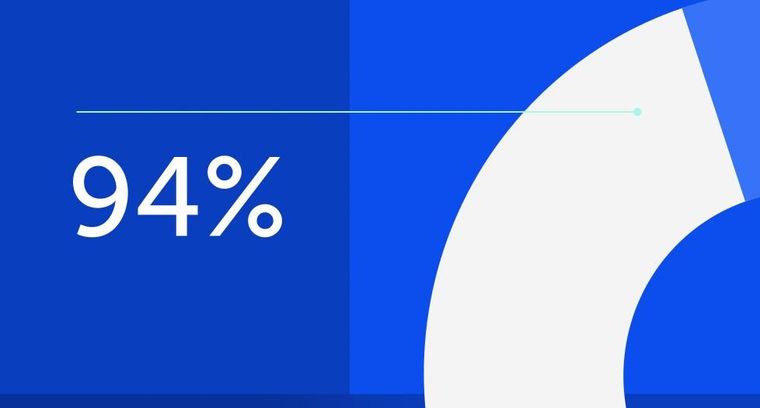
94% of researchers rate our articles as excellent or good
Learn more about the work of our research integrity team to safeguard the quality of each article we publish.
Find out more
ORIGINAL RESEARCH article
Front. Clim., 11 March 2021
Sec. Carbon Dioxide Removal
Volume 3 - 2021 | https://doi.org/10.3389/fclim.2021.647276
This article is part of the Research TopicDeveloping and Deploying Negative Emission Technologies: System-level Assessment and Rationalization View all 7 articles
Carbon dioxide removal (CDR) from the atmosphere is likely to be needed to limit global warming to 1.5 or 2°C and thereby for meeting the Paris Agreement. There is a debate which methods are most suitable and cost-effective for this goal and thus deeper understanding of system effects related to CDR are needed for effective governance of these technologies. Bio-Energy with Carbon Capture and Storage (BECCS) and Direct Air Carbon Capture and Storage (DACCS) are two CDR methods, that have a direct relation to the electricity system—BECCS via producing it and DACCS via consuming. In this work, we investigate how BECCS and DACCS interact with an intermittent electricity system to achieve net negative emissions in the sector using an energy system model and two regions with different wind and solar resource conditions. The analysis shows that DACCS has a higher levelized cost of carbon (LCOC) than BECCS, implying that it is less costly to capture CO2 using BECCS under the assumptions made in this study. However, due to a high levelized cost of electricity (LCOE) produced by BECCS, the total system cost is lower using DACCS as negative emission provider as it is more flexible and enables cheaper electricity production from wind and solar PV. We also find that the replacement effect outweighs the flexibility effect. Since variations in solar-based systems are more regular and shorter (daily cycles), one could assume that DACCS is better suited for such systems, whereas our results point in the opposite direction showing that DACCS is more competitive in the wind-based systems. The result is sensitive to the price of biomass and to the amount of negative emissions required from the electricity sector. Our results show that the use of the LCOC as often presented in the literature as a main indicator for choosing between different CDR options might be misleading and that broader system effects need to be considered for well-grounded decisions.
If the increase in global warming is to be restricted to less than 2°C with reasonable certainty, global greenhouse gas emissions must decrease by roughly half by the mid-21st century, as compared to the current levels, and continue to decline thereafter (Rogelj et al., 2013). To achieve the 1.5°C target set by the Paris Agreement, negative emissions will likely be needed in the second half of the century, to compensate for the emissions in the first part of the century or for sectors that are difficult to mitigate completely, such as agriculture (Fuss et al., 2014). Several ways exist to provide negative emissions: Bio-Energy with Carbon Capture and Storage (BECCS), Direct Air Carbon Capture and Storage (DACCS), afforestation, enhanced weathering etc. Captured CO2 from biomass or air could also be used for production of fuels and material, however, their lifetime tends to be short leading to CO2 being released to the atmosphere almost immediately and are thus not considered as negative emissions. For stringent climate scenarios BECCS together with afforestation has been seen as a main way of enabling negative CO2 emissions. For example, the median amount of electricity provided by BECCS in the Intergovernmental Panel on Climate Change's (IPCCs) AR5 scenarios likely to achieve the 2°C target is 8 EJ in Year 2050 globally. DACCS on the other hand is only emerging as a negative emissions option in global climate scenarios (e.g., Realmonte et al., 2019). Although several technologies have the potential to enable negative emissions, BECCS has the advantage of simultaneously providing benefits other than mitigation (e.g., electricity and heat, biofuels, or pulp and paper). However, biomass is a limited resource and there are significant uncertainties related to how much of it can be provided to the energy system in a sustainable manner or without having a negative effect on other systems such as food supply or biodiversity (Slade et al., 2014; Creutzig et al., 2015). Furthermore, it is uncertain as to where in the energy system the available biomass should be used as there are several hard to abate sectors such as aviation or the chemical industry that may need biomass as feedstock. In addition, building a transport infrastructure for CO2 can be difficult and costly. This has prompted interest in DACCS that does not have to be coupled with an emission source and can thus be placed near a storage location. Creutzig et al. (2019) also point out the modularity and related potential for fast learning as benefits of DACCS compared to BECCS. In addition, negative emissions from DACCS can be more easily verified, whereas to certify negative emissions from BECCS, the whole value chain must be evaluated. On the other hand, DACCS does not produce additional benefits besides negative emissions.
DACCS captures CO2 from ambient air via a contactor to then release it into a relatively pure stream in a regeneration step. DACCS has the disadvantages of using air with very low CO2 concentration (ca 400 ppm), compared to combined heat and power plants (CHPs) and power plants where CO2 concentration in the exhaust can be about 20% (Garðarsdóttir et al., 2015). Two main types of DACCS technologies exist, a high temperature (HT) system using a liquid sorbent to capture the CO2 and a low temperature (LT) system using a solid sorbent (Fasihi et al., 2019). Both systems have a thermal and electrical energy demand. The HT system is based on more developed carbon capture and storage (CCS) technology and the system has a large thermal energy demand where a temperature of ~900°C is needed to regenerate the sorbent (Negative Emissions Technologies Reliable Sequestration: A Research Agenda, 2018). The high temperature in DACCS is usually achieved through natural gas combustion, but it could also be attained through combustion of biogas or by using a fully electrified system through an electric arc furnace. The LT system could use moisture, low-grade heat, vacuum or pressure to regenerate the sorbent (Fasihi et al., 2019). This system could be fully electrified by using a heat pump to provide for the low-grade heat for the regeneration process. As can be seen, both BECCS and DACCS have a connection to the electricity system—BECCS via the opportunity to produce electricity and DACCS via consuming it. Large amounts of electricity could be required if DACCS is implemented on a large scale and its heat demand electrified.
Significant changes are on the way in the electricity system. In recent years, the share of low-carbon electricity generation from wind and solar sources has expanded pointedly, and it is expected to continue to do so in the coming decades owing to lowered costs and policy incentives that are fuelled by climate and energy security concerns. However, large-scale expansion of wind and solar power creates a new set of challenges. The energy supplied from wind and solar technologies is variable in both the short and long terms. High levels of wind and solar power complicate systems operation by changing the shape of the residual load and exacerbating the uncertainty of supply. On the one hand, if significant amounts of intermittent capacity are installed in the system there may be an over-supply of electricity on windy and sunny days, which would result in periods of low electricity prices. On the other hand, when wind and solar power production is too low to meet the demand, other power plants must be deployed. Their full-load hours will, however, be reduced by wind and solar infeed, while requirements in relation to flexibility will increase compared to current thermal generation. Thus, the variability of solar and wind generation can be expected to have a strong influence on investment decisions in the electricity generation system, including investments in BECCS, over the coming decades but also on technologies using electricity as a fuel such as electrified DACCS.
When comparing negative emissions technologies, the cost of carbon per ton of captured CO2 is often used as a measurement (Fuss et al., 2018). Whereas this measure may give an indication about the competitiveness of the technology, it also omits the context of the energy system these technologies are placed in and the effects they may have on the optimal system composition. Previous literature has mostly focused on assessing the role of BECCS in an energy system context (e.g., Bauer et al., 2018; Vaughan et al., 2018; Johansson et al., 2019; Lehtveer and Fridahl, 2020). Early studies that included both DACCS and BECCS have usually found DACCS to be too expensive to be competitive. For example, Fuss et al. (2013) investigated how abatement measures and negative emission technologies (NETs) (i.e., BECCS and DACCS) can be used to mitigate climate change during the period 2010–2099. The model uses marginal abatement cost curves together with CO2 capturing costs for the NETs to determine the optimal mitigation strategy by minimizing the total system cost. The study assumes a marginal cost for DACCS of 550 US$/tCO2 and a cost of 105US$/tCO2 for BECCS for the whole period. It is found that BECCS is the NET deployed in combination with abatement measures to achieve an atmospheric CO2 concentration of 435 ppm in 2100 with a discount rate of 5%. However, in a sensitivity analysis with a discount rate of 10%, abatement measures and BECCS are used in the early part of the century while DACCS gets deployed in the latter part of the century. More recently, Realmonte et al. (2019) used two different Integrated Assessment Models (IAMs) to investigate how DACCS can fit into a mitigation portfolio. The models include the HT system, the LT system, BECCS, and afforestation as NETs. The capturing costs are estimated to be between 180 and 300 $/tCO2 currently for the HT system with potential to reach 100$/tCO2 and 200–350 $/tCO2 currently for LT system with potential reduction to 50$/tCO2 as a floor cost. The cost includes capital cost and O&M costs but excludes costs for energy, which are determined by the model. The model uses a 20% annual growth rate cap for the technologies and two different carbon budgets to comply with the 1.5 and 2 °C targets. The results show that DACCS is deployed in both models but in the latter part of the century, while BECCS and afforestation are used in earlier periods. The LT system is preferred over the HT system. The main limiting factor for DACCS is found to be the speed it can be scaled up. Both of these studies have a limited time resolution for wind and solar infeed due to the scope of the models. The main difference between the studies is that Realmonte et al. includes more NETs and that they are using a lower capturing cost for DACCS which results in DACCS being more cost competitive. Breyer et al. (2020) use a linear programming model to analyse the dynamics of a variable renewable electricity system used to supply a DACCS system on an hourly scale. The Maghreb region, which has good conditions for solar insolation, is modeled with a focus on the years 2040 and 2050. A LT solid sorbent DACCS system is modeled where the electric and thermal energy needed is supplied through an off-grid decentralized electricity system. The electricity is produced by solar PV and wind power and to balance the system, batteries, and thermal energy storages (TES) are used. To supply the low temperature heat needed in the DAC system the TES and heat pumps are deployed. The study results in a projected capturing cost of CO2 of 105, 70, 55€/tCO2 in the years 2030, 2040, and 2050, and the DACCS system is concluded to be run almost continuously with 8,300 full-load hours (FLH) in a cost-optimized operation. The results show that the majority of the electricity generation supplying the DACCS system is solar PV and the DACCS system's electricity demand is almost as large as the total electricity demand of the entire Maghreb region. The study concludes that DACCS might be an economically beneficial opportunity for the region without considering the growing need for electricity in the region and resulting competition for resources. Thus, there is a lack of investigation and quantification of dynamics behind the choice between BECCS and DACCS in the intermittent energy system on sufficiently high time resolution and including the competition for wind and solar resources which has also been pointed out by Creutzig et al. (2019). The aim of the present work is to contribute to filling the current knowledge gap in three ways:
• First, analyse the role BECCS and DACCS take in the intermittent electricity system with requirement to produce negative emissions.
• Second, to determine which negative emissions technology is more cost-effective from the systems point of view under different wind and solar conditions.
• Third, to analyse the relation between system benefits and LCOC and its implications for policy decisions.
We evaluate the role of BECCS and DACCS in a carbon-constrained electricity system by applying an investment model for electricity system set up as a linear programming problem. The model finds the lowest cost feasible solution for investing in and operating a system under given constraints over a year. The model, called eNODE (Electricity in Nodes), was first developed by Göransson et al. and is presented in detail in previous work (Göransson et al., 2017). The model is designed to give a good representation of variability and variation management on the intra-annual time-scale. eNODE represents the electricity system operation over a year with a temporal resolution of 3-hours. The start-up time, start-up costs, and minimum generation level of thermal generation are accounted for as suggested by Weber (2005). Thermal generation with improved flexibility was added to eNODE in a subsequent study (Garðarsdóttir et al., 2018). Furthermore, Johansson and Göransson (2020) have complemented eNODE with variation management strategies, including batteries, demand-side management (DSM), and hydrogen storage.
To accommodate a detailed description of inter-hourly variability, the geographical resolution is reduced, and eNODE is applied to one copperplate-region at a time. A green-field approach is adopted, which assumes as the starting point an empty system without any generation capacity in place. Thus, eNODE is not designed to create a realistic representation of any actual regional electricity system (e.g., existing or planned capacities) but instead to investigate the linkages and dynamics between the different parts of the electricity generation system. However, in order to assure realistic combinations of wind and solar resources and electricity demand, the wind, solar, and load data from actual regions in Europe for year 2012, a rather typical year, are applied. In this work, two example regions have been selected for their large differences in wind and solar resources: one region with good wind conditions (IE-Ireland) typical to costal Northern-Europe and one region with good solar conditions (ES3-central Spain) typical to Southern Europe. The reason to choose different resource conditions is that wind and solar infeed patterns are fundamentally different. Solar infeed has more regular daily cycles whereas wind variations tend to be on longer scales covering days and weeks. In addition, both sources exhibit a seasonal variation in Europe with solar infeed being higher during the summer period and wind infeed during the winter period.
The technology cost data, fuel prices, and data on renewable resources and generation profiles applied in this work are listed in the Supplementary Material. Four different types of variation management technologies are included in this work: (redox) flow batteries; lithium-ion (Li-ion) batteries; hydrogen storage; and DSM. DSM, as implemented here, implies that up to 20% of the hourly demand for electricity can be delayed for up to 12 h [see (Johansson and Göransson, 2020) for a complete description of the DSM implementation]. Fuel cells have been added to the technological data, in addition to the electrolyser and hydrogen storage. The addition of fuel cells creates an endogenous demand for hydrogen as a means of electricity storage. The costs and efficiencies for the electrolyser, fuel cell, and hydrogen storage, as well as for batteries are given in Supplementary Material Table A3.
eNODE contains three bio-based generation technologies with CCS option. Biomass-fuelled steam power plants with CCS (biomass CCS), as well as combined cycle gas turbines with CCS fuelled with bio-based methane (biomethane CCS) are modeled as negative emission technologies. In addition, a carbon-neutral mix of co-fired biomethane and natural gas with CCS (biomethane-NG CCS) has been added to the technology options. The capture rate, additional costs for the CCS part, and efficiency penalties are assumed to be equal to their corresponding fossil-fuelled versions. More information on the modeling of the bio-based CCS technologies can be found in Johansson et al. (2019).
For this study DACCS technologies were added to the model. As previously described, several system configurations for DACCS exists. In this study, three different configurations were considered, a fully electrified LT solid sorbent system and two versions of the HT liquid solvent system with different supply for the thermal energy demand; a fully electrified system and a system combusting biogas. Both economic parameters such as CAPEX, OPEX and start-up costs, and technical parameters such as start-up time, minimum load level and capture rate were added to the model and can be found in the Supplementary Material.
In this study, we model a system at year 2050 and assume that 10% of removal of CO2 emissions compared to Year 1990 level emissions per year for the given region. The target was chosen in accordance to long-term modeling studies that indicate the need of net negative emissions in the middle of the century (Fuss et al., 2014). The costs of all technologies in the model represent about 2050 level. All the investment costs of all generation technologies and flexibility measures technologies are given in the Supplementary Material. We use cost data from Fasihi et al. (2019) for investment costs given for near term for DACCS in our Base case as the future cost of DACCS is highly uncertain and to our assessment rather optimistic in this study.
In the Base case all technology options are included. We also run a case where DACCS technologies are excluded to provide a comparison called NoDACCS. Both cases were applied to each of the two modeled regions. The cost of biomass is set to 30€/MWhth for these runs including both pre-processing and transport. The biomass value can be compared to the bioenergy index PIX (Pellet Nordic Index), which has remained rather stable within the range of 26–31 €/MWhth over the past years.
Further, based on the dispatch of technologies in the model, the LCOC was calculated for negative emission technologies present to determine the average cost for capturing one ton of CO2 using a given technology in a specific case. The LCOC takes into account the running time of the NET and value of electricity produced at that hour given by the model as well as fuel cost for DACCS and reduction of total cost for BECCS as electricity can be sold as an additional product, see Equation (1).
where CAPEX is the capital expenditures, OPEXvar and OPEXfix is the variable and fixed operating expenditures, CFuel is the fuel costs, CTransportation is the transportation cost, CStorage is the cost for storing CO2 and CElectricity is the revenue for selling electricity to the grid. CRF is the capital recovery factor and is calculated according to Equation (2).
where i is the interest rate, which is set to 5% in this work and n is the lifetime of the technology. Furthermore, to illustrate the system effects, the LCOE based on model dispatch was calculated for solar PV, wind and BECCS technologies according to Equation (3). The LCOE is a measure to determine the average cost for generating one unit of electricity using given technology in a specific case.
where CCO2 is the cost for carbon derived from the model run.
A Monte-Carlo analysis on sensitive parameters was also conducted to assess the robustness of the results. In Monte-Carlo analysis a large set of parameters is created, and the model is solved for each of them allowing thus to analyse the effect of the parameter values on the results. The parameters varied and their ranges can be found in Table 1. The cost of BECCS and other CCS technologies in the model is coupled to high temperature DACCS as it is essentially the same technology. The model was run for 200 different combinations of these parameters for both sunny (ES3) and windy region (IE).
Although this work provides important insights into the interactions among wind power, solar PV, and negative emission technologies; there are several limitations to this study. The addition of trade with neighboring regions would increase the possibilities for managing variations and for sharing investments. Thus, trade is likely to lower the total systems cost and offer additional measures for variation management and possibly storage, especially if trade with regions with hydropower is enabled. However, as the amount of hydropower available is limited, this would not change our findings fundamentally.
The model, eNODE, does not consider the existing capital stock. Similarly, the historical CO2 emissions and emissions from other parts of the energy system are not considered. Depending on the investment pathway in the overall energy system, there may be little scope for any fossil CO2 emissions or too little biomass to compensate for them and therefore limits to natural gas and BECCS combo that is allowed in our study for managing the variability. This could increase the competitiveness of DACCS as it is not dependent on biomass resource.
Furthermore, as this case study is applied to regions in Europe, the effectiveness of solar power is lower in our study than in many other regions of the world. However, due to more regular diurnal variations, solar PV based power is more easily managed by alternative strategies, such as short-term energy storage rather than biofueled complements. Wind variations, that are longer, and also seasonal demand variations, that are more common in colder regions, can however give some competitive advantage to BECCS compared to the LT DACCS system which may or may not be overweighed by other system effects.
Figure 1 shows the annual electricity generation for the two cases—Base and NoDACCS—in the two studied regions—ES3 and IE. In the Base case, both DACCS technologies and BECCS are available for providing negative emissions. However, DACCS outcompetes BECCS at the given price level for both regions. In the Base case when DACCS “LT” is acting as the only NET, the annual electricity generation increases compared to the NoDACCS case where the negative emissions are provided by BECCS. This is due to that DACCS consumes electricity and acts as a new load to the system, resulting in a higher total energy demand. The annual electricity generation is 4.7 TWh/year (5%) larger in the sunny region (ES3) and 2.6 TWh/year (8%) larger in the windy region (IE) compared to the case where DACCS technologies are not available. However, the total system cost is 5% lower in ES3 and 7% lower in IE in the Base case compared to the NoDACCS case.
Figure 1. Annual electricity generation in the Base case and in the NoDACCS case in regions ES3 (sunny) and IE (windy).
Moreover, the DACCS LT solid sorbent system is consistently outcompeting the HT liquid solvent system for both regions. Therefore, the HT liquid solvent system is never shown in the results. In the NoDACCS case, BECCS is working as the only provider of negative emissions, but the technology is simultaneously generating electricity to the grid.
Figures 2, 3 show the dynamics in the modeled system. To do that, 2-week periods covering both good and less good variable renewable resource conditions were chosen. Figures 2A,D shows the electricity generation and the total electricity demand in ES3 during 2 weeks for the Base case and NoDACCS case respectively. During some hours, solar PV and wind power, together with the energy storage in the batteries, as seen in Figures 2B,E, provide for the entire energy demand in both cases. At other hours, peak generation of natural gas is used to fulfill the whole demand. The energy demand for DACCS LT together with the variation of electricity price for the Base case is shown in Figure 2C. The electricity price is low during hours with large electricity production from variable renewable generation and high when complementary and peak generation is needed. DACCS LT is responding to low-electricity prices that enable lower cost of carbon removal, which can be seen in Figure 2C, and is therefore mainly running when the electricity price is low. Figure 2F shows the electricity produced by BECCS and the variation of the electricity price in the NoDACCS case, showing that BECCS runs when electricity prices are high and complement to renewables is needed. One can also note that BECCS runs more continuously whereas DACCS LT is more often switched on and off.
Figure 2. (A) Electricity generation; (B) energy storage; and (C) electricity demand for DACCS and price for the Base case in region ES3 with in week 45 and 46 in year 2050. (D) Electricity generation; (E) energy storage; and (F) electricity generation by BECCS and price for the NoDACCS case in region ES3 with in week 45 and 46 in year 2050.
Figure 3. (A) Electricity generation; (B) energy storage; and (C) electricity demand for DACCS and price for the Base case in region IE in week 9 and 10 in year 2050. (D) Electricity generation; (E) energy storage; and (F) electricity generation by BECCS and price for the NoDACCS case in region IE in week 9 and 10 in year 2050.
Figures 3A,D shows 2 weeks of electricity generation in region IE in the Base case and NoDACCS case, respectively. Generation from wind and solar power is larger in the first week than in the second week in both cases due to better resource conditions. Therefore, variable electricity generation from wind and solar together with the energy storage in batteries covers the whole energy demand for almost all hours in the first week. In the second week, generation from variable renewables decreases and electricity generation from natural gas is needed to supply for the whole energy demand. Figures 3B,E show the energy storage in batteries for the Base case and NoDACCS case, respectively. Due to the IE system being wind dominated, the pattern of the energy storage level is more irregular in the IE case compared to the ES3 system, which is solar dominated. Moreover, the installed battery capacity relative to the amount of installed capacity of electricity generation is much larger for ES3 than IE, implying that batteries are better suited to handle solar variations that are more regular than wind variations. Figure 3C shows the electricity demand of DACCS LT together with the variation in electricity price while Figure 3F shows the electricity produced by BECCS along with the electricity price. Similar dynamics as in the solar-based system can be observed here.
To be able to compare how much it costs to capture one ton of CO2 for each region we calculate the LCOC based on the dispatch in the model. It is generally assumed that by using a technology with the lowest LCOC, the total cost of reaching low CO2 levels will be minimized. Figure 4 shows the LCOC for DACCS LT and BECCS respectively in different cases. The LCOC for DACCS LT is consistently larger than the LCOC for BECCS, meaning that it is more expensive to capture one ton of CO2 using DACCS LT than using BECCS. The two largest costs for DACCS LT are the CAPEX and variable OPEX. As for BECCS, the largest costs are the fuel cost and CAPEX. This is in line with analysis from Creutzig et al. (2019) who also deem the land scarcity and resulting cost of biomass to be the largest determinant of cost of BECCS. The electricity produced by BECCS is sold to the electricity grid, resulting in an income for BECCS which is subtracted from the other costs for the total LCOC, see Equation (1). The costs for transporting and storing CO2 varies between the regions based on (Kjärstad et al., 2013) but constitute only a minor part of the total cost in both regions. The costs for storage and transport of CO2 are more expensive in IE compared to ES3. Note that the transport cost of CO2 for DACCS is assumed to be zero since the technology could be placed on the storage site. In both cases DACCS LT is run for ca 4,500 FLH, whereas BECCS is run for ca 3,900 FLH if it has to replace DACCS LT. This is about half of the hours of the year and means that the levelized cost of both technologies could potentially be reduced by higher operating hours as capital costs would be then divided over more hours, however, this not cost-efficient from the system perspective.
Figure 4. LCOC calculated for DACCS and BECCS in regions ES3 (sunny) and IE (windy) for the Base case and the NoDACCS case respectively.
The studied system provides two services: electricity and negative emissions. Thus, it is also relevant to analyse the LCOE in different set-ups. LCOE for selected technologies is shown in Figure 5. For BECCS it is assumed that the case specific price for CO2 (i.e., a result from the Base or NoDACCS case) is received as an income and thus deducted from the LCOE, see Equation (3). The largest cost for both wind power and solar PV is the fixed OPEX, which is dependent on the load factor of the technology for unit of electricity generated. A larger load factor results in a lower fixed OPEX due to more hours to spread the costs over. This can be seen for wind power in the IE case where the load factor is larger, resulting in a decreased fixed OPEX and therefore also a reduced total LCOE. The LCOE for BECCS is consistently larger than that for wind power and solar PV for both ES3 cases. However, for the case where BECCS is present in IE (NoDACCS), the LCOE for BECCS is lower than the LCOE for solar PV. Moreover, the fuel cost has the largest impact on the LCOE for BECCS.
Figure 5. LCOE calculated for onshore wind power, solar PV and BECCS for the regions ES3 (sunny) and IE (windy) for the Base case and the NoDACCS case respectively.
The results from the Monte Carlo analysis are shown in Figures 6–8. In the analysis both the cost of biomass and the CAPEX of CCS technologies was varied for 200 combinations for each region. In most of the cases DACCS LT is preferred both in windy and sunny regions, but the choice of BECCS or DACCS HT is significantly higher in the wind-based systems. In some instances (about 10–25% of the runs) both DACCS LT and BECCS/DACCS HT enter the system. BECCS and DACCS HT were grouped together in Figure 6 due to their similar characteristics when it comes to flexibility and investment cost.
Figure 6. Occurrence of different NETs or combination of them in Monte Carlo analysis (200 runs) in the regions ES3 (sunny) to the left and IE (windy) to the right respectively.
Figure 7. The installed capacity of NETs compared to the cost of biomass in the regions ES3 (sunny) to the left and IE (windy) to the right respectively.
Figure 8. The installed capacity of NETs compared to the CAPEX cost ratio of the electrified versions of LT solid sorbent DACCS and HT liquid solvent DACCS for the regions ES3 (sunny) to the left and IE (windy) to the right respectively. The CAPEX of BECCS is pegged to the CAPEX of HT DACCS in the model.
The results show a clear correlation between the cost of biomass and the choice of negative emissions technology (Figure 7). With a low biomass cost, BECCS is the preferred option in the sunny region whereas the results are varied for the windy region and depend on the cost ratio between the negative emission technologies. Sometimes both BECCS and DACCS LT are invested in in such cases. DACCS will become the preferred technology at higher biomass costs. It can also be seen that the biomass price required for DACCS to enter the system is higher for the solar PV-based system (ES3) than in the wind-based system (IE).
The cost relation between the CAPEX of DACCS LT and DACCS HT (and thus also BECCS as it is based on the same technology) has a much smaller effect on the results (Figure 8). DACCS HT enters the system only when it is significantly cheaper than DACCS LT, otherwise BECCS will be preferred as it has the additional benefit of being able to produce electricity at high price hours. Both DACCS LT and BECCS can be chosen at all CAPEX ratios in the sunny region and the cost of biomass is the main determining factor. BECCS is chosen in the windy region only when both biomass price and technology cost are favorable.
In this analysis, we have used an electricity system model with high time resolution to analyse the role BECCS and DACCS can take in an intermittent electricity system. Our results demonstrate that these two technologies can have a fundamentally different role, especially when it comes to DACCS LT. DACCS LT is consuming electricity mainly at low price hours and reducing the need of energy storage. BECCS on the other hand, is run when wind and solar supply is low and electricity prices are high but also crowds out some renewable production due to its relative inflexibility. DACCS LT is chosen over BECCS and DACCS HT in most instances of sensitivity analysis as the most cost-effective option for the whole system.
Our results clearly show that other considerations than just LCOC may play a role in cost effectiveness of a negative emission technology. When comparing BECCS and DACCS as a part of a future electricity system, two main factors play a role besides the cost of technologies themselves. First, the flexibility of the technology and its ability to adapt to the variable electricity production. Here, DACCS LT has a clear advantage with a cyclical operation at 3 h intervals, whereas BECCS and DACCS HT need longer time for starting up and also have costs associated to starting and stopping operation. Secondly, the cost of electricity produced by the whole system becomes important. With DACCS as a negative emission provider more solar and wind electricity at cheaper cost is produced by the system compared to the system where BECCS provides some of the electricity. For low production hours of wind and solar-based electricity batteries and gas turbines are used to cover the lack of production instead of BECCS. The combination of this is cheaper on the system level than using BECCS to cover the low production hours. It is also interesting to note that the second effect outweighs the first one. Since variations in solar-based systems are more regular and shorter (daily cycles), one could assume that DACCS LT is better suited for such systems, whereas our results point in the opposite direction. DACCS LT is more often chosen in the windy system. This can again be explained when looking at LCOE production for different technologies (Figure 5). In the solar-based system LCOE of wind, solar PV and BECCS is much closer to each other, whereas in wind-based system the LCOE of wind is by far lower than others and solar PV becomes more expensive than BECCS due to larger variations in seasonal generation. This means that replacing the renewable (mostly wind-based) electricity with electricity from BECCS becomes more costly in this system. As the LCOC does not include either of the dynamics discussed above, it can give a misleading impression that BECCS is by far superior to DACCS LT for providing the system with negative emissions. In fact, the opposite is the case in our study. It should be kept in mind that this relation is also dependent on the amount of negative emissions required from the system. In our study the electricity is the main product and clearly outweighs the negative emissions in amount. In a system that is focused more on negative emission generation, the LCOC would have a greater importance than LCOE. However, it is not likely to be the case with larger-scale systems where electrification will play an important role in reaching the climate targets.
It is sometimes argued that the levelized cost could be a more accurate measure if only extra investments for CCS part are included and the revenue from the electricity sales ignored. This approach will, however, also miss the system dynamics that determine when the plant is run most cost effectively. Another common approach is to have purpose built renewable energy generation that usually assumes the best conditions for DACCS electricity consumption (Fasihi et al., 2019; Breyer et al., 2020). This may lower the cost for CO2 captured via DACCS but has an opportunity cost of not using the renewable resource in the societally cost-effective way. In many regions the potential for renewables is limited by either land use constraints or by public acceptance of exploration of new sights. Therefore, an adequate comparison of different NETs requires an analysis of the whole system where they are placed.
As there is a large movement toward electrification in many sectors there will likely also be competition for low price electricity meaning that in case of large-scale electrification the fuel cost for DACCS may increase. Although the cost of fuel (electricity) is only a small part of the total cost of capturing carbon with DACCS LT, this may also have an effect on other dynamics in the system and should thus be studied further. From our results we see that there is a much larger over production of electricity compared to demand in the solar PV-based system and thus DACCS LT is more likely to remain competitive there if the renewable resource potential is limited. Otherwise, the opportunity cost of replacing electricity from variable renewables still applies and has a stronger effect in windy systems.
Carbon dioxide removal (CDR) from the atmosphere is likely to be needed to limit global warming to 1.5 or 2°C and thereby meeting the Paris Agreement. Several methods exist for CDR and there is a debate on which methods are most suitable and cost-effective. Thus, deeper understanding of system effects related to CDR are needed for effective governance of these technologies. Bio-Energy with Carbon Capture and Storage (BECCS) and Direct Air Carbon Capture and Storage (DACCS) are two CDR technologies, that can have a direct relation with the electricity system—BECCS via producing it and DACCS via consuming. In this work, we investigate how BECCS and DACCS interact with an intermittent electricity system to achieve net negative emissions using an energy system model and two regions with different wind and solar resource conditions. The analysis shows that DACCS has usually a higher LCOC than BECCS, implying that it is less costly to capture CO2 using BECCS under the assumptions made in this study. However, due to a higher LCOE produced by BECCS, the total system cost is lower using DACCS as negative emission provider as it can be more flexible and enables cheaper electricity production via renewables and storage. The result is mainly sensitive to the price of biomass and to lesser extent to the investment cost relation between DACCS and BECCS. This indicates that the use of the LCOC as often presented in the literature as a main indicator for choosing between different CDR options might be misleading and that impact to the whole system operation needs to be considered for well-grounded decisions. We also see that low temperature DACCS is better suited for solar PV-based systems from the flexibility point of view as the variations there are more short term and regular and also due to the tendency to larger over generation of electricity. However, these benefits can be overweighed by the opportunity cost of lower total electricity production costs in windy systems, where the difference in LCOE of wind power and BECCS is large that difference in LCOE of variable renewables and BECCS in a sunny region.
The original contributions presented in the study are included in the article/Supplementary Material, further inquiries can be directed to the corresponding author/s.
ML conceived the idea of the study and performed the modeling. AE collected the data. Both authors analyzed the results and wrote the paper.
ML was employed by Göteborg Energi AB, a municipal utility company, from September 2020. The main part of the work was conducted prior this employment.
The remaining author declares that the research was conducted in the absence of any commercial or financial relationships that could be construed as a potential conflict of interest.
We would like to thank Max Biermann and Mikael Odenberger for their comments on this work that have helped to improve it greatly.
The Supplementary Material for this article can be found online at: https://www.frontiersin.org/articles/10.3389/fclim.2021.647276/full#supplementary-material
Negative Emissions Technologies and Reliable Sequestration: A Research Agenda (2018). Washington, DC, National Academies Press. 368pp.
Bauer, N., Rose, S. K., Fujimori, S., van Vuuren, D. P., Weyant, J., Wise, M. l., et al. (2018). Global energy sector emission reductions and bioenergy use: overview of the bioenergy demand phase of the EMF-33 model comparison. Clim. Change 163, 1553–1568. doi: 10.1007/s10584-018-2226-y
Breyer, C., Fasihi, M., and Aghahosseini, A. (2020). Carbon dioxide direct air capture for effective climate change mitigation based on renewable electricity: a new type of energy system sector coupling. Mitigat. Adapt. Strateg. Glob. Change 25, 43–65. doi: 10.1007/s11027-019-9847-y
Creutzig, F., Breyer, C., Hilaire, J., Minx, J., Peters, G., and Socolow, R. H. (2019). The mutual dependence of negative emission technologies and energy systems. Energy Environ. Sci. 12, 1805–1817. doi: 10.1039/C8EE03682A
Creutzig, F., Ravindranath, N. H., Berndes, G., Bolwig, S., Bright, R., Cherubini, F., et al. (2015). Bioenergy and climate change mitigation: an assessment. GCB Bioenergy 7, 916–944. doi: 10.1111/gcbb.12205
Fasihi, M., Efimova, O., and Breyer, C. (2019). Techno-economic assessment of CO2 direct air capture plants. J. Clean. Prod. 224, 957–980. doi: 10.1016/j.jclepro.2019.03.086
Fuss, S., Canadell, J. G., Peters, G. P., Tavoni, M., Andrew, R. M., Ciais, P., et al. (2014). Betting on negative emissions. Nat. Clim. Change 4:850. doi: 10.1038/nclimate2392
Fuss, S., Lamb, W. F., Callaghan, M. W., Hilaire, J., Creutzig, F., Amann, T., et al. (2018). Negative emissions—Part 2: Costs, potentials and side effects. Environ. Res.Lett. 13:063002. doi: 10.1088/1748-9326/aabf9f
Fuss, S., Reuter, W. H., Szolgayova, J., and Michael, O. (2013). Optimal mitigation strategies with negative emission technologies and carbon sinks under uncertainty. Clim. Change 118, 73–87. doi: 10.1007/s10584-012-0676-1
Garðarsdóttir, S. Ó., Göransson, L., Normann, F., and Johnsson, F. (2018). Improving the flexibility of coal-fired power generators: impact on the composition of a cost-optimal electricity system. Appl. Energy 209, 277–289. doi: 10.1016/j.apenergy.2017.10.085
Garðarsdóttir, S. Ó., Normann, F„ Andersson, K., Prölß, K., and Emilsdóttir, S, Johnsson F. (2015). Post-combustion CO2 capture applied to a state-of-the-art coal-fired power plant—the influence of dynamic process conditions. Int. J. Greenhouse Gas Control 33, 51–62. doi: 10.1016/j.ijggc.2014.12.001
Göransson, L., Goop, J., Odenberger, M., and Johnsson, F. (2017). Impact of thermal plantcycling on the cost-optimal composition of a regional electricity generation system. Appl. Energy 197, 230–240. doi: 10.1016/j.apenergy.2017.04.018
Johansson, V., and Göransson, L. (2020). Impacts of variation management on cost-optimal investments in wind power and solar photovoltaics. Renew. Energy Focus 32, 10–22. doi: 10.1016/j.ref.2019.10.003
Johansson, V., Lehtveer, M., and Göransson, L. (2019). Biomass in the electricity system: a complement to variable renewables or a source of negative emissions? Energy 168, 532–541. doi: 10.1016/j.energy.2018.11.112
Kjärstad, J., Morbee, J., Odenberger, M., Johnsson, F., and Tzimas, E. (2013). Modelling large-scale CCS development in europe linking techno- economic modelling to transport infrastructure. Energy Proc. 37, 2941–2948. doi: 10.1016/j.egypro.2013.06.180
Lehtveer, M., and Fridahl, M. (2020). Managing variable renewables with biomass in the European electricity system: emission targets and investment preferences. Energy 213:118786. doi: 10.1016/j.energy.2020.118786
Realmonte, G., Drouet, L., Gambhir, A., Glynn, J., Hawkes, A., Köberle, A. C., et al. (2019). An inter-model assessment of the role of direct air capture in deep mitigation pathways. Nat. Commun. 10:3277. doi: 10.1038/s41467-019-10842-5
Rogelj, J., McCollum, D. L., Reisinger, A., Meinshausen, M., and Riahi, K. (2013). Probabilistic cost estimates for climate change mitigation. Nature 493, 79–83. doi: 10.1038/nature11787
Slade, R., Bauen, A., and Gross, R. (2014). Global bioenergy resources. Nat. Clim. Change 4:99. doi: 10.1038/nclimate2097
Vaughan, N. E., Gough, C., Mander, S., Littleton, E. M., Welfle, A., Gernaat, D. E. H. J., et al. (2018). Evaluating the use of biomass energy with carbon capture and storage in low emission scenarios. Environ. Res. Lett. 13:044014. doi: 10.1088/1748-9326/aaaa02
Keywords: negative emissions, BECCS, DACCS, variation management, electricity system modeling
Citation: Lehtveer M and Emanuelsson A (2021) BECCS and DACCS as Negative Emission Providers in an Intermittent Electricity System: Why Levelized Cost of Carbon May Be a Misleading Measure for Policy Decisions. Front. Clim. 3:647276. doi: 10.3389/fclim.2021.647276
Received: 29 December 2020; Accepted: 15 February 2021;
Published: 11 March 2021.
Edited by:
Mijndert Van Der Spek, Heriot-Watt University, United KingdomReviewed by:
Charithea Charalambous, Heriot-Watt University, United KingdomCopyright © 2021 Lehtveer and Emanuelsson. This is an open-access article distributed under the terms of the Creative Commons Attribution License (CC BY). The use, distribution or reproduction in other forums is permitted, provided the original author(s) and the copyright owner(s) are credited and that the original publication in this journal is cited, in accordance with accepted academic practice. No use, distribution or reproduction is permitted which does not comply with these terms.
*Correspondence: Mariliis Lehtveer, bWFyaWxpaXMubGVodHZlZXJAY2hhbG1lcnMuc2U=
Disclaimer: All claims expressed in this article are solely those of the authors and do not necessarily represent those of their affiliated organizations, or those of the publisher, the editors and the reviewers. Any product that may be evaluated in this article or claim that may be made by its manufacturer is not guaranteed or endorsed by the publisher.
Research integrity at Frontiers
Learn more about the work of our research integrity team to safeguard the quality of each article we publish.