- 1Department of the Fifth Division, Peking University School and Hospital of Stomatology and National Center for Stomatology and National Clinical Research Center for Oral Diseases and National Engineering Research Center of Oral Biomaterials and Digital Medical Devices and Beijing Key Laboratory of Digital Stomatology and National Healty Center (NHC) Key Laboratory of Digital Stomatology and National Medical Products Administration (NMPA) Key Laboratory for Dental Materials, Beijing, China
- 2Peking University School and Hospital of Stomatology and National Center for Stomatology and National Clinical Research Center for Oral Diseases and National Engineering Research Center of Oral Biomaterials and Digital Medical Devices and Beijing Key Laboratory of Digital Stomatology and National Healty Center (NHC) Key Laboratory of Digital Stomatology and National Medical Products Administration National (NMPA) Key Laboratory for Dental Material, Beijing, China
- 3Stomatology Hospital, School of Stomatology, Zhejiang University School of Medicine, Zhejiang Provincial Clinical Research Center for Oral Diseases, Key Laboratory of Oral Biomedical Research of Zhejiang Province, Cancer Center of Zhejiang University, Engineering Research Center of Oral Biomaterials and Devices of Zhejiang Province, Hangzhou, China
- 4Department of Prosthodontics, Peking University School and Hospital of Stomatology and National Center for Stomatology and National Clinical Research Center for Oral Diseases and National Engineering Research Center of Oral Biomaterials and Digital Medical Devices and Beijing Key Laboratory of Digital Stomatology and National Healty Center (NHC) Key Laboratory of Digital Stomatology and National Medical Products Administration National (NMPA) Key Laboratory for Dental Materials, Beijing, China
- 5Department of Periodontology, Peking University School and Hospital of Stomatology and National Center for Stomatology and National Clinical Research Center for Oral Diseases and National Engineering Research Center of Oral Biomaterials and Digital Medical Devices and Beijing Key Laboratory of Digital Stomatology and National Healty Center (NHC) Key Laboratory of Digital Stomatology and National Medical Products Administration National (NMPA) Key Laboratory for Dental Materials, Beijing, China
Objective: The purpose of this systematic bioinformatics analysis was to describe the compositions and differences in submucosal microbial profiles of peri-implants’ diseases and healthy implant.
Material and methods: PubMed, Embase, ETH Z, Scopus, CNKI, and Wanfang databases were searched to screen relevant literature on the analysis of peri-implant microflora based on the sequencing analysis technique of 16S ribosomal RNA (16S rRNA) gene. High-throughput sequencing of the 16S rRNA gene of microorganisms from healthy implants, peri-implant mucositis, and peri-implantitis was downloaded from the screened articles. EasyAmplicon and Usearch global algorithm were used to match the reads from each dataset to a full length of 16S rRNA or ITS gene sequence. The microorganisms based on the Human Oral Microbiome Database (HOMD) were re-classified, and the microbial diversity, flora composition, and differential species of the samples were re-analyzed, including taxonomic classification and alpha and beta diversity calculations. The co-occurrence network was also re-analyzed.
Results: A total of seven articles with 240 implants were included. Among them, 51 were healthy implants (HI), 43 were in the peri-implant mucositis (PM) group, and 146 were in the peri-implantitis (PI) group. A total of 26,483 OTUs were obtained, and 877 microorganisms were annotated. The alpha diversity including Chao1 (healthy implants, 121.04 ± 92.76; peri-implant mucositis, 128.21 ± 66.77; peri-implantitis, 131.15 ± 84.69) and Shannon (healthy implants, 3.25 ± 0.65; peri-implant mucositis, 3.73 ± 0.61; peri-implantitis, 3.53 ± 0.67) of the samples from the three groups showed a significant difference. The beta diversity of the three samples was statistically different among groups. The genera of Treponema and Fretibacterium were significantly more abundant in the PI group than in the other two groups, and the genus of Streptococcus was more abundant in the HI group. The relative abundance of Porphyromonas in the peri-implantitis group was 6.1%. The results of the co-occurrence network showed differences in the network topology among the three groups of samples. The most connected three genera in the healthy implants were Halomonas, Fusobacterium, and Fretibacterium. The most connected three genera in peri-implant mucositis were Alistipes, Clostridia UCG-014, and Candidatus Saccharimonas. The most connected three genera in the peri-implantitis group were Lachnoanaerobaculum, Fusobacterium, and Atopobium. The betweenness of Porphvromonas gingivalis (red complex) in the PI group (7,900) was higher than in the HI group (23).
Conclusions: The community compositions of peri-implant submucosal microorganisms were significantly different in healthy implants, peri-implant mucositis, and peri-implantitis. The submucosal microbial communities in peri-implantitis were characterized by high species richness and diversity compared with the healthy implants; the relative abundance of red complex, some members of the yellow complex, and some novel periodontal pathogens was higher in the peri-implantitis and peri-implant mucositis groups than in the healthy implant group. The core flora of the co-occurrence network of healthy implants, peri-implant mucositis, and peri-implantitis varied considerably. The peri-implantitis site presented a relative disequilibrium microbial community, and Porphyromonas may play an important role in the co-occurrence network.
1 Introduction
Peri-implantitis has been defined as a plaque-associated pathological condition affecting tissues around dental implants (Berglundh et al., 2018; Doornewaard et al., 2017). A systematic review of the incidence of peri-implantitis showed that, at the patient level, the average prevalence of peri-implantitis was 19.53%, and at the implant level, it was 12.53% (Diaz et al., 2022). Peri-implantitis can lead to progressive bone loss, making it the main cause for dental implant failure (Heitz-Mayfield et al., 2018). Numerous experimental and clinical studies have shown that peri-implantitis is a pathological condition related to plaque formation in the tissues surrounding dental implants (Berglundh et al., 2018; Darby, 2022). Studies, which were conducted by traditional microbial culture techniques, have found that there was a significant difference in the composition of plaque biofilm between infected implants and healthy implants (Mombelli and Mericske-Stern, 1990; Hannig, 1997). Imbalances in the oral microbiome and plaque accumulation are closely associated with the development of peri-implant mucositis and peri-implantitis. Early attempts to identify and classify the bacterial components of subgingival plaque from peri-implantitis relied on bacterial cultivation techniques, with a focus on known periodontal pathogenic bacterial species (Mombelli and Mericske-Stern, 1990; Shibli et al., 2008). However, approximately 53% of oral microorganisms have not yet been named, and 35% have not yet been cultured (Chen et al., 2010). Differences in cultivation environments can also lead to biases in microbial composition, missing other possible microbial communities associated with peri-implantitis. Therefore, traditional bacterial cultivation techniques have technological limitations when they were applied to identify the dental implant microbiome’s composition.
At present, sequence analysis of the 16S ribosomal RNA (rRNA) gene is a powerful mechanism to identify new pathogens in patients with suspected bacterial disease, and more recently this technology has been applied in the clinical laboratory for routine identification of bacterial isolates (Patel, 2001). Therefore, 16S rRNA sequencing has become the main method to study the composition and distribution of microbial communities. It has been widely applied in the study of oral microbial communities under non-culture conditions (Sun et al., 2023). The Human Oral Microbiome Database (HOMD) is an international standard human oral microbiome bioinformatics database, which covers more than 600 types of prokaryotic microorganisms in the oral cavity and is used for data sharing of oral microorganisms (Chen et al., 2010). Similarly, the Oral Microbiome Bank of China (OMBC) was established in 2018 to build a Chinese-related oral microbiome bioinformatics platform (Xian et al., 2018).
In recent years, it has been found that the submucosal flora around an implant is an independent ecosystem with its unique community structure (Belibasakis and Manoil, 2021). The uniqueness of the microbial community in peri-implantitis infections is well acknowledged compared with periodontal pathogens. Koyanagi T et al (Koyanagi et al., 2010). first studied the submucosal biofilm of dental implants using 16S rRNA gene clone library technique in 2010. Some specific groups of microorganisms, belonging to Chloroflexi, Tenericutes, Synergistetes, and Firmicutes, were only found in peri-implantitis lesions. Kumar PS et al (Kumar et al., 2012). used second-generation sequencing technology to compare the submucosal flora between implants and natural teeth, showing that the abundance of Gram-negative anaerobic bacteria in normal implant teeth was higher than that in peri-implantitis and periodontitis sites. Many literatures showed submucosal microbiomes similarity between per-implantitis and periodontitis sites and found the complexity and uniqueness of peri-implant-related bacterial communities (Yu et al., 2019; Kotsakis and Olmedo, 2021).
Recently, a few studies have used 16S rRNA sequencing to establish the core microbiota around an implant. Results vary from study to study. The amplification of the V1–V3 region of the 16S rRNA gene was performed in some studies (Zheng et al., 2015; Nie et al., 2020), while the amplification of the V3–V4 region of the 16S rRNA gene was performed in other studies (Maruyama et al., 2014; Komatsu et al., 2020; Yu et al., 2019). Due to the heterogeneity of the papers, it is impossible to analyze data from published articles in a straightforward aggregated manner. Recently, high-throughput sequencing of the full gene of 16S rRNA has become a widely accepted technique (Johnson et al., 2019). Sequencing the entire 16S rRNA gene provides real and significant advantages over sequencing a partial 16S rRNA gene. None of the variable regions covered by partial 16S sequencing were able to recapture the diversity represented when sequencing the full-length gene (Yarza et al., 2014). High-throughput sequencing of the full gene is more accurate but expensive. Research to explore core microbiota around an implant base on full-length 16S rRNA gene sequencing were rarely reported. Amplicon sequencing and Usearch global algorithm could be used to match the reads from a partial region to a full length of 16S rRNA (Nishio et al., 2023; Rognes et al., 2016; Yuan et al., 2020). With the help of these methods, data from literatures based on different gene regions could be integrated and re-analyzed. In this paper, the partial region gene reads from previous implant-related literatures based on various gene regions were matched to a full length and then re-classified and summarized based on a full length of 16S rRNA gene sequencing and HOMD. The microorganisms in healthy implants and their disease states were re-explored.
2 Materials and methods
2.1 Literature collection
Search terms identified using MeSH search terms relevant to the focus area were combined and applied using Boolean operators, “OR” or “AND”, as appropriate in the searched databases. The exact search term used was (“peri-implant” OR “peri-implantitis” OR “implant mucositis” OR “implant disease” OR “implant”) AND (“16s rRNA” OR “microbiome” OR “microbial profile” OR “sequencing” OR “PacBio SMRT”). Systematic searches were performed at the academic databases PubMed, EMbase, ETHZ, and Scopus. At the same time, corresponding Chinese vocabulary of “16s rRNA” AND “implant” OR “peri-implantitis” were used as keywords in the Chinese database CNKI, Wanfang, Peking University Map to search and screen Chinese literatures. Two co-investigators (Xinran Guo and Jinchen Ye) independently performed systematic searches of the aforementioned databases and sources. The search results were screened based on manuscript titles to select studies for abstract review, and then, based on the abstract reading, studies were identified for full-text analysis. Any disagreement among the co-investigators in the selection of studies for screening and/or for final inclusion was resolved by mutual discussion. Studies meeting the eligibility criteria were included for data extraction. All literatures published between 2010 and 2024 (up to July 2024) were reviewed and searched (Figure 1).
2.2 Inclusion criteria
Following are the inclusion criteria: (1) 16s rRNA gene-based Sanger sequencing (first-generation sequencing), high-throughput sequencing (second-generation sequencing), and PacBio SMRT (third-generation sequencing); (2) the research objects are humans; and (3) the diagnosis of the subject meets the criteria for “healthy implant”, “peri-implantitis”, and/or “peri-implant mucositis”, in accordance with previous research (Berglundh et al., 2018; Caton et al., 2018).
2.3 Exclusion criteria
The exclusion criteria are as follows: (1) the original literature was not available, (2) the original data and primer sequences of the experimental study were not available, and (3) the original data lacks relevant sample information.
2.4 Bioinformatics analysis and statistical analysis
The original sequencing data, downloaded from the screened articles, were divided into three groups, including healthy implant (HI), peri-implant mucositis implant (PM), and peri-implantitis implant (PI) based on the clinical diagnosis of the samples. Serial reading quality was checked by using the FastQC V. 0.11.5 program. The two ends of sequencing reads were processed by join with join _ -pair _ ends.py program in QIIME (version 1.9.0) and then trimmed to a Phred score of at least 20 by using split _ libraries _ fastq. py program in QIIME (version 1.9.0). The EasyAmplicon pipeline (https://github.com/YongxinLiu/EasyAmplicon) and Usearch global algorithm in VSEARCH 2.1.3 were then used to match the reads from each dataset to a full length of 16S rRNA. This process is based on a predefined set of sequences with known classifications (the manually curated Greengenes database 13.8 or the Unite database 7.2) (Rognes et al., 2016). The sequences are clustered into OTUs. The sequences of operational taxonomic units with 97% similarity were matched with the sequences in the Human Oral Microbiome Database (HOMD-V15.2). The class confidence was set to 0.7, and the data were sampled for species annotation analysis. The Chao1 index, richness index, Shannon index, and Simpson index were calculated at the OTU level to assess alpha diversity. Principal component analysis (based on Weighted UniFrac distance) was performed at the OTU level to assess beta diversity. An analysis of similarities (ANOSIM) was applied to compare the differences in flora structure among groups. Kruskal–Wallis test was performed to compare the microbial relative abundance at the phylum, genus, and species levels, respectively, and the false discovery rate (FDR) was calculated for the P-value to reduce the false positive rate and to analyze the species with differences between groups. SPSS 26.0 software (IBM Corporation) and GraphPad Prism 9 were used for statistics and graphing. The difference was statistically significant when bilateral P < 0.05. Linear discriminant analysis (LDA) effect size (LEfSe) was used to compare the relative abundances of the dominant species among the three groups. The co-occurrence network was constructed based on the Spearman correlation between OTUs of each sample. The OTUs whose frequency is less than 0.6 in all samples are eliminated, and the Spearman correlation between the OTUs were computed with the “Microeco” package in the R language; a co-occurrence network was constructed based on the threshold of Spearman correlation between OTUs with R value greater than 0. 6 and P-value less than 0. 05. The co-occurrence network was visualized by using Gephi 0.10 software, and each dot in the network represented an OTU. The node size was the abundance of each OTU, and the nodes were colored according to the module.
3 Results
3.1 General information and clinical indicators of implants
Through the literature search from 2010 to 2023, 863 articles were selected, and seven articles with 240 implants were included through further data collation. Three of the studies were cohort studies, two of them were case–control studies, and two studies were cross-sectional studies. Agency for Healthcare Research and Quality (AHQR) and Newcastle-Ottawa Scale (NOS) were used to evaluate the quality of these studies (Berkman et al., 2004; Wells et al., 2009) (Table 1). There were 51 healthy implants (HI), 43 peri-implant mucositis (PM), and 146 peri-implantitis (PI). General information is shown in Tables 2 and 3.
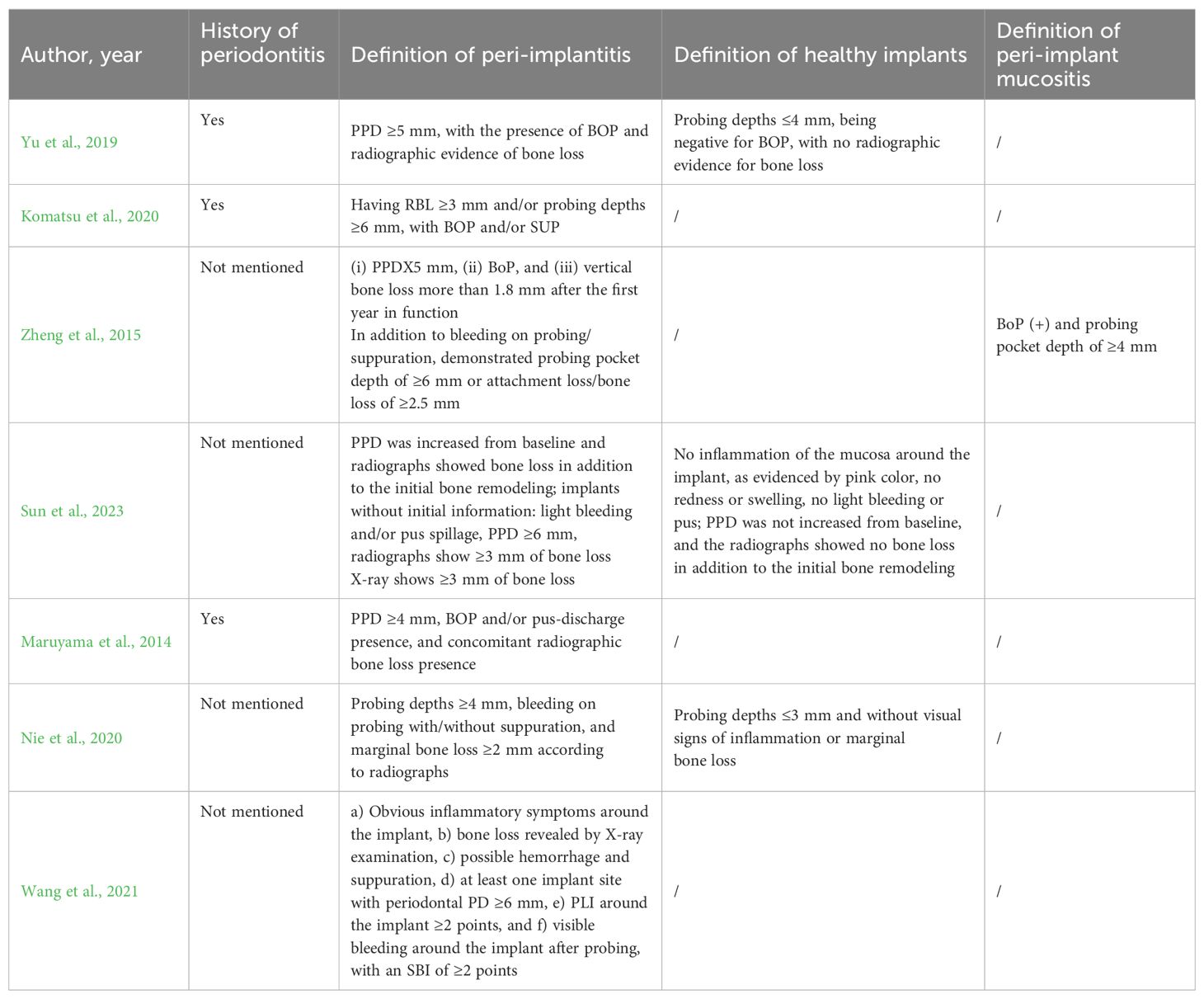
Table 3. History of periodontitis, definition of peri-implantitis, healthy implants, and peri-implant mucositis.
3.2 Composition of submucosal flora of implant
A total of 26,483 OTUs were obtained, and 877 microorganisms were annotated.
At the phylum level (see Figure 2A), Firmicutes, Proteobacteria, Bacteroidota, Actinobacteria, Fusobacteria, and Patescibacteria were the predominant microflora with abundance >5% in healthy implants, peri-implant mucositis, and peri-implantitis, occupying more than 80% of all sequences. The remaining bacteria belong to Synergistetes, Spirochaetes, Campilobacterota, Desulfobacteria, and unclassified bacteria.
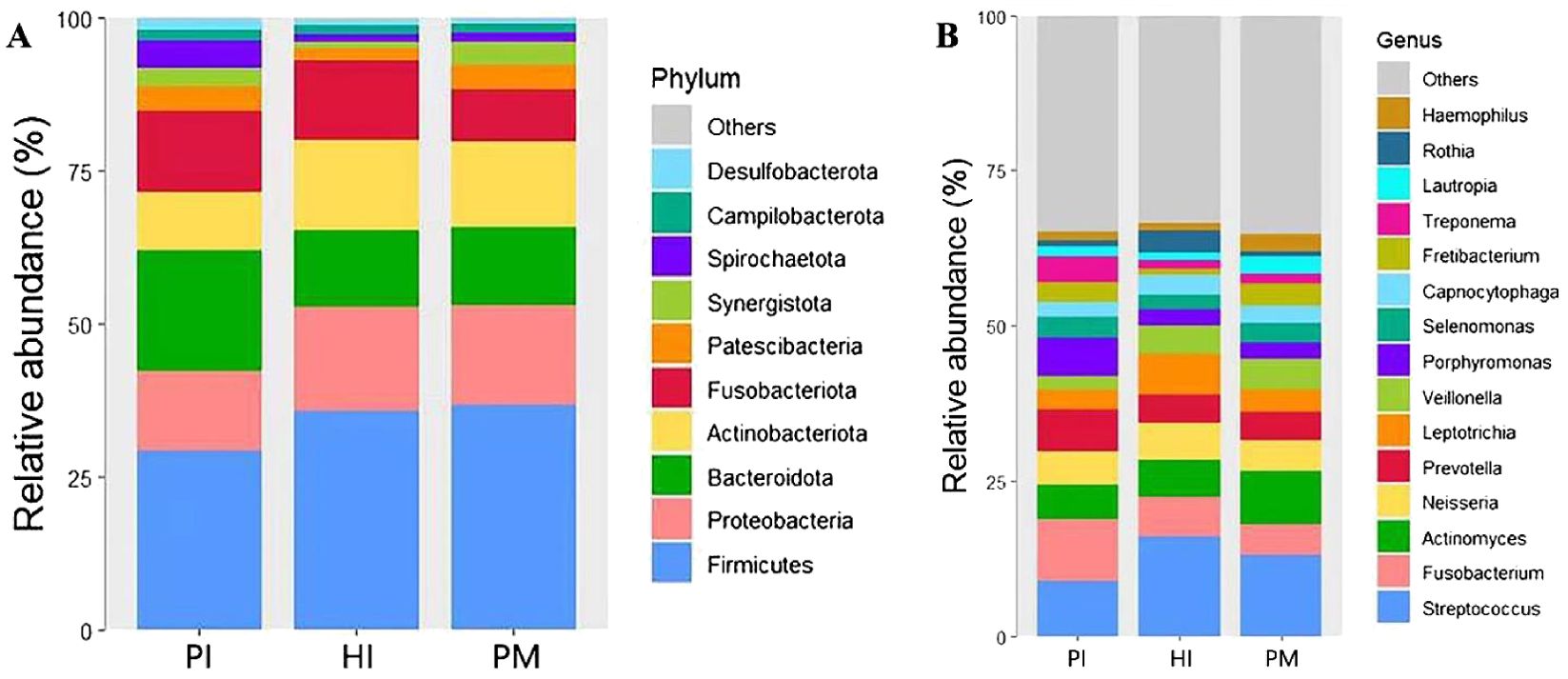
Figure 2. Species composition of submucosal microflora at the phylum and genus levels in healthy implants (HI), peri-implant mucositis (PM), and peri-implantitis (PI). (A) At the phylum level. (B) At the genus level.
At the genus level (Figure 2B), a total of 15 dominant genera with abundance >2% were detected, including Streptococcus, Fusobacterium, Actinomyces, Neisseria, Prevotella, Leptotrichia, Veillonella, Porphyromonas, Selenomonas, Capnocytophia, Fretibacterium, Treponema, Lautropia, Rothia, and Haemophilus. Its total abundance accounts for more than 70% of the total sample sequencing.
The heat map of species abundance in healthy implants, peri-implant mucositis, and peri-implantitis is shown at the phylum level in Figure 3A and at the genus level in Figure 3B. At the phylum level, the relative abundance of Bacteroidota and Spirochaetota in the PI group was significantly higher than the PM group (19.58% vs. 12.81%, P < 0.05; 4.34% vs.1.50%, P < 0.05). The relative abundance of Fusobacteriota in the PI group was higher (13.15%) than the PM groups (8.64%), but the difference was not statistically significant (P > 0.05). At the genus level, the relative abundance of Treponema in the PI group was significantly higher (4.32%) than the PM group (1.50%, P < 0.05). The relative abundance of Porphyromonas in the PI group was higher (6.10%) than the PM group (2.63%), but the difference was not statistically significant (P > 0.05).
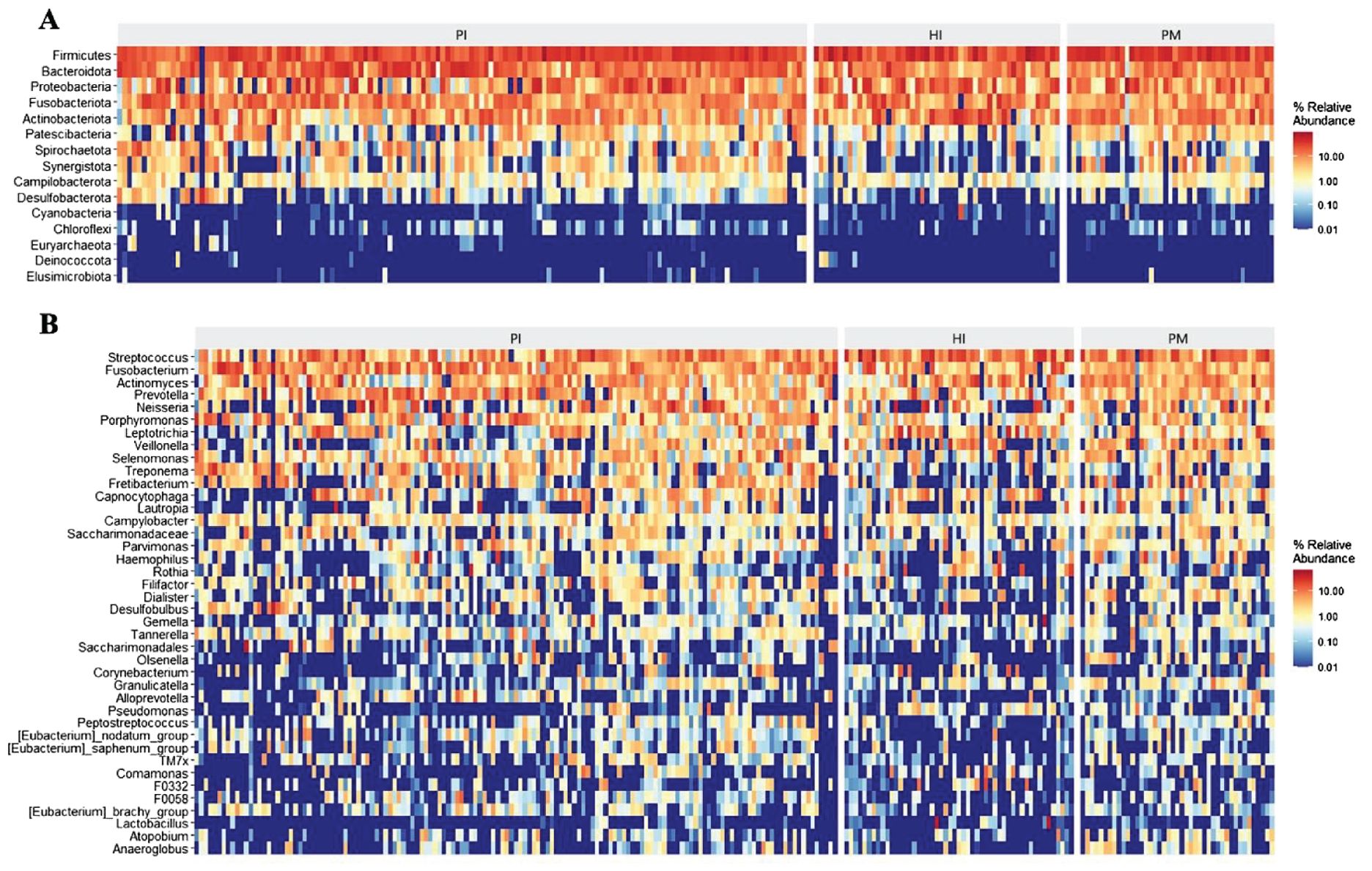
Figure 3. Microbial composition and abundance of submucosal microflora at phylum and genus levels in healthy implants (HI), peri-implant mucositis (PM), and peri-implantitis (PI). Heatmaps at the phylum level (A) and at the genus level (B).
3.3 Differences in the composition of submucosal flora of implants
There was a significant difference in the alpha diversity of the submucosal flora between the implant disease group and the healthy group as indicated by the higher richness–Chaol index (healthy implants: 121.04 ± 92.76, peri-implant mucositis: 128.21 ± 66.77, peri-implantitis: 131.15 ± 84.69; Figure 4A) and diversity–Shannon index (healthy implants: 3.25 ± 0.65, peri-implant mucositis: 3.73 ± 0.61, peri-implantitis: 3.53 ± 0.67; Figure 4B). The results of the principal component analysis based on Weighted UniFrac distance showed that the species composition of submucosal flora in healthy implants, peri-implant mucositis, and peri-implantitis, respectively, were significantly different (beta diversity, R2 = 0.04, P = 0.01, ANOSIM), and the principal component variables principal component 1 (PC 1) and PC2 were 8.2% and 7%, respectively (Figure 5).
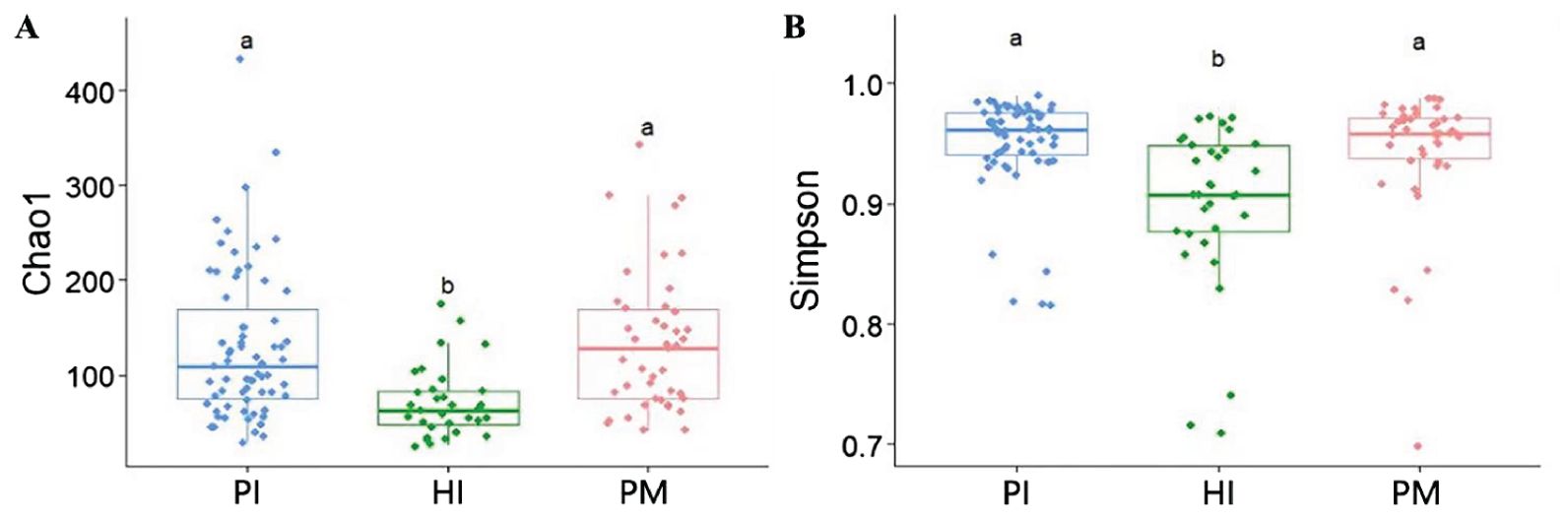
Figure 4. Comparison of α diversity of submucosal microflora of implants in peri-implantitis (PI), healthy implants (HI), and peri-implant mucositis (PM). (A) Microbial richness presented by Chao1 index. (B) Microbial diversity presented by Shannon index. Significant differences are marked with “a, b, c”; no common superscript denotes a significant difference (P < 0.05).
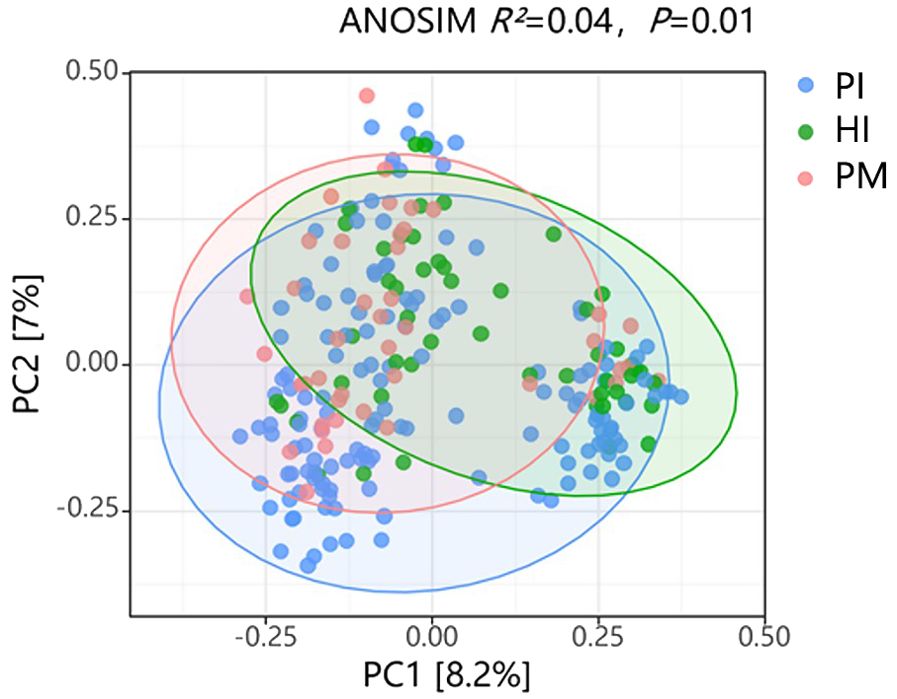
Figure 5. Comparison of β diversity of submucosal microflora in healthy implants (HI), peri-implantitis (PI), and peri-implant mucositis (PM). ANOSIM (analysis of similarities) R2 = 0.04, P = 0.01. PC, principal component.
The analysis of the species composition of the submucosal flora in healthy implants, peri-implant mucositis, and peri-implantitis using LEfSe analysis is shown in Figure 6. Corrected differences between the two groups at different classification levels were screened with the aid of the Kruskal–Wallis test and the FDR-corrected P-values P < 0.05. At the phylum level, the abundance of Bacteroidota and Spirochaetota in the peri-implantitis group was significantly higher than that in the other two groups, and Syntroph was more abundant in the healthy implant group. At the class level, the abundance of Bacteroidia and Clostridia in the peri-implantitis group was significantly higher than that in the other two groups. Bacillus was more abundant in the healthy implant group than in the control group. There was a significant increase in Coriobacteriia in the peri-implant mucositis group. At the family level, the abundance of Fusobacteriaceae, Porphyromonadaceae, and Spirochaetaceae in the peri-implant group was significantly higher than that in other groups. The abundance of Streptococcus was higher in the healthy implant group. At the order level, the abundance of Synergistales in the peri-implantitis group was significantly higher than that in the other two groups. The abundance of Lactobacillales was higher in the healthy implant group, and Coriobacteriales was significantly increased in peri-implant mucositis. At the genus level, Treponema and Fretibacterium were significantly more abundant in the peri-implantitis group than in the other two groups, and Streptococcus was more abundant in the healthy implant group.
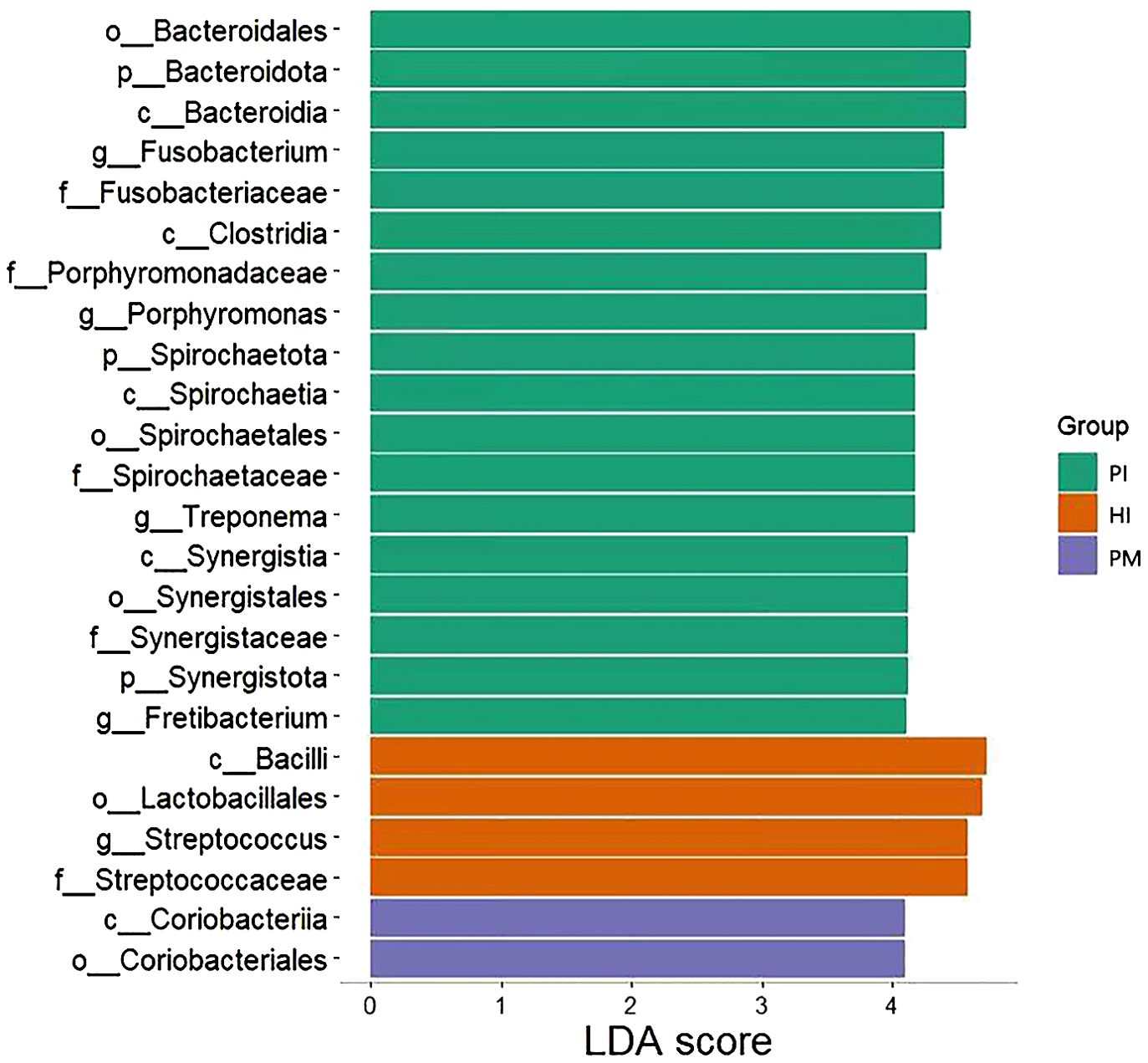
Figure 6. LEfSe analysis of submucosal flora of implants in healthy implants (HI), peri-implantitis (PI), and peri-implant mucositis (PM). LDA, linear discriminant analysis.
3.4 Co-occurrence network analysis reveals distinct microbial interaction patterns in healthy implant and peri-implant disease
In this study, the method of co-occurrence network analysis was used to explore the potential relationship between the microbial communities under healthy implants, peri-implant mucositis, and peri-implantitis (Figure 7). The results show that there are differences in the network topology of the three groups. The connectivity, interaction, node number, and density of bacteria in the healthy implant group and the peri-implant mucositis group were more than those in the peri-implantitis group. The microbial community of the peri-implantitis sites was less complex compared with the peri-implant mucositis sites. Positive correlations between species were found in the three groups (Supplementary Tables S1-S3).
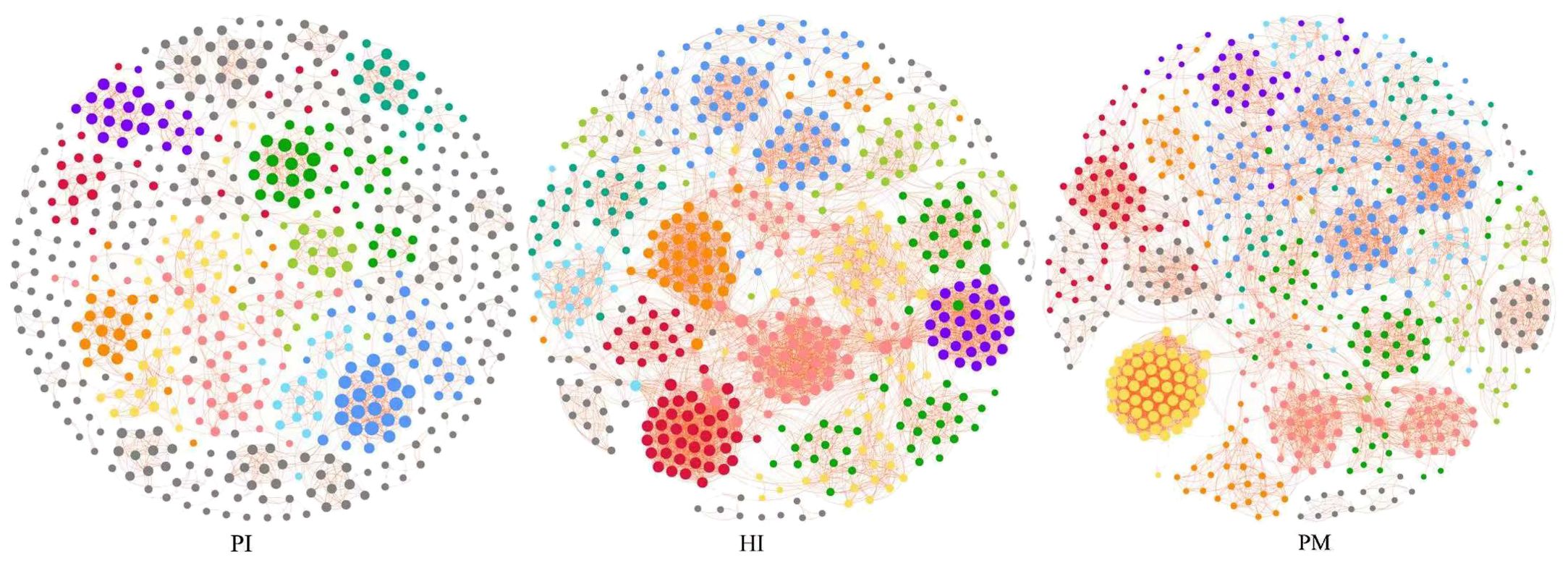
Figure 7. Co-occurrence network analysis: symbiotic network of three groups of microbial communities (network modules are shown in different colors). HI, healthy implants; PI, peri-implantitis; PM, peri-implant mucositis.
The relative abundances of the top six modules of each group are shown in Figure 8. The highest relative abundance module in the HI group was module 1, including Campylobacter_concisus (green complex), sputigena, Prevotella_saccharolytica, etc. The highest relative abundance module in the PI group was module 5, including Treponema_medium, Prevotella_intermedia (oragen complex), etc. The highest relative abundance module in the PM group was module 6, including Johnsonella_ignava, Actinomyces_israelii, Cardiobacterium_hominis, etc. Interestingly, despite the fact that the relative abundance of module 4 in the PM group was not very high, the degrees of species were more than 40. Module 4 included Erysipelotrichaceae_UCG-006, Peptostreptococcus_stomatis, Alistipes, etc.
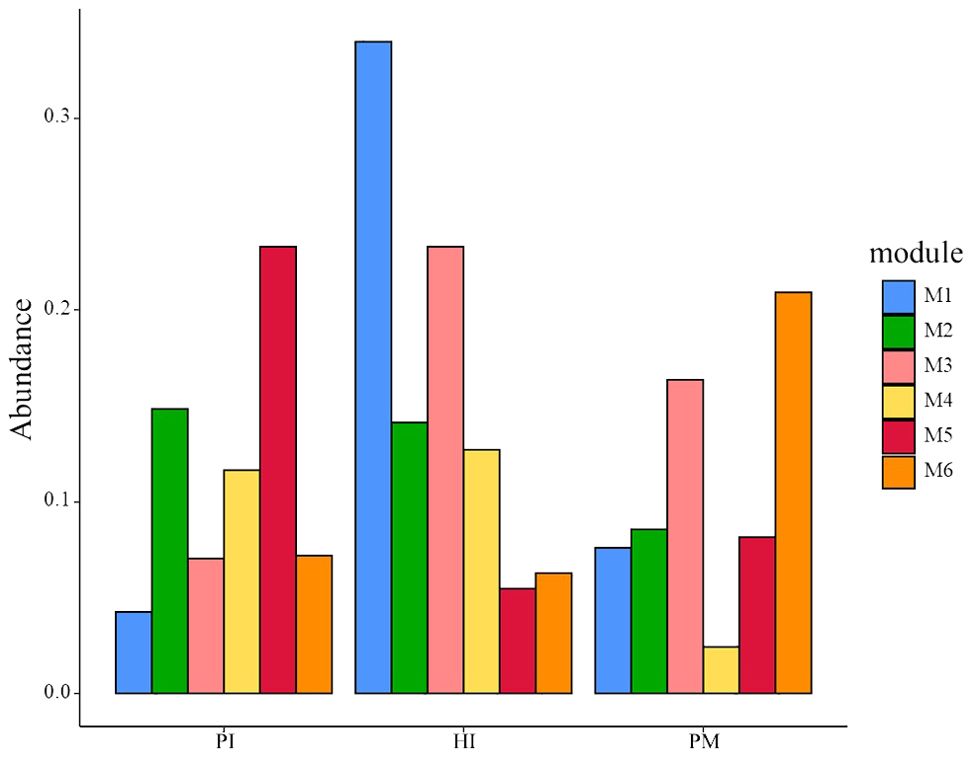
Figure 8. Relative abundances of the top six modules in three groups. HI, healthy implants; PI, peri-implantitis; PM, peri-implant mucositis.
We found that the core bacteria of the three groups are quite different, and these core bacteria are the key nodes of the group’s co-occurrence network. The most connected three genera of healthy implants were Fretibacterium (degree 57, betweenness 6001), Halomonas (degree 55, betweenness 8246) and Allorhizobium–Neorhizobium–Pararhizobium–Rhizobium (degree 51, betweenness 1707). The most connected three genera in peri-implant mucositis were Alistipes (degree 56, betweenness 5094), Clostridia UCG-014 (degree 55, betweenness 420), and Candidatus Saccharimonas (degree 55, betweenness 32). The most connected three genera in the peri-implantitis group were Lachnoanaerobaculum (degree 21, betweenness 3949), Fusobacterium (degree 21, betweenness 246), and Atopobium (degree 21, betweenness12). The betweenness of Bacteroides forsythus (red complex) in the PI group (9062) was higher than in the HI group (731). The betweenness of Porphvromonas gingivalis (red complex) in the PI group (7,900) was higher than in the HI group (23).
4 Discussion
Data from previous studies focusing on peri-implant microbial flora analysis based on 16s rRNA gene sequencing analysis technology was screened and downloaded in this study. The original sequencing data, downloaded from the screened articles, were divided into three groups, including healthy implant (HI), peri-implant mucositis implant (PM), and peri-implantitis implant (PI). Since two studies were conducted on the V1–V3 region and another five studies were conducted on the V3–V4 region, the Usearch global algorithm in VSEARCH 2.1.3 was then used to match the reads from each dataset to a full length of 16S rRNA or ITS gene sequence. The underlying database referred to (HOMD-V15.2) is also composed of full-length sequences. Then, the high-throughput sequencing samples of 16s rRNA gene of submucosal microorganisms in healthy implants, peri-implant mucositis, and peri-implantitis were analyzed. The microorganisms based on the Human Oral Microbiome Database (HOMD) were re-classified, and the microbial diversity, flora composition, and differential species of the samples were re-analyzed, including taxonomic classification and alpha and beta diversity calculations. The co-occurrence network was also re-analyzed. A total of 877 microorganisms were annotated.
Our results show that the dominant phyla of peri-implantitis are Firmicutes (29.13%), Bacteroidota (19.58%), Fusobacteriota (13.15%), and Proteobacteria (13.08%). The dominant phyla identified in this study in response to peri-implantitis was similar with the study of Sanz-Martin et al. (2017). Compared with the healthy implant, the abundance of Firmicutes was decreased and the abundance of Bacteroidetes was significantly increased in the peri-implantitis group. Similarly, a significantly higher relative abundance of Bacteroidota and Fusobacterium nucleatum, respectively, was found in the peri-implantitis group compared with healthy implant by Al-Ahmada et al. (P < 0.05). The major phyla of healthy implants were Firmicutes (51.9%), Bacteroidota (18.5%), Clostridia (11.1%), and Proteobacteria (7.4%), whereas the predominant phyla of peri-implantitis were Firmicutes (30.6%), Bacteroidota (40.3%), Clostridia (13.9%), and Proteobacteria (5.6%) (Al-Ahmad et al., 2018). Data were not included in this study because their data were not publicly shared. The dominant phylum around the implant changed according to different physiological conditions.
This study shows that the dominant genus of peri-implantitis are Fusobacterium (9.93%), Streptococcus (8.81%), Prevotella (6.79%), and Prophyromonas (6.10%). At the genus level, Porphyromonas and Treponema (4.33%), which are closely related to periodontal disease, were identified around peri-implantitis. However, the relative abundances of these periodontitis-related bacteria were lower in peri-implantitis sites than in periodontitis sites. This was consistent with the results of many previous studies (Kumar et al., 2012; Socransky et al., 1998; da Silva et al., 2014). Notably, the Human Oral Microbiome Database (HOMD) collected bacterial species distributed in the oral cavity region and the upper respiratory tract and found that the dominant phyla were Actinobacteria, Firmicutes, and Proteobacteria (Escapa et al., 2018). According to these studies, the flora distribution between periodontitis and peri-implantitis at the phylum level was different, and there were differences in the occurrence and development mechanism between peri-implant disease and periodontal disease. Similar results were also found in a previous study (Koyanagi et al., 2010).
Overall, microbiota had similar alpha diversity between the peri-implant mucositis (PM) and peri-implantitis (PI) groups. There were significant differences between them and the healthy implant group. The findings are consistent with previous studies (Hashimoto et al., 2022; Padial-Molina et al., 2024). Similar to a previous study (Li et al., 2015), the beta diversity of submucosal flora in healthy implants, peri-implantitis, and peri-implantitis was significantly different.
The abundance of Fretibacterium was higher in the peri-implantitis group than in the other two groups (Figure 6), which means it was one of the biomarkers of peri-implantitis. The effect of Fretibacterium on the development of periodontitis has been reported in the literature. This study suggests that this bacterium is related to periodontal pocket probing depth (PPD) and bleeding on probing (BOP) and positively correlated (P < 0.05) (Khemwong et al., 2019). Oliveira R et al. also reported related results, which proved that periodontitis is closely related to Fretibacterium (Oliveira et al., 2016). However, it has not been reported that this bacterium is significantly related to the occurrence of peri-implantitis. We have detected that there is a high species abundance in peri-implantitis by statistical analysis. Considering that it is an anaerobic Bacillus, we believe that its role and mechanism in the occurrence and development of peri-implantitis may be similar to those of periodontitis. However, its interaction with peri-implantitis needs further study.
In this study, we constructed a co-occurrence network of microorganisms based on Spearman’s rank correlation coefficients (Spearman coefficient >0.6). The connectivities between species in the three groups were quite different. The co-occurrence network of the peri-implantitis group was dominated by the red complex and showed a relative disequilibrium. Nie J et al. also found that the topology of the co-occurrence network of healthy implants is significantly different from that of peri-implantitis implants (Nie et al., 2020). It is well acknowledged that peri-implantitis is a multifactorial, dysbiosis-related pathological process (Kroger et al., 2018). Yu XL et al. found that there was a wide positive correlation between the bacteria of the synthetic bacteria phylum, including Fusarium Acremonium, HMT 361/362, and Thiovibrio desthiolatus TG5 sp. in periodontitis and the peri-implantitis group. However, there was also a negative correlation in some genera, such as Rosella, Microporus, Actinomyces, Corynebacterium, Streptococcus, Neisseria, Kingella, Leptotrichum, Fusobacterium, etc (Yu et al., 2019). The three core bacteria in the peri-implantitis group in this study were Lachnoanaerobaculum, Fusobacterium, and Atopobium. Among them, Lachnoanaerobaculum has the highest connectivity in network co-occurrence. Lachnoanaerobaculum is obligate anaerobic, gram-positive (Ida et al., 2022), and it is reported as significantly increased in smokers’ subgingival plaque around an implant (Duan et al., 2017). Fusobacterium, another core bacteria, was reported to play an important role in biofilm formation (Jiang et al., 2021) and could be distinctly detected in high-risk individuals (Wang et al., 2021). Atopobium was reported as over-represented in peri-implantitis sites compared with that in healthy sites (Barbagallo et al., 2022; Rubino et al., 2021). Large degrees of species including Erysipelotrichaceae_UCG-006, Peptostreptococcus_stomatis, and Alistipes were found in the peri-implant mucositis group. Erysipelotrichaceae_UCG-006 was not yet reported in the dental field. Peptostreptococcus stomatis was significantly increased in the tumor sites of oral squamous cell carcinoma patients than in normal tissues (Luo et al., 2023). Alistipes was predicted to play a causal role in enhancing the risk of periodontitis (Zhang et al., 2019). Whether these organisms play an important role in the development of peri-implant mucositis needs to be further explored in the future.
Peri-implant mucositis is considered to be the precursor to peri-implantitis, a condition which may progress rapidly, leading to advanced bone loss and resulting in loss of an implant (Heitz-Mayfield, 2024). In this study, peri-implantitis sites harbor more anaerobic and hemophilic bacteria (Fusobacteriota, Porphyromonas, Treponema, and Spirochaetota) than sites with peri-implant mucositis. The microbial communities of peri-implantitis sites showed more imbalance compared with peri-implant mucositis sites with lower biomass. The abundance of Coriobacteriia and Coriobacteriales, respectively, was higher in the peri-implant mucositis group than in the other two groups (Figure 6). Coriobacteriales had a protective effect on allergic rhinitis and microscopic colitis (Jin et al., 2023; Sandler et al., 2023). Whether Coriobacteriales could impede the development of peri-implantitis from peri-implant mucositis sites needs further studies.
It should be noted that this study has limitations. Studies have shown that individual differences have a significant impact on the composition of the microbiota composition, which is caused by differences in the host immune response (Yu et al., 2019; Alves et al., 2022; Kensara et al., 2021). The literature data we collected were all from Asian populations, mainly Chinese and Japanese peri-implant samples. These individual selection biases may lead to a certain bias in the research results. To eliminate this bias, data collected from research based on other races is advocated.
To sum up, this study was conducted based on 16S rRNA sequencing literature data on submucosal microflora in healthy implants, peri-implant mucositis, and peri-implantitis. The composition and differences are re-analyzed comprehensively. The results showed that there was a significant difference in flora diversity between peri-implant disease and healthy implants. Some members of the red complex (Fusobacterium and Prophyromonas) and yellow complex (Streptococcus) are closely related to peri-implantitis. The abundance of Fretibacterium (an anaerobic Bacilli) was higher in the peri-implantitis group than in the other two groups. The co-occurrence network in the peri-implantitis group was different from that in the healthy implant group and the peri-implant mucositis group. The peri-implantitis site presented a relative disequilibrium microbial community, and members of the red complex (Porphyromonas and Bacteroides forsythus) played an important role in the co-occurrence network.
Data availability statement
Publicly available datasets were analyzed in this study. This data can be found here: NCBI Short Reads Achieve under the accession of biosamples: SAMN09464023 - SAMN09464094; NCBI Short Reads Achieve: PRJNA861252; Short Reads Archive (Accession number SRP043555); the DNA Data Bank of Japan (DDBJ) under accession no. DRA000946 (http://www.ddbj.nig.ac.jp/); the DNA Data Bank of Japan (DDBJ) with the following accession numbers: 16S rDNA sequencing (DRA010104) and metagenomic analysis (DRA006832); NCBI PRJNA786326; Sequence Read Archive with the accession number PRJNA487121.
Ethics statement
Ethical approval was not required for the study involving humans in accordance with the local legislation and institutional requirements. Written informed consent to participate in this study was not required from the participants or the participants’ legal guardians/next of kin in accordance with the national legislation and the institutional requirements.
Author contributions
PJ: Writing – original draft, Writing – review & editing. XG: Data curation, Writing – original draft. JCY: Methodology, Writing – original draft. HL: Writing – review & editing. JWY: Writing – original draft, Writing – review & editing. JH: Writing – review & editing.
Funding
The author(s) declare financial support was received for the research, authorship, and/or publication of this article. Supported by the National Natural Science Foundation of China (No. 82201022; 82301072), PR China; Key Research and Development Project of Hainan (No. ZDYF2021SHFZ229), PR China; the Hainan Provincial Natural Science Foundation of China (No. 822CXTD534), PR China; the Key Health Projects of Science and Technology Development of Lanzhou (No. 2021002), PR China; the Teaching Reform Funding Project of Peking University School and Hospital of Stomatology (2022-PT-06), PR China; and by the Association Between Edentulous Jaws and Health Outcomes In A Chinese Elderly Population Funding Project of Peking University School and Hospital of Stomatology (3030546840026), PR China.
Conflict of interest
The authors declare that the research was conducted in the absence of any commercial or financial relationships that could be construed as a potential conflict of interest.
Publisher’s note
All claims expressed in this article are solely those of the authors and do not necessarily represent those of their affiliated organizations, or those of the publisher, the editors and the reviewers. Any product that may be evaluated in this article, or claim that may be made by its manufacturer, is not guaranteed or endorsed by the publisher.
Supplementary material
The Supplementary Material for this article can be found online at: https://www.frontiersin.org/articles/10.3389/fcimb.2024.1445751/full#supplementary-material
Supplementary Table 1 | Genera in co-abundance group used for network construction of peri-implantitis group.
Supplementary Table 2 | Genera in co-abundance group used for network construction of healthy group.
Supplementary Table 3 | Genera in co-abundance group used for network construction of peri-implant mucositis group.
References
Al-Ahmad, A., Muzafferiy, F., Anderson, A. C., Wolber, J. P., Ratka-Kruger, P., Fretwurst, T., et al. (2018). Shift of microbial composition of peri-implantitis-associated oral biofilm as revealed by 16S rRNA gene cloning. J. Med. Microbiol. 67, 332–340. doi: 10.1099/jmm.0.000682
Alves, C. H., Russi, K. L., Rocha, N. C., Bastos, F., Darrieux, M., Parisotto, T. M., et al. (2022). Host-microbiome interactions regarding peri-implantitis and dental implant loss. J. Transl. Med. 20, 425. doi: 10.1186/s12967-022-03636-9
Barbagallo, G., Santagati, M., Guni, A., Torrisi, P., Spitale, A., Stefani, S., et al. (2022). Microbiome differences in periodontal, peri-implant, and healthy sites: a cross-sectional pilot study. Clin. Oral. Investig. 26, 2771–2781. doi: 10.1007/s00784-021-04253-4
Belibasakis, G. N., Manoil, D. (2021). Microbial community-driven etiopathogenesis of peri-implantitis. J. Dent. Res. 100, 21–28. doi: 10.1177/0022034520949851
Berglundh, T., Armitage, G., Araujo, M. G., Avila-Ortiz, G., Blanco, J., Camargo, P. M., et al. (2018). Peri-implant diseases and conditions: Consensus report of workgroup 4 of the 2017 World Workshop on the Classification of Periodontal and Peri-Implant Diseases and Conditions. J. Periodontol 89 Suppl 1, S313–S318. doi: 10.1002/JPER.17-0739
Berkman, N. D., Dewalt, D. A., Pignone, M. P., Sheridan, S. L., Lohr, K. N., Lux, L., et al. (2004). Literacy and health outcomes. Evid Rep. Technol. Assess. (Summ) 87), 1–8. Available at: https://www.ncbi.nlm.nih.gov/pubmed/15819598.
Caton, J. G., Armitage, G., Berglundh, T., Chapple, I. L. C., Jepsen, S., Kornman, K. S., et al. (2018). A new classification scheme for periodontal and peri-implant diseases and conditions - Introduction and key changes from the 1999 classification. J. Clin. Periodontol 45 Suppl 20, S1–S8. doi: 10.1111/jcpe.12935
Chen, T., Yu, W. H., Izard, J., Baranova, O. V., Lakshmanan, A., Dewhirst, F. E. (2010). The Human Oral Microbiome Database: a web accessible resource for investigating oral microbe taxonomic and genomic information. Database (Oxford) 2010, baq013. doi: 10.1093/database/baq013
Darby, I. (2022). Risk factors for periodontitis & peri-implantitis. Periodontol 2000 90, 9–12. doi: 10.1111/prd.12447
da Silva, E. S., Feres, M., Figueiredo, L. C., Shibli, J. A., Ramiro, F. S., Faveri, M. (2014). Microbiological diversity of peri-implantitis biofilm by Sanger sequencing. Clin. Oral. Implants Res. 25, 1192–1199. doi: 10.1111/clr.12231
Diaz, P., Gonzalo, E., Villagra, L. J. G., Miegimolle, B., Suarez, M. J. (2022). What is the prevalence of peri-implantitis? A systematic review and meta-analysis. BMC Oral. Health 22, 449. doi: 10.1186/s12903-022-02493-8
Doornewaard, R., Christiaens, V., De Bruyn, H., Jacobsson, M., Cosyn, J., Vervaeke, S., et al. (2017). Long-term effect of surface roughness and patients’ Factors on crestal bone loss at dental implants. A systematic review and meta-analysis. Clin. Implant Dent. Relat. Res. 19, 372–399. doi: 10.1111/cid.12457
Duan, X., Wu, T., Xu, X., Chen, D., Mo, A., Lei, Y., et al. (2017). Smoking may lead to marginal bone loss around non-submerged implants during bone healing by altering salivary microbiome: A prospective study. J. Periodontol 88, 1297–1308. doi: 10.1902/jop.2017.160808
Escapa, I. F., Chen, T., Huang, Y., Gajare, P., Dewhirst, F. E., Lemon, K. P. (2018). New insights into human nostril microbiome from the expanded human oral microbiome database (eHOMD): a resource for the microbiome of the human aerodigestive tract. mSystems 3 (6), e00187-18. doi: 10.1128/mSystems.00187-18
Hannig, M. (1997). Transmission electron microscopic study of in vivo pellicle formation on dental restorative materials. Eur. J. Oral. Sci. 105, 422–433. doi: 10.1111/j.1600-0722.1997.tb02139.x
Hashimoto, Y., Okada, S., Yasuda, K., Kawagoe, M., Kajiya, M., Tsuga, K. (2022). Microbial differences between active and remission peri-implantitis. Sci. Rep. 12, 5284. doi: 10.1038/s41598-022-09192-y
Heitz-Mayfield, L. J. A. (2024). Peri-implant mucositis and peri-implantitis: key features and differences. Br. Dent. J. 236, 791–794. doi: 10.1038/s41415-024-7402-z
Heitz-Mayfield, L. J., Aaboe, M., Araujo, M., Carrion, J. B., Cavalcanti, R., Cionca, N., et al. (2018). Group 4 ITI Consensus Report: Risks and biologic complications associated with implant dentistry. Clin. Oral. Implants Res. 29 Suppl 16, 351–358. doi: 10.1111/clr.13307
Ida, Y., Okuyama, T., Araki, K., Sekiguchi, K., Watanabe, T., Ohnishi, H. (2022). First description of Lachnoanaerobaculum orale as a possible cause of human bacteremia. Anaerobe 73, 102506. doi: 10.1016/j.anaerobe.2021.102506
Jiang, Q., Yu, Y., Xu, R., Zhang, Z., Liang, C., Sun, H., et al. (2021). The temporal shift of peri-implant microbiota during the biofilm formation and maturation in a canine model. Microb. Pathog. 158, 105100. doi: 10.1016/j.micpath.2021.105100
Jin, Q., Ren, F., Dai, D., Sun, N., Qian, Y., Song, P. (2023). The causality between intestinal flora and allergic diseases: Insights from a bi-directional two-sample Mendelian randomization analysis. Front. Immunol. 14. doi: 10.3389/fimmu.2023.1121273
Johnson, J. S., Spakowicz, D. J., Hong, B. Y., Petersen, L. M., Demkowicz, P., Chen, L., et al. (2019). Evaluation of 16S rRNA gene sequencing for species and strain-level microbiome analysis. Nat. Commun. 10, 5029. doi: 10.1038/s41467-019-13036-1
Kensara, A., Hefni, E., Williams, M. A., Saito, H., Mongodin, E., Masri, R. (2021). Microbiological profile and human immune response associated with peri-implantitis: A systematic review. J. Prosthodont 30, 210–234. doi: 10.1111/jopr.13270
Khemwong, T., Kobayashi, H., Ikeda, Y., Matsuura, T., Sudo, T., Kano, C., et al. (2019). Fretibacterium sp. human oral taxon 360 is a novel biomarker for periodontitis screening in the Japanese population. PloS One 14, e0218266. doi: 10.1371/journal.pone.0218266
Komatsu, K., Shiba, T., Takeuchi, Y., Watanabe, T., Koyanagi, T., Nemoto, T., et al. (2020). Discriminating microbial community structure between peri-implantitis and periodontitis with integrated metagenomic, metatranscriptomic, and network analysis. Front. Cell Infect. Microbiol. 10. doi: 10.3389/fcimb.2020.596490
Kotsakis, G. A., Olmedo, D. G. (2021). Peri-implantitis is not periodontitis: Scientific discoveries shed light on microbiome-biomaterial interactions that may determine disease phenotype. Periodontol 2000 86, 231–240. doi: 10.1111/prd.12372
Koyanagi, T., Sakamoto, M., Takeuchi, Y., Ohkuma, M., Izumi, Y. (2010). Analysis of microbiota associated with peri-implantitis using 16S rRNA gene clone library. J. Oral. Microbiol. 24, 2. doi: 10.3402/jom.v2i0.5104
Kroger, A., Hulsmann, C., Fickl, S., Spinell, T., Huttig, F., Kaufmann, F., et al. (2018). The severity of human peri-implantitis lesions correlates with the level of submucosal microbial dysbiosis. J. Clin. Periodontol 45, 1498–1509. doi: 10.1111/jcpe.13023
Kumar, P. S., Mason, M. R., Brooker, M. R., O’Brien, K. (2012). Pyrosequencing reveals unique microbial signatures associated with healthy and failing dental implants. J. Clin. Periodontol 39, 425–433. doi: 10.1111/j.1600-051X.2012.01856.x
Li, Z. J., Wang, S. G., Li, Y. H., Tu, D. X., Liu, S. Y., Nie, H. B., et al. (2015). Study on microbial diversity of peri-implantitis subgingival by high-throughput sequencing. Sichuan Da Xue Bao Yi Xue Ban 46, 568–572. Available at: https://www.ncbi.nlm.nih.gov/pubmed/26480660.
Luo, S., Li, W., Li, Q., Zhang, M., Wang, X., Wu, S., et al. (2023). Causal effects of gut microbiota on the risk of periodontitis: a two-sample Mendelian randomization study. Front. Cell Infect. Microbiol. 13. doi: 10.3389/fcimb.2023.1160993
Maruyama, N., Maruyama, F., Takeuchi, Y., Aikawa, C., Izumi, Y., Nakagawa, I. (2014). Intraindividual variation in core microbiota in peri-implantitis and periodontitis. Sci. Rep. 4, 6602. doi: 10.1038/srep06602
Mombelli, A., Mericske-Stern, R. (1990). Microbiological features of stable osseointegrated implants used as abutments for overdentures. Clin. Oral. Implants Res. 1, 1–7. doi: 10.1034/j.1600-0501.1990.010101.x
Nie, J., Zhang, Q., Zheng, H., Xu, L. X., Wang, X. Y., Chen, F. (2020). Pyrosequencing of the subgingival microbiome in peri-implantitis after non-surgical mechanical debridement therapy. J. Periodontal Res. 55, 238–246. doi: 10.1111/jre.12708
Nishio, S., Moriya, S., Kunihisa, M., Takeuchi, Y., Imai, A., Takada, N. (2023). Rapid and easy construction of a simplified amplicon sequencing (simplified AmpSeq) library for marker-assisted selection. Sci. Rep. 13, 10575. doi: 10.1038/s41598-023-37522-1
Oliveira, R. R., Fermiano, D., Feres, M., Figueiredo, L. C., Teles, F. R., Soares, G. M., et al. (2016). Levels of candidate periodontal pathogens in subgingival biofilm. J. Dent. Res. 95, 711–718. doi: 10.1177/0022034516634619
Padial-Molina, M., Montalvo-Acosta, S., Martin-Morales, N., Perez-Carrasco, V., Magan-Fernandez, A., Mesa, F., et al. (2024). Correlation between inflammasomes and microbiota in peri-implantitis. Int. J. Mol. Sci. 25 (2), 961. doi: 10.3390/ijms25020961
Patel, J. B. (2001). 16S rRNA gene sequencing for bacterial pathogen identification in the clinical laboratory. Mol. Diagn. 6, 313–321. doi: 10.1054/modi.2001.29158
Rognes, T., Flouri, T., Nichols, B., Quince, C., Mahe, F. (2016). VSEARCH: a versatile open source tool for metagenomics. PeerJ 4, e2584. doi: 10.7717/peerj.2584
Rubino, C. V., Katz, B. G., Langlois, K., Wang, H. H., Carrion, J. A., Walker, S. G., et al. (2021). Evaluation of different materials used for sealing of implant abutment access channel and the peri-implant sulcus microbiota: A 6-month, randomized controlled trial. Clin. Oral. Implants Res. 32, 941–950. doi: 10.1111/clr.13787
Sandler, R. S., Sun, S., Keku, T. O., Woosley, J. T., Anderson, C., Peery, A. F., et al. (2023). Dietary calcium and risk of microscopic colitis. Clin. Transl. Gastroenterol. 14, e00569. doi: 10.14309/ctg.0000000000000569
Sanz-Martin, I., Doolittle-Hall, J., Teles, R. P., Patel, M., Belibasakis, G. N., Hammerle, C. H. F., et al. (2017). Exploring the microbiome of healthy and diseased peri-implant sites using Illumina sequencing. J. Clin. Periodontol 44, 1274–1284. doi: 10.1111/jcpe.12788
Shibli, J. A., Melo, L., Ferrari, D. S., Figueiredo, L. C., Faveri, M., Feres, M. (2008). Composition of supra- and subgingival biofilm of subjects with healthy and diseased implants. Clin. Oral. Implants Res. 19, 975–982. doi: 10.1111/j.1600-0501.2008.01566.x
Socransky, S. S., Haffajee, A. D., Cugini, M. A., Smith, C., Kent, R. L., Jr. (1998). Microbial complexes in subgingival plaque. J. Clin. Periodontol 25, 134–144. doi: 10.1111/j.1600-051x.1998.tb02419.x
Sun, F., Liu, J., Li, S. Q., Wei, Y. P., Hu, W. J., Wang, C. (2023). Profiles and differences of submucosal microbial in peri-implantitis and health implants: A cross-sectional study. Beijing Da Xue Bao Yi Xue Ban 55, 30–37. doi: 10.19723/j.issn.1671-167X.2023.01.005
Wang, C. W., Hao, Y., Di Gianfilippo, R., Sugai, J., Li, J., Gong, W., et al. (2021). Machine learning-assisted immune profiling stratifies peri-implantitis patients with unique microbial colonization and clinical outcomes. Theranostics 11, 6703–6716. doi: 10.7150/thno.57775
Wells, G. A., Shea, B., O’Connell, D., Peterson, J., Welch, V., Losos, M., et al. (2009). The Newcastle-Ottawa Scale (NOS) for assessing the quality if nonrandomized studies in meta-analyses. Available online at: http://www.ohri.ca/programs/clinical_epidemiology/oxford.htm. (Accessed May 3, 2021)
Xian, P., Xuedong, Z., Xin, X., Yuqing, L., Yan, L., Jiyao, L., et al. (2018). The oral microbiome bank of China. Int. J. Oral. Sci. 10, 16. doi: 10.1038/s41368-018-0018-x
Yarza, P., Yilmaz, P., Pruesse, E., Glockner, F. O., Ludwig, W., Schleifer, K. H., et al. (2014). Uniting the classification of cultured and uncultured bacteria and archaea using 16S rRNA gene sequences. Nat. Rev. Microbiol. 12, 635–645. doi: 10.1038/nrmicro3330
Yu, X. L., Chan, Y., Zhuang, L., Lai, H. C., Lang, N. P., Keung Leung, W., et al. (2019). Intra-oral single-site comparisons of periodontal and peri-implant microbiota in health and disease. Clin. Oral. Implants Res. 30, 760–776. doi: 10.1111/clr.13459
Yuan, J., Wen, T., Zhang, H., Zhao, M., Penton, C. R., Thomashow, L. S., et al. (2020). Predicting disease occurrence with high accuracy based on soil macroecological patterns of Fusarium wilt. ISME J. 14, 2936–2950. doi: 10.1038/s41396-020-0720-5
Zhang, L., Liu, Y., Zheng, H. J., Zhang, C. P. (2019). The oral microbiota may have influence on oral cancer. Front. Cell Infect. Microbiol. 9. doi: 10.3389/fcimb.2019.00476
Keywords: oral microbiome, dental implants, peri-implantitis, 16S rRNA, data analysis
Citation: Jia P, Guo X, Ye J, Lu H, Yang J and Hou J (2024) Microbiome of diseased and healthy implants—a comprehensive microbial data analysis. Front. Cell. Infect. Microbiol. 14:1445751. doi: 10.3389/fcimb.2024.1445751
Received: 08 June 2024; Accepted: 02 August 2024;
Published: 29 August 2024.
Edited by:
Ke Deng, The University of Hong Kong, Hong Kong SAR, ChinaReviewed by:
Junyu Shi, Shanghai Jiao Tong University, ChinaQi Yan, Wuhan University, China
Hanqi Gao, Shanghai Jiao Tong University, China
Copyright © 2024 Jia, Guo, Ye, Lu, Yang and Hou. This is an open-access article distributed under the terms of the Creative Commons Attribution License (CC BY). The use, distribution or reproduction in other forums is permitted, provided the original author(s) and the copyright owner(s) are credited and that the original publication in this journal is cited, in accordance with accepted academic practice. No use, distribution or reproduction is permitted which does not comply with these terms.
*Correspondence: Jingwen Yang, amluZ3dlbi55YW5nQGZveG1haWwuY29t