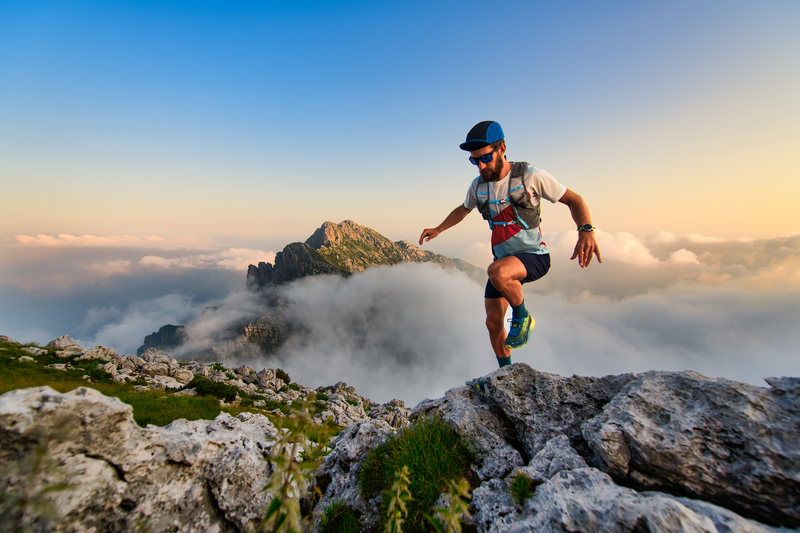
94% of researchers rate our articles as excellent or good
Learn more about the work of our research integrity team to safeguard the quality of each article we publish.
Find out more
ORIGINAL RESEARCH article
Front. Cell. Infect. Microbiol. , 02 May 2022
Sec. Microbiome in Health and Disease
Volume 12 - 2022 | https://doi.org/10.3389/fcimb.2022.885093
This article is part of the Research Topic The role of microorganisms in the development and progression of cancer View all 10 articles
As the leading cause of cancer death, lung cancer seriously endangers human health and quality of life. Although many studies have reported the intestinal microbial composition of lung cancer, little is known about the interplay between intestinal microbiome and metabolites and how they affect the development of lung cancer. Herein, we combined 16S ribosomal RNA (rRNA) gene sequencing and liquid chromatography-mass spectrometry (LC-MS) technology to analyze intestinal microbiota composition and serum metabolism profile in a cohort of 30 lung cancer patients with different stages and 15 healthy individuals. Compared with healthy people, we found that the structure of intestinal microbiota in lung cancer patients had changed significantly (Adonis, p = 0.021). In order to determine how intestinal flora affects the occurrence and development of lung cancer, the Spearman rank correlation test was used to find the connection between differential microorganisms and differential metabolites. It was found that as thez disease progressed, L-valine decreased. Correspondingly, the abundance of Lachnospiraceae_UCG-006, the genus with the strongest association with L-valine, also decreased in lung cancer groups. Correlation analysis showed that the gut microbiome and serum metabolic profile had a strong synergy, and Lachnospiraceae_UCG-006 was closely related to L-valine. In summary, this study described the characteristics of intestinal flora and serum metabolic profiles of lung cancer patients with different stages. It revealed that lung cancer may be the result of the mutual regulation of L-valine and Lachnospiraceae_UCG-006 through the aminoacyl-tRNA biosynthesis pathway, and proposed that L-valine may be a potential marker for the diagnosis of lung cancer.
Lung cancer has the characteristics of high morbidity and high mortality, which seriously endangers human health and quality of life. As the leading cause of cancer death, there would be an estimated 2.2 million new cases and 1.79 million deaths worldwide in 2020 (Sung et al., 2021). Many early-stage lung cancer patients have no symptoms or only have mild symptoms with no specificity, making it difficult to attract attention. However, the diagnosis of lung cancer requires complicated procedures such as pathological biopsy and imaging examinations, and there is a lack of simple bedside detection methods. Therefore, many lung cancer patients are often in the middle or advanced stages when they are diagnosed, so there are always different degrees of cancer metastasis, leading to a poor prognosis (Rudin et al., 2021). The current non-invasive diagnostic method for lung cancer is mainly liquid biopsy. There is a small amount of circulating tumor cells (CTC) shed from the tumor site in the blood of cancer patients, and necrotic cancer cells release a small amount of circulating tumor DNA (ctDNA) into the blood; therefore, it can help to judge the occurrence of cancer by detecting CTC and ctDNA. In addition, non-invasive biomarkers based on protein and microRNA have also been widely studied (I and Cho, 2015; Farooq and Herman, 2020). In recent years, a growing number of studies have discovered the connection between intestinal flora and disease diagnosis, treatment, and prognosis. At present, many studies involve the sequencing of gut microbes in lung cancer patients, and Zheng et al. (2020) have developed an operational taxonomic unit (OTU)-based prediction model for the early diagnosis of lung cancer. However, relying on single omics for prediction does not seem to be sufficient, and so far, the connection and interaction between the gut microbiome and metabolome of lung cancer patients with different stages have not been recorded.
A cohort study found that the diversity of intestinal flora was at a similar level in healthy children and adults, but the composition and function of the microbiome were different (Wen et al., 2019). Herein, we assume that there are differences in the composition or diversity of the intestinal flora of lung cancer patients with different stages. We recruited 30 different-stage lung cancer patients and 15 healthy individuals and performed the corresponding detection and analysis on their stool and serum specimens. The composition of the intestinal flora and serum metabolites was compared by bioinformatics analysis. We are trying to combine microbiology and metabolomics to find out the pathogenesis of lung cancer and potential biomarkers, so as to provide new insights for the diagnosis and treatment of lung cancer in the future.
Thirty newly diagnosed lung cancer patients from Hunan Cancer Hospital and 15 individuals undergoing physical examination from the Health Management Center of the Third Xiangya Hospital of Central South University were included in our study. Stool and serum samples were collected according to the protocol approved by the Ethics Committee of the Third Xiangya Hospital of Central South University, and written informed consent from all participants was obtained. The exclusion criteria were as follows: 1) individuals with primary carcinoma of other organs, 2) individuals suffering from other cancers, and 3) individuals receiving antibiotics or probiotics within the past 3 months. The control group was matched according to age and sex ratio.
Fresh stool samples of each participant were collected and then placed on ice immediately. The temperature was ensured to be below 4°C, and the samples collected were stored at −80°C within 1 h until DNA extraction. Intravenous blood collection was carried out by professional nurses in strict accordance with aseptic standard procedures. Serum was collected by centrifugation and stored at −80°C until being tested.
QIAamp 96 Power Fecal QIAcube HT kit (Qiagen, Hilden, Germany) was used to extract total DNA from stool samples according to the manufacturer’s instructions. NanoDrop2000 (Thermo Fisher, USA) and 1% agarose gel electrophoresis were used to detect the concentration and quality of DNA. Barcode-specific primers (primers 5′-TACGGRAGGCAGCAG-3′ and 5′-AGGGTATCTAATCCT-3′) were used to perform PCR amplification on the 16S V3–V4 region.
The PCR products were separated on a 2% agarose gel and further purified using AxyPrep DNA Gel Extraction Kit (Axygen Biosciences, Union City, CA, USA). The purified PCR products were quantified using Qubit dsDNA Assay Kit (Life Technologies, Waltham, MA, USA). Finally, equal amounts of samples were mixed according to the concentration of PCR products, and NovaSeq PE250 paired-end sequencing was performed.
The Trimmomatic software (Bolger et al., 2014) was used to remove impurity from the original double-ended sequence (FASTQ format), and the FLASH software (Reyon et al., 2012) was used to merge after impurity removal. Then, sequences containing ambiguous bases, single-base homologous regions, and chimeras were removed to achieve accurate impurity removal to ensure the accuracy of the results. After sequencing data that were being preprocessed to generate high-quality sequences, the OTU with sequence similarity ≥97% was defined as a taxon by VSEARCH software (Caporaso et al., 2010). All representative reads were annotated and blasted against the SILVA database (version 132) using RDP classifier v2.11 (confidence threshold of 70%) (Wang et al., 2007). The microbial diversity was estimated using alpha diversity that included the Chao1 index (Chao and Bunge, 2002), Shannon index (Hill et al., 2003), and Simpson index. The UniFrac distance matrix performed by the QIIME software was used for weighted UniFrac principal coordinates analysis (PCoA).
Metabolites were extracted after sample pretreatment. The LC-MS system composed of a Dionex U3000 UHPLC ultra-high performance liquid chromatograph (Thermo Fisher Scientific, USA) and a QE PLUS high-resolution mass spectrometer (Thermo Fisher Scientific, USA) was used as the analytical instrument in this experiment. The operating conditions of the instrument were set as follows: chromatographic conditions—chromatographic column, ACQUITY UPLC HSS T3 (100 mm × 2.1 mm, 1.8 μm); column temperature, 45°C mobile phase, 0.1% formic acid–water (A) and acetonitrile (B); flow rate, 0.35 ml/min; injection volume, 2 μl; and mass spectrum condition—ion source, ESI. The sample mass spectrum signal acquisition adopted the positive and negative ion scanning modes, respectively. After obtaining the original data, Progenesis QI v2.3 software was used to perform standardized preprocessing and qualitative and relative quantitative analyses.
Statistical analysis was performed using GraphPad Prism software (San Diego, CA, USA), QIIME, and R package (V.2.15.3). Student’s t-test and Fisher’s exact test were used to compare sample baseline data. Adonis analysis, Wilcoxon rank-sum test, and Kruskal–Wallis test were used to compare the differences between microbial groups. The metabolomics data were processed and analyzed using the Progenesis QI v2.3 software. Student’s t-test and fold change analysis were used to compare metabolites between groups. Pearson correlation coefficient was used to measure the degree of linear correlation between two metabolites. The Spearman rank correlation test was used to assess the correlation between microorganisms and metabolites. p <0.05 was considered as statistically significant.
This study included 30 lung cancer patients and 15 healthy individuals. The average age of the early-stage lung cancer (ESLC) group, non-early-stage lung cancer (NESLC) group, and healthy control (HC) group was 61.25 ± 6.09, 57.82 ± 9.1, and 59.6 ± 5.45 years old, respectively. No significant difference was found. Similarly, the gender distribution of the three groups has also been verified (Fisher’s exact test, p = 0.923). Adenocarcinoma was the main type of lung cancer (6/8 in ESLC, 12/22 in NESLC) (Table 1).
In order to explore the intestinal microbial composition of lung cancer patients with different stages, we performed 16S rRNA sequencing on stool samples of 30 lung cancer patients (8 of ESLC, 22 of NSLC) and 15 healthy individuals. The VSEARCH (version 2.4.2) software (Rognes et al., 2016) was used to classify OTU according to 97% of similarity. The sequence with the largest abundance in each OTU was selected as the representative sequence of the OTU, compared, and annotated with the SILVA (v132) database using the RDP classifier (v2.11) as the annotation tool. A total of 6,053 annotated OTUs were obtained. Compared with HC, the total OTUs and unique OTUs of ESLC and NESLC increased, and there were 2,378 annotation OTUs shared by the three groups (Figure 1A).
Figure 1 Intestinal flora community structure. (A) The Venn diagram showed each group of unique and common OTUs. (B–D) The top 15 representative species and their proportions in the three groups at the level of phylum, species, and genus. (E) PCoA shows differences between individuals or groups. The abscissa (PC1) and the ordinate (PC2) are the two main coordinates that explain the greatest difference between samples. The points in the graph represent samples, and different colors represent different sample grouping information; similar samples are clustered together.
We analyzed the community structure of intestinal microbial (Supplemental Material 1). At the phylum level, Bacteroides, Firmicutes, and Proteobacteria were the main components in the three groups. At the family level, besides similar families with higher abundance (Bacteroidaceae, Lachnospiraceae, Ruminococcaceae), the abundance of Enterobacteriaceae was higher in ESLC (15.01%), Enterobacteriaceae and Prevotellaceae in NESLC (10.55%, 9.71%), and Prevotellaceae in HC (14.87%). At the genus level, apart from the similar higher abundance genus (Bacteroides), Faecalibacterium (10.71%), Prevotella_9 (12.56%), and Bacteroides (7.33%) were the most abundant genera identified in HC; Faecalibacterium (8.25%), Klebsiella (7.76%), and Escherichia–Shigella (6.77%) in ESLC; and Prevotella_9 (7.77%) and Escherichia–Shigella (7.23%) in NESLC (Figures 1B–D).
The microbial abundance was statistically analyzed (Supplemental Material 2). The Chao1 index showed that there was no significant difference in community richness among groups, while the Shannon and Simpson indexes both showed that each group had similar community diversity. When comparing the structure of the microbial community, β diversity showed differences among the groups (Figure 1E, Adonis, p = 0.021).
The Kruskal–Wallis algorithm was used to further identify microbes with different abundances, and 3 phyla, 21 families, and 55 genera were identified (Supplemental Material 3). At the genus level, Escherichia–Shigella, Anaerotruncus, Ruminiclostridium, Lactobacillus, Pediococcus, Sphingobium, Prevotellaceae, Prevotella_1, and Olsenella were rich in the two lung cancer groups and also Cryptobacterium in ESLC and Lachnospira, Roseburia, Brevundimonas, Lachnospiraceae_UCG-006, and Lachnospiraceae_UCG-004 in HC.
Linear discriminant analysis (LDA) effect size (LEfSe) was used to identify key microbial taxa. Eight, five, and two key genera were identified in HC, ESLC, and NESLC, respectively (Figure 2). The genus with an average relative abundance of less than 0.01% was excluded. The key species were Roseburia (LDA score 4.22, p = 0.012), Lachnospira (LDA score 4.21, p = 0.001), Anaerostipes (LDA score 3.83, p = 0.007), and Lachnoclostridium (LDA score 3.60, p = 0.042) in HC; Lactobacillus in ESLC (LDA score 3.89, p = 0.029); and Escherichia_Shigella in NESLC (LDA score 4.53, p = 0.010).
Figure 2 Key genera selection. Differential microbial score chart: the higher the score, the greater the contribution of the microbe to the difference.
Metabolites and fermentation products produced by intestinal flora can enter the blood and have a functional impact on the host’s physiology. Therefore, in order to further explore the changes in the intestinal microbe–host interaction, we examined the metabolic profile in the serum. Based on the abundance of metabolites detected by non-targeted metabolomics, the orthogonal partial least-squares discriminant analysis (OPLS-DA) was performed. According to the scatter plot, samples from different groups were largely separable, indicating different metabolic patterns (Figures 3A–C). The permutation test showed that there was no overfitting to the data, and verified the OPLS-DA model (Figures 3D–F). Generally, the closer the slopes of the R2Y and Q2Y lines were to zero, the more likely the model was to be overfitted. A total of 5,514 metabolites were identified, consisting of 2,793 of positive ion and 2,721 of negative ion (Supplemental Material 4).
Figure 3 Principal component analysis. (A–C) OPLS-DA score chart shows the difference in metabolites between groups. The abscissa represents the variation between groups, and the ordinate represents the variation within groups. (D–F) Comparison of the true model parameters in the validation test and those of permutated models.
Variable importance of projection (VIP) value was obtained through the OPLS-DA model. The biologically significant differential metabolites were mined according to the screening criteria: the VIP value of the first principal component of the OPLS-DA model >1 and the p-value of the t-test <0.05. The larger the value, the greater the contribution of the variable to the grouping. ESLC vs. HC, NESLC vs. HC, and ESLC vs. NESLC screened out 272, 319, and 68 differential metabolites, respectively (Supplemental Material 5). Hierarchical clustering was performed on the expression of the differential metabolites with the top 50 of VIP value to show the relationship among samples and the expression differences of metabolites among samples more intuitively. The result is shown below (Figure 4). 9-Hydroxy-7-megastigmen-3-one glucoside, 1-[6-(3)-ladderane-hexanoyl-2-(8-(3)-ladderane-octany])-sn-glycerophosphocholine, and perilloside A were more abundant in the lung cancer groups, while indoleacrylic acid, L-isoleucine, L-valine, PC(O−16:0/2:0), and LysoPC (16:0) decreased.
Figure 4 Heatmap of differential metabolites. (A) Differential metabolites in ESLC vs. HC. (B) Differential metabolites in NESLC vs. HC. (C) Differential metabolites in ESLC vs. NESLC. The abscissa represents the sample name, and the ordinate represents the differential metabolite. The color from blue to red indicates that the expression abundance of metabolites is from low to high.
Correlation analysis can help measure the correlation between differential metabolites. Differential metabolites with the top 50 of VIP value were selected for visual analysis. It was found that perilloside C, which was richer in the lung cancer groups, was negatively correlated with PC(O−16:0/2:0), which was richer in the HC group (Supplemental Material 6).
The KEGG ID of the metabolites was used for pathway enrichment analysis. The differential metabolites of ESLC vs. HC were mainly involved in aminoacyl-tRNA biosynthesis; valine, leucine, and isoleucine biosynthesis; ABC transporters; and sphingolipid signaling pathway (p < 0.01); those of NESLC vs. HC were involved in caffeine metabolism; valine, leucine, and isoleucine biosynthesis; aminoacyl-tRNA biosynthesis; Fc gamma R-mediated phagocytosis; and choline metabolism in cancer (p < 0.01). On the other hand, the differential metabolites of ESLC vs. NESLC were involved in butanoate metabolism, aminoacyl-tRNA biosynthesis, and apoptosis (p < 0.01) (Figure 5).
Figure 5 (A–C) The p-value is the significance of enrichment in metabolic pathways. The red line indicates that the p-value is 0.01, and the blue line indicates that the p-value is 0.05. When the top of the bar is higher than the blue line, the signal pathway it represents is significant. (D–F) The ordinate is the name of the metabolic pathway, and the abscissa is the rich factor (rich factor = the number of significantly different metabolites/the total number of metabolites in the pathway); the greater the rich factor, the greater the degree of enrichment. The color from green to red indicates that the p-value decreases sequentially; the larger the point, the more metabolites enriched on the pathway.
We found that the aminoacyl-tRNA biosynthesis pathway seemed to be closely related to the progression of lung cancer, so we focused on the differential metabolites involved in it, L-valine, L-lysine, L-isoleucine, L-histidine, and L-glutamate, to seek new biomarkers (Supplemental Material 7). We reviewed the expression levels of these metabolites in different samples (Figure 6). The results showed that L-lysine, L-isoleucine, and L-histidine decreased significantly in the lung cancer groups. Compared with ESLC, L-glutamate increased significantly in NESLC. L-valine showed significant differences in the pairwise comparisons, and the trend of change was first down and then up, but always lower than the healthy level.
Correlation analysis was performed to help us better understand the correlation between the microbiome and plasma metabolome. Based on metabolomics and genomics datasets, network analysis was performed to determine broader associations between the two (Figure 7 and Supplemental Material 8). The CorNetwork diagram showed the relationship between Anaerostipes, Coprococcus_3, Lachnospiraceae_UCG-004, Lachnospiraceae_UCG-006, and various metabolites. The Spearman correlation coefficients between the top 100 differential metabolites and the top 100 differential microbes were calculated (Supplemental Material 9). The results showed that there was a significant correlation between L-valine and Lachnospira, Anaerostipes, Coprococcus_3, Fusicatenibacter, Lachnospiraceae_UCG-004, [Eubacterium]_xylanophilum_group, Lachnospiraceae_UCG-006, and Burkholderia–Caballeronia–Paraburkholderia. Among them, Lachnospiraceae_UCG-006 was the most correlated genus (correlation = 0.49, p < 0.001), which was also a differential genus enriched in HC.
Figure 7 The CorNetwork diagram. The connection indicates correlation. The red connecting line represents a positive correlation between nodes, while the blue line represents a negative correlation.
Our research has proved the changes of intestinal microbiota and serum metabolic spectrum in lung cancer patients. We combined the two omics to find the possible pathogenesis and potential biomarkers of lung cancer.
The control group consisted of individuals undergoing physical examination from the Health Management Center, while lung cancer patients were newly diagnosed without treatment. Medication use is an important consideration when we screen study subjects, which is mainly because any medication, such as antibiotics and anticancer medication, will cause changes in intestinal flora to varying degrees. We have observed differences in the composition and structure of gut microbes between lung cancer patients with different stages and healthy people. Faecalibacterium has a higher abundance in HC, and its relative and absolute abundances in ESLC and NESLC decreased sequentially. Faecalibacterium is considered to be a marker of healthy intestines and is a type of butyrate-producing bacteria (Wang et al., 2007). In previous studies, it was also found that its abundance decreased in lung cancer patients (Gui et al., 2020; Zheng et al., 2020). In addition, ESLC has abundant Klebsiella and Escherichia–Shigella, while NESLC has abundant Prevotella_9 and Escherichia–Shigella. Klebsiella, Escherichia–Shigella, and Prevotella_9 all contain many opportunistic pathogens. The latest research has linked the increase in Prevotella abundance with local and systemic diseases, including rheumatoid arthritis, hypertension, and metabolic disorders (Horta-Baas et al., 2017; Li et al., 2017; Ding et al., 2020).
Previous studies have shown that the changes of intestinal flora in patients with lung cancer are often related to Firmicutes, Bacteroides, and Proteobacteria (Zhang et al., 2018; Liu et al., 2019; Zhuang et al., 2019; Zheng et al., 2020; Georgiou et al., 2021). When using the Kruskal–Wallis algorithm to further identify microbes with different abundances, it was found that the abundance of Firmicutes decreased and Proteobacteria increased in the lung cancer group. Compared with previous studies, the change of Firmicutes was consistent and Proteobacteria was also consistent with most studies, which only decreased in the study of Zhang et al. However, Bacteroides has been found to increase in lung cancer, while Actinomycetes has been found to decrease (Zhuang et al., 2019; Zheng et al., 2020), which was not found in our study, even in the comparison between HC and NESLC. We try to find the reasons for the differences from the included population and experimental design. The study of Zheng et al. included patients with early-stage lung cancer. Although one of its exclusion criteria was antibiotic use in the last 8 weeks, the use of anticancer drugs was not specified. In the study of Zhuang et al., subjects did not use any drugs in the past 3 months and seemed to have not received chemotherapy, but it was uncertain whether there were therapeutic behaviors other than chemotherapy. Our subjects were all newly diagnosed and treated patients, so we consider that the difference may be due to the selection criteria of subjects or the heterogeneity of patients. It is worth mentioning that although no difference was found at the level of Bacteroides and Actinomycota, differential genera mined such as Roseburia, Lachnospira, Olsenella, and Cryptobacterium belonged to Bacteroides or Actinomycota.
When looking for key differential microorganisms, it was found that Anaerostipes, Lachnoclostridium, Roseburia, and Lachnospira were the key differential genera in HC. These genera are closely related to the production of short-chain fatty acids (Liu et al., 2019; Chen et al., 2021; Yi et al., 2021). Short-chain fatty acids play an important role in human health because they regulate the function and differentiation of almost all intestinal immune cells (Surono et al., 2021). It has been found that short-chain fatty acids maintain intestinal homeostasis by promoting the production of IL-10 in Th1 cells (Sun et al., 2018). Xia et al. (2020) made preparations from plant flower buds to induce SCFA-producing bacteria to produce SCFAs to achieve an anticancer effect. Correspondingly, the key differential genus in ESLC was Lactobacillus. Lactobacillus itself is a kind of beneficial bacteria, which acts in preventing pathogenic bacteria from invading and colonizing the intestines, enhances the body’s immunity, and has anticancer effects (Hendler and Zhang, 2018; Eslami et al., 2019; Han et al., 2021). In colorectal cancer, Lactobacillus showed reduced abundance (Loftus et al., 2021). However, in a large cohort study, it was found that the increased abundance of Lactobacillus bacteria in the oral cavity was closely related to the risk of lung cancer (Hosgood et al., 2021). The key differential genus in NESLC was Escherichia_Shigella. The increased abundance of Escherichia/Shigella was considered to be a characteristic of intestinal flora imbalance in Crohn’s disease (Pascal et al., 2017). These indicate that compared with HC, patients with early/advanced lung cancer have different degrees of intestinal flora imbalance. Random forest algorithm, a kind of machine learning algorithm, was used to screen important microorganisms that distinguish differences between groups. The screened genera were mostly the same as the key genera in each group. However, it should be noted that due to the hierarchical structure of the algorithm, there may be no linear relationship between the filtered features and the output. For example, Pseudomonas, although selected as the most important genus, was not a differential genus, and the difference between groups was not statistically significant.
Pathway enrichment analysis was performed using differential metabolites to understand the mechanism of metabolic pathway changes in different samples. Results showed that the aminoacyl-tRNA biosynthesis pathway was enriched in HC vs. ESLC, HC vs. NESLC, and ESLC vs. ESLC, which seemed to be closely related to the progression of lung cancer. In previous studies, the aminoacyl-tRNA biosynthesis pathway was an enrichment pathway for UAP1 expression-related genes in lung adenocarcinoma (Wang et al., 2020). It was closely related to cisplatin resistance in non-small cell lung cancer (Shi et al., 2019). Moreover, it has also been found to be upregulated in gastric cancer, and researchers even proposed a new therapeutic strategy for gastric cancer targeting the aminoacyl-tRNA biosynthesis pathway (Gao et al., 2021). Differential metabolites in this pathway, L-lysine, L-isoleucine, and L-histidine, were significantly reduced in the lung cancer groups, but there was no significant difference between ESLC and NESLC, which meant that these indicators could not be used as reference indicators for staging. L-glutamate was only significantly different between ESLC and NESLC; thus, it was not the best predictor. The serum concentration of L-valine showed differences in the pairwise comparison, so it may be used as a potential marker. In the correlation analysis, we found that the most strongly related genus of L-valine was Lachnospiraceae_UCG-006, which was the differential genus we screened earlier. The level of valine in early-stage non-small cell lung cancer was lower than that in advanced stage (Puchades-Carrasco et al., 2016). In the study of Ni et al. (2019), valine declined in the lung cancer group as a potential biomarker, although this research was based on two existing data sets. In addition, valine has been reported as a potential biomarker for the differential diagnosis of seronegative rheumatoid arthritis and psoriatic arthritis (Souto-Carneiro et al., 2020). Upregulated concentrations of branched-chain amino acids were detected in stool samples from colorectal cancer patients and in gastric tissue fluid from mice with gastric cancer (Yachida et al., 2019; Gu et al., 2020).
It is worth mentioning that although this study describes the changes in the microbiome and metabolome in lung cancer and the relationship between the two, we cannot explain the causal relationship between them. This requires more and larger cohort studies to explore in the future.
This study has some limitations. Prognosis is an important part of disease research. However, due to the inability to track the prognosis of all patients in the short term, we are temporarily unable to study the prognosis of the disease. On the other hand, the strict entry conditions lead to a small number of participants. We hope to expand the sample size and conduct further studies through multicenter cooperation in our future research.
This study describes the characteristics of intestinal flora and serum metabolic profiles of patients with lung cancer in different stages. It reveals that lung cancer may be the result of the mutual regulation of L-valine and Lachnospiraceae_UCG-006 through the aminoacyl-tRNA biosynthesis pathway, and proposes that L-valine may be a potential marker for the diagnosis of lung cancer.
The datasets presented in this study can be found in online repositories. The names of the repository/repositories and accession number(s) can be found below: https://data.mendeley.com/datasets/8rftx9ybnm/1, genomic sequencing; https://data.mendeley.com/datasets/nj4cz7mmj5/1, metabolomics.
The studies involving human participants were reviewed and approved by the IRB of The Third Xiangya Hospital of Central South University. The patients/participants provided their written informed consent to participate in this study.
Design of the study: SC, RG, and Y-FF. Methodology: SC and X-HZ. Formal analysis: SC and X-HZ. Data curation: SC and X-HZ. Software: SC. Writing—original draft preparation: SC and X-HZ. Writing—review and editing: J-HZ, H-YJ, H-TL, SC, RG, and Y-FF. All authors contributed to the article and approved the submitted version.
This work was supported by the National Natural Science Foundation of China (Grant No. 82002452), and the Fundamental Research Funds for the Central Universities of Central South University (Grant No. 2021zzts1090).
The authors declare that the research was conducted in the absence of any commercial or financial relationships that could be construed as a potential conflict of interest.
All claims expressed in this article are solely those of the authors and do not necessarily represent those of their affiliated organizations, or those of the publisher, the editors and the reviewers. Any product that may be evaluated in this article, or claim that may be made by its manufacturer, is not guaranteed or endorsed by the publisher.
We thank Hunan Cancer Hospital for the help provided in specimen collection, Qin-yu Zhao for data analysis, and all the patients involved in the study.
The Supplementary Material for this article can be found online at: https://www.frontiersin.org/articles/10.3389/fcimb.2022.885093/full#supplementary-material
Bolger, A. M., Lohse, M., Usadel, B. (2014). Trimmomatic: A Flexible Trimmer for Illumina Sequence Data. Bioinformatics 30, 2114–2120. doi: 10.1093/bioinformatics/btu170
Caporaso, J. G., Kuczynski, J., Stombaugh, J., Bittinger, K., Bushman, F. D., Costello, E. K., et al. (2010). QIIME Allows Analysis of High-Throughput Community Sequencing Data. Nat. Methods 7, 335–336. doi: 10.1038/nmeth.f.303
Chao, A., Bunge, J. (2002). Estimating the Number of Species in a Stochastic Abundance Model. Biometrics 58, 531–539. doi: 10.1111/j.0006-341X.2002.00531.x
Chen, L., Zhou, X., Wang, Y., Wang, D., Ke, Y., Zeng, X. (2021). Propionate and Butyrate Produced by Gut Microbiota After Probiotic Supplementation Attenuate Lung Metastasis of Melanoma Cells in Mice. Mol. Nutr. Food Res. 65, e2100096. doi: 10.1002/mnfr.202100096
Ding, N., Zhang, X., Zhang, X. D., Jing, J., Liu, S. S., Mu, Y. P., et al. (2020). Impairment of Spermatogenesis and Sperm Motility by the High-Fat Diet-Induced Dysbiosis of Gut Microbes. Gut 69, 1608–1619. doi: 10.1136/gutjnl-2019-319127
Eslami, M., Yousefi, B., Kokhaei, P., Hemati, M., Nejad, Z. R., Arabkari, V., et al. (2019). Importance of Probiotics in the Prevention and Treatment of Colorectal Cancer. J. Cell Physiol. 234, 17127–17143. doi: 10.1002/jcp.28473
Farooq, M., Herman, J. G. (2020). Noninvasive Diagnostics for Early Detection of Lung Cancer: Challenges and Potential With a Focus on Changes in DNA Methylation. Cancer Epidemiol. Biomarkers Prev. 29, 2416–2422. doi: 10.1158/1055-9965.EPI-20-0704
Gao, X., Guo, R., Li, Y., Kang, G., Wu, Y., Cheng, J., et al. (2021). Contribution of Upregulated aminoacyl-tRNA Biosynthesis to Metabolic Dysregulation in Gastric Cancer. J. Gastroenterol. Hepatol. 36, 3113–3126. doi: 10.1111/jgh.15592
Georgiou, K., Marinov, B., Farooqi, A. A., Gazouli, M. (2021). Gut Microbiota in Lung Cancer: Where Do We Stand? Int. J. Mol. Sci. 22, 10429. doi: 10.3390/ijms221910429
Gu, J., Huang, C., Hu, X., Xia, J., Shao, W., Lin, D. (2020). Nuclear Magnetic Resonance-Based Tissue Metabolomic Analysis Clarifies Molecular Mechanisms of Gastric Carcinogenesis. Cancer Sci. 111, 3195–3209. doi: 10.1111/cas.14443
Gui, Q., Li, H., Wang, A., Zhao, X., Tan, Z., Chen, L., et al. (2020). The Association Between Gut Butyrate-Producing Bacteria and Non-Small-Cell Lung Cancer. J. Clin. Lab. Anal. 34, e23318. doi: 10.1002/jcla.23318
Han, X., Ding, S., Ma, Y., Fang, J., Jiang, H., Li, Y., et al. (2021). Lactobacillus plantarum and Lactobacillus brevis Alleviate Intestinal Inflammation and Microbial Disorder Induced by ETEC in a Murine Model. Oxid. Med. Cell Longev. 2021, 6867962. doi: 10.1155/2021/6867962
Hendler, R., Zhang, Y. (2018). Probiotics in the Treatment of Colorectal Cancer. Medicines (Basel) 5, 101. doi: 10.3390/medicines5030101
Hill, T. C., Walsh, K. A., Harris, J. A., Moffett, B. F. (2003). Using Ecological Diversity Measures With Bacterial Communities. FEMS Microbiol. Ecol. 43, 1–11. doi: 10.1111/j.1574-6941.2003.tb01040.x
Horta-Baas, G., Romero-Figueroa, M. D. S., Montiel-Jarquín, A. J., Pizano-Zárate, M. L., García-Mena, J., Ramírez-Durán, N. (2017). Intestinal Dysbiosis and Rheumatoid Arthritis: A Link Between Gut Microbiota and the Pathogenesis of Rheumatoid Arthritis. J. Immunol. Res. 2017, 4835189. doi: 10.1155/2017/4835189
Hosgood, H. D., Cai, Q., Hua, X., Long, J., Shi, J., Wan, Y., et al. (2021). Variation in Oral Microbiome is Associated With Future Risk of Lung Cancer Among Never-Smokers. Thorax 76, 256–263. doi: 10.1136/thoraxjnl-2020-215542
I, H., Cho, J. Y. (2015). Lung Cancer Biomarkers. Adv. Clin. Chem. 72, 107–170. doi: 10.1016/bs.acc.2015.07.003
Liu, F., Li, J., Guan, Y., Lou, Y., Chen, H., Xu, M., et al. (2019). Dysbiosis of the Gut Microbiome Is Associated With Tumor Biomarkers in Lung Cancer. Int. J. Biol. Sci. 15, 2381–2392. doi: 10.7150/ijbs.35980
Li, J., Zhao, F., Wang, Y., Chen, J., Tao, J., Tian, G., et al. (2017). Gut Microbiota Dysbiosis Contributes to the Development of Hypertension. Microbiome 5, 14. doi: 10.1186/s40168-016-0222-x
Loftus, M., Hassouneh, S. A., Yooseph, S. (2021). Bacterial Community Structure Alterations Within the Colorectal Cancer Gut Microbiome. BMC Microbiol. 21, 98. doi: 10.1186/s12866-021-02153-x
Ni, J., Xu, L., Li, W., Zheng, C., WU, L. (2019). Targeted Metabolomics for Serum Amino Acids and Acylcarnitines in Patients With Lung Cancer. Exp. Ther. Med. 18, 188–198. doi: 10.3892/etm.2019.7533
Pascal, V., Pozuelo, M., Borruel, N., Casellas, F., Campos, D., Santiago, A., et al. (2017). A Microbial Signature for Crohn's Disease. Gut 66, 813–822. doi: 10.1136/gutjnl-2016-313235
Puchades-Carrasco, L., Jantus-Lewintre, E., Pérez-Rambla, C., García-García, F., Lucas, R., Calabuig, S., et al. (2016). Serum Metabolomic Profiling Facilitates the non-Invasive Identification of Metabolic Biomarkers Associated With the Onset and Progression of non-Small Cell Lung Cancer. Oncotarget 7, 12904–12916. doi: 10.18632/oncotarget.7354
Reyon, D., Tsai, S. Q., Khayter, C., Foden, J. A., Sander, J. D., Joung, J. K. (2012). FLASH Assembly of TALENs for High-Throughput Genome Editing. Nat. Biotechnol. 30, 460–465. doi: 10.1038/nbt.2170
Rognes, T., Flouri, T., Nichols, B., Quince, C., Mahé, F. (2016). VSEARCH: A Versatile Open Source Tool for Metagenomics. PeerJ 4, e2584. doi: 10.7717/peerj.2584
Rudin, C. M., Brambilla, E., Faivre-Finn, C., Sage, J. (2021). Small-Cell Lung Cancer. Nat. Rev. Dis. Primers 7, 3. doi: 10.1038/s41572-020-00235-0
Shi, Y., Wang, Y., Huang, W., Wang, R., Yuan, Y. (2019). Integration of Metabolomics and Transcriptomics To Reveal Metabolic Characteristics and Key Targets Associated With Cisplatin Resistance in Nonsmall Cell Lung Cancer. J. Proteome Res. 18, 3259–3267. doi: 10.1021/acs.jproteome.9b00209
Souto-Carneiro, M., Tóth, L., Behnisch, R., Urbach, K., Klika, K. D., Carvalho, R. A., et al. (2020). Differences in the Serum Metabolome and Lipidome Identify Potential Biomarkers for Seronegative Rheumatoid Arthritis Versus Psoriatic Arthritis. Ann. Rheum. Dis. 79, 499–506. doi: 10.1136/annrheumdis-2019-216374
Sung, H., Ferlay, J., Siegel, R. L., Laversanne, M., Soerjomataram, I., Jemal, A., et al. (2021). Global Cancer Statistics 2020: GLOBOCAN Estimates of Incidence and Mortality Worldwide for 36 Cancers in 185 Countries. CA Cancer J. Clin. 71, 209–249. doi: 10.3322/caac.21660
Sun, M., Wu, W., Chen, L., Yang, W., Huang, X., Ma, C., et al. (2018). Microbiota-Derived Short-Chain Fatty Acids Promote Th1 Cell IL-10 Production to Maintain Intestinal Homeostasis. Nat. Commun. 9, 3555. doi: 10.1038/s41467-018-05901-2
Surono, I. S., Jalal, F., Bahri, S., Romulo, A., Kusumo, P. D., Manalu, E., et al. (2021). Differences in Immune Status and Fecal SCFA Between Indonesian Stunted Children and Children With Normal Nutritional Status. PloS One 16, e0254300. doi: 10.1371/journal.pone.0254300
Wang, X., Chen, X., Liu, H. (2020). Expression and Bioinformatics-Based Functional Analysis of UAP1 in Lung Adenocarcinoma. Cancer Manag. Res. 12, 12111–12121. doi: 10.2147/CMAR.S282238
Wang, Q., Garrity, G. M., Tiedje, J. M., Cole, J. R. (2007). Naive Bayesian Classifier for Rapid Assignment of rRNA Sequences Into the New Bacterial Taxonomy. Appl. Environ. Microbiol. 73, 5261–5267. doi: 10.1128/AEM.00062-07
Wen, Y., Jin, R., Chen, H. (2019). Interactions Between Gut Microbiota and Acute Childhood Leukemia. Front. Microbiol. 10, 3555. doi: 10.3389/fmicb.2019.01300
Xia, W., Khan, I., Li, X. A., Huang, G., Yu, Z., Leong, W. K., et al. (2020). Adaptogenic Flower Buds Exert Cancer Preventive Effects by Enhancing the SCFA-Producers, Strengthening the Epithelial Tight Junction Complex and Immune Responses. Pharmacol. Res. 159, 104809. doi: 10.1016/j.phrs.2020.104809
Yachida, S., Mizutani, S., Shiroma, H., Shiba, S., Nakajima, T., Sakamoto, T., et al. (2019). Metagenomic and Metabolomic Analyses Reveal Distinct Stage-Specific Phenotypes of the Gut Microbiota in Colorectal Cancer. Nat. Med. 25, 968–976. doi: 10.1038/s41591-019-0458-7
Yi, Y., Shen, L., Shi, W., Xia, F., Zhang, H., Wang, Y., et al. (2021). Gut Microbiome Components Predict Response to Neoadjuvant Chemoradiotherapy in Patients With Locally Advanced Rectal Cancer: A Prospective, Longitudinal Study. Clin. Cancer Res. 27, 1329–1340. doi: 10.1158/1078-0432.CCR-20-3445
Zhang, W. Q., Zhao, S. K., Luo, J. W., Dong, X. P., Hao, Y. T., Li, H., et al. (2018). Alterations of Fecal Bacterial Communities in Patients With Lung Cancer. Am. J. Transl. Res. 10, 3171–3185.
Zheng, Y., Fang, Z., Xue, Y., Zhang, J., Zhu, J., Gao, R., et al. (2020). Specific Gut Microbiome Signature Predicts the Early-Stage Lung Cancer. Gut. Microbes 11, 1030–1042. doi: 10.1080/19490976.2020.1737487
Keywords: lung cancer, intestinal microorganism, intestinal flora, serum metabolites, biomarker
Citation: Chen S, Gui R, Zhou X-h, Zhang J-h, Jiang H-y, Liu H-t and Fu Y-f (2022) Combined Microbiome and Metabolome Analysis Reveals a Novel Interplay Between Intestinal Flora and Serum Metabolites in Lung Cancer. Front. Cell. Infect. Microbiol. 12:885093. doi: 10.3389/fcimb.2022.885093
Received: 27 February 2022; Accepted: 04 April 2022;
Published: 02 May 2022.
Edited by:
Jaime Garcia-Mena, Centro de Investigaciones y Estudios Avanzados, Instituto Politécnico Nacional de México (CINVESTAV), MexicoReviewed by:
Maria Gazouli, National and Kapodistrian University of Athens, GreeceCopyright © 2022 Chen, Gui, Zhou, Zhang, Jiang, Liu and Fu. This is an open-access article distributed under the terms of the Creative Commons Attribution License (CC BY). The use, distribution or reproduction in other forums is permitted, provided the original author(s) and the copyright owner(s) are credited and that the original publication in this journal is cited, in accordance with accepted academic practice. No use, distribution or reproduction is permitted which does not comply with these terms.
*Correspondence: Yun-feng Fu, ZnV5dW5mZW5nQGNzdS5lZHUuY24=
Disclaimer: All claims expressed in this article are solely those of the authors and do not necessarily represent those of their affiliated organizations, or those of the publisher, the editors and the reviewers. Any product that may be evaluated in this article or claim that may be made by its manufacturer is not guaranteed or endorsed by the publisher.
Research integrity at Frontiers
Learn more about the work of our research integrity team to safeguard the quality of each article we publish.