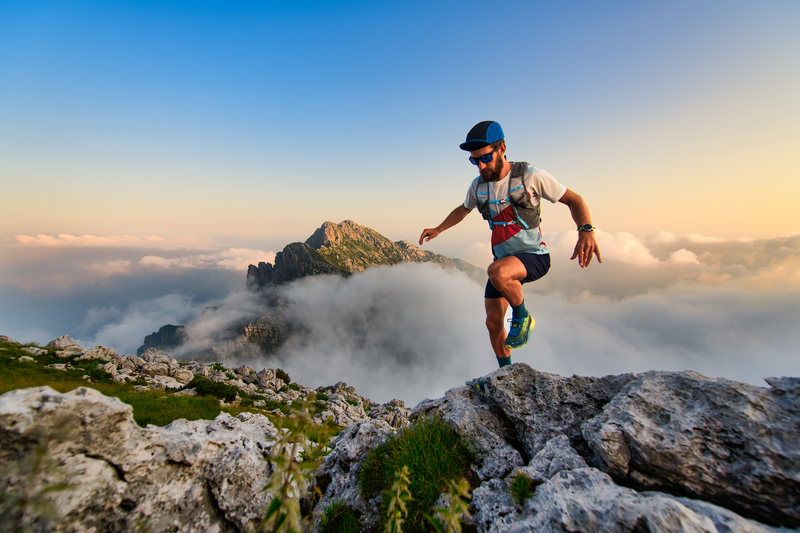
94% of researchers rate our articles as excellent or good
Learn more about the work of our research integrity team to safeguard the quality of each article we publish.
Find out more
ORIGINAL RESEARCH article
Front. Clim. , 31 March 2022
Sec. Predictions and Projections
Volume 4 - 2022 | https://doi.org/10.3389/fclim.2022.820490
This article is part of the Research Topic Multi-Scale Air-Sea Variability and its Application in Indo-Pacific Regions View all 8 articles
The hourly measurements of air-sea interaction parameters made at the Kuroshio Extension Observatory (KEO) buoy since 2004 have produced an extensive dataset that can be used to understand the dynamics of the oceanic mixed-layer under extreme weather conditions. Our analysis of the KEO-buoy measurements reveals the presence of sporadic low-salinity signals (SLSSs) with duration of 1–2 days in the mixed-layer when, for example, tropical cyclones (TCs) approach. Here SLSS events are identified within the buoy data by using a flag which is set to unity when the value of normalized salinity falls below −1 (minus one). Application of this definition to the hourly time series of salinity measured at 10 m depth by the KEO buoy has allowed us to identify 244 SLSS events over the 11-year analysis period. Both winter (January-March) and spring (April-June) seasons show a peak in the composite time series of wind velocity subdiurnal variation (WVDV) near the start time of SLSS events. SLSS events can be terminated by the entrainment of saltier subsurface water parcels to the mixed-layer in response to storms. The discovery of the WVDV peaks following SLSS commencement is the reason the present study has adopted a time scale of 1-day wind velocity analysis. This temporal resolution has enabled us to identify a TC-related delay in the development of the WVDV peaks, which may represent a fundamental characteristic of the influence of TCs on these events.
- The monthly occurrence of SLSS events shows peaks in both May-July and October, with an annual average of about two events per month.
- SLSSs cannot be reproduced by 1D turbulence closure models which have been used as part of 3D ocean circulation models.
- Some of SLSS events can be terminated by the entrainment of saltier subsurface water parcels to the mixed-layer in response to storms.
The Kuroshio Extension Observatory (KEO) buoy has been measuring air-sea interaction quantities since 2004. The KEO buoy records contain sporadic low salinity signals (SLSSs) in the mixed-layer when, for example, tropical cyclones approach. SLSSs cannot be reproduced by one-dimensional turbulence closure models which have been used as part of three-dimensional ocean circulation models in previous studies. In the western North Pacific, precipitation is substantial in both the Baiu and Autumn front seasons around June and November, respectively. The monthly occurrence of SLSS events shows peaks in both May-July and October, with an annual average of about two events per month. The composite time series of precipitation in both spring (April-June) and autumn (October-December) seasons show signals from −3 days to 0 days, indicating surface skin layer freshening prior to the start time of SLSS events. This may explain how the Baiu- and Autumn-rain fronts stimulate the genesis of SLSS events. However, a number of SLSS events occur with less rain in both summer and winter seasons. High values of wind velocity subdiurnal variation in the winter season suggest impacts from energetic cyclones that trigger SLSS events with less precipitation.
Water cycle in the atmosphere and ocean plays an important role in the global climate (Hosoda et al., 2009). Precipitation with the deposit of dust from the atmosphere yields freshening of sea surface water that then affects both physics and biology in the oceanic mixed-layer. The three-dimensional distribution of salinity in the global ocean has been measured using Argo floats. Argo floats in a traditional setting do not measure depths shallower than 5 m. This is to prevent contamination of the sensor by pollutants near the sea surface when water is sucked in by a pump and the conductivity is measured by the electrodes of the sensor. This problem is being eliminated, and the International Argo Steering Committee has recently recommended measurements up to a depth of 1 or 2 m below the sea surface. This will increase opportunity for observing the traces of precipitation in the salinity profiles of Argo floats.
While the movement of rain fronts has been monitored by multi-satellite observations with sufficient spatiotemporal resolution (Kubota et al., 2007), the associated live signals of sea surface freshening have been less captured by remote sensing. This is attributed to the short duration of rain events (from hours to days) as well as the limited number of satellites with microwave sensors for getting sea surface salinity. The life-cycle of near-surface freshwater has, so far, been investigated mainly in tropical regions by moored sensors with a vertical spacing of a few meters (Soloviev and Lukas, 1996; Soloviev et al., 2015; Thompson et al., 2019). For a better understanding of how the lenses of surface freshwater emerge and diffuse in the oceanic mixed-layer, some studies have shown the utility of using an instrument with a vertical resolution of 10 cm (Anderson and Riser, 2014). These fragmental investigations in terms of horizontal locations may be extended to the global ocean in future studies to yield climatological statistics about the life-cycle of surface freshwater. This is required for improving operational algorithms in general circulation models concerning air-sea interaction as well as sea surface salinity satellites. For example, Bellenger et al. (2017) have adopted a new formulation for salinity and temperature in the oceanic surface skin layer to investigate its impact on atmospheric circulation.
For midlatitude western boundary current regions with the passage of tropical cyclones (TCs), the statistics of surface freshening events have been less reported in previous studies. We note that the Kuroshio Extension Observatory (KEO) buoy has been moored at 32°N and 144°E in the western North Pacific Ocean and maintained by the National Oceanic and Atmospheric Administration (NOAA) since 2004 (Cronin et al., 2008). Hourly time series of mooring data collected from 2004 to 2019 are made available on the KEO website following quality control by NOAA (the structural details of the KEO dataset are explained in Appendix A of the present manuscript). The KEO observation was part of the 2-year Kuroshio Extension System Study (http://www.po.gso.uri.edu/dynamics/KESS/). This research program was aimed at clarifying various processes associated with the Kuroshio Current and its recirculation regions (Qiu and Chen, 2005).
This study has investigated the climatological characteristics of surface freshwater based on the 11-year KEO dataset, with the aim of improving our understanding of both mixed-layer dynamics and turbulence closure schemes used in Ocean Global Climate Models (OGCMs). This manuscript is organized as follows. Section The Kuroshio Extension Observatory buoy shows that the KEO-buoy dataset contains sporadic low salinity signals (SLSSs) associated with the passage of TC. These SLSSs cannot be reproduced by one-dimensional (1D) turbulence closure models. This may suggest need for incorporating the surface skin layer formulation in the modeling community (Bellenger et al., 2017). At the end of this section, this study presents a detailed definition and analysis of the SLSS phenomenon. Section Climatological Characteristics of Sporadic Events shows occurrence frequency and the time profiles of composite events, followed by Section Summary. The work described below, therefore, has wider environmental significance since it should lead to improved operational algorithms in general circulation models concerning air-sea interaction as well as sea surface salinity satellites.
Trajectories of seven TCs in the western North Pacific that have passed over or near to the buoy location are shown in Figure 1, which enables to focus on the signature of passing storms within the extensive KEO record of in situ buoy data. These seven TCs have been chosen for analysis in the present study primarily on the basis of the availability of coeval KEO data. All TCs reach maturity before approaching the KEO buoy, as indicated by the central pressure on the trajectories in Figure 1. The fact that the KEO buoy is able to successfully operate in extreme weather conditions (when wind speeds exceed 20 m/s) is clearly of central importance here.
Figure 1. Trajectories of seven tropical cyclones in the western North Pacific that have passed over or close to the KEO buoy location (blue dot). The seven tropical cyclones considered here are listed in Table 1. The central pressures of these tropical cyclones at 6-h intervals along their trajectories is indicated by red, yellow, and green dots with the red-to-yellow transition set at 950 hPa and the yellow-to-green transition set at 970 hPa. Storm T1610 approached the KEO buoy twice: first from the east and then later from the southwest after performing an anticlockwise migration. The trajectory and central pressure data are obtained from the Japan Meteorological Agency.
Precipitation has been measured by the KEO buoy for all TCs, as presented in Table 1 (except for T1605, Omais). The KEO buoy is equipped with a rain gage of the siphon-type (sampling every minute, while discharge from the gauge is smoothed every 16 mins), which provides measurements of precipitation that can be susceptible to strong winds. As a result, this may lead to an underestimation of the actual precipitation. We have therefore compared the KEO precipitation data to satellite precipitation data (Grovel Satellite Mapping of Precipitation: GSMaP, Kubota et al., 2007; Ushio et al., 2009) for all TCs in Table 1. For example, T1417 (Kammuri) yielded 115.71 mm at KEO while GSMaP recorded 216.36 mm for precipitation accumulated over 3 days during the approach. We note that the GSMaP values are somewhat greater than those from KEO for all TCs. Hereafter we present the precipitation values obtained both by KEO and by GSMaP and, where necessary, adopt the latter for our analysis.
Table 1. List of major tropical cyclones passing over or near to the KEO buoy during the study period.
We have downloaded wind velocity, precipitation, evaporation rates, longwave radiation, shortwave radiation, latent and sensible heat fluxes, and the vertical profiles of temperature, salinity, and density from 2004 to 2017 for the present study (Appendix A). For example, Figures 2A–E show hourly time series for 13–26 September 2009 when T0914 (Choiwan) approached the KEO buoy (Bond et al., 2011). As this cyclone passed directly over the KEO buoy on 19 September, the wind direction turned from southwest to southeast and the wind speed increased to about 20 m/s (Figure 2A). Precipitation rates of about 10 mm/h were measured by both the KEO buoy and by GSMaP (red and black lines, respectively, in Figure 2B). The decrease in shortwave radiation (red line in Figure 2C) indicates an increase in cloud cover associated with T0914, while enhancement of negative latent heat flux (violet line in Figure 2C) indicates evaporation associated with strong wind. The vertical profile of salinity (Figure 2D) shows patches of low salinity water on 14 and 19 September near the sea surface, which may be attributed to heavy rain. T0914 has been simulated by Wada et al. (2013) using a three-dimensional air-sea coupled model wherein the ocean model component has a total of four layers including the surface mixed-layer. They have investigated the CO2 outgassing at sea surface associated with T0914 passage at the location of the KEO buoy.
Figure 2. Hourly time series for 13-26 September 2009 when cyclone T0914 (Choiwan) approached the KEO buoy. (A) 10 m wind velocity [m/s] in the KEO buoy data with solid and dotted-lines representing the zonal and meridional components, respectively. (B) Precipitation rates [mm/hour] with the red-dotted and black-solid lines representing data from the KEO buoy and GSMaP, respectively. (C) Heat flux [W/m2] with the black, red, violet, and blue lines representing long-wave radiation, short-wave radiation, and latent and sensible heat-flux components. The vertical profiles of (D) salinity [PSU] and (E) temperature [°C] from the sea surface to 100 m depth, observed by the KEO buoy. The black lines in (F,G) represent the normalized anomalies of salinity and temperature at 10 m depth, calculated using equations (2a) and (2b), respectively. The blue and green lines in (F,G) represent the flag parameters indicative of sporadic low salinity and temperature signals (SLSSs and SLTSs) based on equations (3a) and (3b), respectively.
The relationship between sea surface salinity change and precipitation may be estimated as follows. Let us consider a water column element adjacent to the sea surface with height Δ and salinity S. Conservation of salt in the water column element yields
which may be written as
where dΔ on the right hand side may be interpreted as the increase in the height of the water column element owing to precipitation. Equation (1b) suggests that an accumulated precipitation of dΔ = 60 mm (which represents an average of KEO and GSMaP precipitation values associated with T0914 in Table 1) allows for a water column element of, for example, Δ = 20 m and S = 35.0 PSU to freshen by 0.105 (= 35.0 · 0.06/20) PSU. This estimate is largely consistent with decrease in near-surface salinity on 19 September (Figure 2D). On the other hand, the vertical profile of temperature (Figure 2E) suggests that the mixed-layer depth is about 60 m with typhoon-induced entrainment of cold water parcels from subsurface layers on 20 September, corresponding to the timing of the wind direction change (Figure 2A). Both salinity and temperature profiles manifest near-inertial oscillations from 20 September onwards. This behavior has been described in previous studies (Honda et al., 2018).
Mellor and Yamada (1982) and Nakanishi and Niino (2009) have presented ensemble-mean closure models that simulate the vertical distribution of both turbulent kinetic energy and mixing length. We have performed two sets of numerical experiments for the upper ocean using a vertical one-dimensional (1D) model, with an integration period of 2 weeks associated with the approach of each TC in Table 1. The first experiment adopts the turbulence closure scheme of Mellor and Yamada (1982), while the second adopts the turbulence closure scheme of Nakanishi and Niino (2009), as detailed in Appendix B. As shown in Figures S1–S7 (see Supporting Information), neither scheme is able to reproduce the low salinity signals near the sea surface as observed by the buoy. However, these features are not artifacts since the KEO salinity data have been quality controlled (following the method of Fofonoff and Millard, 1983) to within an accuracy of ±0.02 PSU including manual correction. We are therefore confident that these low salinity events of 1–2 day duration represent genuine surface signatures of a new air-sea interaction induced by TCs. To our knowledge, neither observation-based statistical approaches nor OGCM simulations/re-analyses have reported the presence of low-salinity episodes such as these in midlatitude western boundary current regions with the passage of TCs. We have named these newly-discovered features as “sporadic low salinity signals (SLSSs).” In the following, we shall present results from a series of investigations into the climatological characteristics of SLSSs using the 11-year KEO dataset, which may serve to improve our understanding of both mixed-layer dynamics and turbulence closure schemes used in OGCMs.
We focus on periods when a sharp decrease took place in the time series of salinity and give a definition for SLSS events. Sporadic low temperature signals (SLTSs) are also identified within the temperature time series in the same way as salinity. Let S and T be the time series of salinity and temperature, respectively, at 10 m depth observed by the KEO buoy. We extract the anomaly component from each of these series using a 7-day high-pass running filter and normalize it using the standard deviation based on a 30-day running mean as follows:
where the asterisk indicates the normalized anomaly, while the overbar symbol together with superscript 7d indicates a top-hat 7-day running mean filter. The use of a 7-day high-pass filter enables us to focus on sporadic signals (as shown in Figure 2) by removing the possible effects of mesoscale eddies and current meanders, while the 30-day standard deviation enables us to investigate all seasons of the year. For example, the scale of salinity and temperature variations caused by summer TCs in the mixed-layer differs from that caused by the more energetic cyclones of winter. The use of normalization, as given by equations (2a,b), is aimed at treating salinity and temperature variations equally.
We now define SLSS events as occurring when S* becomes <−1. We introduce a similar definition for SLTS events—that they exist only when T* is <−1. These definitions may be written in terms of the flags (Fsalt and Ftemp) for SLSS and SLTS, respectively,
where H represents the Heaviside step function. Terms on the right of equations (3a)-(3b) may be interpreted as flags which become either unity or zero when the normalized anomaly becomes less than or >−1, respectively. As shown in Figure 2F, the normalized anomaly of salinity S* (black line) has a negative peak of −1.5 on September 19, which yields Fsalt = 1 (blue line) with a duration of about 1 day. As shown in Figure 2G, the normalized anomaly of temperature T* has a negative peak of −0.8 on September 21, which yields Ftemp = 0.
We next compare the observed vertical profile of salinity (Figure 2D) with the SLSS flag values (Figure 2F), noting that SLSS recorded on 14, 17, and 19 September is extracted with high accuracy. A similar comparison of the observed vertical profile of temperature (Figure 2E) with SLTS flag values (Figure 2G) shows that no SLTS signatures fulfilled the required criterion during this period.
We have applied the definitions of SLSS and SLTS events in (3a) and (3b), respectively, to the hourly time series of salinity and temperature measured at 10 m depth by the KEO buoy from June 2004 to December 2017 which we refer to “the 11-year period” considering the availability the buoy data, as explained in Appendix A. This analysis has identified 244 SLSS events and 191 SLTS events, all of which have durations >6 h. Hereafter we investigate the climatological characteristics of these selected SLSS events.
Firstly we investigate the relationship between SLSS occurrence and precipitation. Relative to the start time of each SLSS event, we have estimated precipitation accumulated from −3 days to +3 days using GSMaP hourly data at the location of the KEO buoy. GSMaP has allowed us to maintain our analysis throughout the 11-year period on a continual basis, which is an advantage over the more intermittent KEO precipitation data. Figure 3A shows the total count of SLSS events sorted into bins of 6-day accumulated precipitation. White and blue bars in Figure 3A indicate SLSS events developing under light- and heavy-rain conditions, with the light-to-heavy transition set to 40 mm/6-day. This border value has been set to classify all TCs in Table 1 (except for T1414 and T1605) into the heavy-rain condition. A total of 152 events occurred under light-rain conditions, while the heavy-rain condition gave 92 events over the 11 years. We thus find that only about one third (= 92/244) of SLSS events occurred under heavy-rain conditions.
Figure 3. The occurrence frequencies of 244 SLSS events in the 11-year analysis period sorted by (A) total count arranged into bins of 6-day accumulated precipitation (from −3 days to +3 days relative to the start time of each SLSS event and with a unit of mm) based on GSMaP hourly data at the location of the KEO buoy. (B) Monthly averaged count where white and blue bars indicate SLSS events in light- and heavy-rain conditions, respectively, with a light-to-heavy transition set to 40 mm/6-day. (C) Same as (A) and (B) except for being sorted by total count in bins of wind velocity subdiurnal variation (WVDV) with a unit of m2/s2 and (D) monthly averaged count where green, pink and orange bars indicate SLSS events in weak-, intermediate-, and strong-wind conditions, respectively, with transitions at 5 and 25 m2/s2. WVDV for each SLSS event has been calculated as equation (4) with an average from −0.5 days to +0.5 days relative to the start time of each SLSS event and using JRA55-do 10 m wind velocity 3 hourly data at the location of the KEO buoy. The monthly climatology profiles of GSMaP precipitation (with a unit of mm and indicated by tics on the right vertical axis) and of JRA55-do WVDV (with a unit of m2/s2 and indicated by tics on the right vertical axis) at the location of the KEO buoy are calculated independently from SLSS events throughout the 11-year analysis period and are plotted in (B) as an orange line and in (D) as a black line. Monthly error bars in (B) and (D) are for all rain/wind conditions encountered over these 11 years.
In the western North Pacific, precipitation is substantial during both the Baiu- and Autumn-front seasons around June and November, respectively (see left panels in Figure 4). The monthly occurrence of SLSS events in Figure 3B shows peaks in May-July and October, with an annual average of about 2 events per month. The orange line in Figure 3B shows that the monthly climatology of precipitation exhibits a profile that is analogous to that of the heavy-rain SLSS occurrence. The present study refers to January-March, April-June, July-September and October-December as the winter, spring, summer and autumn seasons, respectively, because we are primarily interested in SLSS events caused by TCs in the summer season. Figure 3B shows that a number of SLSS events occur under light rain conditions in both summer and winter seasons.
Figure 4. Seasonal climatology of precipitation rate, evaporation rate, sea surface salinity, and sea surface height in the western North Pacific for 2003-2017. Left panels show precipitation rate from GSMaP (color shading with a unit of mm/month) and evaporation rate from Objectively Analyzed Air-Sea Fluxes (OA-flux) data (solid-contours for ranges greater than 150 mm/month and dashed contours for smaller ranges with an interval of 50 mm/month). Right panels show sea surface salinity from Argo data (color shading with a unit of PSU) and sea surface height from JCOPE2 reanalysis (solid- and dashed-contours for positive and negative values, respectively, with an interval of 4 cm). (A,B) January-March, (C,D) April-June, (E,F) July-September, and (G,H) October-December. The location of the KEO buoy is marked at 32°N and 144°E.
However, SLSS events can be triggered by processes other than precipitation. As explained later in the manuscript, we have found that a number of SLSS events are associated with the short-term variation of surface wind, rather than its magnitude without time-scale separation. Let u and v be the zonal and meridional components, respectively, of 10 m wind velocity. We define wind velocity subdiurnal variation (WVDV) based on the squares of the high-pass filtered velocity components. Thus
where the overbar symbol with superscript 1d indicates a 1-day running mean filter. We have applied equation (4) to 3-hourly wind velocity data from the Japanese 55-year atmospheric reanalysis JRA55-do (for driving ocean-sea-ice models: Tsujino et al., 2018) at the location of the KEO buoy for all 244 SLSS events identified here. The inclusion of JRA55-do wind output has enabled us to continually analyze through the 11-year analysis period, thus avoiding the discontinuities in the KEO wind velocity data. Figure 3C shows the total count of SLSS events sorted into bins of WVDV averaged from −0.5 days to +0.5 days, relative to the start time of each SLSS event. Green, pink and orange bars in Figure 3C indicate SLSS events recorded under weak-, intermediate- and strong-wind conditions with transitions at 5 m2/s2 and 25 m2/s2. The weak-wind condition incorporates 70 SLSS events, the intermediate-wind condition has 79 SLSS events while the strong-wind condition contains 95 SLSS events over the 11-year period. The monthly occurrence of SLSS events in Figure 3D shows peaks in February-May and October-December in terms of strong- and intermediate-wind conditions which are consistent with the monthly climatology of WVDV (which has been calculated independently from SLSS events) as shown by the black line. We note in Figures 3B,D that (i) high values of the WVDV climatology (black line) in the winter season may indicate the approach of energetic cyclones that trigger SLSS events with less precipitation, (ii) a number of SLSS events occur with less rain and less wind variation in the summer season, a topic to which we shall return later in the manuscript.
We have mentioned that both the Baiu- and Autumn-rain fronts have an impact on the occurrence of SLSS events. This comment warrants further investigation because the freshwater budget in the mixed-layer of the subtropical ocean is relevant to precipitation as well as evaporation. Figure 4 shows the seasonal climatology of precipitation rate, evaporation rate, sea surface salinity, and sea surface height in the western North Pacific for 2003–2017. Evaporation is substantial from regions off the Pacific coast of Japan in both autumn and winter seasons, which can be attributed to the oceanic heat content of the Kuroshio Current (Yu, 2007). The impact of evaporation appears to lead to an increase in sea surface salinity as shown in the right panel of Figure 4. Meanwhile, salty water parcels in regions around the dateline may be advected westward as part of the southern recirculation of the Kuroshio Extension Current (Qiu and Chen, 2005). The region of the KEO buoy is characterized by the presence of a thick subsurface layer of warm and saline water called North Pacific Subtropical Mode Water (Masuzawa, 1969). These conditions are typically encountered in the vicinity of the KEO buoy and they tend to lead to the formation of saltier structures in the subsurface layers of southern recirculation regions, as shown in Figure 2D.
We have calculated a composite time series based on the criterion defined by equation (3a) relative to the start time of each SLSS event, as shown by the blue lines in each panel of Figure 5 (see ticks on the right vertical axis). The top four panels in Figure 5 show the composite time series of 55, 62, 60, 67 SLSS events (giving a sum of 244 events over the 11-year analysis period) for the winter, spring, summer, and autumn seasons, respectively. The composite time series of the SLSS flag decays monotonically in about 1–2 days for all four seasons, a time-scale that is closer to the near-inertial period rather than to the decay-times of instability processes associated with oceanic eddies and currents. There is no notable second peak in the SLSS flag time series between −5 days to +5 days in Figures 5A–D, indicating that successive SLSS events within the time scale of 10 days are statistically independent of each other. Note that the annual average of SLSS occurrence is about 2 counts per month, as has been explained for Figure 3. The relationship between SLSS and SLTS events may be investigated by looking at the composite time series of (3b) relative to the start time of each SLSS event, as shown by the green lines in Figure 5. The correlation between SLSS and SLTS flags is at most 0.2 and is greatest in winter, indicating that energetic cyclones have the effect of cooling the sea surface or of causing deep vertical mixing.
Figure 5. Composite time series of the sporadic event flags defined by equations (3a) and (3b) and the precipitation rate in a 10-day period (from −5 days to +5 days relative to the start time of each SLSS event). Time series of SLTS and SLSS flags are plotted by solid-blue and solid-green lines, respectively, with ticks on the left vertical axis in a non-dimensional unit. Precipitation rates from the KEO-buoy and GSMaP data are plotted by solid-black and dotted-red lines, respectively, with ticks on the left vertical axis in units of mm/hour. Seasonal classification of SLSS events in the 11 years to (A) January-March, (B) April-June, (C) July-September, and (D) October-December in all rain/wind conditions. Total count of SLSS events in each season is noted in each panel. Statistics in (C) are subdivided into SLSS events in (E) heavy- and (F) light-rain conditions with the light-to-heavy transition set to 40 mm/6-day in terms of accumulated precipitation based on GSMaP data at the location of the KEO buoy.
The composite time series of precipitation rates from GSMaP and KEO data at the buoy location are shown as black and red lines, respectively, in Figure 5. The precipitation time series in the winter season shows a narrow peak at the start times of SLSS events, whereas both the spring and autumn time series show signals from −3 days to 0 days, indicating surface skin layer freshening prior to SLSS start times. In the summer season (black and red lines in Figure 5C) the precipitation data show signals that are less correlated with the SLSS-flag time series. The summer statistics are subdivided into SLSS events (see Figures 5E,F) recorded under heavy- and light-rain conditions, as before, using a light-to-heavy transition of 40 mm/6-day, based on accumulated GSMaP precipitation recorded at the location of the KEO buoy. We note that over the 11-year analysis period, some 18 events are associated with, for example, TCs with precipitation rates of about 1 mm/h over the 3 days prior to the event start times. The composite time series in the summer season under light-rain conditions (Figure 5F) indicates that precipitation and SLTS signals are little correlated with SLSS (the difference between KEO and GSMaP precipitation rates in this group will be investigated in a future study).
Results from a similar composite time-series analysis for WVDV data are shown by black and red lines in Figure 6 for the zonal and meridional components. Both winter and spring seasons show peaks at the start times of SLSS events, indicating the roles of atmospheric cyclones and anticyclones. The discovery of these potentially meaningful peaks in Figures 6A,B is the reason the present study has adopted a time scale of 1 day for WVDV analysis, as shown in equation (4). The autumn season shows WVDV signals of about 12 m2/s2 from −5 days to +5 days, indicating less relevance to SLSS occurrence. The WVDV time series in the summer season shows a sharp peak but its timing is delayed by about one day. The summer statistics are subdivided into SLSS events (see Figures 6E,F) under heavy- and light-rain conditions, as before (40 mm/6-day transition). We note in Figure 6E (18 events) that the delayed peak of WVDV in Figure 6C (60 events) relates to heavy-rain conditions associated with, for example, TCs. Indeed, a similar time lag was also present in the case of T0914 (Choiwan) as shown in Figure 2. Under the influence of TCs, SLSS events are terminated by the entrainment of saltier subsurface water parcels by the wind storm. A period of heavy precipitation followed by a phase of saltier water entrainment over the duration of 1–2 days typically forms an SLSS event. The composite time series in the summer season under light-rain conditions (42 events in Figure 6F) shows that WVDV and SLTS signals are little correlated with SLSS. This last result indicates the possibility that SLSS events are triggered by freshwater parcels that have remained in the surface skin layers since the seasonal development of the Baiu front.
Figure 6. (A–F) Same as Figure 5 except for black and red lines showing the zonal and meridional components, respectively of wind velocity subdiurnal variation (WVDV) given by equation (4) with a unit of m2/s2. WVDV has been calculated using 10 m wind velocity in the JRA55-do 3-hourly dataset.
We have performed a detailed examination of the dynamics of the oceanic mixed-layer under extreme weather conditions using results from the Kuroshio Extension Observatory (KEO) buoy, which has been gathering air-sea interaction data since 2004. Our main finding is that the KEO buoy records sporadic low salinity signals (SLSSs) in the mixed-layer when, for example, tropical cyclones (TCs) approach. To our knowledge, the presence and characteristics of these SLSS events have not been reported in previous studies for midlatitude western boundary current regions with the passage of TCs. In equations (3a) and (3b), we have defined the flags used to identify SLSS and sporadic low temperature signal (SLTS). These flags are designed to register unity when normalized salinity and temperature are <−1 (minus one). Application of these definitions to the hourly time series of salinity and temperature measured at 10 m depth by the KEO buoy over the 11-year analysis period has allowed us to identify 244 SLSS events and 191 SLTS events. The climatological characteristics of all SLSS events are summarized in Table 2 and are discussed below.
Table 2. Dependence of SLSS occurrence on precipitation, wind, and other factors investigated in the present study.
We have calculated composite time series based on equation (3a) relative to the start time of each SLSS event and find that the flag decays monotonically over a 1–2 day interval in all four seasons, which is closer to the time scale of the near-inertial period rather than to the decay-times of instability processes associated with oceanic eddies and currents. Another finding is that there is no notable second peak in the SLSS flag time series between −5 to +5 days. This indicates that successive SLSS events occurring within time scales of 10 days are statistically independent of each other. We have performed three further sets of composite time series analysis: the first of these is for sporadic low temperature signal (SLTS), the second covers precipitation, while the third considers wind velocity subdiurnal variation (WVDV) based on equation (4). Both winter (January-March) and spring (April-June) seasons show a peak in WVDV at the start time of SLSS events, indicating the roles of atmospheric cyclones and anticyclones. The finding of these potentially meaningful peaks is the reason the present study has adopted a time scale of 1 day for WVDV analysis.
The summer (July-September) statistics are subdivided into SLSS events recorded under heavy- and light-rain conditions with the light-to-heavy transition set to 40 mm/6-day in terms of accumulated precipitation at the location of the KEO buoy. We note that 18 events within the 11-year analysis period are associated with, for example, TCs with composite precipitation rates of about 1 mm/h over the 3 days prior to the start time of the SLSS events. A delayed WVDV peak was initially observed in the case of T0914 (Choiwan) and such temporal lags in fact represent a fundamental aspect of the influence of TCs on SLSS events.
A further TC influence involves the termination of SLSS events through the entrainment of saltier subsurface water parcels by the wind storm. Thus a preceding period of heavy precipitation followed by saltier water entrainment produces an SLSS event duration of 1–2 days. On the other hand, a number of SLSS events occur with less rain and less prominent wind variation in the summer season, when the composite time series recorded under light-rain conditions (42 events in the 11 years) indicates that WVDV and SLTS events are little correlated with SLSS events. These results may indicate the possibility of SLSS events being triggered by freshwater parcels that have remained in the surface skin layers since the seasonal development of the Baiu front.
We have mentioned that in the western North Pacific, precipitation is substantial in both the Baiu and Autumn front seasons around June and November, respectively. The monthly occurrence of SLSS events shows peaks in both May-July and October, with an annual average of about two events per month. The composite time series of precipitation in both spring (April-June) and autumn (October-December) seasons show signals from −3 days to 0 days, indicating surface skin layer freshening prior to the start time of SLSS events. This may explain how the Baiu- and Autumn-rain fronts stimulate the genesis of SLSS events.
A number of SLSS episodes occur with less rain in both summer and winter seasons. High values of WVDV climatology in the winter season suggest impacts from energetic cyclones that trigger SLSS events with less precipitation. The correlation between SLSS and SLTS flags is at most 0.2 and is greatest in winter, indicating that these energetic cyclones have cooled the sea surface or have caused deep vertical mixing. A future study should investigate whether SLSS events can be triggered by mechanisms other than precipitation.
The present study has illustrated how to extract the features of marine weather events that produce SLSSs in the oceanic mixed-layer. This new and concise approach will be useful for identifying SLSS events in the other regions of the global ocean to give necessary information for improving air-sea flux formulations. The approach of the present study will be useful for improving the performance of surface skin layer models (Bellenger et al., 2017) through a detailed analysis of high temporal-resolution data (hourly records) gathered by a mooring buoy.
Publicly available datasets were analyzed in this study. This data can be found here: https://www.pmel.noaa.gov/ocs/data-overview.
KK has performed main work. YK and YF have supported atmospheric event statistics. SO and HT have supported oceanic analysis. HA has supported overall work. All authors contributed to the article and approved the submitted version.
The authors declare that the research was conducted in the absence of any commercial or financial relationships that could be construed as a potential conflict of interest.
All claims expressed in this article are solely those of the authors and do not necessarily represent those of their affiliated organizations, or those of the publisher, the editors and the reviewers. Any product that may be evaluated in this article, or claim that may be made by its manufacturer, is not guaranteed or endorsed by the publisher.
The authors thank Prof. Joji Ishizaka and Dr. Takahito Kataoka for helpful discussions. The sources of the data used in this manuscript are summarized in Appendix A. This work was supported by a Sasagawa Scientific Research Grant from the Japan Science Society, by JSPS KAKENHI Grants 19H05698, 21K18652, 19H05696, 18H03737, and 18H03726, and by JAXA 2nd RA on Earth Observations.
The Supplementary Material for this article can be found online at: https://www.frontiersin.org/articles/10.3389/fclim.2022.820490/full#supplementary-material
Anderson, J. E., and Riser, S. C. (2014). Near-surface variability of temperature and salinity in the near-tropical ocean: Observations from profiling floats. J. Geophys. Res. Oceans 119, 7433–7448. doi: 10.1002/2014JC010112
Bellenger, H., Drushka, K., Asher, W., Reverdin, G., Katsumata, M., and Watanebe, M. (2017). Extension of the prognostic model of sea surface temperature to rain-induced cool and fresh lenses. J. Geophys. Res. Oceans 122, 484–507. doi: 10.1002/2016JC012429
Bond, N. A., Cronin, M. F., Sabine, C., Kawai, Y., Ichikawa, H., Freitag, P., et al. (2011). Upper ocean response to typhoon Choi-wan as measured by the Kuroshio Extension Observatory mooring. J. Geophys. Res. Oceans 116, C02031. doi: 10.1029/2010JC006548
Cronin, M. F., Meinig, C., Sabine, C. L., Ichikawa, H., and Tomita, H. (2008). Surface mooring network in the Kuroshio Extension. IEEE Syst. J. 2, 424–430. doi: 10.1109/JSYST.2008.925982
Fofonoff, N. P., and Millard, R. C. (1983). Algorithms for the Computation of Fundamental Properties of Seawater. Paris: UNESCO, 53pp. (UNESCO Technical Papers in Marine Sciences; 44), doi: 10.25607/OBP-1450
Furuichi, N., Hibiya, T., and Niwa, Y. (2012). Assessment of turbulence closure models for resonant inertial response in the oceanic mixed-layer using a large eddy simulation model. J. Oceanogr. 68, 285–294, doi: 10.1007/s10872-011-0095-3
Honda, M. C., Sasai, Y., Siswanto, E., Kuwano-Yoshida, A., Aiki, H., and Cronin, M. F. (2018). Impact of cyclonic eddies and typhoons on biogeochemistry in the oligotrophic ocean based on biogeochemical/physical/meteorological time-series at station KEO. Prog. Earth Planet. Sci. 5, 42. doi: 10.1186/s40645-018-0196-3
Hosoda, S., Suga, T., Shikama, N., and Mizuno, K. (2009). Global surface layer salinity change detected by Argo and its implication for hydrological cycle intensification. J. Oceanogr. 65, 579–586. doi: 10.1007/s10872-009-0049-1
Kubota, T., Shige, S., Hashizume, H., Aonashi, K., Takahashi, N., Seto, S., et al. (2007). Global precipitation map using satellite-borne microwave radiometers by the GSMaP project: production and validation, IEEE Trans. Geosci. Remote Sens. 45, 2259–2275. doi: 10.1109/TGRS.2007.895337
Masuzawa, J. (1969). Subtropical mode water. Deep-Sea Res. 16, 463–472. doi: 10.1016/0011-7471(69)90034-5
Maúre, E. R., Ishizaka, J., Aiki, H., Mino, Y., Yoshie, N., Goes, J. I., et al. (2018). One-dimensional turbulence-ecosystem model reveals the triggers of the spring bloom in mesoscale eddies. J. Geophys. Res. Oceans 123, 6841–6860. doi: 10.1029/2018JC014089
Mellor, G. L., and Yamada, T. (1982). Development of a turbulence closure model for geophysical fluid problems. Rev. Geophys. Space Phys. 20, 851–875. doi: 10.1029/RG020i004p00851
Miyazawa, Y., Zhang, R., Guo, X., Tamura, H., Ambe, D., Lee, J.-S., et al. (2009). Water mass variability in the western North Pacific detected in a 15-year eddy resolving ocean reanalysis. J. Oceanogr. 65, 737–756. doi: 10.1007/s10872-009-0063-3
Nakanishi, M., and Niino, H. (2009). Development of an improved turbulence closure model for the atmospheric boundary layer. J. Meteor. Soc. Japan 87, 895–912. doi: 10.2151/jmsj.87.895
Qiu, B., and Chen, S. (2005). Variability of the Kuroshio Extension jet, recirculation gyre and mesoscale eddies on decadal timescales. J. Phys. Oceanogr. 35, 2090–2103. doi: 10.1175/JPO2807.1
Skyllingstad, E. D., and Denbo, D. W. (1995). An ocean large-eddy simulation of Langmuir circulations and convection in the surface mixed layer. J. Geophys. Res. Oceans 100, 8501–8522. doi: 10.1029/94JC03202
Soloviev, A., and Lukas, R. (1996). Observation of spatial variability of diurnal thermocline and rain-formed halocline in the western Pacific warm pool. J. Phys. Oceanogr. 26, 2529–2538. doi: 10.1175/1520-0485(1996)026andlt;2529:OOSVODandgt;2.0.CO;2
Soloviev, A. V., Matt, S., and Fujimura, A. (2015). Three-dimensional dynamics of freshwater lenses in the ocean's near-surface layer. Oceanography 28, 142–149. doi: 10.5670/oceanog.2015.14
Stoney, L., Walsh, K., Babanin, A. V., Ghantous, M., Govekar, P., and Young, I. (2017). Simulated ocean response to tropical cyclones: The effect of a novel parameterization of mixing from unbroken surface waves. J. Adv. Model Earth Syst. 9, 759–780. doi: 10.1002/2016MS000878
Thompson, E. J., Moum, J. N., Fairall, C. W., and Rutledge, S. A. (2019). Wind limits on rain layers and diurnal warm layers. J. Geophys. Res. Oceans 124, 897–924. doi: 10.1029/2018JC014130
Tsujino, H., Urakawa, S., Nakano, H., Small, R. J., Kim, W. M., Yeager, S. G., et al. (2018). JRA-55 based surface dataset for driving ocean-sea-ice models (JRA55-do). Ocean Modell. 130, 79–139. doi: 10.1016/j.ocemod.2018.07.002
Ushio, T., Kubota, T., Shige, S., Okamoto, K., Aonashi, K., Inoue, T., et al. (2009). A. Kalman filter approach to the Global Satellite Mapping of Precipitation (GSMaP) from combined passive microwave and infrared radiometric data. J. Meteor. Soc. Japan 87A, 137–151. doi: 10.2151/jmsj.87A.137
Wada, A., Cronin, M. F., and Sutton, A. J. (2013). Numerical simulations of oceanic pCO2 variations and interactions between Typhoon Choi-wan and the ocean. J. Geophys. Res. Oceans 118, 2667–2684. doi: 10.1002/jgrc.20203
Yu, L. (2007). Global variations in oceanic evaporation (1958-2005). The role of the changing wind speed. J. Clim. 20, 5376–15390. doi: 10.1175/2007JCLI1714.1
Yu, L., Jin, X., and Weller, R. A. (2008). Multidecade Global Flux Datasets From the Objectively Analyzed Air-Sea Fluxes (OAFlux) Project: Latent and Sensible Heat Fluxes, Ocean Evaporation, and Related Surface Meteorological Variables. Technical Report OA-2008-01, Woods Hole Oceanographic Institution, Woods Hole, Massachusetts, United States. p. 64.
The details of all datasets used in the present manuscripts are given below, and are summarized in Table S1 (see Supporting Information). The 6-hourly track, lowest pressure and maximum wind speed of TCs in Figure 1 are from the Japan Meteorological Agency (JMA): https://www.data.jma.go.jp/fcd/yoho/typhoon/route_map/index.html.
The KEO link on the NOAA homepage offers measurements from sea surface to 525m depth with eleven sensors. We used hourly data from the sea surface to 100m depth from seven sensors: sea-surface, 5m, 10m, 25m, 50m, 75m, (100m: temperature only), and downloaded these from https://www.pmel.noaa.gov/ocs/data-overview. The KEO buoy data from June 2004 to December 2017 (163 months) were available from the website with the exclusion of the following maintenance periods: November 2005-September 2007 (23 months), January-February 2008 (2 months), June–October 2011 (5 months), June 2013 (1 month), and February–June 2017 (5 months), which yields a total period of 127 (=163-23-2-5-1-5) months for analysis. This is the “11-year study period” referred to in the present manuscript.
GSMaP version 6 precipitation data has a temporal resolution of one hour and a horizontal resolution of 0.1°. This product consists of a reanalysis version from 2004 to 2013 (GSMaP_RNL) and a standard version from 2013 to 2017 (GSMaP_MVK). We did not discriminate between these two data sources for long-term analysis and downloaded both from https://sharaku.eorc.jaxa.jp/GSMaP/index_j.htm. The GSMaP precipitation at the location of the KEO buoy (32.3° N, 144.5° E) was used in the present study to validate or compensate for the precipitation data obtained by the KEO (Figures 3, 5, 6).
JRA55-do version 1.4 10m wind velocity data was downloaded from https://esgf-node.llnl.gov/search/input4mips/ which has a temporal interval of three hours and a horizontal resolution of about 55 km for the whole of 2004–2017. The JRA55-do wind velocity at the location of the KEO buoy (32.3° N, 144.5° E) was used in the present study (Figures 3, 6) to compensate for the short gaps in wind velocity data present in the KEO data.
The evaporation data provided by Woods Hole Oceanographic Institution (WHOI) OA-flux Version 3 (Yu et al., 2008) is a monthly product and has a horizontal resolution of 1°. We have computed seasonal climatology for 2003–2017 in Figure 4 (contours in left panels) using data from: http://apdrc.soest.hawaii.edu/dods/public_data/WHOI_OAFlux/version3/monthly/evapr_oaflux.info.
Sea surface salinity data from the Asia-Pacific Data-Research Center (APDRC) of the University of Hawai'i is a monthly product based on Argo and has a horizontal resolution of 1°. We have computed seasonal climatology for 2003–2017 in Figure 4 (color shading in right panels) using data from http://apdrc.soest.hawaii.edu/dods/public_data/Argo_Products/monthly_mean/Mixed_Layer_Gridded_monthly_mean.info.
Sea surface height data from the Japan Coastal Ocean Predictability Experiment 2 (JCOPE2: Miyazawa et al., 2009) is a daily product and has a horizontal resolution of 1/12°. We have computed seasonal climatology for 2003–2017 in Figure 4 (contours in right panels) using data from http://apdrc.soest.hawaii.edu/datadoc/fra-jcope2.php.
Vertical mixing in the upper ocean layer is an important process since it has an impact on sea surface temperature and is thus relevant to global climate. In turn, sea surface temperature is influenced by the seasonal and interannual variations of air-sea fluxes, mixed-layer depth, surface currents and eddies, and internal gravity-wave activity. Mechanisms that determine the vertical profiles of the mixed-layer have been investigated, for example, by using numerical models with a vertical mixing scheme.
We have performed two sets of numerical experiments for the upper ocean using a vertical one-dimensional (1D) model, with an integration period of 14 days in order to simulate the approach of each TC in Table 1. One experiment adopts the turbulence closure scheme of Mellor and Yamada (1982) level-2.5, and is hereafter referred to as MY2.5. The other experiment adopts the turbulence closure scheme of Nakanishi and Niino (2009), and is hereafter referred to as MYNN. The model domain extends from the sea surface to 400m depth with a uniform grid spacing of 1m. The model code is the same as that used for the dynamical core of a 1D turbulence-biology model in Maúre et al. (2018). The initial and boundary conditions of the 1D model are explained as follows. The model has been integrated from an initial condition of no motion with the vertical profiles of temperature and salinity from the KEO buoy observations interpolated to the model grid. The model has been forced at the sea surface by the wind stress vector, and by heat and water fluxes adapted from the hourly KEO-buoy surface-flux data (Appendix A).
The 1D model assumes that all quantities are horizontally uniform. Application of this assumption to the incompressibility equation yields no vertical motion (not shown). In the real ocean, both the mixed-layer depth and the thermocline depth vary with the vertical component of velocity that may originate from, for example, internal gravity waves. In order to compensate for the lack of vertical motion, we have constructed an hourly time series of vertical velocity at 100m depth using the hourly time series of temperature and salinity perturbations observed by the KEO buoy. This is written as
where w and ρ are vertical velocity and potential density, respectively. The subscripts t and z indicate partial differentiation in terms of time and the vertical coordinate, respectively. The prime and overbar symbols indicate perturbation and mean components, respectively, over the 14-day integration period. The 100m vertical velocity derived from equation (5) has been linearly interpolated in the vertical direction to vanish at the sea surface, and then applied in the vertical advection term of the temperature and salinity equations in the 1D model. In the following we explain the results of 1D-model simulations for T0914 (Choiwan), T1218 (Ewiner), T1414 (Fengshen), T1417 (Kammuri), T1420 (Nuri), T1605 (Omais) and T1610 (Lionrock).
In each of Figures S1–S7 (see Supporting Information), the top two panels show the hourly vertical profiles of salinity and temperature observed by the KEO buoy in the top 100 m for two weeks relative to the approach time of individual TCs. The middle two panels show the result of the 1D model experiment using the MY2.5 scheme. The bottom two panels show the result of the 1D model experiment using the MYNN scheme. In the case of T0914 (Choiwan), the mixed-layer cooling starts on 20 September in the observations (Figure S1b) and on 22 September in the MYNN experiment (Figure S1f). The mixed-layer cooling is not clear in the MY2.5 experiment (Figure S1d). The observed SLSSs on both 14 and 19 September are not reproduced in the MY2.5 and MYNN experiments. The undulation of the mixed-layer bottom depth (at 70m depth) with a near inertial period is reproduced in both MY2.5 and MYNN experiments. In order to increase the number of observation-to-model comparisons, we have performed a set of 1D model experiments for the other TCs listed in Table 1. SLSSs are observed on 26 September 2012 in Figure S2 for T1218 (Ewinar), on 24 September 2014 in Figure S3 for T1414 (Fengshen), on 29 September 2014 in Figure S4 for T1417 (Kammuri), on 12 November 2014 in Figure S5 for T1420 (Nuri), on 6 August 2016 in Figure S6 for T1605 (Omais), and on 31 August 2016 in Figure S7 for T1610 (Lionrock). However, all model experiments fail to reproduce the SLSS events recorded in the data.
The inability of 1D model experiments to reproduce the SLSS phenomenon may be attributed to overestimation of vertical mixing at depths where there is little stratification (relevant to MY2.5 and MYNN models), the insufficient resolution of vertical grids (particularly uniform grids with 1m depth spacing), the assumption of horizontal uniformity (relevant to 1D models). Concerning (i) and (ii) in the above, the performance of 1D models (i.e. the ensemble-mean turbulence closure models) has often been verified using large eddy simulation (LES) models (i.e. three-dimensional turbulence models, Skyllingstad and Denbo, 1995; Furuichi et al., 2012) within idealized settings. These LES studies have been performed only for a restricted parameter range relevant to the dynamical regime of the mixed-layer under extreme conditions, owing to the limitations of numerical models (Stoney et al., 2017). For example, the effect of breaking waves at the sea surface during the passage of a TC has not yet been resolved in LES schemes, while ensemble-mean closure models for the mixed-layer have similarly not yet been incorporated. Concerning (iii), owing to both the duration of rain fronts and the presence of oceanic submesoscale eddies, SLSSs are expected to be nonuniform in the horizontal direction.
Keywords: precipitation, composite time series, surface skin layer, tropical cyclones, wind velocity subdiurnal variation
Citation: Kameyama K, Kanno Y, Ohishi S, Tomita H, Fukutomi Y and Aiki H (2022) Sporadic Low Salinity Signals in the Oceanic Mixed Layer Observed by the Kuroshio Extension Observatory Buoy. Front. Clim. 4:820490. doi: 10.3389/fclim.2022.820490
Received: 23 November 2021; Accepted: 01 February 2022;
Published: 31 March 2022.
Edited by:
Sang-Wook Yeh, Hanyang University, South KoreaReviewed by:
Kumar Ravi Prakash, Indian Institute of Technology Delhi, IndiaCopyright © 2022 Kameyama, Kanno, Ohishi, Tomita, Fukutomi and Aiki. This is an open-access article distributed under the terms of the Creative Commons Attribution License (CC BY). The use, distribution or reproduction in other forums is permitted, provided the original author(s) and the copyright owner(s) are credited and that the original publication in this journal is cited, in accordance with accepted academic practice. No use, distribution or reproduction is permitted which does not comply with these terms.
*Correspondence: Kohei Kameyama, cmVua2FtZWVAeWFob28uY28uanA=; Hidenori Aiki, YWlraUBuYWdveWEtdS5qcA==
Disclaimer: All claims expressed in this article are solely those of the authors and do not necessarily represent those of their affiliated organizations, or those of the publisher, the editors and the reviewers. Any product that may be evaluated in this article or claim that may be made by its manufacturer is not guaranteed or endorsed by the publisher.
Research integrity at Frontiers
Learn more about the work of our research integrity team to safeguard the quality of each article we publish.