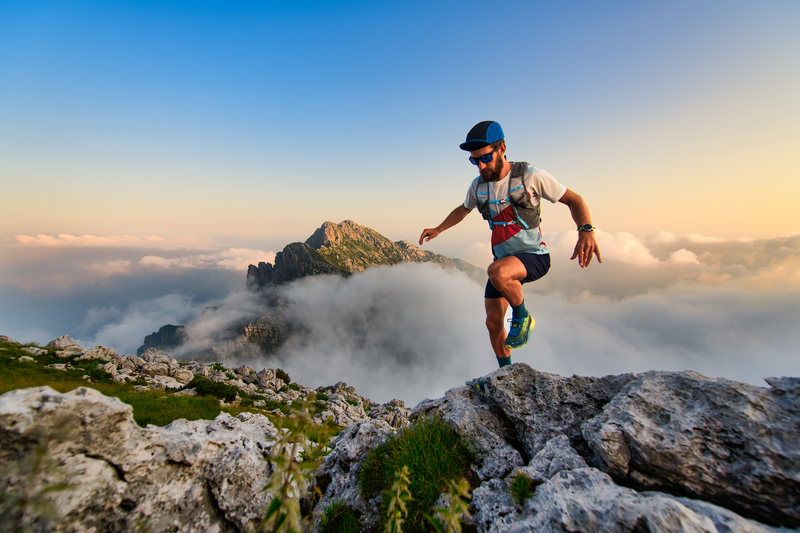
94% of researchers rate our articles as excellent or good
Learn more about the work of our research integrity team to safeguard the quality of each article we publish.
Find out more
ORIGINAL RESEARCH article
Front. Cell. Infect. Microbiol. , 11 July 2022
Sec. Bacteria and Host
Volume 12 - 2022 | https://doi.org/10.3389/fcimb.2022.907239
Objective: Increasing evidence shows a close relationship between gut microbiota and major depressive disorder (MDD), but the specific mechanisms remain unknown. This study was conducted to explore differential gut microbiota compositions related to the severity of MDD.
Methods: Healthy controls (HC) (n = 131) and MDD patients (n = 130) were included. MDD patients with Hamilton Depression Rating Scale (HDRS) score <25 and ≥25 were assigned into moderate (n = 72) and severe (n = 58) MDD groups, respectively. Univariate and multivariate analyses were used to analyze the gut microbiota compositions at the genus level.
Results: Thirty-six and 27 differential genera were identified in moderate and severe MDD patients, respectively. The differential genera in moderate and severe MDD patients mainly belonged to three (Firmicutes, Actinobacteriota, and Bacteroidota) and two phyla (Firmicutes and Bacteroidota), respectively. One specific covarying network from phylum Actinobacteriota was identified in moderate MDD patients. In addition, five genera (Collinsella, Eggerthella, Alistipes, Faecalibacterium, and Flavonifractor) from the shared differential genera by two MDD groups had a fair efficacy in diagnosing MDD from HC (AUC = 0.786).
Conclusions: Our results were helpful for further exploring the role of gut microbiota in the pathogenesis of depression and developing objective diagnostic methods for MDD.
Major depressive disorder (MDD) is a common but serious neuropsychiatric disorder that can greatly affect the patients’ quality of life (Martins-de-Souza, 2014; Zhu et al., 2020; Liu et al., 2021; Tian et al., 2021). It is mainly characterized by emptiness or hopelessness, loss of interest, and sleep disturbances (Rana et al., 2021). Previous studies reported that MDD was closely related to hippocampal atrophy, disorder of the hypothalamic–pituitary–adrenal (HPA) axis, and reduction of glial cells in the prefrontal cortex (Ongür et al., 1998; Campbell and Macqueen, 2004; Pariante and Lightman, 2008). However, commonly accepted theories about the pathogenesis of MDD are still not available. Meanwhile, the first-line treatment according to these theories can only alleviate symptoms in about half of MDD patients (Al-Harbi, 2012), and there are no validated biomarkers for objective diagnosis of MDD nowadays. Thus, it is urgently needed to further study the pathogenesis of MDD from new perspectives.
Gut microbiota plays an important role in maintaining the host’s health, and many researchers pay attention to the cross talk between the gut and brain (Han et al., 2020; Qiao et al., 2020; Kovács et al., 2021; Khoshkam et al., 2021). Mounting evidence shows that gut microbiota can affect the host’s brain functions and behaviors via the “microbiota–gut–brain” axis (Chen et al., 2021; Rajput et al., 2021; Ding et al., 2021; Dong et al., 2021). In our previous work, we found significant differences in gut microbiota compositions between MDD patients and healthy controls (HC) (Chen et al., 2020; Bai et al., 2021), and these differences were specifically relative to bipolar disorder and schizophrenia (Zheng et al., 2019; Zheng et al., 2020). Other researchers also found that some bacterial taxa, such as Flavonifractor and Faecalibacterium, changed in patients with depression (Coello et al., 2019; Zhou et al., 2020; Coello et al., 2021). Using an animal depression model, we reported that gut microbiota could induce depression-like behaviors by regulating the host’s metabolism (Zheng et al., 2016) and that glycerophospholipid metabolism might be the vital node between microbiota and depression (Tian et al., 2022). These findings suggested that further exploring the role of gut microbiota in the onset of depression may be helpful for revealing the pathogenesis of MDD.
Many metabolites produced by gut microbiota are closely related to health (Lu et al., 2021; Zhong et al., 2021; Xie et al., 2021). Short-chain fatty acids (SCFAs), as the main products of gut microbiota, have been found to change in many diseases such as cardiovascular disease and autism (Chambers et al., 2018; Tran and Mohajeri, 2021). Our previous studies found some differential microbial metabolites in the urine and plasma of MDD patients (Zheng et al., 2013; Zheng et al., 2013; Chen et al., 2015). Moreover, we found differential urinary and plasma metabolites related to the severity of MDD (Liu et al., 2015; Chen et al., 2017). Considering the close relationships between metabolites and gut microbiota in MDD, we conducted this study to explore whether the differences in gut microbiota compositions were also related with the severity of MDD.
This study was approved by the Ethical Committee of Chongqing Medical University (No. 20200320), and all the included subjects provided written informed consent. Subjects meeting the fourth Diagnostic and Statistical Manual of Mental Disorders criteria for MDD (DSM-IV) were included as MDD patients. HC were from the Medical Examination Center. In total, 131 HC and 130 MDD patients were included from our previous studies (Chen et al., 2020; Bai et al., 2021). There were 21 MDD patients receiving antidepressants (mainly citalopram, fluoxetine, paroxetine, sertraline, and venlafaxine) for 1 month prior to sample collections. In the MDD group, 78 patients with Hamilton Depression Rating Scale (HDRS) score <25 were assigned into the moderate MDD group, and the other 52 patients with HDRS score ≥25 were assigned into the severe MDD group (Kriston and von Wolff, 2011; Liu et al., 2015; Chen et al., 2017). The age, body mass index (BMI), and sex ratio were matched among the three groups. The detailed information of these subjects is found in Table 1.
The procedures for the measurement of gut microbiota compositions were identical to our previous studies (Chen et al., 2020; Bai et al., 2021). Briefly, after the raw 16S rRNA gene sequencing reads were obtained using the Illumina MiSeq PE300 platform/NovaSeq PE250 platform (Illumina, San Diego, USA), they were then demultiplexed, quality-filtered by FASTP (version 0.20.0), and merged by FLASH (version 1.2.7) with the following criteria. (i) The 300-bp reads were truncated at any site receiving an average quality score of <20 over a 50-bp sliding window, and the truncated reads shorter than 50 bp were discarded. (ii) Only overlapping sequences longer than 10 bp were assembled according to their overlapped sequence. The maximum mismatch ratio of the overlap region was 0.2. Reads that could not be assembled were discarded. (iii) Exact barcode matching, two-nucleotide mismatch in primer matching, and reads containing ambiguous characters were removed. The operational taxonomic units (OTUs) with 97% similarity cutoff were clustered using UPARSE (version 7.1), and chimeric sequences were removed. The taxonomy of each OTU representative sequence was analyzed by RDP Classifier (version 2.2) against the 16S rRNA database using a confidence threshold of 0.7. At last, we obtained the relative abundances of gut microbiota at different levels. In this study, we analyzed the abundance score for each genus in the three groups.
Firstly, the Student’s t-test, non-parametric test, chi-square test, or one-way analysis was used to check whether there were significant differences on the demographic data among the three groups (Ma et al., 2021). Secondly, the orthogonal partial least-square discriminant analysis (OPLS-DA) was used to identify the differential genera responsible for the discrimination between MDD patients and HC. Here, the default seven-round cross-validation in OPLS-DA was applied. The genus with important variables on the projection (VIP) > 1.0 (equivalent to a p-value of less than 0.05) was identified as the differential genus. Thirdly, the co-occurrence network was built using the identified differential genera to reflect the microbial changes in HC, moderate MDD patients, and severe MDD patients (Abdullaeva et al., 2021). Fourth, to identify the genera with the promise as the potential biomarkers for diagnosing MDD, the stepwise logistic-regression analysis based on Akaike’s information criterion (AIC) rule was used to analyze the shared differential genera in moderate and severe MDD patients (Ferlizza et al., 2020; Fuchs-Leitner et al., 2021). By dealing with the trade-off between simplicity and goodness of fit of the built model, the AIC rule was often applied to conduct model selection during stepwise logistic-regression analysis (Chen et al., 2020). The model with the minimum AIC value was the preferred model, and genera in this model were viewed as the potential biomarkers. Receiver operating characteristic (ROC) curve analysis was conducted to evaluate the diagnostic performance of the identified potential biomarkers (Kumstel et al., 2020; Wang et al., 2021; Fang et al., 2021). The area under the curve (AUC) was used to assess the diagnostic performance: 0.9–1, excellent; 0.8–0.9, good; 0.7–0.8, fair; 0.6–0.7, poor; and 0.5–0.6, failed. Moreover, sensitivity analysis in the logistic regression model was conducted by excluding these 21 MDD patients receiving antidepressants in 1 month prior to sample collection. Finally, the correlation between HDRS score and the abundance score of all differential genera was investigated. SPSS 19.0 and R software 3.6 were used to do all the analyses, and P-value <0.05 was viewed as significant difference.
The relative abundances of gut microbiota in MDD patients and HC are described in Figure 1A. Firmicutes, Bacteroidota, Actinobacteriota, and Proteobacteria were the main phyla in both MDD patients and HC. Here, two parameters (Shannon and Simpson) were calculated to assess α-diversity, and principal-coordinate analysis was used to assess β-diversity. There was no significant difference on α-diversity between HC and moderate MDD patients (Shannon, p = 0.32; Simpson, p = 0.16), but principal-coordinate analysis showed that there were significant differences on β-diversity between the two groups (p = 0.012). Meanwhile, non-significant differences on α-diversity (Shannon, p = 0.44; Simpson, p = 0.25) and significant differences on β-diversity (p = 0.031) were observed between HC and severe MDD patients.
Figure 1 Changes of gut microbiota compositions in HC and moderate and severe MDD patients. (A) Relative abundances of gut microbiota at the genus level in MDD patients and HC. (B) OPLS-DA model showed that there was only a small overlap between HC and moderate MDD patients, suggesting the divergent microbial changes between the two groups; (C) differential genera responsible for discriminating moderate MDD patients from HC; (D) OPLS-DA model showed that there was only a small overlap between HC and severe MDD patients, suggesting the divergent microbial changes between the two groups; (E) differential genera responsible for discriminating severe MDD patients from HC. HC, healthy controls; MDD, major depressive disorder; Fae, Faecalibacterium; Vic, Victivallis; Meg1, Megamonas; Pyr, Pyramidobacter; Hyd, Hydrogenoanaerobacterium; Pre, Prevotella; Kle, Klebsiella; Ace, Acetanaerobacterium; But, Butyricimonas; Ali, Alistipes; Cop, Coprobacillus; Ana1, Anaerococcus; Act, Actinomyces; Eub, Eubacterium; Par1, Parabacteroides; Psy, Psychrobacter; Odo, Odoribacter; Ent1, Enterococcus; Ent2, Enterorhabdus; Cor, Corynebacterium; Ana2, Anaerofustis; All, Allisonella; Por, Porphyromonas; Bar, Barnesiella; Ols, Olsenella; Dor, Dorea; Oxa, Oxalobacter; Egg, Eggerthella; Sla, Slackia; Fla, Flavonifractor; Pep, Peptoniphilus; Bla, Blautia; Ana3, Anaerotruncus; Par2, Parvimonas; Gor, Gordonibacter; Col, Collinsella; Lac1, Lactococcus; Sut, Sutterella; Tur, Turicibacter; Meg2, Megasphaera; Bil, Bilophila; Lac2, Lactobacillus; Bac, Bacteroides; Ana, Anaeroglobus.
After adjusting for age, sex, and BMI, the OPLS-DA model displayed that the HC and moderate MDD patients could be obviously separated by the microbiota genera, which suggested the divergent microbial changes between HC and moderate MDD patients (Figure 1B). By analyzing the loading plot of the model, 36 genera with VIP > 1.0 were identified as the differential genera in moderate MDD patients. Compared with HC, the abundance scores of Victivallis, Pyramidobacter, Hydrogenoanaerobacterium, Megamonas, Klebsiella, Prevotella, and Faecalibacterium were decreased, while those of Dorea, Butyricimonas, Alistipes, Parabacteroides, Blautia, Coprobacillus, Flavonifractor, Odoribacter, Actinomyces, Collinsella, Barnesiella, Eubacterium, Anaerococcus, Allisonella, Anaerofustis, Oxalobacter, Anaerotruncus, Acetanaerobacterium, Eggerthella, Peptoniphilus, Enterococcus, Gordonibacter, Porphyromonas, Parvimonas, Slackia, Psychrobacter, Corynebacterium, Olsenella, and Enterorhabdus were increased in moderate MDD patients (Figure 1C). These differential genera mainly belonged to phyla Firmicutes (n = 16, 44.44%), Actinobacteriota (n = 8, 22.22%), and Bacteroidota (n = 7, 19.44%). The detailed information of these differential genera is described in Table 2.
Similarly, after adjusting for age, sex, and BMI, using OPLS-DA (Figure 1D), we identified 27 differential genera with VIP >1.0 in severe MDD patients. Compared with HC, the abundance scores of Lactococcus, Pyramidobacter, Victivallis, Sutterella, Klebsiella, Hydrogenoanaerobacterium, Dorea, and Faecalibacterium were decreased, while those of Eggerthella, Bacteroides, Blautia, Collinsella, Odoribacter, Alistipes, Megasphaera, Flavonifractor, Butyricimonas, Parabacteroides, Bilophila, Porphyromonas, Anaerotruncus, Eubacterium, Peptoniphilus, Acetanaerobacterium, Lactobacillus, Turicibacter, and Anaeroglobus were increased in severe MDD patients (Figure 1E). These differential genera mainly belonged to phyla Firmicutes (n = 14, 51.85%) and Bacteroidota (n = 6, 22.22%). The detailed information of these differential genera is described in Table 3.
The co-occurrence networks deduced from the relative abundance of moderately or severely related genera were generated using Spearman’s correlation coefficient, which was used to reflect microbial changes in HC and moderate and severe MDD patients. As shown in Figure 2, the majority of altered genera belonging to phylum Actinobacteriota (n = 6, 75%) were specific to moderate MDD patients. The co-occurrence network showed that in moderate MDD patients, six genera from phylum Actinobacteriota and seven genera from phylum Firmicutes significantly covaried with one another, which generated two characteristic covarying networks from phyla Actinobacteriota and Firmicutes. No such specific covarying network was found in severe MDD patients. Meanwhile, among the identified differential genera, 19 (nine belonging to phylum Firmicutes and five belonging to phylum Bacteroidota) were consistently changed in MDD patients compared with HC (Figure 2). The co-occurrence network showed that there were two characteristic covarying networks from phyla Bacteroidota and Firmicutes in MDD groups.
Figure 2 Co-occurrence network showing microbial changes in moderate and severe MDD patients. The microbial genera changed in moderate or severe MDD were identified by OPLS-DA. In total, 63 differential genera were identified in the two groups. Nineteen of 63 genera were consistently altered in both moderate and severe MDD patients relative to HC, and 17 and 8 genera were specific to moderate MDD alone and severe MDD alone, respectively. Compared to HC, moderate MDD was mainly characterized by altered covarying genera assigned to phylum Firmicutes, Actinobacteriota, and Bacteroidota, while severe MDD was mainly characterized by altered covarying genera assigned to phyla Firmicutes and Bacteroidota. Lines between nodes indicate Spearman’s correlation > +0.30 (light red) or < −0.30 (light blue)); line thickness indicates p value (p < 0.05).
To identify the potential biomarkers for diagnosing MDD, we used the logistic regression analysis to further analyze the consistently changed genera in both moderate and severe MDD patients when compared with HC. After adjusting for age, sex, and BMI, the results showed that the most significant deviations between HC and MDD patients were explained by five differential genera (Collinsella, Eggerthella, Alistipes, Faecalibacterium, and Flavonifractor) (Figure 3). The ROC curve analysis was then used to evaluate the diagnostic performance of these differential genera. The results showed that the panel consisting of these five genera could yield an AUC of 0.786 for classifying MDD patients from HC (Figure 3). The sensitivity analysis by excluding the medicated MDD patients showed a similar diagnostic performance of this panel in diagnosing MDD. These results suggested that these five differential genera might hold promise as potential biomarkers for diagnosing MDD.
Figure 3 Five differential genera as potential biomarkers for diagnosing MDD. The model consisting of these five genera had the minimum AIC value; thus, they were viewed as the potential biomarkers. The panel consisting of these five genera could yield an AUC of 0.786 for classifying MDD patients from HC, suggesting fair diagnostic performance in diagnosing MDD. HC, healthy controls; MDD, major depressive disorder; AUC, area under the curve.
To find out the potential correlations between the severity of depression and gut microbiota, Pearson correlation analysis was used to analyze the correlations between HDRS score and differential genera. The genera significantly correlated with the HDRS score were used to build the correlation network (Figure 4). Six differential genera (Parvimonas, Dorea, Gordonibacter, Blautia, Actinomyces, and Enterococcus) in moderate MDD patients presented significantly positive or negative correlations with the HDRS score. Four differential genera (Klebsiella, Butyricimonas, Bilophila, and Odoribacter) in severe MDD patients presented significantly positive correlations with HDRS.
Figure 4 Differential genera in moderate and severe MDD patients significantly correlated with HDRS. Six genera (four of them belonged to phylum Firmicutes) in moderate MDD patients were significantly positively or negatively correlated with HDRS. Four genera in severe MDD patients were significantly positively correlated with HDRS. MDD, major depressive disorder; HDRS, Hamilton Depression Rating Scale.
It might be interesting to see the differential genera between moderate and severe MDD patients; thus, we directly used the data from moderate and severe MDD patients to build the OPLS-DA model. Results showed no significant difference on both α-diversity (Shannon, p = 0.51; Simpson, p = 0.47) and β-diversity (p = 0.22) between moderate and severe MDD patients. Meanwhile, after adjusting for age, sex, and BMI, the built OPLS-DA model displayed that moderate and severe MDD patients could not be clearly separated (40.38% severe MDD patients were wrongly assigned into moderate MDD patients) (Figure 5A). However, we still identified four differential genera (Catenibacterium, VIP = 1.73; Dorea, VIP = 2.16; Gordonibacter, VIP = 1.45; Megamonas, VIP = 1.58) between moderate and severe MDD patients. Compared with severe MDD patients, Catenibacterium, Dorea, and Gordonibacter were significantly higher, while Megamonas was significantly lower in the moderate MDD patients (Figure 5B).
Figure 5 Genus-level analysis of gut microbiota between moderate and severe MDD patients. (A) OPLS-DA model showed that the moderate and severe MDD patients could not be significantly separated; (B) there were four differential genera between the two groups.
This study was conducted to find the divergent microbes of different MDD severity. The results showed that there were 36 and 27 differential genera in moderate and severe MDD patients, respectively. The differential genera in moderate and severe MDD patients mainly belonged to three (Firmicutes, Actinobacteriota, and Bacteroidota) and two phyla (Firmicutes and Bacteroidota), respectively. Meanwhile, one specific covarying network from phylum Actinobacteriota was identified in moderate MDD patients. In addition, the moderate and severe MDD patients shared no differential genera that were significantly correlated with the HDRS score. Therefore, these findings suggested that although moderate and severe MDD patients shared some common differential genera, the two groups had significantly different microbial signatures.
Currently, clinicians still use the structured clinical interview rather than objective laboratory tests to diagnose MDD. However, the interview method often results in a certain percentage of misdiagnosis (Mitchell et al., 2009) due to the highly heterogeneous of clinical presentation of MDD. One promising way to markedly increase the accuracy of diagnosis is to identify disease biomarkers for objectively diagnosing MDD. In recent decades, much work has been done to identify potential biomarkers for MDD (Dmitrzak-Weglarz et al., 2021; Bai et al., 2021; Huang et al., 2022; Travica et al., 2022). However, few studies have taken the severity of MDD into consideration. In our previous studies, the differential urinary and plasma metabolites related to the severity of MDD were observed (Liu et al., 2015; Chen et al., 2017). Here, we provided an interesting method to identify potential biomarkers for MDD. A panel consisting of five consistently changed genera was found to have fair efficacies in diagnosing MDD patients from HCs.
Gut microbiota could be influenced by many factors, such as dietary habit and antibiotic agents (Farag et al., 2020; Khan et al., 2020; Dordević et al., 2021; Liu et al., 2021). Madison et al. reported that dietary habit could affect the gut microbiota compositions independently or in conjunction with stress (Madison and Kiecolt-Glaser, 2019). Lv et al. found that there was a close relationship between BMI and gut microbiota compositions in Chinese male college students (Lv et al., 2019). Duan et al. observed that the gut microbiota compositions were different in cynomolgus macaques with different ages (Duan et al., 2019). Our previous study found the differential gut microbiota compositions between young and middle-aged MDD patients (Chen et al., 2020). In the present study, our findings further suggested that the gut microbiota compositions could also be affected by the severity of MDD. These results might provide a novel clue for understanding the role of gut microbiota in the onset of depression. However, only gut microbiota at the genus level was analyzed here. Therefore, our identified potential microbial biomarkers—although very promising—were preliminary results and need further validation.
Firmicutes and Bacteroidetes are the two phyla of dominating bacteria in human gut microbiota. Zheng et al. found that the relative abundance of Bacteroidetes was significantly changed in MDD patients compared with HCs (Zheng et al., 2016). Jiang et al. reported that both the relative abundances of Firmicutes and Bacteroidetes were significantly disordered in MDD patients compared with HCs (Jiang et al., 2015). In our previous study, we found that compared with HCs, the relative abundance of Bacteroidetes was significantly increased and decreased in young and middle-aged MDD patients, respectively, and the relative abundance of Firmicutes was only found to be significantly changed in young MDD patients (Chen et al., 2020). In this study, we observed that the differential genera in moderate and severe MDD patients mainly belonged to three (Firmicutes, Actinobacteriota, and Bacteroidota) and two (Firmicutes and Bacteroidota) phyla, respectively. These results indicated that the gut microbiota compositions could be affected by many factors, and further studies on the associations between MDD and gut microbiota should minimize the influence of confounding factors.
The shared differential genus Collinsella by two MDD groups is an important intestinal bacterium to produce ursodeoxycholic acid. Ursodeoxycholic acid has antioxidant and anti-apoptotic effects and can suppress pro-inflammatory cytokines like IL-2 and TNF-α (Hirayama et al., 2021). The close relationships between MDD and inflammation have been reported in many previous studies (Leonard, 2018; Colasanto et al., 2020). Another shared differential genus Faecalibacterium is an important intestinal bacterium to produce butyric acid. Butyric acid is a major short-chain fatty acid (SCFA) produced by gut microbiota (Sun et al., 2021). SCFAs are speculated to play an important role in the cross talk between the gut and brain. Our previous study found associations between disordered hypothalamus neurotransmitters and fecal SCFAs in depressed mice (Wu et al., 2020). These results showed that the identified shared differential genus were worthy of further exploring.
Previous studies reported that the dominant taxa were different in the different phases of the life cycle (Lim et al., 2015; Vemuri et al., 2018). Our previous study found that there were age-specific differential changes on gut microbiota composition in MDD patients (Chen et al., 2020). In this study, we identified three significantly decreased and one significantly increased genus in severe MDD patients compared with moderate MDD patients. Three of them (Catenibacterium, Dorea, Megamonas) belonged to the phylum Firmicutes. These results indicated that the continuing changes of gut microbiota in moderate MDD patients, especially phylum Firmicutes, might contribute to the deterioration of depression. Therefore, developing personalized treatment methods to timely treat moderate MDD patients might be able to alleviate or delay the progress of depression.
Many studies have reported the microbial markers of depression (Jiang et al., 2015; Chen et al., 2018; Zhou et al., 2020; Yang et al., 2020). Zhou et al. found that gut microbiota-based biomarkers, such as Faecalibacterium and Butyricicoccus, might be helpful for the diagnosis and treatment of postpartum depressive disorder patients (Zhou et al., 2020). Here, Faecalibacterium was also identified as a potential biomarker for MDD. Another study reported that a combinatorial marker panel consisting of bacterial species and fecal metabolite markers could effectively discriminate MDD from HC (Yang et al., 2020). Our previous study found that the suitability of Actinobacteria and Bacteroidia as the sex-specific biomarkers for diagnosing MDD was worthy of further exploring (Chen et al., 2018). Jiang et al. observed that Alistipes and Faecalibacterium might be potential biomarkers for MDD patients (Jiang et al., 2015). Here, Collinsella and Eggerthella belonged to phylum Actinobacteriota and Alistipes belonged to phylum Bacteroidota were identified as potential biomarkers for MDD. Although these results showed a potential and novel method for objective diagnosis of depression, further studies were warranted to evaluate the suitability of gut microbiota as a biomarker for depression.
Several limitations should be mentioned here. Firstly, the number of subjects in each group was relatively small, which requires future studies to validate and support the conclusions. Secondly, although the potential effects of main confounding factors (age, BMI, sex ratio) were eliminated, the effects of other potential factors, such as family history of psychiatric diseases, host genetics, smoking, and dietary habit, were not explored here; thus, future studies were needed to assess the effects of these factors. Thirdly, all the included subjects came from the same place, and thus there might be ethno-specific biases, which could limit the applicability of our conclusion. Fourthly, due to technical reasons, the identification of gut microbiota at the species level was unsuccessful. Therefore, it might also be meaningful to further investigate the differential gut microbiota compositions at the species level. Fifthly, we did not analyze the functions of differential gut microbiota related to the severity of MDD, which was worthy of further exploring using whole-genome sequencing (WGS) or phylogenetic investigation of communities by reconstruction of unobserved states (PICRUST). Sixthly, the “healthy human microbiota” is only a theoretical phenomenon. Due to the complexity of assessing the health status of gut microbiota, the “healthy human microbiota” has not yet been defined. Thus, the microbial biomarkers should be cautiously interpreted. Seventhly, although the sensitivity analysis showed that the results obtained by excluding these 21 MDD patients were similar to the original results, we did not know whether 1 month was enough to remove the effects of antidepressive treatments on gut microbiota; thus, future studies should recruited drug-naïve MDD patients to evaluate our results.
In conclusion, this study found that there were divergent microbial phenotypes between moderate and severe MDD patients. Totally, 36 and 27 differential genera in moderate and severe MDD patients, respectively, were identified. One specific covarying network from phylum Actinobacteriota was identified in moderate MDD patients. In addition, five differential genera (Collinsella, Eggerthella, Alistipes, Faecalibacterium, and Flavonifractor) held promise as the potential biomarkers for diagnosing MDD. Our results may also be helpful for further exploring the role of gut microbiota in the pathogenesis of depression.
The datasets presented in this study can be found in online repositories. The names of the repository/repositories and accession number(s) can be found below: https://www.ncbi.nlm.nih.gov/, PRJNA806486.
The studies involving human participants were reviewed and approved by Ethical Committee of Chongqing Medical University. The patients/participants provided their written informed consent to participate in this study.
QZ, J-JC, and PX conceived and designed the study; QZ, YW, and W-HS participated in data collection. J-JC and C-JZ analyzed the data. QZ, J-JC, and PX prepared the paper. All authors have read and approved the final manuscript.
This work was supported by the National Key Research and Development Program of China (2017YFA0505700), the Non-profit Central Research Institute Fund of Chinese Academy of Medical Sciences (2019PT320002), the Natural Science Foundation Project of China (81820108015, 81701360), the Natural Science Foundation of Chongqing (cstc2021jcyj-msxmX0084), the Science and Technology Research Program of Chongqing Municipal Education Commission (Grant No. KJQN202100420), and the Chongqing Yuzhong District Science & Technology Commission (20190115).
The authors declare that the research was conducted in the absence of any commercial or financial relationships that could be construed as a potential conflict of interest.
All claims expressed in this article are solely those of the authors and do not necessarily represent those of their affiliated organizations, or those of the publisher, the editors and the reviewers. Any product that may be evaluated in this article, or claim that may be made by its manufacturer, is not guaranteed or endorsed by the publisher.
Abdullaeva, Y., Ambika Manirajan, B., Honermeier, B., Schnell, S., Cardinale, M. (2021). Domestication Affects the Composition, Diversity, and Co-Occurrence of the Cereal Seed Microbiota. J. Adv. Res. 31, 75–86. doi: 10.1016/j.jare.2020.12.008
Al-Harbi, K. S. (2012). Treatment-Resistant Depression: Therapeutic Trends, Challenges, and Future Directions. Patient Prefer. Adherence 6, 369–388. doi: 10.2147/PPA.S29716
Bai, S., Xie, J., Bai, H., Tian, T., Zou, T., Chen, J. J. (2021). Gut Microbiota-Derived Inflammation-Related Serum Metabolites as Potential Biomarkers for Major Depressive Disorder. J. Inflamm. Res. 14, 3755–3766. doi: 10.2147/JIR.S324922
Campbell, S., Macqueen, G. (2004). The Role of the Hippocampus in the Pathophysiology of Major Depression. J. Psychiatry Neurosci. 29 (6), 417–426.
Chambers, E. S., Preston, T., Frost, G., Morrison, D. J. (2018). Role of Gut Microbiota-Generated Short-Chain Fatty Acids in Metabolic and Cardiovascular Health. Curr. Nutr. Rep. 7 (4), 198–206. doi: 10.1007/s13668-018-0248-8
Chen, J. J., He, S., Fang, L., Wang, B., Bai, S. J., Xie, J., et al (2020). Age-Specific Differential Changes on Gut Microbiota Composition in Patients With Major Depressive Disorder. Aging (Albany NY) 12 (3), 2764–2776. doi: 10.18632/aging.102775
Chen, T., Wang, R., Duan, Z., Yuan, X., Ding, Y., Feng, Z., et al (2021). Akkermansia Muciniphila Protects Against Psychological Disorder-Induced Gut Microbiota-Mediated Colonic Mucosal Barrier Damage and Aggravation of Colitis. Front. Cell Infect. Microbiol. 11, 723856. doi: 10.3389/fcimb.2021.723856
Chen, J. J., Zheng, P., Liu, Y. Y., Zhong, X. G., Wang, H. Y., Guo, Y. J., et al (2018). Sex Differences in Gut Microbiota in Patients With Major Depressive Disorder. Neuropsychiatr. Dis. Treat. 14, 647–655. doi: 10.2147/NDT.S159322
Chen, J. J., Zhou, C. J., Liu, Z., Fu, Y. Y., Zheng, P., Yang, D. Y., et al (2015). Divergent Urinary Metabolic Phenotypes Between Major Depressive Disorder and Bipolar Disorder Identified by a Combined GC-MS and NMR Spectroscopic Metabonomic Approach. J. Proteome Res. 14 (8), 3382–3389. doi: 10.1021/acs.jproteome.5b00434
Chen, J. J., Zhou, C. J., Zheng, P., Cheng, K., Wang, H. Y., Li, J., et al (2017). Differential Urinary Metabolites Related With the Severity of Major Depressive Disorder. Behav. Brain Res. 332, 280–287. doi: 10.1016/j.bbr.2017.06.012
Coello, K., Hansen, T. H., Sørensen, N., Munkholm, K., Kessing, L. V., Pedersen, O., et al (2019). Gut Microbiota Composition in Patients With Newly Diagnosed Bipolar Disorder and Their Unaffected First-Degree Relatives. Brain Behav. Immun. 75, 112–118. doi: 10.1016/j.bbi.2018.09.026
Coello, K., Hansen, T. H., Sørensen, N., Ottesen, N. M., Miskowiak, K. W., Pedersen, O., et al (2021). Affective Disorders Impact Prevalence of Flavonifractor and Abundance of Christensenellaceae in Gut Microbiota. Prog. Neuropsychopharmacol. Biol. Psychiatry 110, 110300. doi: 10.1016/j.pnpbp.2021.110300
Colasanto, M., Madigan, S., Korczak, D. J. (2020). Depression and Inflammation Among Children and Adolescents: A Meta-Analysis. J. Affect. Disord. 277, 940–948. doi: 10.1016/j.jad.2020.09.025
Ding, H., Yi, X., Zhang, X., Wang, H., Liu, H., Mou, W. W. (2021). Imbalance in the Gut Microbiota of Children With Autism Spectrum Disorders. Front. Cell Infect. Microbiol. 11, 572752. doi: 10.3389/fcimb.2021.572752
Dmitrzak-Weglarz, M., Szczepankiewicz, A., Rybakowski, J., Kapelski, P., Bilska, K., Skibinska, M., et al (2021). Expression Biomarkers of Pharmacological Treatment Outcomes in Women With Unipolar and Bipolar Depression. Pharmacopsychiatry 54 (6), 261–268. doi: 10.1055/a-1546-9483
Dong, R., Lin, H., Chen, X., Shi, R., Yuan, S., Li, J., et al (2021). Gut Microbiota and Fecal Metabolites Associated With Neurocognitive Impairment in HIV-Infected Population. Front. Cell Infect. Microbiol. 11, 723840. doi: 10.3389/fcimb.2021.723840
Dordević, D., Jančíková, S., Vítězová, M., Kushkevych, I. (2021). Hydrogen Sulfide Toxicity in the Gut Environment: Meta-Analysis of Sulfate-Reducing and Lactic Acid Bacteria in Inflammatory Processes. J. Adv. Res. 27, 55–69. doi: 10.1016/j.jare.2020.03.003
Duan, J., Yin, B., Li, W., Chai, T., Liang, W., Huang, Y., et al (2019). Age-Related Changes in Microbial Composition and Function in Cynomolgus Macaques. Aging (Albany NY) 11 (24), 12080–12096. doi: 10.18632/aging.102541
Fang, F., Li, Z., Yu, J., Long, Y., Zhao, Q., Ding, X., et al (2021). MicroRNAs Secreted by Human Embryos Could be Potential Biomarkers for Clinical Outcomes of Assisted Reproductive Technology. J. Adv. Res. 31, 25–34. doi: 10.1016/j.jare.2021.01.003
Farag, M. A., Abdelwareth, A., Sallam, I. E., El Shorbagi, M., Jehmlich, N., Fritz-Wallace, K., et al (2020). Metabolomics Reveals Impact of Seven Functional Foods on Metabolic Pathways in a Gut Microbiota Model. J. Adv. Res. 23, 47–59. doi: 10.1016/j.jare.2020.01.001
Ferlizza, E., Solmi, R., Miglio, R., Nardi, E., Mattei, G., Sgarzi, M., et al (2020). Colorectal Cancer Screening: Assessment of CEACAM6, LGALS4, TSPAN8 and COL1A2 as Blood Markers in Faecal Immunochemical Test Negative Subjects. J. Adv. Res. 24, 99–107. doi: 10.1016/j.jare.2020.03.001
Fuchs-Leitner, I., Yazdi, K., Gerstgrasser, N. W., Tholen, M. G., Graffius, S. T., Schorb, A., et al (2021). Risk of PTSD Due to the COVID-19 Pandemic Among Patients in Opioid Substitution Treatment. Front. Psychiatry 12, 729460. doi: 10.3389/fpsyt.2021.729460
Han, D., Gao, P., Li, R., Tan, P., Xie, J., Zhang, R., et al (2020). Multicenter Assessment of Microbial Community Profiling Using 16S rRNA Gene Sequencing and Shotgun Metagenomic Sequencing. J. Adv. Res. 26, 111–121. doi: 10.1016/j.jare.2020.07.010
Hirayama, M., Nishiwaki, H., Hamaguchi, T., Ito, M., Ueyama, J., Maeda, T., et al (2021). Intestinal Collinsella may Mitigate Infection and Exacerbation of COVID-19 by Producing Ursodeoxycholate. PLoS One 16 (11), e0260451. doi: 10.1371/journal.pone.0260451
Huang, X., Yin, H., Wan, X. X., Fu, B., Tang, B., Lei, J. (2022). Maternal Plasma Serotonin Level Not Suitable as Postpartum Depression Diagnostic Biomarker: Results From a Prospective Cohort Study. J. Affect. Disord. 298 (Pt A), 284–291. doi: 10.1016/j.jad.2021.11.001
Jiang, H., Ling, Z., Zhang, Y., Mao, H., Ma, Z., Yin, Y., et al (2015). Altered Fecal Microbiota Composition in Patients With Major Depressive Disorder. Brain Behav. Immun. 48, 186–194. doi: 10.1016/j.bbi.2015.03.016
Khan, I., Pathan, S., Li, X. A., Leong, W. K., Liao, W. L., Wong, V., et al (2020). Far Infrared Radiation Induces Changes in Gut Microbiota and Activates GPCRs in Mice. J. Adv. Res. 22, 145–152. doi: 10.1016/j.jare.2019.12.003
Khoshkam, Z., Aftabi, Y., Stenvinkel, P., Paige Lawrence, B., Rezaei, M. H., Ichihara, G., et al (2021). Recovery Scenario and Immunity in COVID-19 Disease: A New Strategy to Predict the Potential of Reinfection. J. Adv. Res. 31, 49–60. doi: 10.1016/j.jare.2020.12.013
Kovács, Z., Glover, L., Reidy, F., MacSharry, J., Saldova, R. (2021). Novel Diagnostic Options for Endometriosis-Based on the Glycome and Microbiome. J. Adv. Res. 33, 167–118. doi: 10.1016/j.jare.2021.01.015
Kriston, L., von Wolff, A. (2011). Not as Golden as Standards Should be: Interpretation of the Hamilton Rating Scale for Depression. J. Affect. Disord. 128 (1-2), 175–177. doi: 10.1016/j.jad.2010.07.011
Kumstel, S., Vasudevan, P., Palme, R., Zhang, X., Wendt, E. H. U., David, R., et al (2020). Benefits of non-Invasive Methods Compared to Telemetry for Distress Analysis in a Murine Model of Pancreatic Cancer. J. Adv. Res. 21, 35–47. doi: 10.1016/j.jare.2019.09.002
Leonard, B. E. (2018). Inflammation and Depression: A Causal or Coincidental Link to the Pathophysiology? Acta Neuropsychiatr. 30 (1), 1–16. doi: 10.1017/neu.2016.69
Lim, E. S., Zhou, Y., Zhao, G., Bauer, I. K., Droit, L., Ndao, I. M., et al (2015). Early Life Dynamics of the Human Gut Virome and Bacterial Microbiome in Infants. Nat. Med. 21 (10), 1228–1234. doi: 10.1038/nm.3950
Liu, X., Liu, S., Tang, Y., Pu, Z., Xiao, H., Gao, J., et al (2021). Intragastric Administration of Casein Leads to Nigrostriatal Disease Progressed Accompanied With Persistent Nigrostriatal-Intestinal Inflammation Activited and Intestinal Microbiota-Metabolic Disorders Induced in MPTP Mouse Model of Parkinson's Disease. Neurochem. Res. 46 (6), 1514–1539. doi: 10.1007/s11064-021-03293-2
Liu, L., Wang, H., Rao, X., Yu, Y., Li, W., Zheng, P., et al (2021). Comprehensive Analysis of the Lysine Acetylome and Succinylome in the Hippocampus of Gut Microbiota-Dysbiosis Mice. J. Adv. Res. 30, 27–38. doi: 10.1016/j.jare.2020.12.002
Liu, X., Zheng, P., Zhao, X., Zhang, Y., Hu, C., Li, J., et al (2015). Discovery and Validation of Plasma Biomarkers for Major Depressive Disorder Classification Based on Liquid Chromatography-Mass Spectrometry. J. Proteome Res. 14 (5), 2322–2330. doi: 10.1021/acs.jproteome.5b00144
Lu, L., Tang, M., Li, J., Xie, Y., Li, Y., Xie, J., et al (2021). Gut Microbiota and Serum Metabolic Signatures of High-Fat-Induced Bone Loss in Mice. Front. Cell Infect. Microbiol. 11, 788576. doi: 10.3389/fcimb.2021.788576
Lv, Y., Qin, X., Jia, H., Chen, S., Sun, W., Wang, X. (2019). The Association Between Gut Microbiota Composition and BMI in Chinese Male College Students, as Analysed by Next-Generation Sequencing. Br. J. Nutr. 122 (9), 986–995. doi: 10.1017/S0007114519001909
Madison, A., Kiecolt-Glaser, J. K. (2019). Stress, Depression, Diet, and the Gut Microbiota: Human-Bacteria Interactions at the Core of Psychoneuroimmunology and Nutrition. Curr. Opin. Behav. Sci. 28, 105–110. doi: 10.1016/j.cobeha.2019.01.011
Ma, K., Liu, S., Liang, H., Wang, G., Wang, T., Luo, S., et al (2021). Ca2+-Activated Cl- Channel TMEM16A Inhibition by Cholesterol Promotes Angiogenesis in Endothelial Cells. J. Adv. Res. 29, 23–32. doi: 10.1016/j.jare.2020.09.003
Martins-de-Souza, D. (2014). Proteomics, Metabolomics, and Protein Interactomics in the Characterization of the Molecular Features of Major Depressive Disorder. Dialogues Clin. Neurosci. 16 (1), 63–73. doi: 10.31887/DCNS.2014.16.1/dmartins
Mitchell, A. J., Vaze, A., Rao, S. (2009). Clinical Diagnosis of Depression in Primary Care: A Meta-Analysis. Lancet 374 (9690), 609–619. doi: 10.1016/S0140-6736(09)60879-5
Ongür, D., Drevets, W. C., Price, J. L. (1998). Glial Reduction in the Subgenual Prefrontal Cortex in Mood Disorders. Proc. Natl. Acad. Sci. U. S. A. 95 (22), 13290–13295. doi: 10.1073/pnas.95.22.13290
Pariante, C. M., Lightman, S. L. (2008). The HPA Axis in Major Depression: Classical Theories and New Developments. Trends Neurosci. 31 (9), 464–468. doi: 10.1016/j.tins.2008.06.006
Qiao, C. M., Sun, M. F., Jia, X. B., Li, Y., Zhang, B. P., Zhao, L. P., et al (2020). Sodium Butyrate Exacerbates Parkinson's Disease by Aggravating Neuroinflammation and Colonic Inflammation in MPTP-Induced Mice Model. Neurochem. Res. 45 (9), 2128–2142. doi: 10.1007/s11064-020-03074-3
Rajput, C., Sarkar, A., Sachan, N., Rawat, N., Singh, M. P. (2021). Is Gut Dysbiosis an Epicenter of Parkinson's Disease? Neurochem. Res. 46 (3), 425–438. doi: 10.1007/s11064-020-03187-9
Rana, T., Behl, T., Sehgal, A., Srivastava, P., Bungau, S. (2021). Unfolding the Role of BDNF as a Biomarker for Treatment of Depression. J. Mol. Neurosci. 71 (10), 2008–2021. doi: 10.1007/s12031-020-01754-x
Sun, X., Wang, D., Wei, L., Ding, L., Guo, Y., Wang, Z., et al (2021). Gut Microbiota and SCFAs Play Key Roles in QingFei Yin Recipe Anti-Streptococcal Pneumonia Effects. Front. Cell Infect. Microbiol. 11, 791466. doi: 10.3389/fcimb.2021.791466
Tian, T., Mao, Q., Xie, J., Wang, Y., Shao, W.-h., Zhong, Q, et al. (2022). Multi-Omics Data Reveals the Disturbance of Glycerophospholipid Metabolism Caused by Disordered Gut Microbiota in Depressed Mice. J. Adv. Res 39, 135–145.. doi: 10.1016/j.jare.2021.10.002
Tian, X. Y., Xing, J. W., Zheng, Q. Q., Gao, P. F. (2021). 919 Syrup Alleviates Postpartum Depression by Modulating the Structure and Metabolism of Gut Microbes and Affecting the Function of the Hippocampal GABA/Glutamate System. Front. Cell Infect. Microbiol. 11, 694443. doi: 10.3389/fcimb.2021.694443
Tran, S. M., Mohajeri, M. H. (2021). The Role of Gut Bacterial Metabolites in Brain Development, Aging and Disease. Nutrients 13 (3), 732. doi: 10.3390/nu13030732
Travica, N., Berk, M., Marx, W. (2022). Neurofilament Light Protein as a Biomarker in Depression and Cognitive Function. Curr. Opin. Psychiatry 35 (1), 30–37. doi: 10.1097/YCO.0000000000000756
Vemuri, R., Gundamaraju, R., Shastri, M. D., Shukla, S. D., Kalpurath, K., Ball, M., et al (2018). Gut Microbial Changes, Interactions, and Their Implications on Human Lifecycle: An Ageing Perspective. BioMed. Res. Int. 2018, 4178607. doi: 10.1155/2018/4178607
Wang, W., Sun, P., Han, F., Wang, C., Wang, Y., Wang, X., et al (2021). Transcriptome Sequencing Identifies Potential Biomarker for White Matter Lesions Diagnosis in the Hypertension Population. Neurochem. Res. 46 (8), 2079–2088. doi: 10.1007/s11064-021-03346-6
Wu, M., Tian, T., Mao, Q., Zou, T., Zhou, C. J., Xie, J., et al (2020). Associations Between Disordered Gut Microbiota and Changes of Neurotransmitters and Short-Chain Fatty Acids in Depressed Mice. Transl. Psychiatry 10 (1), 350. doi: 10.1038/s41398-020-01038-3
Xie, J., Cho, H., Lin, B. M., Pillai, M., Heimisdottir, L. H., Bandyopadhyay, D., et al (2021). Improved Metabolite Prediction Using Microbiome Data-Based Elastic Net Models. Front. Cell Infect. Microbiol. 11, 734416. doi: 10.3389/fcimb.2021.734416
Yang, J., Zheng, P., Li, Y., Wu, J., Tan, X., Zhou, J., et al (2020). Landscapes of Bacterial and Metabolic Signatures and Their Interaction in Major Depressive Disorders. Sci. Adv. 6 (49), eaba8555. doi: 10.1126/sciadv.aba8555
Zheng, P., Gao, H. C., Qi, Z. G., Jia, J. M., Li, F. F., Chen, J. J., et al (2013). Peripheral Metabolic Abnormalities of Lipids and Amino Acids Implicated in Increased Risk of Suicidal Behavior in Major Depressive Disorder. Metabolomics 9 (3), 688–696. doi: 10.1007/s11306-012-0474-9
Zheng, P., Wang, Y., Chen, L., Yang, D., Meng, H., Zhou, D., et al (2013). Identification and Validation of Urinary Metabolite Biomarkers for Major Depressive Disorder. Mol. Cell Proteomics 12 (1), 207–214. doi: 10.1074/mcp.M112.021816
Zheng, P., Yang, J., Li, Y., Wu, J., Liang, W., Yin, B., et al (2020). Gut Microbial Signatures Can Discriminate Unipolar From Bipolar Depression. Adv. Sci. (Weinh.) 7 (7), 1902862. doi: 10.1002/advs.201902862
Zheng, P., Zeng, B., Liu, M., Chen, J., Pan, J., Han, Y., et al (2019). The Gut Microbiome From Patients With Schizophrenia Modulates the Glutamate-Glutamine-GABA Cycle and Schizophrenia-Relevant Behaviors in Mice. Sci. Adv. 5 (2), eaau8317. doi: 10.1126/sciadv.aau8317
Zheng, P., Zeng, B., Zhou, C., Liu, M., Fang, Z., Xu, X., et al (2016). Gut Microbiome Remodeling Induces Depressive-Like Behaviors Through a Pathway Mediated by the Host's Metabolism. Mol. Psychiatry 21 (6), 786–796. doi: 10.1038/mp.2016.44
Zhong, Z., Chen, W., Gao, H., Che, N., Xu, M., Yang, L., et al (2021). Fecal Microbiota Transplantation Exerts a Protective Role in MPTP-Induced Parkinson's Disease via the TLR4/PI3K/AKT/NF-κb Pathway Stimulated by α-Synuclein. Neurochem. Res. 46 (11), 3050–3058. doi: 10.1007/s11064-021-03411-0
Zhou, Y., Chen, C., Yu, H., Yang, Z. (2020). Fecal Microbiota Changes in Patients With Postpartum Depressive Disorder. Front. Cell Infect. Microbiol. 10, 567268. doi: 10.3389/fcimb.2020.567268
Keywords: major depressive disorder, gut microbiota, Firmicutes, Actinobacteriota, Bacteroidota
Citation: Zhong Q, Chen J-j, Wang Y, Shao W-h, Zhou C-j and Xie P (2022) Differential Gut Microbiota Compositions Related With the Severity of Major Depressive Disorder. Front. Cell. Infect. Microbiol. 12:907239. doi: 10.3389/fcimb.2022.907239
Received: 06 April 2022; Accepted: 17 June 2022;
Published: 11 July 2022.
Edited by:
Zongxin Ling, Zhejiang University, ChinaReviewed by:
Attayeb Mohsen, National Institutes of Biomedical Innovation, Health and Nutrition, JapanCopyright © 2022 Zhong, Chen, Wang, Shao, Zhou and Xie. This is an open-access article distributed under the terms of the Creative Commons Attribution License (CC BY). The use, distribution or reproduction in other forums is permitted, provided the original author(s) and the copyright owner(s) are credited and that the original publication in this journal is cited, in accordance with accepted academic practice. No use, distribution or reproduction is permitted which does not comply with these terms.
*Correspondence: Peng Xie, eGllcGVuZ0BjcW11LmVkdS5jbg==
†These authors have contributed equally to this work
Disclaimer: All claims expressed in this article are solely those of the authors and do not necessarily represent those of their affiliated organizations, or those of the publisher, the editors and the reviewers. Any product that may be evaluated in this article or claim that may be made by its manufacturer is not guaranteed or endorsed by the publisher.
Research integrity at Frontiers
Learn more about the work of our research integrity team to safeguard the quality of each article we publish.