- 1Department of Medical Laboratory Diagnostics – Fahrenheit Biobank BBMRI.pl, Medical University of Gdansk, Gdansk, Poland
- 2Scientific Circle of Studies Regarding Personalized Medicine Associated with Department of Medical Laboratory Diagnostics, Medical University of Gdansk, Gdansk, Poland
- 3Sanprobi Sp. z o. o., Szczecin, Poland
- 4Department of Oncology and Radiotherapy, Medical University of Gdansk, Gdansk, Poland
- 5Unit of Surgery with Unit of Surgery with Unit of Oncological Surgery, Specialist Hospital in Koscierzyna, Koscierzyna, Poland
- 6Academy of Medical and Social Applied Sciences, Elbląg, Poland
- 7Department of Surgical Oncology, Medical University of Gdansk, Gdansk, Poland
- 8Department of Gastrointestinal Surgical Oncology, Greater Poland Cancer Centre, Poznan, Poland
- 9Department of Human Nutrition and Metabolomics, Pomeranian Medical University in Szczecin, Szczecin, Poland
- 10Department of Biochemical Research, Pomeranian Medical University in Szczecin, Szczecin, Poland
- 11BioTechMed Centre/Department of Mechanics of Materials and Structures, Gdansk University of Technology, Gdansk, Poland
Introduction: Recent years, microbiota-associated aspects have been analysed in multiple disorders regarding cancers. Existing evidence pints that gut microorganisms might take part in tumour origin and therapy efficacy. Nevertheless, to date, data on faecal metabolomics in cancer patients is still strongly limited. Therefore, we aimed to analyse gut untargeted metabolome in gastrointestinal cancer patients (i.e., gastric and colorectal cancer).
Patients and methods: There were 12 patients with either gastric (n=4) or colorectal cancer (n=8) enrolled and 8 analysed (n=4 each). Stool samples were collected prior to anti-cancer treatments. Untargeted metabolomics analyses were conducted by means of mass spectrometry.
Results: A plethora of metabolites in cancer patients we analysed were noted, with higher homogenity in case of gastric cancer patients. We found that the level of Deoxyguanosine,m/z 266.091,[M-H]-, Uridine,m/z 245.075,[M+H]+, Deoxyguanosine,m/z 268.104,[M]+, 3-Indoleacetic acid,m/z 176.07,[M+H]+, Indoxyl,m/z 132.031,[M-H]-, L-Phenylalanine,m/z 164.073,[M-H]-, L-Methionine,m/z 150.058,[M+NH4]+, was significantly higher in colorectal cancer patients and Ethyl hydrogen malonate,m/z 133.031,[M+H]+ in gastric cancer.
Conclusion: The overall insights into untargeted metabolomics showed that most often higher levels of analysed metabolites were detected in colorectal cancer patients compared to gastric cancer patients. The link between gut metabolome and both local and distal metastasis might exist, however it requires confirmation in further multi-centre studies regarding larger sample size.
1 Introduction
Microbiome and metabolome-related aspects have become objects of interest in oncology (Kaźmierczak-Siedlecka et al., 2023). The reasons are as follows: [1] Currently, it is known that some microbes are involved in development of tumour by creating dysbiotic environment and activating biochemical pathways (Rajagopala et al., 2017). There are therapeutic methods (such as prebiotics, probiotics, synbiotics, postbiotics, next-generation probiotics) which modify the composition of gut microbiome and the activity of microorganisms through for instance affecting production of metabolites and consequently leading to eubiosis restoration. However, it is still under investigation, and it requires further analysis to strengthen the possibility of usage. [2] According to some data, there is a bidirectional link between gut microbiome and drugs (also anti-cancer agents). These interactions are described as pharmacomicrobiomics (Ting et al., 2022). Basis on this bidirectional communications may provide personalized and more effective anti-cancer management. [3] Microbiome profile and metabolomic signature may be considered as biomarkers (Wong and Yu, 2023), which can select subjects with higher risk of tumour development or to detect cancer in early stages. Therefore, it seems that there can be found many benefits from routinely analysis of gut microbiome in cancer patients and include it to screening program.
In contrast to targeted metabolomics, untargeted metabolomics is characterized by wide range of discovery, mainly hypothesis generating, comprehensive analysis, qualitative identifications and relative quantitation of small molecules in sample (Schrimpe-Rutledge et al., 2016). In the level of metabolomics, small molecules are characterized from many types of samples, such as stool, urine, serum, cell extracts, and others. Considering metabolomics it should be emphasized that there are different methods of both separation and detection. Notably, it seems that metabolomics analysis based on mass spectrometry is one of the most significant technology allowing to detect and identify small molecules which are produced by gut microbiota (Bauermeister et al., 2022).
As it was previously mentioned, the imbalance of gut microbiota composition and changes of microbiota-derived metabolites are observed in gastrointestinal cancer patients (Kaźmierczak-Siedlecka et al., 2023; Ohigashi et al., 2013; Tong et al., 2021; Yang et al., 2022; Dai et al., 2021). Recently, in Kaźmierczak-Siedlecka et al. study it was shown that microbiota-derived metabolites based on the proportion between acetate, proprionate, and butyrate is changed in colorectal cancer patients in preoperative period (Kaźmierczak-Siedlecka et al., 2023). Untargeted metabolomics seems to be extremely significant in oncology due to the fact that it allows to collect data without pre-existing knowledge (Schrimpe-Rutledge et al., 2016). It is noteworthy that anti-cancer treatment (such as surgery, chemotherapy, radiotherapy) affects gut microbiome and metabolome-related aspects and vice-versa. Therefore, the aim of this study was to analyse untargeted metabolomics in patients with gastrointestinal cancers (i.e. gastric cancer and colorectal cancer) prior to the introduction of anti-cancer treatment. It allows to obtain more precise data without the potential influence of above mentioned treatment. Moreover, the comparison of untargeted metabolomics in case of gastric and colorectal cancer has been investigated.
2 Patients and methods
Participants (n=12) were recruited in Department of Surgical Oncology (Medical University of Gdansk) and Unit of Surgery with Unit of Surgery with Unit of Oncological Surgery, Specialist Hospital in Koscierzyna, Poland. Inclusion criteria were age ≥18 yr., patients with diagnosed gastric/colorectal cancer prior to the introduction of anti-cancer treatment, written consent to take part in this study. Exclusion criteria included age <18 yr., patients with gastric/colorectal cancer who were under anti-cancer treatment. The stool samples (at least 4 g) were collected after confirming of diagnosis and before introduction of anti-cancer treatment. The stool samples were taken by own patients, placed in sterile tube, and then provided to researchers as soon as possible. Next, they were stored in -80°C in the Fahrenheit Biobank BBMRI.pl, Medical University of Gdansk, until conduction of untargeted metabolomics analysis according to the well-established protocol at Sanprobi Sp. z o. o. The study protocol has been approved by the Independent Bioethics Committee for Scientific Research at the Medical University of Gdansk (identifiers: NKBBN/129/2021, NKBBN/428/2022, KB/428-314/2023).
2.1 Preparation of material for analysis
Briefly, 500 µl of a mixture of methanol, water and acetonitrile in the proportions of 50:25:25 v/v/v with the addition of deuterated internal standards was added to 60 mg of feces. Then, the samples were shaken at 2000 rpm at 4°C for 30 min. to dissolve the metabolites in the solution and precipitate the proteins. In the next step, the samples were centrifuged for 4 minutes at a speed of 4000 rpm and at a temperature of 4°C. After the samples were centrifuged, the supernatant was decanted to the chromatography tubes through a 0.22 μm syringe filter. The samples were subsequently analysed on the same day by a liquid chromatography–mass spectrometry. QC samples were prepared by mixing test samples in equal proportions and prepared in the same way as the test samples.
2.2 Liquid chromatography-mass spectrometry analysis
The analysis was carried out on an ExionLC liquid chromatograph equipped with a binary pump, autosampler, and column thermostat coupled with a Triple TOF 6600+ mass spectrometer (Sciex, Framingham, MA, USA). The separation was carried out on a Phenomenex Luna® Omega 1.6μm polar C18 150 x 2.1mm column for 45 min in gradient separation. The mobile phases were: Phase A – Water with 10mM ammonium acetate, Phase B - acetonitrile with 0.1% formic acid. The column injection was 2μl and the column temperature was 20°C. The phase flow was 0.2 ml/min. Spectral analysis was performed in the positive ion mode with a capillary voltage of 5500 V, Curtain gas (CUR) was 25 psi, Ion source gas 1 (GS1) 45 psi, Ion source gas 2 (GS2) 60 psi and the ion source temperature was 400°C and the mode negative ions at a capillary voltage of 4500 V, Curtain gas (CUR) was 25 psi, Ion source gas 1 (GS1) 45 psi, Ion source gas 2 (GS2) 60 psi and the ion source temperature was 350°C. Spectrometer collected spectral data in SWATH mode.
2.3 Analysis of the results and statistical analysis
The obtained spectral spectra were analysed and matched to reference spectra contained in the SCIEX All-In-One HR-MS/MS, NIST and own databases using SCIEX OS software. In the next step, based on the results obtained and the identification of metabolites present in the tested samples, a file was created in Microsoft Excel 2019 PL (Poland) for statistical analysis and data visualization on the Metaboanalyst platform (https://www.metaboanalyst.ca/). The t-test and fold change >2 were used to determine differences between the study groups. The statistical analysis was conducted using above mentioned Microsoft Excel 2019 PL (Poland) and STATISTICA version 13.0.
3 Results
This study included 12 patients (n=8 – colorectal cancer, n=4 – gastric cancer). The basic characteristics of these participants is as follows: the median age – 61.78 ± 11.50 years, the median Body Mass Index (BMI) – 29 ± 1.41 kg/m2, the most commonly co-existing disease – hypertension. Among these patients, 4 were excluded due to incomplete data regarding tumour characteristics. Therefore, the analysis is based on 2 groups: first including gastric cancer patients (n=4) and second regarding colorectal cancer patients (n=4) (Table 1).
The analysis of stool samples revealed the occurrence of wide range of metabolites in gastric and colorectal cancer patients (Table 2).
The metabolic profile of analysed stool samples varies, especially in case of colorectal cancer patients (Figure 1). These differences can be caused by variability of either types of tumours or tumours anatomical localisation. There is higher grouping in case of gastric cancer, which confirms more homogeneous metabolic profile comparing to the analysed group of colorectal cancer. Moreover, in Figure 1 there are subgroups (in gastric cancer) created by Sample_1G and Sample_3G, Sample_4G and Sample_N2G, which show similar characteristics in these subgroups.
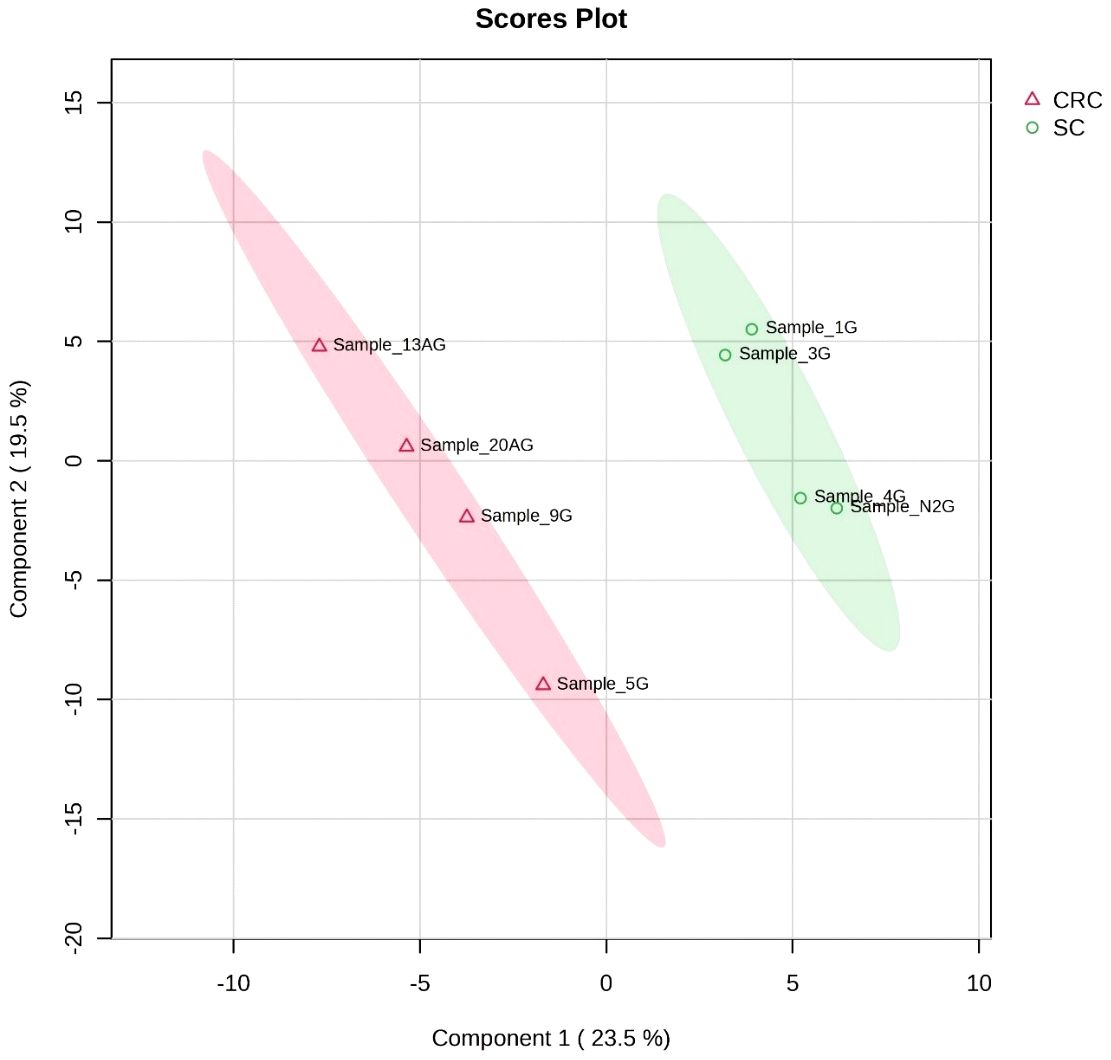
Figure 1 Figure PLS_DA – the comparing analysed groups – gastric cancer [SC] and colorectal cancer [CRC]. SC, stomach cancer; CRC, colorectal cancer.
The occurrence of metabolites, which varied in both analysed groups, is presented in Figure 2. The metabolites, which significantly varied colorectal cancer and gastric cancer are placed in Figure 2 with blue and red colours and next they are precisely analysed and presented in Figure 3.
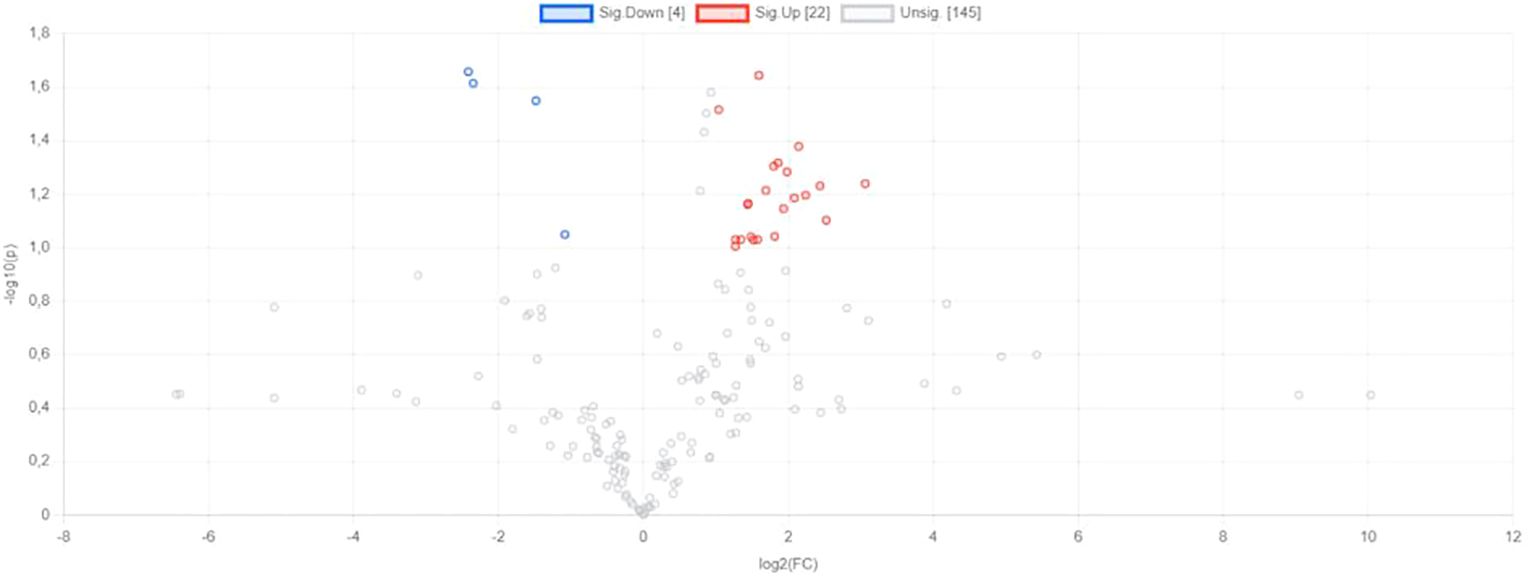
Figure 2 Volcano plot presenting metabolites varied groups of gastric cancer [SC] and colorectal cancer [CRC]. Significance parameters: p<0.1; FC > 2.
The comparison of the levels of particular metabolites detected in colorectal cancer patients and gastric cancer has been presented in Figure 3. Considering 25 metabolites (Figure 3), it is observed that higher level of them are mostly noted in colorectal cancer patients compared to the gastric cancer (21 metabolites vs. 4 metabolites, respectively). For instance, the levels of L- Leucine, L- tryptophan, L-Phenylalanine are higher in colorectal cancer than in gastric cancer. Moreover, considering extremely precise statistical condition, the statistically significant difference (p<0.05 and FC – 2) between analysed groups were found in case of Deoxyguanosine,m/z 266.091,[M-H]-, Uridine,m/z 245.075,[M+H]+, Deoxyguanosine,m/z 268.104,[M]+, 3-Indoleacetic acid,m/z 176.07,[M+H]+, Indoxyl,m/z 132.031,[M-H]-, L-Phenylalanine,m/z 164.073,[M-H]-, L-Methionine,m/z 150.058,[M+NH4]+, Ethyl hydrogen malonate,m/z 133.031,[M+H]+.
4 Discussion
Molecular diagnosis of cancer based on metabolomics can be promising in near future (Cheung et al., 2019). Metabolomic data may be used as biomarkers allowing to detect several cancers, such as oesophageal, gastric, pancreatic, bladder, lung, thyroid, and others (Wang et al., 2022; Yang et al., 2022). Different metabolites/metabolic pathways/metabolism may provide a signature which is specific for diseases/conditions. For instance, in a study by Yang et al., it was noted that glycophospholipid metabolism is related to both tumorigenesis and progression of oesophageal squamous cell carcinoma (ESCC) and that may be therapeutic target in ESCC progression (Yang et al., 2022). Hang et al. reported that untargeted plasma metabolomics can serve as a potential risk prediction of hepatocellular carcinoma (Hang et al., 2022). The aspects of untargeted metabolomics can be also useful in case of other digestive cancers. Plasma metabolomic signatures in precancerous gastric lesions progressing to cancer were identified in a study by Huang et al. (2021). Notably, six plasma metabolites were related to the both overall risk of gastric cancer and early gastric cancer whereas three of these metabolites, such as α-linolenic acid, linoleic acid, palmitic acid were associated with the prediction of risk of gastric lesion progression and early gastric cancer. In another study untargeted metabolome was also analysed in case of gastric cancer (Yu et al., 2021). Serum samples were taken from patients with chronic gastritis/gastric cancer. It was shown that lipid metabolism may affect the development of chronic gastritis to gastric cancer; moreover, hexadecasphinganine, linoleamide, and N-Hydroxy arachidonoyl amine were assessed as diagnostic markers for both chronic gastritis and gastric cancer (Yu et al., 2021). In the current study, we also investigated gut metabolome in cancer patients, but from stool samples. The overall insights showed that higher level of analysed metabolites was mostly noted in colorectal cancer patients compared to gastric cancer patients. For instance, in case of indole-3-acetic acid and tryptophan, the levels are higher in colorectal cancer than in gastric cancer. Indole-3-acetic acid is a tryptophan metabolite produced by gut microbiota according to the following pathway in intestinal epithelial cells: (1) ingested dietary protein, (2) tryptophan, (3) intestinal microbiota, (4) indole-3-acetic acid (Seo and Wargo, 2023; Tomii et al., 2023). This result can be associated with different overall characteristics of gut microbiota in particular types of cancer, i.e. gastric and colorectal cancer. In the current study, it was observed that the level of L-phenylalanine was also higher in colorectal cancer compared to gastric cancer. In previously published data it was reported that some amino-acids including phenylalanine may be considered as a biomarkers in colorectal cancer patients (Hashim et al., 2019). Recently, Chen et al. (2022) presented that gut microbiome-associated serum metabolites can be used to detect colorectal cancer (Chen et al., 2022).
In the current study, it was observed higher grouping in case of gastric cancer in comparison to colorectal cancer, which confirms more homogeneous metabolic profile in gastric cancer patients. Moreover, on Scores Plot analysis there were created two subgroups in case of gastric cancer, such as Sample_1G and Sample_3G, Sample_4G and Sample_N2G. It may suggest similar characteristics in these two subgroups. Notably, the first subgroup created by Sample_1G and Sample_3G regards gastric cancer with similar TNM assessment (i.e. Sample_1G: T3N0M0, Sample_3G: T3N1M0). TNM tool is used to assess as follows: T – tumour, N – nodes (involvement of lymph nodes), and M – metastasis (Piñeros et al., 2019). The link between untargeted metabolomics in gastric cancer and both local and distal metastasis may exist, however it requires confirmation with larger sample size. In the current study, it was demonstrated that the metabolic profile of colorectal cancer patients is varied. It can be associated with different localisation of tumours – there are four cases analysed, i.e. sigmoid-rectal cancer, sigmoid colon cancer, rectal cancer, and ascending colon cancer.
5 Limitations and future directions
This study has been some limitations. First of all, the study was conducted with relatively small sample size. However, it is treated as preliminary results to present basic characteristics of untargeted metabolomics in gastric and colorectal cancer patients as well as to find the directions prior to the next study (KB/428-526/2023, Medical University of Gdansk, Gdansk, Poland) in which we analyse the impact of anti-cancer treatment regarding chemotherapy and radiotherapy on untargeted metabolomics aspects. This project is currently ongoing in cooperation with multi-disciplinary team of both oncologists and oncological surgeons. It is also recommended to investigate untargeted metabolomics among patients with similar both stage and grade of the cancers, nevertheless it might be challenging to collect stool samples with larger sample size. However, it would provide promising strategy to be included in clinical aspects. Metabolomics-based biomarkers might provide earlier detection of cancers allowing to complete resection of tumour. Moreover, metabolomics-related techniques can be attractive due to the fact that they are non-invasive and relatively low cost.
6 Conclusions
The aspect of untargeted metabolomics is a new area, which can be considered in oncology. Notably, the results presented in the current study were obtained prior to the introduction of anti-cancer management, such as surgical treatment. The overall insights into untargeted metabolomics showed that most often higher levels of analysed metabolites were detected in colorectal cancer patients compared to gastric cancer patients. It can be related to the different activity of gut microbiome in particular types of gastrointestinal cancer. Additionally, it was observed a higher grouping in case of gastric cancer comparing to colorectal cancer, which confirms more homogeneous metabolic profile in this cancer. The link between untargeted metabolomics in gastric cancer and both local and distal metastasis may exist, but it requires confirmation in further multi-centre studies regarding larger sample size.
Data availability statement
The mass spectrometry proteomics data have been deposited to the ProteomeXchange Consortium via the PRIDE partner repository with the dataset identifier PXD051921.
Ethics statement
The studies involving humans were approved by Independent Bioethics Committee for Scientific Research at the Medical University of Gdansk. The studies were conducted in accordance with the local legislation and institutional requirements. The participants provided their written informed consent to participate in this study.
Author contributions
KK-S: Writing – review & editing, Writing – original draft, Resources, Project administration, Methodology, Investigation, Funding acquisition, Formal analysis, Conceptualization. DM: Writing – original draft. DS: Writing – review & editing, Software, Formal analysis. JM: Writing – review & editing. BS: Writing – review & editing, Data curation. PU: Writing – review & editing, Data curation. KP: Writing – review & editing. ES: Writing – review & editing, Supervision. KS-Z: Writing – review & editing, Supervision. LK: Writing – review & editing, Supervision, Conceptualization.
Funding
The author(s) declare financial support was received for the research, authorship, and/or publication of this article. This study has been funded by MTN grant from the Medical University of Gdansk, Poland (KK-S; grant no: 71-01213), and from the Ministry of Science and Higher Education, Poland (grant no. 2/566516/SPUB/SP/2023).
Conflict of interest
The authors declare that the research was conducted in the absence of any commercial or financial relationships that could be construed as a potential conflict of interest.
Publisher’s note
All claims expressed in this article are solely those of the authors and do not necessarily represent those of their affiliated organizations, or those of the publisher, the editors and the reviewers. Any product that may be evaluated in this article, or claim that may be made by its manufacturer, is not guaranteed or endorsed by the publisher.
References
Bauermeister, A., Mannochio-Russo, H., Costa-Lotufo, L. V., Jarmusch, A. K., Dorrestein, P. C. (2022). Mass spectrometry-based metabolomics in microbiome investigations. Nat. Rev. Microbiol. 20, 143–160. doi: 10.1038/s41579-021-00621-9
Chen, F., Xudong, D., Chang-Chun, Z., Ke-Xin, L., Yu-Juan, Z., Xiao-Ying, L., et al. (2022). Integrated analysis of the faecal metagenome and serum metabolome reveals the role of gut microbiome-associated metabolites in the detection of colorectal cancer and adenoma. Gut 71, 1315–1325. doi: 10.1136/gutjnl-2020-323476
Cheung, P. K., Man Hin, M., Hing Fung, T., Ka Fai, Y., Hin Fung, T., Man Kee, M. C., et al. (2019). The applications of metabolomics in the molecular diagnostics of cancer. Expert Rev. Mol. Diagnostics 19, 785–793. doi: 10.1080/14737159.2019.1656530
Dai, D., Yang, Y., Yu, J., Dang, T., Qin, W., Teng, L., et al. (2021). Interactions between gastric microbiota and metabolites in gastric cancer. Cell Death Dis. 12 (12), 1104. doi: 10.1038/s41419-021-04396-y
Hang, D., Xiaolin, Y., JiaYi, L., Chong, S., Juncheng, D., Xiangfeng, L., et al. (2022). Untargeted plasma metabolomics for risk prediction of hepatocellular carcinoma: A prospective study in two chinese cohorts. Int. J. Cancer 151, 2144–2154. doi: 10.1002/ijc.34229
Hashim, N. A. A., Ab-Rahim, S., Suddin, L. S., Saman, M. S. A., Mazlan, M. (2019). Global serum metabolomics profiling of colorectal cancer. Mol. Clin. Oncol. 11, 3–14. doi: 10.3892/mco.2019.1853
Huang, S., Guo, Y., Li, Z-W., Shui, G., Tian, H., Li, B-W., et al. (2021). Identification and validation of plasma metabolomic signatures in precancerous gastric lesions that progress to cancer. JAMA Netw. Open 4 (6), e2114186. doi: 10.1001/jamanetworkopen.2021.14186
Kaźmierczak-Siedlecka, K., Skonieczna-Żydecka, K., Palma, J., Sobocki, B., Świerblewski, M., Siedlecka-Kroplewska, K., et al. (2023). Microbiota-derived metabolites in colorectal cancer patients in preoperative period. Eur. Rev. Med. Pharmacol. Sci. 27, 1443–1449. doi: 10.26355/eurrev_202302_31384
Ohigashi, S., Sudo, K., Kobayashi, D., Takahashi, O., Takahashi, T., Asahara, T., et al. (2013). Changes of the intestinal microbiota, short chain fatty acids, and fecal PH in patients with colorectal cancer. Dig. Dis. Sci. 58 (6), 1717–1726. doi: 10.1007/s10620-012-2526-4
Piñeros, M., Parkin, D. M., Ward, K., Chokunonga, E., Ervik, M., Farrugia, H., et al. (2019). Essential TNM: A registry tool to reduce gaps in cancer staging information. The lancet. Oncology 20, e103–e111. doi: 10.1016/S1470-2045(18)30897-0
Rajagopala, S. V., Vashee, S., Oldfield, L. M., Suzuki, Y., Venter, J. C., Telenti, A., et al. (2017). The human microbiome and cancer. Cancer Prev. Res. 10, 226–234. doi: 10.1158/1940-6207.CAPR-16-0249
Schrimpe-Rutledge, A. C., Codreanu, S. G., Sherrod, S. D., McLean, J. A. (2016). Untargeted metabolomics strategies-challenges and emerging directions. J. Am. Soc. Mass Spectrometry 27, 1897–1905. doi: 10.1007/s13361-016-1469-y
Seo, Y. D., Wargo, J. A. (2023). From bugs to drugs: bacterial 3-IAA enhances efficacy of chemotherapy in pancreatic cancer. Cell Rep. Med. 4, 101039. doi: 10.1016/j.xcrm.2023.101039
Ting, N. L. N., Lau, H. C. H., Yu, J. (2022). Cancer pharmacomicrobiomics: targeting microbiota to optimise cancer therapy outcomes. Gut 71, 1412–1425. doi: 10.1136/gutjnl-2021-326264
Tomii, A., Higa, M., Naito, K., Kurata, K., Kobayashi, J., Takei, C., et al. (2023). Activation of the TLR4-JNK but not the TLR4-ERK pathway induced by indole-3-acetic acid exerts anti-proliferative effects on caco-2 cells. Bioscience Biotechnology Biochem. 87, 839–849. doi: 10.1093/bbb/zbad055
Tong, Y., Gao, H., Qi, Q., Liu, X., Li, J., Gao, J., et al. (2021). High fat diet, gut microbiome and gastrointestinal cancer. Theranostics 11, 5889–5910. doi: 10.7150/thno.56157
Wang, S., Li, M., Yan, L., He, M., Lin, H., Xu, Y., et al. (2022). Metabolomics study reveals systematic metabolic dysregulation and early detection markers associated with incident pancreatic cancer. Int. J. Cancer 150, 1091–1100. doi: 10.1002/ijc.33877
Wong, C. C., Yu, J. (2023). Gut microbiota in colorectal cancer development and therapy. Nat. Rev. Clin. Oncol. 20, 429–452. doi: 10.1038/s41571-023-00766-x
Yang, T., Hui, R., Nouws, J., Sauler, M., Zeng, T., Wu, Q. (2022). Untargeted metabolomics analysis of esophageal squamous cell cancer progression. J. Trans. Med. 20, 127. doi: 10.1186/s12967-022-03311-z
Yang, Y., Dai, D., Jin, W., Huang, Y., Zhang, Y., Chen, Y., et al. (2022). Microbiota and metabolites alterations in proximal and distal gastric cancer patients. J. Trans. Med. 20, 439. doi: 10.1186/s12967-022-03650-x
Keywords: colorectal cancer, gastric cancer, gut microbiome, microbiota-derived metabolites, untargeted metabolomics
Citation: Kaźmierczak-Siedlecka K, Muszyński D, Styburski D, Makarewicz J, Sobocki BK, Ulasiński P, Połom K, Stachowska E, Skonieczna-Żydecka K and Kalinowski L (2024) Untargeted metabolomics in gastric and colorectal cancer patients – preliminary results. Front. Cell. Infect. Microbiol. 14:1394038. doi: 10.3389/fcimb.2024.1394038
Received: 29 February 2024; Accepted: 08 April 2024;
Published: 07 May 2024.
Edited by:
Amedeo Amedei, University of Florence, ItalyReviewed by:
Anna Duda-Madej, Wroclaw Medical University, PolandYotsawat Pomyen, Chulabhorn Research Institute, Thailand
Copyright © 2024 Kaźmierczak-Siedlecka, Muszyński, Styburski, Makarewicz, Sobocki, Ulasiński, Połom, Stachowska, Skonieczna-Żydecka and Kalinowski. This is an open-access article distributed under the terms of the Creative Commons Attribution License (CC BY). The use, distribution or reproduction in other forums is permitted, provided the original author(s) and the copyright owner(s) are credited and that the original publication in this journal is cited, in accordance with accepted academic practice. No use, distribution or reproduction is permitted which does not comply with these terms.
*Correspondence: Karolina Kaźmierczak-Siedlecka, leokadia@gumed.edu.pl; Leszek Kalinowski, leszek.kalinowski@gumed.edu.pl; Ewa Stachowska, ewa.stachowska@pum.edu.pl