily state or reflect those of the United States Government or any agency thereof.
AZ was employed by Netzero.Partners. AB was employed by Forschungszentrum Jülich GmbH.
The remaining authors declare that the research was conducted in the absence of any commercial or financial relationships that could be construed as a potential conflict of interest.
All claims expressed in this article are solely those of the authors and do not necessarily represent those of their affiliated organizations, or those of the publisher, the editors and the reviewers. Any product that may be evaluated in this article, or claim that may be made by its manufacturer, is not guaranteed or endorsed by the publisher.
The authors would like to acknowledge the support and feedback from the entire International CCU Assessment Harmonization Group, which includes representatives from the National Energy Technology Laboratory, the National Renewable Energy Laboratory, Argonne National Laboratory, the National Research Council of Canada, IASS Potsdam, TU Berlin, RWTH Aachen, The University of Sheffield, the Global CO2 Initiative at the University of Michigan, and EIT Climate-KIC. Volker Sick and Tim Skone offered expert oversight of this project along with all the other teams in the group.
CCU, Carbon capture and utilization (or use); LCA, Life cycle assessment; TEA, Techno-economic assessment; TRL, Technology readiness level; R&D, Research and development; NOAK, nth-of-a-kind; FOAK, First-of-a-kind.
1. ^ILCD. ILCD Handbook - General guide on LCA - Detailed guidance.
Althaus, H.-J., Hischier, R., Ossess, M., Primas, A., Jungbluth, N., and Chudacoff, M. (2007). Life cycle inventories of chemicals. Final report ecoinvent data No. 8 v2.0. Dübendorf, CH: EMPA, Swiss Centre for Life Cycle Inventories.
Artz, J., Müller, T. E., Thenert, K., Kleinekorte, J., Meys, R., Sternberg, A., et al. (2018). Sustainable conversion of carbon dioxide: an integrated review of catalysis and life cycle assessment. Chem. Rev. 118, 434–504. doi: 10.1021/acs.chemrev.7b00435
Beemsterboer, S., Baumann, H., and Wallbaum, H. (2020). Ways to get work done: a review and systematisation of simplification practices in the LCA literature. Int. J. Life Cycle Assess. 25, 2154–2168. doi: 10.1007/s11367-020-01821-w
Bergerson, J. A., Brandt, A., Cresko, J., Carbajales-Dale, M., MacLean, H. L., Matthews, H. S., et al. (2020). Life cycle assessment of emerging technologies: Evaluation techniques at different stages of market and technical maturity. J. Industr. Ecol. 24,11–25. doi: 10.1111/jiec.12954
Blumberg, T., Morosuk, T., and Tsatsaronis, G. (2017). Exergy-based evaluation of methanol production from natural gas with CO2 utilization. Energy 141, 2528–2539. doi: 10.1016/j.energy.2017.06.140
Borgonovo, E., Castaings, W., and Tarantola, S. (2012). Model emulation and moment-independent sensitivity analysis: An application to environmental modelling. Environ. Modell. Softw. 34:105–115. doi: 10.1016/j.envsoft.2011.06.006
Buchner, G. A., Stepputat, K. J., Zimmermann, A. W., and Schomäcker, R. (2019). Specifying technology readiness levels for the chemical industry. Ind. Eng. Chem. Res. 58, 6957–6969. doi: 10.1021/acs.iecr.8b05693
Buchner, G. A., Zimmermann, A. W., Hohgräve, A. E., and Schomäcker, R. (2018). Techno-economic assessment framework for the chemical industry—based on technology readiness levels. Ind. Eng. Chem. Res. 57, 8502–8517. doi: 10.1021/acs.iecr.8b01248
Cremonese, L., Olfe-Kräutlein, B., Strunge, T., Naims, H., Zimmermann, A., Langhorst, T., et al. (2020). Making sense of techno-economic assessment and life cycle assessment studies for CO2 utilization: a guide on how to commission, understand, and derive decisions from TEA and LCA studies. doi: 10.3998/2027.42/156039
Cucurachi, S., Borgonovo, E., and Heijungs, R. (2016). A protocol for the global sensitivity analysis of impact assessment models in life cycle assessment. Risk Analysis 36, 357–377. doi: 10.1111/risa.12443
Dearden, J. C. (2016). The history and development of quantitative structure-activity relationships (QSARs). Int. J. Quant. Struct. Prop. Relatsh. 1, 1–44. doi: 10.4018/IJQSPR.2016010101
European Association of Research Technology Organisations (2014). The TRL Scale as a Research and Innovation Policy Tool, EARTO Recommendations. Available online at: http://www.earto.eu/fileadmin/content/03_Publications/The_TRL_Scale_as_a_R_I_Policy_Tool_-_EARTO_Recommendations_-_Final.pdf.
European Commission - Joint Research Center (2010). ILCD Handbook - General guide on LCA - Detailed guidance. Publications office, Luxembourg: European Commission - Joint Research Center.
European Commission (2014a). HORIZON (2020). WORK PROGRAMME (2014). – (2015). 19. General Annexes Revised. Available online at: http://ec.europa.eu/research/participants/data/ref/h2020/wp/2014_2015/annexes/h2020-wp1415-annex-ga_en.pdf.
European Commission (2014b). HORIZON (2020). WORK PROGRAMME (2014). – (2015). 19. General Annexes Revised. Available online at: http://ec.europa.eu/research/participants/data/ref/h2020/wp/2014_2015/annexes/h2020-wp1415-annex-ga_en.pdf.
Heijungs, R. (2021). Selecting the best product alternative in a sea of uncertainty. Int. J. Life Cycle Assess 26, 616–632. doi: 10.1007/s11367-020-01851-4
Heijungs, R., Guinée, J. B., Mendoza Beltrán, A., Henriksson, P. J. G., and Groen, E. (2019). Everything is relative and nothing is certain. Toward a theory and practice of comparative probabilistic LCA. Int. J. Life Cycle Assess 24, 1573–1579. doi: 10.1007/s11367-019-01666-y
Hepburn, C., Adlen, E., Beddington, J., Carter, E. A., Fuss, S., Mac Dowell, N., et al. (2019). The technological and economic prospects for CO2 utilization and removal. Nature 575, 87–97. doi: 10.1038/s41586-019-1681-6
Hetherington, A. C., Borrion, A. L., Griffiths, O. G., and McManus, M. C. (2014). Use of LCA as a development tool within early research: challenges and issues across different sectors. Int. J. Life Cycle Assess 19, 130–143. doi: 10.1007/s11367-013-0627-8
Hung, C. R., Ellingsen, L. A.-W., and Majeau-Bettez, G. (2020). LiSET: a framework for early-stage life cycle screening of emerging technologies. J. Industr. Ecol. 24, 26–37. doi: 10.1111/jiec
ISO (2006a). Amd1:2020. Environmental Management – Life Cycle Assessment – Principles and Framework 13.020.10 Environmental Management 13.020.60 Product Life-Cycles: International Organization for Standardization. Geneva.
ISO (2006b). Amd2:2020. Environmental Management – Life Cycle Assessment – Requirements and Guidelines 13.020.10 Environmental Management 13.020.60 Product Life-Cycles: International Organization for Standardization. Geneva.
Kätelhön, A., Meys, R., Deutz, S., Suh, S., and Bardow, A. (2019). Climate change mitigation potential of carbon capture and utilization in the chemical industry. Proc. Natl. Acad. Sci. U.S.A. 116, 11187–11194. doi: 10.1073/pnas.1821029116
Kleinekorte, J., Fleitmann, L., Bachmann, M., Kätelhön, A., Barbosa-Póvoa, A., Assen, N., et al. (2020). Life cycle assessment for the design of chemical processes, products, and supply chains. Annual Rev. Chem. Biomol. Eng. 11, 203–233. doi: 10.1146/annurev-chembioeng-011520-075844
Lee, C.-T., Tsai, C.-C., Wu, P.-J., Yu, B.-Y., and Lin, S.-T. (2021). Screening of CO2 utilization routes from process simulation: design, optimization, environmental and techno-economic analysis. J. CO2 Utilization 53:101722. doi: 10.1016/j.jcou.2021.101722
Mendoza Beltran, A., Cox, B., Mutel, C., Vuuren, D. P., Font Vivanco, D., Deetman, S., et al. (2020). When the background matters: using scenarios from integrated assessment models in prospective life cycle assessment. J. Industr. Ecol. 24, 64–79. doi: 10.1111/jiec.12825
Mendoza Beltran, A., Prado, V., Font Vivanco, D., Henriksson, P. J. G., Guinée, J. B., and Heijungs, R. (2018). Quantified uncertainties in comparative life cycle assessment: what can be concluded? Environ. Sci. Technol. 52, 2152–2161. doi: 10.1021/acs.est.7b06365
Meys, R., Frick, F., Westhues, S., Sternberg, A., Klankermayer, J., and Bardow, A. (2020). Towards a circular economy for plastic packaging wastes – the environmental potential of chemical recycling. Resources Conserv. Recycling 162:105010. doi: 10.1016/j.resconrec.2020.105010
Meys, R., Kätelhön, A., Bachmann, M., Winter, B., Zibunas, C., Suh, S., et al. (2021). Achieving net-zero greenhouse gas emission plastics by a circular carbon economy. Science 374, 71–76. doi: 10.1126/science.abg9853
Michailos, S., McCord, S., Sick, V., Stokes, G., and Styring, P. (2019). Dimethyl ether synthesis via captured CO2 hydrogenation within the power to liquids concept: a techno-economic assessment. Energy Conversion Manage. 184, 262–276. doi: 10.1016/j.enconman.2019.01.046
Moni, S. M., Mahmud, R., High, K., and Carbajales-Dale, M. (2020). Life cycle assessment of emerging technologies: a review. J. Industr. Ecol. 24, 52–63. doi: 10.1111/jiec.12965
Ostovari, H., Sternberg, A., and Bardow, A. (2020). Rock ‘n' use of CO2: carbon footprint of carbon capture and utilization by mineralization. Sustain. Energy Fuels 4, 4482–4496. doi: 10.1039/D0SE00190B
Parvatker, A. G., and Eckelman, M. J. (2019). Comparative evaluation of chemical life cycle inventory generation methods and implications for life cycle assessment results. ACS Sustain. Chem. Eng. 7, 350–367. doi: 10.1021/acssuschemeng.8b03656
Pérez-Fortes, M., Schöneberger, J. C., Boulamanti, A., Harrison, G., and Tzimas, E. (2016a). Formic acid synthesis using CO2 as raw material: Techno-economic and environmental evaluation and market potential. Int. J. Hydrogen Energy 41, 16444–16462. doi: 10.1016/j.ijhydene.2016.05.199
Pérez-Fortes, M., Schöneberger, J. C., Boulamanti, A., and Tzimas, E. (2016b). Methanol synthesis using captured CO2 as raw material: techno-economic and environmental assessment. Appl. Energy 161, 718–732. doi: 10.1016/j.apenergy.2015.07.067
Perry, R. H., Green, D. W., and Maloney, J. O. (1997). Perry's Chemical Engineers' Handbook, 7th Edn. New York, NY: McGraw-Hill.
Piccinno, F., Hischier, R., Seeger, S., and Som, C. (2016). From laboratory to industrial scale: a scale-up framework for chemical processes in life cycle assessment studies. J. Cleaner Produc. 135, 1085–1097. doi: 10.1016/j.jclepro.2016.06.164
Ravikumar, D., Keoleian, G. A., Miller, S. A., and Sick, V. (2021a). Assessing the relative climate impact of carbon utilization for concrete, chemical, and mineral production. Environ. Sci. Technol. 55, 12019–12031. doi: 10.1021/acs.est.1c01109
Ravikumar, D., Seager, T. P., Cucurachi, S., Prado, V., and Mutel, C. (2018). Novel method of sensitivity analysis improves the prioritization of research in anticipatory life cycle assessment of emerging technologies. Environ. Sci. Tech. 52, 6534–6543. doi: 10.1021/acs.est.7b04517
Ravikumar, D., Zhang, D., Keoleian, G., Miller, S., Sick, V., and Li, V. (2021b). Carbon dioxide utilization in concrete curing or mixing might not produce a net climate benefit. Nat. Commun. 12:855. doi: 10.1038/s41467-021-21148-w
Rebitzer, G., Ekvall, T., Frischknecht, R., Hunkeler, D., Norris, G., Rydberg, T., et al. (2004). Life cycle assessment part 1: framework, goal and scope definition, inventory analysis, and applications. Environ. Int. 30, 701–720. doi: 10.1016/j.envint.2003.11.005
Roh, K., Al-Hunaidy, A. S., Imran, H., and Lee, J. H. (2019). Optimization-based identification of CO 2 capture and utilization processing paths for life cycle greenhouse gas reduction and economic benefits. AIChE J 65:e16580. doi: 10.1002/aic.16580
Roh, K., Bardow, A., Bongartz, D., Burre, J., Chung, W., Deutz, S., et al. (2020). Early-stage evaluation of emerging CO2 utilization technologies at low technology readiness levels. Green Chem. 22, 3842–3859. doi: 10.1039/C9GC04440J
Roy, K., Kar, S., and Das, R. N. (2015). Understanding the Basics of QSAR for Applications in Pharmaceutical Sciences and Risk Assessment. Academic press. doi: 10.1016/C2014-0-00286-9
Ruiz-Mercado, G. J., Smith, R. L., and Gonzalez, M. A. (2012). Sustainability indicators for chemical processes: I taxonomy. Ind. Eng. Chem. Res. 51, 2309–2328. doi: 10.1021/ie102116e
Sayyed Ahmad, K., Farid, B., and Nathan, P. (2022). Optimized feed-forward neural networks to address CO2-equivalent emissions data gaps – Application to emissions prediction for unit processes of fuel life cycle inventories for Canadian provinces. J. Cleaner Produc. 332:130053. doi: 10.1016/j.jclepro.2021.130053
Sick, V., Armstrong, K., Cooney, G., Cremonese, L., Eggleston, A., Faber, G., et al. (2020). The need for and path to harmonized life cycle assessment and techno-economic assessment for carbon dioxide capture and utilization. Energy Technol. 8:1901034. doi: 10.1002/ente.201901034
Skone, T., Mutchek, M., Krynock, M., Cooney, G., Pegallapati, A., Rai, S., et al. (2019). Carbon Dioxide Utilization Life Cycle Analysis Guidance for the U.S. DOE Office of Fossil Energy. Pittsburgh: National Energy Technology Laboratory.
Sylvia, S., Zainab, D., Yuanlei, C., Adam, R., Brandt, H., MacLean, L., et al. (2021). Improving robustness of LCA results through stakeholder engagement: a case study of emerging oil sands technologies. J. Cleaner Produc. 281:125277. doi: 10.1016/j.jclepro.2020.125277
Towler, G. P., and Sinnott, R. K. (2008). Chemical Engineering Design. Principles, practice and economics of plant and process design. Amsterdam, Boston: Elsevier/Butterworth-Heinemann.
Tsoy, N., Steubing, B., van der Giesen, C., and Guinée, J. (2020). Upscaling methods used in ex ante life cycle assessment of emerging technologies: a review. Int. J. Life Cycle Assess. 25, 1680–1692. doi: 10.1007/s,11367-020-01796-8
US Department of Energy (2011a). Technology Readiness Assessment Guide. Available online at: http://www2.lbl.gov/DIR/assets/docs/TRL~guide.pdf,.
US Department of Energy (2011b). Technology Readiness Assessment Guide. Available online at: http://www2.lbl.gov/DIR/assets/docs/TRL~guide.pdf.
van Bavel, S., Verma, S., Negro, E., and Bracht, M. (2020). Integrating CO2 electrolysis into the gas-to-liquids–power-to-liquids process. ACS Energy Lett. 5, 2597–2601. doi: 10.1021/acsenergylett.0c01418
van der Giesen, C., Cucurachi, S., Guinée, J., Kramer, G. J., and Tukker, A. (2020). A critical view on the current application of LCA for new technologies and recommendations for improved practice. J. Cleaner Produc. 259:120904. doi: 10.1016/j.jclepro.2020.120904
Wender, B. A., Foley, R. W., Hottle, T. A., Sadowski, J., Prado-Lopez, V., Eisenberg, D. A., et al. (2014). Anticipatory life-cycle assessment for responsible research and innovation. J. Responsible Innovation 1, 200–207. doi: 10.1080/23299460.2014.920121
Wynne, B. (1992). Uncertainty and environmental learning: reconceiving science and policy in the preventive paradigm. Global Environ. Change 2, 111–127. doi: 10.1016/0959-3780(92)90017-2
Zimmermann, A., Kant, M., Strunge, T., Tzimas, E., Leitner, W., Arlt, W., et al. (2017). CO2 Utilisation Today. Report 2017.
Zimmermann, A., Müller, L., Wang, Y., Langhorst, T., Wunderlich, J., Marxen, A., et al. (2020).Techno-Economic Assessment & Life Cycle Assessment Guidelines for CO2 Utilization (Version 1.1). doi: 10.3998/2027.42/162573
Zimmermann, A. W. (2021). Development of Techno-Economic Assessment (TEA) for Carbon Capture and Utilization (CCU) Challenges, Guidelines, Frameworks and Case Studies: Challenges, Guidelines, Frameworks and Case Studies (Doctoral Thesis). Berlin: Technische Universität Berlin.
Zimmermann, A. W., Buchner, G. A., and Schomäcker, R. (2021). Apples and apples: a shortcut assessment framework for early-stage carbon capture and utilization technologies based on efficiency, feasibility, and risk. Energy Technol. 9:2000691. doi: 10.1002/ente.202000691
Keywords: carbon capture and utilization, technology readiness levels, technology maturity, emerging technologies, techno-economic assessment, life cycle assessment
Citation: Zimmermann AW, Langhorst T, Moni S, Schaidle JA, Bensebaa F and Bardow A (2022) Life-Cycle and Techno-Economic Assessment of Early-Stage Carbon Capture and Utilization Technologies—A Discussion of Current Challenges and Best Practices. Front. Clim. 4:841907. doi: 10.3389/fclim.2022.841907
Received: 22 December 2021; Accepted: 01 March 2022;
Published: 28 March 2022.
Edited by:
Ben W. Kolosz, University of Pennsylvania, United StatesReviewed by:
John L. Field, Oak Ridge National Laboratory, United StatesCopyright © 2022 Zimmermann, Langhorst, Moni, Schaidle, Bensebaa and Bardow. This is an open-access article distributed under the terms of the Creative Commons Attribution License (CC BY). The use, distribution or reproduction in other forums is permitted, provided the original author(s) and the copyright owner(s) are credited and that the original publication in this journal is cited, in accordance with accepted academic practice. No use, distribution or reproduction is permitted which does not comply with these terms.
*Correspondence: Arno W. Zimmermann, YXJuby56aW1tZXJtYW5uQHR1LWJlcmxpbi5kZQ==; Tim Langhorst, dGxhbmdob3JzdEBldGh6LmNo
Disclaimer: All claims expressed in this article are solely those of the authors and do not necessarily represent those of their affiliated organizations, or those of the publisher, the editors and the reviewers. Any product that may be evaluated in this article or claim that may be made by its manufacturer is not guaranteed or endorsed by the publisher.
Research integrity at Frontiers
Learn more about the work of our research integrity team to safeguard the quality of each article we publish.