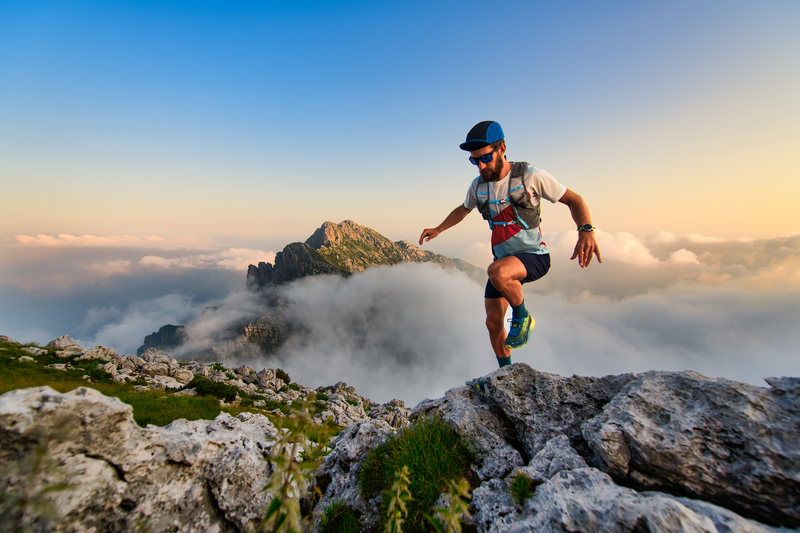
95% of researchers rate our articles as excellent or good
Learn more about the work of our research integrity team to safeguard the quality of each article we publish.
Find out more
REVIEW article
Front. Cell. Infect. Microbiol. , 15 August 2022
Sec. Microbiome in Health and Disease
Volume 12 - 2022 | https://doi.org/10.3389/fcimb.2022.943427
Evidence of gut microbiota involvement in regulating glucose metabolism and type 2 diabetes mellitus (T2DM) progression is accumulating. The understanding of microbial dysbiosis and specific alterations of gut microbiota composition that occur during the early stages of glucose intolerance, unperturbed by anti-diabetic medications, is especially essential. Hence, this systematic review was conducted to summarise the existing evidence related to microbiota composition and diversity in individuals with prediabetes (preDM) and individuals newly diagnosed with T2DM (newDM) in comparison to individuals with normal glucose tolerance (nonDM). A systematic search of the PubMed, MEDLINE and CINAHL databases were conducted from inception to February 2021 supplemented with manual searches of the list of references. The primary keywords of “type 2 diabetes”, “prediabetes”, “newly-diagnosed” and “gut microbiota” were used. Observational studies that conducted analysis of the gut microbiota of respondents with preDM and newDM were included. The quality of the studies was assessed using the modified Newcastle-Ottawa scale by independent reviewers. A total of 18 studies (5,489 participants) were included. Low gut microbial diversity was generally observed in preDM and newDM when compared to nonDM. Differences in gut microbiota composition between the disease groups and nonDM were inconsistent across the included studies. Four out of the 18 studies found increased abundance of phylum Firmicutes along with decreased abundance of Bacteroidetes in newDM. At the genus/species levels, decreased abundance of Faecalibacterium prausnitzii, Roseburia, Dialister, Flavonifractor, Alistipes, Haemophilus and Akkermansia muciniphila and increased abundance of Lactobacillus, Streptococcus, Escherichia, Veillonella and Collinsella were observed in the disease groups in at least two studies. Lactobacillus was also found to positively correlate with fasting plasma glucose (FPG), HbA1c and/or homeostatic assessment of insulin resistance (HOMA-IR) in four studies. This renders a need for further investigations on the species/strain-specific role of endogenously present Lactobacillus in glucose regulation mechanism and T2DM disease progression. Differences in dietary intake caused significant variation in specific bacterial abundances. More studies are needed to establish more consistent associations, between clinical biomarkers or dietary intake and specific gut bacterial composition in prediabetes and early T2DM.
It is expected that by 2030, 578 million people worldwide will have diabetes, with type 2 diabetes mellitus (T2DM) accounting for about 90% of this staggering figure (Saeedi et al., 2019). With an estimated world population of 8,548 million by 2030, this would predict approximately 6% of the world’s population having T2DM by 2030 (Worldometers, 2021). In addition, approximately 8% of the world adult population is projected to have prediabetes by 2030, thereby also being at risk of developing full-blown T2DM (International Diabetes Federation (IDF), 2019). T2DM is a non-communicable disease characterized by an elevated blood glucose level or hyperglycaemia, defined by a fasting plasma glucose (FPG) level of ≥ 7.0mmol/l or 2-hour postprandial glucose level of ≥ 11.1mmol/l following oral glucose tolerance test (OGTT) (Alberti and Zimmet, 1998). Intermediate hyperglycaemia or prediabetes state, is defined by an impaired fasting glucose (IFG) and/or impaired glucose tolerance (IGT), after OGTT (Alberti and Zimmet, 1998). It was estimated that up to 21% of individuals with prediabetes will develop T2DM within three years (Eades et al., 2014).
Individuals with T2DM have an increased risk of developing complications such as kidney failure, retinopathy, neuropathy, cardiovascular disease and limb amputations (Centers for Disease Control Prevention, 2014). Recent evidence suggests a strong association between alterations in the gut microbiota composition and several metabolic disorders, including diabetes (Han and Lin, 2014). Several potential microbial molecular mechanisms have been suggested to contribute towards the onset and progression of T2DM (Gurung et al, 2020). On the other hand, use of metformin was found to be associated with modification in gut microbiota composition that contributed towards the therapeutic effects as well as known intestinal adverse effects of this most commonly used antidiabetic drug (Forslund et al., 2015). This emphasizes the need to detach observations of gut microbial alterations occurring in disease alone, free from the effects of drugs. As such, these specific changes in gut microbiota composition of prediabetic individuals and/or newly diagnosed diabetic individuals who have not begun pharmacotherapy, may serve as a predictive tool for identifying individuals at high-risk for developing T2DM. This would also enable future studies focusing on the specific microbial species to distinguish their role in disease as either cause or effect or both. This systematic review therefore aims to evaluate and summarise the existing evidence related to microbiota composition and diversity in individuals with prediabetes (preDM) and individuals newly diagnosed with T2DM (newDM) in comparison to individuals with normal glucose tolerance (nonDM). Findings on the association between the gut microbiota composition and clinical or dietary factors are also summarised.
A systematic review of observational studies was performed according to a protocol published in the International Prospective Register of Systematic Reviews (PROSPERO) (CRD42020160458, 10/7/2020) (Pathmanathan et al., 2020) and reported according to the Preferred Reporting Items for Systematic Reviews and Meta-Analyses (PRISMA) guidelines (Moher et al., 2009).
A systematic search of published literature from inception to February 2021 was conducted using electronic databases including PubMed Central by the National Center for Biotechnology Information (PMC-NCBI), MEDLINE Complete and CINAHL (EBSCO Host). Briefly, main keywords included in the search were “type 2 diabetes”, “prediabetes”, “newly-diagnosed” and “gut microbiota”. The complete search strategy is provided in Table 1. Additional eligible studies were identified by hand-searching the reference lists of included studies. The search was limited to only studies published in English.
Studies that met the following criteria were included: (1) observational studies including case-control, cohort and cross-sectional study design; (2) studies on adult participants with newDM or preDM (3) studies that included a nonDM control group and (4) studies in which faecal samples were collected for gut microbial analysis. We excluded studies in which the participants were receiving treatment or dietary intervention prior to the investigation, studies in which participants provided microbiota samples from other body sites, animal studies and studies in which participants had other types of diabetes besides T2DM. Review articles, unpublished data, and articles in other languages were also excluded.
All search results were exported to a reference manager software (Endnote X9.3.1). Two authors (GL and SGP) selected the articles based on their titles and abstracts. Any disagreements between the reviewers were resolved through discussion with the third reviewer (NA). The full texts of eligible studies were assessed, and studies deemed irrelevant were excluded. A standard form was used to extract the data of included studies (Supplementary Excel 1).
Data recorded were general study characteristics (author name, year of publication, journal name, study design, country, sample size, gender distribution, mean age) and characteristics of methodology used by selected studies (microbiota quantification methods and diversity indices used) and the outcome measured. The primary outcomes were gut microbial abundance and differences between study groups at the phylum, class, order, family and genus taxonomic ranks. Species were grouped according to their respective genus. The secondary outcomes included clinical biomarkers, dietary intake and other parameters measured along with their correlation with the gut microbial composition.
The study quality was assessed using the modified Newcastle Ottawa scale (Wells et al., 2014; Bjerre et al., 2017). The scale involves a maximum rating of nine stars divided into three categories: (1) samples selection, (2) comparability (comparison of the baseline parameters) and (3) exposure (defined measure of exposure and response rate between cases and controls). The studies were categorised based on their quality which were very good (score of 9); good (score of 7 to 8); fair (score of 5 to 6); and poor (score less than 5). Two researchers independently assessed the studies (GL and NB) and any discrepancies were resolved by another researcher (NA).
Of 3,994 articles identified, 18 studies were included in this systematic review (See Figure 1 for PRISMA flow diagram). The characteristics of the study population are summarised in Table 2. The 18 observational studies had been conducted between 2013 and 2021. They included twelve case-control (Zhang et al., 2013; Lambeth et al., 2015; Bhute et al., 2017; Allin et al., 2018; Chen et al., 2019; Nuli et al., 2019; Zhao et al., 2019; Zhong et al., 2019; Gaike et al., 2020; Ghaemi et al., 2020; Li et al., 2020; Wang et al., 2021), four cross-sectional studies (Diener et al., 2021; Egshatyan et al., 2016; Chávez-Carbajal et al., 2020; Wu et al., 2020) and two cohort studies (Karlsson et al., 2013; Ericson et al., 2020). Six studies were conducted in Asia [India (Bhute et al., 2017; Gaike et al., 2020), Iran (Ghaemi et al., 2020), Taiwan (Chen et al., 2019) and China (Zhang et al., 2013; Nuli et al., 2019; Zhao et al., 2019; Zhong et al., 2019; Li et al., 2020; Wang et al., 2021)], five in Europe [Denmark (Allin et al., 2018), Russia (Egshatyan et al., 2016) and Sweden (Karlsson et al., 2013; Wu et al., 2020; Ericson et al., 2020)] and three in North America [USA (Lambeth et al., 2015) and Mexico (Diener et al., 2021; Chávez-Carbajal et al., 2020)]. These studies had compared the gut microbial profiles of either preDM alone (Allin et al., 2018; Ericson et al., 2020), newDM alone (Chen et al., 2019; Li et al., 2020), preDM and newDM (Zhang et al., 2013; Egshatyan et al., 2016; Nuli et al., 2019; Zhong et al., 2019; Wu et al., 2020), preDM and knownDM (Karlsson et al., 2013; Lambeth et al., 2015; Ghaemi et al., 2020; Chávez-Carbajal et al., 2020; Wang et al., 2021), newDM and knownDM (Bhute et al., 2017; Zhao et al., 2019) or all three preDM, newDM and knownDM (Diener et al., 2021; Gaike et al., 2020) in comparison to gut microbial profile of nonDM. The findings on knownDM were not analysed in this review.
Figure 1 Flow chart of process undertaken to identify eligible studies, according to the PRISMA guidelines.
The 18 studies consisted of 5,489 participants. In 15 of the 18 studies, 43% of the participants were male (n=2, 252) and 57% were female (n= 2,978). Three studies (Zhang et al., 2013; Bhute et al., 2017; Ghaemi et al., 2020) did not specify the participants’ gender. The mean age of participants was 50 ± 7.82 years. There was a total of 3,149 participants in the control or nonDM group. The remaining 2,340 participants with varying glucose levels included 1,599 preDM, 406 newDM and 335 knownDM. PreDM was diagnosed using either IFG (Lambeth et al., 2015; Allin et al., 2018; Gaike et al., 2020; Chávez-Carbajal et al., 2020; Ericson et al., 2020), IGT (Karlsson et al., 2013), combined glucose intolerance (CGI) (Wu et al., 2020), IFG and/or IGT (Diener et al., 2021; Zhang et al., 2013; Egshatyan et al., 2016; Zhong et al., 2019; Ghaemi et al., 2020; Wu et al., 2020; Wang et al., 2021). NewDM was diagnosed using OGTT (Diener et al., 2021; Zhang et al., 2013; Egshatyan et al., 2016; Bhute et al., 2017; Nuli et al., 2019; Zhao et al., 2019; Zhong et al., 2019; Li et al., 2020; Wu et al., 2020), HbA1c (Egshatyan et al., 2016; Zhao et al., 2019; Gaike et al., 2020) and fasting plasma glucose (FPG) (Chen et al., 2019). In 10 out of 11 studies that had participants with newDM, no information was provided regarding period between newDM diagnosis to sample collection while one study reported to only have included participants with newDM who had disease duration of <12 months and a HbA1c range of 6.5 – 9.0% (Egshatyan et al., 2016).
Table S1 summarises the quality assessment of the studies included. The mean score for the studies included was 8 (range of 6-9) out of a possible total of 9. All studies received either very good or good scores. One study received three out of five maximum scores in the section on study selection as it did not describe sampling strategy or justify the sample size (Chávez-Carbajal et al., 2020), which are criteria to be fulfilled for cross sectional studies, based on the decision rule in the modified Newcastle Ottawa scale used in this review. In the comparability section, five studies (Zhang et al., 2013; Bhute et al., 2017; Chen et al., 2019; Gaike et al., 2020; Ghaemi et al., 2020) stated that cases and controls were matched based on diabetes status alone and did not take into account other confounding factors such as gender or age. Meanwhile, in the exposure section, 11 studies (Zhang et al., 2013; Lambeth et al., 2015; Bhute et al., 2017; Allin et al., 2018; Chen et al., 2019; Nuli et al., 2019; Zhao et al., 2019; Zhong et al., 2019; Gaike et al., 2020; Ghaemi et al., 2020; Li et al., 2020) did not mention the number of study participants who dropped out, if any.
Table 3 summarises the methodologies adapted by the studies to analyse the microbiome data. These studies used varying DNA kits for DNA extraction and different microbiome sequencing platforms including Illumina Miseq (Diener et al., 2021; Lambeth et al., 2015; Egshatyan et al., 2016; Allin et al., 2018; Nuli et al., 2019) or Hi-Seq (Karlsson et al., 2013; Gaike et al., 2020; Wu et al., 2020; Ericson et al., 2020; Wang et al., 2021) sequencing platforms, the 454 GS FLX Titanium pyro-sequencer (Zhang et al., 2013), PGM sequencing (Ion Touch 2) (Bhute et al., 2017; Chávez-Carbajal et al., 2020), Ion S5 sequencer with Ion Torrent Technology (Zhao et al., 2019), shotgun metagenomics sequencing using the BGISEQ-500 platform (Zhong et al., 2019) as well as quantitative polymerase chain reaction (qPCR) (Chen et al., 2019; Ghaemi et al., 2020). When the 16S rDNA was targeted for sequencing, the hypervariable regions targeted included V4 (Diener et al., 2021; Lambeth et al., 2015; Allin et al., 2018; Gaike et al., 2020), V3-V4 (Egshatyan et al., 2016; Nuli et al., 2019; Zhao et al., 2019), V3 (Bhute et al., 2017; Chávez-Carbajal et al., 2020), V1-V3 (Ericson et al., 2020) or V3-V5 (Zhang et al., 2013) regions, while five studies did not specify the target regions (Karlsson et al., 2013; Zhong et al., 2019; Li et al., 2020; Wu et al., 2020; Wang et al., 2021). The two studies using qPCR to assess the microbiota had used ten and six pairs of specific bacterial 16S rRNA primers, respectively, to target Atopobium cluster, Bacteroides fragilis, Bifidobacterium, Clostridium coccoides, Clostridium leptum, Clostridium perfringens, Enterobacteriaceae, Enterococcus, Lactobacillus and Prevotella (Chen et al., 2019) and Akkermansia, Bacteroides, Bifidobacterium, E.coli, Faecalibacterium and Lactobacillus (Ghaemi et al., 2020).
Table 3 Characteristics of the methodology used by the 18 selected articles for gut microbiota composition and diversity assessments.
Following sequencing, the microorganisms were classified and reported as operational taxonomic units (OTUs) (Zhang et al., 2013; Lambeth et al., 2015; Egshatyan et al., 2016; Bhute et al., 2017; Allin et al., 2018; Nuli et al., 2019; Zhao et al., 2019; Gaike et al., 2020; Li et al., 2020; Chávez-Carbajal et al., 2020; Ericson et al., 2020), metagenomic clusters (MGCs) (Karlsson et al., 2013; Wu et al., 2020), microbiota (log10 cell/g) (Chen et al., 2019; Ghaemi et al., 2020), metagenome (Wang et al., 2021), metagenomic linkage group (MLGs) (Zhong et al., 2019) or amplicon sequence variants (ASVs) (Diener et al., 2021).
The α-diversity or the average microbial diversity within a single sample was reported by 13 of the 18 studies and the key measures used were Shannon, Simpson, observed species richness, abundance-based coverage estimator (ACE), Chao1 and phylogenetic diversity indexes (Table 3).
Six of the studies found a statistically significant lower α-diversity in the disease groups namely preDM (Allin et al., 2018; Chávez-Carbajal et al., 2020), newDM (Zhang et al., 2013; Bhute et al., 2017; Gaike et al., 2020) and in both preDM and newDM (Nuli et al., 2019) in comparison to nonDM. Two other studies reported a non-significant reduction in α-diversity in preDM (Lambeth et al., 2015) and newDM (Zhao et al., 2019). On the other hand, another two studies, (Zhong et al., 2019) and (Wang et al., 2021) found no significant difference in α-diversity among preDM and newDM in comparison to nonDM. Diener et al. (2021) found that genera Ruminococcaceae was the most positively correlated with α-diversity while Fusobacterium, Flavonifractor, and Parasutterella were the most negatively correlated with α-diversity (Diener et al., 2021). Allin et al. (2018) went on to demonstrate that α-diversity in the total group of subjects was negatively correlated with T2DM biomarkers particularly plasma triacylglycerol and high sensitivity C-reactive protein (hsCRP), as well as body mass index (BMI), hip circumference (HC), fasting blood glucose (FBG), fasting plasma insulin (FINS), plasma C-peptide and HOMA-IR (Allin et al., 2018). Ericson et al. (2020) examined the correlation between the food patterns and α-diversity in preDM and nonDM, but discovered no statistically significant association (Ericson et al., 2020).
The β-diversity or the measure of how gut microbial composition vary between study groups was reported by 11 studies using principal coordinate analysis (PCoA), principal component analysis (PCA) of either weighted or unweighted UniFrac or Bray Curtis dissimilarities distance matrices (Table 3).
Six studies found a significant difference in β-diversity among preDM (Chávez-Carbajal et al., 2020), newDM (Bhute et al., 2017; Zhao et al., 2019; Li et al., 2020) and in both preDM and newDM (Nuli et al., 2019; Wu et al., 2020) in comparison to nonDM. Four studies found no difference in the β-diversity between disease groups and nonDM groups (Zhang et al., 2013; Allin et al., 2018; Zhong et al., 2019; Wang et al., 2021). Gaike et al. (2020) observed using Principal Coordinate Analysis (PCoA), that bacterial diversity of newDM was distinct from that of nonDM, whereas preDM formed an overlapping cluster with nonDM indicating similarity in bacterial diversity (Gaike et al., 2020).
All eighteen studies provided information on microbial abundance by genus/species ranks. Eleven studies (Zhang et al., 2013; Karlsson et al., 2013; Lambeth et al., 2015; Egshatyan et al., 2016; Bhute et al., 2017; Nuli et al., 2019; Zhao et al., 2019; Zhong et al., 2019; Gaike et al., 2020; Li et al., 2020; Chávez-Carbajal et al., 2020) reported the taxonomic rank of microbial abundance by phylum level. Nine studies (Zhang et al., 2013; Karlsson et al., 2013; Lambeth et al., 2015; Bhute et al., 2017; Allin et al., 2018; Nuli et al., 2019; Zhao et al., 2019; Li et al., 2020; Chávez-Carbajal et al., 2020) reported by ranks of class, order and family.
Eight studies reported that Bacteroidetes and Firmicutes were the predominant phyla in all groups studied, i.e., preDM and/or newDM and nonDM (Zhang et al., 2013; Karlsson et al., 2013; Lambeth et al., 2015; Egshatyan et al., 2016; Nuli et al., 2019; Zhao et al., 2019; Zhong et al., 2019; Li et al., 2020). Proteobacteria was reported as the next predominant phyla in five out of these eight studies (Karlsson et al., 2013; Lambeth et al., 2015; Nuli et al., 2019; Zhao et al., 2019; Zhong et al., 2019). All eighteen studies reported significant differences in gut microbiota composition by microbial taxa in the disease groups i.e. preDM and/or newDM when compared to the nonDM control group (Table 4 and Table S2). In four studies, a significant increase in the phylum Firmicutes along with a significant decrease in phylum Bacteroidetes were observed in the newDM group (Bhute et al., 2017; Nuli et al., 2019; Zhao et al., 2019; Gaike et al., 2020). Two of these four studies each found a significant increase (Zhao et al., 2019; Gaike et al., 2020) or significant decrease (Bhute et al., 2017; Nuli et al., 2019) in Proteobacteria respectively. Meanwhile, two other studies reported a significant decrease in phylum Verrucomicrobia in preDM group (Zhang et al., 2013; Egshatyan et al., 2016). Two studies reported increased Firmicutes/Bacteroidetes ratio (F/B ratio) among newDM (Zhao et al., 2019; Li et al., 2020) and one study reported increased F/B ratio among both preDM and newDM (Gaike et al., 2020).
Table 4 The changes (increase or decrease) noted in gut microbiota of preDM and newDM in comparison to nonDM by microbial taxa and number of reporting studies. All findings are significant (p <0.050).
Figure 2 depicts findings from all 18 studies on the significantly differing genera/species belonging to the six predominant gut bacterial phyla in a heatmap-like format. When focusing exclusively on changes reported by two or more studies, the composition of a particular genera/species demonstrated distinct changes in disease groups (Table 4 and Table S2). The number of Streptococcus (Karlsson et al., 2013; Allin et al., 2018; Zhong et al., 2019), Escherichia (Diener et al., 2021; Zhong et al., 2019; Ghaemi et al., 2020) and Veillonella (Diener et al., 2021; Nuli et al., 2019) in preDM were increased. Similarly, Lactobacillus (Bhute et al., 2017; Chen et al., 2019; Gaike et al., 2020) and Collinsella (Zhang et al., 2013; Zhong et al., 2019), were increased in newDM. On the other hand, Faecalibacterium prausnitzii (Karlsson et al., 2013; Allin et al., 2018; Zhong et al., 2019; Ghaemi et al., 2020; Wu et al., 2020), Akkermansia (Zhang et al., 2013; Allin et al., 2018), Alistipes (Karlsson et al., 2013; Wu et al., 2020), Flavonifractor (Nuli et al., 2019; Wu et al., 2020) and Roseburia (Karlsson et al., 2013; Zhong et al., 2019) were decreased in preDM while Akkermansia (Zhong et al., 2019; Gaike et al., 2020), Dialister (Zhong et al., 2019; Li et al., 2020), Haemophilus (Zhang et al., 2013; Zhong et al., 2019), Roseburia (Zhang et al., 2013; Zhong et al., 2019) and Faecalibacterium (Zhang et al., 2013; Bhute et al., 2017; Wu et al., 2020) were decreased in newDM. Bacteroides (Zhang et al., 2013; Karlsson et al., 2013; Allin et al., 2018; Zhao et al., 2019; Ghaemi et al., 2020; Li et al., 2020) and Prevotella (Zhang et al., 2013; Egshatyan et al., 2016; Bhute et al., 2017; Zhao et al., 2019) of the phylum Bacteroidetes; Blautia (Diener et al., 2021; Egshatyan et al., 2016; Allin et al., 2018; Zhao et al., 2019; Gaike et al., 2020), Eubacterium (Zhang et al., 2013; Karlsson et al., 2013; Zhao et al., 2019; Ericson et al., 2020), Clostridium (Karlsson et al., 2013; Allin et al., 2018; Chen et al., 2019; Zhong et al., 2019; Wu et al., 2020) and Coprococcus (Allin et al., 2018; Zhao et al., 2019; Zhong et al., 2019; Wu et al., 2020) of the phylum Firmicutes all exhibited changes in both directions.
Figure 2 A heatmap-like view depicting the genera/species of the six predominant gut microbial phyla, found to increase or decrease in the preDM and newDM groups, by number of reporting studies.
Fifteen studies assessed clinical indices and dietary habits in their study groups and correlated them with individual gut microbial taxa abundance (Diener et al., 2021; Zhang et al., 2013; Karlsson et al., 2013; Lambeth et al., 2015; Egshatyan et al., 2016; Bhute et al., 2017; Allin et al., 2018; Chen et al., 2019; Nuli et al., 2019; Zhao et al., 2019; Gaike et al., 2020; Li et al., 2020; Chávez-Carbajal et al., 2020; Ericson et al., 2020; Wang et al., 2021). These are depicted in Tables S3, S4 respectively (Supplementary Material).
Nine studies reported that preDM (Zhang et al., 2013; Egshatyan et al., 2016; Allin et al., 2018; Wu et al., 2020; Ericson et al., 2020) and newDM (Zhang et al., 2013; Chen et al., 2019; Zhao et al., 2019; Wu et al., 2020) had significantly increased BMI. Five studies found a positive correlation between the increase of BMI with genera Blautia, Eubacterium, Roseburia (Ericson et al., 2020), Streptococcus, Veillonella, Prevotella (Zhao et al., 2019) or negative correlation with genera Prevotella (Nuli et al., 2019) and Clostridium (Allin et al., 2018), and specifically Clostridium coccoides (Chen et al., 2019). Meanwhile, the increase in FPG was inversely correlated to Bacteroides uniformis (Li et al., 2020) and Prevotella copri (Bhute et al., 2017), but positively correlated to the genus Escherichia (Gaike et al., 2020) and Coprococcus comes (Allin et al., 2018). Additionally, it was noted that FPG and/or HOMA-IR were positively associated with the genus Lactobacillus (Diener et al., 2021; Karlsson et al., 2013; Chen et al., 2019), Blautia wexlerae (Allin et al., 2018), Clostridium (Allin et al., 2018) and specifically Clostridium leptum and Clostridium coccoides (Chen et al., 2019). Similarly, both FPG and HbA1c were observed to either correlate positively (Zhao et al., 2019) or negatively (Gaike et al., 2020) with genus Akkermansia, negatively with genus Clostridium (Karlsson et al., 2013) and positively with genus Lactobacillus (Chen et al., 2019; Gaike et al., 2020) and specifically Lactobacillus gasseri (Karlsson et al., 2013). Besides that, it was discovered that inflammatory marker C-reactive protein was positively associated with the genus Veillonella (Diener et al., 2021) and negatively associated with the genus Clostridium (Allin et al., 2018). Likewise, interleukin-6 (IL-6) inversely correlated with the genus Blautia (Diener et al., 2021) whereas adiponectin was positively correlated with genus Clostridium (Lambeth et al., 2015).
Regarding dietary intake, five of the 15 studies assessed dietary intake using various measures (Egshatyan et al., 2016; Chen et al., 2019; Nuli et al., 2019; Chávez-Carbajal et al., 2020; Ericson et al., 2020). These included a 24-hour dietary recall (Chávez-Carbajal et al., 2020), a 3-day food record of at least 1 weekend or 1 weekday (Chen et al., 2019), a 4-day web-based food record, developed by the Swedish National Food Institute (Ericson et al., 2020), a quantitative evaluation of food intake based on a computer program ‘Analysis of Human Nutrition’ (Egshatyan et al., 2016) and a 12-month dietary recall that recorded frequency of 84 food items consumed daily, weekly, monthly, annually or never (Nuli et al., 2019).
Chen et al. (2019) reported significantly lower daily fibre intake and significantly higher fat intake in newDM when compared to the healthy controls. In newDM, they observed a positive correlation between cholesterol intake and Clostridium coccoides and Clostridium leptum while both carbohydrate and cholesterol intake were positively associated with Bacteroides fragilis. Fibre intake was also found to be positively associated with genus Bifidobacterium. On the other hand, the study noted negative correlation between carbohydrate and fat intake with family Enterobacteriaceae as well as between fat intake and genus Enterococcus (Chen et al., 2019).
Meanwhile, Nuli et al. (2019) found that daily intake of cereals, meat, salt and oil were excessive while vegetables, fish, shrimp and dairy products were insufficient in newDM when compared to nonDM. They reported the following positive correlations: energy and protein intake with genus Prevotella, carbohydrate intake with genus Dialister, cholesterol intake with genus Megasphaera and fibre intake with phylum Spirochaetae as well as negative correlation between fat intake and phylum Actinobacteria in both preDM and newDM (Zhang et al., 2013).
Chávez-Carbajal et al. (2020) noted increased daily energy and macronutrients intake among preDM when compared to nonDM. On the other hand, Egshatyan et al. (2016) noted higher levels of daily energy and carbohydrate consumption in newDM when compared to preDM. They also found a negative correlation between energy and cholesterol intake with Bifidobacterium, starch intake with Blautia and sugar intake with Catenibacterium. Meanwhile, a positive correlation was noted between starch intake with Bifidobacterium and carbohydrate intake with Prevotella. In this study, the study groups were stratified by dietary intake before analysing the association of gut microbiota with glucose intolerance. Among participants with glucose intolerance, the abundance of Blautia was increased even in those consuming lower carbohydrate or fat while high abundance of Serratia was increased among participants who consumed equal amounts of energy and carbohydrate. Regardless of energy intake per day, the glucose intolerance group had a significantly decreased abundance of the phylum Verrucomicrobia (Egshatyan et al., 2016).
Ericson et al. (2020) observed that a diet consisted of what they defined as a ‘health-conscious food pattern’ was associated with a lower prevalence of prediabetes. This association, according to their findings, is linked to BMI and gut microbiota, especially a higher abundance of Roseburia. They also found that in preDM, fibre intake had a positive association with genera Roseburia and Lachnospira and a negative association with Eubacterium (Karlsson et al., 2013).
This systematic review aimed to provide an overview of changes reported in the gut microbiota of subjects with preDM and newDM, unperturbed by pharmacotherapy, when compared to individuals with normal glucose tolerance. The comprehensive search of the databases and references list resulted in inclusion of a majority of high-quality studies that contributed to the review’s strength. We discuss herein the consistent differences in the composition of gut microbiota and their correlation with various parameters.
Bacteria in the gut predominantly belong to six phyla i.e., Firmicutes, Bacteroidetes, Actinobacteria, Proteobacteria, Fusobacteria, and Verrucomicrobia, with the two phyla Firmicutes and Bacteroidetes accounting for 90% of the total gut microbial composition (Rinninella et al., 2019). The changes in the abundance of specific Firmicutes and Bacteroidetes species and the overall increase or decrease in F/B ratio are often associated with several diseases. Studies in obesity and known T2DM have found that the F/B ratio increases (Silva et al., 2020), decreases (Levy et al., 2016; d’Hennezel et al., 2017; Parada Venegas et al., 2019) or even remains unchanged (Stojanov et al., 2020; Anhê et al., 2021). In the present review, a significant increase in Firmicutes along with a significant decrease in Bacteroidetes among newDM were observed (Bhute et al., 2017; Nuli et al., 2019; Zhao et al., 2019; Gaike et al., 2020). However only three (Zhao et al., 2019; Gaike et al., 2020; Li et al., 2020) out of the 18 studies reported on the F/B ratio, even so with contradictory findings. In past studies, an increase in Firmicutes or a higher F/B ratio has been linked with development of obesity, as Firmicutes are more efficient than Bacteroidetes in harvesting energy from food, thus contributing to the extra calories (Magne et al., 2020). While obesity is a major risk factor for T2DM, the disease is also characterized by a state of low-grade inflammation that precedes the onset of glucose intolerance. The pro-inflammatory cytokines are said to impair insulin signalling, increase permeability and inflammation in the intestinal epithelium, eventually leading to development of insulin resistance (Tamanai-Shacoori et al., 2017). While the notion remains that this continuous low-grade inflammatory state is caused by lipopolysachharide (LPS) produced by the Gram-negative gut microbiota (Cani et al., 2007), it is now evident that Bacteroidetes, the most abundant group of Gram-negative bacteria in the gut produces distinct subtypes of LPS with immunoinhibitory functions that prevents inflammation (d’Hennezel et al., 2017). Logically, a decrease in either of the two dominant Firmicutes or Bacteroidetes phyla, could possibly increase the relative abundance of other Gram-negative bacteria such as those belonging to the phylum Proteobacteria, hence inducing production of more pro-inflammatory LPS subtypes (Magne et al., 2020; Anhê et al., 2021). An increase in other phyla, however, may not necessarily further affect the F/B ratio (Stojanov et al., 2020). Moreover, the F/B ratio does not consider compositional changes that may be occurring in the larger variety of family, genus and species taxonomic levels of each phylum. The relevance of the F/B ratio to serve as a disease marker for metabolic diseases is therefore inconclusive. Another important microbiota-associated factor in health and disease is the production of short-chain fatty acids (SCFAs). SCFAs, namely acetate, propionate and butyrate are metabolic products of fibre fermentation by bacteria in the gut. They are shown to exert many beneficial effects on human metabolism and immune system (Parada Venegas et al., 2019; Silva et al., 2020). Firmicutes are the primary producers of butyrate while Bacteroidetes mainly produce acetate and propionate (Levy et al., 2016; Parada Venegas et al., 2019). Gut microbiota dysbiosis has been shown to alter SCFA production, thereby affecting the epigenetic regulation of genes modulating insulin resistance and inflammatory reactions seen in T2DM (Remely et al., 2014). In relation to this, although an increase in phyla Firmicutes was noted, several members of this phyla were constantly found to be decreased in the disease groups. They were Faecalibacterium prausnitzii, Roseburia, Dialister and Flavonifractor. The role of these organisms as biomarkers of health is well established. Their beneficial effects are mainly attributed to their ability to produce SCFAs, especially butyrate, that play a major role in maintaining intestinal barrier integrity, energy homeostasis, attenuating inflammation and modulating glycaemic response (Tamanai-Shacoori et al., 2017; Martín et al., 2018; Mukherjee et al., 2020). Previous studies also found F. prausnitzii (Graessler et al., 2013; Remely et al., 2014; Candela et al., 2016), Dialister (Almugadam et al., 2020) and Roseburia (Forslund et al., 2015) to be reduced among known T2DM patients on medication. Taken together, these findings indicate that these bacteria are depleted in the gut microbiome prior to the onset of diabetes and may remain so even after treatment is initiated.
Other bacteria noted to be decreased in the disease groups in this review were Alistipes of phylum Bacteroidetes, Akkermansia muciniphila, of phylum Verrucomicrobia and Haemophilus, of phylum Proteobacteria. Alistipes, however was found to be increased in abundance among known T2DM patients (Wu et al., 2010; Qin et al., 2012). Indeed this bacterium is known to have protective effects against certain diseases including cardiovascular disease, while also being pathogenic in others due to its inflammatory potential (Parker et al., 2020). Previous studies showed that the mucin-degrading and gut barrier protecting A. muciniphila improve glucose tolerance in high fat diet-induced diabetic mice upon metformin treatment (Shin et al., 2014; de la Cuesta-Zuluaga et al., 2017). On the other hand, a decrease in abundance of this bacterium increases gut permeability, a known characteristic in T2DM progression (Cani et al., 2008). The decrease in Haemophilus is in agreement with a study investigating the gut microbiota pattern in women with active vs sedentary lifestyle that noted an increase in this genus, along with other health-promoting bacterial species Faecalibacterium prausnitzii, Roseburia hominis and Akkermansia muciniphila in active women (Bressa et al., 2017). This is unexpected given that the genus Haemophilus is a mucosal pathogen and its abundance has been associated with varying pathogenicity in infections (Nørskov-Lauritsen, 2014), multiple sclerosis (Chen et al., 2016) and colorectal carcinoma (Liu et al., 2020).
On the other hand, the bacteria found to have increased in abundance among the disease groups included Firmicutes: Streptococcus, Veillonella and Lactobacillus, specifically L. ruminis, L. gasseri and L.salivarius as well as Escherichia (a Proteobacteria) and Collinsella (an Actinobacteria). Streptococcus, Escherichia and Collinsella are all known gut inhabitants whose abundance are associated with several inflammatory diseases including T2DM (Qin et al., 2012; Candela et al., 2016). Increased abundance of Escherichia coli is also linked with increased microbial infections in diabetic patients (Wiwanitkit, 2011). All three genera are positively associated with animal-based diet consumption and studies have reported that these bacteria can be successfully reduced through fibre-rich and plant-based dietary interventions (Candela et al., 2016; van Soest et al., 2020). Bacteria belonging to genus Veillonella are Gram-negative bacteria (unlike most Firmicutes) (Megrian et al., 2020), however there is little evidence for their role in health and disease to date. The immunomodulatory and probiotic properties of Lactobacillus are well established. However, along with its association seen herein with preDM and newDM, the present review also found noteworthy positive association between the genus Lactobacillus and glycaemic markers including FPG, HbA1c and the associated HOMA-IR index. The abundance of Lactobacillus has also been linked to chronic inflammation seen in known diabetic subjects (Zeuthen et al., 2006; Larsen et al., 2010; Lê et al., 2013). Although probiotic strains of Lactobacillus have been found to have beneficial anti-diabetic effects in mouse models (Yun et al., 2009; Park et al., 2015; Lee et al., 2018), it is likely that the effects of endogenous Lactobacillus species towards health and disease is strain dependent and further studies are required to investigate their direct effect on T2DM.
Overall, the increased levels of glycaemic and pro-inflammatory markers along with low diversity of gut bacteria among the preDM and newDM generally observed in this review suggest the possibility of gut bacteria- associated inflammation-induced environment preceding the development of T2DM. Although the studies included in this review found significant association between clinical biomarkers and the abundance of specific bacterial groups, no consistent findings were observed between studies, with the exception of the correlation between glycaemic markers and Lactobacillus abundance. Similarly, no consistent findings between studies were noted in correlation between dietary intake and specific gut bacterial composition. However, the studies were able to conclude that a less healthy food pattern including increased carbohydrate, fat or energy intake or a reduced fibre intake correlated with prevalence of preDM or newDM and differences in dietary intake caused significant variation in specific bacterial abundance.
One of the limitations of the present review is that it only summarises studies investigating gut microbiota based on composition found in faecal samples. There is a need for more studies to look into microbiota of mucosal biopsies in addition to faeces, in order to differentiate mucosa-associated bacteria from the total composition present in faeces. This is because mucosa-associated bacteria may have a more pertinent role in the pathogenesis of disease. It is also important to emphasise that findings from observational studies selected herein do not conclusively establish whether changes seen in microbiota composition were a cause or effect of glucose intolerance. Hence, bacteria found to be decreased or increased in the disease groups could not be conclusively termed as being ‘protective’ or ‘pro-diabetic’, respectively.
Studies involving taxonomic analyses of microbiota have several biases. One of it is that compositional analyses of microbiota requires that the microbial proportions to be summed to a 100% total, thus reporting the relative abundance and not the absolute composition of the microorganisms present in the faecal samples of participants. Thus, if one bacterial group appears to be reduced, the others will naturally appear to be increased and vice versa. Differences in sampling fractions may also tend to introduce false positives and false negatives (Lin and Peddada, 2020).
Another challenge in analysing gut microbiome data across studies is the biases caused by the heterogenous methodology adapted. This limits the comparability of studies and leads to ambiguous results across similar studies. A meta-analysis was also not workable for the same reason. Although all studies targeted the 16S rRNA gene, factors that differed across the studies i.e. the choice of sequencing region, pipelines and databases used for bioinformatics analysis influenced the results produced. Moreover, the usage of different diversity indexes and statistical analysis affects the comparability of microbial diversity between the studies. The choice of the diversity index affects the interpretation of the microbiome data and leads to a lack of generalizable results across the studies. Besides methodological limitations, the use of alpha diversity as a biomarker in health and disease may be confounded by colonic transit time (Roager et al., 2016) and stool consistency (Falony et al., 2016).
More importantly, the taxonomic diversity exhibited by these analyses does not take into account, the functional redundancy among members of the microbiota. Taxonomically distinct bacterial species are able to perform similar metabolic functions and as such, taxonomic variation does not reflect functional variation (Louca et al., 2018). Therefore, profiling of the microbial metabolic function would be of more significance to assess impact of the microbiota on the human host in health and disease.
The 18 studies included herein were found to have heterogeneity in methodology and inconsistencies in the findings on gut microbial changes observed among preDM and newDM when compared to nonDM. By focusing on changes that were similarly reported in two or more studies, it was evident that certain bacteria were found to be increased (Lactobacillus, Streptococcus, Escherichia, Veillonella and Collinsella) and/or decreased (Faecalibacterium prausnitzii, Roseburia, Dialister, Flavonifractor, Alistipes, Haemophilus and Akkermansia muciniphila) in preDM and newDM. These alterations were however not consistent across all studies included, hence emphasising the uncertainty that lies in this field of study. The increased presence of Lactobacillus in preDM and newDM along with its positive correlation with glycaemic markers were also inconsistent observations. This renders a need for more investigation on the species/strain-specific role of this genus in T2DM disease progression and glucose regulation mechanism. Healthier food intake inversely correlated with prevalence of preDM and newDM, while differences in dietary intake caused significant variation in specific bacterial abundances. More studies should investigate the correlation of clinical biomarkers and dietary intake with gut bacterial composition in prediabetes and early T2DM to establish more consistent associations.
SP and NA conceived the review protocol. GL and SP carried the systematic searches and extracted the data. GL, NB and NA performed the quality assessment of the selected papers. MM, NB, MO, FM, FA, NM, ZI and SP critically checked the extracted data. GL wrote the first draft of the manuscript. SP reviewed and edited the entire manuscript. MM, NA and BL critically reviewed the final manuscript. All authors contributed to writing and revising the manuscript.
This systematic review was conducted as part of a study funded by the Fundamental Research Grant Scheme from the Ministry of Education, Malaysia (FRGS/1/2018/SKK08/USIM/02/1). Geetha Letchumanan is supported by the same grant.
The authors declare that the research was conducted in the absence of any commercial or financial relationships that could be construed as a potential conflict of interest.
All claims expressed in this article are solely those of the authors and do not necessarily represent those of their affiliated organizations, or those of the publisher, the editors and the reviewers. Any product that may be evaluated in this article, or claim that may be made by its manufacturer, is not guaranteed or endorsed by the publisher.
We gratefully acknowledge Gerald W. Tannock, Professor Emeritus of the University of Otago, New Zealand for his valuable comments on an earlier draft of this paper.
The Supplementary Material for this article can be found online at: https://www.frontiersin.org/articles/10.3389/fcimb.2022.943427/full#supplementary-material
Alberti, K. G. M. M., Zimmet, P. Z. (1998). Definition, Diagnosis and Classification of Diabetes Mellitus and its Complications. Part 1: Diagnosis and Classification of Diabetes Mellitus. Provisional Report of a WHO Consultation. Diabetic Med. 15 (7), 539–553. doi: 10.1002/(SICI)1096-9136(199807)15:7%3C539::AID-DIA668%3E3.0.CO;2-S
Allin, K. H., Tremaroli, V., Caesar, R., Jensen, B. A. H., Damgaard, M. T. F., Bahl, M. I., et al. (2018). Aberrant Intestinal Microbiota in Individuals With Prediabetes. Diabetologia 61 (4), 810–820. doi: 10.1007/s00125-018-4550-1
Almugadam, B. S., Liu, Y., Chen, S.-M., Wang, C.-H., Shao, C.-Y., Ren, B.-W., et al. (2020). Alterations of Gut Microbiota in Type 2 Diabetes Individuals and the Confounding Effect of Antidiabetic Agents. J. Diabetes Res. 2020, 7253978. doi: 10.1155/2020/7253978
Anhê, F. F., Barra, N. G., Cavallari, J. F., Henriksbo, B. D., Schertzer, J. D. (2021). Metabolic Endotoxemia is Dictated by the Type of Lipopolysaccharide. Cell Rep. 36 (11), 109691. doi: 10.1016/j.celrep.2021.109691
Bhute, S. S., Suryavanshi, M. V., Joshi, S. M., Yajnik, C. S., Shouche, Y. S., Ghaskadbi, S. S. (2017). Gut Microbial Diversity Assessment of Indian Type-2-Diabetics Reveals Alterations in Eubacteria, Archaea, and Eukaryotes. Front. Microbiol. 8 (214). doi: 10.3389/fmicb.2017.00214
Bjerre, R. D., Bandier, J., Skov, L., Engstrand, L., Johansen, J. D. (2017). The Role of the Skin Microbiome in Atopic Dermatitis: A Systematic Review. Br. J. Dermatol. 177 (5), 1272–1278. doi: 10.1111/bjd.15390
Bressa, C., Bailén-Andrino, M., Pérez-Santiago, J., González-Soltero, R., Pérez, M., Montalvo-Lominchar, M. G., et al. (2017). Differences in Gut Microbiota Profile Between Women With Active Lifestyle and Sedentary Women. PloS One 12 (2), e0171352. doi: 10.1371/journal.pone.0171352
Candela, M., Biagi, E., Soverini, M., Consolandi, C., Quercia, S., Severgnini, M., et al. (2016). Modulation of Gut Microbiota Dysbioses in Type 2 Diabetic Patients by Macrobiotic Ma-Pi 2 Diet. Br. J. Nutr. 116 (1), 80–93. doi: 10.1017/S0007114516001045
Cani, P. D., Amar, J., Iglesias, M. A., Poggi, M., Knauf, C., Bastelica, D., et al. (2007). Metabolic Endotoxemia Initiates Obesity and Insulin Resistance. Diabetes 56 (7), 1761–1772. doi: 10.2337/db06-1491
Cani, P. D., Bibiloni, R., Knauf, C., Waget, A., Neyrinck, A. M., Delzenne, N. M., et al. (2008). Changes in Gut Microbiota Control Metabolic Endotoxemia-Induced Inflammation in High-Fat Diet–Induced Obesity and Diabetes in Mice. Diabetes 57 (6), 1470–1481. doi: 10.2337/db07-1403
Centers for Disease Control Prevention (2014). National Diabetes Statistics Report: Estimates of Diabetes and its Burden in the United States Vol. 2014 (Atlanta, GA: US Department of Health and Human Services).
Chávez-Carbajal, A., Pizano-Zárate, M. L., Hernández-Quiroz, F., Ortiz-Luna, G. F., Morales-Hernández, R. M., De Sales-Millán, A., et al. (2020). Characterization of the Gut Microbiota of Individuals at Different T2D Stages Reveals a Complex Relationship With the Host. Microorganisms 8 (1), 94. doi: 10.3390/microorganisms8010094
Chen, J., Chia, N., Kalari, K. R., Yao, J. Z., Novotna, M., Paz Soldan, M. M., et al. (2016). Multiple Sclerosis Patients Have a Distinct Gut Microbiota Compared to Healthy Controls. Sci. Rep. 6, 28484. doi: 10.1038/srep28484
Chen, P.-C., Chien, Y.-W., Yang, S.-C. (2019). The Alteration of Gut Microbiota in Newly Diagnosed Type 2 Diabetic Patients. Nutrition 63-64, 51–56. doi: 10.1016/j.nut.2018.11.019
de la Cuesta-Zuluaga, J., Mueller, N. T., Corrales-Agudelo, V., Velásquez-Mejía, E. P., Carmona, J. A., Abad, J. M., et al. (2017). Metformin Is Associated With Higher Relative Abundance of Mucin-Degrading Akkermansia Muciniphila and Several Short-Chain Fatty Acid-Producing Microbiota in the Gut. Diabetes Care 40 (1), 54–62. doi: 10.2337/dc16-1324
d’Hennezel, E., Abubucker, S., Murphy, L. O., Cullen, T. W., Lozupone, C. (2017). Total Lipopolysaccharide From the Human Gut Microbiome Silences Toll-Like Receptor Signaling. mSystems 2 (6), e00046–e00017. doi: 10.1128/mSystems.00046-17
Diener, C., Reyes-Escogido, M., Jimenez-Ceja, L. M., Matus, M., Gomez-Navarro, C. M., Chu, N. D., et al. (2021). Progressive Shifts in the Gut Microbiome Reflect Prediabetes and Diabetes Development in a Treatment-Naive Mexican Cohort. Front. Endocrinol. 11 (1009). doi: 10.3389/fendo.2020.602326
Eades, C. E., Leese, G. P., Evans, J. M. M. (2014). Incidence of Impaired Glucose Regulation and Progression to Type 2 Diabetes Mellitus in the Tayside Region of Scotland. Diabetes Res. Clin. Practice 104 (1), e16–ee9. doi: 10.1016/j.diabres.2014.01.012
Egshatyan, L., Kashtanova, D., Popenko, A., Tkacheva, O., Tyakht, A., Alexeev, D., et al. (2016). Gut Microbiota and Diet in Patients With Different Glucose Tolerance. Endocr. Connect. 5 (1), 1–9. doi: 10.1530/EC-15-0094
Ericson, U., Brunkwall, L., Hellstrand, S., Nilsson, P. M., Orho-Melander, M. (2020). A Health-Conscious Food Pattern Is Associated With Prediabetes and Gut Microbiota in the Malmö Offspring Study. J. Nutr. 150 (4), 861–872. doi: 10.1093/jn/nxz293
Falony, G., Joossens, M., Vieira-Silva, S., Wang, J., Darzi, Y., Faust, K., et al. (2016). Population-Level Analysis of Gut Microbiome Variation. Science 352 (6285), 560–564. doi: 10.1126/science.aad3503
Forslund, K., Hildebrand, F., Nielsen, T., Falony, G., Le Chatelier, E., Sunagawa, S., et al. (2015). Disentangling Type 2 Diabetes and Metformin Treatment Signatures in the Human Gut Microbiota. Nature 528 (7581), 262–266. doi: 10.1038/nature15766
Gaike, A. H., Paul, D., Bhute, S., Dhotre, D. P., Pande, P., Upadhyaya, S., et al. (2020). The Gut Microbial Diversity of Newly Diagnosed Diabetics But Not of Prediabetics Is Significantly Different From That of Healthy Nondiabetics. mSystems 5 (2), e00578–e00519. doi: 10.1128/mSystems.00578-19
Ghaemi, F., Fateh, A., Sepahy, A. A., Zangeneh, M., Ghanei, M., Siadat, S. D. (2020). Intestinal Microbiota Composition in Iranian Diabetic, Pre-Diabetic and Healthy Individuals. J. Diabetes Metab. Disord. 19 (2), 1199–1203. doi: 10.1007/s40200-020-00625-x
Graessler, J., Qin, Y., Zhong, H., Zhang, J., Licinio, J., Wong, M. L., et al. (2013). Metagenomic Sequencing of the Human Gut Microbiome Before and After Bariatric Surgery in Obese Patients With Type 2 Diabetes: Correlation With Inflammatory and Metabolic Parameters. Pharmacogenomics J. 13 (6), 514–522. doi: 10.1038/tpj.2012.43
Gurung, M., Li, Z., You, H., Rodrigues, R., Jump, D. B., Morgun, A., et al. (2020). Role of Gut Microbiota in Type 2 Diabetes Pathophysiology. EBioMedicine 51, 102590. doi: 10.1016/j.ebiom.2019.11.051
Han, J. L., Lin, H. L. (2014). Intestinal Microbiota and Type 2 Diabetes: From Mechanism Insights to Therapeutic Perspective. World J. Gastroenterol. 20 (47), 17737–17745. doi: 10.3748/wjg.v20.i47.17737
International Diabetes Federation (IDF) (2019). Clinical Practice Recommendations for Managing Type 2 Diabetes in Primary Care. 9th edn (Brussel, Belgium: International Diabetes Federation).
Karlsson, F. H., Tremaroli, V., Nookaew, I., Bergström, G., Behre, C. J., Fagerberg, B., et al. (2013). Gut Metagenome in European Women With Normal, Impaired and Diabetic Glucose Control. Nature 498 (7452), 99–103. doi: 10.1038/nature12198
Lambeth, S. M., Carson, T., Lowe, J., Ramaraj, T., Leff, J. W., Luo, L., et al. (2015). Composition, Diversity and Abundance of Gut Microbiome in Prediabetes and Type 2 Diabetes. J. Diabetes Obes. 2 (3), 1–7. doi: 10.154362F2376-0949.15.031
Larsen, N., Vogensen, F. K., van den Berg, F. W., Nielsen, D. S., Andreasen, A. S., Pedersen, B. K., et al. (2010). Gut Microbiota in Human Adults With Type 2 Diabetes Differs From non-Diabetic Adults. PloS One 5 (2), e9085. doi: 10.1371/journal.pone.0009085
Lee, E., Jung, S.-R., Lee, S.-Y., Lee, N.-K., Paik, H.-D., Lim, S.-I. (2018). Lactobacillus Plantarum Strain Ln4 Attenuates Diet-Induced Obesity, Insulin Resistance, and Changes in Hepatic mRNA Levels Associated With Glucose and Lipid Metabolism. Nutrients 10 (5), 643. doi: 10.3390/nu10050643
Lê, K.-A., Li, Y., Xu, X., Liu, T., Yang, W., He, F., et al. (2013). Alterations in Fecal Lactobacillus and Bifidobacterium Species in Type 2 Diabetic Patients in Southern China Population. Front. Physiol. 3 (496). doi: 10.3389/fphys.2012.00496
Levy, M., Thaiss, C. A., Elinav, E. (2016). Metabolites: Messengers Between the Microbiota and the Immune System. Genes Dev. 30 (14), 1589–1597. doi: 10.1101/gad.284091.116
Li, L., Li, C., Lv, M., Hu, Q., Guo, L., Xiong, D. (2020). Correlation Between Alterations of Gut Microbiota and miR-122-5p Expression in Patients With Type 2 Diabetes Mellitus. Ann. Transl. Med. 8 (22), 1481. doi: 10.21037/atm-20-6717
Lin, H., Peddada, S. D. (2020). Analysis of Microbial Compositions: A Review of Normalization and Differential Abundance Analysis. NPJ Biofilms Microbiomes 6 (1), 60–. doi: 10.1038/s41522-020-00160-w
Liu, W., Zhang, R., Shu, R., Yu, J., Li, H., Long, H., et al. (2020). Study of the Relationship Between Microbiome and Colorectal Cancer Susceptibility Using 16srrna Sequencing. BioMed. Res. Int. 2020, 7828392. doi: 10.1155/2020/7828392
Louca, S., Polz, M. F., Mazel, F., Albright, M. B. N., Huber, J. A., O'Connor, M. I., et al. (2018). Function and Functional Redundancy in Microbial Systems. Nat. Ecol. Evol. 2 (6), 936–943. doi: 10.1038/s41559-018-0519-1
Magne, F., Gotteland, M., Gauthier, L., Zazueta, A., Pesoa, S., Navarrete, P., et al. (2020). The Firmicutes/Bacteroidetes Ratio: A Relevant Marker of Gut Dysbiosis in Obese Patients? Nutrients 12 (5), 1474. doi: 10.3390/nu12051474
Martín, R., Bermúdez-Humarán, L. G., Langella, P. (2018). Searching for the Bacterial Effector: The Example of the Multi-Skilled Commensal Bacterium Faecalibacterium Prausnitzii. Front. Microbiol. 9 (346). doi: 10.3389/fmicb.2018.00346
Megrian, D., Taib, N., Witwinowski, J., Beloin, C., Gribaldo, S. (2020). One or Two Membranes? Diderm Firmicutes Challenge the Gram-Positive/Gram-Negative Divide. Mol. Microbiol. 113 (3), 659–671. doi: 10.1111/mmi.14469
Moher, D., Liberati, A., Tetzlaff, J., Altman, D. G. (2009). Preferred Reporting Items for Systematic Reviews and Meta-Analyses: The PRISMA Statement. J. Clin. Epidemiol. 62 (10), 1006–1012. doi: 10.1016/j.jclinepi.2009.06.005
Mukherjee, A., Lordan, C., Ross, R. P., Cotter, P. D. (2020). Gut Microbes From the Phylogenetically Diverse Genus Eubacterium and Their Various Contributions to Gut Health. Gut Microbes 12 (1), 1802866. doi: 10.1080/19490976.2020.1802866
Nørskov-Lauritsen, N. (2014). Classification, Identification, and Clinical Significance of Haemophilus and Aggregatibacter Species With Host Specificity for Humans. Clin. Microbiol. Rev. 27 (2), 214–240. doi: 10.1128/CMR.00103-13
Nuli, R., Cai, J., Kadeer, A., Zhang, Y., Mohemaiti, P. (2019). Integrative Analysis Toward Different Glucose Tolerance-Related Gut Microbiota and Diet. Front. Endocrinol. 10. doi: 10.3389/fendo.2019.00295
Parada Venegas, D., de la Fuente, M. K., Landskron, G., González, M. J., Quera, R., Dijkstra, G., et al. (2019). Short Chain Fatty Acids (SCFAs)-Mediated Gut Epithelial and Immune Regulation and Its Relevance for Inflammatory Bowel Diseases. Front. Immunol. 10 (277). doi: 10.3389/fimmu.2019.00277
Parker, B. J., Wearsch, P. A., Veloo, A. C. M., Rodriguez-Palacios, A. (2020). The Genus Alistipes: Gut Bacteria With Emerging Implications to Inflammation, Cancer, and Mental Health. Front. Immunol. 11. doi: 10.3389/fimmu.2020.00906
Park, K.-Y., Kim, B., Hyun, C.-K. (2015). Lactobacillus Rhamnosus GG Improves Glucose Tolerance Through Alleviating ER Stress and Suppressing Macrophage Activation in Db/Db Mice. J. Clin. Biochem. Nutr. 56 (3), 240–246. doi: 10.3164/jcbn.14-116
Pathmanathan, S.G., Letchumanan, G., Abdullah, N., Ismail, Z., Baharom, N., Manzor, N. F. M., et al (2020). Gut Microbiota Composition inNewly Diagnosed Type 2 Diabetic and Prediabetic Individuals Versus non-Diabetic Individuals. A Systematic Review. Available at: https://www.crd.york.ac.uk/prospero/display_record.php?ID=CRD42020160458
Qin, J., Li, Y., Cai, Z., Li, S., Zhu, J., Zhang, F., et al. (2012). A Metagenome-Wide Association Study of Gut Microbiota in Type 2 Diabetes. Nature 490 (7418), 55–60. doi: 10.1038/nature11450
Remely, M., Aumueller, E., Merold, C., Dworzak, S., Hippe, B., Zanner, J., et al. (2014). Effects of Short Chain Fatty Acid Producing Bacteria on Epigenetic Regulation of FFAR3 in Type 2 Diabetes and Obesity. Gene 537 (1), 85–92. doi: 10.1016/j.gene.2013.11.081
Rinninella, E., Raoul, P., Cintoni, M., Franceschi, F., Miggiano, G. A. D., Gasbarrini, A., et al. (2019). What is the Healthy Gut Microbiota Composition? A Changing Ecosystem Across Age, Environment, Diet, and Diseases. Microorganisms 7 (1):14. doi: 10.3390/microorganisms7010014
Roager, H. M., Hansen, L. B. S., Bahl, M. I., Frandsen, H. L., Carvalho, V., Gøbel, R. J., et al. (2016). Colonic Transit Time is Related to Bacterial Metabolism and Mucosal Turnover in the Gut. Nat. Microbiol. 1 (9), 16093. doi: 10.1038/nmicrobiol.2016.93
Saeedi, P., Petersohn, I., Salpea, P., Malanda, B., Karuranga, S., Unwin, N., et al. (2019). Global and Regional Diabetes Prevalence Estimates for 2019 and Projections for 2030 and 2045: Results From the International Diabetes Federation Diabetes Atlas, 9(Th) Edition. Diabetes Res. Clin. Pract. 157, 107843. doi: 10.1016/j.diabres.2019.107843
Shin, N. R., Lee, J. C., Lee, H. Y., Kim, M. S., Whon, T. W., Lee, M. S., et al. (2014). An Increase in the Akkermansia Spp. Population Induced by Metformin Treatment Improves Glucose Homeostasis in Diet-Induced Obese Mice. Gut 63 (5), 727–735. doi: 10.1136/gutjnl-2012-303839
Silva, Y. P., Bernardi, A., Frozza, R. L. (2020). The Role of Short-Chain Fatty Acids From Gut Microbiota in Gut-Brain Communication. Front. Endocrinol. 11, 25. doi: 10.3389/fendo.2020.00025
Stojanov, S., Berlec, A., Štrukelj, B. (2020). The Influence of Probiotics on the Firmicutes/Bacteroidetes Ratio in the Treatment of Obesity and Inflammatory Bowel Disease. Microorganisms 8 (11):1715. doi: 10.3390/microorganisms8111715
Tamanai-Shacoori, Z., Smida, I., Bousarghin, L., Loreal, O., Meuric, V., Fong, S. B., et al. (2017). Roseburia Spp.: A Marker of Health? Future Microbiol. 12, 157–170. doi: 10.2217/fmb-2016-0130
van Soest, A. P. M., Hermes, G. D. A., Berendsen, A. A. M., van de Rest, O., Zoetendal, E. G., Fuentes, S., et al. (2020). Associations Between Pro- and Anti-Inflammatory Gastro-Intestinal Microbiota, Diet, and Cognitive Functioning in Dutch Healthy Older Adults: The NU-AGE Study. Nutrients 12 (11):3471. doi: 110.3390/nu12113471
Wang, L., Yu, X., Xu, X., Ming, J., Wang, Z., Gao, B., et al. (2021). The Fecal Microbiota Is Already Altered in Normoglycemic Individuals Who Go on to Have Type 2 Diabetes. Front. Cell. Infect. Microbiol. 11 (1):3471. doi: 10.3389/fcimb.2021.598672
Wells, G., Shea, B., O’Connell, D., Peterson, J., Welch, V., Losos, M., et al. (2014). Newcastle-Ottawa Quality Assessment Scale Cohort Studies. The Ottawa Hospital Research Institute
Wiwanitkit, V. (2011). Outbreak of Escherichia Coli and Diabetes Mellitus. Indian J. Endocrinol. Metab. 15 (Suppl 1), S70–S71. doi: 10.4103/2230-8210.83050
Worldometers (2021) World Population. Available at: https://www.worldometers.info/world-population/.
Wu, X., Ma, C., Han, L., Nawaz, M., Gao, F., Zhang, X., et al. (2010). Molecular Characterisation of the Faecal Microbiota in Patients With Type II Diabetes. Curr. Microbiol. 61 (1), 69–78. doi: 10.1007/s00284-010-9582-9
Wu, H., Tremaroli, V., Schmidt, C., Lundqvist, A., Olsson, L. M., Krämer, M., et al. (2020). The Gut Microbiota in Prediabetes and Diabetes: A Population-Based Cross-Sectional Study. Cell Metab. 32 (3), 379–90.e3. doi: 10.1016/j.cmet.2020.06.011
Yun, S. I., Park, H. O., Kang, J. H. (2009). Effect of Lactobacillus Gasseri BNR17 on Blood Glucose Levels and Body Weight in a Mouse Model of Type 2 Diabetes. J. Appl. Microbiol. 107 (5), 1681–1686. doi: 10.1111/j.1365-2672.2009.04350.x
Zeuthen, L. H., Christensen, H. R., Frøkiaer, H. (2006). Lactic Acid Bacteria Inducing a Weak Interleukin-12 and Tumor Necrosis Factor Alpha Response in Human Dendritic Cells Inhibit Strongly Stimulating Lactic Acid Bacteria But Act Synergistically With Gram-Negative Bacteria. Clin. Vaccine Immunol. 13 (3), 365–375. doi: 10.1128/CVI.13.3.365-375.2006
Zhang, X., Shen, D., Fang, Z., Jie, Z., Qiu, X., Zhang, C., et al. (2013). Human Gut Microbiota Changes Reveal the Progression of Glucose Intolerance. PloS One 8 (8), e71108–e7110e. doi: 10.1371/journal.pone.0071108
Zhao, L., Lou, H., Peng, Y., Chen, S., Zhang, Y., Li, X. (2019). Comprehensive Relationships Between Gut Microbiome and Faecal Metabolome in Individuals With Type 2 Diabetes and its Complications. Endocrine 66 (3), 526–537. doi: 10.1007/s12020-019-02103-8
Keywords: gut microbiota, type 2 diabetes, prediabetes, 16S rRNA sequencing, systematic review
Citation: Letchumanan G, Abdullah N, Marlini M, Baharom N, Lawley B, Omar MR, Mohideen FBS, Addnan FH, Nur Fariha MM, Ismail Z and Pathmanathan SG (2022) Gut Microbiota Composition in Prediabetes and Newly Diagnosed Type 2 Diabetes: A Systematic Review of Observational Studies. Front. Cell. Infect. Microbiol. 12:943427. doi: 10.3389/fcimb.2022.943427
Received: 13 May 2022; Accepted: 20 June 2022;
Published: 15 August 2022.
Edited by:
Benjamin A. H. Jensen, University of Copenhagen, DenmarkReviewed by:
Fernando Forato Anhe, Laval University, CanadaCopyright © 2022 Letchumanan, Abdullah, Marlini, Baharom, Lawley, Omar, Mohideen, Addnan, Nur Fariha, Ismail and Pathmanathan. This is an open-access article distributed under the terms of the Creative Commons Attribution License (CC BY). The use, distribution or reproduction in other forums is permitted, provided the original author(s) and the copyright owner(s) are credited and that the original publication in this journal is cited, in accordance with accepted academic practice. No use, distribution or reproduction is permitted which does not comply with these terms.
*Correspondence: Siva Gowri Pathmanathan, Z293cmlAdXNpbS5lZHUubXk=
Disclaimer: All claims expressed in this article are solely those of the authors and do not necessarily represent those of their affiliated organizations, or those of the publisher, the editors and the reviewers. Any product that may be evaluated in this article or claim that may be made by its manufacturer is not guaranteed or endorsed by the publisher.
Research integrity at Frontiers
Learn more about the work of our research integrity team to safeguard the quality of each article we publish.