- 1Department of Endocrinology and Metabolism, The First Hospital of Jilin University, Changchun, Jilin, China
- 2Department of Gastroenterology, The First Hospital of Jilin University, Changchun, Jilin, China
Background: Numerous studies have demonstrated the influence of gut microbiota on the development of obesity. In this study, we utilized Mendelian randomization (MR) analysis to investigate the gut microbiota characteristics among different types of obese patients, aiming to elucidate the underlying mechanisms and provide novel insights for obesity treatment.
Methods: Two-sample multivariable Mendelian randomization (MR) analysis was employed to assess causal relationships between gut microbiota and various obesity subtypes. Gut microbiota data were obtained from the international consortium MiBioGen, and data on obese individuals were sourced from the Finnish National Biobank FinnGen. Eligible single-nucleotide polymorphisms (SNPs) were selected as instrumental variables. Various analytical methods, including inverse variance weighted (IVW), MR-Egger regression, weighted median, MR-RAPS, and Lasso regression, were applied. Sensitivity analyses for quality control included MR-Egger intercept tests, Cochran’s Q tests, and leave-one-out analyses and others.
Results: Mendelian randomization studies revealed distinct gut microbiota profiles among European populations with different obesity subtypes. Following multivariable MR analysis, we found that Ruminococcaceae UCG010 [Odds Ratio (OR): 0.842, 95% confidence interval (CI): 0.766-0.926, Adjusted P value: 0.028] independently reduced the risk of obesity induced by excessive calorie intake, while Butyricimonas [OR: 4.252, 95% CI: 2.177-8.307, Adjusted P value: 0.002] independently increased the risk of medication-induced obesity. For localized adiposity, Pasteurellaceae [OR: 0.213, 95% CI: 0.115-0.395, Adjusted P value: <0.001] acted as a protective factor. In the case of extreme obesity with alveolar hypoventilation, lactobacillus [OR: 0.724, 95% CI: 0.609-0.860, Adjusted P value: 0.035] reduced the risk of its occurrence. Additionally, six gut microbiota may have potential roles in the onset of different types of obesity. Specifically, the Ruminococcus torques group may increase the risk of its occurrence. Desulfovibrio and Catenabacterium may serve as protective factors in the onset of Drug-induced obesity. Oxalobacteraceae, Actinomycetaceae, and Ruminiclostridium 9, on the other hand, could potentially increase the risk of Drug-induced obesity. No evidence of heterogeneity or horizontal pleiotropy among SNPs was found in the above studies (all P values for Q test and MR-Egger intercept > 0.05).
Conclusion: Gut microbiota abundance is causally related to obesity, with distinct gut microbiota profiles observed among different obesity subtypes. Four bacterial species, including Ruminococcaceae UCG010, Butyricimonas, Pasteurellaceae and lactobacillus independently influence the development of various types of obesity. Probiotic and prebiotic supplementation may represent a novel approach in future obesity management.
1 Introduction
Obesity is a chronic metabolic disorder, and with its global incidence steadily rising, it has become a significant economic and health concern worldwide (Vlassopoulos and Lean, 2016). Obesity is often associated with cardiovascular diseases, diabetes, musculoskeletal disorders (especially osteoarthritis), and cancers (including ovarian, liver, and colon cancers, etc). Consequently, obesity and its related issues have gained increasing attention (Haslam and James, 2005). Obesity is recognized as a multifactorial disorder, with its etiology involving a multitude of factors including genetics, environment, behavior, and psychology. The gut microbiome stands out as a significant environmental determinant contributing to its onset. The human gut microbiota comprises approximately 100 trillion species and carries genes numbering about 150 times that of the human genome (Marchesi et al., 2016). The gut microbiota plays a pivotal role in maintaining normal intestinal function and host health, participating in metabolic homeostasis. Consequently, alterations in the gut microbiota contribute significantly to the development of metabolic disorders. The gut microbiota is primarily influenced by environmental factors, particularly diet, and exhibits variation based on ethnicity, possibly owing to disparities in dietary customs across regions (Deschasaux et al., 2018; Rothschild et al., 2018). While the host genome plays a central role in shaping the composition of the gut microbiota, numerous geographic and environmental factors, including diet, lifestyle, sanitation, and medication use, can lead to variations in the gut microbiota. Research suggests that the impact of genes on the gut microbiota appears to be less pronounced, whereas environmental factors (especially diet) hold greater influence (Rothschild et al., 2018).
In recent years, an increasing body of evidence has substantiated a causal relationship between the gut microbiota and obesity. Bäckhed et al. transplanted the gut microbiota of normal mice into germ-free mice, resulting in a significant increase in body fat percentage even without altering food intake (Backhed et al., 2004). Li et al. observed that the genus Akkermansia exhibited a protective effect against childhood obesity and BMI (Li et al., 2023). There are several potential mechanisms underlying the association between the gut microbiota and obesity: The gut microbial community enhances energy intake from food by producing efficient enzymes for the degradation of dietary nutrients (Blaut, 2015). Dietary fibers, through metabolites such as short-chain fatty acids (SCFAs) produced by the gut microbiota, not only serve as an energy source but also interact with G protein-coupled receptor (GPR) 41 and GPR43 (Lin et al., 2012) to modulate lipid and glucose metabolism. Additionally, AMPK is involved in mediating the effects of SCFAs, contributing not only to the aforementioned metabolic regulations but also to cholesterol metabolism (den Besten et al., 2013); In addition to the aforementioned effects, SCFA can also influence the production of hormones such as leptin, peptide YY (PYY), ghrelin, insulin, and glucagon-like peptide-1 (GLP-1). These hormones all play crucial roles in the development and occurrence of obesity (Fukumoto et al., 2003; Neuman et al., 2015). Furthermore, the gut microbiota can also impact the integrity of the intestinal barrier, modulate plasma lipopolysaccharide (LPS) levels, and further influence the occurrence and progression of metabolic endotoxemia and low-grade inflammation in the body, thereby either promoting or reducing the development of obesity (Tsukumo et al., 2015).
While the fundamental cause of obesity is an excessive calorie intake compared to expenditure, variations in the human gut microbiota ecosystem may be a pivotal factor influencing energy homeostasis. Specifically, individuals prone to obesity might exhibit distinctive alterations in gut microbiota, as opposed to normal or lean populations (Ley et al., 2005). According to the ICD-10 coding (The, 2019), we included localized adiposity, obesity (including calorie-induced obesity, drug-induced obesity, extreme obesity with alveolar hypoventilation) in our study, and analyzed the gut microbiota characteristics of patients with different types of obesity. This study explores the gut microbiota characteristics in various obesity types, aiming to enhance comprehension of obesity pathogenesis and provide insights into novel therapeutic avenues for obese individuals.
2 Methods
2.1 Source of datasets
In this study, we obtained whole-genome association study (GWAS) data on gut microbiota from the International MiBioGen Consortium. The database coordinated 16S rRNA gene sequencing profiles and genetic typing data for 18,340 participants from 24 cohorts across the United States, Canada, Israel, South Korea, Germany, Denmark, the Netherlands, Belgium, Sweden, Finland, and the United Kingdom. This comprehensive meta-analysis of autosomal human genetic variations and their associations with the gut microbiota involved a large-scale, multi-ethnic, whole-genome approach (Kurilshikov et al., 2021). Details of recruitment criteria, sample size, and ethical approval can be found in the Supplementary Note 1, 2, Supplementary Table 9. GWAS datasets on obesity were obtained from the FinnGen research project, which originates from the Finnish National Biobank Network. Participants in FinnGen were recruited from 2017 to 2023. This database encompasses data from 473,681 participants, including 15,045 individuals with obesity due to excessive caloric intake, 240 individuals with drug-induced obesity, 1,064 individuals with extreme obesity associated with alveolar hypoventilation, and 132 individuals with localized adiposity. The control group consisted of varying numbers of individuals, ranging from 355,786 to 355,902 (Kurki et al., 2023). The inclusion and exclusion criteria for participants were determined based on the ICD-10 codes of the primary diagnosis during hospitalization. Pharmaceuticals inducing obesity include Corticosteroids, antihypertensive drugs (β-adrenergic blockers), neuropsychiatric medications (Clozapine, olanzapine, quetiapine), antidepressants (amitriptyline, nortriptyline, and mirtazapine), and antiepileptic drugs (valproate and carbamazepine). Specific disease codes are provided in Table 1. Participants in FinnGen provided informed consent for biobank research on basis of the Finnish Biobank Act. The Coordinating Ethics Committee of the Hospital District of Helsinki and Uusimaa (HUS) approved the FinnGen study protocol (number HUS/990/2017). Due to variations in race and population stratification, the exposure and outcome databases were derived from European populations. The study population includes both males and females, mitigating potential biases arising from population stratification (Emdin et al., 2017).
2.2 Study approach
In this study, a two-sample Mendelian randomization (MR) design was employed to investigate the causal effects of gut microbiota on various types of obesity (Figure 1). Genetic variants associated with gut microbiota were selected as instrumental variables (IVs). Multiple analytical methods, including inverse variance-weighted (IVW), MR-Egger regression, weighted median, and MR-RAPS, were utilized. Additionally, sensitivity analyses were conducted using MR-Egger intercept test, Cochran’s Q test, and leave-one-out analysis for quality control purposes. A MR analysis must adhere to the following three assumptions: 1) the relevance assumption: there is a strong association between instrumental variables and exposure factors; 2) the independence assumption: instrumental variables must be independent of confounding factors influencing both exposure and outcomes; 3) the exclusion assumption: instrumental variables should not directly impact the outcome variable.
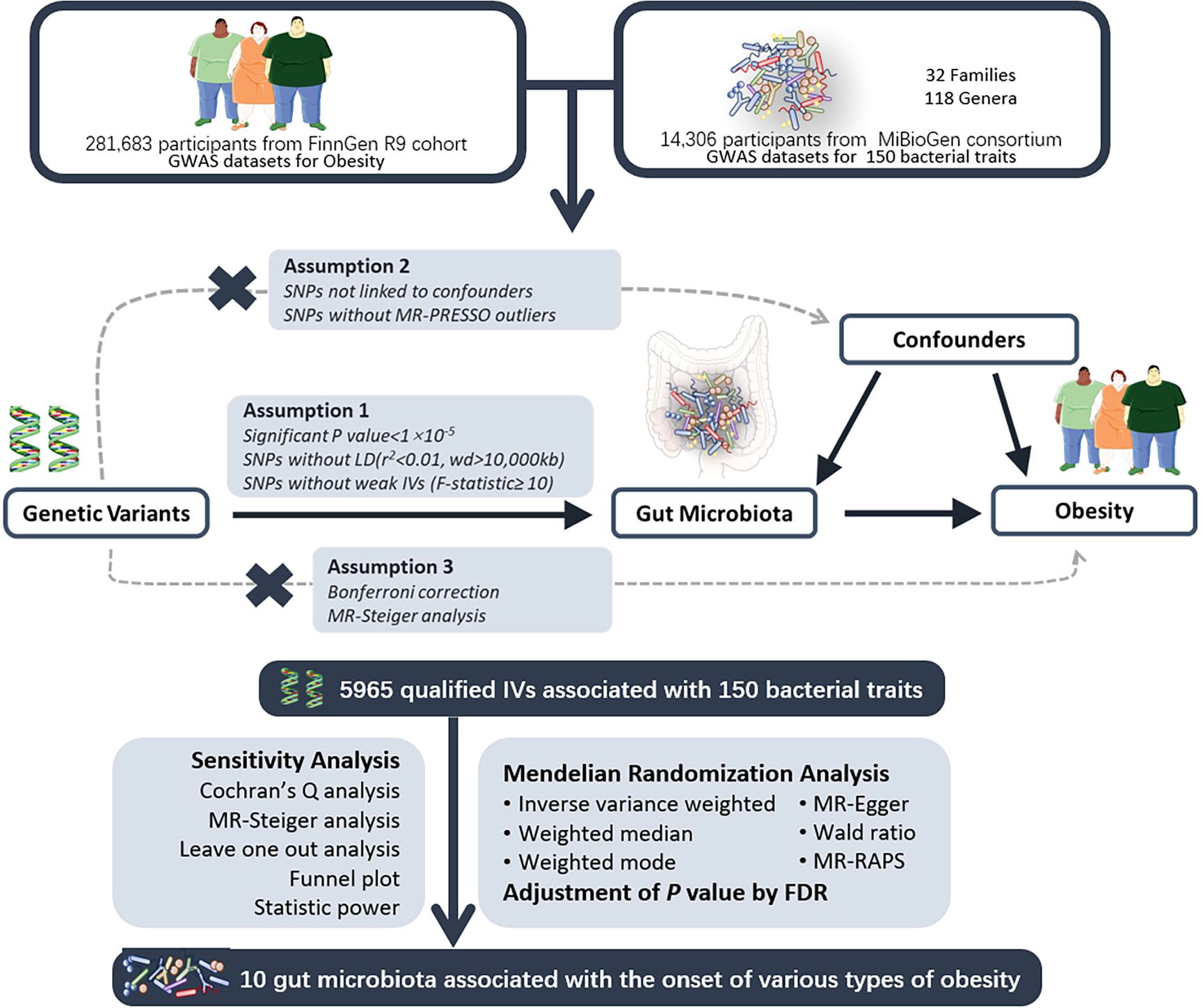
Figure 1 The study design of the MR study of the associations of gut microbiota on obesity. UV, univariable; MV, multivariable; MR, Mendelian Randomization; GWAS, Genome-Wide Association Study; SNP, Single Nucleotide Polymorphism, used as instrumental variables for exposure and outcome; LD, Linkage Disequilibrium; MR-PRESSO, Mendelian Randomization Pleiotropy RESidual Sum and Outlier; FDR, False Discovery Rate. Parts of the figure were drawn by using pictures from Servier Medical Art.
2.3 Instrumental variable selection
In line with the study design and assumptions described above, our initial step involved extracting SNPs significantly correlated with both exposure and outcomes using a p-value threshold of p<1×10-5. A linkage disequilibrium (LD) threshold (r2<0.01, kb=0000) was applied to ensure SNP independence by eliminating linkage disequilibrium. Subsequently, we calculated the F-value for each SNP as an indicator of strength, and SNPs with F<10 were deemed weak instrumental variables and removed (Burgess et al., 2017). During the process of outcome data extraction, any missing SNPs in the outcome database were replaced with highly correlated proxy SNPs. Additionally, during dataset matching, this study excluded ambiguous and palindromic SNPs. Each SNP was examined using the PhenoScanner database (Staley et al., 2016), and SNPs associated with distinct confounding factors were excluded based on different outcomes (as shown in Table 1). MR-PRESSO was utilized to detect and eliminate SNPs with levels of horizontal pleiotropy outliers. MR-Steiger analysis was conducted on all SNPs to verify the direction of causal estimates, and SNPs with erroneous directions were removed according to the method described by (Li et al., 2022). Subsequently, a Bonferroni correction (P<0.05/n, where n represents the number of remaining SNPs) was applied to remove SNPs directly correlated with outcomes. Following this stringent selection process, the SNPs that remained were deemed as qualified instrumental variables.
2.4 Univariable MR analysis
We employed a variety of MR methods to assess the causal relationship between gut microbiota and obesity, including Inverse Variance Weighting (IVW), MR-Egger regression, weighted median, weighted mode, and MR-PAPS method.
The IVW method is a weighted linear regression without an intercept term that uses the reciprocal of the variance of the genetic associations’ product with the outcome as weights (Burgess et al., 2013). Although some have suggested weighted methods with second-order weights, in practice, utilizing first-order standard errors is often reasonable (Burgess et al., 2016). The IVW method is divided into fixed-effects (FE) and multiplicative random-effects (MRE) models based on the consistency of SNP effect differences. In contrast to the IVW method, MR-Egger includes an intercept term that allows for detection of SNP-level pleiotropy (Burgess and Thompson, 2017).
Although the aforementioned methods are commonly used in MR analysis, the weighted average of ratio estimates will differ from zero if the ratio estimate of a single variant is non-zero, leading to a “0% breakdown point” inferences issue where a single incorrect data point can cause estimates to exhibit arbitrary large bias. To address the “0% breakdown point” issue, we also employed two consensus methods. We considered the weighted median method with a 50% breakdown point (based on the majority valid assumption) and the weighted mode method with a higher breakdown point (based on the plurality valid assumption) (Bowden et al., 2016a; Burgess et al., 2019).
Finally, we also employed a common modeling approach, the MR-Robust Adjusted Profile Score (RAPS) method. This method directly models pleiotropic effects of genetic variants using a random-effects distribution, which is likely to perform well when pleiotropic effects are truly normally distributed about zero. To mitigate the increased statistical Type I error rate due to multiple testing, we applied the False Discovery Rate (FDR) method to correct the results, and an adjusted p-value <0.05 was considered a significant causal effect.
2.5 Multivariable MR analysis
Building upon the results of univariable MR analysis, we conducted multivariable MR (MVMR) analysis using the same parameters with various methods.Multivariable MR is an extension of the standard univariable MR, incorporating instrumental variables related to one or more gut microbiota and enabling the estimation of direct causal effects of each microbiota in a single analysis (Burgess and Thompson, 2015; Sanderson et al., 2019). Additionally, all included SNPs must be independent of each other. In this study, multivariable analyses were performed using the IVW method, MR-Egger method, Weighted median method, and Lasso method to elucidate gut microbiota with independent causal effects.
2.6 Sensitivity analysis
Cochran’s Q test was employed to assess heterogeneity among IVs, considering SNPs with Q test P-value < 0.05 as heterogeneous; MR-Egger intercept was computed to determine the presence of pleiotropy among SNPs, and an intercept significance P-value < 0.05 indicates horizontal pleiotropy. Given the adoption of various MR analysis methods, in the absence of heterogeneity and pleiotropy among SNPs, we considered the results from the IVW-random effects model as the primary results; in the presence of heterogeneity, we integrated results from the IVW-random effects model and the weighted median method; in the presence of pleiotropy, we regarded results from the MR-Egger method as the primary results (Burgess et al., 2019). Additionally, we employed the MR-Steiger model to validate the estimated overall direction for result robustness; to ascertain the presence of influential SNPs, we conducted a leave-one-out sensitivity test. The MR-Egger regression requires fulfillment of the Instrument Strength Independent of Direct Effect (InSIDE) assumption (Burgess and Thompson, 2017) and the No Measurement Error (NoME) assumption (Bowden et al., 2016b). We generated a funnel plot and calculated the I2 statistic to confirm the validity of these assumptions. Correction for causal estimates is necessary when I2 < 90%, and MR-Egger is the primary analytical method (Bowden et al., 2016b).
2.7 Visualization of results
We created a heatmap for the overall MR results and depicted significant MR findings using forest plots. Additionally, for each set of MR analyses, scatter plots and regression curve plots were generated, along with forest plots illustrating the effects of individual SNPs. These visualizations will be presented in the Supplementary Materials.
2.8 Statistical analysis software
All statistical analyses and visualizations in this study were conducted using R software (version 4.1.2) with the “TwoSampleMR,” “MR-PRESSO,” “mr.raps,” and “forestploter” packages, as well as several foundational R packages.
3 Results
3.1 Instrument variable selection
Initially, we screened a total of 7671 SNPs associated with gut microbiota. No weak instruments with F<10 were identified. Among them, 388 SNPs were excluded due to missing data in the outcome database, 1716 SNPs were removed as ambiguous or palindromic SNPs during dataset integration, and 99 SNPs were deleted after PhenoScanner retrieval revealed associations with confounding factors. MR-PRESSO testing identified 25 SNPs with horizontal pleiotropy. MR-Steiger analysis did not reveal any SNPs with incorrect causal directions. After Bonferroni correction, 18 SNPs directly related to the outcome were removed. In the end, 5965 qualified SNPs were included in the study.
3.2 Mendelian randomization analysis
In this study, MR analysis was conducted for a total of 750 batches, and detailed results for all methods can be found in Supplementary Table 2. A total of 60 batches exhibited positive results in the MR analysis. However, after FDR correction, only 10 batches showed significant causal relationships (adjusted P-value < 0.05). We created a heatmap with red indicating significant findings (Figure 2) and a forest plot based on the main methods in the significant MR results (Figure 3). We conducted a comprehensive sensitivity analysis on all MR results. The results of the Q-test are provided in Supplementary Table 6, indicating heterogeneity in 13 batches. After comparing the results of IVW (MRE) and Weighted median methods for these batches, we found consistent conclusions between the two methods. The MR-Egger intercept test results can be found in Supplementary Table 7, indicating horizontal pleiotropy in the analysis of 7 batches. Therefore, we employed the MR-Egger method as the primary analytical approach for these 7 batches without the need for causal correction (I2 > 90%). The remaining batches were analyzed using the IVW (MRE) method as the primary approach. This study did not identify SNPs that strongly influenced the significant results. Leave-one-out analysis results are available in Supplementary Figure 3. The MR-Steiger test did not identify overall directional errors in the analysis results.
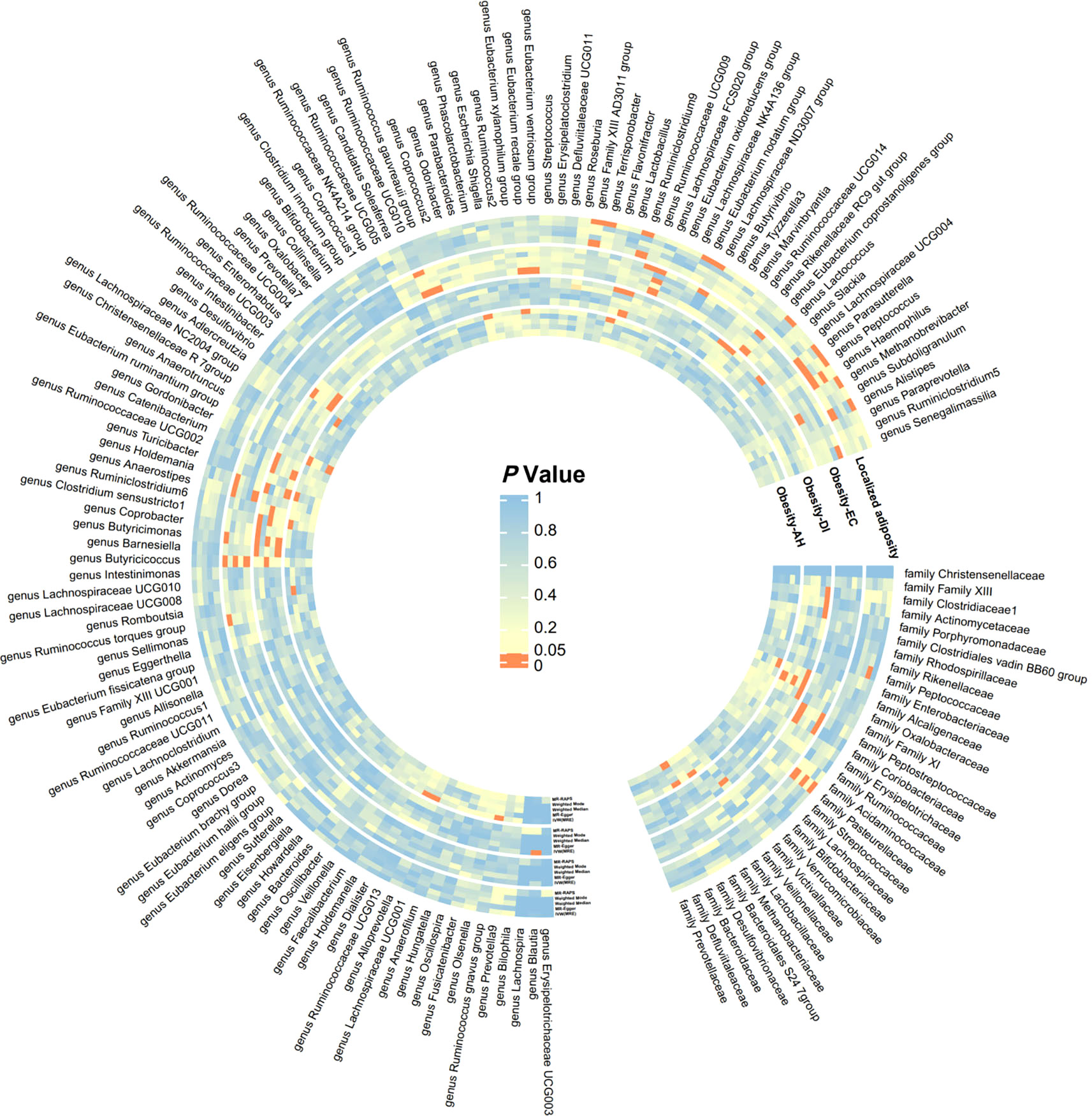
Figure 2 Significance heatmap of MR analysis. IVW, inverse variance weighted; MR, Mendelian randomization; RAPS, Robust Adjusted Profile Score.
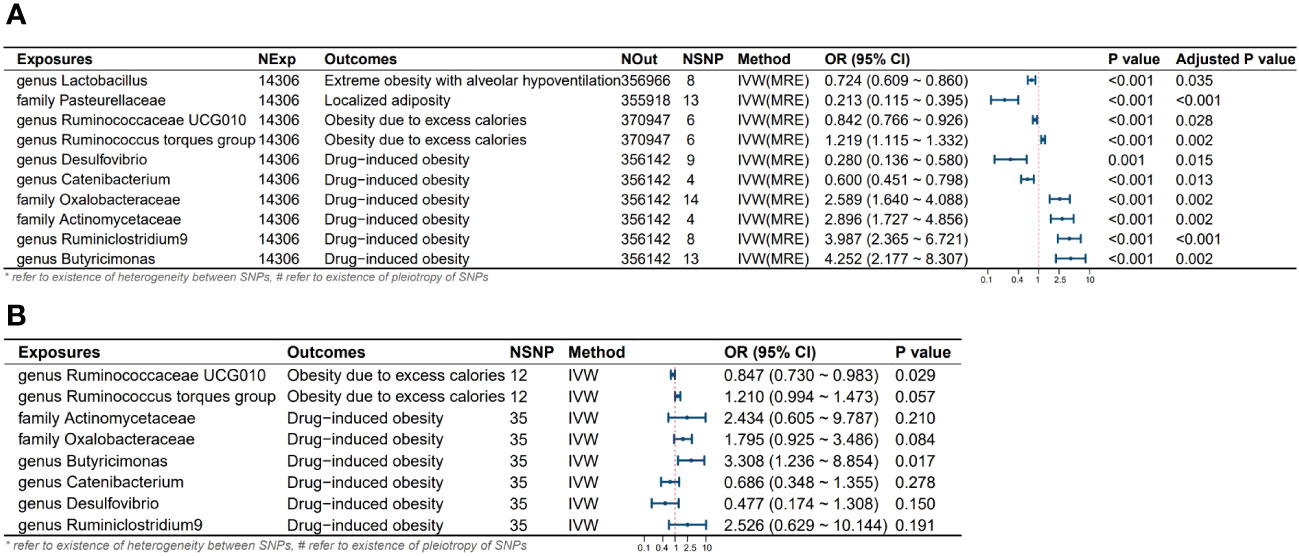
Figure 3 Results and forest plot of the MR analysis. (A) Results and forest plot of the significant UVMR analysis; (B) Results and forest plot of the MVMR analysis; IVW(MRE), inverse variance weighted (multiplicative random effects model); CI, confidence interval; NExp, sample size of exposure dataset; NOut, sample size of outcome dataset; NSNP, number of SNP included in MR analysis; *refer to existence of heterogeneity of SNPs, #refer to existence of pleiotropy between SNPs.
3.3 Impact of gut microbiota abundance changes on obesity onset
Through univariate and multivariate MR analyses, we identified four intestinal microbial communities that influence the occurrence of obesity. Additionally, we discovered six potential influential microbial communities, which were no longer significant after the multivariate analysis. Ruminococcaceae UCG010 independently reduces the risk of obesity due to excess calorie intake (Odds Ratio (OR): 0.842, 95% confidence interval (CI): 0.766-0.926, Adjusted P value: 0.028). Butyricimonas independently increases the risk of obesity due to excess calorie intake (OR: 4.252, 95% CI: 2.177-8.307, Adjusted P value: 0.002). Pasteurellaceae is a protective factor for localized adiposity population (OR: 0.213, 95% CI: 0.115-0.395, Adjusted P value: <0.001). Lactobacillus reduces the risk of extreme obesity with alveolar hypoventilation (OR: 0.724, 95% CI: 0.609-0.860, Adjusted P value: 0.035). Although the following bacteria are no longer significant after multivariable MR, we still consider them potential factors influencing the onset of obesity. Specifically, the Ruminococcus torques group may potentially increase the risk of its onset. Desulfovibrio, and Catenabacterium may act as protective factors in the development of Drug-induced obesity in the population. Oxalobacteraceae, Actinomycetaceae, and Ruminiclostridium 9 may potentially increase the risk of Drug-induced obesity. No heterogeneity and horizontal pleiotropy were observed among the SNPs in the above-mentioned study (all P values for Q test and MR-Egger intercept > 0.05) (Table 2).
4 Discussion
In this study, we found significant differences in the gut microbiota characteristics among different types of obese populations, revealing a close association between gut microbiota and obesity. Through univariate and multivariate Mendelian randomization (MR) analyses, we identified significant alterations in the abundance of Ruminococcaceae UCG010, Butyricimonas, Lactobacillus, and Pasteurellaceae in populations with Obesity due to excess calories, drug-induced obesity, extreme obesity with alveolar hypoventilation, and localized adiposity, respectively, which confirms the independent association of these four-gut microbiota with obesity. Additionally, we observed potential correlations between obesity and six other gut microbiota.
Previous studies, both in rodents and human clinical research, consistently demonstrated a common pattern in the gut microbiota of obese individuals, characterized by a relative decrease in the abundance of Bacteroidetes and an increase in Firmicutes. Reiner and Mary, among others, observed an increase in Firmicutes and a decrease in Bacteroidetes abundance in mice exposed to a high-fat diet (Hildebrandt et al., 2009; Jumpertz et al., 2011). A study conducted on the Ukrainian population found a significant increase in Firmicutes and a decrease in Bacteroidetes levels in adult obese individuals in the country. Furthermore, the Firmicutes/Bacteroidetes ratio was positively correlated with BMI (Koliada et al., 2017). Ley et al. also reported a higher abundance of Firmicutes and a lower abundance of Bacteroidetes in obese populations, with the proportion of Bacteroidetes gradually increasing with the frequency of low-calorie (that is, low-fat and low-carbohydrate) diets (Ley et al., 2006). At the genus level, the gut microbiota of overweight or obese individuals exhibited differences compared to the healthy group. Million et al. identified a positive association between Lactobacillus reuteri and obesity, while Lactobacillus reuteri, Escherichia coli, and Methanobrevibacter smithii showed a negative correlation with obesity (Million et al., 2013). Yun et al. conducted 16S rRNA gene sequencing on healthy, overweight, and obese populations and identified a positive association of Paraprevotellaceae with overweight individuals, while Acidaminococcus and Adlercreutzia showed a positive correlation with obesity; Eggerthella exhibited a negative correlation with both overweight and obese patients (Yun et al., 2017).
In contrast to the aforementioned studies, our investigation of obese patients in a European population, where both the exposure and outcome were European individuals, revealed a decrease in the abundance of Firmicutes and Bacteroidetes in their gut microbiota, accompanied by an increase in Bacteroidetes abundance. We believe that the primary reason for the observed differences lies in the variation in the study populations. Previous related studies primarily focused on individuals with obesity primarily attributed to excessive calorie intake. In contrast, our study conducted a more detailed stratification of obese patients, incorporating multiple outcome variables for Mendelian randomization analysis. Consequently, we observed a decrease in the abundance of Ruminococcaceae UCG010 (a family within the Ruminococcaceae family) in individuals with obesity resulting from excessive calorie intake. This bacterium belongs to the Firmicutes phylum, and its production of short-chain fatty acids (SCFAs), primarily butyrate, serves as a major energy source for colonocytes (Kang et al., 2017). Butyrate not only contributes to extra fat deposition in the body but also binds to G protein-coupled receptors (GPR) 41 and GPR43 (Lin et al., 2012), promoting the expression of satiety hormones such as peptide YY (PYY) and leptin in adipocytes (Louis and Flint, 2017; Cuevas-Sierra et al., 2019). Additionally, it stimulates hepatic fat synthesis and intestinal motility (Chakraborti, 2015). Furthermore, butyrate can induce L cells to secrete glucagon-like peptide-1 (GLP-1), attenuating obesity (Yadav et al., 2013). Therefore, we proposed that Ruminococcaceae UCG010 serves as a protective factor against Obesity due to excess calories.
It is noteworthy that this study represents the first analysis of the gut microbiota characteristics in individuals with drug-induced obesity, extreme obesity with alveolar hypoventilation, and localized adiposity. The findings have revealed some intriguing results. We observed an increased abundance of Butyricimonas in the gut microbiota of individuals with drug-induced obesity. Butyricimonas, the Gram-negative anaerobic bacterium within the Bacteroidota phylum, Odoribacteraceae family, is considered to significantly ameliorate obesity induced by a high-fat diet and reduce hepatic fat deposition (Rodriguez et al., 2020; Lee et al., 2022). However, in this study, Butyricimonas not only had no discernible impact on the population with obesity due to excess calorie intake but rather increased the risk of developing drug-induced obesity. Several potential reasons may underlie this paradox: Gram-negative bacteria contain lipopolysaccharides (LPS) as an intrinsic component of their cell wall, which is considered an endotoxin. In healthy individuals, blood levels of LPS are typically low. LPS is a potent activator of toll-like receptor 4 (TLR4) (Lim and Staudt, 2013). The binding of LPS to TLR4 can activate a wide array of cellular signaling pathways, inducing inflammation responses and the expression and secretion of cytokines within adipocytes and macrophages, leading to insulin resistance development and increased obesity (Medzhitov and Horng, 2009; Abdallah Ismail et al., 2011). Furthermore, the increase in LPS can directly enhance intestinal permeability, further exacerbating metabolic endotoxemia and inflammatory responses (Saad et al., 2016). Additionally, it is possible that Butyricimonas amplifies the effects of drugs in the process of inducing obesity, thereby further promoting or exacerbating obesity. Our hypothesis gains additional support from a report detailing the isolation of a new anaerobic bacterial species, Butyricimonas phoceensis sp. nov., from a severely obese 57-year-old French woman with a BMI of 55.8 kg/m² (Togo et al., 2016). Further research is required to delve into the specific mechanistic correlations between Butyricimonas and drug-induced obesity.
In individuals with extreme obesity accompanied by alveolar hypoventilation, we observed a decreased abundance of Lactobacillus, a genus within the Lactobacillaceae family, Firmicutes phylum. Previous research has consistently demonstrated the beneficial effects of many Lactobacillus strains in mitigating obesity in calorie-induced obesity populations. Wei et al. observed that Loigolactobacillus coryniformis and Lacticasebacillus paracasei reduced the body weight, Lee’s index, and fat index in high-fat diet-fed mice, significantly alleviating obesity (Song et al., 2021). Kang et al. also confirmed the significant role of Lactobacillus acidophilus in combating obesity, suppressing inflammation, increasing energy metabolism, and regulating lipid metabolism (Kang et al., 2022). Lactobacillus can restore the intestinal barrier, reduce intestinal permeability, inhibit bacteria and LPS entry into the liver, further suppressing the TLR4/NF-κB signaling pathway, and reducing inflammation. Additionally, this bacterium can increase the production of short-chain fatty acids (SCFAs) and regulate bile acid metabolism (Carvalho and Saad, 2013). In this study, we identified Lactobacillus as a protective factor in individuals with extreme obesity accompanied by alveolar hypoventilation. This represents the first discovery of this bacterium’s newfound prominence in this context. However, the specific mechanisms remain incompletely understood, necessitating further in-depth research.
Lastly, we observed a decreased abundance of Pasteurellaceae in individuals with localized adiposity. There is limited research regarding the association between Pasteurellaceae and obesity. This bacterium belongs to the Gram-negative anaerobic bacteria within the phylum Proteobacteria, comprising 13 genera and 65 species (Bonaventura et al., 2010). Most of its members live in symbiosis on the mucosal surfaces of birds and mammals, particularly in the upper respiratory tract (Kokotovic et al., 2007). Some of the human pathogens within this family include Haemophilus influenzae (St Geme, 2002) and Aggregatibacter species (Peng et al., 2019), which can cause various diseases such as meningitis, otitis media, chancroid, pneumonia, periodontitis, among others (Mullen et al., 2007). Maria et al. conducted a comparative genomic analysis of Pasteurellaceae and identified numerous unique conserved signature indels (CSIs). These CSIs were found to be uniquely shared among all sequenced Pasteurella species/strains and were not present in other bacteria. Hence, it is not out of the realm of possibility that Pasteurellaceae’s potential role in ameliorating localized adiposity may be linked to these unique CSIs. However, due to the limited research in this area, further validation is required (Bonaventura et al., 2010).
With the increasing annual incidence of obesity, obesity, as one of the key global economic and health concerns, is receiving growing attention. Obesity not only affects the quality of people’s life but also increases the risk of various short-term and long-term complications, thereby reducing life expectancy. Therefore, the treatment of obesity is currently a focal point of interest. To date, apart from lifestyle interventions, there are some anti-obesity medications available that are effective. However, they still have limitations, such as the potential for adverse events and higher costs (Aron-Wisnewsky et al., 2021). Therefore, in order to reverse the global obesity trend and its associated management costs, there is a need to develop and implement safe and cost-effective public health interventions.
It is known that probiotics can alter the composition and population of the gastrointestinal microbiota and can be used to prevent or ameliorate associated gastrointestinal diseases. However, both have demonstrated promising preventative or therapeutic effects on non-gastrointestinal diseases, such as obesity, type 2 diabetes, and cardiovascular diseases, in animal models. Although human data are equivocal, it offers a novel perspective for their potential clinical use in managing diseases (Forgie et al., 2019) .
In this study, based on databases comprising European populations for both exposure and outcomes, we conducted univariable and multivariable Mendelian randomization (MR) analyses. This study is the first to comprehensively stratify obesity and unveil distinctive gut microbiota features among various obesity types. It confirms the causal relationship between gut microbiota and obesity. It is our hope that this work will provide novel diagnostic and therapeutic avenues for different types of obesity in future clinical practice. Furthermore, our study rigorously controlled for confounding factors related to obesity, rendering the conclusions more reliable compared to previous observational studies. Nevertheless, this study has certain limitations. Firstly, our study was confined to European populations. Given the substantial differences in dietary habits and genetic traits among diverse populations, the findings of this study may not be directly applicable to populations outside of Europe. Secondly, this study did not provide a molecular mechanism explaining how gut microbiota precisely influences the occurrence of thrombotic diseases. Thirdly, due to the limitations of the gut microbiota genome-wide association study (GWAS) databases used in this study, some gut microbiota beyond the scope of these databases may have been inadvertently overlooked.
5 Conclusion
There is a causal relationship between gut microbiota abundance and obesity, with distinct gut microbiota profiles observed in different obese populations. The bacteria, including Ruminococcaceae UCG010, Butyricimonas, Pasteurellaceae and lactobacillus independently influence the development of various types of obesity. Additionally, there are six gut microbiota species with potential associations with the development of obesity. The adjunctive therapy of probiotics and prebiotics may emerge as a novel approach in the future treatment of obesity.
Data availability statement
Publicly available datasets were analyzed in this study. This data can be found here: https://mibiogen.gcc.rug.nl/menu/main/home; https://www.finngen.fi/en/access_results.
Author contributions
SL: Writing – original draft. FL: Writing – original draft. YC: Investigation, Writing – review & editing. LR: Writing – review & editing. LS: Supervision, Writing – review & editing. XG: Supervision, Writing – review & editing. GW: Funding acquisition, Supervision, Writing – review & editing.
Funding
The author(s) declare financial support was received for the research, authorship, and/or publication of this article. This work was supported by grants from the Department of Science and Technology of Jilin Province (20190901006JC to GW).
Acknowledgments
We want to acknowledge the participants and investigators of the FinnGen study and MlBioGen study.
Conflict of interest
The authors declare that the research was conducted in the absence of any commercial or financial relationships that could be construed as a potential conflict of interest.
Publisher’s note
All claims expressed in this article are solely those of the authors and do not necessarily represent those of their affiliated organizations, or those of the publisher, the editors and the reviewers. Any product that may be evaluated in this article, or claim that may be made by its manufacturer, is not guaranteed or endorsed by the publisher.
Supplementary material
The Supplementary Material for this article can be found online at: https://www.frontiersin.org/articles/10.3389/fcimb.2024.1352109/full#supplementary-material
References
Abdallah Ismail, N., Ragab, S. H., Abd Elbaky, A., Shoeib, A. R., Alhosary, Y., Fekry, D. (2011). Frequency of Firmicutes and Bacteroidetes in gut microbiota in obese and normal weight Egyptian children and adults. Arch. Med. Sci. 7 (3), 501–507. doi: 10.5114/aoms.2011.23418
Aron-Wisnewsky, J., Warmbrunn, M. V., Nieuwdorp, M., Clement, K. (2021). Metabolism and metabolic disorders and the microbiome: the intestinal microbiota associated with obesity, lipid metabolism, and metabolic health-pathophysiology and therapeutic strategies. Gastroenterology 160 (2), 573–599. doi: 10.1053/j.gastro.2020.10.057
Backhed, F., Ding, H., Wang, T., Hooper, L. V., Koh, G. Y., Nagy, A., et al. (2004). The gut microbiota as an environmental factor that regulates fat storage. Proc. Natl. Acad. Sci. U.S.A. 101 (44), 15718–15723. doi: 10.1073/pnas.0407076101
Blaut, M. (2015). Gut microbiota and energy balance: role in obesity. Proc. Nutr. Soc. 74 (3), 227–234. doi: 10.1017/S0029665114001700
Bonaventura, M. P., Lee, E. K., Desalle, R., Planet, P. J. (2010). A whole-genome phylogeny of the family Pasteurellaceae. Mol. Phylogenet Evol. 54 (3), 950–956. doi: 10.1016/j.ympev.2009.08.010
Bowden, J., Davey Smith, G., Haycock, P. C., Burgess, S. (2016a). Consistent estimation in mendelian randomization with some invalid instruments using a weighted median estimator. Genet. Epidemiol. 40 (4), 304–314. doi: 10.1002/gepi.21965
Bowden, J., Del Greco, M. F., Minelli, C., Davey Smith, G., Sheehan, N. A., Thompson, J. R. (2016b). Assessing the suitability of summary data for two-sample Mendelian randomization analyses using MR-Egger regression: the role of the I2 statistic. Int. J. Epidemiol. 45 (6), 1961–1974. doi: 10.1093/ije/dyw220
Burgess, S., Butterworth, A., Thompson, S. G. (2013). Mendelian randomization analysis with multiple genetic variants using summarized data. Genet. Epidemiol. 37 (7), 658–665. doi: 10.1002/gepi.21758
Burgess, S., Davey Smith, G., Davies, N. M., Dudbridge, F., Gill, D., Glymour, M. M., et al. (2019). Guidelines for performing Mendelian randomization investigations: update for summer 2023. Wellcome Open Res. 4, 186. doi: 10.12688/wellcomeopenres.15555.1
Burgess, S., Small, D. S., Thompson, S. G. (2017). A review of instrumental variable estimators for Mendelian randomization. Stat. Methods Med. Res. 26 (5), 2333–2355. doi: 10.1177/0962280215597579
Burgess, S., Thompson, S. G. (2015). Multivariable Mendelian randomization: the use of pleiotropic genetic variants to estimate causal effects. Am. J. Epidemiol. 181 (4), 251–260. doi: 10.1093/aje/kwu283
Burgess, S., Thompson, S. G. (2017). Interpreting findings from Mendelian randomization using the MR-Egger method. Eur. J. Epidemiol. 32 (5), 377–389. doi: 10.1007/s10654-017-0255-x
Burgess, S., Thompson, S. G., Collaboration, C. C. G. (2016). Methods for meta-analysis of individual participant data from Mendelian randomisation studies with binary outcomes. Stat. Methods Med. Res. 25 (1), 272–293. doi: 10.1177/0962280212451882
Carvalho, B. M., Saad, M. J. (2013). Influence of gut microbiota on subclinical inflammation and insulin resistance. Mediators Inflammation 2013, 986734. doi: 10.1155/2013/986734
Chakraborti, C. K. (2015). New-found link between microbiota and obesity. World J. Gastrointest Pathophysiol 6 (4), 110–119. doi: 10.4291/wjgp.v6.i4.110
Cuevas-Sierra, A., Ramos-Lopez, O., Riezu-Boj, J. I., Milagro, F. I., Martinez, J. A. (2019). Diet, gut microbiota, and obesity: links with host genetics and epigenetics and potential applications. Adv. Nutr. 10 (suppl_1), S17–S30. doi: 10.1093/advances/nmy078
den Besten, G., van Eunen, K., Groen, A. K., Venema, K., Reijngoud, D. J., Bakker, B. M. (2013). The role of short-chain fatty acids in the interplay between diet, gut microbiota, and host energy metabolism. J. Lipid Res. 54 (9), 2325–2340. doi: 10.1194/jlr.R036012
Deschasaux, M., Bouter, K. E., Prodan, A., Levin, E., Groen, A. K., Herrema, H., et al. (2018). Depicting the composition of gut microbiota in a population with varied ethnic origins but shared geography. Nat. Med. 24 (10), 1526–1531. doi: 10.1038/s41591-018-0160-1
Emdin, C. A., Khera, A. V., Kathiresan, S. (2017). Mendelian randomization. JAMA 318 (19), 1925–1926. doi: 10.1001/jama.2017.17219
Forgie, A. J., Fouhse, J. M., Willing, B. P. (2019). Diet-microbe-host interactions that affect gut mucosal integrity and infection resistance. Front. Immunol. 10, 1802. doi: 10.3389/fimmu.2019.01802
Fukumoto, S., Tatewaki, M., Yamada, T., Fujimiya, M., Mantyh, C., Voss, M., et al. (2003). Short-chain fatty acids stimulate colonic transit via intraluminal 5-HT release in rats. Am. J. Physiol. Regul. Integr. Comp. Physiol. 284 (5), R1269–R1276. doi: 10.1152/ajpregu.00442.2002
Haslam, D. W., James, W. P. (2005). Obesity. Lancet 366 (9492), 1197–1209. doi: 10.1016/S0140-6736(05)67483-1
Hildebrandt, M. A., Hoffmann, C., Sherrill-Mix, S. A., Keilbaugh, S. A., Hamady, M., Chen, Y. Y., et al. (2009). High-fat diet determines the composition of the murine gut microbiome independently of obesity. Gastroenterology 137 (5), 1716–1724.e1711-1712. doi: 10.1053/j.gastro.2009.08.042
Jumpertz, R., Le, D. S., Turnbaugh, P. J., Trinidad, C., Bogardus, C., Gordon, J. I., et al. (2011). Energy-balance studies reveal associations between gut microbes, caloric load, and nutrient absorption in humans. Am. J. Clin. Nutr. 94 (1), 58–65. doi: 10.3945/ajcn.110.010132
Kang, Y., Kang, X., Yang, H., Liu, H., Yang, X., Liu, Q., et al. (2022). Lactobacillus acidophilus ameliorates obesity in mice through modulation of gut microbiota dysbiosis and intestinal permeability. Pharmacol. Res. 175, 106020. doi: 10.1016/j.phrs.2021.106020
Kang, C., Wang, B., Kaliannan, K., Wang, X., Lang, H., Hui, S., et al. (2017). Gut microbiota mediates the protective effects of dietary capsaicin against chronic low-grade inflammation and associated obesity induced by high-fat diet. mBio, 8(3). doi: 10.1128/mBio.00470-17
Kokotovic, B., Friis, N. F., Ahrens, P. (2007). Mycoplasma alkalescens demonstrated in bronchoalveolar lavage of cattle in Denmark. Acta Vet. Scand. 49 (1), 2. doi: 10.1186/1751-0147-49-2
Koliada, A., Syzenko, G., Moseiko, V., Budovska, L., Puchkov, K., Perederiy, V., et al. (2017). Association between body mass index and Firmicutes/Bacteroidetes ratio in an adult Ukrainian population. BMC Microbiol. 17 (1), 120. doi: 10.1186/s12866-017-1027-1
Kurilshikov, A., Medina-Gomez, C., Bacigalupe, R., Radjabzadeh, D., Wang, J., Demirkan, A., et al. (2021). Large-scale association analyses identify host factors influencing human gut microbiome composition. Nat. Genet. 53 (2), 156–165. doi: 10.1038/s41588-020-00763-1
Kurki, M. I., Karjalainen, J., Palta, P., Sipila, T. P., Kristiansson, K., Donner, K. M., et al. (2023). FinnGen provides genetic insights from a well-phenotyped isolated population. Nature 613 (7944), 508–518. doi: 10.1038/s41586-022-05473-8
Lee, H., An, J., Kim, J., Choi, D., Song, Y., Lee, C. K., et al. (2022). A Novel Bacterium, Butyricimonas virosa, Preventing HFD-Induced Diabetes and Metabolic Disorders in Mice via GLP-1 Receptor. Front. Microbiol. 13, 858192. doi: 10.3389/fmicb.2022.858192
Ley, R. E., Backhed, F., Turnbaugh, P., Lozupone, C. A., Knight, R. D., Gordon, J. I. (2005). Obesity alters gut microbial ecology. Proc. Natl. Acad. Sci. U.S.A. 102 (31), 11070–11075. doi: 10.1073/pnas.0504978102
Ley, R. E., Turnbaugh, P. J., Klein, S., Gordon, J. I. (2006). Microbial ecology: human gut microbes associated with obesity. Nature 444 (7122), 1022–1023. doi: 10.1038/4441022a
Li, Q., Gao, J., Luo, J., Lin, D., Wu, X. (2023). Mendelian randomization analyses support causal relationship between gut microbiota and childhood obesity. Front. Pediatr. 11, 1229236. doi: 10.3389/fped.2023.1229236
Li, F., Liu, Y., Wang, Z., Zhao, Q., Li, Y., Tang, T. (2022). A mendelian randomization study with populations of European ancestry rules out a causal relationship between inflammatory bowel disease and colorectal cancer. Front. Genet. 13, 949325. doi: 10.3389/fgene.2022.949325
Lim, K. H., Staudt, L. M. (2013). Toll-like receptor signaling. Cold Spring Harb. Perspect. Biol. 5 (1), a011247. doi: 10.1101/cshperspect.a011247
Lin, H. V., Frassetto, A., Kowalik, E. J., Jr., Nawrocki, A. R., Lu, M. M., Kosinski, J. R., et al. (2012). Butyrate and propionate protect against diet-induced obesity and regulate gut hormones via free fatty acid receptor 3-independent mechanisms. PloS One 7 (4), e35240. doi: 10.1371/journal.pone.0035240
Louis, P., Flint, H. J. (2017). Formation of propionate and butyrate by the human colonic microbiota. Environ. Microbiol. 19 (1), 29–41. doi: 10.1111/1462-2920.13589
Marchesi, J. R., Adams, D. H., Fava, F., Hermes, G. D., Hirschfield, G. M., Hold, G., et al. (2016). The gut microbiota and host health: a new clinical frontier. Gut 65 (2), 330–339. doi: 10.1136/gutjnl-2015-309990
Medzhitov, R., Horng, T. (2009). Transcriptional control of the inflammatory response. Nat. Rev. Immunol. 9 (10), 692–703. doi: 10.1038/nri2634
Million, M., Angelakis, E., Maraninchi, M., Henry, M., Giorgi, R., Valero, R., et al. (2013). Correlation between body mass index and gut concentrations of Lactobacillus reuteri, Bifidobacterium animalis, Methanobrevibacter smithii and Escherichia coli. Int. J. Obes. (Lond) 37 (11), 1460–1466. doi: 10.1038/ijo.2013.20
Mullen, L. M., Nair, S. P., Ward, J. M., Rycroft, A. N., Williams, R. J., Henderson, B. (2007). Comparative functional genomic analysis of Pasteurellaceae adhesins using phage display. Vet. Microbiol. 122 (1-2), 123–134. doi: 10.1016/j.vetmic.2006.12.022
Neuman, H., Debelius, J. W., Knight, R., Koren, O. (2015). Microbial endocrinology: the interplay between the microbiota and the endocrine system. FEMS Microbiol. Rev. 39 (4), 509–521. doi: 10.1093/femsre/fuu010
Peng, Z., Wang, X., Zhou, R., Chen, H., Wilson, B. A., Wu, B. (2019). Pasteurella multocida: genotypes and genomics. Microbiol. Mol. Biol. Rev. 83 (4). doi: 10.1128/MMBR.00014-19
Rodriguez, J., Hiel, S., Neyrinck, A. M., Le Roy, T., Potgens, S. A., Leyrolle, Q., et al. (2020). Discovery of the gut microbial signature driving the efficacy of prebiotic intervention in obese patients. Gut 69 (11), 1975–1987. doi: 10.1136/gutjnl-2019-319726
Rothschild, D., Weissbrod, O., Barkan, E., Kurilshikov, A., Korem, T., Zeevi, D., et al. (2018). Environment dominates over host genetics in shaping human gut microbiota. Nature 555 (7695), 210–215. doi: 10.1038/nature25973
Saad, M. J., Santos, A., Prada, P. O. (2016). Linking gut microbiota and inflammation to obesity and insulin resistance. Physiol. (Bethesda) 31 (4), 283–293. doi: 10.1152/physiol.00041.2015
Sanderson, E., Davey Smith, G., Windmeijer, F., Bowden, J. (2019). An examination of multivariable Mendelian randomization in the single-sample and two-sample summary data settings. Int. J. Epidemiol. 48 (3), 713–727. doi: 10.1093/ije/dyy262
Song, W., Song, C., Li, L., Wang, T., Hu, J., Zhu, L., et al. (2021). Lactobacillus alleviated obesity induced by high-fat diet in mice. J. Food Sci. 86 (12), 5439–5451. doi: 10.1111/1750-3841.15971
Staley, J. R., Blackshaw, J., Kamat, M. A., Ellis, S., Surendran, P., Sun, B. B., et al. (2016). PhenoScanner: a database of human genotype-phenotype associations. Bioinformatics 32 (20), 3207–3209. doi: 10.1093/bioinformatics/btw373
St Geme, J. W., 3rd (2002). Molecular and cellular determinants of non-typeable Haemophilus influenzae adherence and invasion. Cell Microbiol. 4 (4), 191–200. doi: 10.1046/j.1462-5822.2002.00180.x
Togo, A. H., Diop, A., Dubourg, G., Nguyen, T. T., Andrieu, C., Caputo, A., et al. (2016). Butyricimonas phoceensis sp. nov., a new anaerobic species isolated from the human gut microbiota of a French morbidly obese patient. New Microbes New Infect. 14, 38–48. doi: 10.1016/j.nmni.2016.07.010
Tsukumo, D. M., Carvalho, B. M., Carvalho Filho, M. A., Saad, M. J. (2015). Translational research into gut microbiota: new horizons on obesity treatment: updated 2014. Arch. Endocrinol. Metab. 59 (2), 154–160. doi: 10.1590/2359-3997000000029
Vlassopoulos, A., Lean, M. E. (2016). Unraveling the effect of adiposity on health: The epidemiology paradoxes. Obes. (Silver Spring) 24 (6), 1212. doi: 10.1002/oby.21530
Yadav, H., Lee, J. H., Lloyd, J., Walter, P., Rane, S. G. (2013). Beneficial metabolic effects of a probiotic via butyrate-induced GLP-1 hormone secretion. J. Biol. Chem. 288 (35), 25088–25097. doi: 10.1074/jbc.M113.452516
Keywords: gut microbiota, obesity, Mendelian randomization, causal relationship, stratification
Citation: Liu S, Li F, Cai Y, Ren L, Sun L, Gang X and Wang G (2024) Unraveling the mystery: a Mendelian randomized exploration of gut microbiota and different types of obesity. Front. Cell. Infect. Microbiol. 14:1352109. doi: 10.3389/fcimb.2024.1352109
Received: 07 December 2023; Accepted: 23 January 2024;
Published: 05 February 2024.
Edited by:
Qixiao Zhai, Jiangnan University, ChinaReviewed by:
Maria Oana Sasaran, “George Emil Palade” University of Medicine, Pharmacy, Sciences and Technology of Târgu Mureș, RomaniaAmanda Carroll-Portillo, University of New Mexico, United States
Copyright © 2024 Liu, Li, Cai, Ren, Sun, Gang and Wang. This is an open-access article distributed under the terms of the Creative Commons Attribution License (CC BY). The use, distribution or reproduction in other forums is permitted, provided the original author(s) and the copyright owner(s) are credited and that the original publication in this journal is cited, in accordance with accepted academic practice. No use, distribution or reproduction is permitted which does not comply with these terms.
*Correspondence: Guixia Wang, gwang168@jlu.edu.cn
†These authors have contributed equally to this work and share first authorship