- 1Department of Pharmacy, The Third Affiliated Hospital of Soochow University/The First People’s Hospital of Changzhou, Changzhou, China
- 2Changzhou Key Laboratory of Human Use Experience Research & Transformation of Menghe Medical School, Changzhou Hospital Affiliated to Nanjing University of Chinese Medicine, Changzhou, China
- 3Department of Tumor Biological Treatment, The Third Affiliated Hospital of Soochow University, Changzhou, China
- 4Pediatric Central Laboratory, Affiliated Changzhou Children’s Hospital of Nantong University, Changzhou, China
Aims: Hyperglycemia is one of the adverse effects of tacrolimus (TAC), but the underlying mechanism is not fully identified. We used multi-omics analysis to evaluate the changes in the gut microbiota and metabolic profile of rats with TAC-induced diabetes.
Methods: To establish a diabetic animal model, Sprague Dawley rats were divided randomly into two groups. Those in the TAC group received intraperitoneal injections of TAC (3 mg/kg) for 8 weeks, and those in the CON group served as the control. 16S rRNA sequencing was used to analyze fecal microbiota. The metabolites of the two groups were detected and analyzed by nontargeted and targeted metabolomics, including amino acids (AAs), bile acids (BAs), and short-chain fatty acids (SCFAs).
Results: The rats treated with TAC exhibited hyperglycemia as well as changes in the gut microbiota and metabolites. Specifically, their gut microbiota had significantly higher abundances of Escherichia-Shigella, Enterococcus, and Allobaculum, and significantly lower abundances of Ruminococcus, Akkermansia, and Roseburia. In addition, they had significantly reduced serum levels of AAs including asparagine, aspartic acid, glutamic acid, and methionine. With respect to BAs, they had significantly higher serum levels of taurocholic acid (TCA), and glycochenodeoxycholic acid (GCDCA), but significantly lower levels of taurodeoxycholic acid (TDCA) and tauroursodeoxycholic acid (TUDCA). There were no differences in the levels of SCFAs between the two groups. Correlations existed among glucose metabolism indexes (fasting blood glucose and fasting insulin), gut microbiota (Ruminococcus and Akkermansia), and metabolites (glutamic acid, hydroxyproline, GCDCA, TDCA, and TUDCA).
Conclusions: Both AAs and BAs may play crucial roles as signaling molecules in the regulation of TAC-induced diabetes.
1 Introduction
Tacrolimus (TAC) is a macrolide antibiotic produced by the soil fungus Streptomyces tsukubaensis. It is the most widely used calcineurin inhibitor for organ transplant patients and is frequently used to prevent organ rejection after transplantation. It is characterized by a narrow therapeutic index and high inter- and intra-patient pharmacokinetic variability, and the use of excessive TAC is associated with nephrotoxicity, diabetes, opportunistic infections, increased cardiovascular risk, and malignancies. However, insufficient TAC may result in the rejection and eventually the loss of the graft (Brunet et al., 2019; Degraeve et al., 2020). Research has shown that the prolonged use of TAC can lead to hyperglycemia, which is a significant risk factor for post-transplant diabetes mellitus (PTDM) (Jouve et al., 2018). As early as 1987, Lewis rats were found by Nalesnik et al. to exhibit signs of elevated blood glucose following long-term TAC administration (Nalesnik et al., 1987). While thoroughly understanding the pathogenic mechanism underlying TAC-induced diabetes is crucial for managing PTDM, existing research is largely limited to the toxic effect of TAC on islet β cells. Indeed, TAC can induce diabetes by affecting the signaling pathways related to the maintenance and function of β cells (Rodriguez-Rodriguez et al., 2021; Tong et al., 2021), such as calcineurin (CaN)/nuclear factor of activated T cells (NFAT) (Goodyer et al., 2012), phosphatidylinositol 3-kinase (PI3K)/protein kinase B (AKT)/mammalian target of rapamycin (mTOR) (Rodriguez-Rodriguez et al., 2019), transforming growth factor-β (TGF-β)/mothers against decapentaplegic homolog (Smad) (Chen et al., 1997), and tyrosine-protein kinase (Syk)/B-cell linker protein (BLNK)/nuclear factor-κB (NF-κB) (Chen et al., 2024).
Gut microbiota is a very complex microbial ecosystem that exists in gastrointestinal tract and is considered a special metabolic “organ” of the body (Huda et al., 2021). Thanks to the advance of 16S rRNA and metagenome sequencing technology, the influence of the gut microbiota on type 2 diabetes mellitus (T2DM), including its participation in glucose regulation and insulin sensitivity, has been recognized (Fu et al., 2018; Gurung et al., 2020). Some studies also reported that TAC can alter the composition and bacterial taxa of the gut microbiota (Zhang et al., 2018). Meanwhile, TAC-induced glucose metabolism disorders can be aggravated by altering the gut microbial composition and function (Han et al., 2019). Presumably, the gut microbiota participates in the process of TAC-induced diabetes.
The regulation of glucose homeostasis by the gut microbiota depends on multiple metabolites and their interactions with host cell receptors, including short-chain fatty acids (SCFAs), amino acids (AAs), bile acids (BAs), and so on (Zhai et al., 2021; Zheng et al., 2021). Studies have demonstrated that SCFAs can promote the secretion of glucagon-like peptide-1 (GLP-1) by activating free fatty acid receptor 2 (FFAR2), which indirectly modulates blood glucose levels by enhancing insulin secretion and reducing glucagon secretion (He et al., 2020). In terms of branched-chain AAs (BCAAs), both in vivo and in vitro studies have indicated that decreased BCAA levels can improve insulin sensitivity by inhibiting the mTOR/S6K1 signaling pathway, thereby relieving the inhibition of the insulin signaling pathway (Zhu et al., 2016). In addition, BAs can regulate glucose homeostasis by directly interacting with the farnesoid X receptor (FXR) and the G protein-coupled bile acid receptor 1 (TGR5) in the liver, intestine, and pancreas. They also indirectly stimulate FXR-dependent intestinal fibroblast growth factor 15 (FGF15/19) production (Sah et al., 2022). Metabolomics reveals the link between genotype and phenotype in biological systems. By quantifying the changes in the absolute and/or relative amounts of thousands of small molecule metabolites in blood and tissues, it provides valuable insights into disease diagnosis, pathogenesis, and drug intervention (Ribbenstedt et al., 2018). Several metabolomic methods have been developed to evaluate biomarkers of diabetes (Schrimpe-Rutledge et al., 2016; Hu et al., 2021). However, there are limited studies on the changes in the gut microbiota and the related metabolites in TAC-induced diabetic models (Canfora et al., 2019; Li et al., 2024).
In this study, to screen markers related to TAC-induced diabetes, we used 16S rRNA gene sequencing to analyze the changes in the gut microbiota of TAC-induced diabetic rats and nontargeted/targeted metabolomics to detect the serum metabolic profiles. In addition, we further analyzed the correlation between the gut microbiota, metabolites, and the glucose metabolism indexes and discussed the mechanism of TAC-induced diabetes from a new angle.
2 Materials and methods
2.1 Materials
TAC was purchased from Popeye Biotechnology (Shenzhen) Co., Ltd. (Shenzhen, China). Methanol, acetonitrile, formic acid, and ammonia were supplied by Merck (Dannstadt, Germany). Methoxyamine HCl, fatty acid methyl esters, and pyridine were provided by Sigma-Aldrich (St. Louis, MO, USA). N-Methyl-N-(trimethylsilyl)trifluoroacetamide (MSTFA), hexane, methylene chloride, and chloroform were purchased from Thermo-Fisher Scientific (Fair Lawn, NJ, USA). Bile acid (BA) standards, including cholic acid (CA), glycocholic acid (GCA), deoxycholic acid (DCA), chenodeoxycholic acid (CDCA), ursodeoxycholic acid (UDCA), glycochenodeoxycholic acid (GCDCA), taurocholic acid (TCA), tauroursodeoxycholic acid (TUDCA), glycoursodeoxycholic acid (GUDCA), taurochenodeoxycholic acid (TCDCA), taurodeoxycholic acid (TDCA), lithocholic acid (LCA), and taurolithocholic acid (TLCA), and isotope internal standards were supplied by Sigma-Aldrich (St. Louis, MO, USA). Deionized water was generated in the lab using a Milli-Q system (EMD Millipore, Danvers, MA, USA).
2.2 Animal experiment and sample collection
Eight-week-old male Sprague Dawley (SD) rats (180-220 g) were supplied by Cavens Experimental Animal Co., Ltd. (Changzhou, China). The rats were kept in a standard specific pathogen free (SPF) environment with a 12 h/12 h light/dark cycle and acclimatized for 7 days before the experiment. The rats were randomly divided into the control group (CON) and the tacrolimus group (TAC), with six rats in each group, and received intraperitoneal injections of either 3 mg/kg/d TAC (TAC group) or an equal volume of normal saline (CON group) for 8 weeks. After 8 weeks, the feces, the serum, and the pancreatic tissue were collected. The experiment protocol complied with the institutional guidelines on the care and use of laboratory animals was certified by the Ethics Committee of the Third Affiliated Hospital of Soochow University (Approval No. 2021151).
2.3 Fasting blood glucose and oral glucose tolerance test
Blood samples were collected from the caudal vein after the rats were fasted for 12 h with free access to water. The FBG was measured using an Accu-Chek Active blood glucose meter. For the OGTT, the rats were given 40% glucose (2 g/kg body weight) via gavage, and the blood samples collected at 0, 30, 90, 120, and 180 min after gavage were analyzed.
2.4 Biochemical indicators and serum insulin
An AU5800 automatic biochemistry analyzer (Beckman Coulter, Brea, CA, USA) was used to analyze the serum biochemical parameters. The serum insulin levels were determined using ELISA kits (YX-091419R, Yuanxin Biotechnology, Shanghai, China). The homeostasis model assessment was used to calculate the following indexes from the FBG (mmol/L) and the fasting insulin (FINS, mU/L):
where HOMA-IR is the insulin resistance index, HOMA-β is the insulin secretion index, and HOMA-ISI is the insulin sensitivity.
2.5 Histological assessment
The pancreatic damage was assessed using hematoxylin-eosin (H&E) staining according to a published protocol (Hu et al., 2021). The stained slides were observed and photographed under an Olympus CX31 microscope (Olympus, Hamburg, Germany).
2.6 Gut microbiota analysis
The microbial DNA in the collected feces was extracted using the E.Z.N.A.® Stool DNA Kit (Omega Biotek, Norcross, GA, USA) according to the manufacturer’s instructions. The V3-V4 region of the 16S rRNA gene of the bacteria was amplified on a GeneAmp 9700 thermocycler (Applied Biosystems, Waltham, MA, USA) using the primers 338F (5’-ACTCCTACGGGAGGCAGCAG-3’) and 806R (5’-GGACTACHVGGGTWTCTAAT-3’). The structure of the gut microbiota was constructed after amplification and sequencing on the Illumina MiSeq platform, and the abundance of the OTUs was determined using USEARCH 7.17.1 (http://drive5.com/uparse/).
2.7 Non-targeted and targeted metabolomics
2.7.1 Non-targeted metabolomics analysis
Serum samples were thawed in an ice bath and centrifuged at 4°C and 3,000 g for 5 min. A 50 μL aliquot of the serum was added to precooled methanol/chloroform (3/1 v/v, 175 μL) and L-2-chlorophenylalanine (10 μL, internal standard). The mixture was centrifuged at 4°C and 14,000 g for 20 min. A 200 μL aliquot of the supernatant was freeze-dried, and the supernatant of all remaining samples was mixed in equal volume to give a quality control (QC) sample. To the residue was added an aqueous solution of methoxylamine hydrochloride pyridine (20 mg/mL, 50 μL), and the mixture was maintained at 30°C for 2 h before it was derivatized with FAMEs (50 μL) containing MSTFA (1% TMCS) at 37.5°C for 60 min and analyzed by GC-MS (Wu et al., 2021).
The obtained raw GC-MS data files were processed using Chroma TOF (v4.71, Leco Corp., St. Joseph, MO, USA) for baseline denoising, smoothing, peak extraction, deconvolution, and peak alignment. Compounds were identified by comparing the MS similarity and the FAMEs retention index distance using the JiaLib database. The data were imported into iMAP (Metabo-Profile, Shanghai, China) for multivariate statistical analysis, including principal component analysis (PCA) and orthogonal partial least squares discriminant analysis (OPLS-DA). The metabolites were defined as differential metabolites if their variable importance in the projection (VIP) value in the OPLS-DA model was greater than 1 and they showed statistically significant difference between the TAC and CON groups. The reliability and prediction ability of the model were assessed with R2 (the goodness of fit parameter) and Q2 (the goodness of prediction parameter). The enrichment analysis and topological analysis of the differential metabolites were performed using the MetaboAnalyst software (https://www.metaboanalyst.ca/).
2.7.2 Targeted metabolomics analysis
Serum BAs were determined according to the validated high-performance liquid chromatography tandem mass spectrometry (HPLC-MS/MS) method reported in the literature (Hu et al., 2021). Fecal BAs were determined as follows. The feces sample was sonicated in methanol (9 mL per gram fecal sample) for 30 min, and the mixture was then centrifuged at 16,400 rpm for 20 min. To an aliquot of the supernatant (50 μL) was added the internal standard (20 μL) and acetonitrile (200 μL). The internal standard contained GCA-d5, CDCA-d4, GCDCA-d7, DCA-d5, and LCA-d4, all at 100 ng/mL. The mixture was vortexed for 30 s and then centrifuged at 16,400 rpm for 10 min. The supernatant was diluted with an equal volume of ultrapure water, transferred to a glass vial, and analyzed by HPLC-MS/MS.
To measure the serum AAs, the serum (50 μL) was vortexed in 50% acetonitrile (200 μL), and the mixture was centrifuged at 4°C at 16,400 rpm for 10 min. The supernatant was centrifuged again at 4°C at 16,400 rpm for 5 min, and the serum AAs were then determined by HPLC-MS/MS.
For the analysis of serum SCFAs, the serum (50 μL) was added into acetonitrile (100 μL) using a pipette, and the mixture were ultrasonicated at 5°C and 40 kHz for 30 min before centrifugation at 4°C and 13,000 rpm for 15 min. To the supernatant was added the aqueous solutions of 3-NPH·HCl (200 mM, 20 μL) and EDC·HCl (120 mM and containing 6% pyridine, 20 μL). The mixture was then reacted at 40°C for 30 min, diluted with 50% acetonitrile to 750 μL, and analyzed by UPLC-MS/MS.
The detailed chromatographic and mass spectrometric conditions for non-targeted and targeted metabolomic analysis are given in Supplementary Tables S1–S6.
2.8 Statistical analysis
Data were expressed as mean ± standard deviation, and SPSS 25.0 was used for statistical analysis. Statistically significance (P< 0.05) in the difference between two groups was verified by the Student’s t test for normally distributed continuous variables and by the Mann-Whitney U test for non-normally distributed variables. The Spearman correlation analysis was used to analyze the correlation among gut microbiota, BAs, AAs, and physiological data.
3 Results
3.1 Establishment of TAC-induced diabetic rat model
After 8 weeks, the rats in the TAC group had much lower body weight than the rats in the CON group, and their FBG and area under the curve (AUC) of OGTT were significantly higher (Figures 1A–D). The TAC group had significantly lower FIN and HOMA-β than the CON group (Figures 1E, F), but there was no difference in HOMA-IR and HOMA-ISI between the two groups (Supplementary Figure S1). In the histological assessments, compared to the rats in the CON group, the rats in the TAC group had fewer the islet cells, many vacuoles appeared in the cytoplasm of their islet cells, and the nuclear staining was uneven (Figure 1G). Apparently, the TAC treatment destroyed islet cells and reduced insulin secretion.
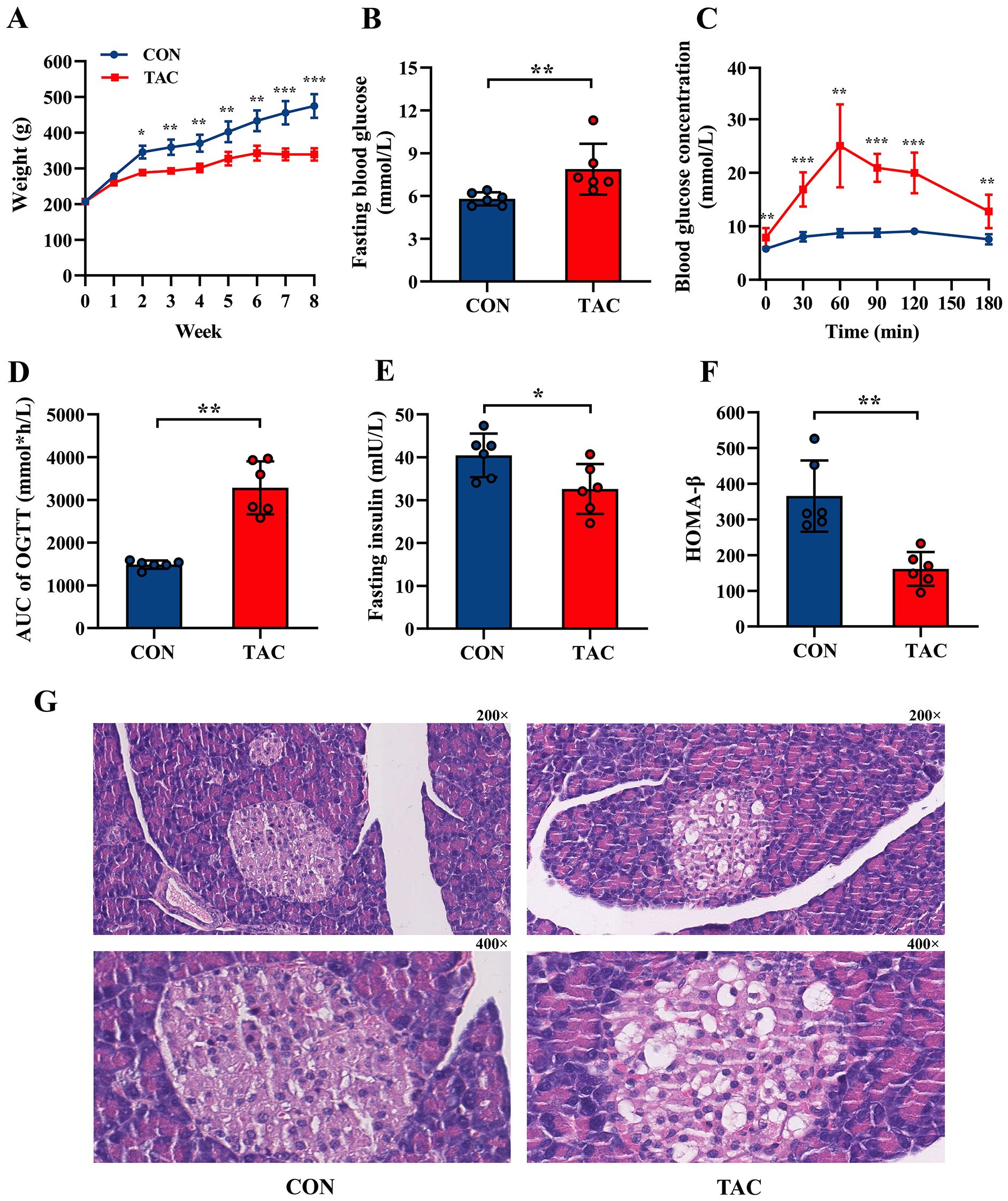
Figure 1. Effects of TAC on SD rats. (A) Body weight; (B) Fasting blood glucose; (C) Oral glucose tolerance test (OGTT); (D) Area under curve (AUC) of OGTT; (E) Fasting insulin; (F) HOMA-β index; (G) Pancreatic histological structure with 200× and 400× magnification, respectively. *P< 0.05, **P< 0.01, ***P< 0.001.
In addition, compared to the CON group, the TAC group had significantly higher TC, HDL-C, and urea levels, as well as significantly lower AST, LDH, Na+, Cl− and Mg2+ levels. No difference was observed in the TG, LDL-C, Cr, ALT, ALP, GGT, TP, ALB, GLB, and UA levels between the two groups (Table 1).
3.2 Effects of TAC on composition of the gut microbiota
In the principal co-ordinates analysis (PCoA), the fecal samples of the two groups were divided into different clusters, indicating that TAC changed the composition of the gut microbiota in rats (Figure 2A). The Chao1, ACE, and Shannon indexes were lower in the TAC group than in the CON group, but the differences were not statistically significant (Supplementary Table S7). At the phylum level, the TAC group had significantly higher relative abundances of Proteobacteria and Actinobacteriota and lower relative abundances of Bacteroidetes, Verrucomicrobiota, and Cyanobacteria. The ratio of Bacteroidetes to Firmicutes was significantly lower in the TAC group (Figures 2B, C).
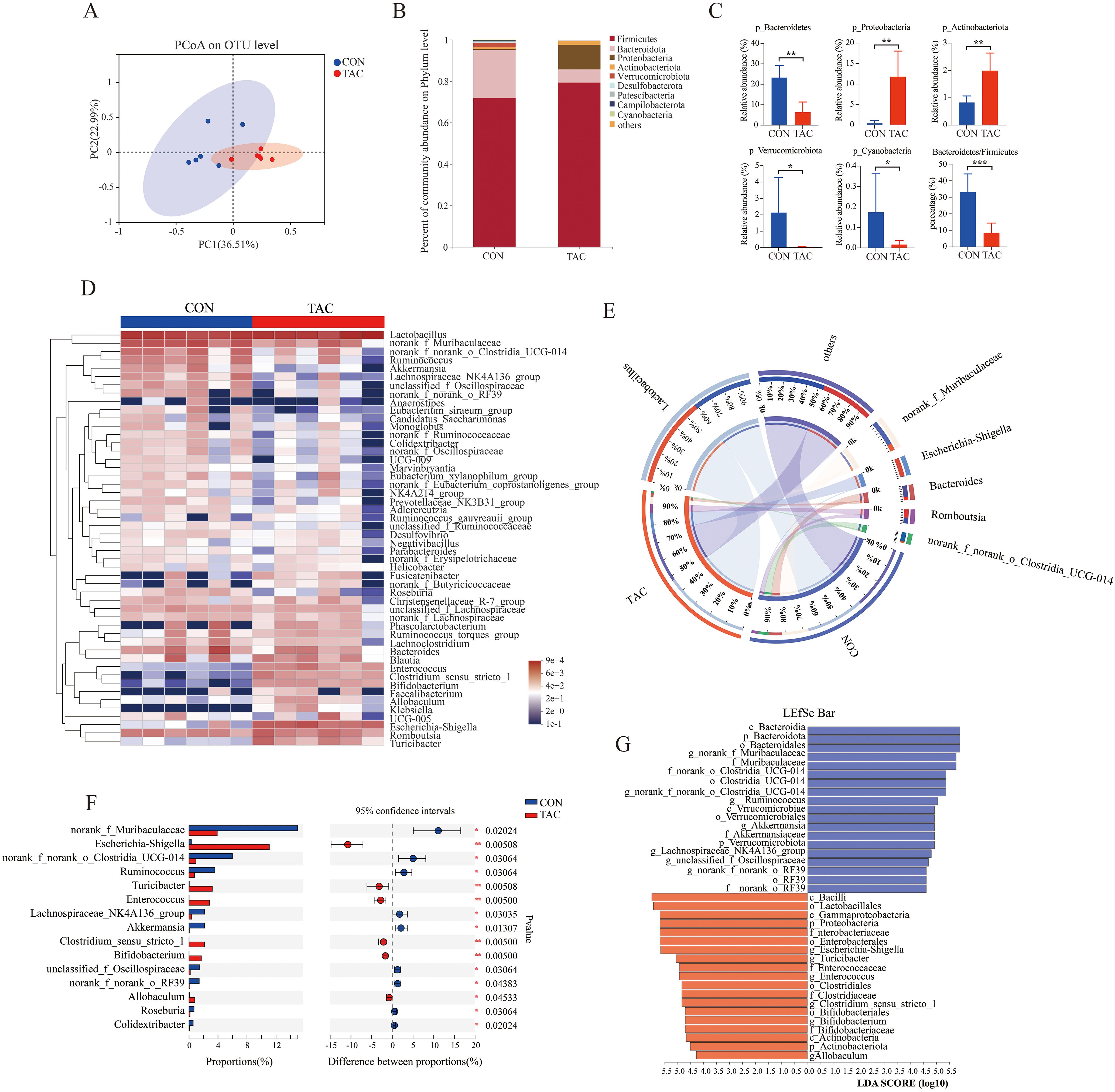
Figure 2. Effects of TAC on the structure of the gut microbiota. (A) PCoA based on OTU; (B) Relative abundance of gut microbiota at the phylum level; (C) The relative abundance of key phyla in the gut microbiota; (D) Heatmap of the relative abundance of the top 50 dominant genera; (E) Community Circos diagram; (F) The relative abundance of different microbiota at the genus level; (G) LEfSe data (LDA > 4.0; *P< 0.05, **P< 0.01, ***P< 0.001.
The top 50 differentiated taxa with the highest genus level could be extracted from the heatmap (Figure 2D). The relatively dominant taxa were Lactobacillus, norank_f_Muribaculaceae, and Bacteroides in the CON group but Lactobacillus, Escherichia-Shigella, and Romboutsia in the TAC group (Figure 2E). With reference to the CON group, the relative abundances of Escherichia-Shigella, Turicibacter, Enterococcus, Bifidobacterium, and Allobaculum were much higher, and the relative abundances of Ruminococcus, Akkermansia, Colidextribacter, and Roseburia were much lower in the TAC group (Figure 2F). The linear discriminant analysis (LDA) effect size (LEfSe) was used to further identify different taxa that may be microbiological markers. With the LDA score cutoff at 4.0, the following key enrichments were noted (Figure 2G): in the CON group, p_Bacteroidota, o_Bacteroidales, c_Bacteroidia, o_Clostridia_UCG-014, c_Verrucomicrobiae, and f_Akkermansiaceae; in the TAC group, c_Bacilli, o_Lactobacillales, c_Gammaproteobacteria, and p_Proteobacteria.
We further ran the correlation analysis between the differential gut genera and the physiological data. The FBG and the AUC of OGTT were correlated positively with the relative abundances of Escherichia-Shigella, Enterococcus, and Bifidobacterium but negatively with the relative abundances of Ruminococcus, Roseburia, and Akkermansia. The FIN and HOMA-β levels were correlated positively with Akkermansia but negatively with Enterococcus. The changes in TG, TC, and HDL-C could also be associated with the gut microbiota (Supplementary Figure S2). The results suggested that the changes in the gut microbiota likely played an important role in TAC-induced diabetes.
The functional genes in the gut microbiota were predicted with PICRUSt2. The functional genes related to metabolic pathways changed significantly in the TAC group. The TAC group had a notable decrease in the percent relative frequency of global and overview maps, amino acid metabolism, and biosynthesis of other secondary metabolites, along with a significant increase in lipid metabolism and metabolism of other AAs (Supplementary Figure S3). In the amino acid metabolism pathways, histidine metabolism, arginine biosynthesis, cysteine and methionine metabolism, and glycine, serine and threonine metabolism were significantly enriched in the CON group, whereas arginine and proline metabolism were significantly enriched in the TAC group. In the lipid metabolism pathways, the biosynthesis of unsaturated fatty acids, primary bile acid biosynthesis, and secondary bile acid biosynthesis were significantly enriched in the TAC group. In addition, the TAC group had significantly higher relative abundance of the biosynthesis of amino acids, fatty acid metabolism, carbon metabolism, and glutathione metabolism than the CON group (Figure 3).
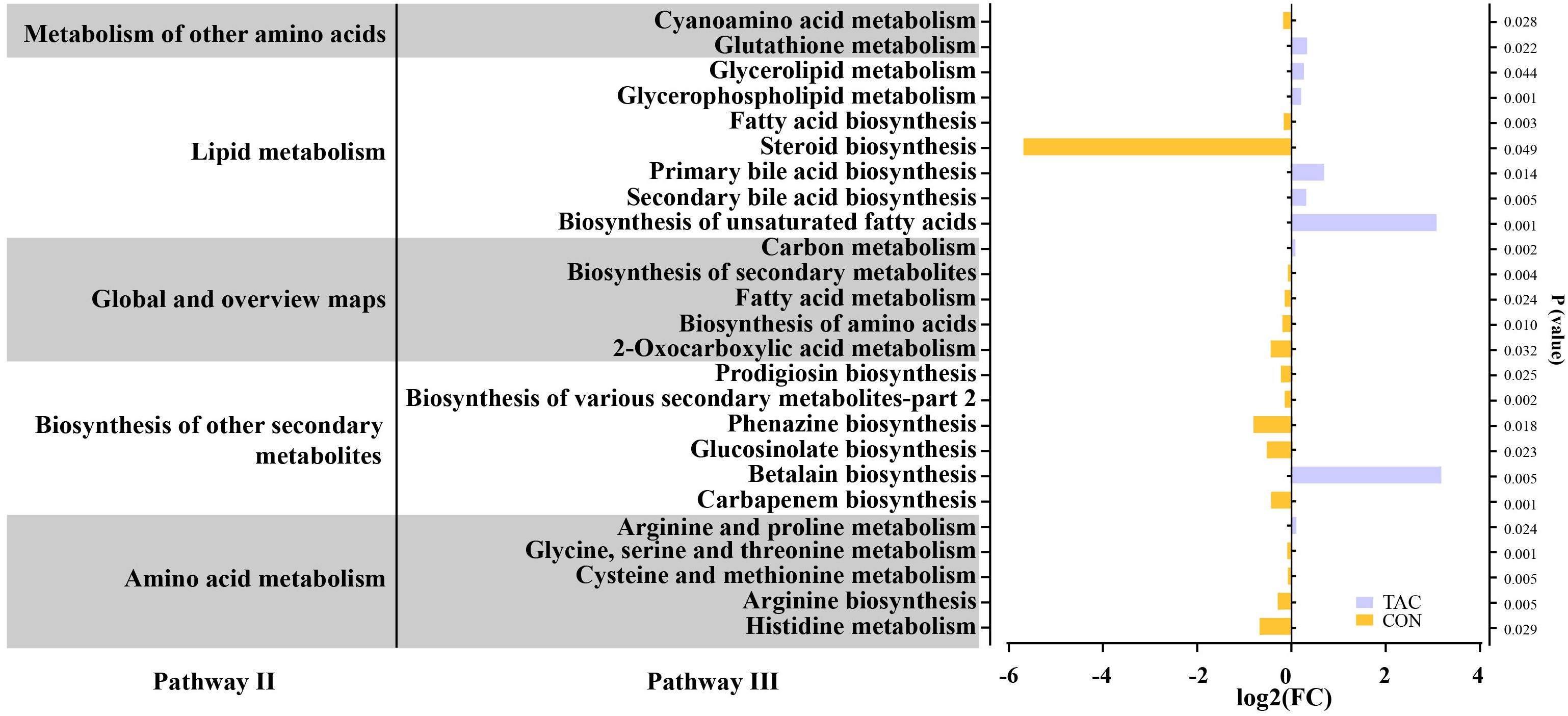
Figure 3. TAC-induced changes in the functional genes of the intestinal microbiota. The horizontal axis shows the relative abundance (fold changes) of differentially expressed functional genes enriched in KEGG pathways (level 3).
3.3 Nontargeted metabolomics analysis
We further evaluated the serum metabolites in the CON and TAC groups based on nontargeted metabolomics to examine how TAC affected the glucose metabolism disorder.
The serum samples of the two groups were well separated in PCA and OPLS-DA (Figures 4A, B). The OPLS-DA permutation test (1000 times) indicated that the model exhibited good reliability and provided good prediction (R2Y = 0.993, Q2Y = 0.854) (Figure 4C). With the criteria VIP > 1 and P< 0.05, 46 common differential metabolites could be considered potential biomarkers (Figure 4D). Compared to the CON group, the levels of 17 metabolites, including glucosaminic acid, galactonic acid, sorbitol, CA, and CDCA, were significantly increased in the TAC group. On the other hand, the metabolites with significant decrease in the TAC group included pyroglutamic acid, asparagine, aspartic acid, 4-hydroxyproline, glutamic acid, 1-methylhistidine, and behenic acid (Table 2).
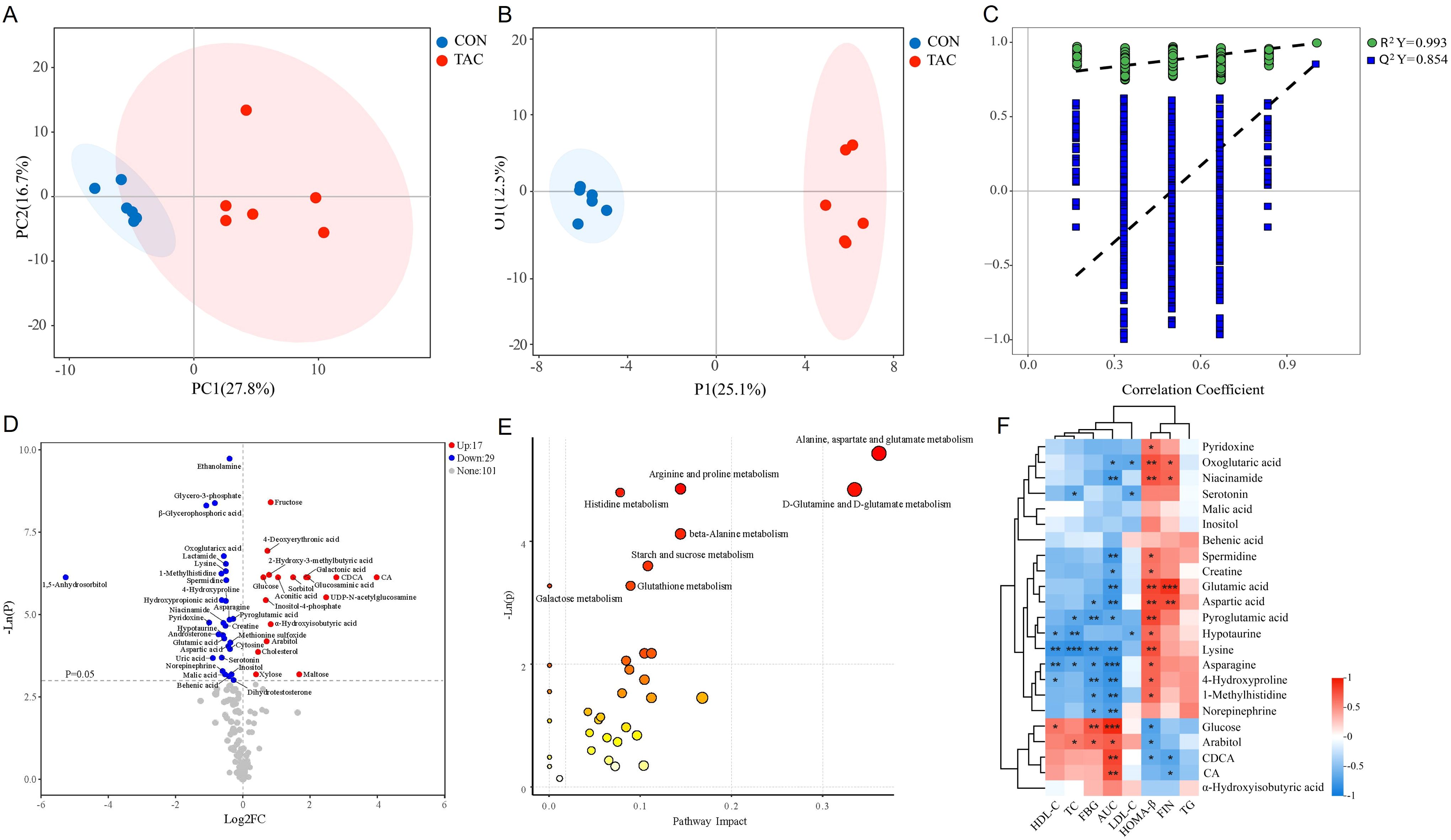
Figure 4. TAC induced changes in the serum metabolite profile and metabolic pathway. (A) PCA; (B) OPLS-DA scores; (C) 1000 random permutation test evaluation; (D) Volcano plot; (E) KEGG pathway analysis of 46 significantly different metabolites; (F) Heatmap of the correlation between the intestinal flora metabolites and the glycolipid metabolism indicators. *P< 0.05, **P< 0.01, ***P< 0.001.
Subsequently, MetaboAnalyst was used for the pathway analysis of differential metabolites to clarify the functional changes in the serum metabolic profile in TAC-induced diabetic rats. The pathways of alanine, aspartate, and glutamate metabolism, D-glutamine and D-glutamate metabolism, arginine and proline metabolism, histidine metabolism, beta-alanine metabolism, starch and sucrose metabolism, galactose metabolism, and glutathione metabolism were significantly altered between the two groups (Figure 4E). Notably, arginine and proline metabolism, histidine metabolism, and glutathione metabolism also significantly differed in the functional prediction of the gut microbiota.
Metabolites associated with the microbiota, such as SCFAs, BAs, tryptophan metabolites, and phenols, have been shown to play a crucial role in the development of diabetes (Wu et al., 2023). Therefore, we screened metabolites related to the intestinal flora and analyzed their correlations with glycolipid metabolism indicators. The results indicated that BAs (CA and CDCA) and carbohydrates were positively correlated with AUC and negatively correlated with HOMA-β. Additionally, vitamins, amines, and AAs were mostly negatively correlated with FBG and AUC but positively correlated with HOMA-β (Figure 4F).
3.4 Targeted metabolomics analysis
3.4.1 Serum amino acid
The TAC group showed significantly reduced serum levels of asparagine, aspartic acid, glutamine, glutamate, histidine, isoleucine, hydroxyproline, tryptophan, methionine, and phenylalanine (Figure 5A). The results for asparagine, aspartic acid, glutamic acid, and hydroxyproline were consistent with the nontargeted analysis, suggesting that they may play an important role in TAC-induced diabetes.
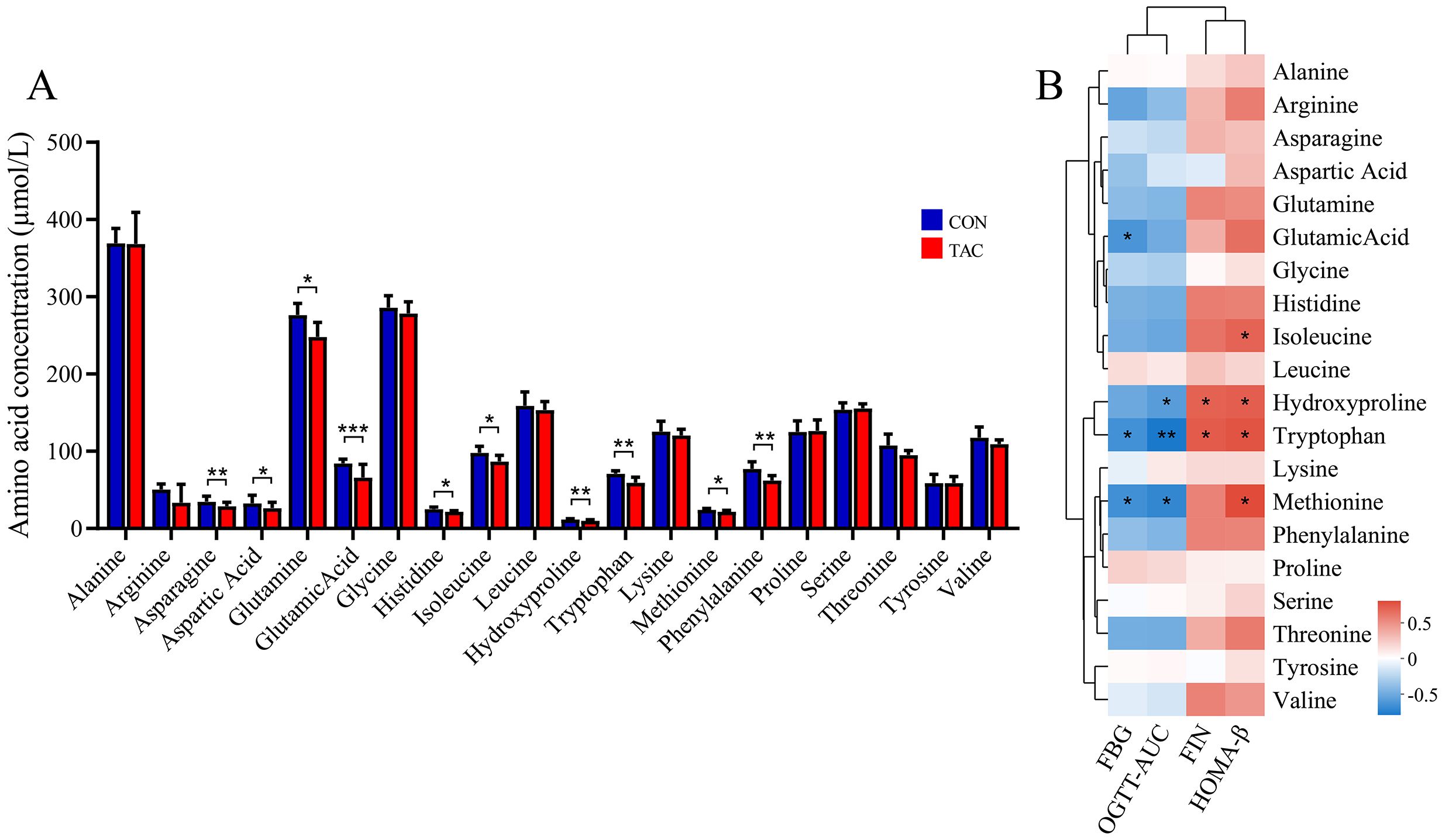
Figure 5. Effects of TAC on the amino acid metabolic profile. (A) Changes in the amino acid levels; (B) Correlation between amino acid and metabolic indexes. *P< 0.05, **P< 0.01, ***P< 0.001.
Spearman correlation was used to further determine the association between the AAs and the glucose metabolism-related indicators. The results showed that the levels of FBG and AUC of OGTT were negatively correlated with glutamic acid, hydroxyproline, tryptophan, and methionine, while FIN and HOMA-β were positively correlated with hydroxyproline, tryptophan, and tyrosine (Figure 5B).
3.4.2 Serum and fecal bile acid
The absolute quantification was carried out for 13 BAs in both serum and feces. We found that TAC significantly increased the primary/secondary BA ratio and the total BAs level, but significantly decreased the conjugated/unconjugated BAs ratio (Figure 6A). The TAC group had significantly higher serum levels of 12-OH/non-12-OH-BAs. Among the non-12-OH-BAs, CDCA, GCA, and GCDCA had significantly elevated levels and TUDCA and TDCA had significantly reduced levels in the TAC group. For the 12-OH-BAs, the level of CA was significantly higher in the TAC group.
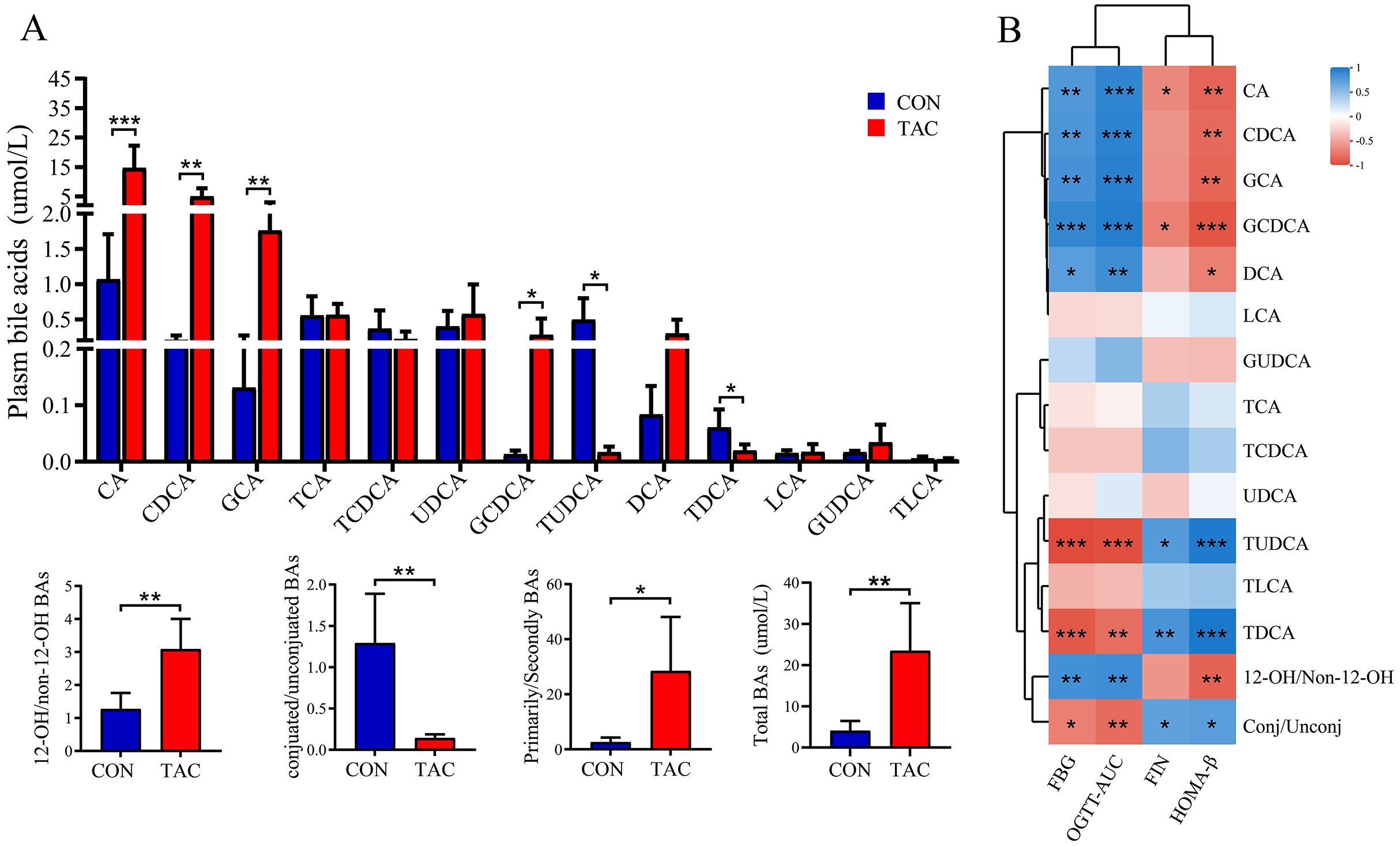
Figure 6. Effects of TAC on the serum bile acid metabolic profile. (A) Changes in bile acid levels; (B) Correlation between bile acids and metabolic indexes. *P< 0.05, **P< 0.01, ***P< 0.001.
The correlation between serum BAs and the index of glucose metabolism was then analyzed. Both FBG and the AUC of the OGTT were correlated significantly positively with 12-OH/non-12-OH-BAs, CA, DCA, and GCA, and significantly negatively with conjugated/unconjugated BAs, TUDCA, and TDCA. In addition, HOMA-β was negatively correlated with 12-OH/non-12-OH-BAs but positively correlated with conjugated/unconjugated BAs (Figure 6B).
As the gut is the site of BA metabolism, the fecal BAs were also examined. Most BAs in the feces had the same variation as in the serum. The exceptions were that the TAC group had significantly higher TCA, TCDCA, TLCA and conjugated/unconjugated BAs along with significantly lower UDCA and LCA (Supplementary Figure S4).
3.4.3 Serum short-chain fatty acids
Short-chain fatty acids are important regulators in the glucose homeostasis (Canfora et al., 2019). There was no difference in the serum SCFAs between the two groups (Supplementary Figure S5).
3.4.4 Correlation analysis of the gut microbiota with host metabolites
The gut microbiota carries out complex metabolic activities in the human intestine, producing numerous beneficial or harmful metabolites in the human circulatory system (Nicholson et al., 2012). Thus, Spearman’s correlation analysis was performed to identify the potential relationships between microbes (the top 50 abundant microflora at the genus level) and AA and BA-related metabolites.
A significant association existed between the changes in the gut microbiota abundance and the serum AA levels. The levels of histidine, hydroxyproline, and phenylalanine were correlated positively with the relative abundances of Akkermansia and Ruminococcus and negatively with the relative abundances of Enterococcus and Bifidobacterium. In addition, the decrease in serum glutamine was negatively correlated with Bifidobacterium (Figure 7A).
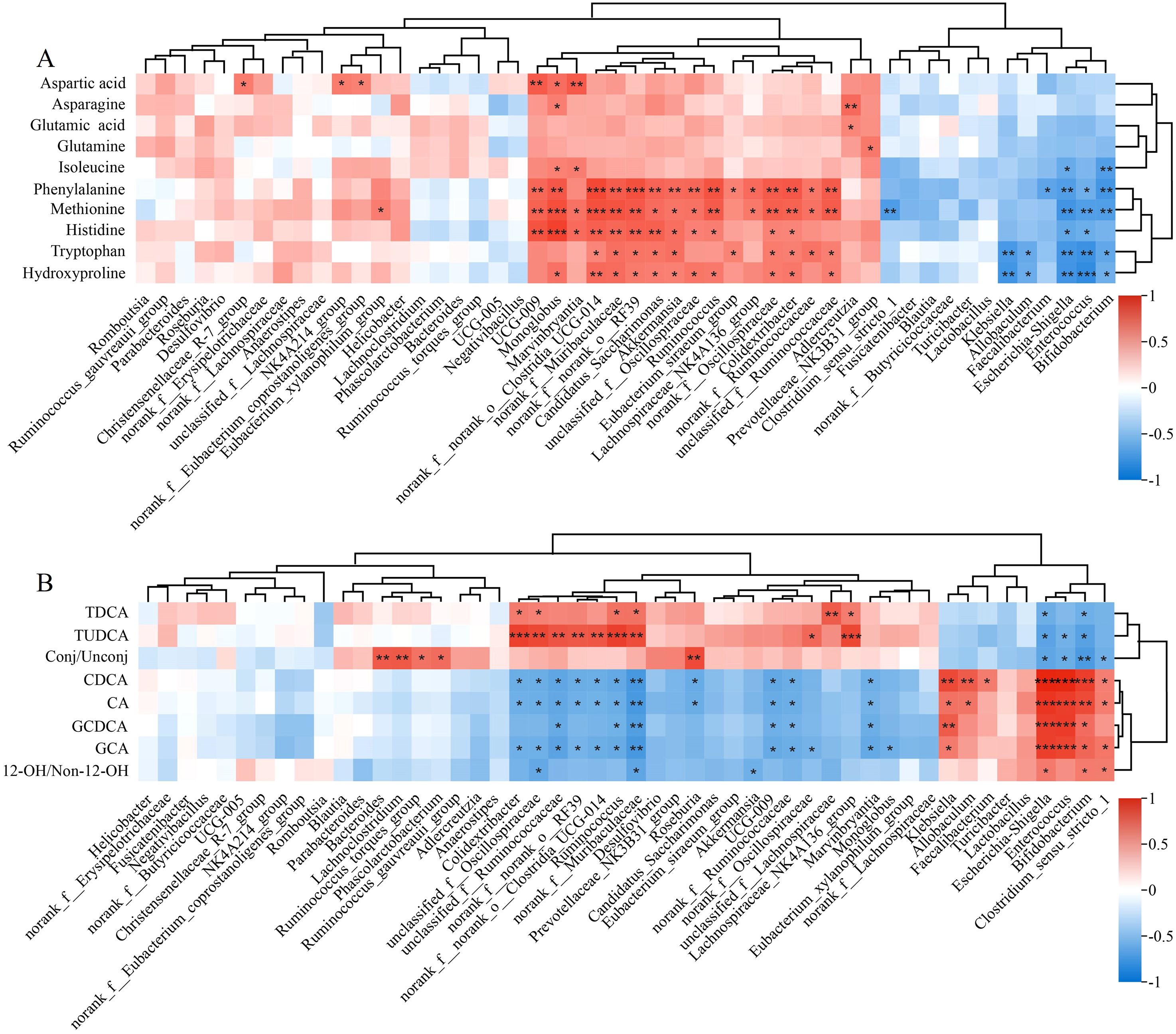
Figure 7. Heatmaps for the correlation between gut microbiota and metabolites related to (A) amino acids and (B) bile acids. *P< 0.05, **P< 0.01, ***P< 0.001.
The gut microbiota was also significantly associated with serum BA levels. Akkermansia and Colidextribacter were correlated positively with CA and CDCA but negatively with TDCA and TUDCA. Escherichia-Shigella, Enterococcus, and Bifidobacterium were correlated positively with GCA and GCDCA. Significantly negative correlation existed between Akkermansia and the level of 12-OH/non-12-OH BAs (Figure 7B).
4 Discussion
In this study, we noted that the prolonged use of TAC significantly decreased the body weight of the rats, possibly due to its toxic effects (such as diabetes, hepatorenal toxicity, etc.) (Quan et al., 2020). It has been reported that TAC has a direct role in causing diabetes (Quintana-Perez et al., 2022), but the underlying mechanism has not been fully elucidated. We then used 16S rRNA sequencing and metabolomics to screen relevant biomarkers involved in TAC-induced diabetes.
The gut microbiota of TAC-induced diabetic rats had significant changes in composition at the phylum and genus levels compared to the control. After the TAC treatment, both the relative abundance of Bacteroidetes and the ratio of Bacteroidetes/Firmicutes decreased significantly. Firmicutes and Bacteroidetes are the dominant bacteria in human and animal intestine, and many studies have shown that the level of Bacteroides and the ratio of Bacteroides/Firmicutes are negatively correlated with blood glucose levels (Tilg and Moschen, 2014; Gurung et al., 2020). The negative correlation between Bacteroides/Firmicutes and FBG was also observed in our TAC-treated rats (r = −0.763, P = 0.004). At the genus level, the TAC-induced diabetic rats had higher abundances of Escherichia-Shigella and Allobaculum and lower abundances of Ruminococcus, Akkermansia, and Roseburia. Zhang et al. also reported previously that for the mice after 14 days of TAC administration, the relative abundances increased significantly for Allobaculum and decreased significantly for Clostridium, Ruminococcus, and Oscillospira in the gut microbiota (Zhang et al., 2018). Meanwhile, we found that the changes in Ruminococcus, Roseburia, and Akkermansia were closely related to FBG and FIN levels according to the correlation analysis. It has been reported that Roseburia and Akkermansia help to restore insulin sensitivity and improve glucose metabolism, and a decrease in Ruminococcus abundance may be related to changes in the blood glucose of the host (Huda et al., 2021). Another study suggested that the decreased abundance of Roseburia may be directly related to the development of T2DM (Karlsson et al., 2013). These results suggested that changes in the structure of the gut microbiota may be related to diabetes induced by TAC.
With the development of metagenomics and metabolomics, it has been found that small molecular compounds metabolized by the gut microbiota can act as a “bridge” between the gut microbiota and disease (Koh and Backhed, 2020). Therefore, we identified the differences in a variety of metabolites, including AAs, lipids, amines, BAs, and fatty acids, between the CON and TAC groups through non-targeted metabolomics. The interactions between these metabolites and the cell receptors may impact the health of the host (Massey and Brown, 2021).
Amino acids play an important role in the development of T2DM and IR (Ding et al., 2023). After TAC exposure, the pathways related to AA metabolism (alanine, aspartic acid, and glutamic acid metabolism, D-glutamine and D-glutamic acid metabolism, arginine and proline metabolism, histidine metabolism) in the serum of SD rats were interfered. This was further confirmed by the decreasing serum levels of aspartic acid, glutamic acid, glutamine, histidine, and hydroxyproline in targeted metabolomics analysis. Low levels of asparagine and aspartic acid are associated with an increased risk of T2DM (Ottosson et al., 2018; Chen et al., 2021). A meta-analysis of metabolomics studies revealed that higher levels of glutamine and glutamate in the blood are associated with a lower risk of diabetes (Guasch-Ferre et al., 2016). Our results revealed that glutamate levels were negatively correlated with FBG, and the TAC group had lower glutamate levels. Glutamate can be converted to glutathione, and oxidative stress caused by glutathione depletion plays an important role in the development of T2DM (Lv et al., 2023). The reduction of insulin resistance is also intimately associated with histidine and its metabolites (Holecek, 2020). Because insulin resistance (including the inhibition of proteolysis) can increase the release of BCAAs in muscle and increase their circulating levels (Mccormack et al., 2013), high levels of plasma BCAAs (leucine, isoleucine, and valine) may be associated with a high risk of PTDM in renal transplant recipients (Oste et al., 2020). However, animal studies have found that supplementation of BCAAs in mice receiving a high fat diet can promote insulin action and signal transduction (Macotela et al., 2011). These conflicting results suggest that the relationship between BCAAs and the occurrence and development of T2DM is complex. Our targeted metabolomics analysis showed that the serum isoleucine levels were significantly reduced by the TAC treatment. Clinical studies have also found that serum leucine levels were significantly reduced in renal transplant patients after TAC treatment (Kim et al., 2010), which may be related to the increase of BCAAs aminotransferase activity in the renal parenchyma and the depletion of BCAAs caused by the decrease of branched-chain α-ketoacid dehydrogenase activity (Adeva et al., 2012; Saleem et al., 2019). The above results suggested that AAs may be a potential marker for TAC-induced diabetes. Previous research has also shown that supplementing mice with a BCAA mixture can increase the abundances of Akkermansia and Bifidobacterium and decrease the abundance of Enterobacteriaceae in the gut microbiota (Yang et al., 2016). In addition, studies have indicated that for mice having a high-fat diet, when the diet was supplemented with resistant dextrin, the levels of bacteria such as Paraclactis, Brautella, and Dubosiella in the gut microbiota were also changed, which consequently affected the levels of their metabolites, including BAs (enterohepatic circulation), indole derivatives (tryptophan metabolism), and lipoic acid (lipid metabolism) (Zhang et al., 2021). Tryptophan is an essential amino acid that is produced through protein fermentation in food. It is converted into indole and its derivatives by bacteria in the large intestine and then utilized by the body. Indole derivatives are microbial tryptophan catalysts beneficial to human health. A higher circulating indole propionate is associated with a lower risk of T2DM (Tuomainen et al., 2018). Tryptophanase, which can decompose tryptophan to produce indole, exists in the intestinal bacteria such as Bacteroides polymorphus, Clostridium ovalis, Clostridium citricum, Enterococcus faecalis, and Escherichia coli. The protective effect and mechanism of these metabolites on diabetes require further exploration and verification (Lee and Lee, 2010; Gao et al., 2023).
Significant changes in specific BAs species were found in this study. In the TAC rats, the serum levels of CA, CDCA, GCA, TCA, GCDCA, and DCA increased significantly, whereas the levels of TDCA and TUDCA decreased significantly. As the main component of bile, primary BAs are synthesized in the liver based on cholesterol by a series of enzymatic reactions and are excreted into the intestine through the gallbladder. They are also bio-transformed in the colon to secondary BAs by the gut microbiota, and both primary and secondary BAs can be returned to the liver upon reabsorption in the ileum and colon (Chiang, 2013; Di Ciaula et al., 2017). Over the last few decades, BAs have attracted considerable attention in the integrated regulation of lipid, glucose, energy metabolism, etc. However, current clinical studies have shown conflicting results. For instance, Sonne et al. found that T2DM patients had higher postprandial total BAs, CA, CDCA, DCA, and UDCA levels than healthy patients (Sonne et al., 2014). In contrast, a Chinese cohort study showed that the T2DM risk was associated negatively with the uncombined BAs (CA, CDCA, and DCA), although it was associated positively with the combined primary BAs (GCA, TCA, GCDCA, and TCDCA) and with secondary BAs (TUDCA) (Lu et al., 2021). In addition, in our study, the administration of TAC significantly increased the serum and fecal 12-OH/non-12OH BAs. Studies have suggested 12-OH/non-12-OH BAs ratio as a potential biomarker for hyperglycemia and insulin resistance, since a higher ratio is associated with islet signaling, higher blood glucose, and lower insulin sensitivity (Haeusler et al., 2012; Haeusler et al., 2013; Qi et al., 2015; Zhong et al., 2022). We also found that the serum BA levels were closely related to FBG, AUC of OGTT, and FIN. It has been discovered that intestinal bacteria, such as Bacteroides, Bifidobacterium, Clostridium, Lactobacillus, and Ruminococcus, play a crucial role in the metabolism of BAs (Okekunle et al., 2019). A key step in BA biotransformation is the microbial debinding by bile acid hydrolase (BSH). Intestinal bacteria including Lactobacillus, Bifidobacterium, Enterococcus, and Bacteroides all exhibit BSH activity (Wahlstrom et al., 2016). Previous studies have demonstrated that antioxidants can affect BSH activity and lead to changes in BA composition to ultimately ameliorate obesity (Huang et al., 2019). Primary BAs that are not reabsorbed enter the colon and are metabolized to secondary BAs by 7-dehydroxylation. For example, CA and CDCA are metabolized to DCA and LCA respectively. The 7-dehydroxylation process involves multiple reactions between Firmicutes (mainly Clostridium and Eubacterium genera) and genes induced by BAs (Collins et al., 2023). Furthermore, recent studies have indicated that changes in BA levels may be associated with bacteria rich in β-glucuronidase (GUS) (Li et al., 2024). A significant increase in the intestinal GUS activity was found in TAC-induced diabetic mice. On the contrary, Bacteroides play a pivotal role in the synthesis of GUS in intestinal microbes and the impact of GUS on drug glucuronidation (Elmassry et al., 2021). Therefore, further research is necessary to validate the significance of gut microbiota and BAs in TAC-induced diabetes.
SCFAs are mainly produced by the fermentation of dietary fiber in food by probiotics (Louis et al., 2014). The regulation of blood glucose by SCFAs has received extensive attention. In T2DM patients, reduced SCFA levels caused by a decreased abundance of SCFA-producing bacteria are associated with insulin resistance and the development of T2DM (Zhao et al., 2018). In mice with diabetes, butyrate supplementation could promote glycogen storage in hepatocytes and improve blood glucose and hepatic glycogen metabolic homeostasis (Zhang et al., 2019). Jiao et al. noted the decrease of SCFAs, especially butyrate, in TAC-induced diabetic mice, and found that butyrate supplementations in the diet significantly improved the glucose tolerance of TAC-induced diabetic mice and increased the expression of GLP-1 in colon and GPR43 in the intestinal crypt (Jiao et al., 2020). However, in our experiments, we observed no difference in SCFAs between the CON group and the TAC group. The observed lack of difference may be attributed to the fact that TAC primarily exerts its effects on the gut microbiota (Wang et al., 2023).
This study only has omics data and approximate correlation analysis. Additional research is needed to verify if the identified metabolites and bacteria have the same trends in clinical findings. Another limitation of this work is that it does not experimentally confirm any causal relationship between intestinal microbiota and host diseases, and relevant experimental evidence is needed in the future.
5 Conclusions
We for the first time evaluated the changes of the gut microbiota and the host metabolism in TAC-induced diabetic rats. The analyses showed that the alterations in the gut microbiota and metabolites, particularly Ackermannia, Ruminococcus, Roseburia, BAs, and AAs, may participate in the progression of TAC-induced diabetes. However, no conclusive evidence could be recognized to suggest that TAC leads to diabetes via the changes in microbial metabolites. The role of microbial metabolites in the development of TAC-induced diabetes remains to be further studied.
Data availability statement
The raw sequencing data in our study have been deposited in the BioProject, accession number: PRJNA1157748.
Ethics statement
The animal study was approved by the Ethics Committee of the Third Affiliated Hospital of Soochow University/The First People’s Hospital of Changzhou. The study was conducted in accordance with the local legislation and institutional requirements.
Author contributions
ZJ: Formal analysis, Investigation, Project administration, Writing – original draft. MQ: Investigation, Writing – original draft. ZZ: Formal analysis, Validation, Writing – original draft. XY: Methodology, Writing – original draft. CX: Investigation, Writing – original draft. LZ: Investigation, Writing – original draft. JJ: Funding acquisition, Supervision, Writing – review & editing. WZ: Writing – review & editing, Conceptualization. NH: Writing – original draft, Writing – review & editing, Conceptualization.
Funding
The author(s) declare financial support was received for the research, authorship, and/or publication of this article. This work was supported by the Changzhou Sci&Tech Program (CM20223005) and the China Postdoctoral Science Foundation (2021M700546).
Conflict of interest
The authors declare that the research was conducted in the absence of any commercial or financial relationships that could be construed as a potential conflict of interest.
Publisher’s note
All claims expressed in this article are solely those of the authors and do not necessarily represent those of their affiliated organizations, or those of the publisher, the editors and the reviewers. Any product that may be evaluated in this article, or claim that may be made by its manufacturer, is not guaranteed or endorsed by the publisher.
Supplementary material
The Supplementary Material for this article can be found online at: https://www.frontiersin.org/articles/10.3389/fcimb.2024.1436477/full#supplementary-material
References
Adeva, M. M., Calvino, J., Souto, G., Donapetry, C. (2012). Insulin resistance and the metabolism of branched-chain amino acids in humans. Amino Acids 43, 171–181. doi: 10.1007/s00726-011-1088-7
Brunet, M., Van Gelder, T., Asberg, A., Haufroid, V., Hesselink, D. A., Langman, L., et al. (2019). Therapeutic drug monitoring of tacrolimus-personalized therapy: second consensus report. Ther. Drug Monit. 41, 261–307. doi: 10.1097/FTD.0000000000000640
Canfora, E. E., Meex, R. C. R., Venema, K., Blaak, E. E. (2019). Gut microbial metabolites in obesity, NAFLD and T2DM. Nat. Rev. Endocrinol. 15, 261–273. doi: 10.1038/s41574-019-0156-z
Chen, X., Hu, K., Shi, H. Z., Zhang, Y. J., Chen, L., He, S. M., et al. (2024). Syk/BLNK/NF-kappaB signaling promotes pancreatic injury induced by tacrolimus and potential protective effect from rapamycin. BioMed. Pharmacother. 171, 116125. doi: 10.1016/j.biopha.2024.116125
Chen, Y., Wang, N., Dong, X., Zhu, J., Chen, Y., Jiang, Q., et al. (2021). Associations between serum amino acids and incident type 2 diabetes in Chinese rural adults. Nutr. Metab. Cardiovasc. Dis.: NMCD 31, 2416–2425. doi: 10.1016/j.numecd.2021.05.004
Chen, Y. G., Liu, F., Massague, J. (1997). Mechanism of TGFbeta receptor inhibition by FKBP12. EMBO J. 16, 3866–3876. doi: 10.1093/emboj/16.13.3866
Chiang, J. Y. (2013). Bile acid metabolism and signaling. Compr. Physiol. 3, 1191–1212. doi: 10.1002/cphy.c120023
Collins, S. L., Stine, J. G., Bisanz, J. E., Okafor, C. D., Patterson, A. D. (2023). Bile acids and the gut microbiota: metabolic interactions and impacts on disease. Nat. Rev. Microbiol. 21, 236–247. doi: 10.1038/s41579-022-00805-x
Degraeve, A. L., Moudio, S., Haufroid, V., Chaib Eddour, D., Mourad, M., Bindels, L. B., et al. (2020). Predictors of tacrolimus pharmacokinetic variability: current evidences and future perspectives. Expert Opin. Drug Metab. Toxicol. 16, 769–782. doi: 10.1080/17425255.2020.1803277
Di Ciaula, A., Garruti, G., Lunardi Baccetto, R., Molina-Molina, E., Bonfrate, L., Wang, D. Q., et al. (2017). Bile acid physiology. Ann. Hepatol. 16, s4–s14. doi: 10.5604/01.3001.0010.5493
Ding, Y., Wang, S., Lu, J. (2023). Unlocking the potential: amino acids’ Role in predicting and exploring therapeutic avenues for type 2 diabetes mellitus. Metabolites 13, 1017. doi: 10.3390/metabo13091017
Elmassry, M. M., Kim, S., Busby, B. (2021). Predicting drug-metagenome interactions: Variation in the microbial beta-glucuronidase level in the human gut metagenomes. PloS One 16, e0244876. doi: 10.1371/journal.pone.0244876
Fu, L., Qiu, Y., Shen, L., Cui, C., Wang, S., Wang, S., et al. (2018). The delayed effects of antibiotics in type 2 diabetes, friend or foe? J. Endocrinol. 238, 137–149. doi: 10.1530/JOE-17-0709
Gao, J., Yang, T., Song, B., Ma, X., Ma, Y., Lin, X., et al. (2023). Abnormal tryptophan catabolism in diabetes mellitus and its complications: Opportunities and challenges. BioMed. Pharmacother. 166, 115395. doi: 10.1016/j.biopha.2023.115395
Goodyer, W. R., Gu, X., Liu, Y., Bottino, R., Crabtree, G. R., Kim, S. K. (2012). Neonatal beta cell development in mice and humans is regulated by calcineurin/NFAT. Dev. Cell 23, 21–34. doi: 10.1016/j.devcel.2012.05.014
Guasch-Ferre, M., Hruby, A., Toledo, E., Clish, C. B., Martinez-Gonzalez, M. A., Salas-Salvado, J., et al. (2016). Metabolomics in prediabetes and diabetes: A systematic review and meta-analysis. Diabetes Care 39, 833–846. doi: 10.2337/dc15-2251
Gurung, M., Li, Z., You, H., Rodrigues, R., Jump, D. B., Morgun, A., et al. (2020). Role of gut microbiota in type 2 diabetes pathophysiology. EBioMedicine 51, 102590. doi: 10.1016/j.ebiom.2019.11.051
Haeusler, R. A., Astiarraga, B., Camastra, S., Accili, D., Ferrannini, E. (2013). Human insulin resistance is associated with increased plasma levels of 12alpha-hydroxylated bile acids. Diabetes 62, 4184–4191. doi: 10.2337/db13-0639
Haeusler, R. A., Pratt-Hyatt, M., Welch, C. L., Klaassen, C. D., Accili, D. (2012). Impaired generation of 12-hydroxylated bile acids links hepatic insulin signaling with dyslipidemia. Cell Metab. 15, 65–74. doi: 10.1016/j.cmet.2011.11.010
Han, Y., Jiang, X., Ling, Q., Wu, L., Wu, P., Tang, R., et al. (2019). Antibiotics-mediated intestinal microbiome perturbation aggravates tacrolimus-induced glucose disorders in mice. Front. Med. 13, 471–481. doi: 10.1007/s11684-019-0686-8
He, J., Zhang, P., Shen, L., Niu, L., Tan, Y., Chen, L., et al. (2020). Short-chain fatty acids and their association with signalling pathways in inflammation, glucose and lipid metabolism. Int. J. Mol. Sci. 21, 6356. doi: 10.3390/ijms21176356
Holecek, M. (2020). Histidine in health and disease: metabolism, physiological importance, and use as a supplement. Nutrients 12, 848. doi: 10.3390/nu12030848
Hu, N., Zhang, Q., Wang, H., Yang, X., Jiang, Y., Chen, R., et al. (2021). Comparative evaluation of the effect of metformin and insulin on gut microbiota and metabolome profiles of type 2 diabetic rats induced by the combination of streptozotocin and high-fat diet. Front. Pharmacol. 12, 794103. doi: 10.3389/fphar.2021.794103
Huang, F., Zheng, X., Ma, X., Jiang, R., Zhou, W., Zhou, S., et al. (2019). Theabrownin from Pu-erh tea attenuates hypercholesterolemia via modulation of gut microbiota and bile acid metabolism. Nat. Commun. 10, 4971. doi: 10.1038/s41467-019-12896-x
Huda, M. N., Kim, M., Bennett, B. J. (2021). Modulating the microbiota as a therapeutic intervention for type 2 diabetes. Front. Endocrinol. (Lausanne) 12, 632335. doi: 10.3389/fendo.2021.632335
Jiao, W., Zhang, Z., Xu, Y., Gong, L., Zhang, W., Tang, H., et al. (2020). Butyric acid normalizes hyperglycemia caused by the tacrolimus-induced gut microbiota. Am. J. Transplant. 20, 2413–2424. doi: 10.1111/ajt.15880
Jouve, T., Noble, J., Rostaing, L., Malvezzi, P. (2018). Tailoring tacrolimus therapy in kidney transplantation. Expert Rev. Clin. Pharmacol. 11, 581–588. doi: 10.1080/17512433.2018.1479638
Karlsson, F. H., Tremaroli, V., Nookaew, I., Bergstrom, G., Behre, C. J., Fagerberg, B., et al. (2013). Gut metagenome in European women with normal, impaired and diabetic glucose control. Nature 498, 99–103. doi: 10.1038/nature12198
Kim, C. D., Kim, E. Y., Yoo, H., Lee, J. W., Ryu, D. H., Noh, D. W., et al. (2010). Metabonomic analysis of serum metabolites in kidney transplant recipients with cyclosporine A- or tacrolimus-based immunosuppression. Transplantation 90, 748–756. doi: 10.1097/TP.0b013e3181edd69a
Koh, A., Backhed, F. (2020). From association to causality: the role of the gut microbiota and its functional products on host metabolism. Mol. Cell 78, 584–596. doi: 10.1016/j.molcel.2020.03.005
Lee, J. H., Lee, J. (2010). Indole as an intercellular signal in microbial communities. FEMS Microbiol. Rev. 34, 426–444. doi: 10.1111/j.1574-6976.2009.00204.x
Li, P., Zhang, R., Zhou, J., Guo, P., Liu, Y., Shi, S. (2024). Vancomycin relieves tacrolimus-induced hyperglycemia by eliminating gut bacterial beta-glucuronidase enzyme activity. Gut Microbes 16, 2310277. doi: 10.1080/19490976.2024.2310277
Louis, P., Hold, G. L., Flint, H. J. (2014). The gut microbiota, bacterial metabolites and colorectal cancer. Nat. Rev. Microbiol. 12, 661–672. doi: 10.1038/nrmicro3344
Lu, J., Wang, S., Li, M., Gao, Z., Xu, Y., Zhao, X., et al. (2021). Association of serum bile acids profile and pathway dysregulation with the risk of developing diabetes among normoglycemic Chinese adults: findings from the 4C study. Diabetes Care 44, 499–510. doi: 10.2337/dc20-0884
Lv, Y., Zheng, Y., Zhao, X., Li, Z., Wang, G. (2023). The relationship between islet beta-cell function and metabolomics in overweight patients with Type 2 diabetes. Biosci. Rep. 43, BSR20221430. doi: 10.1042/BSR20221430
Macotela, Y., Emanuelli, B., Bang, A. M., Espinoza, D. O., Boucher, J., Beebe, K., et al. (2011). Dietary leucine–an environmental modifier of insulin resistance acting on multiple levels of metabolism. PloS One 6, e21187. doi: 10.1371/journal.pone.0021187
Massey, W., Brown, J. M. (2021). The gut microbial endocrine organ in type 2 diabetes. Endocrinology 162, bqaa235. doi: 10.1210/endocr/bqaa235
Mccormack, S. E., Shaham, O., Mccarthy, M. A., Deik, A. A., Wang, T. J., Gerszten, R. E., et al. (2013). Circulating branched-chain amino acid concentrations are associated with obesity and future insulin resistance in children and adolescents. Pediatr. Obes. 8, 52–61. doi: 10.1111/j.2047-6310.2012.00087.x
Nalesnik, M. A., Todo, S., Murase, N., Gryzan, S., Lee, P. H., Makowka, L., et al. (1987). Toxicology of FK-506 in the lewis rat. Transplant. Proc. 19, 89–92.
Nicholson, J. K., Holmes, E., Kinross, J., Burcelin, R., Gibson, G., Jia, W., et al. (2012). Host-gut microbiota metabolic interactions. Science 336, 1262–1267. doi: 10.1126/science.1223813
Okekunle, A. P., Zhang, M., Wang, Z., Onwuka, J. U., Wu, X., Feng, R., et al. (2019). Dietary branched-chain amino acids intake exhibited a different relationship with type 2 diabetes and obesity risk: a meta-analysis. Acta Diabetol. 56, 187–195. doi: 10.1007/s00592-018-1243-7
Oste, M. C. J., Flores-Guerrero, J. L., Gruppen, E. G., Kieneker, L. M., Connelly, M. A., Otvos, J. D., et al. (2020). High plasma branched-chain amino acids are associated with higher risk of post-transplant diabetes mellitus in renal transplant recipients. J. Clin. Med. 9, 511. doi: 10.3390/jcm9020511
Ottosson, F., Smith, E., Melander, O., Fernandez, C. (2018). Altered asparagine and glutamate homeostasis precede coronary artery disease and type 2 diabetes. J. Clin. Endocrinol. Metab. 103, 3060–3069. doi: 10.1210/jc.2018-00546
Qi, Y., Jiang, C., Cheng, J., Krausz, K. W., Li, T., Ferrell, J. M., et al. (2015). Bile acid signaling in lipid metabolism: metabolomic and lipidomic analysis of lipid and bile acid markers linked to anti-obesity and anti-diabetes in mice. Biochim. Biophys. Acta 1851, 19–29. doi: 10.1016/j.bbalip.2014.04.008
Quan, Y., Luo, K., Cui, S., Lim, S. W., Shin, Y. J., Ko, E. J., et al. (2020). The therapeutic efficacy of water-soluble coenzyme Q10 in an experimental model of tacrolimus-induced diabetes mellitus. Korean J. Intern. Med. 35, 1443–1456. doi: 10.3904/kjim.2019.269
Quintana-Perez, J. C., Garcia-Dolores, F., Valdez-Guerrero, A. S., Aleman-Gonzalez-Duhart, D., Arellano-Mendoza, M. G., Rojas Hernandez, S., et al. (2022). Modeling type 2 diabetes in rats by administering tacrolimus. Islets 14, 114–127. doi: 10.1080/19382014.2022.2051991
Ribbenstedt, A., Ziarrusta, H., Benskin, J. P. (2018). Development, characterization and comparisons of targeted and non-targeted metabolomics methods. PloS One 13, e0207082. doi: 10.1371/journal.pone.0207082
Rodriguez-Rodriguez, A. E., Donate-Correa, J., Rovira, J., Cuesto, G., Luis-Ravelo, D., Fernandes, M. X., et al. (2019). Inhibition of the mTOR pathway: A new mechanism of beta cell toxicity induced by tacrolimus. Am. J. Transplant. 19, 3240–3249. doi: 10.1111/ajt.15483
Rodriguez-Rodriguez, A. E., Porrini, E., Torres, A. (2021). Beta-cell dysfunction induced by tacrolimus: A way to explain type 2 diabetes? Int. J. Mol. Sci. 22, 10311. doi: 10.3390/ijms221910311
Sah, D. K., Arjunan, A., Park, S. Y., Jung, Y. D. (2022). Bile acids and microbes in metabolic disease. World J. Gastroenterol. 28, 6846–6866. doi: 10.3748/wjg.v28.i48.6846
Saleem, T., Dahpy, M., Ezzat, G., Abdelrahman, G., Abdel-Aziz, E., Farghaly, R. (2019). The profile of plasma free amino acids in type 2 diabetes mellitus with insulin resistance: association with microalbuminuria and macroalbuminuria. Appl. Biochem. Biotechnol. 188, 854–867. doi: 10.1007/s12010-019-02956-9
Schrimpe-Rutledge, A. C., Codreanu, S. G., Sherrod, S. D., McLean, J. A. (2016). Untargeted metabolomics strategies-challenges and emerging directions. J. Am. Soc. Mass Spectrom 27, 1897–1905. doi: 10.1007/s13361-016-1469-y
Sonne, D. P., Rehfeld, J. F., Holst, J. J., Vilsboll, T., Knop, F. K. (2014). Postprandial gallbladder emptying in patients with type 2 diabetes: potential implications for bile-induced secretion of glucagon-like peptide 1. Eur. J. Endocrinol. 171, 407–419. doi: 10.1530/EJE-14-0309
Tilg, H., Moschen, A. R. (2014). Microbiota and diabetes: an evolving relationship. Gut 63, 1513–1521. doi: 10.1136/gutjnl-2014-306928
Tong, L., Li, W., Zhang, Y., Zhou, F., Zhao, Y., Zhao, L., et al. (2021). Tacrolimus inhibits insulin release and promotes apoptosis of Min6 cells through the inhibition of the PI3K/Akt/mTOR pathway. Mol. Med. Rep. 24, 658. doi: 10.3892/mmr
Tuomainen, M., Lindstrom, J., Lehtonen, M., Auriola, S., Pihlajamaki, J., Peltonen, M., et al. (2018). Associations of serum indolepropionic acid, a gut microbiota metabolite, with type 2 diabetes and low-grade inflammation in high-risk individuals. Nutr. Diabetes 8, 35. doi: 10.1038/s41387-018-0046-9
Wahlstrom, A., Sayin, S. I., Marschall, H. U., Backhed, F. (2016). Intestinal crosstalk between bile acids and microbiota and its impact on host metabolism. Cell Metab. 24, 41–50. doi: 10.1016/j.cmet.2016.05.005
Wang, J., Zhu, N., Su, X., Gao, Y., Yang, R. (2023). Gut-microbiota-derived metabolites maintain gut and systemic immune homeostasis. Cells 12, 793. doi: 10.3390/cells12050793
Wu, J., Yang, K., Fan, H., Wei, M., Xiong, Q. (2023). Targeting the gut microbiota and its metabolites for type 2 diabetes mellitus. Front. Endocrinol. (Lausanne) 14, 1114424. doi: 10.3389/fendo.2023.1114424
Wu, Z., Cheng, W., Wang, Z., Feng, S., Zou, H., Tan, X., et al. (2021). Intestinal microbiota and serum metabolic profile responded to two nutritional different diets in mice. Front. Nutr. 8, 813757. doi: 10.3389/fnut.2021.813757
Yang, Z., Huang, S., Zou, D., Dong, D., He, X., Liu, N., et al. (2016). Metabolic shifts and structural changes in the gut microbiota upon branched-chain amino acid supplementation in middle-aged mice. Amino Acids 48, 2731–2745. doi: 10.1007/s00726-016-2308-y
Zhai, L., Wu, J., Lam, Y. Y., Kwan, H. Y., Bian, Z. X., Wong, H. L. X. (2021). Gut-microbial metabolites, probiotics and their roles in type 2 diabetes. Int. J. Mol. Sci. 22, 12846. doi: 10.3390/ijms222312846
Zhang, W. Q., Zhao, T. T., Gui, D. K., Gao, C. L., Gu, J. L., Gan, W. J., et al. (2019). Sodium butyrate improves liver glycogen metabolism in type 2 diabetes mellitus. J. Agric. Food Chem. 67, 7694–7705. doi: 10.1021/acs.jafc.9b02083
Zhang, Z., Chen, X., Cui, B. (2021). Modulation of the fecal microbiome and metabolome by resistant dextrin ameliorates hepatic steatosis and mitochondrial abnormalities in mice. Food Funct. 12, 4504–4518. doi: 10.1039/D1FO00249J
Zhang, Z., Liu, L., Tang, H., Jiao, W., Zeng, S., Xu, Y., et al. (2018). Immunosuppressive effect of the gut microbiome altered by high-dose tacrolimus in mice. Am. J. Transplant. 18, 1646–1656. doi: 10.1111/ajt.14661
Zhao, L., Zhang, F., Ding, X., Wu, G., Lam, Y. Y., Wang, X., et al. (2018). Gut bacteria selectively promoted by dietary fibers alleviate type 2 diabetes. Science 359, 1151–1156. doi: 10.1126/science.aao5774
Zheng, X., Chen, T., Jiang, R., Zhao, A., Wu, Q., Kuang, J., et al. (2021). Hyocholic acid species improve glucose homeostasis through a distinct TGR5 and FXR signaling mechanism. Cell Metab. 33, 791–803.e7. doi: 10.1016/j.cmet.2020.11.017
Zhong, S., Chevre, R., Castano Mayan, D., Corliano, M., Cochran, B. J., Sem, K. P., et al. (2022). Haploinsufficiency of CYP8B1 associates with increased insulin sensitivity in humans. J. Clin. Invest. 132, e152961. doi: 10.1172/JCI152961
Keywords: tacrolimus, diabetes, gut microbiota, metabolomics, amino acids, bile acids, short chain fatty acids
Citation: Jiang Z, Qian M, Zhen Z, Yang X, Xu C, Zuo L, Jiang J, Zhang W and Hu N (2024) Gut microbiota and metabolomic profile changes play critical roles in tacrolimus-induced diabetes in rats. Front. Cell. Infect. Microbiol. 14:1436477. doi: 10.3389/fcimb.2024.1436477
Received: 22 May 2024; Accepted: 14 August 2024;
Published: 17 September 2024.
Edited by:
Anas Abdel Raman, King Faisal Specialist Hospital and Research Centre, Saudi ArabiaReviewed by:
Refat Nimer, Jordan University of Science and Technology, JordanRajaa Sebaa, Shaqra University, Saudi Arabia
Copyright © 2024 Jiang, Qian, Zhen, Yang, Xu, Zuo, Jiang, Zhang and Hu. This is an open-access article distributed under the terms of the Creative Commons Attribution License (CC BY). The use, distribution or reproduction in other forums is permitted, provided the original author(s) and the copyright owner(s) are credited and that the original publication in this journal is cited, in accordance with accepted academic practice. No use, distribution or reproduction is permitted which does not comply with these terms.
*Correspondence: Wenting Zhang, d2VudGluZ196aGFuZ0BudHUuZWR1LmNu; Nan Hu, aG5fMzI0QDE2My5jb20=