- 1Division of Infectious Diseases, Department of Internal Medicine, Yonsei University College of Medicine, Seoul, Republic of Korea
- 2CJ Bioscience, Inc., Seoul, Republic of Korea
- 3Interdisciplinary Program in Bioinformatics, Seoul National University, Seoul, Republic of Korea
- 4Department of Biomedical Sciences, Graduate School of Medical Science, Brain Korea 21 Project, Gangnam Severance Hospital, Yonsei University College of Medicine, Seoul, Republic of Korea
- 5Department of Immunology, Blavatnik Institute, Harvard Medical School, Boston, MA, United States
- 6Division of Infectious Diseases, Department of Internal Medicine, Seoul Medical Center, Seoul, Republic of Korea
- 7The Picower Institute for Learning and Memory, Department of Brain and Cognitive Sciences, Massachusetts Institute of Technology, Cambridge, MA, United States
- 8Bio2Q, Keio University, Tokyo, Japan
Introduction: Coronavirus disease 2019 (COVID-19) alters the gut microbiome. This study aimed to assess the association between the disease severity of COVID-19 and changes in stool microbes through a seven-month follow-up of stool collection.
Methods: We conducted a multicentre, prospective longitudinal study of 58 COVID-19 patients and 116 uninfected controls. Differences in the gut microbiota were analysed using 16S ribosomal RNA sequencing. The first stool samples were collected at an early convalescent phase of COVID-19, and the second sample was collected at least seven months after COVID-19 infection.
Results and discussion: At the order level, Eubacteriales and Bifidobacteriales decreased, while Bacteroidales and Burkholderiales increased in the COVID-19 group compared to the controls. Alpha diversity also decreased in COVID-19 patients compared to controls, with imperfect recovery of the gut microbiome after seven months. The compositional change in the gut microbiome between the early and late convalescent phases was largest in the moderate and severe groups. The severity of COVID-19 was the most influential clinical variable for microbiome composition (Sum of Sqs = 0.686, P = 0.006), and its effect persisted even after partialling out other effects such as antibiotic use and age. Thus, our study indicates a possible interaction between respiratory viral infection and the composition of the gut microbiota community, warranting future mechanistic and prospective longitudinal studies. Additionally, we were able to detect microbiome changes in patients who were re-infected with SARS-CoV-2. Notably, the dominant bacteria in the re-infected group were Lachnospiraceae and Faecalimonas umbilicata, compared to the one-time infected group.
Introduction
Since the World Health Organization (WHO) declared a pandemic in March 2020, the threat of Coronavirus disease 2019 (COVID-19) has persisted as variants of Severe Acute Respiratory Syndrome Coronavirus 2 (SARS-CoV-2) continuously emerge (Karim and Karim, 2021). Angiotensin-converting enzyme 2 (ACE2) receptor play a key role in the entry of SARS-CoV-2 into host cells. The spike protein of SARS-CoV-2 binds to the ACE2 receptor, forming a complex that enters and replicates in human cells (Jia et al., 2005; Du et al., 2009; Wang et al., 2020). ACE2 receptors are present in the epithelial cells of multiple tissues, including goblet cells of the nasal cavity, alveolar cells of the lung, mucosal cells of the gastrointestinal tract, tubular epithelial cells of the kidney, and endothelial cells of blood vessels. Therefore, COVID-19 patients may experience various gastrointestinal symptoms, such as vomiting and diarrhea (Dai et al., 2020; Han et al., 2020; Sungnak et al., 2020).
Commensal microbes have been suggested to play critical roles in modulating natural resistance to viral infection in preclinical mouse models (Stefan et al., 2020). Alterations in the gut microbiome communities of patients with COVID-19 are thought to be associated with pneumonia (Dhar and Mohanty, 2020; Gu et al., 2020; Zuo et al., 2020a; Zuo et al., 2020b; Sun et al., 2022).
Bacterial dysbiosis is sustained after COVID-19 resolution and is correlated with an increased incidence of opportunistic pathogens (Yamamoto et al., 2021; Zuo et al., 2021). However, since stool samples for these studies were collected during the early infection period, it is unclear whether COVID-19 is associated with long-term microbiome changes (Chen et al., 2022; Liu et al., 2022). Thus, prolonged dysbiosis in post-COVID-19 patient groups and its correlation with disease severity should also be evaluated. Furthermore, antibiotic use also needs to be considered for its potential confounding effects on gut dysbiosis because most severe COVID-19 patients are exposed to antimicrobial agents due to secondary bacterial pneumonia during hospitalization. Additionally, since the number of re-infection cases is increasing, it is informative to assess the differences in the gut microbiome in those infected once versus twice.
Herein, we compared gut microbiota composition at the early convalescent and late recovery phases after confirming SARS-CoV-2 infection, considering other clinical factors such as antibiotic effects and disease severity. We verified the association between disease severity and stool microbiome alterations in COVID-19 patients and assessed the recovery of gut dysbiosis through a seven-month follow-up stool collection. Additionally, we attempted to identify the dominant bacterial species in patients infected with SARS-CoV-2 once versus twice.
Materials and methods
Study design and populations
The enrolment criteria were persons who recovered with improvement of symptoms after being diagnosed with COVID-19 by PCR test, regardless of clinical symptoms. The exclusion criteria were 1) pregnant women, 2) illiterate, and 3) those who did not consent to participate in the study.
Patients were confirmed to have COVID-19 infection by PCR tests using nasopharyngeal and oropharynx swab specimens. This test was performed by RT-PCR using Power Check TM (Kogene Biothech, Republic of Korea), which has been authorized by the Food and Drug Administration (FDA). The threshold was 38 cycles of PCR, thus, with Ct values greater than 38 being treated as negative and Ct values smaller than 37 as positive for infection. Ct values between 37 and 38 were determined as intermediate and PCR was repeated. The PCR test targeted the Envelope (E) gene and RNA-dependent RNA polymerase gene (RdRp) gene of SARS-CoV-2. These tests in this study were performed at three different medical centers from July 2020 to Feb 2022. Sinchon and Gangnam Severance Hospitals are university-affiliated tertiary hospitals with a total of 2,800 beds. The Seoul Medical Center is a public hospital with 500 beds in Seoul, Republic of Korea.
Initial stool samples were collected from the subjects at the end of quarantine or hospitalization. Hospitalization and isolation are separate issues. If a patient was admitted and confirmed with COVID-19, they were moved to a negative pressure isolation facility and quarantined. Usually, if the confirmed COVID-19 status had been known before admission, the date of admission and the start date of isolation were determined the same. The quarantine is maintained for 7 days from the date of confirmation, and once it ends, patients were moved out of the negative pressure isolation facility and into a general ward. Follow-up stool samples were collected via the express mail system seven months after the date of COVID-19 confirmation. For the freshness of stool samples, the patients were limited to local residents within 60 km from the laboratory of this hospital, who could deliver stool specimens to the research lab within 2 hours after defecation. If the patients had mild symptoms and were discharged with brief hospitalization within seven days, stool samples were collected within one month of the diagnosis of COVID-19.
Healthy SARS-CoV-2 naive controls
We compared the gut microbiome of early and late convalescent phases of COVID-19 patients with healthy controls (1:2 ratio). Propensity score matching analysis was used based on three variables: age, sex, and body mass index (BMI). The data for the healthy control group were provided by the data platform of CJ Bioscience, Inc. (Seoul, South Korea) for comparative analysis. Healthy individuals were selected from gut microbiota study of healthy soldiers in the Republic of Korea (Yoon et al., 2021). The exclusion criteria included history of acute illness, chronic illness, cancer, abdominal surgery, and the use of antibiotics or probiotics within 3 months of the study. For individuals older than 60 years, the exclusion criteria for the presence of hypertension and diabetes were dismissed if they were well controlled. All healthy control samples were collected prior to the COVID-19 pandemic.
Data collection and clinical variables
Clinical characteristics, including age, sex, height, body weight, vaccination, smoking and clinical symptoms during hospitalization, and underlying medical conditions, were investigated using self-report questionnaires. The severity of disease was stratified as mild (severity score, l; no limitation of activities, and 2; limitation of activities), moderate (3: hospitalized with no oxygen therapy, and 4: oxygen therapy by mask or nasal prongs), and severe (5; non-invasive mechanical ventilation or high-flow oxygen therapy, 6; mechanical ventilation; and 7; mechanical ventilation with renal replacement therapy or Extracorporeal Membrane Oxygenation) by the WHO ordinal severity scale based on the clinical and respiratory status of patients (Rubio-Rivas et al., 2022).
Upon completion of stool sample collection, study participants were screened via a telephone survey for re-infection episodes of COVID-19. The confirmation of re-infection with COVID-19 was double-checked through the Korea Centers for Disease Control and Prevention (KCDC). We only re-examined re-infections, focusing on the patient’s symptoms, recovery severity, and microbiome analysis. There were some limitations in collecting additional personal data with regard to vaccination status.
Stool sample processing and DNA extraction
Stool was collected in sterile collection cups using a Para-Pak® stool transport vial (Meridian Bioscience Inc., Cincinnati, Ohio, USA) and immediately sent to the research team. Stool samples were processed in the laboratory within 2 hour of arrival and stored at −80°C until analysis. Microbial DNA was extracted from approximately 200 mg of feces per sample using the FastDNA™ SPIN Kit for Soil (MP Biomedicals, Solon, Ohio, USA), according to the manufacturer’s protocol.
Metagenomic sequencing
Polymerase chain reaction (PCR) amplification was performed using primers targeting the V3–V4 regions of the 16S rRNA gene with the extracted DNA. For bacterial amplification, the primers 341F (5′-TCGTCGGCAGCGTC-AGATGTGTATAAGAGACAG-CCTACGGGNGGCWGCAG-3′) and 805R (5′-GTCTCGTGGGCTCGGAGATGTGTATAAGAGACAGGACTACHVGGGTATCTAATCC-3′) were used. The fusion primers were constructed in the following order: P5 (P7) graft-binding, i5 (i7) index, Nextera consensus, sequencing adaptor, and target region sequence. Amplification was performed in a C1000 touch thermal cycler PCR system (Bio-Rad Laboratories, Inc., Hercules, CA, USA) under the following conditions: initial denaturation at 95°C for 3 min, followed by 25 cycles of denaturation at 95°C for 30 s, primer annealing at 55°C for 30 s, and extension at 72°C for 30 s, with a final elongation at 72°C for 5 min. The PCR product was confirmed by 1% agarose gel electrophoresis and visualized using a Gel Doc system (BioRad, Hercules, CA, USA). The amplified products were purified with the ProNex size-selective purification system (Promega, USA) with a 1:1.5 v/v ratio of sample to beads. Quality and product size were assessed on a Bioanalyzer 2100 (Agilent, Palo Alto, CA, USA) using a DNA 7500 chip. Mixed amplicons were pooled, and sequencing was carried out at CJ Bioscience (Seoul, Korea) using the Illumina MiSeq Sequencing system (Illumina, USA) with 2 × 250 bp paired-end mode according to the manufacturer’s instructions.
Metagenome analysis
Taxonomic profiling was performed using EzBioCloud’s MTP 16S service with the PKSSU4.0 reference database. Operational Taxonomic Unit (OTU) read counts were imported into R V.4.0.3 for statistical analysis. Propensity score matching in MatchIt R package V.4.4.0 was used to select individuals from the healthy controls closest to the COVID-19 patients. The OTU read counts of the samples were rarefied to 12,746 (minimum read count among the samples). For the alpha diversity analysis, Shannon diversity and species richness were calculated. The microbial diversity of the two groups, including paired data from the same patients (early and late convalescent phase), was compared using the Wilcoxon rank sum test. We also assessed normality using Q-Q plots and the Shapiro-Wilk test (Supplementary Figure S1) and calculated parametric Student’s t-test results (Supplementary Figure S2). As for Beta diversity, the Bray-Curtis index was used to calculate microbiome dissimilarities, and principal coordinate analysis (PCoA) was carried out to visualize the dissimilarities. Permutational multivariate analysis of variance (PERMANOVA) was used to determine whether the dissimilarities were associated with certain sample parameters. Distance-based redundancy analysis (dbRDA) was conducted to assess the influence of confounding factors on the other factors. The vegan R package V.2.5-7 was used for alpha diversity, Bray-Curtis, PERMANOVA, dbRDA, and rarefying. Linear discriminant analysis effect size (LEfSe) was used to identify the microbial biomarkers associated with certain sample parameters. The MaAsLin2 R package V.1.10.0, was used to identify multivariable associations between parameters and taxonomic features.
Statistical analysis
Categorical variables were compared using the Chi-square test and presented as number (percent). Continuous variables were expressed as mean ± standard deviation, and they were analyzed using the non-parametric Mann–Whitney U or Kruskal–Wallis test. All two-tailed P-values of < 0.05 were considered statistically significant. The statistical analyses were performed using SPSS Version 23.0 software (SPSS Inc., Chicago, IL, USA).
Data and materials availability
All sequencing data is available at PRJNA950198.
Results
Clinical characteristics of the study populations
Stool samples from the early and late convalescent phases of COVID-19 patients (n = 58) were compared with uninfected controls (n = 116) of the COVID-19 naive group (Supplementary Table S1). Patients with COVID-19 were stratified into three different groups according to the WHO severity scale. Clinical symptoms of fever (45.8% in the mild group vs. 86.4% in the moderate group vs. 75.0% in the severe group, P = 0.011) and COVID-19-related pneumonia (12.5% vs. 63.6% vs. 100%, P < 0.001) were more prevalent in the moderate and severe groups than in the mild COVID-19 group. All patients with severe COVID-19 received mechanical ventilation care (0% vs. 0% vs. 100%, P < 0.001) and intravenous steroid therapy (0% vs. 36.4% vs. 100%, P < 0.001). Most patients with severe COVID-19 were treated with remdesivir (0% vs. 36.4% vs. 91.7%, P < 0.001) and systemic antibiotic therapy (4.2% vs. 54.5% vs. 83.3%, P < 0.001) (Table 1).
The mean time intervals from the date of first stool collection and confirmation date of COVID-19 infection in each group were 43.7 ± 15.0 days, 46.2 ± 22.1 days, and 53.3 ± 16.3 days, respectively. Late convalescent phase data at the time of the second stool sample collection were 222.7 ± 29.5 days, 234.8 ± 37.6 days, and 238.5 ± 16.2 days, respectively, from COVID-19 confirmation date.
Based on COVID-19 genomic surveillance by the Korea Centers for Disease Control and Prevention (KCDC), it was estimated that all subjects in the COVID-19 patient group were infected with either the wild-type or B.1.617.2 (delta) variants of SARS-CoV-2. According to a survey conducted in July 2022, when the B.1.1.529 (Omicron) variant of the SARS-CoV-2 outbreak in South Korea decreased, only five patients among the study populations were re-infected with the B.1.1.529 variant of SARS-CoV-2.
Relative stool microbiome abundance and distribution between COVID-19 patients and COVID-19 naive controls
The gut microbiome changes at the order and genus levels in COVID-19 patients compared with controls are depicted in Figure 1. Specifically, the mean abundance of Eubacteriales (58.3% in the control group vs. 49.0% in the COVID-19 group, P < 0.001) and Bifidobacteriales (6.5% vs. 2.2%, P < 0.001) decreased, while Bacteroidales (20.5% vs. 33.4%, P < 0.001) and Burkholderiales (0.2% vs. 1.0%, P < 0.001) increased in the COVID-19 group compared to the controls (Figure 1A). At the genus level, the mean abundance of Bacteroides still showed a significant increase (13.1% vs. 22.6%, P < 0.001) in the COVID-19 group compared to the controls. Meanwhile, Blautia (10.4% vs. 3.7%, P < 0.001), Bifidobacterium (6.5% vs. 2.2%, P < 0.001), and Lactobacillus (1.4% vs. 0.8%, P = 0.002) displayed a decreasing trend in the COVID-19 group compared to the controls (Figure 1B).
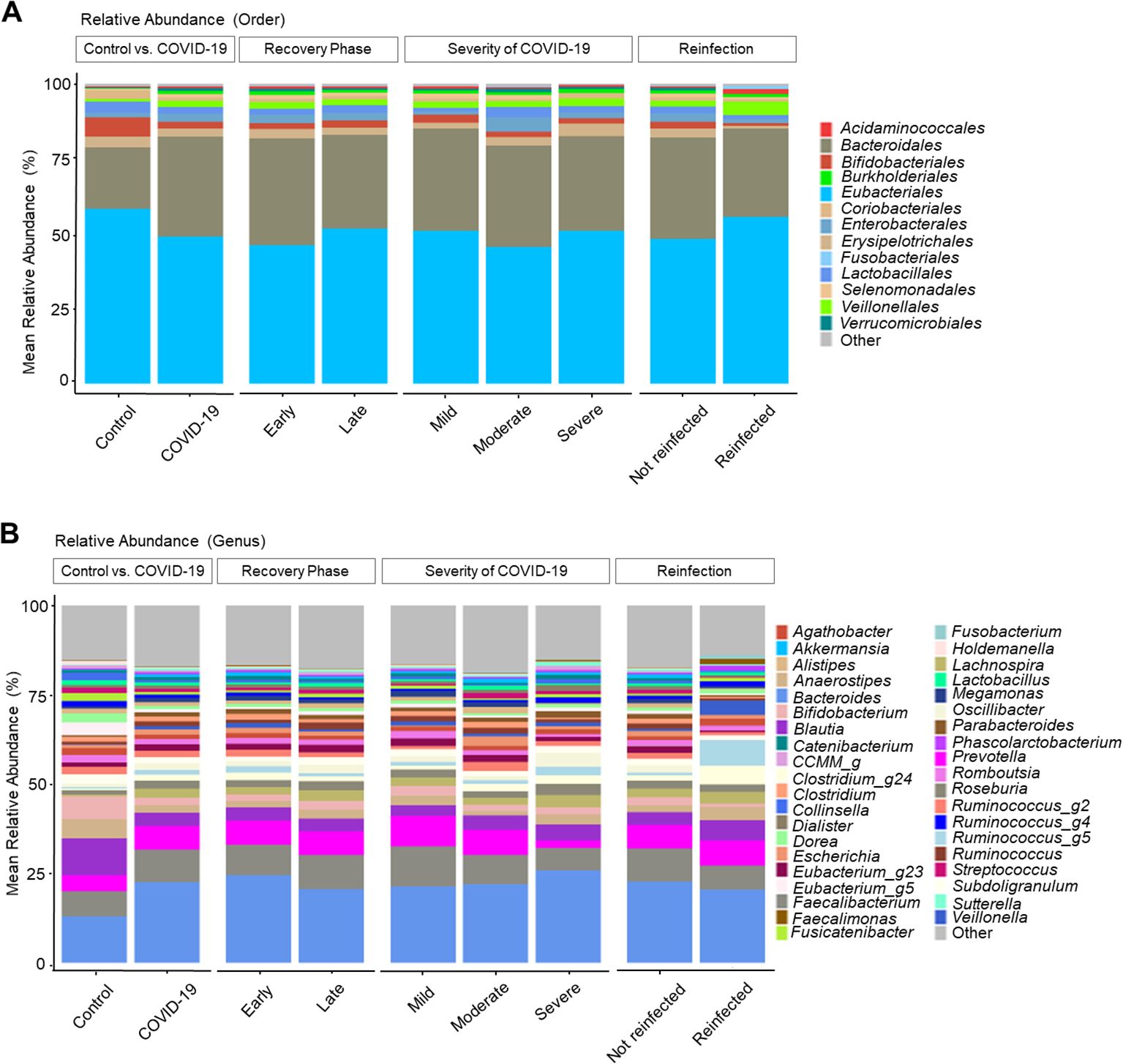
Figure 1. Taxonomic profiles of COVID-19 and uninfected healthy controls. (A) Order level. (B) Genus level.
Analysis by recovery time revealed an increase in Eubacteriales and Bifidobacteriales, along with a decrease in Bacteroidales and Burkholderiales in the late convalescent phases. The overall microbiome composition exhibited a recovery tendency similar to that of the uninfected group; however, full recovery was not observed.
Regarding the COVID-19 severity, the moderate and severe groups showed decreased levels of Bifidobacterium and increased Bacteroides compared to the mild group. Additionally, Prevotella and Faecalibacterium displayed a decreasing trend with the increased severity of COVID-19 infection.
Stool microbiome diversity and similarity in COVID-19 patients compared to naive controls
The mean community richness and microbial diversity were significantly lower in the COVID-19 group compared to the control group (P = 0.036), as indicated by the Shannon diversity index (Figure 2A). Additionally, alpha diversity value was significantly lower in the early convalescent phase of COVID-19 compared to the control group (P = 0.002), with a near recovery in the late convalescent phase compared with the early phase of COVID-19 infection (P = 0.002) (Figure 2B). Analysis based on the severity of COVID-19 during the early phase of infection and the corresponding control stool samples revealed a decrease in Shannon index as the severity of COVID-19 infection increased (Figure 2C). Notably, significant differences in microbiome community were observed between the control and the moderate (P = 0.022), and the severe groups (P = 0.007) during the early phase. However, these severity-associated differences in the microbiome community were absent during the late convalescent phase (Figure 2D).
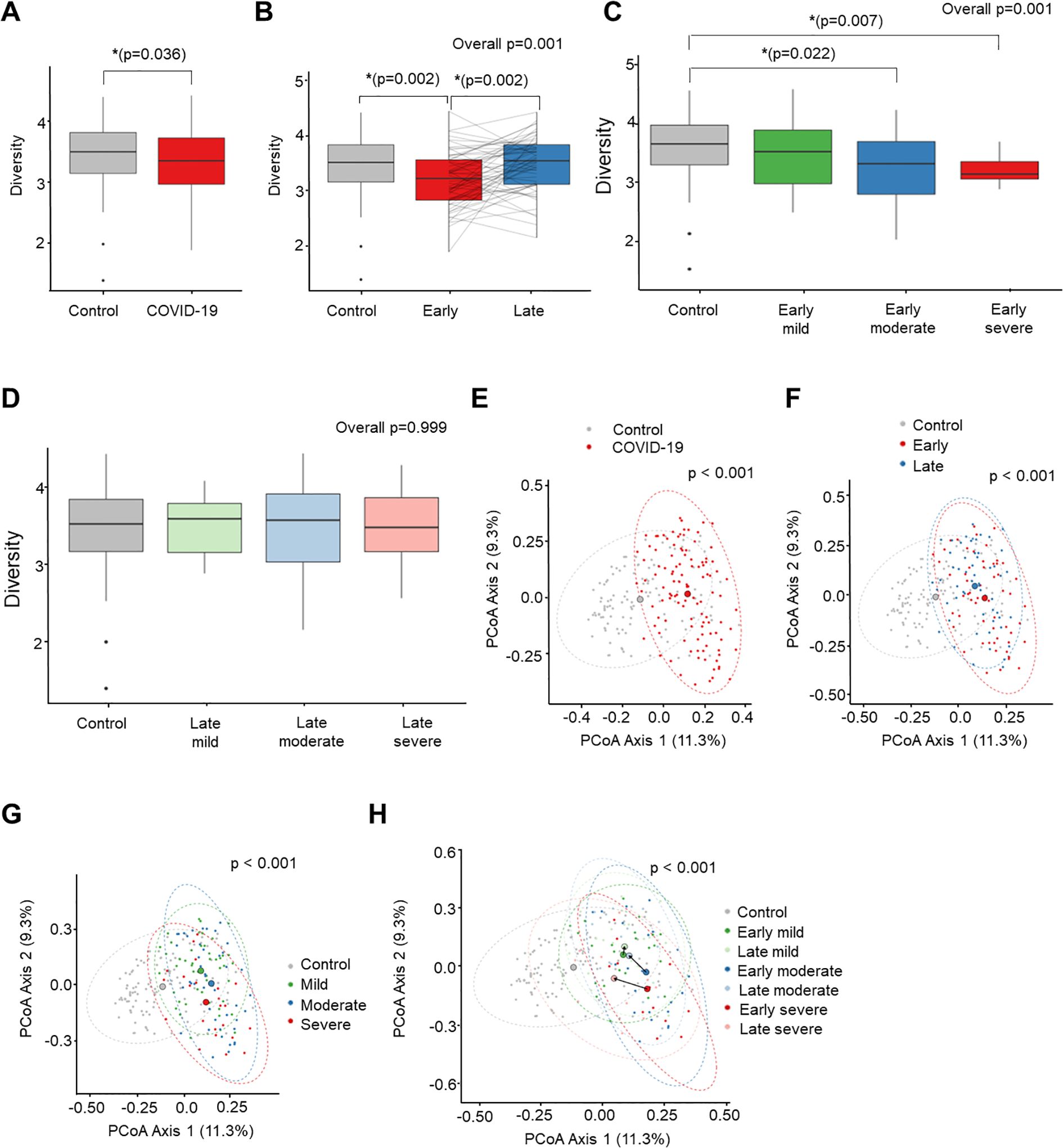
Figure 2. Comparison of alpha diversity (Shannon index) between COVID-19 and uninfected healthy controls (A-D). PCoA analysis based on Bray-Curtis dissimilarity distance between COVID-19 and uninfected healthy controls (E-H). (A) Control vs. COVID-19. (B) Recovery time analysis. (C) Severity analysis in early convalescent phase. (D) Severity analysis in late convalescent phase. (E) Control vs. COVID-19. (F) Recovery time analysis. (G) Severity analysis. (H) Recovery time and severity analysis. *P < 0.05.
Next, we conducted compositional analyses to assess differences among microbial communities. The Principal Coordinates Analysis (PCoA) of Bray-Curtis distance revealed a significant distinction in the overall microbial composition between patients with COVID-19 and uninfected controls (P < 0.001) (Figure 2E). Comparing the overall microbiome composition between the early and late convalescent phases showed no significant difference in beta diversity in the PCoA analysis (early vs. late, P = 0.123) (Figure 2F). However, subgroup analysis by disease severity revealed major alterations between the groups (mild vs. moderate, P = 0.018; mild vs. severe, P = 0.001; moderate vs. severe, P = 0.043) (Figure 2G). Furthermore, significant compositional differences in the distance between the early and late phases were observed according to severity, particularly in the moderate and severe groups (early phase vs. late phase: mild group, P = 0.130; moderate group, P = 0.027; severe group, P = 0.010) (Figure 2H). Pairwise PERMANOVA P-values for each group are presented in Supplementary Table S2.
Linear discriminant analysis on the effect size of the gut microbiome
In Figure 3, the lengths of the bar columns represent the linear discriminant analysis (LDA) score, which indicates significant differences in bacterial abundances of the stool microbiome between the two groups. LDA scores (log10) > 2 and P-value < 0.05 are listed in Figure 3A displays the microbial taxa with significant differences between COVID-19 infection (Positive score) and uninfected controls (Negative score). In the control group, Bacillota (genus Blautia & Anaerostipes) and Actinomycetota (genus Bifidobacterium), bacteria easily found in probiotic products or producing butyrate, were dominant. Conversely, as described earlier, Bacteroidota and Pseudomonadota were dominant in the COVID-19 group.
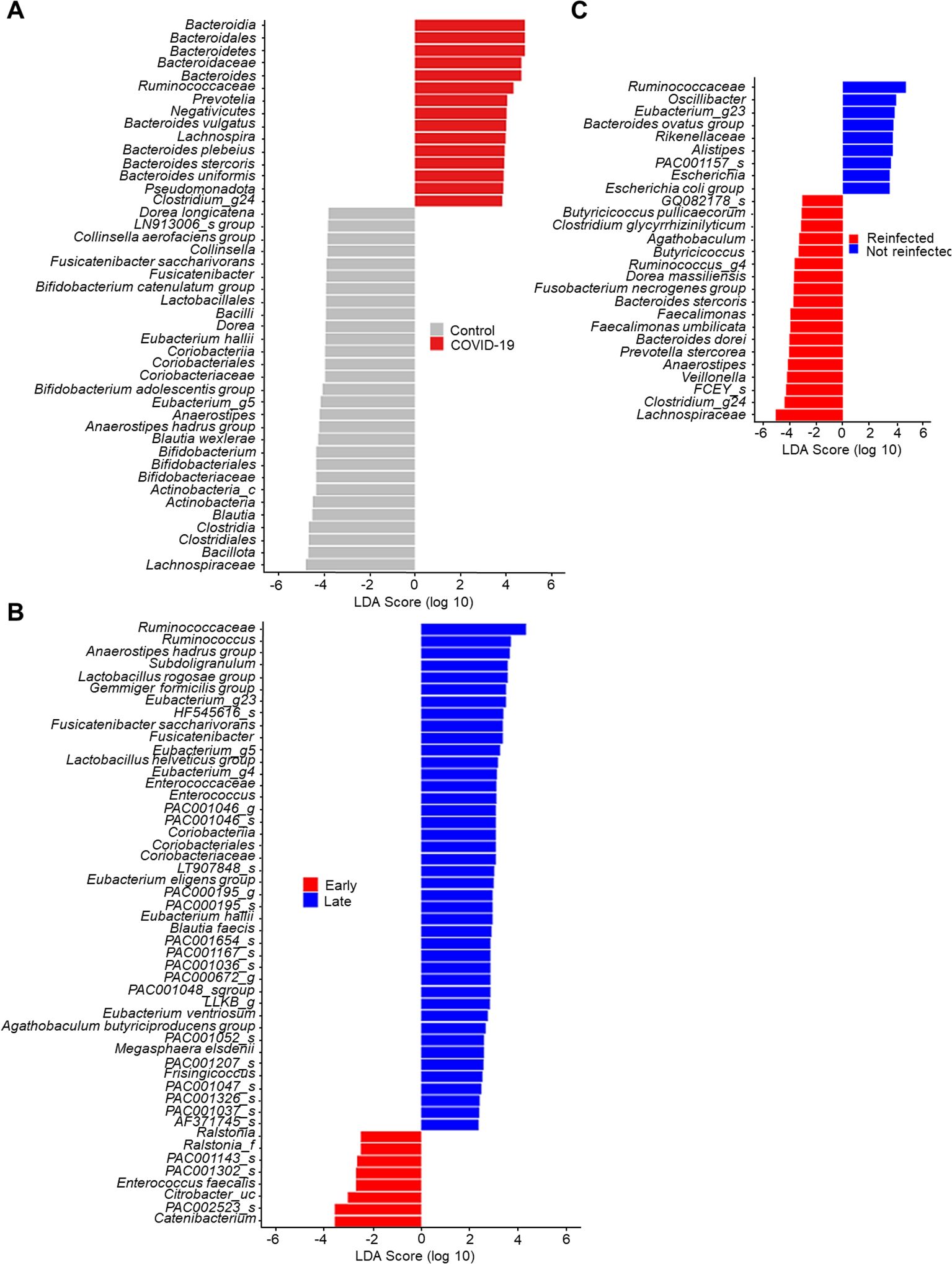
Figure 3. Linear discriminant analysis effect size of stool microbiome. (A) Control vs. COVID-19. (B) Early convalescent phase vs. Lase convalescent phase. (C) Wild type or delta variant of SARS-CoV-2 infection vs. Omicron variant of SARS-CoV-2 re-infection.
The LDA scores of the recovery time (Figure 3B) indicate that Enterococcus faecalis, bacteria known for their antibiotic resistance, were dominant in the early convalescent phase of COVID-19 (Negative score). In contrast, several butyrate-producing bacteria such as Subdoligranulum, Anaerostipes, and Eubacterium became dominant in the late convalescent phase of COVID-19 (Positive score).
In our cohort, five patients were re-infected with the B.1.1.529 (Omicron) variant of SARS-CoV-2. Therefore, we conducted comparative microbiome analyses between those infected once versus twice with SARS-CoV-2. Compared to patients infected with either the wild type or the delta variant of SARS-CoV-2 (negative score), those re-infected with the B.1.1.529 variant (positive score) were found to have Lachnospiraceae and Faecalimonas umbilicata as the dominant species (Figure 3C).
Minimal influence of antibiotic use and age on WHO severity’s effect on gut microbiome
To determine whether the effect of the WHO severity scale on the microbiome was independent of other factors such as antibiotic use and age, we conducted multivariable analyses using dbRDA and MaAsLin2.
We constructed a dbRDA model to assess the impact of the WHO severity scale on overall microbial composition, measured by Bray-Curtis distance, while controlling for variables including antibiotic use, age, time of stool collection, gender, SARS-CoV-2 reinfection, and BMI (Figure 4A; Table 2). We used the by=“margin” option in the permutation-based ANOVA test to calculate marginal effects, ensuring that the order of variables did not bias the results. The WHO severity scale had the largest impact on microbial composition (Sum of Sqs = 0.686, P = 0.006), while antibiotic use showed a relatively smaller but still significant effect (Sum of Sqs = 0.545, P = 0.008). Age had an even smaller effect, which was not statistically significant (Sum of Sqs = 0.439, P = 0.068). To further assess the effect of the WHO severity scale, we partialled out antibiotic use and age from the dbRDA model, and the WHO severity scale remained significant in both cases (Figures 4B, C; Table 2).
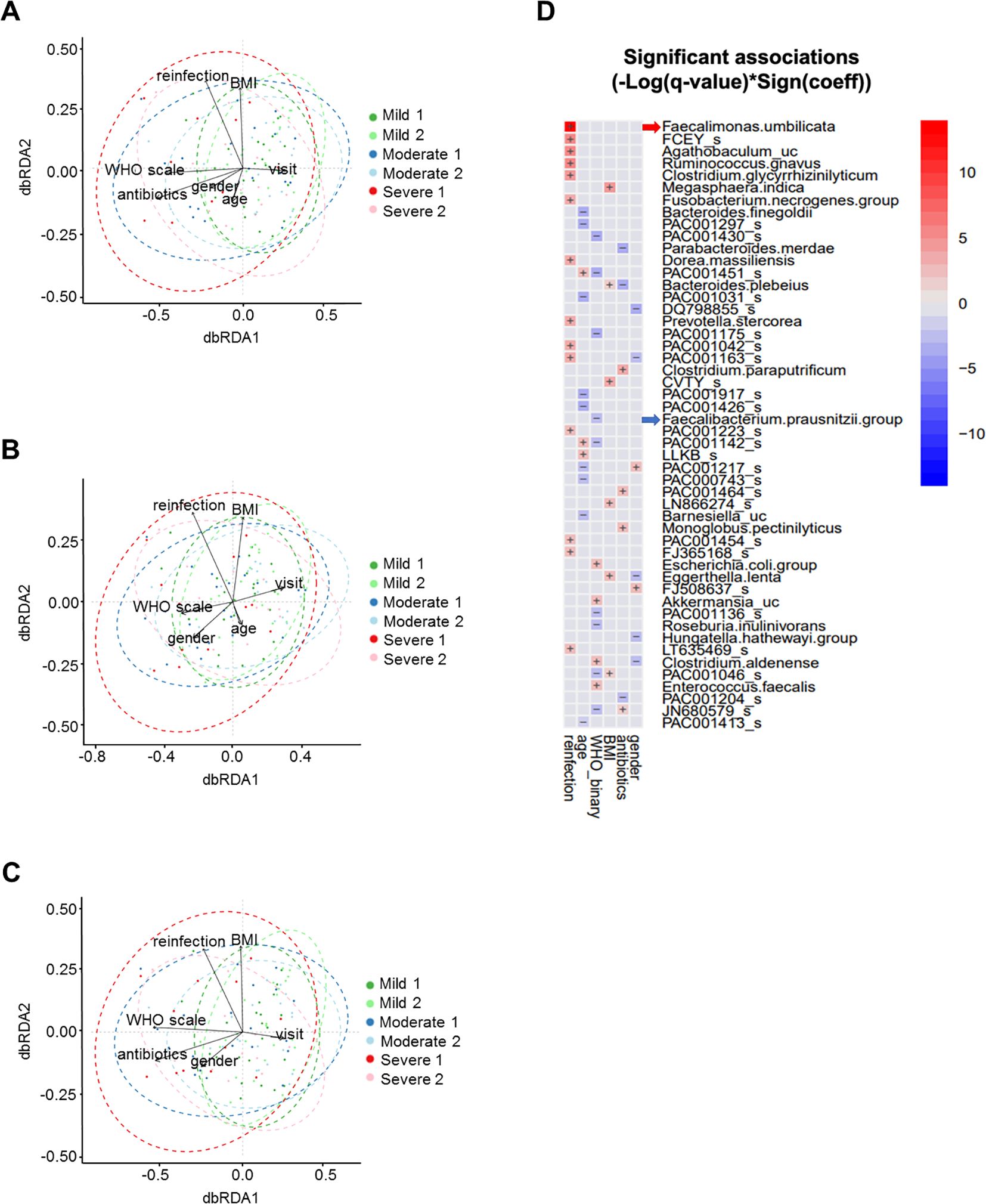
Figure 4. Multivariable analyses of stool microbiome. (A) dbRDA with WHO severity scale, antibiotic use, age, time of stool collection, gender, SARS-CoV-2 reinfection, and BMI. (B) dbRDA with antibiotic use partialled out. (C) dbRDA with age partialled out. (D) MaAsLin2 heatmap showing stool microbial operational taxonomic units with significant association with clinical variables.
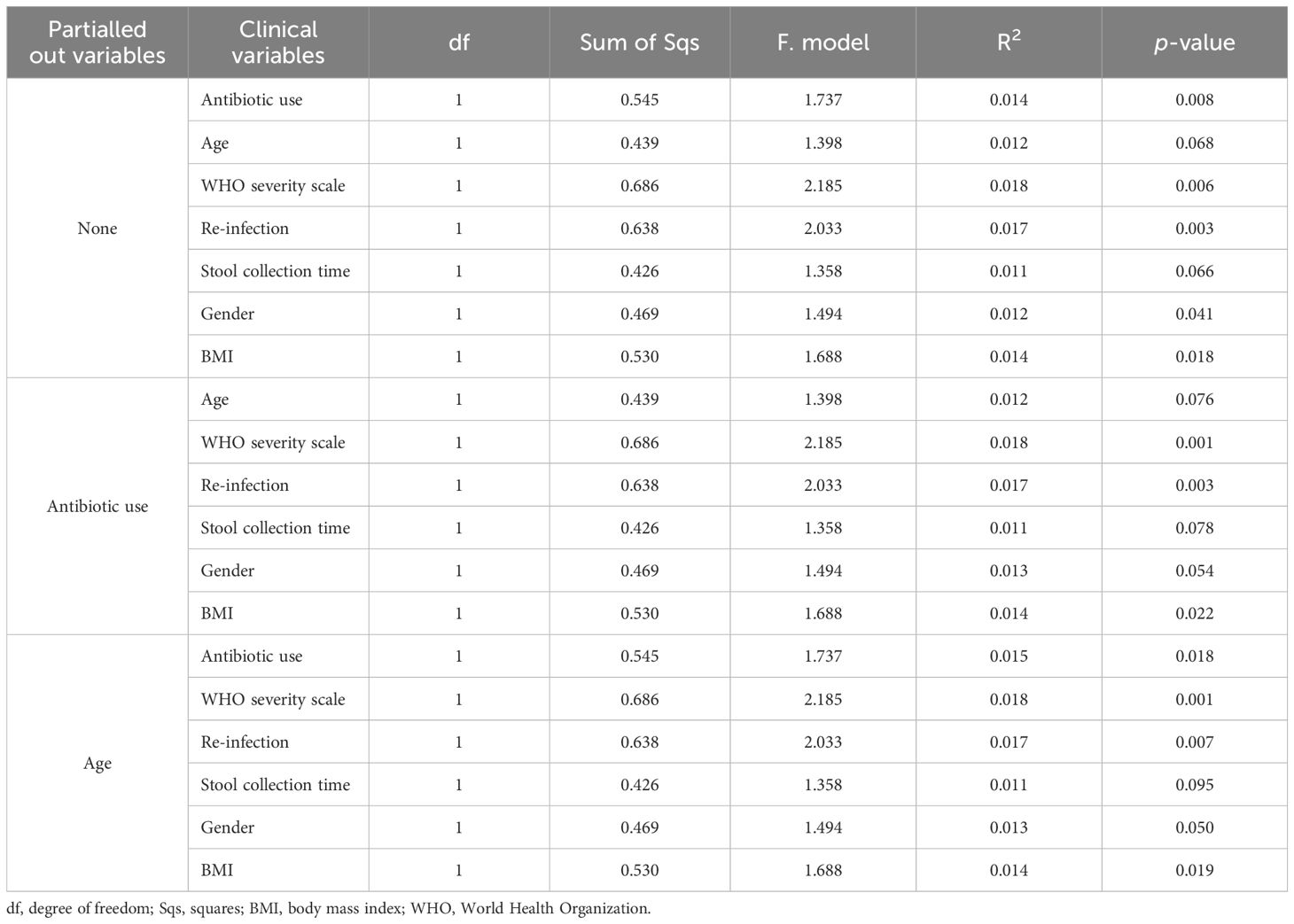
Table 2. Permutation-based ANOVA for dbRDA to test the effect of confounding clinical factors and their interactions on stool microbiome composition.
In addition, we performed MaAsLin2 analysis to examine multivariable associations between individual microbial taxonomic features and clinical variables, including gender, antibiotic use, BMI, WHO severity scale, age, and SARS-CoV-2 reinfection. This analysis identified microbial taxa that were specifically associated with the WHO severity scale, including Faecalibacterium prausnitzii (Figure 4D).
These analyses indicate that the WHO severity scale significantly influences microbial composition, with effects independent of antibiotic use and age.
Discussion
The results of this study indicate that SARS-CoV-2 infection is likely associated with changes in the stool microbiome compared to the uninfected controls selected with propensity-matched scoring analyses. Specifically, our results suggest that SARS-CoV-2 infection leads to a decreased abundance of symbionts and an increased proportion of opportunistic pathogens. Seven months after SARS-CoV-2 infection, the gut microbiome community became more similar to the baseline composition. However, the extent of recovery was somewhat limited, highlighting the persistent effects of respiratory viral infection on the gut microbiota community.
We found a significant reduction in microbiome diversity in patients with severe symptoms. Moreover, we and others (Donati Zeppa et al., 2020; Shi et al., 2021) found that Faecalibacterium decreased as the disease severity increased. However, we did not detect an increased abundance of Clostridium or Coprobacillus in the severe COVID-19 group as reported in other studies (Donati Zeppa et al., 2020; Shi et al., 2021). Importantly, our study confirmed that the degree of gut microbiota alteration was higher in the severe group, even after considering the impact of antibiotic use and age. These results support the notion that gut microbiota diversity is negatively correlated with COVID-19 severity.
The gut microbiota composition undergoes significant changes upon SARS-CoV-2 infection, likely due to the gut-lung axis interaction as reported following respiratory infections such as influenza, respiratory syncytial virus, or chronic obstructive pulmonary diseases (Keely et al., 2012; Hanada et al., 2018; Yildiz et al., 2018; Gu et al., 2020). For example, the progression of pneumonia and acute respiratory distress syndrome triggered by respiratory infections can impact the host immune system, leading to alterations in the gut microbiome. Conversely, this interaction involves both endotoxins and metabolites from the gut microbiome, influencing the immune response of the lung. The disrupted immune-gut homeostasis may further affect disease progression due to heightened inflammatory responses (Dhar and Mohanty, 2020). ACE2, a receptor for SARS-CoV-2, regulates amino acid transport in the intestine, playing a critical role in modulating innate immunity (Perlot and Penninger, 2013). Thus, SARS-CoV-2 infection may also influence the gut microbiome through the ACE2 activity-dependent modulation of host immunity.
Our study revealed that several butyrate-producing bacteria, such as Oscillibacter, Subdoligranulum, and Anaerostipes were abundantly present in late convalescent COVID-19 patients compared to early recovered phase patients. This finding aligns with the observation that patients with severe COVID-19 often exhibit impaired production of bacterial metabolites such as short-chain fatty acids (SCFAs), which include butyrate (Zhang et al., 2022). Given that SCFAs possess anti-inflammatory properties, the reduced abundance of butyrate-producing bacteria may have contributed to the severity of COVID-19. Although it was not a significant difference in our data analysis due to an insufficient sample size; however, other literature indicates that it is also related to the patient’s hospitalization duration and the severity of respiratory infections (Haak et al., 2018; Kullberg et al., 2024).
Stool samples were collected post-recovery, allowing us to detect microbiome changes in patients re-infected with SARS-CoV-2. In the re-infected group, Lachnospiraceae and Faecalimonas umbilicata were dominant bacteria compared to the one-time infected group. While gut microbiota composition alone may not be the sole determinant of re-infection, it raises intriguing questions about the potential role of dysbiosis in predisposing individuals to re-infection. Exploring whether individuals with incomplete recovery of their microbiome community are at a higher risk of re-infection could provide valuable insights, especially in the context of seasonal flu or endemic SARS-CoV-2. Further comparative studies are needed to help validate this hypothesis.
While the severity of COVID-19 has diminished compared to the initial virulence of the SARS-CoV-2 virus (Ciceri et al., 2020; Lan et al., 2021) and has transitioned from a pandemic to an endemic state, identifying high-risk groups remains crucial for better preparedness for future pandemics. Our findings offer a potential pathway for identifying biomarkers that could pinpoint dominant microbiome species linked to disease severity and the likelihood of re-infection.
We also note that this study has several limitations. First, we did not conduct live viral culture or immune correlate analyses alongside microbiome analyses. Secondly, stool samples from deceased COVID-19 patients (the most severe group) were not included in our analysis. Additionally, the sample size of moderate and severe COVID-19 patients was relatively small, underscoring the need for larger-scale studies. Thirdly, the majority of our subjects were infected with wild-type and delta variant viruses, and data from individuals infected with omicron and their sub-lineage variants were not included. The Korea Disease Control and Prevention Agency confirmed the SARS-CoV-2 mutation pattern through sample surveillance, and divided the epidemic period based on the week in which more than 50% of the weekly variant virus tests were detected. The period when the samples were collected coincides with the period when wild type SARS-CoV-2 and delta variant were dominant. Lastly, we did not account for other factors that likely influence the gut microbiome, such as diet, lifestyle, eating habits, and living environment.
Despite these limitations, our study holds significance for several reasons. Firstly, we recruited a larger number of subjects compared to previously published studies. Secondly, we utilized follow-up data, including samples collected at seven-month post-recovery, for our analyses. We successfully identified bacteria that could serve as indicators of severe COVID-19, laying the groundwork for future predictive modeling efforts and mechanistic studies using preclinical models. Furthermore, we demonstrated that even after accounting for the effects of antibiotics and age, the severity of COVID-19 remained significantly associated with changes in microbial communities. Lastly, despite its correlative nature, our data suggest the potential utility of the microbiome as a biomarker for assessing susceptibility to re-infection.
In summary, our study reveals distinct differences in the stool microbiome composition between COVID-19 patients and uninfected controls, particularly evident during the early convalescent phase, with pronounced variations observed in the severe disease group. Notably, the severity of COVID-19 infection emerged as the most influential clinical factor in shaping gut dysbiosis, surpassing even the impact of antibiotic usage. These findings underscore the potential of predominant opportunistic microorganisms as biomarkers for assessing the severity of respiratory infectious diseases.
Data availability statement
The datasets presented in this study can be found in online repositories. The names of the repository/repositories and accession number(s) can be found in the article/Supplementary Material.
Ethics statement
The protocol for this prospective study was reviewed and approved by the Institutional Review Board of Gangnam Severance Hospital, Yonsei University College of Medicine in Seoul, South Korea (Reg. No. 3-2020-0110), Severance Hospital, Yonsei University College of Medicine, Seoul, South Korea (Reg. No. 4-2020-0966) and Seoul Medical Center in Seoul, South Korea (Reg. No. 2020-07-007). The studies were conducted in accordance with the local legislation and institutional requirements. The participants provided their written informed consent to participate in this study. Written informed consent was obtained from the individual(s) for the publication of any potentially identifiable images or data included in this article.
Author contributions
KL: Conceptualization, Formal analysis, Investigation, Writing – original draft. YK: Conceptualization, Formal analysis, Investigation, Writing – original draft. SD: Conceptualization, Formal analysis, Investigation, Writing – original draft. JY: Writing – review & editing. H-SO: Formal analysis, Writing – review & editing. JL: Formal analysis, Writing – review & editing. S-JY: Formal analysis, Writing – review & editing. IC: Formal analysis, Writing – review & editing. JC: Formal analysis, Writing – review & editing. EL: Data curation, Investigation, Writing – review & editing. SJ: Data curation, Investigation, Writing – review & editing. WW: Data curation, Investigation, Writing – review & editing. J-PC: Data curation, Investigation, Writing – review & editing. SH: Data curation, Investigation, Writing – review & editing. GC: Conceptualization, Writing – review & editing. JH: Conceptualization, Writing – review & editing. LK: Conceptualization, Supervision, Writing – review & editing. YS: Conceptualization, Supervision, Writing – review & editing.
Funding
The author(s) declare financial support was received for the research, authorship, and/or publication of this article. This study was supported by a faculty research grant from Yonsei University College of Medicine (Grant No. 6-2020-0238). This work was also supported by National Research Foundation of Korea (NRF) grants funded by the Korean government (MSIT) (NRF 2023R1A2C1005804 and RS-2024-00408822).
Acknowledgments
We thank all laboratory researchers belonging to the Department of Internal Medicine at Severance Hospital and Seoul Medical Center for collecting the clinical data and stool samples.
Conflict of interest
Authors YK, H-SO, JL, S-JY, IC, JC were employed by the company CJ Bioscience, Inc. JH and GC are consultants for CJ Cheiljedang and Interon Laboratories, Inc.
The remaining authors declare that the research was conducted in the absence of any commercial or financial relationships that could be construed as a potential conflict of interest.
Publisher’s note
All claims expressed in this article are solely those of the authors and do not necessarily represent those of their affiliated organizations, or those of the publisher, the editors and the reviewers. Any product that may be evaluated in this article, or claim that may be made by its manufacturer, is not guaranteed or endorsed by the publisher.
Supplementary material
The Supplementary Material for this article can be found online at: https://www.frontiersin.org/articles/10.3389/fcimb.2024.1455295/full#supplementary-material
References
Chen, Y., Gu, S., Chen, Y., Lu, H., Shi, D., Guo, J., et al. (2022). Six-month follow-up of gut microbiota richness in patients with COVID-19. Gut 71, 222–225. doi: 10.1136/gutjnl-2021-324090
Ciceri, F., Ruggeri, A., Lembo, R., Puglisi, R., Landoni, G., Zangrillo, A., et al. (2020). Decreased in-hospital mortality in patients with COVID-19 pneumonia. Pathog. Glob Health 114, 281–282. doi: 10.1080/20477724.2020.1785782
Dai, Y. J., Hu, F., Li, H., Huang, H. Y., Wang, D. W., Liang, Y. (2020). A profiling analysis on the receptor ACE2 expression reveals the potential risk of different type of cancers vulnerable to SARS-CoV-2 infection. Ann. Transl. Med. 8, 481. doi: 10.21037/atm.2020.03.61
Dhar, D., Mohanty, A. (2020). Gut microbiota and Covid-19-possible link and implications. Virus Res. 285, 198018. doi: 10.1016/j.virusres.2020.198018
Donati Zeppa, S., Agostini, D., Piccoli, G., Stocchi, V., Sestili, P. (2020). Gut microbiota status in COVID-19: an unrecognized player? Front. Cell Infect. Microbiol. 10. doi: 10.3389/fcimb.2020.576551
Du, L., He, Y., Zhou, Y., Liu, S., Zheng, B. J., Jiang, S. (2009). The spike protein of SARS-CoV–a target for vaccine and therapeutic development. Nat. Rev. Microbiol. 7, 226–236. doi: 10.1038/nrmicro2090
Gu, S., Chen, Y., Wu, Z., Chen, Y., Gao, H., Lv, L., et al. (2020). Alterations of the gut microbiota in patients with coronavirus disease 2019 or H1N1 influenza. Clin. Infect. Dis. 71, 2669–2678. doi: 10.1093/cid/ciaa709
Haak, B. W., Littmann, E. R., Chaubard, J. L., Pickard, A. J., Fontana, E., Adhi, F., et al. (2018). Impact of gut colonization with butyrate-producing microbiota on respiratory viral infection following allo-HCT. Blood 131, 2978–2986. doi: 10.1182/blood-2018-01-828996
Han, C., Duan, C., Zhang, S., Spiegel, B., Shi, H., Wang, W., et al. (2020). Digestive symptoms in COVID-19 patients with mild disease severity: clinical presentation, stool viral RNA testing, and outcomes. Am. J. Gastroenterol. 115, 916–923. doi: 10.14309/ajg.0000000000000664
Hanada, S., Pirzadeh, M., Carver, K. Y., Deng, J. C. (2018). Respiratory viral infection-induced microbiome alterations and secondary bacterial pneumonia. Front. Immunol. 9. doi: 10.3389/fimmu.2018.02640
Jia, H. P., Look, D. C., Shi, L., Hickey, M., Pewe, L., Netland, J., et al. (2005). ACE2 receptor expression and severe acute respiratory syndrome coronavirus infection depend on differentiation of human airway epithelia. J. Virol. 79, 14614–14621. doi: 10.1128/JVI.79.23.14614-14621.2005
Karim, S. S. A., Karim, Q. A. (2021). Omicron SARS-CoV-2 variant: a new chapter in the COVID-19 pandemic. Lancet 398, 2126–2128. doi: 10.1016/s0140-6736(21)02758-6
Keely, S., Talley, N. J., Hansbro, P. M. (2012). Pulmonary-intestinal cross-talk in mucosal inflammatory disease. Mucosal Immunol. 5, 7–18. doi: 10.1038/mi.2011.55
Kullberg, R. F. J., Wikki, I., Haak, B. W., Kauko, A., Galenkamp, H., Peters-Sengers, H., et al. (2024). Association between butyrate-producing gut bacteria and the risk of infectious disease hospitalisation: results from two observational, population-based microbiome studies. Lancet Microbe. 5, 100864. doi: 10.1016/S2666-5247(24)00079-X
Lan, F.-Y., Filler, R., Mathew, S., Iliaki, E., Osgood, R., Bruno-Murtha, L. A., et al. (2021). Evolving virulence? Decreasing COVID-19 complications among Massachusetts healthcare workers: a cohort study. Pathog. Global Health 115, 4–6. doi: 10.1080/20477724.2020.1847778
Liu, Q., Mak, J. W. Y., Su, Q., Yeoh, Y. K., Lui, G. C., Ng, S. S. S., et al. (2022). Gut microbiota dynamics in a prospective cohort of patients with post-acute COVID-19 syndrome. Gut 71, 544–552. doi: 10.1136/gutjnl-2021-325989
Perlot, T., Penninger, J. M. (2013). ACE2 - from the renin-angiotensin system to gut microbiota and malnutrition. Microbes Infect. 15, 866–873. doi: 10.1016/j.micinf.2013.08.003
Rubio-Rivas, M., Mora-Luján, J. M., Formiga, F., Arévalo-Cañas, C., Lebrón Ramos, J. M., Villalba García, M. V., et al. (2022). WHO ordinal scale and inflammation risk categories in COVID-19. Comparative study of the severity scales. J. Gen. Internal Med. 37, 1980–1987. doi: 10.1007/s11606-022-07511-7
Shi, H. Y., Zhu, X., Li, W. L., Mak, J. W. Y., Wong, S. H., Zhu, S. T., et al. (2021). Modulation of gut microbiota protects against viral respiratory tract infections: a systematic review of animal and clinical studies. Eur. J. Nutr. 60, 4151–4174. doi: 10.1007/s00394-021-02519-x
Stefan, K. L., Kim, M. V., Iwasaki, A., Kasper, D. L. (2020). Commensal microbiota modulation of natural resistance to virus infection. Cell 183, 1312–1324.e1310. doi: 10.1016/j.cell.2020.10.047
Sun, Z., Song, Z. G., Liu, C., Tan, S., Lin, S., Zhu, J., et al. (2022). Gut microbiome alterations and gut barrier dysfunction are associated with host immune homeostasis in COVID-19 patients. BMC Med. 20, 24. doi: 10.1186/s12916-021-02212-0
Sungnak, W., Huang, N., Bécavin, C., Berg, M., Queen, R., Litvinukova, M., et al. (2020). SARS-CoV-2 entry factors are highly expressed in nasal epithelial cells together with innate immune genes. Nat. Med. 26, 681–687. doi: 10.1038/s41591-020-0868-6
Wang, Q., Zhang, Y., Wu, L., Niu, S., Song, C., Zhang, Z., et al. (2020). Structural and functional basis of SARS-coV-2 entry by using human ACE2. Cell 181, 894–904.e899. doi: 10.1016/j.cell.2020.03.045
Yamamoto, S., Saito, M., Tamura, A., Prawisuda, D., Mizutani, T., Yotsuyanagi, H. (2021). The human microbiome and COVID-19: A systematic review. PloS One 16, e0253293. doi: 10.1371/journal.pone.0253293
Yildiz, S., Mazel-Sanchez, B., Kandasamy, M., Manicassamy, B., Schmolke, M. (2018). Influenza A virus infection impacts systemic microbiota dynamics and causes quantitative enteric dysbiosis. Microbiome 6, 1–17. doi: 10.1186/s40168-017-0386-z
Yoon, H., Lee, D. H., Lee, J. H., Kwon, J. E., Shin, C. M., Yang, S.-J., et al. (2021). Characteristics of the gut microbiome of healthy young male soldiers in South Korea: the effects of smoking. Gut Liver 15, 243. doi: 10.5009/gnl19354
Zhang, F., Wan, Y., Zuo, T., Yeoh, Y. K., Liu, Q., Zhang, L., et al. (2022). Prolonged impairment of short-chain fatty acid and L-isoleucine biosynthesis in gut microbiome in patients with COVID-19. Gastroenterology 162, 548–561.e544. doi: 10.1053/j.gastro.2021.10.013
Zuo, T., Wu, X., Wen, W., Lan, P. (2021). Gut microbiome alterations in COVID-19. Genomics Proteomics Bioinf. 19, 679–688. doi: 10.1016/j.gpb.2021.09.004
Zuo, T., Zhan, H., Zhang, F., Liu, Q., Tso, E. Y. K., Lui, G. C. Y., et al. (2020a). Alterations in fecal fungal microbiome of patients with COVID-19 during time of hospitalization until discharge. Gastroenterology 159, 1302–1310.e1305. doi: 10.1053/j.gastro.2020.06.048
Keywords: stool microbiome, metagenomics, SARS-CoV-2, severity of coronavirus disease 2019 (COVID-19), convalescent patients
Citation: Lee KH, Kim YO, Dho SH, Yong JJH, Oh H-S, Lee JH, Yang S-J, Cha I, Chun J, Lee EH, Jeong SJ, Woo W, Choi J-P, Han SH, Choi GB, Huh JR, Kim LK and Song YG (2024) Altered gut microbiome in convalescent patients with coronavirus disease 2019. Front. Cell. Infect. Microbiol. 14:1455295. doi: 10.3389/fcimb.2024.1455295
Received: 26 June 2024; Accepted: 07 November 2024;
Published: 28 November 2024.
Edited by:
Kundlik Gadhave, Johns Hopkins University, United StatesReviewed by:
Paolo Vassalini, Central Hospital of Belcolle, ItalyJordi Espadaler Mazo, AB-Biotics SA, Spain
Copyright © 2024 Lee, Kim, Dho, Yong, Oh, Lee, Yang, Cha, Chun, Lee, Jeong, Woo, Choi, Han, Choi, Huh, Kim and Song. This is an open-access article distributed under the terms of the Creative Commons Attribution License (CC BY). The use, distribution or reproduction in other forums is permitted, provided the original author(s) and the copyright owner(s) are credited and that the original publication in this journal is cited, in accordance with accepted academic practice. No use, distribution or reproduction is permitted which does not comply with these terms.
*Correspondence: Young Goo Song, SW1mZWxsQHl1aHMuYWM=; Lark Kyun Kim, TEtLSU1AeXVocy5hYw==; Jun R. Huh, SnVuX0h1aEBobXMuaGFydmFyZC5lZHU=
†These authors have contributed equally to this work