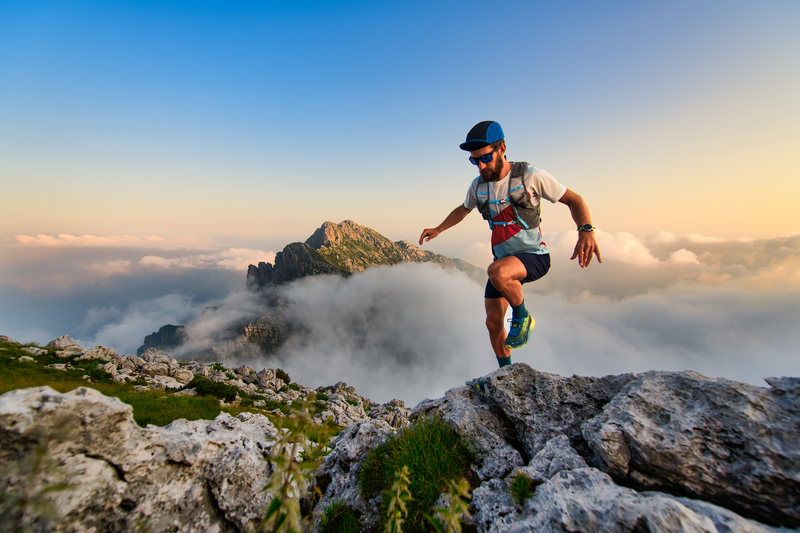
94% of researchers rate our articles as excellent or good
Learn more about the work of our research integrity team to safeguard the quality of each article we publish.
Find out more
REVIEW article
Front. Clim. , 11 July 2022
Sec. Predictions and Projections
Volume 4 - 2022 | https://doi.org/10.3389/fclim.2022.754264
This article is part of the Research Topic Knowledge Gaps from the IPCC Special Report on the Ocean and Cryosphere in a Changing Climate and Recent Advances, Volume II View all 7 articles
We provide an overview of decision support tools and methods that are available for managing climate-related risks and for delivering adaptation and resilience options and solutions. The importance of understanding political, socio-economic and cultural contexts and the decision processes that these tools support is emphasized. No tool or method is universally suited to all circumstances. Some decision processes are structured with formal governance requirements; while others are less so. In all cases, discussions and interactions with stakeholders and other players will have formal and informal aspects. We categorize decision support tools in several broad ways with the aim of helping decision makers and their advisors select tools that are appropriate to their culture, resources and other circumstances. The assessment examines the constraints and methodological assumptions that need be considered.
Climate change only differs from other risk management problems by the fact there is only one Earth and a number of the risks are existential to life on the planet. But it is still about managing risk, for which there is an immense body of literature and decades of experience to draw upon; the wheel need not be reinvented. Risks (Simpson et al., 2021) can be directly related to greenhouse gas emissions, such as the risk related to exceeding an average increase in air temperature since preindustrial times of 1.5°C, or indirectly related to that, such as health outcomes from a warming climate (Vanos et al., 2020) (see Box 1). Further, climate risks may also relate to risks from actions used to ameliorate other risks.
Box 1. Example of climate-risk control systems-managing risk of heat stroke in elderly people.
Heat stroke in elderly people is a well-established phenomenon during heat waves (Vanos et al., 2020). Care for immobile elderly people at home during elevated heat, such as in heatwaves or during summer in many parts of the world, has many factors to consider. Thermo-regulation in a patient can be assisted by reducing heat in the vicinity. Both of these are examples of control systems in which the carer can actively make decisions and facilitate immediate outcomes.
A control system has a target condition for the state of the system, a controller (regulator), and a mechanism for changing the state of the system. The controller measures the state of the system, compares that measurement with the target condition and adjusts the controlling mechanism to move the state of the system toward the target condition.
A thermostat in an air conditioner managing the temperature of a room is one such control system on which the carer may depend. Thermoregulation in a human body acts in a similar way. Under heat stress, the body reduces heat by increasing blood flow to the skin where heat can dissipate into the air. In addition, cooling is achieved by the evaporation of sweat. Heat transfer is more effective as the room temperature falls below body temperature. Evaporation can occur in situations warmer than body temperature but its effectiveness is limited by humidity. Thus, room temperature and humidity are important for managing a patient's heat stress. For simplicity, we combine these terms as “the cooling environment”.
The cooling environment of a room is dependent on that environment throughout the building, the surrounding city and the climate generally. Humidity is difficult to reduce passively and is a product of passive cooling in the larger urban environment. The thermal masses of buildings and cities create prolonged, elevated ambient temperatures that reduce the effectiveness of temperature control systems within individual rooms and homes, which have to work against continued radiation of heat from the thermal mass as well as simply reducing air temperature in a room. Each of these levels of thermoregulation are control systems that work on different temporal and spatial scales and involve different types and scales of decision processes. The interaction between them is illustrated in Figure 1 and described in Table 1.
The management of an individual patient is dependent on, inter alia, historical developments of the ambient conditions in the building (months to years), the ambient conditions in the city (years to decades), the ambient climate (multi-decades to centuries) and his or her family/social support systems. Although history in buildings, cities and climate are not likely to have considered the issue of risks of heat stroke, they are still systems to which controls can be applied, i.e. the use of feedbacks to make adjustments to the conditions. Feedbacks in these control systems outside the home will not benefit the immediate conditions of the patient but will benefit the management of risks of heat strokes in future patients. In terms of decision systems, the outer control systems are more diffuse, less determinate, both in the controller and in the mechanisms for control. The controllers involve more people with varying social and family connections to the patient, more backgrounds, expertise, and experiences, and may be less likely to have continuity of people over the course of an iteration of feedbacks. The mechanisms for control become less likely to be a single action from a choice among actions (top down) but more likely to be many diverse actions (top down and bottom up) along interacting pathways with feedbacks increasingly occurring in a haphazard and diffuse manner. This diffusivity creates the perception that the outer systems are not control systems when in fact they are just unavailable for many people, or, when available, are under-utilized and misunderstood when not considered as control systems to manage risk.
Importantly, there is no single approach that could be prescribed to manage the risk of heat stroke in the patient. It would be easy to suggest all homes have highly efficient, high powered air conditioners. However, this is dependent on the owners having funds to purchase and operate such machines, the building and city having reliable power systems to service spikes in and/or prolonged use of the air conditioners, and that the climate is such that it does not cause power outages at these times through lightning strikes.
Figure 1. Nested-control system managing the risk of an elderly patient experiencing a heat stroke during home care. Coupled inner systems of thermoregulation in the patient and the cooling system in the home can be regularly monitored and adjusted by a carer. The home environment is impacted by the ambient temperature and humidity of the building, which is the next outer control system. In turn, the building is affected by two further control systems - the temperature and humidity of the city, which is influenced by the regional climate. The identified risk (red line) in the patient thermometer is when the patient's temperature increases to a critical level at which heat stroke would occur. The cooling system is regulated by the difference in the room temperature and the target temperature (blue-arrow control system). Orange arrows indicate the primary direction of “passive” influence of temperature and humidity from the outer control systems to the inner control systems. Red arrows indicate the “active” feedbacks between these systems when the risk (probability of a temperature causing heat stroke) is unsatisfactory—adjusting the target temperature on the cooling system and the performance of the cooling system are unable to maintain satisfactory room conditions. Factors to consider in each control system are shown in small text. The carer, tenants of the building, building owners, city governors and international governors play critical roles in linking the different control systems through bottom-up and top-down processes.
Table 1. Example Attributes of the nested-control systems influencing the risk of an elderly patient experiencing a heat stroke during home care (lists are not exhaustive).
Deciding on actions to ameliorate climate-related risks is a very human process; many psychological factors may impact on the cognitive and deliberative processes of individuals and organizations (Orlove et al., 2020). These factors play important roles in making sense of the problems to be tackled and in the final decisions on what actions may be taken. They can also influence choices (decisions) on the types of methods to employ to inform decision-making. Actual methods, tools and processes for making decisions on climate change are not often discussed, except in a macro sense, when talking about how to manage climate change, as if the solution and the problem are inextricably linked and seemingly obvious. Yet managing risks, particularly indirect ones, may be achieved through many different pathways, many of which may involve great uncertainty, and which will have varying benefits, costs and effectiveness for ameliorating risk. A decision-maker may potentially have leanings to one or a few of many options, depending on their own preferences, which may not be in the interests of everyone. Moreover, individual steps to manage climate risks will have varying degrees of reversibility, potentially locking in future pathways. How can errors of judgement be reduced, and poor outcomes avoided as far as possible?
A climate management process is more likely to require iterative approaches over many years, if not decades, because a number of risks are expected to emerge in the future and actions are needed now in order to take effect to reduce risk before the future arrives. While “trial-and-error” processes may be a necessary option for managing emergency responses to extreme events, it is not an option for timely mitigation intended for limiting global mean temperatures to not rising above 2°C (IPCC, 2018). Nor is it an option under many circumstances where individuals, communities and sectors may be seriously disadvantaged by a proposed action. So what decision methods are available that could be brought to bear in resolving and deciding on (locally, regionally, globally) the best courses of action to tackle climate change, adapt to the challenges that are unable to be avoided, and enable the greatest chance for climate recovery and resilience of natural and human systems? More importantly and bearing in mind the human influences in process and outcomes, how might the complexity of the interactions of different risks (Simpson et al., 2021) be unpicked and made relatively straightforward in order to appraise how best to tackle the problem with limited resources, and many competing interests and perspectives?
In effect, risk management under climate change is the same as managing a nested “control system” (Box 1). The outer system is Earth's climate, with inner systems in Earth's regions, and progressing further inward toward locations (cities; or, in nature, ecosystems), and specific instances (houses, households and individuals; forests, glades and colonies). At whatever level, the principles of a control system can apply. It is not just a top-down process, defined as measures or regulations adopted by governments or corporate bodies, but includes bottom-up processes where actions can be taken by individuals or collectives of individuals with a mind to “think globally-act locally”. There is an interaction within and between these levels to achieve an effective control system at any level. Decision-makers need to be aware of these interactions in order to provide effective responses; the degree to which the interactions need to be made explicit will depend on the scale of response being considered. Also, they need to be aware that, unlike a readily-understood control system such as a thermostat, adjustments (or iterations) will be needed along the way to correct the trajectory, rather than allow overshoots and a need for subsequent correction and restoration.
Decision processes do not have a uniform structure. The circumstances surrounding decisions may differ in many ways. There may be a range of uncertainties involved, differing in character and scale (see Box 1, Figure 1, Table 1). For climate change, the issues may relate to protecting a small locality or community or perhaps adapting a region or continent; and the potential consequences of the decision may vary from something quite moderate up to very significant, perhaps existential. The people involved can vary from a single decision-maker to several decision-makers, or in the climate change context often local or national governments with a plethora of stakeholders. The objectives may be unclear at the outset, often contentious among the decision makers and stakeholders. Experts may disagree in their advice, and data to resolve their differences may be sparse. The formal governance structures which dictate who should decide, and their responsibilities, authorities and accountabilities, can constrain the decision-making considerably, including the formal interactions with stakeholders. At the same time, availability of social and more traditional media ensures that informal debate among all players – decision makers, stakeholders, experts, and decision analysts – and the public will take place beyond the formal decision process creating expectations and even bottom-up actions, yet also providing a lot of relevant and useful information. Against such a breadth of circumstances, it is inconceivable that a single decision-making tool will be suitable for all cases: although some proponents of a method or software application might suggest otherwise in their marketing. People seeking to manage responses to the climate challenges amongst a portfolio of other challenges can find linking tools to tasks within their context a challenge in itself.
Here, we recognize that decision-making for managing climate-related risks is most likely to be unorganized, unstructured and, in simple terms, messy at the outset. However, many risks need to be managed by a collective of people, using processes that enable collective and repeatable outcomes, whether they be through communities, businesses and industries, civil society groups, and governments. The more often the processes are predictable and repeatable, the more they can be used by others in similar contexts. We lean to decision analytic approaches that can navigate the complex nature of risk management and make explicit the nature and background of a decision.
Our aim is to provide an overview for policy-makers on what tools may be useful to support decision-making in managing climate-related risks, recognizing the complexity of the issues both in the physical world and the socio-political world in which the decisions have to be made. In doing so we also provide a guided literature review, both directly and through many of our citations. Decision-making (hereafter DM when used as an adjective) literature specifically oriented toward “climate change” is sparse (Figure 2). We separate DM literature from other literatures that may relate to the causes and drivers of the phenomena that underpin understanding the risks; we seek literature specifically related to the decision process. A broader literature on decision-making tools is used in this overview, with links to experience in their application to climate-related DM. While we do not undertake an exhaustive and systematic review, we have covered sufficient breadth for the reader to find examples of the application of the main DM tools and techniques available.
Figure 2. Number of journal articles (per decade) on decision-making and risk management found in the literature database SCOPUS in each decade for seven climate-related risks, with the number specifically related to “climate change” indicated. Journal Articles were found by searching abstracts using the search “ABS ({risk assessment} OR {risk management} OR {decision making} OR {control system} OR {decision system}) AND (risk)”, where the risk was Fishery (extended search term – “fishery OR fisheries”), Drought (“drought”), Flood (“flood management OR flood mitigation”), Wildfire (“wildfire OR wild fire”), Coast (“coast”), Urban (“urban environment”), or Health (“public health”).
The first part of our overview relates to framing decision-making. We identify the components of making decisions to respond to climate risks in a timely manner; some components of decision-making may have greater importance than others depending on the context for the decision-makers. By doing so, we aim to provide a framework in which the context for decision-making can be better understood and the tools better utilized. The second part is about the decision process, presenting phases of and approaches to the process. In particular, we then catalog several decision-making tools in ways that should enable individuals, communities, organizations and government departments and agencies identify a small number that may suit their needs in relation to climate change adaption and mitigation. We aim to help problem-solvers and their advisors become “intelligent customers” of decision analysis. We make no claims that our advice is objective in any sense; any catalog requires judgement to classify each item. However, we hope that we have written this paper in a way that catalyses a “pause for thought” so that users will better understand the various ways to make a decision utilizing a suite of available methods and tools. Lastly, we provide some insights from our experience on the road ahead for managing the climate challenges.
The majority of our decision-making is informal, barely structured with little explicit deliberation and made in ways of which we may be barely conscious; of course, the majority of our decision-making concerns things such as when to eat lunch or the route to take across a station concourse. Consideration of the consequences of different outcomes may be barely noticeable. The degree to which risks may be considered depends on how “lucky one feels”, which is an important motivator as to whether systematic approaches are used (risk aversion) or not (risk tolerant). In this case, risk aversion is less about fear and avoidance but more about determining that the consequence of a risk being realized cannot be tolerated. More systematic approaches may be something like needing to get to a meeting on time and considering the timeliness of different options for routes across the city. For significant decisions and in a professional context, we usually seek to make our decisions more formally, more “rationally” (“blind luck” is not an option), and in an auditable way, perhaps supported by some form of decision analysis and evidence. In groups we deliberate and seek to resolve differences of views. In organizations and governments there are formal governance rules and constitutions determining the decision processes, authorities and accountabilities; but alongside these, informal discussions inevitably take place, influencing the outcome.
That any form of decision analysis necessarily imposes some form of consistency and rationality upon the explicit modeling of the objectives and uncertainties is often not appreciated. Moreover, the consistency and rationality assumed by some approaches may contradict those assumed by others, making the use of some tools incompatible with the use of others. Thus users need to check that they not only understand but agree with the assumptions underpinning those methods that they adopt, or they may be misled by or misuse the results. A very important aspect that distinguishes methodologies of decision analysis is whether they seek to be objective or instead render subjective judgements explicit, taking into consideration diverse values and uncertainties. That said, the application of any method, whether its assumptions fit with the users' perspectives or not, stimulates discussion and focuses attention on understanding the issues, and that alone can be enormously beneficial.
Informal decision-making may not naturally satisfy many of the assumptions made by a decision analytic method. The simplistic response is that informal decision-making is about securing an outcome that may not be easily justified, and that formal decision-making embodies principles to ensure that important decisions are made soundly and rationally. However, in practice, informality and formality run side by side and can be more harmonious, making it not quite so easy to make such a ready distinction (Hodgkinson and Starbuck, 2008; Gregory et al., 2012; French and Argyris, 2018). At the individual level, Kahneman, Tversky and many others have investigated the differences between informal and formal decision making (Kahneman and Tversky, 1974; Morton and Fasolo, 2009; Kahneman, 2011; Montibeller and Winterfeldt, 2015). For many years this work was discussed under the general heading of heuristics and biases, recognizing that informal decision-making uses “quick and dirty” heuristics to make choices, but at the risk of biasing choices on average away from what various principles of rationality would suggest. More recently, the terminology has changed to talking about:
Whether there is a true dichotomy here is moot and there are many other subtleties discussed in the literature (Shleifer, 2012; Evans and Stanovich, 2013). However, this simple distinction is sufficient for our purposes. Decision analysis seeks to encourage System 2 Thinking, helping decision-makers, their advisors and stakeholders each individually think through and reflect on the issues. However, it is easy in discussions and specifically in articulating probability and value judgements, to slip into System 1 Thinking. Better methodologies and tools have elicitation processes for nudging and challenging participants to think carefully and explicitly when giving judgements, but weaker ones simply take the responses and build them into the analysis.
Most decisions are made by groups and there are informal and formal aspects to their interactions and deliberation. Sometimes a decision is reached by simple discussion and consensus, or maybe an informal vote. Other times, “horse-trading” and other agreements can connect decisions (“… and you vote with me next time”). Less democratically, there may be a dominant leader who influences agreement with their views. Business, organizational and political/government decision making are bound by more formal governance structures and constitutions, which prescribe who can take part, what interactions are allowed, how stakeholders may have their voice heard, voting systems, etc. In many societies, decision-making has become more inclusive with stakeholders and the public being consulted formally (Bayley, 2008; Renn, 2008; Rios Insua and French, 2010). This is particularly true in the environmental domain in which many modern techniques of stakeholder engagement, public participation and deliberative democracy have been developed (Beierle and Cayford, 2002; Gregory et al., 2012). Alongside such inclusive deliberations, inevitably informal discussions are also influential. In businesses and organizations, these may be no more than “water-cooler” conversations; but the advent of social media has allowed much wider, often very influential discussion to take place for all types and scales of decision-making. To parallel the distinction between System 1 and 2 Thinking, French and Argyris (2018) have introduced the terminology:
Decision analysis is aimed primarily at supporting System 2 Societal Deliberation helping those charged with the responsibility of taking the decision to do so in an informed, auditable and explicit way. It should, however, recognize the information sources provided by System 1 Societal deliberation such as social media, from which the decision-makers can learn about stakeholder values and other perspectives on the issues, thus ensuring that they are aware of breadth and depth of the issues that they face. Indeed, decision analysis can help in using its tools to communicate the decision-makers' reasoning to their stakeholders, particularly in the formulation and implementation stages of decision-making (French et al., 2005; Morton et al., 2009). In more inclusive decision-making, decision analysis can articulate discussions between decision-makers, stakeholders and experts, drawing the System 1 Societal Deliberations into the formal System 2 process (Mustajoki et al., 2004; Gregory et al., 2012; French and Argyris, 2018). It is important to recognize that broad processes which support this transition to System 2 Thinking and Social Deliberation are context dependent and depend on the skills of the analysis teams rather than something that can be achieved in an almost mechanical way alongside the modeling, computations and analysis. Effective decision analysis requires many more diverse skills than some mathematical and algorithmic introductions to decision analysis suggest.
Decision analysis requires two forms of modeling. First, there is a need to model the external context and the physical issues being addressed; in our case, some climate change impacts. Such modeling is descriptive of the context and can be validated empirically if there are data available, though in many examples of risk management and mitigation, preventive actions are needed before full data may become available. Such models are constructs of two or more entities and the relationships and influences between them. In managing risks, the model may simply be: “if we choose to undertake this action to ameliorate the unacceptable risk, then these consequences will arise because of these reasons.” This model may be founded on the professional judgement of decision makers and their experts or more empirically-based. Options for actions may be further elaborated by alternative beliefs or observations relating actions, the system being managed, and the consequences. The model can be made more robust by assembling knowledge relating to:
• What are the drivers of the risk and how might it be ameliorated?
• What makes the risk unacceptable?
• How specific does the action need to be described in order to fully understand the consequences?
• How does the action interact with the system to deliver consequences?
These questions may be explored with heuristic models, network (pathway) models, statistical models, dynamic mathematical models or a mixture of types, depending on the available knowledge and data. Some approaches to decision analysis are limited to specific types of models, while others are more flexible. The robustness of a model for decision making is determined by the degree to which an action will be systematically chosen for the task and correctly ameliorate risks as expected. Box 2 illustrates the development of a model highlighting some connections of different parts of the management and Earth Systems in managing the risks of damage from flooding.
Box 2. Illustrative model for managing the risk of damage from extreme floods.
Climate change is increasing the likelihood of extreme flooding and, therefore, increasing the risk of accumulated damage from floods in low lying communities (Tabari, 2020). A number of actions through governments may ameliorate this risk in a low-lying area, either through mitigation (reducing greenhouse gas emissions), direct interventions (reducing exposure by increasing flood water storage, building levee banks or moving low-lying communities to higher ground), reducing vulnerability of exposed communities (reducing the effects of exposure in buildings and infrastructure or increasing capacity to recover), or creating incentives or a policy/regulatory environment that stimulates individual or private sector investment in reducing exposure and vulnerability.
Figure 3 presents an illustrative model of the management world and the real world in which human and natural systems interact. The management world is what is known and can be controlled, including human actions (interventions, impacts, activities, incentives, regulation, policy—and, among stakeholders, acceptance of that policy), while the real world remains unknown except for the observations that are made of it. These observations may be perceptions/perspectives, monitoring of important aspects of the human and natural systems relevant for management, or developments in understanding of these systems. The intersection of the management and real worlds are through human actions and observations.
The relationships of different subsystems in both worlds are illustrated using a digraph methodology, where two subsystems (“nodes”) are linked via an “edge” or interaction. In this example, the strength of the interaction relative to other interactions is indicated by the width of the line, and its certainty by the length of the dashes. An arrowhead or a circle indicates whether the interaction is a positive or negative correlation, i.e. if the magnitude of one subsystem or variable increases then the other will increase if the correlation is positive or decrease if it is negative, and vice versa. This relationship and the strength of interaction is the exposure. The size of the arrowhead or circle can be used to indicate vulnerability and its shade an indication of certainty. In framing the process for managing a climate-related risk, knowledge can be used to map such a digraph, with methods available to explore what might happen to all the nodes in the system if you “press” one or more nodes by a directional change—increase or decrease.
A mapping process such as this is useful, at least, during the “sense making” phase for helping decision-makers and stakeholders alike to better understand the nature of the problem and the degree of knowledge and uncertainty that need to be addressed for making robust decisions (Melbourne-Thomas et al., 2013). Not included in this illustration are the potential interactions with other human and natural subsystems or with managing other risks. These can be readily developed in such a diagram to explore and consider whether such interactions need to be addressed.
Figure 3. Example system managing risk of accumulated damage (red circle) of low level areas prone to flooding in the real world (Box 2). Each of the subsystems in the real world have a dynamic affected by internal drivers as well as the external inputs and outputs from other subsystems. The relationships (edges) between subsystems (nodes) and their effects on each other are mapped using a digraph. An end of an edge has an arrow (positive relationship), circle (negative relationship) or neither (no relationship), which indicates how the abutting subsystem will change when the subsystem at the other end of the edge changes. A positive relationship means the affected subsystem will change in the same direction as the impacting subsystem, and a negative relationship means the affected subsystem will change in the opposite direction. These subsystems may also be influenced by other Earth subsystems, human activities and/or management systems not illustrated here. Knowledge of the real world is constrained to the three black arrows. Interventions of the management system in the real world are through actions, which may in themselves be subsystems.
The second form of modeling related to the decision-makers' and stakeholders' beliefs, values and objectives. These are more subjective and do not allow empirical validation. Moreover, the modeling is not descriptive in the sense that beliefs, values and objectives exist fully and explicitly before the modeling process begins. Rather the process of elicitation helps the participants reflect on what they are truly seeking to achieve and constructs the detailed objectives for the analysis (Keeney, 1992; Lichtenstein and Slovic, 2006; French, 2021). This form of modeling is particularly focused on helping the participants move from System 1 Thinking toward System 2 Thinking as it generally introduces rationality conditions that help their values become more consistent. Such modeling is known as prescriptive rather than descriptive modeling. Comparing and deliberating on different prescriptive models may also be important in Social Deliberation if some stakeholders hold to dogma that conflicts with current established approaches. While good decision-making depends on sound empirical description of the context, the process of reaching a decision and consensual acceptance of the selected course of action are not necessarily helped by effectively informing some of the stakeholders that they are “simply wrong” (French and Argyris, 2018), so the deliberations around prescriptive models need to be carefully and sensitively facilitated.
There is much emphasis currently on evidence-based decision-making and we would certainly echo this, but with a careful interpretation. Evidence and the knowledge that it supports is often encoded in descriptive models. We only have direct observations about the past. Decision-making is about planning for the future and so to use observations, we must make judgements about its relevance to the future: do we believe that things will continue in this way or that? Moreover, in many cases relating to risk management, what evidence we have is partial, if indeed we have sufficient data to claim any validated evidence at all. This inevitably means that there is a tension between scientific advisors, who want more time to accumulate and validate evidence, and the decision-makers, who need to make urgent decisions to mitigate risks. Decision analysts need to appreciate this tension in managing the deliberations between decision makers, their scientific experts and stakeholders.
A tension exists between observations, evidence, models about the future and effective risk management related to climate change; what constitutes evidence in climate-related risk management? In the last three decades, the Drivers-Pressures-State change-Impact-Response (DPSIR) framework has grown to underpin evidence-based management (Patrício et al., 2016). At its heart, is the need to attribute change to a driving cause; attribution of climate change to greenhouse gas emmissions from human activity has been a central theme of the Intergovernmental Panel on Climate Change (Bindoff et al., 2013). The application of DPSIR is usually at small spatial scales (10,000 km2 at most) (Patrício et al., 2016) with a view that impacts can be detected and, once detected, restoration would be possible within a similar time frame. Such an approach implies that failure to not have a significant impact can be easily detected and rectified. In climate change, this is equivalent to accepting that an overshoot of a target global mean temperature would not be a disaster and that the climate can be restored before disastrous effects would arise. Yet, we know that the effects of greenhouse gases emitted now will take many decades before their effects will be diminished. Managing this risk requires decisions on actions well in advance of observations demonstrating impacts. Timeliness for action in risk management is an important factor not usually associated with DPSIR analyses. Uncertainty in both descriptive and prescriptive modeling increases the risks of failure. Evidence, therefore, needs to comprise not only observations of the state of the system but consider their power for detecting change and attributing it to the causes, as well as the degree to which models can help manage future risks.
Lastly, we are aware that the prescriptive modeling and analysis used to support decision-making can be interpreted naively as algorithmic computations on simple, often linear models without concern for the wider processes that these calculations support. To be frank, one of us has seen many naïve analyses performed by quantitatively adept scientists which have not truly supported the socio-political processes that surround any complex decision and which consequently have not really informed the decision-makers and stakeholders. Effective decision analysis uses the prescriptive modeling to articulate the deliberations between the participants building a shared understanding of the issues and each other's perceptions and values. It is through that shared understanding that a decision emerges, not simply from the maximization of some objective function. So in the next sections we emphasize many such “softer” socio-political issues that need to be reflected upon and brought into the deliberations in developing appropriate decision analyses for a set of issues.
Decisions are affected by many contextual factors beyond the formality and constraints of the processes used (see French and Geldermann, 2005; Hodgkinson and Starbuck, 2008; French et al., 2009 for a wider discussion of contextual issues) and can be explicitly incorporated in the analyses leading to and supporting decisions. Some important issues to consider are:
• The “decision-maker” may be an individual, a group sharing responsibility, a community, an organization or other legal entity, a government or, in some senses, by society itself.
• The degree of “system understanding” of the risk to be managed, including the root causes and drivers of the risk (cause and effect), and the way the risk and its consequent effects manifest and encoded by the descriptive model of the system.
• The range of possible options for managing risk may vary between a few alternatives and effectively an infinite number.
• There can be many other players involved: stakeholders who will share in the potential impacts in diverse ways; experts who can advise on possible actions, other risks and consequences; and the decision analysts who develop the decision modeling and use this to help articulate deliberation.
• Culture is an important element, especially in relation to the recognition of subjectivity in the discussions and whether this should be modeled and made explicit. Stakeholders' response to risk and uncertainty has many cultural influences (Hofstede, 1984; Thompson et al., 1990; Douglas, 1992). Since climate change is a global issue, variation in local culture can mean that a decision tool and approach appropriate to a set of issues in one region may be inappropriate for seemingly the same issues in another.
• The time and other resources available before a decision must be made can constrain the range of investigations and modeling used.
• The range and depth of uncertainties involved are very important (see Subsection Types of Uncertainties).
• The values and objectives driving the decision may differ between options too. In the private sector, the profit motive and shareholder value may not override other criteria, but financial objectives have high weight; whereas the public sector have more altruistic objectives reflecting responsibilities to, e.g., the public, maintaining society and the environment (see Subsection Values and Objectives).
Box 2 develops these concepts as part of a control system, using a simplified example of managing the risks associated with flooding based on a model of how the management and Earth system might work. The contextual issues that arise in mitigating and adapting to climate change are important in determining suitable decision processes and tools. Firstly, the threatened impacts are global, but with many regional and continental differences in the scale and type of impact. Different areas of societal and business activity will be affected differently. A wide range of stakeholder interests will need to be considered in almost all cases, including those of humanity itself. There are ethical and moral issues to consider alongside more prosaic objectives. The breadth of uncertainties, many of which are deep and difficult to assess, is huge. While less so than in previous decades, climate science is controversial, and consensus will be hard to achieve across all stakeholders.
The breadth of uncertainties can be overwhelming. Courses of action are broadly constrained by the knowledge readily available and the familiarity present in a situation. Identifying the general situation with respect to uncertainty will direct DM planning from the beginning and facilitate communication on what is required.
Cynefin is a way of categorizing decision contexts (“spaces”) according to the decision-makers' and their experts' knowledge of cause and effect and hence their ability to model the system (Snowden, 2002; French, 2013) (see also the community of practice—https://www.cognitive-edge.com/). If a context is known or knowable, then it will be possible usually to build sophisticated models and make sound predictions; but if the context is complex and chaotic only the simplest of models will be possible. Courtney (2003) and others have characterized uncertainty simply on the quality and precision of models that can be built and developed, a very similar categorization to Cynefin. We have chosen to go with the Cynefin formalism since it seems clearer to us to think of knowledge of cause and effect in general terms, rather than when knowledge is specifically expressed as a formal model.
Cynefin recognizes four broad cases (Figure 4):
There is a fifth area in Figure 4, relating to disordered contexts, i.e., those contexts which have yet to be classified. While disordered contexts may be important in other applications of Cynefin, one of the first tasks in problem formulation is to understand and classify the context so the disordered area quickly becomes irrelevant to decision analysis. Moving from the Chaotic Space through the Complex and Knowable Spaces to the Known Space, our knowledge and understanding move from deep uncertainty to certainty. Epistemology from sense-making through inference to full knowledge can be described very simply against the backdrop of Cynefin (French, 2013). Various decision analytic techniques are available for the Known, Knowable and Complex Spaces, but in the Chaotic Space decision-making is a matter of trial and error; or, if there is time, defer any decision and investigate the situation to see if one can learn enough to move the context into the Complex Space. An outcome of this approach is to provide greater openness as to the scale of the problem relative to the knowledge base, which then promotes more obvious courses of action.
Decision-makers can face many forms of uncertainty. Many typologies have been developed to describe these, each focusing on one or more characteristics (Knight, 1921; Berkeley and Humphreys, 1982; French, 1995b; Paté-Cornell, 1996; French et al., 2020). Discussions of uncertainties often focus on the external world within which the problem is being faced, and for which we describe three types of uncertainties—stochastic, epistemic (structural) and analytical. We also describe two important uncertainties internal to the decision process—ambiguity and values—relating to the decision-makers' and stakeholders' perceptions and valuations of the world. These five uncertainties are:
Decision tools and processes can address all five uncertainties, though many concentrate on just one or two. For instance, confining attention to ambiguity and value uncertainties and ignoring stochastic and epistemic uncertainties can help focus discussion sufficiently to clarify goals and objectives and support deliberation between wider stakeholder groups and decision-makers. Partitioning and classifying the components of any uncertainty into these five wide categories is a matter of judgement; but the process of doing so catalyses discussion and helps ensure that all uncertainties are noted in any analysis, even if some are subsequently ignored in order to focus on others.
Any of these uncertainties can be too deep to be modeled and analyzed or resolved by deliberation within the time and resources available for a decision. This may happen because data are very sparse, expert disagreements very wide or, in the case of value uncertainties, ethical issues extremely complex and controversial. In such cases, however the uncertainties should be dealt with in principle; the depth of disagreement between experts and stakeholders, the lack of data, and the need to make a decision relatively quickly mean that in practice methods that can deal with deep uncertainties will need to be adopted until the uncertainties can be resolved (Walker et al., 2013; Marchau et al., 2019; French, 2020). We discuss this further below.
Table 2 helps relates these different types of uncertainties to some of the challenges that arise in facing up to a climate change issue. For instance, consider specific hazards (row 2). Uncertainties may concern (i) the frequency with which an extreme weather condition occurs; (ii) how large a change in the weather extremes will occur; (iii) how well we can predict the weather pattern; (iv) what the goals and objectives would be in adapting to the new pattern and (v) how serious the effects would be in terms of these goals and objectives.
Table 2. Types of uncertainty that can arise in different aspects of an analysis supporting a decision on mitigation or adaption.
Uncertainty does not just relate to what might happen (i.e. stochastic, epistemic and analytical uncertainties); but also to how well potential impacts can be described and valued (i.e. ambiguity and value uncertainties). This can be true at organizational and governmental levels as much as for individuals; and may be particularly the case when the scale of an issue in space or time is large, as is usual in climate change contexts. Issues that extend over regions or countries or over long timespans have strong tendencies to be set in complex socio-political and economic contexts in which values are uncertain and hotly debated, making them complex or even chaotic contexts for decision-making, however straightforward a technical solution might seem.
The balance between how particular decision analyses address uncertainties relating to the external world and those relating to the values driving the decision making is important. Some analyses partially ignore uncertainties relating to the former in order to focus on conflicts in the values held by different stakeholders and help structure debate; others build very sophisticated models of the external world to predict potential consequences, but in doing so lose transparency and risk becoming untrustworthy black boxes to many stakeholders. There are no methods which guarantee to balance such conflicts and provide a oath through such complexities, but skilled decision analysts have the professional facilitation skills that can help find a resolution (Phillips, 2007; French et al., 2009).
Decision making is driven by values, by what the decision-makers want to achieve. Values are necessarily subjective, but in societies that seek to avoid explicit subjectivity in their decision-making, economics and financial theory provide ways of costing many climate change impacts in a broadly objective manner; but there are some “intangible” impacts such as the loss of a historic site or natural estate, or the cultural impact of moving communities that are difficult to cost. The ability to assess intangible impacts, albeit subjectively, is one of the characteristics that distinguish different schools of decision analysis.
Although we have discussed contextual issues and uncertainties first, good decision making in practice follows value-focused thinking. Keeney (1992) describes this as “first deciding what you want then figuring out how to get it.” This runs counter to the more usual alternative-focused approach: namely first identifying some alternatives, then deciding between them. However, value-focused decision making is more creative, not being confined to a set of pre-defined alternatives. Moreover, being aware of the objectives of a decision analysis at the outset means that analytic effort can be focused on what matters, avoiding irrelevancies and providing the means by which any options can be evaluated for their contribution to a solution. In particular, since climate change, environmental and economic models can be very computationally expensive to analyse, value-focused thinking can direct effort to calculating what matters; the scale of the effort required to address the problem can be more easily identified.
Economic and financial methods provide one way of exploring values and objectives: e.g. cost-benefit methods seek for each alternative to evaluate the total cost of implementation and consider it relative to the cost of potential impacts that the alternative ameliorates (Boardman et al., 2017; OECD, 2018). More generally, value and utility methods offer ways of assessing both tangible and intangible costs and benefits, albeit relying more of subjective or, at least, less objective inputs (Keeney, 1992; Keeney and Raiffa, 1993; Bedford et al., 2005).
In passing, we note that many perspectives on rational decision-making separate the Science from the Values that need to be balanced in making a choice. By “Science” we mean the knowledge and investigations that can be brought to bear on resolving the issue and addressing the uncertainties. By “Values”, we mean the decision-makers' objectives that the ultimate choice seeks to meet. Of course, in any democratic society addressing climate change issues, the decision-makers should draw stakeholders' objectives into the ones that they use in the analysis (Renn, 2008; Rios Insua and French, 2010).
In control systems, a decision process will have in mind to update the controls in a regular feedback process. Simple control systems will have only one control with a pre-determined target for adjusting the control based on a measurement of the state of the system – as in the thermostat in our example. More diffuse controllers such as for the climate system, may use many different actions including new actions as differences between the state of the system and the target are measured, considered and responded to. If a risk has only been identified for the first time, then part of the decision process in this first instance will be to determine not only the actions to ameliorate the risk but also whether, and when, to assess in the future the success, failure or other impacts of the actions and whether subsequent adjustments or new actions are needed.
Almost every writer on decision analysis has summarized the decision-making process as a cyclic iteration of several phases (see the many citations to decision analysis in this paper). Here we use three broad phases, which are adequate for our purposes:
Sense-Making and Modeling: Before any auditable, rational decision-making can begin, it is necessary to identify issues, values, objectives, uncertainties, stakeholders, possible actions and their consequences, engage with stakeholders and consult experts as needed, and determine the scope and boundaries of the subsequent analyses. Only when substantial progress has been made on these, is it possible to build a quantitative model and conduct any analysis. This is also a time when the interaction between different risks and decision processes can be mapped, the relative importance of each identified, and the need for integration in their ongoing management.
Analyzing and Exploring: Once a model is built and/or appropriate existing data and knowledge services are identified, exploration and analyses are undertaken in relation to the study's objectives, options and generally building an understanding. Sensitivity and robustness analyses may – should – supplement the decision analysis, setting bounds on some of the residual uncertainty. During the process, the model and information should be validated as much as possible against available data and the decision-makers', experts' and stakeholders' perceptions. The detail and application of this phase is very much dependent on the Cynefin context in which the problem starts out, and, if needed, how much time may be available to move the problem from one context to the next.
Interpreting and Implementing: The results and guidance offered by the analysis need to be interpreted into real world actions. This requires that the decision-makers and analysts make a judgement whether the analysis is adequate or, in technical terminology, requisite for the decision, guiding them to a consensus on the way forward (French et al., 2009). They need to judge whether the model, the analysis and the conclusions are fit for their purposes. Once made, they will also need to communicate the decision to stakeholders and implement the actions.
Figure 5 relates the three phases to the use of data from the real world, and choosing from available options to meet the policy objectives. The left-hand side of the graphic corresponds to the discussions, deliberations, analyses and studies that support the decision making. The right-hand side relates to the real world, which is always too complex to be perfectly modeled or analyzed. We emphasize that the real world includes not just relevant changes in climate, but human society, the environment, business, industry and agriculture and all the systems that need be considered in developing policies in mitigation and adaption.
Figure 5. Setting the three decision making phases into a wider context of a control system. The left-hand side of the graphic corresponds with the human processes of conceptualizing, modeling, analyzing and deliberating on the issues; the right-hand side to the real world and society. In a well-developed simple control system, the “Science, Data, and Knowledge” are measurements (feedbacks, monitoring) of the real world, the “sense making” will have been previously simplified to one or more target objectives, the “analysis and exploration” process is simplified to estimating the difference between the state of the system and the target/desired state, and the “interpretation and implementation” process is simplified to pre-agreed actions to move the system from its current state to the target state.
Generally, the three phases of decision-making proceed from the top to the bottom of the graphic and are indicated by the bulleted lists, but we recognize that analyses, discussions and deliberation will iterate backwords and forwards as understanding of the issue grows. The “decision-maker” at the bottom of the graphic is to be understood as the person or, more likely, group, who are responsible and accountable for the decision under the appropriate governance structure. We emphasize that the interactions of this decision-maker with the real world include appropriate consultations and engagement with stakeholders.
This apparently linear approach from problem to decision implies risk management is organized, that all risks are identified and the processes set in train are carried out with some order, including the monitoring of success. Yet this is obviously rarely the case. Invariably, the process iterates within and between phases as thinking about one issue catalyses further thoughts about other issues or reflections during one phase indicate that other issues should have been considered in an earlier one. Further, problems may be latent, arising at seemingly random times, decisions postponed, and attention of scarce resources diverted to other purposes some way during the process. Moreover, many risks will be interrelated, and will be dealt with on differing timelines and urgencies. Being mindful of these relationships between risks and between their management process can help reduce tensions between them, take advantage of synergies in activities and processes, and avoid inadvertent negative consequences between risks.
Simplistically, decision analytic studies tend to be conducted in one of two modes (Franco and Montibeller, 2010).
• The expert mode in which the analysts work away from the decision-makers, experts and stakeholders, consulting them individually or groups as necessary to gather information. Such studies are common in addressing problems in the Cynefin Known and Knowable spaces. Because such problems occur commonly, well-structured models are relatively easy to build. The analysts' task is mainly to run sophisticated computer codes to explore and analyse the system.
• The facilitated modeling mode in which analysts and decision-makers, accompanied maybe by some experts and stakeholders, meet in one or more workshops to “solve” the problem. Such studies are common in tackling contexts lying in the Cynefin Complex and Chaotic spaces. Initially the emphasis is on understanding the perceptions of the group on what is happening and identifying possible strategies that may be taken up in response, and on the values that will drive their decision-making. Later, quantitative models are built in the presence of the group to capture these and numerical inputs elicited for those quantities that cannot be inferred from “objective” data. The group see and explore the analysis together, before deciding on a course of action.
This rough dichotomy is an oversimplification; many studies involve elements of both. Large projects dealing with complex issues, and integrating across related risks, may begin with several facilitated workshops to explore and identify issues, creating a series of questions. These questions are then explored through sophisticated modeling studies carried out in the expert mode. Later, there may be a return to facilitated modeling to share what has been learnt and evaluate possible strategies, providing guidance to the decision-makers. Some or all of the workshops might be conducted as face-to-face events or remotely (Coakes et al., 2002; French et al., 2009; Nunamaker et al., 2014; Pyrko et al., 2019).
Decision analyses comprise many families of techniques, some with sufficient philosophical and methodological underpinnings to be called a “school”; while others are more collections of techniques with enough common qualities to be grouped together. We have categorized seven broad classes of techniques that support decision making and give details of each approach in Table 3, identifying how they relate to the general considerations in our earlier discussion.
Table 3. Characteristics of the main approaches to decision analysis with respect to their Cynefin context, the manner in which they can be used to address different uncertainties, where they may be used in different phases of the decision-making process, the resources required, and some case studies for further exploring how they might be used.
Bayesian methods provide a structured approach to assembling information around the consequences of choices, either by modeling, analysis of multiple scenarios or structuring deliberation. They can address all types of uncertainties, and are underpinned by axiomatic theoretical bases and powerful computational methods. These most assuredly form a school built on a coherent set of assumptions and philosophical perspectives. Methods can draw in both hard data and expert knowledge weighing them together appropriately. They use the same Bayesian statistical approaches that lie at the heart of many machine learning and artificial intelligence algorithms. Intuitive, graphical interfaces such as decision trees, belief nets and influence diagrams make the methods relatively transparent. Bayesian methods emphasize the auditable, building of consensus. They make explicit the biases (subjectivity) of information, stakeholders and the decision-maker. Traditionally, Bayesian methods use probability to represent uncertainty, multi-attribute utility functions to represent preference and then maximize expected utility to identify an “optimal” decision. As such they apply in the Known and Knowable Spaces. However, the use of multiple scenarios, sensitivity analysis and exploratory decision conferences enable the methods to be applied in the Complex Space (Keeney and Raiffa, 1993; French and Rios Insua, 2000; Smith, 2010; Howard and Abbas, 2016; French, 2020; Workman et al., 2021) (See Table 3a for further details).
Deep uncertainty relates to circumstances in which data are too sparse, experts in too much disagreement or time is too short to model the uncertainty. As such, decision-making under deep uncertainty (DMDU) methods are focused on working in the Complex Space. Approaches here emphasize robustness (“no regrets” options) and the use of scenarios, and often link well with scenario-focused robust Bayesian studies. Indeed, DMDU studies draw in many other approaches to decision analysis, using them to identify robust rather than optimal strategies. DMDU analyses can help decision makers to think contingently and build a more wide-ranging recognition of the risks (Walker et al., 2013; Maier et al., 2016; Marchau et al., 2019; French, 2020; Workman et al., 2021) (See Table 3b for further details).
The process of decision-making can be very complex, extending over time and involving many parties. A range of tools and techniques have grown up to help manage the decision-making process and support risk management and the implementation of the chosen strategy. Some tools organize data and analyses, often being built on a geographic information system. Others manage processes, organizing workflows. Some have inevitably expanded in function to support decision-making itself, even though their primary focus might be on, say, implementation and monitoring risks. They apply in all the Cynefin Spaces. Such tools are closely related to knowledge management systems; knowledge management processes and decision process management differ more in terminology than in substance (Dalkir, 2005; French et al., 2009; Jashapara, 2011) (See Table 3c for further details).
Many of the tools involved in analyzing decisions stem from economic theory and accounting practices: e.g., cost benefit analysis, which seeks to price out all aspects of the consequence of a strategy, or real options theory, which seeks to value financial investments allowing for their risks and the contingent buying and selling. Such methods are perceived as objective when dealing with tangibles, but are more controversial in their valuing of intangibles. Since these methods model uncertainties with probabilities and then work with expectations, they share much in common with Bayesian methods. However, many applications of cost-benefit analysis omit any detailed treatment of uncertainty. Because of the detailed data requirements of these methods, their application is limited to the Known and Knowable Spaces, though there have been some investigations of using real options in the face of deep uncertainty (Neely and de Neufville, 2001; Bedford et al., 2005; Pearce et al., 2006; Hallegatte et al., 2013; Buurman and Babovic, 2016; Boardman et al., 2017) (See Table 3d for further details).
Because of concerns that the statistical accuracy of some data is unknown and that decision-makers and experts cannot make numerical judgements accurately, analyses have been suggested which accept ranges for numerical inputs. While avoiding accuracy issues, weakening the arithmetic also may weaken other foundational assumptions, including some basic principles of rationality. Different types of uncertainty can often be confused, and the analyses can contradict basic probability theory. Interval models of semantic, and imprecision can be useful in exploring ambiguity and value uncertainty, though modeling rather than resolving such uncertainties does not necessary help in decision-making. Some interval methods can be thought of more as sensitivity techniques applied to other decision analytic approaches. Typical approaches here relate to the fuzzy or possibility theory, and evidential reasoning. Interval methods can be applied in the Known, Knowable and Complex Spaces (Shafer, 1976; French, 1984, 1995a; Pedrycz et al., 2011; Xu, 2012) (See Table 3e for further details).
A term covering many approaches: indeed, Bayesian, DMDU and interval methods are sometimes considered multi-criteria decision analyses (MCDA). Some MCDA seek an optimal or best strategy; others form partial rankings, eliminating weak strategies but not discriminating fully between the better ones. Many MCDA methods eschew dealing with uncertainties and focus on modeling and exploring conflicting objectives and balancing these. Some methods have a rather pragmatic basis, although the European School of Multi-Criteria Decision Aid have much firmer philosophical foundations. There are MCDA methods that are appropriate to each of the Known, Knowable and Complex Spaces, though any method may be limited to just one of these spaces. MCDA techniques are especially useful in working with senior decision-makers in setting policy and broad objectives, and in processes of stakeholder engagement (Roy, 1996; Roy and Vanderpooten, 1996; Belton and Stewart, 2002; Bouyssou et al., 2006; Zopounidis and Pardalos, 2010; Velasquez and Hester, 2013; Korhonen and Wallenius, 2020) (See Tables 3f,g for further details).
Soft elicitation, also known as problem structuring, is the process of asking problem owners, experts and stakeholders for the knowledge, perceptions, beliefs, uncertainties and values that a model needs to embody before being populated with numbers. Methods here help in problem formulation, structuring understanding: e.g., cognitive maps, soft OR, soft systems, prompts such as PESTLE and other qualitative tools. The output of soft elicitation can lead to the building of sophisticated quantitative models; and can also structure communications and deliberations with stakeholders. Exploratory data analysis and visual analytics are also relevant. Soft elicitation is, rather obviously, focused on the sense-making and modeling phase of decision making, but it also has enormous advantages in setting the frame for communication between all parties and thus applies in all three phases. Also there are many cases in which the clarity brought by framing the issues well has obviated the need for formal quantitative analysis. These techniques are useful in all of the Cynefin Spaces, though they come to the fore in the Complex and Chaotic Spaces In which sense really needs to be made (Rosenhead and Mingers, 2001; Checkland, 2013; Steed et al., 2013; Bendoly and Clark, 2016; Pyrko et al., 2019; French, 2021) (See Table 3h for further details).
No “one-size-fits-all” tool is available for managing every climate risk or, indeed, managing the same risk but in different contexts, urgencies or availabilities of resources. This section aims to provide a means by which a climate risk manager may appraise the value of different analytic techniques for their situation. We encourage a prospective user of these techniques to consider the nature of the control system they are dealing with, such as described in the box, the Cynefin context in which they find themselves, and the types of uncertainties most conspicuous in their case. Table 3 can then be used to assess the appropriateness, or not, of different groups of techniques described above. The Table lists the various forms of decision analysis, indicating how they manage uncertainties, how they may be used in the different Cynefin contexts, how they fit into the different phases of decision-making and the resources needed in each use. In order to dig deeper into whether an approach may be suitable, citations are given to relevant literature to support our comments. In addition, we cite some relevant case studies in the application of the tools to climate-related risk management. We make no claims of exhaustiveness, and recognize that in identifying these characteristics we are making many subjective choices, but we hope that they offer a constructive guide into the literature that may help problem-owners and analysts find tools potentially valuable in their context.
While once-intractable, Bayesian Methods have made huge strides becoming computationally tractable and transparent to non-specialist users since the last century (Edwards et al., 2007). Moreover, developments in elicitation can be used to address behavioral and cognitive issues that can bias judgemental inputs (Dias et al., 2018; Turkman et al., 2019; Hanea et al., 2020). Many of the other methods evolved before these advances. Thus, Bayesian ideas should not be dismissed on those grounds; the main issue in using them is that they are explicitly subjective, emphasizing transparency, consensus, impartiality, and correspondence to observable reality instead of objectivity (Gelman and Hennig, 2017). Different cultures recognize and value subjectivity and objectivity differently. Some demand that subjective judgements are recognized explicitly, while others only acknowledge objective issues explicitly.
Decisions are based on analyses of the knowledge and information at hand to the decision-maker. The context of the decision process described above influences what can be done in each phase of decision making. Figure 6 illustrates how knowledge and uncertainty of the different subsystems in the example control system for managing the risk of consequences of flooding (Figure 3) influence the Cynefin space that the management problem may fall within, as well as indicating the analytic techniques that may be available.
Figure 6. Illustration of how a management system, including the extent of data and models, will differ between Cynefin contexts, following the example in Box 2. Figure 3 is the Known context on the left. As uncertainty increases according to the Cynefin context (progressive panels toward the right), the ability to evaluate how the risk is changing in the future and to assess the implications of different actions will diminish. These changes are illustrated through change in the uncertainty around each subsystem and the linkages (see Legend in Figure 3) or even understanding what subsystems might be present. The interlinked and iterative processes between the three phases of a management system are shown in the cloud on the left. Text in the symbols are summaries of the text from Figure 3. The application of different approaches to decision analysis (see Section Approaches to Decision Analysis) applicable to the four Cynefin contexts are shown at bottom (solid line = broad application, dashed line = specific methods applicable, dotted line = applicable in some aspects such as in sense-making, double-dash-long-dash line = general useful).
Decision analyses used to support decision-making on climate-related risks shown as case studies in Table 3 were assessed for the circumstances in which they were used. The first dimension of the assessment was the geo-political scale to which the decision was intended to apply—household (or individual), community (village or neighborhood), city (including the greater city jurisdiction), sub-national region (a state, province), nation, trans-national regions (within a continent), international (through global agreements, organizations and the like). This scale differs from the type of body making the decisions, which is reflective of whether the outcome is intended as top-down, autonomous, or bottom-up. Here, a top-down decision is one that applies from a body that is autonomous at higher geopolitical scale to lower scales, whereas a bottom up decision is one made by a body autonomous at lower scales intended to influencing high scale outcomes.
The second dimension relates to the contribution of the technique to decision outcomes. These contributions relate to phases in the decision process but, as described previously, may not be implemented in a set sequence. The types of contributions include:
The results of this assessment are shown in Figure 7. Evidence of the basis of actual decisions and whether decision-analytic techniques were used to support the making of those decisions is difficult to find in the peer-reviewed literature. Most of the case studies were related to theoretical studies with realistic data, reflecting that most literature on climate change is about scenarios and the consequences of those scenarios. Many fewer studies address the actual decision processes of managing climate-related risks. Moreover, the spectrum of different types of contribution to the decision process seem more focussed at subnational/national levels.
Figure 7. A graphic relating case studies to (i) Geopolitical scale of a decision (ii) the commitment to the result of the case study, (iii) the decision-making bodies concerned and (iv) the decision analytic approach used. Symbols represent three components. Colour is the decision analytic approach: red, Bayesian; brown, Decision-Making under Deep Uncertainty; dark blue, Decision Process Management; purple, Economic and Financial Methods; light blue, Interval Methods; light green, full ranking MCDA; dark green, partial ranking MCDA; black, Soft Elicitation. Letters are decision-making bodies: C, Community; G, Government; B, Business/Industry; F, Finance; N, NGO; A, All categories. Numbers in square brackets are the citation: [1] Åström et al. (2014); [2] Alexeeff et al. (2016); [3] Baker and Solak (2011); [4] Batisha (2015); [5] Biehl et al. (2017); [6] Bosomworth et al. (2017); [7] Brown et al. (2019); [8] Butler et al. (2016); [9] Catenacci and Giupponi (2013); [10] de Bruin et al. (2009); [11] de Ruig et al. (2019); [12] El-Zein and Tonmoy (2015); [13] Gilbuena et al. (2013); [14] Groves et al. (2019); [15] Hall et al. (2012); [16] Haque (2016); [17] Jäger et al. (2018); [18] Kim and Chung (2013); [19] Konidari and Mavrakis (2007); [20] Lempert and Groves (2010); [21] Manocha and Babovic (2017); [22] Markl-Hummel and Geldermann (2014); [23] Michailidou et al. (2016); [24] Papathanasiou et al. (2016); [25] Parding et al. (2020); [26] Park et al. (2012); [27] Phan et al. (2019); [28] Prober et al. (2017); [29] Richards et al. (2016); [30] Richards et al. (2013); [31] Sperotto et al. (2017); [32] Sperotto et al. (2019); [33] Streimikiene and Balezentis (2013); [34] Symstad et al. (2017); [35] Taner et al. (2017); [36] Weaver et al. (2013); [37] Xenarios and Polatidis (2015); [38] Yang et al. (2018).
Climate change brings many profound challenges and with them a need to manage a gamut of risks, ranging in scale from very local to global and severity from a relatively simple need to adapt to existential. Addressing these will involve many people, many decision-makers, stakeholders and experts. Some situations may have time and resources for acquiring data, opinions and to test options; others need urgent actions. In consequence, there are many decisions to be made and a great need for modeling and analysis to support these decisions.
In this paper, we have sought to guide policy makers, their advisors and the broader climate change community (scientists, NGOs, advocacy groups) into the literature on decision analysis and the range of tools available to support decision-making. We have sought to emphasize the complexity of decision-making, particularly in the context of time-constrained risk management. We have presented existing approaches and decision analytic tools in a way that we believe will help policy makers find methods that are appropriate to their circumstances. We hope that our paper stimulates their recognition of the complexities involved in the decision-making and at the same time offers constructive suggestions to help develop appropriate decision analyses.
SF and DV conceived of the paper. VK and SF undertook literature review and assessment. AC and DV provided coordination and climate-risk context. All authors contributed to the writing. All authors contributed to the article and approved the submitted version.
DV was employed by CGG, Crawley.
The remaining authors declare that the research was conducted in the absence of any commercial or financial relationships that could be construed as a potential conflict of interest.
All claims expressed in this article are solely those of the authors and do not necessarily represent those of their affiliated organizations, or those of the publisher, the editors and the reviewers. Any product that may be evaluated in this article, or claim that may be made by its manufacturer, is not guaranteed or endorsed by the publisher.
This paper originated in discussions amongst the lead authors in the IPCC Working Group II Chapter 17 on Decision Making. We thank all the lead authors and contributing lead authors in that group for their insights and perspectives that contributed to this paper, and for their dedication to working for the greater good in contributing to the IPCC. The content of the paper grew from the AU4DM catalogue of decision tools (http://au4dmnetworks.co.uk/wp-content/uploads/2020/06/20180503_AU4DM_Shard_workshop_Catalogue.pdf). We are grateful to the AU4DM network for allowing us to use their catalogue as a basis for this work.
Åström, H., Friis Hansen, P., Garrè, L., and Arnbjerg-Nielsen, K. (2014). An influence diagram for urban flood risk assessment through pluvial flood hazards under non-stationary conditions. J. Water Clim. Change. 5, 276–286. doi: 10.2166/wcc.2014.103
Ackermann, F. (2012). Problem structuring methods ‘in the Dock': arguing the case for Soft OR. Eur. J. Operat. Res. 219, 652–658. doi: 10.1016/j.ejor.2011.11.014
Alexeeff, S. E., Pfister, G. G., and Nychka, D. (2016). A Bayesian model for quantifying the change in mortality associated with future ozone exposures under climate change. Biometrics. 72, 281–288. doi: 10.1111/biom.12383
Atkinson, G., Braathen, N. A., Groom, B., and Mourato, S. (2018). Cost-Benefit Analysis and the Environment: Further Developments and Policy Use. Paris, France: OECD Publishing.
Baker, E., and Solak, S. (2011). Climate change and optimal energy technology RandD policy. Eur. J. Operat. Res. 213, 442–454. doi: 10.1016/j.ejor.2011.03.046
Barnett, V. (1999). Comparative Statistical Inference. Chichester: John Wiley and Sons. doi: 10.1002/9780470316955
Batisha, A. F. (2015). Implementing fuzzy decision making technique in analyzing the Nile Delta resilience to climate change. Alexandria Eng. J. 54, 1043–1056. doi: 10.1016/j.aej.2015.05.019
Bayley, C. (2008). “Public Participation,” in E.L. Melnick and B.S. Everitt eds. Encyclopedia of Quantitative Risk Analysis and Assessment, (Chichester: John Wiley and Sons) p. 1383–1391. doi: 10.1002/9780470061596.risk0535
Bedford, T., French, S., and Atherton, E. (2005). Supporting ALARP decision-making by cost benefit analysis and multi-attribute utility theory. J. Risk Res. 8, 207–223. doi: 10.1080/1366987042000192408
Behzadian, M., Kazemzadeh, R. B., Albadvi, A., and Aghdasi, M. (2010). PROMETHEE: a comprehensive literature review on methodologies and applications. Eur. J. Oper. Res. 200, 198–215. doi: 10.1016/j.ejor.2009.01.021
Beierle, T., and Cayford, J. (2002). “Democracy in Practice: Public Participation in Environmental Decisions”. Washington, DC: Routledge.
Bell, M. L., Hobbs, B. F., Elliott, E. M., Ellis, H., and Robinson, Z. (2001). An evaluation of multi-criteria methods in integrated assessment of climate policy. J. Multi-Criteria Decis. Anal. 10, 229–256. doi: 10.1002/mcda.305
Belton, V., and Stewart, T. J. (2002). “Multiple Criteria Decision Analysis: an Integrated Approach”, Boston, MA: Kluwer Academic Press. doi: 10.1007/978-1-4615-1495-4
Bendoly, E., and Clark, S. (2016). Visual Analytics for Management: Translational Science and Applications in Practice,” New York, NY: Taylor and Francis. doi: 10.4324/9781315640891
Berkeley, D., and Humphreys, P. C. (1982). Structuring decision problems and the 'bias heuristic'. Psychol. Bull. 50, 201–252. doi: 10.1016/0001-6918(82)90042-7
Biehl, L. L., Zhao, L., Song, C. X., and Panza, C. G. (2017). Cyberinfrastructure for the collaborative development of U2U decision support tools. Climate Risk Management 15, 90–108. doi: 10.1016/j.crm.2016.10.003
Bindoff, N. L., Stott, P. A., AchutaRao, K. M., Allen, M. R., Gillett, N., Gutzler, D., et al. (2013). “Detection and Attribution of Climate Change: from Global to Regional,” in Climate Change 2013: The Physical Science Basis. Contribution of Working Group I to the Fifth Assessment Report of the Intergovernmental Panel on Climate Change, eds. Stocker, T. F., Qin, D., Plattner, G. K., Tignor, M., Allen, S. K., Boschung, J., et al. (Cambridge, New York, NY: Cambridge University Press) p. 867–952.
Boardman, A. E., Greenberg, D. H., Vining, A. R., and Weimer, D. L. (2017). Cost-Benefit Analysis: Concepts and Practice. Cambridge: Cambridge University Press. doi: 10.1017/9781108235594
Bonczek, R. H., Holsapple, C. W., and Whinston, A. B. (2014). Foundations of Decision Support Systems. New York, NY: Academic Press.
Bosomworth, K., Leith, P., Harwood, A., and Wallis, P. J. (2017). What's the problem in adaptation pathways planning? The potential of a diagnostic problem-structuring approach. Environ. Sci. Policy. 76, 23–28. doi: 10.1016/j.envsci.2017.06.007
Bouyssou, D., Jacquet-Lagrèze, E., Perny, P., Slowiński, R., Vanderpooten, D., and Vincke, P. (2002). Aiding Decisions With Multiple Criteria: Essays in Honor of Bernard Roy. New York, NY: Springer.
Bouyssou, D., Marchant, T., Pirlot, M., Tsoukias, A., and Vincke, P. (2006). Evaluation and Decision Models with Multiple Criteria: Stepping Stones for the Analyst. New York, NY: Springer.
Brown, C., Steinschneider, S., Ray, P., Wi, S., Basdekas, L., and Yates, D. (2019). “Decision Scaling (DS): decision support for climate change,” in Decision Making under Deep Uncertainty (Cham: Springer), 255–287. doi: 10.1007/978-3-030-05252-2_12
Burstein, F., and Holsapple, C.W. (2008). Handbook on Decision Support Systems (in two volumes). Berlin: Springer Verlag. doi: 10.1007/978-3-540-48713-5
Butler, J., Bohensky, E., Suadnya, W., Yanuartati, Y., Handayani, T., Habibi, P., et al. (2016). Scenario planning to leap-frog the sustainable development goals: an adaptation pathways approach. Clim. Risk Manage. 12, 83–99. doi: 10.1016/j.crm.2015.11.003
Buurman, J., and Babovic, V. (2016). Adaptation Pathways and Real Options Analysis: An approach to deep uncertainty in climate change adaptation policies. Policy Soc. 35, 137–150. doi: 10.1016/j.polsoc.2016.05.002
Catenacci, M., and Giupponi, C. (2013). Integrated assessment of sea-level rise adaptation strategies using a Bayesian decision network approach. Environ. Model. Softw. 44, 87–100. doi: 10.1016/j.envsoft.2012.10.010
Checkland, P. (2013). “Soft systems methodology,” in Encyclopedia of Operations Research and Management Science (Boston, MA: Springer), 1430–1436. doi: 10.1007/978-1-4419-1153-7_971
Christensen, R., Johnson, W., Branscum, A., and Hanson, T. (2011). Bayesian Ideas and Data Analysis: An Introduction for Scientists and Statisticians. Boca Raton: CRC press. doi: 10.1201/9781439894798
Coakes, E., Willis, D., and Clarke, S. (2002). Knowledge Management in the SocioTechnical World. London: Springer Verlag. doi: 10.1007/978-1-4471-0187-1
Courtney, H. (2003). Decision-driven scenarios for assessing four levels of uncertainty. Strat. Leaders. 31, 14–22. doi: 10.1108/10878570310455015
Dalkir, K. (2005). Knowledge Management in Theory and Practice. Burlington, MA: Elsevier Butterworth-Heinemann.
de Bruin, K., Dellink, R., Ruijs, A., Bolwidt, L., van Buuren, A., Graveland, J., et al. (2009). Adapting to climate change in The Netherlands: an inventory of climate adaptation options and ranking of alternatives. Clim. Change. 95, 23–45. doi: 10.1007/s10584-009-9576-4
de Ruig, L. T., Barnard, P. L., Botzen, W. W., Grifman, P., Hart, J. F., de Moel, H., et al. (2019). An economic evaluation of adaptation pathways in coastal mega cities: an illustration for Los Angeles. Sci. Total Environ. 678, 647–659. doi: 10.1016/j.scitotenv.2019.04.308
De Smet, Y., and Lidouh, K. (2013). “An introduction to multicriteria decision aid: The PROMETHEE and GAIA methods,” in European Business Intelligence Summer School, eds M. A. Aufaure and E. Zimányi (Berlin: Springer), 150–176. doi: 10.1007/978-3-642-36318-4_7
Dias, L., Morton, A., and Quigley, J. (2018). Elicitation: The Science and Art of Structuring Judgement. Cham: Springer.
Durbach, I. N. (2014). Outranking under uncertainty using scenarios. Eur. J. Oper. Res. 232, 98–108. doi: 10.1016/j.ejor.2013.06.041
Durbach, I. N., and Stewart, T. J. (2020). “Probability and beyond: including uncertainties in decision analysis,” in Behavioral Operational Research, eds L. White, M. Kunc, K. Burger, and J. Malpass (Cham: Springer), 75–91. doi: 10.1007/978-3-030-25405-6_5
Edwards, W., Miles, R. F., and Von Winterfeldt, D. (2007). Advances in Decision Analysis: from Foundations to Applications. Cambridge: Cambridge University Press. doi: 10.1017/CBO9780511611308
El-Zein, A., and Tonmoy, F. N. (2015). Assessment of vulnerability to climate change using a multi-criteria outranking approach with application to heat stress in Sydney. Ecol. Indicat. 48, 207–217. doi: 10.1016/j.ecolind.2014.08.012
Evans, J. S. B., and Stanovich, K. E. (2013). Dual-process theories of higher cognition: advancing the debate. J. Perspect. Psychol. Sci. 8, 223–241. doi: 10.1177/1745691612460685
Fekete, J.-D., and Primet, R. (2016). Progressive analytics: a computation paradigm for exploratory data analysis. arXiv[Preprint]. arXiv:1607.05162. doi: 10.48550/arXiv.1607.05162
Figueira, J. R., Mousseau, V., and Roy, B. (2016). “ELECTRE methods,” in Multiple Criteria Decision Analysis, eds S. Greco, M. Ehrgott, and J. Figueira (New York, NY: Springer), 155–185. doi: 10.1007/978-1-4939-3094-4_5
Fischhoff, B. (2015). The realities of risk-cost-benefit analysis. Science 350, aaa6516. doi: 10.1126/science.aaa6516
Franco, L. A., and Montibeller, G. (2010). Facilitated modelling in operational research. Eur. J. Oper. Res. 205, 489–500. doi: 10.1016/j.ejor.2009.09.030
French, S. (1984). “Fuzzy decision analysis: some criticisms,” in Fuzzy Sets and Decision Analysis, eds H. J. Zimmermann, L. A. Zadeh, and B. R. Gaines (Amsterdam: North Holland).
French, S. (1995a). An introduction to decision theory and prescriptive decision analysis. IMA J. Mathem. Appl. Bus. Ind. 6, 239–247. doi: 10.1093/imaman/6.2.239
French, S. (1995b). Uncertainty and imprecision: modelling and analysis. J. Operat. Res. Soc. 46, 70–79. doi: 10.1057/jors.1995.8
French, S. (2003). Modelling, making inferences and making decisions: the roles of sensitivity analysis. TOP. 11, 229–252. doi: 10.1007/BF02579043
French, S. (2013). Cynefin, Statistics and Decision Analysis. J. Operat. Res. Soc. 64, 547–561. doi: 10.1057/jors.2012.23
French, S. (2020). Axiomatising the bayesian paradigm in parallel small worlds. Operat. Res. doi: 10.1287/opre.2019.1896. [Epub ahead of print].
French, S. (2021). From soft to hard elicitation. J. Operat. Res. Soc. 1–17. doi: 10.1080/01605682.2021.1907244. [Epub ahead of print].
French, S., and Argyris, N. (2018). Decision analysis and political processes. Decis. Analy. 15, 208–222. doi: 10.1287/deca.2018.0374
French, S., and Geldermann, J. (2005). The varied contexts of environmental decision problems and their implications for decision support. Environ. Sci. Policy. 8, 378–391. doi: 10.1016/j.envsci.2005.04.008
French, S., Haywood, S., Oughton, D. H., and Turcanu, C. (2020). Different types of uncertainty in nuclear emergency management. Radioprotection 55, S175–S180. doi: 10.1051/radiopro/2020029
French, S., Maule, A. J., and Mythen, G. (2005). Soft Modelling in Risk Communication and Management: Examples in Handling Food Risk. J. Operat. Res. Soc. 56, 879–888. doi: 10.1057/palgrave.jors.2601901
French, S., Maule, A. J., and Papamichail, K. N. (2009). Decision Behaviour, Analysis and Support. Cambridge: Cambridge University Press. doi: 10.1017/CBO9780511609947
Gelman, A. (2003). A Bayesian formulation of exploratory data analysis and goodness-of-fit testing. Int. Statist. Rev. 71, 369–382. doi: 10.1111/j.1751-5823.2003.tb00203.x
Gelman, A., Carlin, J. B., Stern, H. S., Dunson, D. B., Vehtari, A., and Rubin, D. B. (2013). Bayesian Data Analysis. London: Chapman and Hall. doi: 10.1201/b16018
Gelman, A., and Hennig, C. (2017). Beyond subjective and objective in statistics. J. R. Statis. Soc A 180, 967–1033. doi: 10.1111/rssa.12276
Gervásio, H., and Da Silva, L. S. (2012). A probabilistic decision-making approach for the sustainable assessment of infrastructures. Expert Syst. Applic. 39, 7121–7131. doi: 10.1016/j.eswa.2012.01.032
Gilbuena, R., Kawamura, A., Medina, R., Nakagawa, N., and Amaguchi, H. (2013). Environmental impact assessment using a utility-based recursive evidential reasoning approach for structural flood mitigation measures in Metro Manila, Philippines. J. Environ. Manage. 131, 92–102. doi: 10.1016/j.jenvman.2013.09.020
Goodwin, P., and Wright, G. (2014). Decision Analysis for Management Judgement. Chichester: John Wiley and Sons.
Govindan, K., and Jepsen, M. B. (2016). ELECTRE: A comprehensive literature review on methodologies and applications. Eur. J. Oper. Res. 250, 1–29. doi: 10.1016/j.ejor.2015.07.019
Gregory, R. S., Failing, L., Harstone, M., Long, G., McDaniels, T., and Ohlson, D. (2012). Structured Decision Making: A Practical Guide to Environmental Management Choices. Chichester: Wiley-Blackwell. doi: 10.1002/9781444398557
Groves, D. G., Molina-Perez, E., Bloom, E., and Fischbach, J. R. (2019). “Robust Decision Making (RDM): Application to Water Planning and Climate Policy,” in Decision Making under Deep Uncertainty, eds V. Marchau, W. Walker, P. Bloemen, and S. Popper (Cham: Springer), 135–163. doi: 10.1007/978-3-030-05252-2_7
Gunawan, S., and Azarm, S. (2005). Multi-objective robust optimization using a sensitivity region concept. Struct. Multidiscipl. Optimiz. 29, 50–60. doi: 10.1007/s00158-004-0450-8
Gutjahr, W. J., and Pichler, A. (2016). Stochastic multi-objective optimization: a survey on non-scalarizing methods. Ann. Operat. Res. 236, 475–499. doi: 10.1007/s10479-013-1369-5
Hall, J. W., Lempert, R. J., Keller, K., Hackbarth, A., Mijere, C., and McInerney, D. J. (2012). Robust climate policies under uncertainty: a comparison of robust decision making and info-gap methods. Risk Analy. Int. J. 32, 1657–1672. doi: 10.1111/j.1539-6924.2012.01802.x
Hallegatte, S., Shah, A., Lempert, R., Brown, C., and Gill, S. (2013). Investment decision making under deep uncertainty-application to climate change. Policy Research Working Papers. The World Bank, P. 41. doi: 10.1596/1813-9450-6193
Hanea, A., Nane, G. F., Bedford, T., and French, S. (2020). Expert Judgement in Risk and Decision Analysis. Switzerland: Springer. doi: 10.1007/978-3-030-46474-5
Haque, A. N. (2016). Application of multi-criteria analysis on climate adaptation assessment in the context of least developed countries. J. Multi-Criteria Decis. Analy. 23, 210–224. doi: 10.1002/mcda.1571
Hennig, P., Osborne, M. A., and Girolami, M. (2015). Probabilistic numerics and uncertainty in computations. Proc. R. Soc. A: R. Soc. 471, 20150142. doi: 10.1098/rspa.2015.0142
Hodgkinson, G., and Starbuck, W., (eds.). (2008). The Oxford Handbook of Organizational Decision Making. Oxford: Oxford University Press. doi: 10.1093/oxfordhb/9780199290468.001.0001
Holsapple, C., Sena, M., and Wagner, W. (2019). The perceived success of ERP systems for decision support. Inf. Technol. Manage. 20, 1–7. doi: 10.1007/s10799-017-0285-9
Howell, S., Stark, A., Newton, D., Paxson, D., Carvus, M., and Pereira, J. (2001). Real Options: Evaluating Corporate Investment Opportunities in a Dynamic World. Harlow: FT Prentice Hall.
Hyde, K., Maier, H. R., and Colby, C. (2003). Incorporating uncertainty in the PROMETHEE MCDA method. J. Multi-Criter. Decis. Analy. 12, 245–259. doi: 10.1002/mcda.361
Iooss, B., and Saltelli, A. (2017). “Introduction to sensitivity analysis,” in Handbook of Uncertainty Quantification. (Cham: Springer). doi: 10.1007/978-3-319-12385-1_31
IPCC (2018). Global Warming of 1.5°C. An IPCC Special Report on the impacts of global warming of 1.5°C above pre-industrial levels and related global greenhouse gas emission pathways, in the context of strengthening the global response to the threat of climate change,sustainable development, and efforts to eradicate poverty.
Jäger, W., Christie, E., Hanea, A., den Heijer, C., and Spencer, T. (2018). A Bayesian network approach for coastal risk analysis and decision making. Coastal Eng. 134, 48–61. doi: 10.1016/j.coastaleng.2017.05.004
Kahneman, D., and Tversky, A. (1974). Judgement under uncertainty: heuristics and biases. Science 185, 1124–1131. doi: 10.1126/science.185.4157.1124
Keeney, R. L. (1992). Value-Focused Thinking: a Path to Creative Decision Making. Cambridge: Harvard University Press.
Keeney, R. L., and Raiffa, H. (1993). Decisions With Multiple Objectives: Preferences and Value Trade-offs. Cambridge: Cambridge University Press. doi: 10.1017/CBO9781139174084
Kim, Y., and Chung, E.-S. (2013). Fuzzy VIKOR approach for assessing the vulnerability of the water supply to climate change and variability in South Korea. Appl. Mathem Modell. 37, 9419–9430. doi: 10.1016/j.apm.2013.04.040
Klamroth, K., Knowles, J. D., Rudolph, G., and Wiecek, M. M. (2018). Personalized Multiobjective Optimization: An Analytics Perspective (Dagstuhl Seminar 18031). Schloss Dagstuhl, Wadern, Germany.
Knight, F. H. (1921). Risk, Uncertainty and Profit. Boston, MA: Hart, Schaffner and Marx; Houghton Mifflin Company.
Konidari, P., and Mavrakis, D. (2007). A multi-criteria evaluation method for climate change mitigation policy instruments. Energy Policy 35, 6235–6257. doi: 10.1016/j.enpol.2007.07.007
Korhonen, P. J., and Wallenius, J. (2020). Making Better Decisions. Cham: Springer. doi: 10.1007/978-3-030-49459-9
Kumar, A., Sah, B., Singh, A. R., Deng, Y., He, X., Kumar, P., et al. (2017). A review of multi criteria decision making (MCDM) towards sustainable renewable energy development. Renew. Sustain. Energy Rev. 69, 596–609. doi: 10.1016/j.rser.2016.11.191
Lempert, R. J., and Groves, D. G. (2010). Identifying and evaluating robust adaptive policy responses to climate change for water management agencies in the American west. Technol. Forec. Soc. Change 77, 960–974. doi: 10.1016/j.techfore.2010.04.007
Lichtenstein, S., and Slovic, P. (2006). The Construction of Preference. Cambridge: Cambridge University Press. doi: 10.1017/CBO9780511618031
Maier, H. R., Guillaume, J. H. A., van Delden, H., Riddell, G. A., Haasnoot, M., and Kwakkel, J. H. (2016). An uncertain future, deep uncertainty, scenarios, robustness and adaptation: how do they fit together? Environ. Model. Softw. 81, 154–164. doi: 10.1016/j.envsoft.2016.03.014
Manocha, N., and Babovic, V. (2017). Development and valuation of adaptation pathways for storm water management infrastructure. Environ. Sci. Policy. 77, 86–97. doi: 10.1016/j.envsci.2017.08.001
Marchau, V., Walker, W.E., Bloemen, P., and Popper, S. (2019). Decision Making under Deep Uncertainty. Springer. doi: 10.1007/978-3-030-05252-2
Markl-Hummel, L., and Geldermann, J. (2014). A local-level, multiple criteria decision aid for climate protection. EURO J. Decis. Proces. 2, 121–152. doi: 10.1007/s40070-013-0011-8
Marttunen, M., Lienert, J., and Belton, V. (2017). Structuring problems for multi-criteria decision analysis in practice: a literature review of method combinations. Eur. J. Oper. Res. 263, 1–17. doi: 10.1016/j.ejor.2017.04.041
Massingham, P. (2010). Knowledge risk management: a framework. J. Knowl. Manage. 14, 464–485. doi: 10.1108/13673271011050166
Melbourne-Thomas, J., Constable, A., Wotherspoon, S., and Raymond, B. (2013). Testing paradigms of ecosystem change under climate warming in Antarctica. PLoS ONE. 8, e55093. doi: 10.1371/journal.pone.0055093
Michailidou, A. V., Vlachokostas, C., and Moussiopoulos, N. (2016). Interactions between climate change and the tourism sector: Multiple-criteria decision analysis to assess mitigation and adaptation options in tourism areas. Tour. Manage. 55, 1–12. doi: 10.1016/j.tourman.2016.01.010
Montibeller, G., and Winterfeldt, D. (2015). Cognitive and motivational biases in decision and risk analysis. Risk Analy. 35, 1230–51. doi: 10.1111/risa.12360
Morgan, B. J. (2008). Applied Stochastic Modelling, 2nd Edn. Boca Raton, FL: CRC Press. doi: 10.1201/b17188
Morton, A., Airoldi, M., and Phillips, L. D. (2009). Nuclear risk management on stage: a decision analysis perspective on the UK's Committee on Radioactive Waste Management. Risk Analy. 29, 764–779. doi: 10.1111/j.1539-6924.2008.01192.x
Morton, A., and Fasolo, B. (2009). Behavioural decision theory for multi-criteria decision analysis: a guided tour 60(2): 268-275. J. Operat. Res. Soc. 60, 268–275. doi: 10.1057/palgrave.jors.2602550
Mustajoki, J., Hämäläinen, R. P., and Marttunen, M. (2004). Participatory multi-criteria decision analysis with Web-Hipre: a case of lake regulation policy. Environ. Model. Softw. 19, 537–547. doi: 10.1016/j.envsoft.2003.07.002
Neely, J. E., and de Neufville, R. (2001). Hybrid real options valuation of risky product development projects. Int. J. Technol Policy Manage. 1, 29–46. doi: 10.1504/IJTPM.2001.001743
Nunamaker, J. F., Briggs, R. O., and Romano Jr, N. C. R. (2014). Collaboration Systems: Concept, Value, and Use. New York: Routledge. doi: 10.4324/9781315705569
OECD. (2018). Cost-Benefit Analysis and the Environment. oecd.org: Organisation for Economic Co-operation and Development. doi: 10.1787/9789264085169-en
Orlove, B., Shwom, R., Markowitz, E., and Cheong, S.-M. (2020). Climate decision-making. Ann. Rev. Environ. Resour. 45, 271–303. doi: 10.1146/annurev-environ-012320-085130
Papathanasiou, J., Ploskas, N., and Linden, I. (2016). Real-World Decision Support Systems: Case Studies. Springer. doi: 10.1007/978-3-319-43916-7
Parding, K. M., Dobler, A., McSweeney, C. F., Landgren, O. A., Benestad, R., Erlandsen, H. B., et al. (2020). GCMeval–An interactive tool for evaluation and selection of climate model ensembles. Clim. Serv. 18, 100167. doi: 10.1016/j.cliser.2020.100167
Park, S. E., Marshall, N. A., Jakku, E., Dowd, A. M., Howden, S. M., Mendham, E., et al. (2012). Informing adaptation responses to climate change through theories of transformation. Global Environ. Change. 22, 115–126. doi: 10.1016/j.gloenvcha.2011.10.003
Paté-Cornell, M. E. (1996). Uncertainties in risk analysis: Six levels of treatment. Reliabil. Eng. Syst Safet. 54, 95–111. doi: 10.1016/S0951-8320(96)00067-1
Patrício, J., Elliott, M., Mazik, K., Papadopoulou, K.-N., and Smith, C. J. (2016). DPSIR—two decades of trying to develop a unifying framework for marine environmental management? Front. Marine Sci. 3, 177. doi: 10.3389/fmars.2016.00177
Pearce, D., Atkinson, G., and Mourato, S. (2006). Cost-benefit Analysis and the Environment: Recent Developments. Organisation for Economic Co-operation and development.
Pedrycz, W., Ekel, P., and Parreiras, R. (2011). Fuzzy multicriteria decision-making: models, methods and applications. New York, NY: John Wiley and Sons. doi: 10.1002/9780470974032
Phan, T. D., Smart, J. C., Stewart-Koster, B., Sahin, O., Hadwen, W. L., Dinh, L. T., et al. (2019). Applications of bayesian networks as decision support tools for water resource management under climate change and socio-economic stressors: a critical appraisal. Water 11, 2642. doi: 10.3390/w11122642
Phillips, L. D. (2007). “Decision Conferencing,” in Advances in Decision Analysis: From Foundations to Applications, eds D. von Winterfeldt, R. F. Miles Jr, and W. Edwards (Cambridge: Cambridge University Press) p. 375–399. doi: 10.1017/CBO9780511611308.020
Prober, S. M., Colloff, M. J., Abel, N., Crimp, S., Doherty, M. D., Dunlop, M., et al. (2017). Informing climate adaptation pathways in multi-use woodland landscapes using the values-rules-knowledge framework. Agric. Ecosyst. Environ. 241, 39–53. doi: 10.1016/j.agee.2017.02.021
Pyrko, I., Eden, C., and Howick, S. (2019). Knowledge acquisition using group support systems. Group Decis. Negot. 28, 233–253. doi: 10.1007/s10726-019-09614-9
Raz, T., and Michael, E. (2001). Use and benefits of tools for project risk management. Int. J. Project Manage. 19, 9–17. doi: 10.1016/S0263-7863(99)00036-8
Reilly, T., and Clemen, R. T. (2013). Making Hard Decisions with Decision Tools. Boston, MA: South Western College Publishing.
Richards, R., San,ó, M., Roiko, A., Carter, R. W., Bussey, M., Matthews, J., et al. (2013). Bayesian belief modeling of climate change impacts for informing regional adaptation options. Environ. Model. Softw. 44, 113–121. doi: 10.1016/j.envsoft.2012.07.008
Richards, R., Sano, M., and Sahin, O. (2016). Exploring climate change adaptive capacity of surf life saving in Australia using Bayesian belief networks. Ocean Coastal Manage. 120, 148–159. doi: 10.1016/j.ocecoaman.2015.11.007
Rios Insua, D. (1990). Sensitivity Analysis in Multi-Objective Decision Making. Berlin: Springer Verlag. doi: 10.1007/978-3-642-51656-6
Rios Insua, D., and French, S. (2010). Democracy: a Group Decision and Negotiation Perspective. Dordrecht: Springer. doi: 10.1007/978-90-481-9045-4
Rios Insua, D., and Ruggeri, F. (2000). Robust Bayesian Analysis. New York: Springer-Verlag. doi: 10.1007/978-1-4612-1306-2
Rosenhead, J., and Mingers, J. (2001). Rational Analysis for a Problematic World Revisited. Chichester: John Wiley and Sons.
Roy, B. (1996). Multi-Criteria Modelling for Decision Aiding. Dordrecht: Kluwer Academic Publishers. doi: 10.1007/978-1-4757-2500-1
Roy, B., and Vanderpooten, D. (1996). The European School of MCDA: emergence, basic features, and current works. J. Multi-Criteria Decis. Analy. 5, 22–36. doi: 10.1002/(SICI)1099-1360(199603)5:1<22::AID-MCDA93>3.0.CO;2-F
Saarikoski, H., Mustajoki, J., Barton, D. N., Geneletti, D., Langemeyer, J., Gomez-Baggethun, E., et al. (2016). Multi-Criteria Decision Analysis and Cost-Benefit Analysis: Comparing alternative frameworks for integrated valuation of ecosystem services. Ecosyst. Serv. 22, 238–249. doi: 10.1016/j.ecoser.2016.10.014
Saaty, T. L. (1980). The Analytical Hierarchy Process. New York: McGraw-Hill. doi: 10.21236/ADA214804
Shafer, G. (1976). A Mathematical Theory of Evidence. Princeton university press. doi: 10.1515/9780691214696
Shaw, D., Franco, A., and Westcombe, M. (2006). Special issue: problem structuring methods I: new directions in a problematic world. J. Operat. Res. Soc. 57, 757–758. doi: 10.1057/palgrave.jors.2602193
Shaw, D., Franco, A., and Westcombe, M. (2007). Special issue: problem structuring methods II: Taking problem structuring methods forward. J. Operat. Res. Soc. 58, 545–546. doi: 10.1057/palgrave.jors.2602366
Shleifer, A. (2012). Psychologists at the gate: a review of daniel kahneman's “thinking, fast and slow”. J. Econ. Literat. 50, 1080–1091. doi: 10.1257/jel.50.4.1080
Simpson, N. P., Mach, K. J., Constable, A., Hess, J., Hogarth, R., Howden, M., et al. (2021). A framework for complex climate change risk assessment. One Earth. 4, 489–501. doi: 10.1016/j.oneear.2021.03.005
Smith, J. Q. (2010). Bayesian Decision Analysis: Principles and Practice. Cambridge: Cambridge University Press. doi: 10.1017/CBO9780511779237
Snowden, D. (2002). Complex acts of knowing - paradox and descriptive self-awareness. J. Knowl. Manage. 6, 100–111. doi: 10.1108/13673270210424639
Sperotto, A., Molina, J., Torresan, S., Critto, A., Pulido-Velazquez, M., and Marcomini, A. (2019). A Bayesian Networks approach for the assessment of climate change impacts on nutrients loading. Environ. Sci. Policy. 100, 21–36. doi: 10.1016/j.envsci.2019.06.004
Sperotto, A., Molina, J.-L., Torresan, S., Critto, A., and Marcomini, A. (2017). Reviewing Bayesian Networks potentials for climate change impacts assessment and management: A multi-risk perspective. J. Environ. Manage. 202, 320–331. doi: 10.1016/j.jenvman.2017.07.044
Steed, C. A., Ricciuto, D. M., Shipman, G., Smith, B., Thornton, P. E., Wang, D., et al. (2013). Big data visual analytics for exploratory earth system simulation analysis. Comput. Geosci. 61, 71–82. doi: 10.1016/j.cageo.2013.07.025
Stewart, T. J., French, S., and Rios, J. (2013). Integration of multicriteria decision analysis and scenario planning. Omega. 41, 679–688. doi: 10.1016/j.omega.2012.09.003
Streimikiene, D., and Balezentis, T. (2013). Multi-objective ranking of climate change mitigation policies and measures in Lithuania. Renew. Sustain. Energy Rev. 18, 144–153. doi: 10.1016/j.rser.2012.09.040
Symstad, A. J., Fisichelli, N. A., Miller, B. W., Rowland, E., and Schuurman, G. W. (2017). Multiple methods for multiple futures: Integrating qualitative scenario planning and quantitative simulation modeling for natural resource decision making. Clim. Risk Manage. 17, 78–91. doi: 10.1016/j.crm.2017.07.002
Tabari, H. (2020). Climate change impact on flood and extreme precipitation increases with water availability. Sci. Rep. 10, 13768. doi: 10.1038/s41598-020-70816-2
Taner, M. Ü., Ray, P., and Brown, C. (2017). Robustness-based evaluation of hydropower infrastructure design under climate change. Clim. Risk Manage. 18, 34–50. doi: 10.1016/j.crm.2017.08.002
Turkman, M. A. A., Paulino, C. D., and Müller, P. (2019). Computational Bayesian Statistics: An Introduction. Cambridge: Cambridge University Press. doi: 10.1017/9781108646185
Tzeng, G.-H., and Huang, J.-J. (2011). Multiple Attribute Decision Making: Methods and Applications. CRC press. doi: 10.1201/b11032
Vanos, J. K., Baldwin, J. W., Jay, O., and Ebi, K. L. (2020). Simplicity lacks robustness when projecting heat-health outcomes in a changing climate. Nat. Commun. 11, 6079. doi: 10.1038/s41467-020-19994-1
Velasquez, M., and Hester, P. T. (2013). An analysis of multi-criteria decision making methods. Int. J. Operat. Res. 10, 56–66.
Walker, W. E., Lempert, R. J., and Kwakkel, J. H. (2013). “Deep uncertainty,” in Encyclopedia of operations research and management science, eds. S. Gass and M.C. Fu. (New York: Springer) 395–402. doi: 10.1007/978-1-4419-1153-7_1140
Weaver, C. P., Lempert, R. J., Brown, C., Hall, J. A., Revell, D., and Sarewitz, D. (2013). Improving the contribution of climate model information to decision making: the value and demands of robust decision frameworks. Wiley Interdiscipl. Rev. 4, 39–60. doi: 10.1002/wcc.202
Workman, M., Darch, G., Dooley, K., Lomax, G., Maltby, J., and Pollitt, H. (2021). Climate policy decision making in contexts of deep uncertainty - from optimisation to robustness. Environ. Sci Policy. 120, 127–137. doi: 10.1016/j.envsci.2021.03.002
Xenarios, S., and Polatidis, H. (2015). Alleviating climate change impacts in rural Bangladesh: a PROMETHEE outranking-based approach for prioritizing agricultural interventions. Environ. Develop. Sustain. 17, 963–985. doi: 10.1007/s10668-014-9583-0
Xu, D.-L. (2012). An introduction and survey of the evidential reasoning approach for multiple criteria decision analysis. Ann Operat. Res. 195, 163–187. doi: 10.1007/s10479-011-0945-9
Yang, Z., Ng, A. K., Lee, P. T.-W., Wang, T., Qu, Z., Rodrigues, V. S., et al. (2018). Risk and cost evaluation of port adaptation measures to climate change impacts. Transp. Res. Part D: Transp. Environ. 61, 444–458. doi: 10.1016/j.trd.2017.03.004
Zhang, M.-J., Wang, Y.-M., Li, L.-H., and Chen, S.-Q. (2017). A general evidential reasoning algorithm for multi-attribute decision analysis under interval uncertainty. Eur. J. Oper. Res. 257, 1005–1015. doi: 10.1016/j.ejor.2016.08.028
Keywords: climate change, risk management, climate governance, cynefin, climate mitigation, climate adaptation, decision analysis
Citation: Constable AJ, French S, Karoblyte V and Viner D (2022) Decision-Making for Managing Climate-Related Risks: Unpacking the Decision Process to Avoid “Trial-and-Error” Responses. Front. Clim. 4:754264. doi: 10.3389/fclim.2022.754264
Received: 06 August 2021; Accepted: 02 May 2022;
Published: 11 July 2022.
Edited by:
Zita Sebesvari, United Nations University, JapanReviewed by:
Luis E. Pineda, Yachay Tech University, EcuadorCopyright © 2022 Constable, French, Karoblyte and Viner. This is an open-access article distributed under the terms of the Creative Commons Attribution License (CC BY). The use, distribution or reproduction in other forums is permitted, provided the original author(s) and the copyright owner(s) are credited and that the original publication in this journal is cited, in accordance with accepted academic practice. No use, distribution or reproduction is permitted which does not comply with these terms.
*Correspondence: Andrew J. Constable, YS5jb25zdGFibGVAdXRhcy5lZHUuYXU=
Disclaimer: All claims expressed in this article are solely those of the authors and do not necessarily represent those of their affiliated organizations, or those of the publisher, the editors and the reviewers. Any product that may be evaluated in this article or claim that may be made by its manufacturer is not guaranteed or endorsed by the publisher.
Research integrity at Frontiers
Learn more about the work of our research integrity team to safeguard the quality of each article we publish.