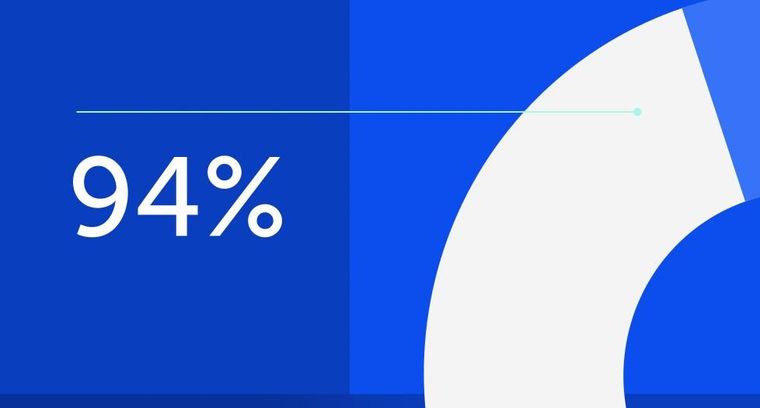
94% of researchers rate our articles as excellent or good
Learn more about the work of our research integrity team to safeguard the quality of each article we publish.
Find out more
ORIGINAL RESEARCH article
Front. Cell. Infect. Microbiol., 12 April 2023
Sec. Intestinal Microbiome
Volume 13 - 2023 | https://doi.org/10.3389/fcimb.2023.1130701
This article is part of the Research TopicNew Techniques in Microbiome Research - Vol II – Host-Microbiome Interactions using ‘meta-omics’ techniquesView all 19 articles
Introduction: Clostridioides difficile infection (CDI) is the main cause of nosocomial diarrhea in developed countries. A key challenge in CDI is the lack of objective methods to ensure more accurate diagnosis, especially when differentiating between true infection and colonization/diarrhea of other causes. The main objective of this study was to explore the role of the microbiome as a predictive biomarker of CDI.
Methods: Between 2018 and 2021, we prospectively included patients with CDI, recurrent CDI (R-CDI), non-CDI diarrhea (NO-CDI), colonization by C. difficile, and healthy individuals. Clinical data and fecal samples were collected. The microbiome was analyzed by sequencing the hypervariable V4 region of the 16S rRNA gene on an Illumina Miseq platform. The mothur bioinformatic pipeline was followed for pre-processing of raw data, and mothur and R were used for data analysis.
Results: During the study period, 753 samples from 657 patients were analyzed. Of these, 247 were from patients with CDI, 43 were from patients colonized with C. difficile, 63 were from healthy individuals, 324 were from NOCDI, and 76 were from R-CDI. We found significant differences across the groups in alpha and beta diversity and in taxonomic abundance. We identified various genera as the most significant biomarkers for CDI (Bacteroides, Proteus, Paraprevotella, Robinsoniella), R-CDI (Veillonella, Fusobacterium, Lactobacillus, Clostridium sensu stricto I), and colonization by C. difficile (Parabacteroides, Faecalicoccus, Flavonifractor, Clostridium XVIII).
Discussion: We observed differences in microbiome patterns between healthy individuals, colonized patients, CDI, R-CDI, and NOCDI diarrhea. We identified possible microbiome biomarkers that could prove useful in the diagnosis of true CDI infections. Further studies are warranted.
Clostridioides difficile infection (CDI) is the main cause of nosocomial diarrhea in developed countries (Dubberke and Olsen, 2012). The clinical severity of CDI can be classified as follows: absence of symptoms; mild, moderate, and severe disease; pseudomembranous colitis; and toxic megacolon, sepsis, and death (Rupnik et al., 2009).
Risk factors associated with CDI include advanced age, hospital stay, treatment with proton pump inhibitors, and prolonged antibiotic treatment or treatment with multiple antibiotics (Bignardi, 1998). The risk factor most closely associated with disease is use of antibiotics, as it is directly related to dysbiosis of the gut microbiota, which enables germination of and colonization by C. difficile (Theriot and Young, 2015; Theriot et al., 2016; Thanissery et al., 2017). Dysbiosis caused by antibiotic treatment is reflected in decreased diversity and loss of specific taxa, both of which alter the variety and quantity of metabolites present in the gut (Jernberg et al., 2007; Dethlefsen et al., 2008; Jernberg et al., 2010; Britton and Young, 2014).
Age also plays an important role in the risk of CDI, as the gut microbiota becomes less diverse with age. In addition, the presence of chronic diseases and the use of multiple drugs, including antibiotics, considerably affects the microbiota, thus increasing the risk of colonization by C. difficile in elderly patients (Odamaki et al., 2016; Gao et al., 2018).
Because of the close relationship between gut microbiota and C. difficile, guidelines recommend fecal microbiota transplantation for recurrent episodes of CDI in order to restore patients’ gut microbiota and prevent further recurrences (McDonald et al., 2018). However, more preventive strategies are needed.
The considerable associated health and economic burden of CDI calls for novel strategies by which CDI can be prevented in susceptible patients (Heimann et al., 2018; Reigadas Ramirez and Bouza, 2018). Profiling differences between the gut microbiota of patients with CDI, that of healthy individuals, and that of individuals with diarrhea due to other causes could help us to predict which patients are at immediate risk for CDI, which will progress better or worse, and which will experience recurrence of CDI.
One of the challenges in diagnosing CDI is that of being able to distinguish between patients with true CDI and those who are colonized (Planche and Wilcox, 2015). The search for new methods for the detection of C. difficile and for distinguishing true infection from colonization in diarrhea of other causes is ongoing (Kelly et al., 2020; Sandlund et al., 2020; Ng et al., 2021). Profiling the microbiome and identifying possible microbiome biomarkers could help us differentiate between infection and colonization by C. difficile and diarrhea of other causes (NOCDI) (Planche and Wilcox, 2015).
The main objective of this study was to explore the role of the microbiome as a predictive biomarker of true CDI.
The study was carried out at Hospital General Universitario Gregorio Marañón in Madrid (Spain), a 1,350-bed tertiary university hospital. Determination of toxigenic C. difficile is routinely performed on all loose stool samples from patients older than 2 years. The microbiology laboratory receives samples both from the hospital itself and from 13 outpatient centers in the same area.
We conducted a prospective study from 2018 to 2021. We enrolled patients whose stool samples were sent to the microbiology laboratory and healthy individuals. We collected clinical data and diagnostic fecal samples from all patients. The participants were classified into the following groups: healthy individuals, patients with primary CDI (CDI), patients with recurrent CDI (R-CDI), patients colonized by toxigenic C. difficile (colonized) and patients with diarrhea who tested negative for C. difficile (NOCDI).
An episode of CDI was defined as the presence of a positive toxigenic CDI test, together with diarrhea (≥3 loose stools in 24 hours) or findings of pseudomembranous colitis by colonoscopy, following the definitions set out in the guidelines of the Society for Healthcare Epidemiology of America (SHEA) and Infectious Diseases Society of America (IDSA) (McDonald et al., 2018).
We considered a patient to be colonized with toxigenic C. difficile when he/she tested positive for toxigenic C. difficile but did not meet clinical criteria for CDI, as defined above.
The severity of the CDI episode was defined according to the SHEA and IDSA guidelines (McDonald et al., 2018).
R-CDI was defined as CDI recurring within 8 weeks of a previous episode, provided the symptoms of the previous episode resolved after completion of initial treatment (van Prehn et al., 2021). Having new symptoms and a positive sample after 60 days was considered a new episode.
Death was considered CDI-related when there were no other attributable causes and/or it occurred within 10 days after the diagnosis of CDI and/or was due to known complications of CDI.
Healthy individuals were defined as those who did not meet any of the following criteria: body mass index lower than 17 or higher than 30, any type of disease including microbiota-related disease (cholelithiasis, colorectal cancer, hepatic encephalopathy, idiopathic constipation, inflammatory bowel disease, irritable bowel syndrome, familial Mediterranean fever, gastric lymphoma or carcinoma, arthritis, asthma, atopy, dermatitis, psoriasis, autoimmune disease, fatigue syndrome, diabetes mellitus, hypercholesterolemia, idiopathic thrombocytopenic purpura, myocardial ischemia, metabolic syndrome, behavioral disorders, multiple sclerosis, myoclonus dystonia, non-alcoholic fatty liver disease, oxalate kidney stones, Parkinson’s disease), gastrointestinal disorders, immunologic disease, immunocompromise, alcohol intake higher than 50 g/day, and use of antibiotics, probiotics, immunosuppressants, proton pump inhibitors, or vaccines in the previous three months.
As for microbiome-related definitions, we considered richness as the number of different species found in a sample (Whittaker et al., 2001). Evenness was defined as the degree to which different species are similar or uniform in abundance. Diversity indicated the degree of species richness and abundance, where alpha diversity referred to the diversity within an individual and beta diversity referred to the difference in diversity between individuals (Whittaker et al., 2001).
Samples were processed using a rapid detection kit for toxigenic C. difficile. This rapid test involves detection of the antigen by immunochromatography (C Diff Quik-Chek Complete assay, TechLab, Blacksburg, VA, USA) and a real-time polymerase chain reaction (PCR) of the toxin B gene (XpertTMC. difficile Assay, GeneXpert, Cepheid, Sunnyvale, CA, USA).
In addition, all samples were cultured on C. difficile selective agar (bioMeriéux, Marcy l’Etoile, France). Suspected toxigenic C. difficile colonies were confirmed using immunochromatography (C Diff Quik-Chek Complete assay, TechLab, Blacksburg, VA, USA).
The demographic data collected included age and sex. Regarding clinical data, underlying conditions were recorded using the McCabe and Jackson score for prognosis of underlying diseases (McCabe and Jackson, 1962); comorbidity was graded according to the Charlson comorbidity index (Charlson et al., 1987). Other clinical data collected included antibiotic treatment, proton pump inhibitor treatment, nasogastric tube use, mechanical ventilation, surgery, and chemotherapy or radiotherapy in the month prior to CDI diagnosis. For the CDI episode, data on severity, treatment received, treatment failure, recurrence, mortality, and CDI-related mortality were recorded.
Immediately upon receipt, the fecal samples were homogenized, aliquoted, and stored at −80°C until the day of analysis. Total DNA was extracted from fecal samples using the Qiagen Fast QiaAmp DNA Stool Mini Kit (QIAGEN, Valencia, CA, USA) according to the manufacturer’s protocol with the inclusion of a physical lysis step. The sample was lysed in FastPrep-24 (MPBio, Derby, UK) with lysis matrix E tubes (MPBio, Derby, UK) twice at 6.5 m/s for 45 seconds. The hypervariable V4 region of the 16s rRNA gene was amplified by polymerase chain reaction with 515-806 primers tailed with sequences to incorporate Illumina flow cell adapters and indexing barcodes (Illumina, San Diego, USA).
Primer dimers and low-molecular-weight products were removed using Agencourt Ampure Beads (Beckman Coulter, Spain) and samples were quantified and quality checked for amplicon size using the 4200 TapeStation (Agilent Technologies, Santa Clara, CA, USA). Amplicons were pooled in equimolar amounts and sequenced (2 × 250) on an Illumina Miseq system (Illumina, San Diego, USA) according to standard protocols.
The raw data were pre-processed and grouped by operational taxonomic units (OTUs) with 97% similarity and classified taxonomically using mothur software (Patrick D. Schloss, PhD, © 2019, Michigan, USA) and SILVA and RDP Ribosomal Database Project databases. Species richness (OTUs observed), evenness (Pielou index), alpha diversity (Shannon index, inverse Simpson index), and beta diversity (Bray-Curtis distance, unweighted unifrac distance) were analyzed using mothur and R software (R Project for Statistical Computing) (R Core Team, 2021, Vienna, Austria).
Statistical analyses were performed with R (R Core Team, 2021, Vienna, Austria). Frequencies were calculated for qualitative variables, and proportions were calculated with their 95% confidence interval following a binomial distribution. For quantitative variables, the median and interquartile range (IQR) or mean and standard deviation (SD) were calculated. Microbiota analyses were performed with R using the Bioconductor packages phyloseq, microbiome, microbiomeStat, vegan, DESeq2, and microeco.
Biomarkers were found using linear discriminant analysis effect size (LEfSe) and random forest (RF). For the LEfSe analysis, the Kruskal-Wallis non-parametric factorial rank sum test was first performed, then the pairwise groups were analyzed using the unpaired Wilcoxon rank sum test. Following these steps, linear discrimination analysis (LDA) was used. LDA score higher than 3 was used. For random forest analysis bootstrapt test number selected was 30 and 1000 trees to grow. Method for adjusted p-values was false discovery rate and Mean Decrease Gini was selected as the indicator value in the analysis.
Differences between groups were determined using the χ2 test; differences for continuous variables were assessed using the t test. The Mann-Whitney test was used for non-normal distributions. The normality of the distribution of continuous variables was tested using the Kolmogorov-Smirnov test with the Lilliefors correction.
During the study period, a total of 753 samples from 657 patients were analyzed. Of these, 247 samples were obtained from 233 patients with CDI, 43 samples from 40 patients colonized with C. difficile, 63 samples from 63 healthy individuals, 324 samples from 264 patients with diarrhoea without C. difficile; and 76 samples from 57 R-CDI.
The median age of the patients was around 70 years, with more females in all groups except NOCDI, as follows: CDI, 56.2% (131/233); colonized, 52.5% (21/40); NOCDI, 49.2% (130/264); and R-CDI, 68.4% (39/57) (p=0.053). Most healthy individuals were female (52.4%), and the median age was 32 years (range, 0-76). The most common underlying conditions were cardiovascular, metabolic, endocrine, nephro-urological, and gastrointestinal diseases (Table 1). The median Charlson comorbidity index was 4 (IQR: 2-6) in the CDI, R-CDI, and NOCDI groups and 4 (IQR: 2.8-5.2) in the colonized group. The percentage of patients with underlying diseases related to microbiota abnormalities was high in all groups, although it was higher in R-CDI: colonized, 60.0% (24/40); NOCDI, 63.6% (168/264); CDI, 67.4% (157/233); and R-CDI, 80.7% (46/57). The most common underlying diseases were diabetes mellitus and myocardial ischemia (Table 1). The lowest number of immunosuppressed patients was found among colonized patients: 32.5% (13/40) vs CDI with 36.9% (86/233), NOCDI with 49.6% (131/264), and R-CDI with 43.9% (25/57) (p=0.018) (Table 1).
The main risk factor for developing CDI was antibiotics in the month prior to sampling, with greater percentages in CDI and R-CDI: CDI, 91.5% (226/247); colonized, 86.0% (37/43); NOCDI, 83.5% (269/324); R-CDI, 93.4% (71/76) (p=0.007). Overall, the most used antibiotic groups in all patients were penicillins, third generation cephalosporins, quinolones and carbapenems (Table 2, Supplementary Table 1). With regard to the other CDI risk factors assessed, treatment with proton pump inhibitors and hospitalization were common in most patients. However, no significant differences were found between the groups for proton pump inhibitors, nasogastric tube use, mechanical ventilation, chemotherapy, radiotherapy, or dialysis (Table 2).
As for severity, most episodes were mild (CDI, 63.6%; R-CDI, 65.8%). There were three cases of toxic megacolon and two cases of pseudomembranous colitis in the CDI group. Most CDI cases were hospital-onset, healthcare facility–associated (53.0% [131/247], while in the R-CDI patients, they were community-onset, healthcare facility–associated (50.0% [38/76]; p < 0.001). All groups contained patients who received treatment for CDI, as follows: CDI patients, 96.7% (238/246); colonized patients, 37.2% (16/43); NOCDI patients, 4.6% (15/324); and R-CDI patients 97.4% (74/76) (p<0.001). Most patients were treated with vancomycin. The recurrence rate was 25.7% in the primary CDI patients (53/206), and 30.0% in R-CDI (15/50). Five CDI patients (5/233; 2.1%) had a probable CDI-related death and four (4/233; 1.7%) had a clearly CDI-related death. In the R-CDI group, 3 (3/57; 5.3%) patients had a probable CDI-related death and 1 (1/57; 1.8%) a clearly CDI-related death (Table 3).
Comparison of the 5 groups revealed significant differences in richness, alpha diversity, and evenness (all p < 0.001); these differences were maintained when the healthy individuals were removed from the comparison (all p < 0.05). In all cases, the R-CDI patients had the lowest richness, the lowest alpha diversity, and the lowest evenness (Figure 1).
Figure 1 Box plot of Alpha diversity index (Shannon and Simpson) and Richness index (Observed) in all groups of patients. CDI, Clostridioides difficile infection; NOCDI, Non C. difficile diarrhoea; RCDI, recurrent CDI; Colonized, patients colonized with C. difficile; Healthy, Healthy subjects. *, p <= 0.05; **, p <= 0.01; ***, p <= 0.001; ****, p <= 0.0001.
When we stratified groups by age, all differences were maintained for those aged 16-69 years and for those over 69 years. When healthy individuals were removed from the comparison, there were significant differences in richness, alpha diversity, and evenness for those aged >69 years and in evenness for those aged 19-69 years. We found a series of significant differences, as follows: between CDI and NOCDI in richness, alpha diversity, and evenness (all p < 0.05); between CDI and R-CDI in richness and alpha diversity (all p < 0.05); between NOCDI and colonized patients in richness and evenness (p<0.05); and between R-CDI and colonized patients in richness and alpha diversity (p<0.05). No significant differences were found between CDI and colonized patients.
In all cases, we found significant differences between healthy individuals and each of the other groups, with higher richness, diversity, and evenness (p < 0.001) (Figure 1). Examination of each group individually revealed significant differences in alpha diversity and evenness among CDI with inflammatory bowel disease (IBD), with lower Shannon and Pielou indices. Differences in alpha diversity and evenness were also seen in patients who had taken a probiotic in the month prior to the episode, with lower Shannon, inverse Simpson, and Pielou indices than patients who had not taken probiotics (Supplementary Table 2). No significant differences in alpha diversity were seen in CDI, colonized patients, or R-CDI related to the presence or absence of toxin detected by Quick Check.
As for the NOCDI group, we found significant differences due to various factors, as follows: higher diversity and evenness values in patients over 69 years old; less diversity in patients who received antibiotics; higher diversity values in patients with underlying disease related to gut microbiota abnormalities and metabolic or cardiac disease; and lower diversity in patients with hematological disease, colectomy or ileostomy, colorectal cancer, or being immunosuppressed (Supplementary Table 2). Finally, in the R-CDI group, the group which presented the lowest microbiome diversity compared to the other CDI, colonized and healthy groups, we also found some differences within the R-CDI group. Patients presenting with colorectal cancer and colectomy or ileostomy had significantly higher diversity and evenness values than R-CDI patients without this condition, except for evenness in colorectal cancer (Supplementary Table 2).
We found significant differences in beta diversity between all the groups in general and in paired terms between all the groups except colonized patients and R-CDI (Figure 2). Regarding the homogeneity of the variance of the intra-patient samples, we found that the most homogenous patients were the healthy individuals, followed in decreasing order by CDI, R-CDI, colonized patients, and NOCDI patients, who were the most heterogeneous (Figure 2).
Figure 2 Beta- diversity. Left: Principal Coordinates Analysis (PCoA) based on unweighted UniFrac distances. Right: Non-metric multidimensional scaling (NMDS) plot based on the Bray-Curtis dissimilarity index. CDI, Clostridioides difficile infection; NOCDI, No C. difficile diarrhoea; RCDI, recurrent CDI; Colonized, patients colonized with C. difficile; Healthy, Healthy subjects.
Regarding the relative abundance of the different taxonomic groups, we found that the major phylum, in all groups except CDI, was Firmicutes (healthy, 51.13%; NOCDI, 41.42%; colonized, 49.06%; and R-CDI, 39.86%), followed by Bacteroidetes (healthy, 31.23%; NOCDI, 36.37%; colonized, 25.09%; and R-CDI, 26.03%); in the CDI patients, the most common phylum was Bacteroidetes (39.59%), followed by Firmicutes (37.53%) (p<0.001). Differences in relative abundance between the groups were significant for Actinobacteria, Bacteroidetes, Firmicutes, Fusobacteria, and Proteobacteria. In the healthy individuals, we found a higher proportion of Actinobacteria and a lower proportion of Proteobacteria than in the other groups (p<0.001). When we compared the CDI, colonized, NOCDI, and R-CDI groups, we still found significant differences in Actinobacteria, Bacteroidetes, Firmicutes, and Proteobacteria (Table 4).
Within the taxonomic family level, we found that Bacteroidaceae was the most abundant in the NOCDI, colonized, and CDI groups (NOCDI, 27.11%; colonized, 18.56%; and CDI, 29.99%), followed by Enterobacteriaceae (NOCDI, 15.29%; colonized, 15.26%; and CDI, 14.79%). However, in the healthy patients, the most abundant family was Lachnospiraceae (25.06%), followed by Bacteroidaceae (19.86%), and in the R-CDI patients, the most abundant was Enterobacteriaceae (23.06%), followed by Bacteroidaceae (20.14%). (Table 5) (Figure 3)
Figure 3 Heatmap of family relative abundance in each sample. CDI, Clostridioides difficile infection; NOCDI, Non C. difficile diarrhoea; RCDI, recurrent CDI; Colonized, patients colonized with C. difficile; Healthy, Healthy subjects.
When we reach the taxonomic level of genus, we find the following differences in abundance between the different groups (Figure 4).
Figure 4 Genera heatmap of Log2 Fold Change represent differentially abundance genus between groups. CDI, Clostridioides difficile infection; NOCDI, Non C. difficile diarrhoea; RCDI, recurrent CDI; Colonized, patients colonized with C. difficile; Healthy, Healthy subjects.
For CDI patients vs. healthy, we found a significantly lower abundance in Bifidobacterium, Collinsella, Slackia, Blautia, Butyrivibrio, Clostridium_XlVb, Coprococcus, Dorea, Fusicatenibacter, Ruminococcus, and Faecalibacterium, and a greater abundance of Staphylococcus, Enterococcus, Lactobacillus, Streptococcus, Parvimonas, Clostridium_XlVa, Robinsoniella, Peptostreptococcus, Clostridium_XVIII, Coprobacillus, Veillonella, Fusobacterium, Campylobacter, Proteus, Pseudomonas, and Akkermansia (all p–0.05).
For CDI vs. NOCDI, we found genera with significant differences between the two groups. Decreases were recorded for Dysgonomonas, Streptococcus, Clostridium_IV, Ruminococcus, Clostridium_XIVb, Megamonas, Acinetobacter, and Enterococcus. Increases were recorded for Blautia, Anaerostipes, Butyrivibrio, Clostridium_XlVa, Coprococcus, Robinsoniella, Butyricicoccus, Coprobacillus, Fusobacterium, Campylobacter, and Akkermansia (all p<0.05).
For CDI vs. colonized, we recorded a reduction in Faecalicoccus, Proteus, Weissella and an increase in Staphylococcus, Clostridium_XlVb, Coprococcus, Peptostreptococcus, Olsenella, Butyricicoccus, Robinsoniella, Megasphaera, and Alloprevotella (all p<0.05).
For colonized patients vs. NOCDI, we found significant differences, such as the decrease in the genera Clostridium XIVb, Coprococcus, Staphylococcus, and Megasphaera and a significant increase in Coprobacillus, Faecalicoccus, Weisella, and Fusobacterium (all p<0.05).
Of note, genera, such as Enterococcus, Fusobacterium, Proteus, Faecalicoccus, Veillonella, and Akkermansia and some Clostridium increased in all groups with respect to healthy individuals, and some of these genera maintained the same differences between the CDI and colonized groups vs. NOCDI. Other groups maintained the opposite pattern, and in the groups with C. difficile, several genera were less abundant, for example, Bifidobacterium, Collinsella, Olsenella, Blautia, Butyrivibrio, Slackia, Coprococcus, Fusicatenibacter, and Megamonas.
As for similarity between the OTUs of the different groups, we found that CDI and NOCDI shared the highest number of OTUs (4,523), followed by NOCDI and healthy individuals (3,296); the lowest values were found for R-CDI and healthy (1,172), colonized and healthy (1,128), and R-CDI and colonized (1,007).
The biomarker search was based on a linear discrimination analysis, which revealed various genera to be possible discriminating biomarkers for patients with high scores (> 3).
The potential biomarkers identified for colonization were Parabacteroides, Faecalicoccus, Flavonifractor, and Clostridium_XVIII. For CDI group, the biomarkers were Bacteroides, Proteus, Paraprevot ella, Butyrivibrio, Senegalimassilia, Holdemanela, Robinsoniella, and Eggerthellas. In R-CDI patients, they were Veillonella, Fusobacterium, Lactobacillus, Enterococcus, Clostridium_XIVa, and Clostridium_sensu_stricto. Finally, in the healthy patients, we identified, among others, Bifidobacterium, Blautia, Faecalibacterium, and Roseburia (Figures 5, 6).
Figure 5 List of possible genera biomarkers that enable discrimination between groups. Left (Linear discriminant analysis (LDA). Right Random Forest (RF)). CDI, Clostridioides difficile infection; NOCDI, Non C. difficile diarrhoea; RCDI, recurrent CDI; Colonized, patients colonized with C. difficile; Healthy, Healthy subjects.
Figure 6 Cladogram with taxa different abundance between groups CDI, Clostridioides difficile infection; NOCDI, Non C. difficile diarrhoea; RCDI, recurrent CDI; Colonized, patients colonized with C. difficile; Healthy, Healthy subjects.
We also performed a random forest analysis, which identified the same discriminating genera for each patient (Figure 5).
In the present study, we outlined the differences in the microbiota of five groups of patients (healthy, colonized, CDI, NOCDI, and R-CDI) from a large cohort, finding marked differences that could be useful as diagnostic markers of true CDI infection.
Several studies have characterized microbiota in patients with CDI, although the groups compared were very heterogeneous. Some studies based their control group on “healthy” individuals, which is a confusing term, since “healthy” can include patients with other diseases but not CDI or diarrhea, patients receiving different treatments for their underlying disease, persons without diarrhea who have not taken antibiotics, and even stool donors for fecal transplantation (Schubert et al., 2014; Milani et al., 2016; Amrane et al., 2019; Sánchez-Pellicer et al., 2021). We compared various groups, including both healthy individuals and patients with diarrhea in whom C. difficile has been ruled out but who share diseases, treatments, and other characteristics that may more closely resemble those of CDI patients.
The comparison with healthy individuals revealed a considerable difference in the state of the intestinal microbiota compared with patients with gastrointestinal disorders, whether due to C. difficile or not, namely, greater richness, alpha diversity, and evenness. These differences are also evident in beta diversity, where the healthy individuals group differs significantly from all the others.
It should also be noted that we did find differences in alpha diversity and richness between the CDI and NOCDI groups, in contrast with observations reported elsewhere, perhaps because our sample was larger than those of other studies (Sangster et al., 2016; Jeon et al., 2019; Herrera et al., 2021). Most studies comparing the microbiota of patients with CDI with that of patients with diarrhea due to other causes and with the microbiota of persons colonized by C. difficile include a low number of individuals for each study group (Zhang et al., 2015; Allegretti et al., 2016; Milani et al., 2016; Sangster et al., 2016; Jeon et al., 2019; Herrera et al., 2021). This observation is very important, since the intestinal microbiome is constantly changing due to factors such as diet, environmental factors, sports, and medication (Dominianni et al., 2015; Rashid et al., 2015; Mohr et al., 2020). Therefore, in order to obtain more reliable results, it is necessary to include a considerable number of persons in each group, as in our study.
Consistent with the literature, the lowest diversity and richness values were recorded for patients with R-CDI (Chang et al., 2008; Allegretti et al., 2016; Gazzola et al., 2020). Both our data and that of other authors show the existing damage to the microbiota or dysbiosis in patients with diarrhea due to C. difficile or other causes and that of patients colonized by C. difficile (Sangster et al., 2016; Sokol et al., 2018; Crobach et al., 2020; Sánchez-Pellicer et al., 2021; Wan et al., 2022). In addition, the greater damage to the microbiota in patients with repeated episodes of CDI is highlighted. In this study, we observed that patients with CDI and IBD presented less alpha diversity and evenness than CDI patients without IBD.
Interestingly, we found that among patients with diarrhea, there were markedly significant differences in beta diversity between those with diarrhea due to CDI and those with diarrhea due to other causes (NOCDI). Some authors have reported similar findings (Schubert et al., 2014; Sangster et al., 2016; Han et al., 2020), whereas others have not (Antharam et al., 2013; Jeon et al., 2019; Gazzola et al., 2020; Herrera et al., 2021). Moreover, we observed that beta diversity differed significantly in patients with CDI and patients colonized by C. difficile. This finding has not been reported in the few studies carried out that include these groups, probably owing to the limited number of samples analyzed (Han et al., 2019; Crobach et al., 2020; Sánchez-Pellicer et al., 2021).
In agreement with the literature (Crobach et al., 2020), we observed that alpha and beta diversity were significantly altered by specific CDI risk factors (antibiotic treatment in the previous month, previous episodes of CDI, treatment with proton pump inhibitors or probiotics in the previous month, nasogastric tube in the previous month), colorectal cancer, solid organ transplant, neoplasia, and hematological disease.
As for differences in abundance, we found that, except in patients with CDI, the most abundant phylum in all five groups was Firmicutes followed by Bacteroidetes. In the CDI group, this order was inverted, with Bacteroidetes being the most abundant phylum, as reported elsewhere (Antharam et al., 2013; Sánchez-Pellicer et al., 2021), although the opposite has also been observed (Zhang et al., 2015; Han et al., 2019; Han et al., 2020). These contradictions in the literature may be due to the different methodologies used for sample extraction, amplification, and analysis, together with the limited number of samples processed. Consistent with other observations in the literature, we also found a decrease in Actinobacteria and an increase in Proteobacteria in patients with CDI compared to healthy individuals (Amrane et al., 2019; Han et al., 2019; Han et al., 2020; Sánchez-Pellicer et al., 2021). When we assessed the genera by groups, we found that Ruminococcus, Clostridium_IV, Streptococcus, Acinetobacter, and Megamonas decreased in the CDI group compared to CDI. The opposite occurred Blautia and Coprococcus, which increased compared to NOCDI. Other genera that were more abundant in CDI compared to both healthy individuals and NOCDI were Lactobacillus, Clostridium_XIVa, Robinsoniella, Coprobacillus, Fusobacterium, Campylobacter, and Akkermansia. The decrease in butyrate-producing bacteria, such as Ruminococcus, and the increase in genera of lactic acid–producing bacteria, such as Blautia, Lactobacillus, and Fusobacterium, has been associated with an increased risk of CDI (Vakili et al., 2020).
Significant differences were found for the presence of genera observed in CDI patients versus NOCDI or those for CDI patients versus colonized patients. This enabled us to carry out an exhaustive search for microbial biomarkers that could help respond to the as yet unresolved need to identify diarrhea truly caused by C. difficile. Few studies have attempted to outline these differences.
Among the few studies comparing the intestinal microbiota of patients with CDI and C. difficile colonization, the number of patients included is lower than in ours, with very few significant differences between the two groups (Zhang et al., 2015; Han et al., 2019; Crobach et al., 2020; Sánchez-Pellicer et al., 2021). Furthermore, the study conducted by Han et al. did not discriminate between toxigenic and non-toxigenic C. difficile strains, meaning that the C. difficile study groups included patients with toxigenic and non-toxigenic C. difficile, thus making it difficult to interpret and extrapolate their results (Han et al., 2019). We identified a series of changes in the microbiota that enabled us to outline the differences between colonized patients and those with true CDI infection. In CDI patients, we observed two genera that decreased significantly, namely, Faecalicoccus and Proteus, both of which are increased in colonized patients. We also found a significant increase in Clostridium_XIVb, Coprococcus, Staphylococcus, and Robinsoniella among patients with true CDI. These differences could facilitate appropriate categorization of these groups of patients, which, as mentioned above, remains unresolved.
To the best of our knowledge, this is the first study to have carried out such a broad comparison to identify biomarkers that differentiate between so many subgroups of patients, especially between CDI patients and colonized patients. The few previous studies on this area focused on finding biomarkers to be able to distinguish between CDI and healthy individuals (Antharam et al., 2013), CDI and NOCDI (Schubert et al., 2014; Herrera et al., 2021), and CDI and R-CDI (Allegretti et al., 2016).
Our results were also consistent with those of previous studies. We observed a decrease in certain families or genera involved in the production of butyric acid, such as Ruminococcaceae (Faecalibacterium and Ruminococcus) and Lachnospiraceae (Blautia and Coprococcus), both in CDI patients and in colonized patients (Antharam et al., 2013; Zhang et al., 2015; Milani et al., 2016). For CDI and colonized patients, we also observed a decrease in Bifidobacterium, a genus with anti-inflammatory and antimicrobial properties. (Imaoka et al., 2008) We identified a series of genera that could be of use as biomarkers. These included Parabacteroides, Faecalicoccus, Flavonifractor, and Clostridium_XVIII for the colonized group, and Veillonella, Fusobacterium, Lactobacillus, Enterococcus, Clostridium_XIVa, and Clostridium_sensu_sricto for the R-CDI group. We also identified genera that better define true CDI infection, such as Bacteroides, Proteus, Paraprevotella, Robinsoniella, and Eggerthella.
Our study is limited by its single-center design, which requires our results to be validated in multicenter studies. However, as our sample is the largest microbiome analysis of CDI to date, our results can be considered robust. Basing our study on 5 different groups of patients enabled us to analyze the different microbiota profiles and thus better characterize those microbiota abnormalities caused solely by C. difficile infection. We also separated primary CDI from R-CDI, where the progressive damage that C. difficile produces in the intestinal microbiota is evident.
In conclusion, we found several genera that could be used as biomarkers to differentiate between C. difficile–colonized patients, true CDI episodes, and diarrhea due to other causes. Our approach will enable us to improve the diagnosis, management, and treatment of CDI. Further research is warranted.
The datasets presented in this study can be found in online repositories. The names of the repository/repositories and accession number(s) can be found below: https://www.ebi.ac.uk/ena, PRJEB57947.
The studies involving human participants were reviewed and approved by Ethics Committee of Hospital General Universitario Gregorio Marañón in Madrid (number MICRO.HGUGM.2016-029). Written informed consent to participate in this study was provided by the participants’ legal guardian/next of kin.
ER and EB designed the study. SV-C performed the collection of data and samples. SV-C and NG performed the data analysis. SV-C, ER, EB, MO, LA, MM, PM, contributed to data interpretation. SV-C wrote the first draft of the manuscript. SV-C, ER, EB, MO, LA, MM, PM, AF and LV, contributed with the intellectual content and approved the final draft. SV-C, ER, and EB had access to and verified the underlying study data. All authors had full access to all the data in the study and had final responsibility for the decision to submit for publication.
Ana I. Fernández, Carmen Moreno, Celso Arando, Darío García de Viedma, Elena Reigadas, Francisco Fernández-Avilés, Javier Bermejo, Javier Vaquero, Lorena Cussó, Manuel Desco, Mara Perellada, Marta Fernández, Nuria López, Rafael Correa, Silvia Vázquez-Cuesta.
This study was funded by Fondo de Investigaciones Sanitarias (FIS), Research Project number PI16/00490 and PI20/01381, PFIS grant number FI21/00310, and by the European Regional Development Fund (FEDER) “A way of making Europe”. The funder was not involved in the study design, collection, analysis, interpretation of data, the writing of this article or the decision to submit it for publication”. This study was funded by Fondo de Investigaciones Sanitarias (FIS), Research Project number PI16/00490 and PI20/01381, PFIS grant number FI21/00310, and by the European Regional Development Fund (FEDER) “A way of making Europe”.
We thank Thomas O’Boyle for his help in the preparation of the manuscript.
The authors declare that the research was conducted in the absence of any commercial or financial relationships that could be construed as a potential conflict of interest.
All claims expressed in this article are solely those of the authors and do not necessarily represent those of their affiliated organizations, or those of the publisher, the editors and the reviewers. Any product that may be evaluated in this article, or claim that may be made by its manufacturer, is not guaranteed or endorsed by the publisher.
The Supplementary Material for this article can be found online at: https://www.frontiersin.org/articles/10.3389/fcimb.2023.1130701/full#supplementary-material
Allegretti, J. R., Kearney, S., Li, N., Bogart, E., Bullock, K., Gerber, G. K., et al. (2016). Recurrent Clostridium difficile infection associates with distinct bile acid and microbiome profiles. Aliment Pharmacol. Ther. 43 (11), 1142–1153. doi: 10.1111/apt.13616
Amrane, S., Hocquart, M., Afouda, P., Kuete, E., Pham, T. P., Dione, N., et al. (2019). Metagenomic and culturomic analysis of gut microbiota dysbiosis during Clostridium difficile infection. Sci. Rep. 9 (1), 12807. doi: 10.1038/s41598-019-49189-8
Antharam, V. C., Li, E. C., Ishmael, A., Sharma, A., Mai, V., Rand, K. H., et al. (2013). Intestinal dysbiosis and depletion of butyrogenic bacteria in Clostridium difficile infection and nosocomial diarrhea. J. Clin. Microbiol. 51 (9), 2884–2892. doi: 10.1128/jcm.00845-13
Bignardi, G. E. (1998). Risk factors for Clostridium difficile infection. J. Hosp Infect. 40 (1), 1–15. doi: 10.1016/s0195-6701(98)90019-6
Britton, R. A., Young, V. B. (2014). Role of the intestinal microbiota in resistance to colonization by Clostridium difficile. Gastroenterology 146 (6), 1547–1553. doi: 10.1053/j.gastro.2014.01.059
Chang, J. Y., Antonopoulos, D. A., Kalra, A., Tonelli, A., Khalife, W. T., Schmidt, T. M., et al. (2008). Decreased diversity of the fecal microbiome in recurrent Clostridium difficile-associated diarrhea. J. Infect. Dis. 197 (3), 435–438. doi: 10.1086/525047
Charlson, M. E., Pompei, P., Ales, K. L., MacKenzie, C. R. (1987). A new method of classifying prognostic comorbidity in longitudinal studies: development and validation. J. Chronic Dis. 40 (5), 373–383. doi: 10.1016/0021-9681(87)90171-8
Crobach, M. J. T., Ducarmon, Q. R., Terveer, E. M., Harmanus, C., Sanders, I., Verduin, K. M., et al. (2020). The bacterial gut microbiota of adult patients infected, colonized or noncolonized by Clostridioides difficile. Microorganisms 8 (5). doi: 10.3390/microorganisms8050677
Dethlefsen, L., Huse, S., Sogin, M. L., Relman, D. A. (2008). The pervasive effects of an antibiotic on the human gut microbiota, as revealed by deep 16S rRNA sequencing. PloS Biol. 6 (11), e280. doi: 10.1371/journal.pbio.0060280
Dominianni, C., Sinha, R., Goedert, J. J., Pei, Z., Yang, L., Hayes, R. B., et al. (2015). Sex, body mass index, and dietary fiber intake influence the human gut microbiome. PloS One 10 (4), e0124599. doi: 10.1371/journal.pone.0124599
Dubberke, E. R., Olsen, M. A. (2012). Burden of Clostridium difficile on the healthcare system. Clin. Infect. Dis. 55 Suppl 2, S88–S92. doi: 10.1093/cid/cis335
Gao, L., Maidment, I., Matthews, F. E., Robinson, L., Brayne, C., Study, M.R.C.C.F.a.A (2018). Medication usage change in older people (65+) in England over 20 years: findings from CFAS I and CFAS II. Age Ageing 47 (2), 220–225. doi: 10.1093/ageing/afx158
Gazzola, A., Panelli, S., Corbella, M., Merla, C., Comandatore, F., De Silvestri, A., et al. (2020). Microbiota in Clostridioides difficile-Associated diarrhea: Comparison in recurrent and non-recurrent infections. Biomedicines 8 (9). doi: 10.3390/biomedicines8090335
Han, S. H., Yi, J., Kim, J. H., Lee, S., Moon, H. W. (2019). Composition of gut microbiota in patients with toxigenic Clostridioides (Clostridium) difficile: comparison between subgroups according to clinical criteria and toxin gene load. PloS One 14 (2), e0212626. doi: 10.1371/journal.pone.0212626
Han, S. H., Yi, J., Kim, J. H., Moon, A. H. (2020). Investigation of intestinal microbiota and fecal calprotectin in non-toxigenic and toxigenic Clostridioides difficile colonization and infection. Microorganisms 8 (6). doi: 10.3390/microorganisms8060882
Heimann, S. M., Cruz Aguilar, M. R., Mellinghof, S., Vehreschild, M. (2018). Economic burden and cost-effective management of Clostridium difficile infections. Med. Mal Infect. 48 (1), 23–29. doi: 10.1016/j.medmal.2017.10.010
Herrera, G., Vega, L., Patarroyo, M. A., Ramírez, J. D., Muñoz, M. (2021). Gut microbiota composition in health-care facility-and community-onset diarrheic patients with Clostridioides difficile infection. Sci. Rep. 11 (1), 10849. doi: 10.1038/s41598-021-90380-7
Imaoka, A., Shima, T., Kato, K., Mizuno, S., Uehara, T., Matsumoto, S., et al. (2008). Anti-inflammatory activity of probiotic Bifidobacterium: enhancement of IL-10 production in peripheral blood mononuclear cells from ulcerative colitis patients and inhibition of IL-8 secretion in HT-29 cells. World J. Gastroenterol. 14 (16), 2511–2516. doi: 10.3748/wjg.14.2511
Jeon, Y. D., Ann, H. W., Lee, W. J., Kim, J. H., Seong, H., Ahn, J. Y., et al. (2019). Characteristics of faecal microbiota in Korean patients with Clostridioides difficile-associated diarrhea. Infect. Chemother. 51 (4), 365–375. doi: 10.3947/ic.2019.51.4.365
Jernberg, C., Löfmark, S., Edlund, C., Jansson, J. K. (2007). Long-term ecological impacts of antibiotic administration on the human intestinal microbiota. Isme J. 1 (1), 56–66. doi: 10.1038/ismej.2007.3
Jernberg, C., Löfmark, S., Edlund, C., Jansson, J. K. (2010). Long-term impacts of antibiotic exposure on the human intestinal microbiota. Microbiol. (Reading) 156 (Pt 11), 3216–3223. doi: 10.1099/mic.0.040618-0
Kelly, C. P., Chen, X., Williams, D., Xu, H., Cuddemi, C. A., Daugherty, K., et al. (2020). Host immune markers distinguish Clostridioides difficile infection from asymptomatic carriage and non-c. difficile diarrhea. Clin. Infect. Dis. 70 (6), 1083–1093. doi: 10.1093/cid/ciz330
McCabe, W. R., Jackson, G. G. (1962). Gram-negative bacteremia: I. etiology and ecology. Arch. Internal Med. 110 (6), 847–855. doi: 10.1001/archinte.1962.03620240029006
McDonald, L. C., Gerding, D. N., Johnson, S., Bakken, J. S., Carroll, K. C., Coffin, S. E., et al. (2018). Clinical practice guidelines for Clostridium difficile infection in adults and children: 2017 update by the infectious diseases society of America (IDSA) and society for healthcare epidemiology of America (SHEA). Clin. Infect. Dis. 66 (7), 987–994. doi: 10.1093/cid/ciy149
Milani, C., Ticinesi, A., Gerritsen, J., Nouvenne, A., Lugli, G. A., Mancabelli, L., et al. (2016). Gut microbiota composition and Clostridium difficile infection in hospitalized elderly individuals: a metagenomic study. Sci. Rep. 6, 25945. doi: 10.1038/srep25945
Mohr, A. E., Jäger, R., Carpenter, K. C., Kerksick, C. M., Purpura, M., Townsend, J. R., et al. (2020). The athletic gut microbiota. J. Int. Soc. Sports Nutr. 17 (1), 24. doi: 10.1186/s12970-020-00353-w
Ng, K. K., Reinert, Z. E., Corver, J., Resurreccion, D., Hensbergen, P. J., Prescher, J. A. (2021). A bioluminescent sensor for rapid detection of PPEP-1, a Clostridioides difficile biomarker. Sensors (Basel) 21 (22). doi: 10.3390/s21227485
Odamaki, T., Kato, K., Sugahara, H., Hashikura, N., Takahashi, S., Xiao, J. Z., et al. (2016). Age-related changes in gut microbiota composition from newborn to centenarian: a cross-sectional study. BMC Microbiol. 16, 90. doi: 10.1186/s12866-016-0708-5
Planche, T., Wilcox, M. H. (2015). Diagnostic pitfalls in Clostridium difficile infection. Infect. Dis. Clin. North Am. 29 (1), 63–82. doi: 10.1016/j.idc.2014.11.008
Rashid, M. U., Zaura, E., Buijs, M. J., Keijser, B. J., Crielaard, W., Nord, C. E., et al. (2015). Determining the long-term effect of antibiotic administration on the human normal intestinal microbiota using culture and pyrosequencing methods. Clin. Infect. Dis. 60 Suppl 2, S77–S84. doi: 10.1093/cid/civ137
Reigadas Ramirez, E., Bouza, E. S. (2018). Economic burden of Clostridium difficile infection in European countries. Adv. Exp. Med. Biol. 1050, 1–12. doi: 10.1007/978-3-319-72799-8_1
R Core Team. R: A language and environment for statistical computing; r foundation for statistical computing. (Vienna, Austria) (2021). Available online: https://www.R-project.org/
Rupnik, M., Wilcox, M. H., Gerding, D. N. (2009). Clostridium difficile infection: new developments in epidemiology and pathogenesis. Nat. Rev. Microbiol. 7 (7), 526–536. doi: 10.1038/nrmicro2164
Sánchez-Pellicer, P., Navarro-López, V., González-Tamayo, R., Llopis-Ruiz, C., Núñez-Delegido, E., Ruzafa-Costas, B., et al. (2021). Descriptive study of gut microbiota in infected and colonized subjects by Clostridioides difficile. Microorganisms 9 (8). doi: 10.3390/microorganisms9081727
Sandlund, J., Davies, K., Wilcox, M. H. (2020). Ultrasensitive Clostridioides difficile toxin testing for higher diagnostic accuracy. J. Clin. Microbiol. 58 (6). doi: 10.1128/jcm.01913-19
Sangster, W., Hegarty, J. P., Schieffer, K. M., Wright, J. R., Hackman, J., Toole, D. R., et al. (2016). Bacterial and fungal microbiota changes distinguish C. difficile infection from other forms of diarrhea: Results of a prospective inpatient study. Front. Microbiol. 7. doi: 10.3389/fmicb.2016.00789
Schubert, A. M., Rogers, M. A., Ring, C., Mogle, J., Petrosino, J. P., Young, V. B., et al. (2014). Microbiome data distinguish patients with Clostridium difficile infection and non-C. difficile-associated diarrhea from healthy controls. MBio 5 (3), e01021–e01014. doi: 10.1128/mBio.01021-14
Sokol, H., Jegou, S., McQuitty, C., Straub, M., Leducq, V., Landman, C., et al. (2018). Specificities of the intestinal microbiota in patients with inflammatory bowel disease and Clostridium difficile infection. Gut Microbes 9 (1), 55–60. doi: 10.1080/19490976.2017.1361092
Thanissery, R., Winston, J. A., Theriot, C. M. (2017). Inhibition of spore germination, growth, and toxin activity of clinically relevant C. difficile strains by gut microbiota derived secondary bile acids. Anaerobe 45, 86–100. doi: 10.1016/j.anaerobe.2017.03.004
Theriot, C. M., Bowman, A. A., Young, V. B. (2016). Antibiotic-induced alterations of the gut microbiota alter secondary bile acid production and allow for Clostridium difficile spore germination and outgrowth in the Large intestine. mSphere 1 (1). doi: 10.1128/mSphere.00045-15
Theriot, C. M., Young, V. B. (2015). Interactions between the gastrointestinal microbiome and Clostridium difficile. Annu. Rev. Microbiol. 69, 445–461. doi: 10.1146/annurev-micro-091014-104115
Vakili, B., Fateh, A., Asadzadeh Aghdaei, H., Sotoodehnejadnematalahi, F., Siadat, S. D. (2020). Characterization of gut microbiota in hospitalized patients with Clostridioides difficile infection. Curr. Microbiol. 77 (8), 1673–1680. doi: 10.1007/s00284-020-01980-x
van Prehn, J., Reigadas, E., Vogelzang, E. H., Bouza, E., Hristea, A., Guery, B., et al. (2021). European Society of clinical microbiology and infectious diseases: 2021 update on the treatment guidance document for Clostridioides difficile infection in adults. Clin. Microbiol. Infect. 27, S1–S21. doi: 10.1016/j.cmi.2021.09.038
Wan, J., Zhang, Y., He, W., Tian, Z., Lin, J., Liu, Z., et al. (2022). Gut microbiota and metabolite changes in patients with ulcerative colitis and Clostridioides difficile infection. Front. Microbiol. 13. doi: 10.3389/fmicb.2022.802823
Whittaker, R. J., Willis, K. J., Field, R. (2001). Scale and species richness: towards a general, hierarchical theory of species diversity. J. Biogeogr. 28 (4), 453–470. doi: 10.1046/j.1365-2699.2001.00563.x
Keywords: CDI, C. difficile, microbiome, R-CDI, 16S rRNA
Citation: Vázquez-Cuesta S, Villar L, García NL, Fernández AI, Olmedo M, Alcalá L, Marín M, Muñoz P, Bouza E and Reigadas E (2023) Characterization of the gut microbiome of patients with Clostridioides difficile infection, patients with non–C. difficile diarrhea, and C. difficile–colonized patients. Front. Cell. Infect. Microbiol. 13:1130701. doi: 10.3389/fcimb.2023.1130701
Received: 29 December 2022; Accepted: 29 March 2023;
Published: 12 April 2023.
Edited by:
Benoit Chassaing, Institut National de la Santé et de la Recherche Médicale (INSERM), FranceReviewed by:
Vijay Antharam, Methodist University, United StatesCopyright © 2023 Vázquez-Cuesta, Villar, García, Fernández, Olmedo, Alcalá, Marín, Muñoz, Bouza and Reigadas. This is an open-access article distributed under the terms of the Creative Commons Attribution License (CC BY). The use, distribution or reproduction in other forums is permitted, provided the original author(s) and the copyright owner(s) are credited and that the original publication in this journal is cited, in accordance with accepted academic practice. No use, distribution or reproduction is permitted which does not comply with these terms.
*Correspondence: Silvia Vázquez-Cuesta, c2lsdmlhdmF6cXVlei5sYWJAZ21haWwuY29t
Disclaimer: All claims expressed in this article are solely those of the authors and do not necessarily represent those of their affiliated organizations, or those of the publisher, the editors and the reviewers. Any product that may be evaluated in this article or claim that may be made by its manufacturer is not guaranteed or endorsed by the publisher.
Research integrity at Frontiers
Learn more about the work of our research integrity team to safeguard the quality of each article we publish.