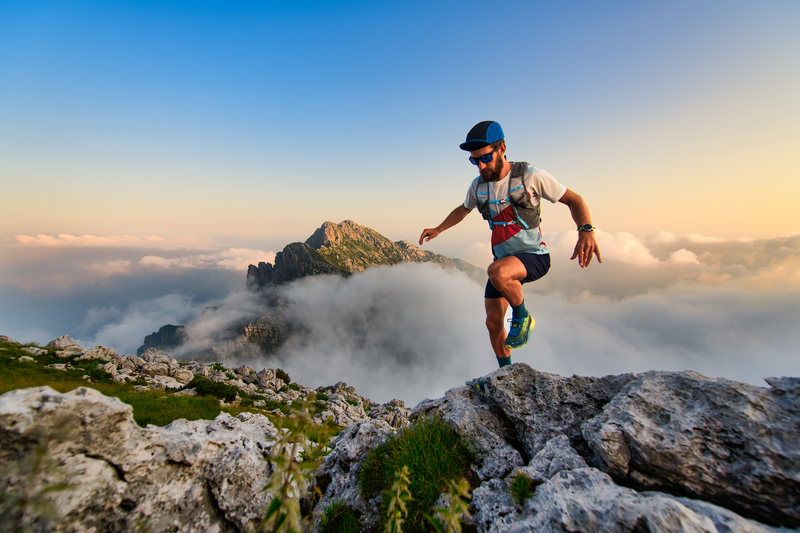
94% of researchers rate our articles as excellent or good
Learn more about the work of our research integrity team to safeguard the quality of each article we publish.
Find out more
ORIGINAL RESEARCH article
Front. Cell. Infect. Microbiol. , 01 May 2023
Sec. Clinical Microbiology
Volume 13 - 2023 | https://doi.org/10.3389/fcimb.2023.1118424
This article is part of the Research Topic Finding Potential Approaches for Tuberculosis Prevention and Treatment View all 9 articles
Purpose: The development of tuberculosis and inflammatory status are closely related. The aim of this study was to investigate the prognostic value of inflammatory biomarkers in patients with rifampicin/multidrug-resistant tuberculosis (RR/MDR-TB).
Patients and methods: This study recruited 504 patients with RR/MDR-TB from Wuhan Jinyintan Hospital. A total of 348 RR/MDR patients from January 2017 to December 2019 were defined as training set, the rest of patients as validation set. The patients were divided into three-risk degrees according to the levels of inflammatory biomarkers (median, 85th percentile). Kaplan-Meier curve and log-rank test were used to assess survival differences among the groups. Cox proportion risk regression was used to identify risk factors for RR/MDR-TB mortality.
Results: In training set, cox proportion risk regression analysis showed that high age (≥60 years) [OR (95%CI):1.053(1.03188-1.077)], smoking [OR (95%CI):2.206(1.191-4.085)], and bronchiectasia [OR (95%CI):2.867(1.548-5.311)] were prognostic factors for RR/MDR-TB patients. In addition, lower survival rates were observed in high CAR group [OR (95%CI):1.464(1.275-1.681)], high CPR group[OR (95%CI):1.268(1.101-1.459)], high CLR group[OR (95%CI):1.004(1.002-1.005)], high NLR group[OR (95%CI):1.103(1.069-1.139)], high PLR group[OR (95%CI):1.003(1.002-1.004)], and high MLR group[OR (95%CI):3.471(2.188-5.508)].Furthermore, AUCs of age, smoking, bronchiectasia, CAR, CPR, CLR, NLR, PLR, and MLR for predicting mortality in RR/MDR-TB patients were 0.697(95%CI:0.618-0.775), 0.603(95%CI:0.512-0.695), 0.629(95%CI:0.538-0.721), 0.748(95%CI:0.675-0.821, P<0.05), 0.754(95%CI:0.683-0.824, P<0.05), 0.759(95%CI:0.689-0.828, P<0.05), 0.789(95%CI:0.731-0.846, P<0.05), 0.740(95%CI:0.669-0.812, P<0.05), and 0.752(95%CI:0.685-0.819, P<0.05), respectively. Importantly, the AUC of predicting mortality of combination of six inflammatory biomarkers [0.823 (95%CI:0.769-0.876)] is higher than any single inflammatory biomarkers. Additionally, the similar results are also obtained in the validation set.
Conclusion: Inflammatory biomarkers could predict the survival status of RR/MDR-TB patients. Therefore, more attention should be paid to the level of inflammatory biomarkers in clinical practice.
Tuberculosis (TB) is a global health concern with high morbidity and mortality. Compared to drug-sensitive TB (DS-TB), RR/MDR-TB typically has longer treatment duration, poorer treatment outcomes, and a higher risk of mortality (Chung-Delgado et al., 2015). According to the World Health Organization (WHO) Global Tuberculosis Report 2021, mortality among RR/MDR-TB patients had increased worldwide with the COVID-19 pandemic (World Health, 2021).
Currently, an increasing number of studies are focused on finding valid markers to predict mortality and exploring risk factors for death in TB patients to improve treatment and reduce mortality, especially in RR/MDR-TB patients. Obviously, Scholars had made some progress in it. It was reported that extrapulmonary TB, age, sex, weight, and comorbidities such as diabetes mellitus (DM) and HIV strongly influence the prognosis of TB (Balaky et al., 2019; Limenih and Workie, 2019; Xie et al., 2020; Machmud et al., 2021). For instance, patients with higher age or extrapulmonary TB tended to have higher mortality (Balaky et al., 2019), and significantly lower survival rates were observed in MDR-TB patients with HIV infection (Machmud et al., 2021). However, previous studies have largely concentrated on clinical features, and few on inflammatory biomarkers. In fact, the inflammatory state was closely related to the development and progression of TB. The release of inflammatory factors along with the formation of an inflammatory microenvironment provided favorable conditions for tissue destruction, bacterial transmission (Amaral et al., 2021), and development and progression of TB (Krug et al., 2021). Fausto Ciccacci’s colleagues and himself reported that high-sensitivity C-reactive protein could predict early mortality in HIV-positive TB patients (Ciccacci et al., 2021).
Although the clinical characteristics associated with mortality in TB patients have been extensively studied, their actual prognostic value was unsatisfactory due to low predictive accuracy, which may limit their clinical application. Therefore, the discovery of novel reliable prognostic biomarkers for patients with RR/MDR-TB is essential for individualized treatment and improved survival. Inexpensive and readily available inflammatory biomarkers, such as C-reactive protein to albumin ratio (CAR), C-reactive protein to prealbumin ratio (CPR), C-reactive protein to lymphocyte ratio (CLR), neutrophil to lymphocyte ratio (NLR), platelet to lymphocyte ratio (PLR) and monocyte to lymphocyte ratio (MLR), have been widely described in various diseases to predict prognosis, especially in malignant tumors. These inflammatory markers may be superior to clinical features for mortality prediction in RR/MDR-TB patients since they exhibit better prognostic value in malignant tumors than clinical features such as TNM classification (Miura et al., 2021).
This study attempted to investigate the effect of inflammatory biomarkers on the prognosis of patients with RR/MDR-TB during anti-tuberculosis treatment (ATT).
We retrospectively collected 504 patients diagnosed with RR/MDR-TB from January 2015 to December 2019 at the Wuhan Jinyintan Hospital, Tongji Medical College, Huazhong University of Science and Technology (Infectious Disease Hospital). Smear microscopy, mycobacterium tuberculosis culture, or GeneXpert MTB/RIF were used for TB diagnosis. RR/MDR-TB was confirmed by drug susceptibility testing (DST). Inclusion criteria were as follows: (1) positive Smear microscopy, mycobacterium tuberculosis culture, or GeneXpert MTB/RIF results; (2) At least rifampin resistance. (3) aged 18 years or older. Exclusion criteria were as follows: (1) dead before treatment; (2) infection of non-tuberculous; (3) refusal of ATT.
1. Division of data set: to ensure the reproducibility of the results, the data of RR/MDR patients from January 2017 to December 2019 in Wuhan Jinyintan Hospital were defined as the training set. And the data from January 2015 to December 2016 (validation set) were applied to validate the results of the training set.
2. Follow-up: The 24-month follow-up was performed for all RR/MDR-TB patients, and the primary outcome of interest was mortality. Censoring was defined as stopping treatment (including cured, completed treatment, treatment failure) or loss to follow-up. The survival time of RR/MDR-TB patients was defined as the date of diagnosis to the date of death, stopping treatment, or loss to follow-up. Definitions of death, cure, completion of treatment, treatment failure, and loss to follow-up are provided in Supplementary Materials.
3. Evaluation and validation of prognostic value: based on the 24-month follow-up results, the RR/MDR-TB patients were divided into two groups, namely mortality group and survival group. RR/MDR patients in survival group were defined as stopping treatment, or loss to follow-up.
In the training set, student’s t-test was used to analyze the difference of the inflammatory biomarkers between mortality group and survival group. Cox proportion risk regression was used for investigating risk factors of mortality. Additionally, all patients were risk stratified into three groups according to the levels of inflammatory markers (median and 85th percentile): high-risk group, middle-risk group and low-risk group. Among groups, Kaplan-Meier curve and log-rank test were used for survival analyses. Receiver operating characteristic (ROC) curve was used for assessing the predictive value of the inflammatory biomarkers on prognosis of RR/MDR-TB patients. To further investigate the prognostic value of inflammatory biomarkers, we combined the six inflammatory biomarkers to assess the predictive power of mortality by calculating their AUCs. All analyses were validated in the validation set.
The baseline demographics and clinical data (such as radiological findings, laboratory test results, symptoms, treatment history, et al.) were collected through the electronic medical record system (EMRS). The follow-up outcomes were obtained from the national electronic case registry. Inflammatory biomarkers were calculated according to the following formula: CAR: C-reactive protein (mg/L) to albumin (g/L) Ratio; CPR: C-reactive protein (mg/L) to prealbumin (mg/L) ratio; CLR: C-reactive protein (mg/L) to lymphocytes (*10^9/L) ratio; NLR: neutrophils (*10^9/L) to lymphocytes (*10^9/L) ratio; PLR: platelets (*10^9/L) to lymphocytes (*10^9/L) ratio; MLR: monocytes (*10^9/L) to lymphocytes (*10^9/L) ratio.
Variables were presented as number with percentage (categorical variables), or mean ± standard deviation (SD)/median (interquartile range, IQR) (continuous variables). Among-groups, differences of continuous variables were compared using student’s t-test or one-way analysis of variance (ANOVA), while categorical variables were assessed by chi-square test or Fisher’s exact test. Cox proportional risk regression was used to investigate risk factors for mortality of patients with RR/MDR-TB. Kaplan-Meier curve and the log-rank test were used for survival analysis. Receiver operating characteristic (ROC) curve was used to assess the predictive value of inflammatory biomarkers on the prognosis of RR/MDR-TB patients.
All statistical analyses were performed by RStudio software (version 4.20). The main R packages included “survival” package, “survminer” package, and “ROCR” package. Two-sided P<0.05 was considered statistically significant.
A total of 504 RR/MDR-TB patients diagnosed and treated at the Wuhan Jinyintan Hospital from January 2015 to December 2019 were enrolled in this study. There were 348 patients in the training set and 156 patients in the validation set. As shown in the Table 1, treatment outcomes and drug sensitivity have a significant difference among two sets. Mortality rates and XDR-TB percentages are declining over time, meaning that the management and treatment of DS-TB are improving compared to the past. There are no differences between training and validation sets in terms of age, gender, follow-up time, smoking, drinking, pulmonary imaging, underlying condition or illness, and inflammatory biomarkers, suggesting that the data from the training set and validation sets are comparable and can be used for mutual validation. Additionally, Supplementary Tables 1-6 showed the baseline demographic and clinical characteristics in three risk-degrees of inflammatory biomarkers in training set.
Table 1 Demographic, clinical, and laboratory characteristics of the training set and validation set.
In training set, age[OR(95%CI):1.053(1.03188-1.077), P<0.05], smoking [OR(95%CI):2.206(1.191-4.085), P<0.05], drinking [OR(95%CI):2.581(1.321-5.403), P<0.05], bronchiectasia [OR(95%CI):2.867(1.548-5.311), P<0.05], pulmonary cavity [OR(95%CI):2.610(1.159-5.877), P<0.05], and pleural effusion [OR(95%CI):2.028(1.019-4.035)] were risk factors for mortality in RR/MDR-TB patients (Table 2). In addition, inflammatory biomarkers also had prognostic value in RR/MDR-TB patients (Table 2), such as CAR [OR (95% CI): 1.464 (1.275-1.681), P<0.05], CPR [OR (95% CI): 1.268 (1.101-1.459), P<0.05], CLR [OR (95% CI): 1.004 (1.002-1.005), P<0.05], NLR [OR (95% CI): 1.103 (1.069-1.139), P<0. 05], PLR [OR (95% CI): 1.003 (1.002-1.004), P<0.05], and MLR [OR (95%CI): 3.471 (2.188-5.508), P<0.05]. Importantly, the results of the validation set were consistent with those of the training set (Supplementary Table 7).
As shown in Figure 1, mortality rates were significantly higher in the bronchiectasia, pulmonary cavity, pleural effusion, smoking, and drinking groups than those in the non-exposure groups (log-rank test, all P value < 0.05). In addition, the mortality rate in the middle/low age group was significantly lower than that in the high age group (>60 years old) (log-rank test, all P value < 0.05).
Figure 1 24-month survival rate of RR/MDR-TB patients according to the levels of clinical characteristics in training set. (A) The survival analysis according to age. (B) The survival analysis according to smoking. (C) The survival analysis according to Drinking. (D) The survival analysis according to Bronchiectasia. (E) The survival analysis according to Pulmonary cavity. (F) The survival analysis according to Pleural effusion.
Based on inflammatory biomarkers level (median and 85th percentile), patients with RR/MDR-TB were classified into three risk groups. The cut-off value for each inflammatory biomarker were detailed in the Supplementary Table 8. Figure 2 showed that the mortality rates in the high-risk group, including CAR (A), CPR (B), CLR (C), NLR (D), PLR (E), and MLR (F), were significantly higher than those in the middle/low-risk group (log-rank test, all P value < 0.01). Likewise, the middle-risk group has a higher mortality rate than in the low-risk group (log-rank test, all P value < 0.01).
Figure 2 24-month survival rate of RR/MDR-TB patients according to risk stratification of inflammatory biomarkers in training set. (A) The survival analysis according to risk stratification of CAR. (B) The survival analysis according to risk stratification of CPR. (C) The survival analysis according to risk stratification of CLR. (D) The survival analysis according to risk stratification of NLR. (E) The survival analysis according to risk stratification of PLR. (F) The survival analysis according to risk stratification of MLR.
To further explore the predictive value of clinical features and inflammatory markers in RR/MDR-TB prognosis, ROC curve analysis was performed. Figure 3A showed that the AUCs of age, smoking, and bronchiectasia were 0.697(95%CI:0.618-0.775, P<0.05), 0.603(95%CI:0.512-0.695, P<0.05), 0.629(95%CI:0.538-0.721, P<0.05), respectively. Unfortunately, pulmonary cavity, pleural effusion, and drinking had poor predictive value (P>0.05). In addition, the AUCs of CAR, CPR, CLR, NLR, PLR, and MLR were 0.748(95%CI:0.675-0.821, P<0.05), 0.754(95%CI:0.683-0.824, P<0.05), 0.759(95%CI:0.689-0.828, P<0.05), 0.789(95%CI:0.731-0.846, P<0.05), 0.740(95%CI:0.669-0.812, P<0.05), 0.752(95%CI:0.685-0.819, P<0.05), respectively (Figure 3B). These results indicated that inflammatory biomarkers had a better predictive value than clinical features, and could be used as prognostic biomarkers of RR/MDR-TB patients. Importantly, the AUC for the combination of the inflammatory biomarkers (AUC: 0.823, 95% CI: 0.769-0.876, P<0.05) was higher than that of any inflammatory biomarkers, which indicated a more accurate prediction (Figure 3B).
Figure 3 The predictive value of clinical characteristics and inflammatory biomarkers on prognosis in RR/MDR-TB patients. (A) Clinical characteristics in training set. The area under the ROC curve (AUC) values in the age, smoking, drinking, bronchiectasia, pulmonary cavity, and pleural effusion were 0.697(95%confidence interval: 0.618-0.775, P<0.05), 0.603(95% confidence interval: 0.512-0.695, P<0.05), 0.577(95%confidence interval: 0.479-0.676, P>0.05), 0.629(95%confidence interval: 0.538-0.721, P<0.05), 0.593(95%confidence interval: 0.508-0.678, P>0.05), and 0.516 (95%confidence interval: 0.463-0.658, P>0.05), respectively. (B) Inflammatory markers in training set. The area under the ROC curve (AUC) values in the CAR, CPR, CLR, NLR,PLR, MLR, and combination were 0.748(95%confidence interval: 0.675-0.821, P<0.05), 0.754(95% confidence interval: 0.683-0.824, P<0.05), 0.759(95%confidence interval: 0.689-0.828, P<0.05), 0.789(95%confidence interval: 0.731-0.846, P<0.05), 0.740(95%confidence interval: 0.669-0.812, P<0.05), 0.752(95%confidence interval: 0.685-0.819, P<0.05), and 0.823(95%confidence interval: 0.769-0.876, P<0.05), respectively. (C) Clinical features in validation set. The area under the ROC curve (AUC) values in the age, smoking, drinking, bronchiectasia, pulmonary cavity, and pleural effusion were 0.642(95%confidence interval: 0.544-0.739, P<0.05), 0.655(95% confidence interval: 0.547-0.764, P<0.05), 0.588(95%confidence interval: 0.471-0.705, P>0.05), 0.668(95%confidence interval: 0.557-0.779, P<0.05), 0.663(95%confidence interval: 0.567-0.760, P>0.05), and 0.639(95%confidence interval: 0.522-0.755, P>0.05), respectively. (D) Inflammatory markers in validaiton set. The area under the ROC curve (AUC) values in the CAR, CPR, CLR, NLR,PLR, MLR, and combination were 0.722(95%confidence interval: 0.627-0.816, P<0.05), 0.750(95% confidence interval: 0.657-0.843, P<0.05), 0.760(95%confidence interval: 0.671-0.850, P<0.05), 0.749(95%confidence interval: 0.649-0.849, P<0.05), 0.667(95%confidence interval: 0.561-0.774, P<0.05), and 0.686(95%confidence interval: 0.571-0.850, P<0.05), 0.771(95%confidence interval:0.674-0.869, P<0.05), respectively.
The results of the prognostic analysis in the validation set were similar to those in the training set. Bronchiectasia, pulmonary cavity, pleural effusion, smoking, and drinking, age, and the different risk-degrees of inflammatory biomarkers also reflected well the prognosis of RR/MDR-TB patients (Supplementary Figures 1 and 2). Furthermore, single inflammatory biomarkers and combined inflammatory biomarkers also presented good predictive power in the validation set, as evidenced by AUCs of 0.722(CAR:95%CI:0.627-0.816), 0.750(CPR:95%CI:0.657-0.843), 0.760(CLR:95%CI:0.671-0.850), 0.749(NLR:95%CI:0.649-0.849), 0.667(CAR:95%CI:0.561-0.774), 0.686(MLR:95%CI:0.571-0.802), and 0.771(combination:95%CI:0.674-0.869), respectively (Figure 3C). Indeed, the AUCs of inflammatory biomarkers were higher than clinical features [age (AUC:0.642, 95%CI:0.544-0.739), smoking (AUC:0.655, 95%CI:0.547-0.764), drinking (AUC:0.588, 95%CI:0.471-0.705), bronchiectasia (AUC:0.668, 95%CI:0.557-0.779), pulmonary cavity (AUC:0.663, 95%CI:0.567-0.760), pleural effusion (AUC:0.639, 95%CI:0.522-0.755)] (Figure 3D).
Overall, we observed 24-month mortality rates of 12.0% in the training set and 20.5% in the validation set for patients with RR/MDR-TB. The rates in this study were higher than the that reported in previous literature for TB patients including both RR/MDR-TB and DS-TB (Khazaei et al., 2005; Martínez-Rodríguez et al., 2016), as explained by a higher mortality rate in RR/MDR-TB than DS-TB (Chung-Delgado et al., 2015).
Previous studies have elucidated in TB patients the prognostic value of various factors such as age, sex, residence, smoking, first sputum result and HIV status (Limenih and Workie, 2019; Xie et al., 2020; Carter et al., 2021). It is crucial for TB patients to find valid mortality predictors, especially in RR/MDR-TB patients, to help individualize management and reduce mortality. Recently, increasing attention have been paid to the interaction between TB and inflammation. The release of inflammatory factors and the formation of an inflammatory micro-environment provide a favorable environment for tissue destruction, the spread of bacteria (Amaral et al., 2021), and development and progression of TB (Krug et al., 2021). To date, the prognostic value of inflammatory biomarkers in TB patients has been rarely reported. A retrospective clinical study found that high-sensitivity C-reactive protein could predict early mortality in TB patients co-infected with HIV (Ciccacci et al., 2021). In our study, high levels of CAR, CPR, CLR, NLR, PLR, and MLR were risk factors for mortality in RR/MDR-TB patients.
C-reactive protein is most commonly used non-specific inflammatory biomarker in clinical practice, which can reflect the body’s inflammatory status. Albumin and prealbumin were visceral proteins valuable to assess the nutritional status of the body. Previous studies have shown that albumin (Sheinenzon et al., 2021) and prealbumin (Dennis et al., 2008) are negatively correlated with inflammatory factors such as CRP and have anti-inflammatory and antioxidant effects. Similarly, lymphocytes were also proven to have an effect on immunity and chronic inflammation (Kurtul et al., 2014). A previous study suggested that lymphocytopenia represents a state of critical inflammation (Li et al., 2021). Therefore, CAR, CPR, and CLR could better represent the body’s inflammatory and nutritional status. In this study, we explored their prognostic value in RR/MDR-TB patients. Our study found that patients with high levels of CAR, CPR, and CLR had a low survival rate. To date, this is the first study to report that RR/MDR-TB patients with high levels of CAR, CPR and CLR have a low survival rate. Currently, CAR (Karabağ et al., 2019; Karimi et al., 2021; Keinänen et al., 2021), CPR (Xie et al., 2011; Karimi et al., 2021; Maruyama et al., 2022), and CLR (Yang et al., 2020; Ko et al., 2021; Taniai et al., 2021) were reported to be prognostic factors for COVID-19, acute renal injury, and malignant tumor. In TB, previous studies have focused on the diagnostic value of inflammatory markers. For example, high-sensitivity C-reactive protein to lymphocyte ratio (HSCLR) was proven to be an important biomarker for distinguishing active PTB from inactive PTB (Yu et al., 2022b). Similarly, CLR can be used to predict the prevalence of T2DM in patients with active PTB (Yu et al., 2022a). To our best knowledge, the current study is the first to explore the correlation between CPR and prognosis of TB.
NLR, PLR, and MLR were newly discovered inflammatory markers in recent years. They are simple, easy to measure, and relatively stable compared with other inflammatory markers, such as interleukins. Previous studies have suggested that NLR, PLR, and MLR are diagnostic markers TB infection. NLR could identify TB in HIV-positive patients (Miyahara et al., 2019), and distinguish PTB from bacterial community-acquired pneumonia (Berhane et al., 2019). PLR was found to be a useful marker for identifying TB infection in COPD patients (Chen et al., 2016). MLR could be used to differentiate spinal TB from non-spinal TB (Chen et al., 2022). Interestingly, several studies have shown that high MLR could predict the severity of TB (Buttle et al., 2021; Chen et al., 2022). In this study, higher mortalities were observed in high NLR, PLR and MLR groups in RR/MDR-TB patients.
In addition, we explored the prognostic value of clinical characteristics in patients with RR/MDR-TB. We observed lower survival rates in the high age, smoking and bronchiectasia groups, which is similarly consistent with previous studies (Bates et al., 2007; Lin et al., 2007; Martínez-Rodríguez et al., 2016; Heunis et al., 2017; Wang et al., 2018). Frequent Comorbidities in older RR/MDR-TB patients, such as cardiovascular diseases, diabetes mellitus, and malignancies, could explain why the high age group had a higher mortality (Pardeshi, 2009). In addition, previous literature has already explained the mechanisms by which smoking affects the prognosis of TB patients. Nicotine can reduce the release of TNF-α, a key cytokine involved in granuloma formation by macrophages through α-7 nicotinic receptors (Silva et al., 2018). Interestingly, results from limited literature have examined the prognostic effect of bronchiectasia on RR/MDR-TB patients. Scholars found that bronchiectasia severity index could predict the mortality in post-tuberculosis bronchiectasia (Wang et al., 2018). TB infection is one of the leading causes of bronchiectasia in Asia-Pacific region. Pseudomonas aeruginosa, once co-infected with TB, may result in a reduced microbiota diversity, a disease progression and a poor prognosis (Dicker et al., 2021).
Based on these findings, the prognosis of RR/MDR-TB patients depend on multiple factors. The results of this study showed that inflammatory biomarkers, age, smoking and bronchiectasia, especially inflammatory biomarkers, were important prognostic factors in RR/MDR-TB, Inflammatory biomarkers had superior prognostic value compared to clinical characteristics in both the training and validation sets as evidenced by higher AUC. In addition, inflammatory biomarkers are easily and conveniently available indicators, and may provide physicians with more information and better guidance to improve the prognosis of RR/MDR-TB patients. CAR, CPR, CLR, NLR, PLR and MLR are good indicators of systemic inflammation (Llop-Talaveron et al., 2018; Okugawa et al., 2020; Jiang et al., 2021), and CAR and CPR can also reflect nutritional status of individuals (Llop-Talaveron et al., 2018). They can be easily and inexpensively calculated by the blood routine and biochemical test before ATT, independent of dehydration or fluid retention. The current study found that high levels of CAR, CPR, CLR, NLR, PLR, and MLR are essential prognostic factors for RR/MDR-TB patients, suggesting the importance for optimizing nutritional status, and improving hyperinflammatory status, thus minimizing mortality rate in RR/MDR-TB patients.
In conclusion, CAR, CPR, CLR, NLR, PLR, and MLR are economical and direct prognostic indicators valuable for assessing inflammation and nutrition status in patients with RR/MDR-TB. RR/MDR-TB patients with higher levels of CAR, CPR, CLR, NLR, PLR, and MLR indicate a higher mortality risk. Therefore, more attention should be paid to inflammatory biomarkers s in clinical practice.
The original contributions presented in the study are included in the article/Supplementary Material. Further inquiries can be directed to the corresponding authors.
The studies involving human participants were reviewed and approved by the Ethic Committee of Wuhan Jinyintan Hospital (KY-2022-06.01). Written informed consent to participate in this study was provided by the participants’ legal guardian/next of kin.
JY, QN, and DS conceived the study. QY, SH, QN, and HL collected samples and clinical data. QY, QN, SH, and HL were responsible for statistical analysis. QY and QN interpreted the data and wrote the manuscript. All authors contributed to the article and approved the submitted version.
The authors declare that the research was conducted in the absence of any commercial or financial relationships that could be construed as a potential conflict of interest.
All claims expressed in this article are solely those of the authors and do not necessarily represent those of their affiliated organizations, or those of the publisher, the editors and the reviewers. Any product that may be evaluated in this article, or claim that may be made by its manufacturer, is not guaranteed or endorsed by the publisher.
The Supplementary Material for this article can be found online at: https://www.frontiersin.org/articles/10.3389/fcimb.2023.1118424/full#supplementary-material
Amaral, E. P., Vinhaes, C. L., Oliveira-de-Souza, D., Nogueira, B., Akrami, K. M., Andrade, B. B. (2021). The interplay between systemic inflammation, oxidative stress, and tissue remodeling in tuberculosis. Antioxid. Redox Signaling 34 (6), 471–485. doi: 10.1089/ars.2020.8124
Balaky, S. T. J., Mawlood, A. H., Shabila, N. P. (2019). Survival analysis of patients with tuberculosis in erbil, Iraqi Kurdistan region. BMC Infect. Dis. 19 (1), 865. doi: 10.1186/s12879-019-4544-8
Bates, M. N., Khalakdina, A., Pai, M., Chang, L., Lessa, F., Smith, K. R. (2007). Risk of tuberculosis from exposure to tobacco smoke: a systematic review and meta-analysis. Arch. Internal Med. 167 (4), 335–342. doi: 10.1001/archinte.167.4.335
Berhane, M., Melku, M., Amsalu, A., Enawgaw, B., Getaneh, Z., Asrie, F. (2019). The role of neutrophil to lymphocyte count ratio in the differential diagnosis of pulmonary tuberculosis and bacterial community-acquired pneumonia: a cross-sectional study at ayder and mekelle hospitals, Ethiopia. Clin. Lab. 65 (4). doi: 10.7754/Clin.Lab.2018.180833
Buttle, T. S., Hummerstone, C. Y., Billahalli, T., Ward, R. J. B., Barnes, K. E., Marshall, N. J., et al. (2021). The monocyte-to-lymphocyte ratio: sex-specific differences in the tuberculosis disease spectrum, diagnostic indices and defining normal ranges. PloS One 16 (8), e0247745. doi: 10.1371/journal.pone.0247745
Carter, B. B., Zhang, Y., Zou, H., Zhang, C., Zhang, X., Sheng, R., et al. (2021). Survival analysis of patients with tuberculosis and risk factors for multidrug-resistant tuberculosis in Monrovia, Liberia. PloS One 16 (4), e0249474. doi: 10.1371/journal.pone.0249474
Chen, L., Liu, C., Liang, T., Ye, Z., Huang, S., Chen, J., et al. (2022). Monocyte-to-Lymphocyte ratio was an independent factor of the severity of spinal tuberculosis. Oxid. Med. Cell. Longevity 2022, 7340330. doi: 10.1155/2022/7340330
Chen, G., Wu, C., Luo, Z., Teng, Y., Mao, S. (2016). Platelet-lymphocyte ratios: a potential marker for pulmonary tuberculosis diagnosis in COPD patients. Int. J. Chronic Obstruct. Pulmon. Dis. 11, 2737–2740. doi: 10.2147/COPD.S111254
Chung-Delgado, K., Guillen-Bravo, S., Revilla-Montag, A., Bernabe-Ortiz, A. (2015). Mortality among MDR-TB cases: comparison with drug-susceptible tuberculosis and associated factors. PloS One 10 (3), e0119332. doi: 10.1371/journal.pone.0119332
Ciccacci, F., Welu, B., Ndoi, H., Karea, I., Orlando, S., Brambilla, D., et al. (2021). High-sensitivity c-reactive protein in HIV care: tuberculosis diagnosis and short-term mortality in a cohort of Kenyan HIV patients in the DREAM programme. Int. J. Infect. Dis.: IJID: Off. Publ. Int. Soc. For Infect. Dis. 104, 329–334. doi: 10.1016/j.ijid.2021.01.008
Dennis, R. A., Johnson, L. E., Roberson, P. K., Heif, M., Bopp, M. M., Cook, J., et al. (2008). Changes in prealbumin, nutrient intake, and systemic inflammation in elderly recuperative care patients. J. Am. Geriatr. Soc. 56 (7), 1270–1275. doi: 10.1111/j.1532-5415.2008.01789.x
Dicker, A. J., Lonergan, M., Keir, H. R., Smith, A. H., Pollock, J., Finch, S., et al. (2021). The sputum microbiome and clinical outcomes in patients with bronchiectasis: a prospective observational study. Lancet Respir. Med. 9 (8), 885–896. doi: 10.1016/S2213-2600(20)30557-9
Heunis, J. C., Kigozi, N. G., Chikobvu, P., Botha, S., van Rensburg, H. D. (2017). Risk factors for mortality in TB patients: a 10-year electronic record review in a south African province. BMC Public Health 17 (1), 38. doi: 10.1186/s12889-016-3972-2
Jiang, Y., Xu, D., Song, H., Qiu, B., Tian, D., Li, Z., et al. (2021). Inflammation and nutrition-based biomarkers in the prognosis of oesophageal cancer: a systematic review and meta-analysis. BMJ Open 11 (9), e048324. doi: 10.1136/bmjopen-2020-048324
Karabağ, Y., Çağdaş, M., Rencuzogullari, I., Karakoyun, S., Artaç, İ., İliş, D., et al. (2019). The c-reactive protein to albumin ratio predicts acute kidney injury in patients with ST-segment elevation myocardial infarction undergoing primary percutaneous coronary intervention. Heart Lung Circ. 28 (11), 1638–1645. doi: 10.1016/j.hlc.2018.08.009
Karimi, A., Shobeiri, P., Kulasinghe, A., Rezaei, N. (2021). Novel systemic inflammation markers to predict COVID-19 prognosis. Front. In Immunol. 12. doi: 10.3389/fimmu.2021.741061
Keinänen, A., Uittamo, J., Marinescu-Gava, M., Kainulainen, S., Snäll, J. (2021). Preoperative c-reactive protein to albumin ratio and oral health in oral squamous cell carcinoma patients. BMC Oral. Health 21 (1), 132. doi: 10.1186/s12903-021-01516-0
Khazaei, H. A., Rezaei, N., Bagheri, G. R., Dankoub, M. A., Shahryari, K., Tahai, A., et al. (2005). Epidemiology of tuberculosis in the southeastern Iran. Eur. J. Epidemiol. 20 (10), 879–883. doi: 10.1007/s10654-005-2152-y
Ko, C.-A., Fang, K.-H., Hsu, C.-M., Lee, Y.-C., Chang, G.-H., Huang, E. I., et al. (2021). The preoperative c-reactive protein-lymphocyte ratio and the prognosis of oral cavity squamous cell carcinoma. Head Neck 43 (9), 2740–2754. doi: 10.1002/hed.26738
Krug, S., Parveen, S., Bishai, W. R. (2021). Host-directed therapies: modulating inflammation to treat tuberculosis. Front. In Immunol. 12. doi: 10.3389/fimmu.2021.660916
Kurtul, A., Yarlioglues, M., Murat, S. N., Ergun, G., Duran, M., Kasapkara, H. A., et al. (2014). Usefulness of the platelet-to-lymphocyte ratio in predicting angiographic reflow after primary percutaneous coronary intervention in patients with acute ST-segment elevation myocardial infarction. Am. J. Cardiol. 114 (3), 342–347. doi: 10.1016/j.amjcard.2014.04.045
Li, L., Ma, Y., Geng, X.-B., Tan, Z., Wang, J.-H., Cui, C., et al. (2021). Platelet-to-lymphocyte ratio relates to poor prognosis in elderly patients with acute myocardial infarction. Aging Clin. Exp. Res. 33 (3), 619–624. doi: 10.1007/s40520-020-01555-7
Limenih, Y. A., Workie, D. L. (2019). Survival analysis of time to cure on multi-drug resistance tuberculosis patients in amhara region, Ethiopia. BMC Public Health 19 (1), 165. doi: 10.1186/s12889-019-6500-3
Lin, H.-H., Ezzati, M., Murray, M. (2007). Tobacco smoke, indoor air pollution and tuberculosis: a systematic review and meta-analysis. PloS Med. 4 (1), e20. doi: 10.1371/journal.pmed.0040020
Llop-Talaveron, J., Badia-Tahull, M. B., Leiva-Badosa, E. (2018). An inflammation-based prognostic score, the c-reactive protein/albumin ratio predicts the morbidity and mortality of patients on parenteral nutrition. Clin. Nutr. (Edinburgh Scotland) 37 (5), 1575–1583. doi: 10.1016/j.clnu.2017.08.013
Machmud, P. B., Gayatri, D., Ronoatmodjo, S. (2021). A survival analysis of successful and poor treatment outcome among patients with drug-resistant tuberculosis and the associated factors: a retrospective cohort study. Acta Med. Indonesiana 53 (2), 184–193.
Martínez-Rodríguez, A., González-Díaz, A., Armas-Pérez, L., Sánchez-Valdés, L., Martínez-Morales, M. A., González-Ochoa, E. R. (2016). Survival of Cuban patients with pulmonary tuberculosis, (2009-2010). MEDICC Rev. 18 (1-2), 22. doi: 10.37757/MR2016.V18.N1-2.5
Maruyama, S., Okamura, A., Kanie, Y., Sakamoto, K., Fujiwara, D., Kanamori, J., et al. (2022). C-reactive protein to prealbumin ratio: a useful inflammatory and nutritional index for predicting prognosis after curative resection in esophageal squamous cell carcinoma patients. Langenbeck’s Arch. Surg. 407 (5), 1901–1909. doi: 10.1007/s00423-022-02508-6
Miura, T., Ohtsuka, H., Aoki, T., Aoki, S., Hata, T., Takadate, T., et al. (2021). Increased neutrophil-lymphocyte ratio predicts recurrence in patients with well-differentiated pancreatic neuroendocrine neoplasm based on the 2017 world health organization classification. BMC Surg. 21 (1), 176. doi: 10.1186/s12893-021-01178-3
Miyahara, R., Piyaworawong, S., Naranbhai, V., Prachamat, P., Kriengwatanapong, P., Tsuchiya, N., et al. (2019). Predicting the risk of pulmonary tuberculosis based on the neutrophil-to-lymphocyte ratio at TB screening in HIV-infected individuals. BMC Infect. Dis. 19 (1), 667. doi: 10.1186/s12879-019-4292-9
Okugawa, Y., Toiyama, Y., Yamamoto, A., Shigemori, T., Ichikawa, T., Yin, C., et al. (2020). Lymphocyte-to-C-reactive protein ratio and score are clinically feasible nutrition-inflammation markers of outcome in patients with gastric cancer. Clin. Nutr. (Edinburgh Scotland) 39 (4), 1209–1217. doi: 10.1016/j.clnu.2019.05.009
Pardeshi, G. (2009). Survival analysis and risk factors for death in tuberculosis patients on directly observed treatment-short course. Indian J. Med. Sci. 63 (5), 180–186. doi: 10.4103/0019-5359.53163
Sheinenzon, A., Shehadeh, M., Michelis, R., Shaoul, E., Ronen, O. (2021). Serum albumin levels and inflammation. Int. J. Biol. Macromol. 184, 857–862. doi: 10.1016/j.ijbiomac.2021.06.140
Silva, D. R., Muñoz-Torrico, M., Duarte, R., Galvão, T., Bonini, E. H., Arbex, F. F., et al. (2018). Risk factors for tuberculosis: diabetes, smoking, alcohol use, and the use of other drugs. Jornal Brasileiro Pneumol.: Publicacao Oficial Da Sociedade Bras. Pneumol. E Tisilogia 44 (2), 145–152. doi: 10.1590/s1806-37562017000000443
Taniai, T., Haruki, K., Hamura, R., Fujiwara, Y., Furukawa, K., Gocho, T., et al. (2021). The prognostic significance of c-reactive protein-To-Lymphocyte ratio in colorectal liver metastases. J. Surg. Res. 258, 414–421. doi: 10.1016/j.jss.2020.08.059
Wang, H., Ji, X.-B., Li, C.-W., Lu, H.-W., Mao, B., Liang, S., et al. (2018). Clinical characteristics and validation of bronchiectasis severity score systems for post-tuberculosis bronchiectasis. Clin. Respir. J. 12 (8), 2346–2353. doi: 10.1111/crj.12911
Xie, Y., Han, J., Yu, W., Wu, J., Li, X., Chen, H. (2020). Survival analysis of risk factors for mortality in a cohort of patients with tuberculosis. Can. Respir. J. 2020, 1654653. doi: 10.1155/2020/1654653
Xie, Q., Zhou, Y., Xu, Z., Yang, Y., Kuang, D., You, H., et al. (2011). The ratio of CRP to prealbumin levels predict mortality in patients with hospital-acquired acute kidney injury. BMC Nephrol. 12, 30. doi: 10.1186/1471-2369-12-30
Yang, M., Chen, X., Xu, Y. (2020). A retrospective study of the c-reactive protein to lymphocyte ratio and disease severity in 108 patients with early COVID-19 pneumonia from January to march 2020 in wuhan, China. Med. Sci. Monit.: Int. Med. J. Exp. Clin. Res. 26, e926393. doi: 10.12659/MSM.926393
Yu, Q., Weng, W., Luo, H., Yan, J., Zhao, X. (2022a). The novel predictive biomarkers for type 2 diabetes mellitus in active pulmonary tuberculosis patients. Infect. Drug Resist. 15, 4529–4539. doi: 10.2147/IDR.S377465
Keywords: rifampicin/multidrug-resistant tuberculosis, prognosis, inflammatory markers, clincial features, inflammation
Citation: Yu Q, Luo H, Hu S, Sun D, Nie Q and Yan J (2023) The survival analysis of rifampicin/multidrug-resistant tuberculosis patients based on the levels of inflammatory biomarkers: a retrospective cohort study. Front. Cell. Infect. Microbiol. 13:1118424. doi: 10.3389/fcimb.2023.1118424
Received: 07 December 2022; Accepted: 07 April 2023;
Published: 01 May 2023.
Edited by:
Bindu Singh, Texas Biomedical Research Institute, United StatesCopyright © 2023 Yu, Luo, Hu, Sun, Nie and Yan. This is an open-access article distributed under the terms of the Creative Commons Attribution License (CC BY). The use, distribution or reproduction in other forums is permitted, provided the original author(s) and the copyright owner(s) are credited and that the original publication in this journal is cited, in accordance with accepted academic practice. No use, distribution or reproduction is permitted which does not comply with these terms.
*Correspondence: Qi Nie, MTAzODc0NTg2QHFxLmNvbQ==; Jisong Yan, amlzb25neWFuMTYzQDE2My5jb20=
Disclaimer: All claims expressed in this article are solely those of the authors and do not necessarily represent those of their affiliated organizations, or those of the publisher, the editors and the reviewers. Any product that may be evaluated in this article or claim that may be made by its manufacturer is not guaranteed or endorsed by the publisher.
Research integrity at Frontiers
Learn more about the work of our research integrity team to safeguard the quality of each article we publish.