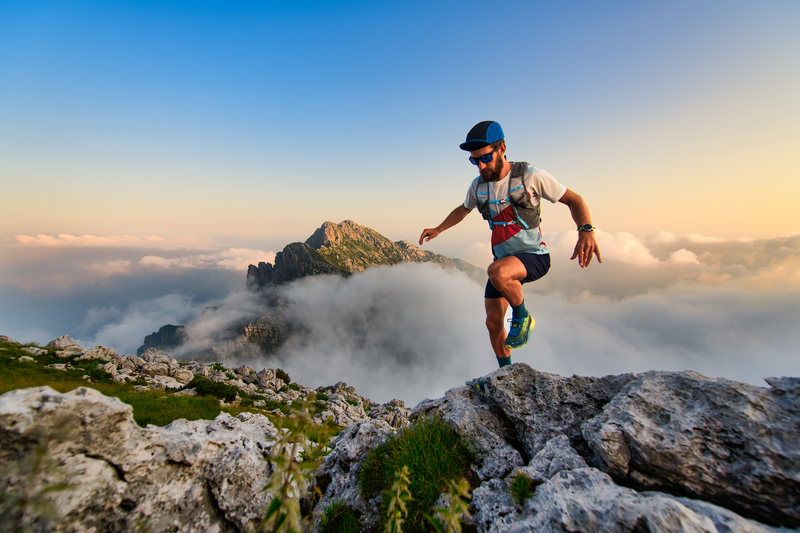
95% of researchers rate our articles as excellent or good
Learn more about the work of our research integrity team to safeguard the quality of each article we publish.
Find out more
ORIGINAL RESEARCH article
Front. Cell. Infect. Microbiol. , 02 June 2022
Sec. Clinical Microbiology
Volume 12 - 2022 | https://doi.org/10.3389/fcimb.2022.859981
This article is part of the Research Topic Protozoal Infections: Treatment and Challenges View all 10 articles
The therapeutic challenges pertaining to leishmaniasis due to reported chemoresistance and toxicity necessitate the need to explore novel pathways to identify plausible inhibitory molecules. Leishmania donovani 24-sterol methyltransferase (LdSMT) is vital for the synthesis of ergosterols, the main constituents of Leishmania cellular membranes. So far, mammals have not been shown to possess SMT or ergosterols, making the pathway a prime candidate for drug discovery. The structural model of LdSMT was elucidated using homology modeling to identify potential novel 24-SMT inhibitors via virtual screening, scaffold hopping, and de-novo fragment-based design. Altogether, six potential novel inhibitors were identified with binding energies ranging from −7.0 to −8.4 kcal/mol with e-LEA3D using 22,26-azasterol and S1–S4 obtained from scaffold hopping via the ChEMBL, DrugBank, PubChem, ChemSpider, and ZINC15 databases. These ligands showed comparable binding energy to 22,26-azasterol (−7.6 kcal/mol), the main inhibitor of LdSMT. Moreover, all the compounds had plausible ligand efficiency-dependent lipophilicity (LELP) scores above 3. The binding mechanism identified Tyr92 to be critical for binding, and this was corroborated via molecular dynamics simulations and molecular mechanics Poisson–Boltzmann surface area (MM-PBSA) calculations. The ligand A1 was predicted to possess antileishmanial properties with a probability of activity (Pa) of 0.362 and a probability of inactivity (Pi) of 0.066, while A5 and A6 possessed dermatological properties with Pa values of 0.205 and 0.249 and Pi values of 0.162 and 0.120, respectively. Structural similarity search via DrugBank identified vabicaserin, daledalin, zanapezil, imipramine, and cefradine with antileishmanial properties suggesting that the de-novo compounds could be explored as potential antileishmanial agents.
Visceral leishmaniasis, the most debilitating form of leishmaniasis, is caused by Leishmania donovani and Leishmania infantum (Ikeogu et al., 2020). It is one of the oldest neglected tropical diseases that remain a major challenge to the global community. It is estimated to affect over 10 million people and cause up to 30,000 deaths annually (Hernández-Bojorge et al., 2020). The present chemotherapeutic options comprising pentavalent antimony, pentamidine (PTM), amphotericin B (Amp B), miltefosine (Milt), paromomycin, and liposomal Amp B suffer from numerous inefficiencies such as long treatment durations, cytotoxicity, resistance, and high cost, necessitating the urgent need for alternative therapeutic agents (Ghorbani and Farhoudi, 2018; Sakyi et al., 2021a).
Target identification and validation are pivotal for rational drug designs (Lionta et al., 2014). Contemporary strategies comprising experimental (metabolomic and transcriptomic approaches) and computational (structure- and ligand-based) approaches have led to the identification of numerous biological targets necessary for the survival of Leishmania parasites (Mandal et al., 2009; Mavromoustakos et al., 2011; Rinschen et al., 2019; Kwofie et al., 2020). However, the incomplete knowledge on Leishmania biology and the limited studies on the exact functions of sterols in intracellular organelles have hampered the exploitation of effective sterol inhibitors against leishmaniasis. While cholesterol is biosynthesized in humans, Leishmania and other protozoa synthesize ergosterol. Due to this difference, a number of drugs including bisphosphonates, statins, azoles, and quinuclidine have been used for leishmaniasis treatment via the inhibition of the ergosterol biosynthetic pathway (Sakyi et al., 2021b). Sterol methyltransferase (SMT) is an enzyme involved in ergosterol biosynthesis which is understudied partly due to the paucity of structural genomics data. This notwithstanding, investigations are ongoing to explore SMT in designing drugs against leishmaniasis due to its absence in the human host coupled with the fact that it is highly conserved among all Leishmania parasites (Magaraci et al., 2003; Kidane et al., 2017).
SMT belongs to the family of transferases and functions by catalyzing methyl transfer from S-adenosyl methionine onto the C24 position of the lanosterol or the cycloartenol side chain during ergosterol biosynthesis. For example, genetic ablation studies of 24-SMT orthologs involved in the sterol biosynthetic pathway have demonstrated that ergosterol, one of the widely recognized classes of lipids in the cellular membrane of protozoans, plays a significant role in plasma membrane stabilization and mitochondrion function (Mukherjee et al., 2019). Studies have demonstrated the crucial functions of SMT to Leishmania survival, and hence, it is considered as a plausible target for drug design (Urbina et al., 1995; Mukherjee et al., 2019; Sakyi et al., 2021a). For instance, vaccine evaluation studies against Leishmania 24-SMT identified 24-SMT as an essential drug target (Goto et al., 2009). Leishmania 24-SMT dysfunction results in the increased generation of reactive oxygen species and vesicular trafficking (Mukherjee et al., 2019). In addition, 24-alkyl sterols have been shown to be essential growth factors of Trypanosoma cruzi to the extent that its perturbation during ergosterol biosynthesis leads to cell cycle defects and DNA fragmentation (Urbina et al., 1995; Pérez-Moreno et al., 2012). Furthermore, RNA-seq analysis has revealed genomic instability at the locus of SMT, resulting in the promotion of amphotericin B resistance in Leishmania parasites (Pountain et al., 2019). Similarly, gigantol and imipramine suppressed the growth and proliferation of promastigotes and amastigotes via inhibition of SMT (Andrade-Neto et al., 2016; Rahman et al., 2021). Similarly, the antiproliferative effects of sterol biosynthesis inhibition on Pneumocystis carinii have hinted sterol methyltransferase suppressors as potential chemotherapeutic options for the treatment of P. carinii infections (Urbina et al., 1997). However, the recent resistance associated with 22,26-azasterol targeting SMT warrants the identification of novel inhibitors.
In-silico techniques in drug design are advantageous due to the reduced cost, time, and energy compared with traditional high-throughput screening (HTS) (Mak and Pichika, 2019). One of the strategies employed in the identification of lead compounds with improved efficacy in rational drug design includes scaffold hopping (Hu et al., 2017). It starts with a known active compound and ends up with new chemotypes with different core structures but with equal or improved efficacy (Hu et al., 2017). A typical example is the discovery of cyproheptadine from pheniramine, an antihistamine used to treat allergic conditions, such as hay fever or urticaria (Sun et al., 2012). Pheniramine has two aromatic rings joined to one carbon or nitrogen atom and a positive charge center. Cyproheptadine, an analog of pheniramine, has significantly improved binding affinity against the H1 receptor. This rigidified molecule with better absorption was achieved by locking both aromatic rings of pheniramine to the active conformation through ring closure and by introducing the piperidine ring to further reduce the flexibility of the molecule (Sun et al., 2012). In addition, these structural changes gave other medical benefits including cyproheptadine as a prophylaxis for migraine, pizotifen for the treatment of migraine, and azatadine as a typical potent sedating antihistamine (Sun et al., 2012). Tramadol was obtained through scaffold hopping of morphine (Sun et al., 2012). The recent interest in de-novo drug design compared with repurposing presents a new paradigm shift, not only in terms of time and cost but also innovation (Talevi and Bellera, 2019). The identification of leads from hits and then optimization to druggable candidates in the drug design pipeline result not only in an increase in potency and selectivity but also improved drug-like properties (Gil and Martinez, 2021). In addition, de-novo drug design has increased the areas explored in the chemical space of molecules leading to improvements in chemotherapeutic efficacy (Lin et al., 2020). This strategy has also been used in the design of drugs including vemurafenib, venetoclax, and dihydroorotate dehydrogenase inhibitors against malaria and aryl sulfonamide, a new aurora A kinase inhibitor (Jacquemard and Kellenberger, 2019). Despite the success achieved using the de-novo design, its use in the search for potential hits against L. donovani 24-sterol methyltransferase (LdSMT) is limited.
The study sought to utilize in-silico approaches to predict putative inhibitors targeting LdSMT. To accomplish this, the three-dimensional (3D) structure of LdSMT was first elucidated via modeling followed by subjection of 22,26-azasterol to de-novo drug design. Next, molecular docking and molecular dynamics simulation studies of the complexes were undertaken to identify potential novel LdSMT inhibitors. Furthermore, the biological activity and pharmacological profiles of the compounds were predicted to reinforce their lead-likeness.
A workflow schema detailing the stepwise techniques employed in this study is shown in Figure 1. The compound 22,26-azaserol was submitted to balanced rapid and unrestricted server for extensive ligand-aimed screening (BRUSELAS) (Banegas-Luna et al., 2019) to generate non-steroidal inhibitors. Meanwhile, a reasonably good structure of LdSMT was modeled and validated. The non-steroidal inhibitors were virtually screened against the LdSMT to identify compounds with high binding affinity to the receptor. The complexes of these compounds then served as input to the e-LEA3D (Douguet, 2010) for the generation of the novel compounds. Molecular dynamics (MD) simulation and molecular mechanics Poisson–Boltzmann surface area (MM-PBSA) were computed on the LdSMT–ligand complexes to determine the molecular interactions as well as the stability during the simulation. Absorption, distribution, metabolism, excretion, and toxicity (ADMET) predictions were performed to evaluate the pharmacological profiles of the selected compounds. The inhibitory constant, ligand efficiency, ligand efficiency scale, fit quality, binding efficiency index, surface efficiency index, and ligand efficiency-dependent lipophilicity were also calculated to assess the quality parameters of the ligands in binding to the target protein. In addition, the biological activity of the selected hits was predicted using the open Bayesian machine learning technique (Lagunin et al., 2000).
The protein sequence of LdSMT (SCMT1/GenBank ID: AAR92099.1) was retrieved from the National Center for Biotechnology Information (NCBI) database (Boratyn et al., 2013). To identify similar proteins as templates for building the 3D structure of LdSMT, the fast-all (FASTA) format of the sequence was aligned with the homologous sequences of crystal protein structures in the Protein Data Bank (PDB) (Berman et al., 2000) using the Basic Local Alignment and Sequencing Tool (BLAST) (Burley et al., 2017).
Due to the unavailability of an experimentally elucidated 3D structure for LdSMT, molecular modeling techniques were used to predict a reasonably accurate protein structure (Crentsil et al., 2020). Modeller version 10.2 (Eswar et al., 2008) was employed in building the 3D structure using three different templates: i) the 3BUS template was used to generate five models of the LdSMT (Eswar et al., 2008), ii) the protein structure with PDB ID 4PNE was also used to model five different structures of the LdSMT (Eswar et al., 2008), and iii) multitemplate homology modeling was employed to generate five structures of the LdSMT (Eswar et al., 2008). A total of three templates comprising 4PNE, 3BUS, and 6UAK were used in the multitemplate homology modeling approach. Modeller 10.2 was employed in generating all the potential structures of the LdSMT (Fiser and Šali, 2003; Eswar et al., 2008). The reasonably best model in each approach was selected based on the discrete optimized protein energy (DOPE) scores (Eswar et al., 2008).
The structural quality and accuracy of the best models from each approach were assessed using PROCHECK (Laskowski et al., 2012) with results reported as Ramachandran plots (Anderson et al., 2005). Further validation with VERIFY 3D (Sasin and Bujnicki, 2004), ERRAT (Colovos and Yeates, 1993; Messaoudi et al., 2013), and PROVE (Sasin and Bujnicki, 2004) was also performed. The reasonably best model was selected based on all the quality assessments for the molecular docking studies. Protein Structure Analysis (ProSA) (Wiederstein and Sippl, 2007) was then used to investigate the problematic regions of the selected model.
The plausible binding sites of the selected protein were determined using the Computed Atlas of Surface Topology of proteins (CASTp) (Dundas et al., 2006; Tian et al., 2018). The predicted sites were visualized using PyMOL (PyMOL Molecular Graphics System, Version 1.5.0.4, Schrödinger, LLC, New York, USA) (Lighthall et al., 2010) and Chimera 1.16 (Pettersen et al., 2004). The predicted binding sites with relatively small areas and volumes, where no ligand could fit, were ignored (Agyapong et al., 2021).
Shape similarity searching and pharmacophore screening were undertaken using the spatial data file (sdf) format of 22,26-azasterol via BRUSELAS (Banegas-Luna et al., 2019). A total of 100 ligands were generated with varying degrees of similarities to the input ligand, 22,26-azasterol. All ligands devoid of the steroidal core with varying degrees of similarities were selected for binding affinity prediction using AutoDock Vina v1.2.0 (Trott and Olson, 2010).
AutoDock Vina (Trott and Olson, 2010) was used for molecular docking. The molecular docking process was performed in two different stages. The first docking stage involved ligands obtained from the scaffold hopping process (Pathania et al., 2021), while the second involved compounds obtained from the de-novo design studies (Agyapong et al., 2021). Altogether, 1,448 ligands were used for the docking studies.
For the first stage, the ligands were obtained from the scaffold hopping and their structural derivatives fetched from the DrugBank (Wishart et al., 2018), PubChem (Kim et al., 2021), ZINC15 (Sterling and Irwin, 2015), and ChemSpider databases (Pence and Williams, 2010). Compounds labeled X1, X2, X3, X4, X5, X6, and X7 which showed good half-maximum inhibitory concentration (IC50) values against Leishmania parasite’s sterol methyltransferase together with amphotericin B, miltefosine, paromomycin, and 22,26-azasterol were also used.
The ligands generated from the de-novo design were virtually screened against the LdSMT in the second stage. For both stages, the ligands and the protein were prepared using the AutoDock Tools (Morris et al., 2009) and saved in the input format of AutoDock Vina (Trott and Olson, 2010). The charge, hydrogen bond network, and histidine protonation state of the protein were assigned after pdbqt conversion. Grid box size was set to (91.445 × 73.502 × 78.352) Å3 with the center at (72.200, 58.009, 13.302) Å. Ligands were then screened against the LdSMT protein with exhaustiveness set to default 8.
The potential protein–ligand complex from the scaffold hopping was submitted to e-LEA3D (Douguet, 2010) for further de-novo design. The binding site radius was set to 15 Å and the final score set to 1 with the same active site coordinates as used in the molecular docking study. Conformational search was set to 10, number of generations to 30, and population size to 30 with the rest of the options left as default.
The atomistic details of binding between the LdSMT and small molecules upon ligand binding were determined using the BIOVIA discovery studio visualizer v19.1.0.18287 (BIOVIA, San Diego, CA, USA) (Šudomová et al., 2019).
The inhibitory constant (Ki) of the ligands was calculated from the binding energies of the selected compounds and the LdSMT protein (Islam and Pillay, 2020). In addition, ligand efficiency (LE) metrics including ligand efficiency scale (LE_Scale), fit quality (FQ), ligand efficiency-dependent lipophilicity (LELP), and surface binding efficiency were also determined (Hopkins et al., 2014).
The ADMET properties were determined using SwissADME (Daina et al., 2017) and the OSIRIS Property Explorer in Data Warrior (Sander et al., 2015). Pan-assay interference compounds (PAINS) (Daina et al., 2017) and synthetic accessibility (Daina et al., 2017) search using SwissADME (Daina et al., 2017) were performed to eliminate false positive compounds that possess good physiochemical properties as well as those with complex structures.
The biological activity of the selected compounds was predicted using prediction of activity spectra for substance (PASS) (Lagunin et al., 2000) with the simplified molecular input line entry system (SMILES) as inputs.
A 100-ns MD simulation was performed for the unbound LdSMT and the protein–hit complexes using GROMACS 2018 (Van Der Spoel et al., 2005; Abraham et al., 2015). QtGrace (Dahiya et al., 2019) was used to plot the graphs generated from the MD simulation. The binding free energies of the complexes were calculated using MM-PBSA (Kumari et al., 2014). The energy contribution of each residue was also determined using g_MMPBSA. The graphs from the MM-PBSA computations were generated using the R programming language (Tippmann, 2014; Alkarkhi and Alqaraghuli, 2020).
Structural similarity search of all the hits was done via DrugBank (Wishart et al., 2018) to identify drugs with potential antileishmanial activity and possible mechanisms of action from similar compounds.
The 3D structure of LdSMT is yet to be experimentally elucidated; therefore, the structure was modeled. A BLAST (Boratyn et al., 2013) search of the protein sequence of LdSMT (SCMT1/GenBank ID: AAR92099.1) was performed via NCBI BLAST (Boratyn et al., 2013) to identify suitable identical templates to the LdSMT. The search revealed 12 experimentally determined protein structures that are identical to the LdSMT (Supplementary Table 1). The most widely used criteria in selecting a template is to choose the template with the highest sequence identity to the query sequence (Broni et al., 2021) and that was used for the modeling. However, the resolution at which the template protein structure was experimentally determined must also be taken into consideration. Also, the coverage of the template sequence to the query is another important factor. Herein, the E-value, sequence identity, query coverage, and the resolution of the 3D structures were used to select the most suitable templates as previously done (Meier and Söding, 2015; Haddad et al., 2020; Kwofie et al., 2021).
All the 12 identical protein structures had sequence identity less than 30% to the LdSMT, and 5WP4 demonstrated the highest with an identity of 29.01% (Supplementary Table 1); however, 5WP4 had a relatively low coverage of 45% to the LdSMT (Supplementary Table 1) and an E-value of 1 × 10−11. The 3BUS template had the least E-value of 6 × 10−20 and identity of 24.12%. The 3BUS protein has previously been used in modeling the SMT of L. infantum (Azam et al., 2014). Although 3BUS had a low resolution (2.65 Å), it was selected as one of the structures for modeling. 3BUS is the crystal structure of the rebeccamycin 4′-O-methyltransferase (RebM) in complex with S-adenosyl-l-homocysteine (Singh et al., 2008). On the other hand, the 4PNE template was also shortlisted as a suitable template due to its high coverage to the LdSMT (61%), high resolution (1.50 Å), and sequence identity similar to that of 3BUS (24.15%). 4PNE is the SpnF enzyme in Saccharopolyspora spinosa involved in the biosynthesis of the insecticide spinosyn A (Fage et al., 2015; Jeon et al., 2017). SpnF has been reported to be structurally similar to S-adenosyl-L-methionine (SAM)-dependent methyltransferases (Fage et al., 2015).
Furthermore, a BLAST search via the SWISS-MODEL (Waterhouse et al., 2018) revealed that 4PNE covered residues 43 to 258 while 3BUS spanned from residues 47 to 276 of the LdSMT. From residues 258 to 353 of the LdSMT sequence, both templates do not share similarities with the LdSMT. Thus, the protein structure with PDB ID 6UAK was selected in addition to 3BUS and 4PNE for the multitemplate homology modeling. The 6UAK shared similarity with the LdSMT mostly from residues 100 to 345. 6UAK is a SAM-dependent methyltransferase (LahSB) from the Lachnospiraceae bacterium C6A11 (Huo et al., 2020). Both 3BUS and 6UAK templates, like the LdSMT, are methyltransferases, while the 4PNE is a methyltransferase-like protein.
An earlier study identified Modeller (Eswar et al., 2008) to predict the most accurate model of L. infantum sterol methyltransferase (Azam et al., 2014). Although the two organisms (L. infantum and L. donovani) belong to the same genus and SMT is highly conserved among Leishmania species (Goto et al., 2007), there was a need to model the structure of LdSMT to ascertain its accuracy. Therefore, Modeller 10.2 (Eswar et al., 2008) was employed for modeling the structure of the LdSMT.
Three modeling approaches were employed to predict the most reasonably accurate LdSMT model. First, 3BUS was used as template to model five structures of the LdSMT. Secondly, 4PNE was also used to model five different structures of the LdSMT. Lastly, a multitemplate homology modeling approach was employed by using three templates comprising 3BUS, 4PNE, and 6UAK protein structures. For each approach, the best model was selected based on the DOPE score, which is an atomic distance-dependent statistical potential calculated from a sample of native protein structures (Shen and Sali, 2006). The DOPE scores were used to distinguish “good” models from “bad” ones with lower DOPE scores signifying a better model (Shen and Sali, 2006; Eswar et al., 2008).
The 3BUS template with a sequence identity of 24.12% and coverage of 63% to the LdSMT was used to generate five potential models of the LdSMT (Supplementary Table 1). The five generated models (referred to as MOD3BUS1, MOD3BUS2, MOD3BUS3, MOD3BUS4, and MOD3BUS5) had genetic algorithm 341 (GA341) scores ranging from 0.87 to 0.99 (Supplementary Table 2). The GA341 score assesses the reliability of a model and has a determined threshold of 0.7. A model is said to be reliable when the GA341 score is higher than the cutoff (0.7) (Broni et al., 2021). For all the 3BUS-based models, the GA341 scores were greater than the cutoff signifying their reliability. Model MOD3BUS2 had the least DOPE score of −30,234.79297 and was selected as the most reasonable structure among the three models (Supplementary Table 2 and Supplementary Figure 2A).
A total of five structures were predicted using 4PNE as template. 4PNE had a sequence identity of 24.15% and a coverage of 61% to the LdSMT (Supplementary Table 1). For the 4PNE-based models, the GA341 scores ranged between 0.73 and 0.98, signifying their high level of reliability (Supplementary Table 2 and Figure 2). Model MOD4PNE5 had the least DOPE score of −31,608.05664 and was thus selected as the most reasonably accurate model for the 4PNE-based structures (Supplementary Table 2 and Figure 2).
Figure 2 Cartoon representation of the structure of the selected Leishmania donovani 24-sterol methyltransferase (LdSMT) model (MOD4PNE5).
For the third set of models, three templates comprising 3BUS, 4PNE, and 6UAK were used for the modeling because it has been reported that the use of multiple templates can help increase the accuracy of a model (Larsson et al., 2008). Model MOD3TEMP2 had a GA341 score of 0.64307, lower than the 0.7 cutoff. However, the other four models had good GA341 scores ranging from 0.7 to 0.94 (Supplementary Table 2). Among the multiple template-generated models, MOD3TEMP3 had the least DOPE score and was thus selected as the most accurate (Supplementary Table 2 and Supplementary Figure 2B).
Next, validation and quality assessment of the predicted 3D structures were undertaken to obtain reasonable structures of the proteins. The best models from each of the three different approaches MOD3BUS2, MOD4PNE5, and MOD3TEMP3 were evaluated to select the most reasonably valid structure of the LdSMT.
The percentage of residues in the most favored, additionally allowed, generously allowed, and disallowed regions in a Ramachandran plot determines the quality of protein structures. From the Ramachandran plots generated from PROCHECK, model MOD3BUS2 had 270 (86.8%), 32 (10.3%), 6 (1.9%), and 3 (1.0%) residues in the most favored, additionally allowed, generously allowed, and disallowed regions, respectively (Table 1 and Supplementary Figure 3A). Model MOD3TEMP3 had 266 (85.5%), 31 (10.0%), 8 (2.6%), and 6 (1.9%) residues in the most favored, additionally allowed, generously allowed, and disallowed regions, respectively (Table 1 and Supplementary Figure 3B). For the MOD4PNE5 model, 264 (84.9%) residues were in the most favored region, 32 (10.3%) in the additionally favored region, 11 (3.5%) in the generously allowed region, and 4 (1.3%) in the disallowed region (Table 1 and Figure 3). The Ramachandran plot statistics of all three structures were comparatively close (Table 1) and are consistent with those of a previously modeled LiSMT structure using Modeller (Azam et al., 2014).
Figure 3 Ramachandran plot of the selected LdSMT model (MOD4PNE5) obtained via PROCHECK. The percentages of residues in the most favored regions, additionally allowed regions, generously allowed regions, and disallowed regions are 84.9%, 10.3%, 3.5%, and 1.3%, respectively.
The qualities of the three structures were further assessed via SAVES v6.0 (Behera et al., 2021). The protein structure MOD3BUS2 had a VERIFY score of 62.61%, ERRAT quality factor of 41.5663, PROVE score of 11%, and four PROCHECK errors, one warning and three passes (Supplementary Table 3). For the MOD3TEMP3 model, ERRAT predicted an overall score of 45.858, VERIFY a score of 53.82%, PROVE a score of 9.6%, and five PROCHECK errors, one warning and two passes (Supplementary Table 3). Model MOD4PNE5 was predicted to have ERRAT, VERIFY, and PROVE scores of 46.9565, 62.04%, and 7.1%, respectively. MOD4PNE5 was also predicted to have four PROCHECK errors, two warnings and two passes (Supplementary Table 3) For a model to be considered high quality, 80% of its amino acids must have a score of 0.2 in the 3D-1D profile (VERIFY score). Although all the top 3 structures did not have a VERIFY score above 80%, MOD3BUS2 (VERIFY score of 62.61) and MOD4PNE5 (VERIFY score of 62.04) could be considered since a previous study has shown that a crystallized structure also performed poorly based on the VERIFY 3D quality indicator (Mora Lagares et al., 2020). For the ERRAT predictions, MOD4PNE5 had the best score and was also predicted using PROVE to be less erroneous (Supplementary Table 3). Generally, model MOD3TEMP3 exhibited the lowest quality scores from SAVES v6.0.
Based on the quality assessments, the protein structure MOD4PNE5 was selected as the most reasonable structure of the LdSMT protein. Aligning the selected LdSMT structure and the chain A of the 4PNE structure revealed a close similarity with a root mean square deviation (RMSD) of 0.356 Å (Supplementary Figure 2C). The quality of the selected model (MOD4PNE5) was further assessed via ProSA-web (Sippl, 1993; Wiederstein and Sippl, 2007). With a Z-score (Zhang and Skolnick, 1998) of −3.97, the LdSMT structure was predicted to be of X-ray quality (Supplementary Figure 4A). The Z-score was used to indicate the overall protein quality (Zhang and Skolnick, 1998; Benkert et al., 2011). ProSA (Sippl, 1993; Wiederstein and Sippl, 2007) was used to plot the energies as a function of the amino acid sequence position for the LdSMT protein (Supplementary Figure 4B). Positive energy values signify problematic or erroneous regions of the protein structure. The residues of the protein demonstrated relatively low energies until residue 230, where positive energy values were observed until the end of the sequence.
The selected LdSMT structure was then refined using ModRefiner (Xu and Zhang, 2011). The refined structure of the selected LdSMT model showed improved Ramachandran plot statistics with 268 (86.2%), 34 (10.9%), 7 (2.3%), and 2 (0.6%) residues in the most favored, additionally allowed, generously allowed, and disallowed regions, respectively (Table 1).
CASTp was used to predict the plausible binding sites within the refined LdSMT structure. CASTp predicted 65 potential binding sites of the LdSMT protein. Ligand binding sites tend to involve the largest pockets or cavities on the protein (Laskowski et al., 1996; Liang et al., 1998); thus, pockets with relatively low areas and volumes, such that no ligand could fit, were not considered (Broni et al., 2021; Kwofie et al., 2021). A total of seven binding sites were shortlisted (Supplementary Table 4), which were visualized using PyMOL (PyMOL Molecular Graphics System, Version 1.5.0.4, Schrödinger, LLC) (Lighthall et al., 2010) and Chimera 1.16 (Pettersen et al., 2004). However, superimposing the LdSMT on the 4PNE template revealed that pocket 1 was similar to the S-adenosyl-L-homocysteine (SAH) ligand’s binding site in the 4PNE protein (Fage et al., 2015). Surprisingly, pocket 1 for LdSMT was predicted to have no opening in Chimera 1.16 (Pettersen et al., 2004), leaving pockets 2 to 7 as the most plausible binding cavities of the LdSMT (Supplementary Table 4). Pockets 5 and 7 also overlapped and occupied the same region (Supplementary Table 4).
Following the protocols of BRUSELAS, 100 ligands were generated using the ChEMBL database (Davies et al., 2015) with varying degrees of similarities to 22,26-azsterol. Out of the 100 ligands, 17 were identified to possess unique scaffolds devoid of the steroidal nucleus present in the 22,26-azasterol. The total score (comprising a combination of WEGA, LiSiCA, Screen3D, and OptiPharm) ranged from 0.38458 to 0.65814. Ten of the molecules with different scaffolds and varying scores are presented (Supplementary Table 5).
A search via the PubChem (Kim et al., 2021), ZINC15 (Sterling and Irwin, 2015), DrugBank (Wishart et al., 2018), and ChemSpider databases (Pence and Williams, 2010) generated 1,342 derivatives of all 17 scaffolds. The 1,370 compounds consisting of 17 scaffolds, 1,342 derivatives, 22,26-azasterol, 7 other known inhibitors (labeled X1–X7), and 3 already known drugs (amphotericin B, miltefosine, and paromomycin) were screened against an energy minimized LdSMT using a grid box of (91.445 × 73.502 × 78.352) Å3 with the center at (72.200, 58.009, 13.302) Å to cover the protein. Screening 1,370 compounds against the active site of the protein identified 25 hits which were selected based on the binding affinities and orientation within the binding site of the protein.
Among the three drugs used in leishmaniasis treatment, amphotericin B had the least binding energy of −5.3 kcal/mol followed by paromomycin (−5.0 kcal/mol) and miltefosine (−4.0 kcal/mol). Interestingly, all the known inhibitors had binding energies lower than the three drugs signifying a higher binding affinity to LdSMT. The binding energies of −5.9, −6.2, and −6.5 kcal/mol were obtained for X6, X3, and X4, respectively. The least binding energy of −7.7 kcal/mol was observed for X5 comparable to 22,26-azasterol (−7.6 kcal/mol). In-vitro studies reported that 22,26-azasterol inhibited L. donovani intracellular amastigotes and Trypanosoma brucei subsp. brucei with IC50 values of 8.9 and 1.76 μM, respectively (Magaraci et al., 2003; Gros et al., 2006), supporting the results reported herewith. Compounds X1, X7, and X2 had binding energies of −7.3, −7.2, and −7.0 kcal/mol, respectively (Supplementary Table 6). Similarly, in-vitro studies revealed that X1, X2, X3, X4, X5, X6, and X7 inhibited the growth of Leishmania parasites with IC50 less than 10 μM, except for X6 and X7 which were found to suppress growth with IC50 values of 28.6 and 30 μM, respectively (Magaraci et al., 2003; Lorente et al., 2004; Andrade-Neto et al., 2016; Torres-Santos et al., 2016).
The 12 best hits out of the 25 selected from the scaffold hopping had lower binding energies compared with the three drugs (Supplementary Table 6). In addition, the binding energies of these ligands were also found to be comparable to the known inhibitors with S1 showing the least binding energy of −9.0 kcal/mol. The closest to this ligand were S2, S3, and S4 with binding energies of −8.9, −8.8, and −8.7 kcal/mol, respectively (Supplementary Table 6). Compounds S12, S11, and S10 had the highest binding energies among the 12 best compounds with binding energies of −7.0, −7.0, and −7.2 kcal/mol, respectively. In addition, the binding energies of compounds S9 (−7.3 kcal/mol), S8 (−7.4 kcal/mol), and S7 (−7.4 kcal/mol) were also obtained. Comparable binding energies to the two lowest binding energies of the known inhibitors were obtained for S5 (−7.7 kcal/mol) and S6 (−7.6 kcal/mol).
The de-novo drug design is the generation of novel chemical entities that fit a set of constraints using computational algorithms (Schneider and Schneider, 2016). Despite the challenges of synthetic accessibility associated with this method, the application of de-novo drug design leads to the development of drug candidates in a cost- and time-efficient manner (Mouchlis et al., 2021). In addition, the de-novo design generates novel compounds with improved biological activity (Mouchlis et al., 2021). A number of studies have been undertaken for the de-novo design of inhibitors against plausible targets (Kranthi et al., 2018; Islam and Pillay, 2020; Pathania et al., 2021). A previous study selected a lead molecule based on the least binding energy as well the accurate pose of the ligand within the protein binding pocket for the de-novo design of inhibitors (Pathania et al., 2021). A similar approach was used in the identification of promising anti-DNA gyrase antibacterial compounds (Islam and Pillay, 2020). Ligands with very low binding energies and accurate pose have the potential to inhibit the receptor.
Among the compounds obtained from scaffold hopping, the well-known heterocyclic quinolinone and the phenylpiperazine/phenylpiperidine moieties found in several bioactive compounds were present. These compounds with diverse pharmacological potencies have been explored for various ailments including leishmaniasis (Kshirsagar, 2015; Chanquia et al., 2019; Mishra et al., 2021). One of such derivatives is 2-(4-(4,6-di(piperidin-1-yl)-1,3,5-triazin-2-ylamino)phenyl)-2-methyl2,3-dihydroquinazolin-4(1H)-one which had an IC50 value of 0.65 μM against intracellular amastigotes when compared with miltefosine (IC50 of 8.4 μM) (Sharma et al., 2013), corroborating the fact that de-novo drug design could result in novel scaffolds as potential antileishmanial agents.
The e-LEA3D was used to generate 155 potential novel compounds against LdSMT after using protein–S1, S2, S3, S4, and 22,26-azasterol complexes. They were filtered based on Lipinski’s rule of five (Benet et al., 2016) and redundancy to generate 78 ligands that were subjected to molecular docking studies. The six best hits (A1, A2, A3, A4, A5, and A6) (Figure 4) were selected for downstream analysis. The search via public databases including PubChem (Kim et al., 2021) and ChemSpider (Pence and Williams, 2010) showed that the six ligands do not have duplicates.
The docking analysis of the six compounds revealed the binding energies of A1 (−8.4 kcal/mol), A2 (−7.5 kcal/mol), and A3 (−7.2 kcal/mol), while A4, A5, and A6 had −7.0 kcal/mol. Though A1, A2, A3, and A6 have similar core structures, they had different binding energies (Table 2). Interestingly, using AutoDock Vina, compounds that had shown binding energies ≤−7.0 kcal/mol have been found to demonstrate significant inhibitory activities against the parasite of consideration (Chang et al., 2007; Wyllie et al., 2018; Tabrez et al., 2021). In lieu of this, the predicted compounds may have the potential of suppressing LdSMT since the binding energies were lower than −7.0 kcal/mol. Altogether, compounds A1, A2, A3, A4, A5, and A6 showed binding energies lower than amphotericin B, miltefosine, and paromomycin. Similarly, all the compounds had binding energies comparable to the known inhibitors and, therefore, have the potential of attenuating LdSMT.
Compounds with similar activity against a receptor may possess identical chemical features in sterically consistent locations within the pocket of a macromolecule (Held et al., 2011; Du et al., 2016). Most of the compounds including 22,26-azasterol and its derivatives were observed to interact with residues Asp58, Ala88, Arg89, Tyr92, Glu85, Phe93, Phe100, Glu102, Lys198, Pro199, and Gly200, which lined binding pockets 5 and 7 (Supplementary Tables 4 and 6). A similar study involving L. infantum SMT, however, showed the ligands to interact with residues Tyr1, Gly4, Gln5, Gly45, Gly47, Asn67, Asn68, Gln72, and Ile112 within the binding pocket of the receptor (Azam et al., 2014). The nature of interactions included pi–anion, pi–pi stacking, pi–alkyl, pi–sigma, carbon–hydrogen, and hydrogen bonds similar to the other study (Azam et al., 2014). Among all the ligands, only amphotericin B had five hydrogen bonds, with residues Asp31, Phe307, Val308, Arg309, and Leu310 found to line pocket 2. Compounds X1 and X5 formed two hydrogen bonds each with LdSMT. Compound X1 interacted with pockets 5 and 7 and residues Asp172 and Gly200, while X5 interacted with pocket 4 and residues Asn12 and Thr319 via hydrogen bonds (Supplementary Table 6).
In addition, compounds X2, X6, X7, S1, S5, S9, S10, and S12 docked into the binding pocket of the receptor but showed no hydrogen bond interactions with any of the residues. The compound 22,26-azasterol formed two hydrogen bonds with Glu102 and Gly200 as well as hydrophobic interactions with Phe100, Lys198, and Pro199. Moreover, while paromomycin, S2, S3, S4, S5, and S11 formed a hydrogen bond with Arg89, that of S6 and S7 showed a similar interaction with Asp58. Apart from paromomycin, all the other ligands which interacted with Arg89 had low binding energies, implying that it might be critical for binding. The known drugs and inhibitors formed hydrophobic interactions with one or more of the residues Phe100, Met101, Asp104, Asp172, Pro199, Gly200, Thr201, Tyr343, and Ile344, while the selected S-class compounds showed hydrophobic interactions with one or more of the residues Asp58, Ala88, Arg89, Tyr92, Phe93, and Phe264 (Supplementary Table 6).
For the e-LEA3D-generated hits, apart from A2 and A3 which were predicted not to form hydrogen bond interactions with any amino acid residues, the remaining four exhibited hydrogen bonding with at least one of the amino acid residues of LdSMT (Table 2). This notwithstanding, all six formed hydrophobic interactions with the LdSMT protein. The hydrophobic interactions for A1 were with residues Arg89, Tyr92, Ala95, Ala96, and Leu123 (Figure 5 and Table 2), and those for A2 were with Phe84, Glu85, Ala88, Arg89, and Tyr92 (Table 2 and Supplementary Figure 5A). The ligand A3, on the other hand, formed hydrophobic interactions with Ala88, Arg89, Tyr92, Phe93, Ala96, and Phe264 (Table 2 and Supplementary Figure 5B). Furthermore, both A4 (Supplementary Figure 5C) and A6 (Supplementary Figure 5E) formed hydrogen bonding with Cys202 and Asp58, respectively, while A5 (Supplementary Figure 5D) formed two hydrogen bonding interactions with Arg222 and Lys317.
A druggable candidate must be able to reach the site of action in the body at optimized concentrations (Hughes et al., 2011). Predictions of pharmacological and physicochemical parameters are essential because they offer clues as to whether the molecule could reach the active site in the desired concentration and remain there to elicit the required biological response. The physicochemical and pharmacokinetic parameters of the six compounds were assessed using SwissADME (Hughes et al., 2011). Physicochemical profiling assessed both Lipinski’s rule of five (RO5) and Veber’s rule to determine if the chemical compounds with certain pharmacological or biological activity have chemical and physical properties to make them orally active (Lipinski et al., 2001; Veber et al., 2002). A5 and A6 were found to obey both Lipinski’s and Veber’s rules, and A1, A2, A3, and A4 violated one of the two rules (Supplementary Table 7).
Solubility, an important physicochemical property, was predicted to assess the bioavailability and bioactivity of the hit compounds (van den Anker et al., 2018). Many lead compounds have failed to reach clinical trials despite being potent because of low bioactivity attributed to insufficient solubility, making solubility predictions critical in the early stages of drug design (Das et al., 2022). Compounds A4, A5, and A6 were, however, predicted to be soluble compared with A1, A2, and A3 whose solubility can be improved upon by structural modification (Das et al., 2022).
Next, molar refractivity (MR) was assessed to give valuable information on the pharmacokinetics and pharmacodynamics of the compounds. This is governed by different interactions in solution such as drug–solvent, drug–drug, and drug–co-solute interactions (Sawale et al., 2016). The compounds passed for molar refractivity as the predicted values for MR (Supplementary Table 7) were all within the acceptable range of 40 to 130. Since compounds with topological polar surface area (tPSA) not more than 140 Å2 are considered to have good oral bioavailability, all compounds are predicted to be orally active and have good bioavailability.
Furthermore, synthetic accessibility was explored to evaluate the synthetic feasibility of the de-novo hits and has gained importance in the prioritization of compounds in drug design (Ertl and Schuffenhauer, 2009). Many druggable candidates especially de-novo constructed chemical entities are unable to reach clinical trials due to their molecular complexity coupled with difficulty in synthesis (Huang et al., 2010; de Souza Neto et al., 2020). SwissADME (Daina et al., 2017) predicted all the six compounds to possess synthetic accessibility less than 6 implying easy synthesis.
The PAINS of the chemical compounds was investigated to establish whether they will react non-specifically with numerous biological targets (Baell, 2016). A number of PAINS compounds include toxoflavin, isothiazolones, hydroxyphenyl hydrazones, curcumin, phenol-sulfonamides, rhodanines, enones, quinones, and catechols (Baell and Walters, 2014). It is reported that about 5% of US FDA-approved drugs obtained from natural and synthetic drugs still contain PAINS-recognized substructures (Baell and Nissink, 2017). All the compounds were predicted not to contain PAINS substructures.
Pharmacokinetics studies were used to evaluate the time course of absorption, distribution, metabolism, and excretion of the selected hits (Luer and Penzak, 2016). The parameters measured were blood–brain barrier (BBB), gastrointestinal absorption (GI), and permeability glycoprotein (P-gp). Compounds predicted to permeate the BBB have the potential to bind to relevant receptors of the brain to activate signal pathways (Banks, 2009). Four of the compounds (A1, A2, A3, and A5) were predicted not to cross the blood–brain barrier, while A4 and A6 were predicted to cross the BBB to attach to the receptors in the brain to elicit a biological response. GI absorption was probed to investigate whether the hit compounds will be absorbed into the bloodstream after metabolism when orally administered (Löbenberg et al., 2013). All ligands were predicted to have a high GI absorption score except A2 and A3 (Supplementary Table 7). Another pharmacokinetic parameter considered for this study was to explore whether the hits generated were P-gp substrates as compounds predicted to inhibit P-gp result in their increased bioavailability (Lin and Yamazaki, 2003; Prachayasittikul and Prachayasittikul, 2016). The six compounds were screened for their P-glycoprotein binding affinity and they were all predicted to be substrates except A4.
The toxicity profiles of all six compounds were predicted using OSIRIS Property Explorer in Data Warrior (Sander et al., 2015). Toxicity prediction has become very critical in the development of drugs as over 45% of drug candidates fail due to toxicity deficiencies (Van Norman, 2019). Moreover, between 1953 and 2013, as many as 462 medicinal products were withdrawn from the market due to adverse drug reactions (Onakpoya et al., 2016). Toxicity profiling considered for this study was mutagenicity, carcinogenicity, irritancy, and reproductive effects. Of the six compounds, only A1 was predicted to be tumorigenic. The rest neither were mutagenic nor possessed any irritant or reproductive effects (Supplementary Table 8). Among all the compounds under consideration, only X6 was predicted to possess reproductive effects. Overall, the predictions indicate that all molecules may have safe pharmacokinetic and pharmacodynamic profiles except for A1, which would require structural modification to improve its pharmacological properties. For instance, the prediction showed that replacement of the chlorine substituent with a hydrogen atom could render the A1 analog non-tumorigenic.
The open Bayesian machine learning technique, PASS, was used to predict the biological activity of the ligands based on the structure–activity relationship between the selected hits and the training set of compounds of known biological activity (Lagunin et al., 2000; Parasuraman, 2011). A ligand is said to be biologically active and requires experimental validation if the probability of activity (Pa) is greater than the probability of inactivity (Pi) (Basanagouda et al., 2011). Among the six compounds, A3 was predicted to possess antileishmanial properties with a Pa of 0.362 and a Pi of 0.066 and also dermatological properties with a Pa of 0.32 and a Pi of 0.091. Compounds A5 and A6 were also predicted as dermatologic, with Pa values of 0.205 and 0.249 and Pi values of 0.162 and 0.120, respectively. The results may suggest that A3, A5, and A6 might be beneficial in treating post-kala-azar leishmaniasis (Momeni et al., 2003; Ali et al., 2012).
Compounds A1 (Pa of 0.571 and Pi of 0.111), A3 (Pa of 0.774 and Pi of 0.026), and A6 (Pa of 0.615 and Pi of 0.079) were also predicted as mucomembrane protectors. A recent in-vitro study revealed that butein acting as a mucomembrane protector on human cells increased immunity against pathogenic infections (Satari et al., 2021). This may suggest that the compounds have the potential of boosting the immune system to prevent disease exacerbation. Compound A1 was predicted as an indolepyruvate C-methyltransferase inhibitor with a Pa of 0.226 and a Pi of 0.074. Compound A3 was also predicted to be phenol O-methyltransferase, histamine N-methyltransferase, and acetylserotonin O-methyltransferase inhibitors with Pa values greater than Pi.
The inhibitory constant (Ki) and other parameters such as LE, LE_Scale, FQ, binding efficiency index (BEI), surface efficiency index (SEI), and LELP were calculated (Supplementary Table 9).
Ki is the concentration required to produce half-maximum inhibition and, hence, an indicator of the potency of a ligand (Fisar et al., 2010). Computation of Ki for the protein–ligand complexes was obtained using Equation (1), where R is the molar gas constant (1.987 × 10−3 kcal/K mol−1) and T (298.15 K) is the absolute temperature (Du et al., 2016).
The Ki predicted for the ligands was low (Supplementary Table 9), hence has the capacity to be lead-like with possible high potency (Reynolds and Reynolds, 2017).
LE is a value that expresses the binding energy of a compound normalized by the compound’s size and expressed by the number of heavy (non-hydrogen) atoms (Hopkins et al., 2004). This property is important to consider in screening for hits as larger compounds tend to show greater binding energy due to a large number of interactions but may not necessarily be the most efficient binder (Hevener et al., 2018). The LE was computed using Equation (2), where BE is the binding energy and NHA is the number of heavy atoms (Abad-Zapatero et al., 2010).
Interestingly, all the ligands except A1, A3, and A5 were within the optimal range (LE < 0.3 kcal/mol/HA) (Schultes et al., 2010) for the ligand efficiency of lead-like molecules.
Results from the computation of LE being size-dependent may not be a true reflection of the binding energy of the compound, and therefore, ligand efficiency scaling (LE_Scale), a size-independent parameter that compares ligands with the help of an exponential function to the maximal LE values, is required (Reynolds et al., 2007). LE_Scaling was computed using Equation (3), where NHA is the number of heavy atoms (Reynolds et al., 2007).
Potential lead-like molecules are suggested to have an LE_Scale lower than 0.3 (Islam and Pillay, 2020). The LE_Scale values of all six molecules were, however, predicted to be in the range of 0.3 to 0.5 with A5 having the highest LE_Scale of 0.455.
FQ is another size-independent parameter that determines the optimal ligand binding within the receptor active site (Schultes et al., 2010), and is computed using Equation (4) (Schultes et al., 2010).
FQ scores range from 0 to 1 with values close to 1 signifying an optimal ligand binding (Schultes et al., 2010). All the compounds were predicted to have an FQ score above 0.7 except A6 (0.695), implying a stronger ligand binding. With the predicted FQ being close to 1, it suggests an optimal ligand binding.
The LELP, on the other hand, assesses the binding energy of a compound in relation to the compound’s lipophilicity (Hevener et al., 2018). LELP is a parameter used in drug design and development to evaluate the quality of compounds by linking potency and lipophilicity in an attempt to estimate drug-likeness (Edwards and Price, 2010). Equation (5), where logP is the lipophilicity, was used in calculating the LELP of the compounds (Schultes et al., 2010).
The recommended range for promising molecules for LELP was >3 (Schultes et al., 2010). All the analogs had LELP above 4 (Supplementary Table 9) suggesting an optimized affinity with respect to lipophilicity.
BEI and SEI are two alternative metrics that are also used to compare the activity of molecules according to size and area (Schultes et al., 2010). Binding efficiency index is defined by BEI = p(IC50)/MW, where MW is the molecular weight (Schultes et al., 2010). The relation in Equation (6) was used in computing the BEI of the ligands (Abad-Zapatero et al., 2010).
SEI, on the other hand, is defined by SEI = p(IC50)/PSA, where PSA is the polar surface area of the ligand (Abad-Zapatero et al., 2010). Calculation of SEI was done using Equation (7) (Abad-Zapatero et al., 2010).
By rule of thumb, potential inhibitors must approximately have the same BEI and SEI values (Abad-Zapatero, 2007). Compounds A1, A4, and A6 were predicted to have BEI equal to SEI. Altogether, the parameters predicted for all the compounds were mostly within the acceptable range prompting the need for experimental analysis.
MD simulation is a computer simulation method for analyzing the physical movements of atoms and molecules (Hollingsworth and Dror, 2018). Of great concern for the MD simulation is how a biomolecular system responds to some perturbation within a short period of time (Karplus and Kuriyan, 2005; Hollingsworth and Dror, 2018). Due to its usefulness, a number of drug design studies have explored molecular dynamics simulation to analyze and validate the binding poses, stability of the complexes, and binding affinity of selected hits within the binding pocket of the receptor (Agyapong et al., 2021; Gupta et al., 2021; Pathania et al., 2021). To check the relative stability of each complex using a 100-ns time span MD simulation, parameters such as RMSD, root mean square fluctuation (RMSF), and radius of gyration (Rg) were computed.
The RMSD trajectory was used to evaluate the stability of the protein–ligand complexes, and the plot shows the system was equilibrated in the range of 0 to 0.7 nm. Averagely, the unbound LdSMT was observed to rise steadily from 0.28 nm until about 0.42 nm for 20 ns before stabilizing thereafter (Figure 6). Comparatively, there is no significant fluctuation observed in any of the protein–ligand complexes except for the LdSMT–A3 complex, which rose from 0.28 to 0.62 nm during 10 ns and then stabilized for the next 10 ns and dropped to about 0.5 nm. It then rose slightly to 0.56 nm and then stabilized after 40 ns. Across the board, the LdSMT–A2 complex had high rigidity and the frames of each complex depict a tight structural packing across the whole protein influencing the low RSMD values of LdSMT.
Figure 6 Root mean square deviation (RMSD) plot of 100 ns molecular dynamics (MD) simulations of the LdSMT–ligand complexes using GROMACS.
The radius of the gyration plot, a graph of Rg against simulation time, is used to analyze the compactness and folding of the unbound protein and complexes during the molecular dynamics simulations. The Rg graph obtained showed that all the complexes had low Rg values implying that the ligands formed a stable and compact complex (Liao et al., 2014; Pandey et al., 2020; Sinha and Wang, 2020). All the complexes except for A3 had a steady decline in Rg to about 40 ns before stabilizing afterward. Rg values for both unbound protein and the complexes were between 1.925 and 2.75 nm (Supplementary Figure 6A). The Rg trajectory of each complex demonstrated that each ligand forms a stable bond with the LdSMT.
The RMSF was explored to investigate which amino acids within the binding site of the receptor interacted with the ligand resulting in the stability of the protein–ligand complex (Farmer et al., 2017). The RMSF plots showed that all the hit compounds caused fluctuations in similar positions of the protein target. The plots revealed that the amino acid residues between 15 and 100, 200 and 250, and 280 and 320 (Supplementary Figure 6B) fluctuated for all complexes and are predicted to be involved in the stability of the complexes (Dong et al., 2018). However, the highest fluctuation was observed around regions 200–280 for A2 and A3 implying it could be involved in ligand binding.
The free energy of binding of all the protein–ligand complexes was calculated using the MM-PBSA continuum solvation method (Kumari et al., 2014). The MM-PBSA was employed to find the free energies of the bound complexes. Ligand A1, which was predicted to have the least binding energy from AutoDock Vina, was shown to have the lowest free binding energy of −282.550 kJ/mol (Table 3) to the LdSMT. Among the three top compounds, only A3 exhibited free binding energy greater than that of the reference candidate, 22,26-azasterol (Table 3). The dominating interaction per contribution to the free energy was electrostatic forces of attraction ranging from −333 to −11 kJ/mol followed by the van der Waals interactions.
Calculation of the energy contribution of each amino acid residue via per-residue energy analysis was performed using MM-PBSA (Congreve and Marshall, 2010; Grinter and Zou, 2014). It has previously been suggested that for a residue to contribute to the binding, the threshold must be >5 or <5 kJ/mol (Kwofie et al., 2019). Based on that, a detailed analysis of each complex was done (Figure 7 and Supplementary Figures 6A–C), and for all the complexes, several binding site residues contributed favorable energies for ligand binding. The Tyr92 and Ala96 contributed to strong binding via pi–pi stacked, pi–pi T-shaped, pi–alkyl, and van der Waals interactions, while Asp58 through its hydrogen bonding strengthened the affinity for ligands bound in pocket 5.
Figure 7 Molecular mechanics Poisson–Boltzmann surface area (MM-PBSA) plot showing the binding free energy contribution per residue of the LdSMT–A1 complex.
Four (A1, A2, A3, and A6) out of the six compounds possessed benzo[b]azepine moiety as a replacement for the steroidal core in the 22,26-azasterol. This same moiety is present in paullone and its derivatives as well as BNZ-1 (Figure 8), which are known to suppress growth in Leishmania parasites with IC50 values of 47 nM and 100 μM, respectively (Clark et al., 2007; Dao Duong Thi et al., 2009), implying the possible antileishmanial potentials of the proposed hits. Moreover, the chemical structural similarity search of compounds A1, A2, A3, and A6 via DrugBank (Wishart et al., 2018) revealed a variable similarity to antipsychotic and antidepressant drugs (Kaur, 2013; Mendonca Junior et al., 2015). For instance, compounds A1, A2, A3, and A6 showed similarity scores above 0.50 to vabicaserin, sertraline, and indatraline (Richardson et al., 2009; Wishart et al., 2018; Lima et al., 2018). In addition, the four compounds showed similarity scores of around 0.55, which are close to those of daledalin, zanapezil, clocapramine, imipramine, and dimethacrine (Figure 8) (Mukherjee et al., 2012; da Silva Rodrigues et al., 2019). Interestingly, these drugs have been explored for their antileishmanial potentials causing Leishmania parasites to undergo mitochondrion depolarization in addition to inhibiting trypanothione reductase, thereby inducing strong oxidative stress in the parasite (Kaur, 2013; Andrade-Neto et al., 2016; da Silva Rodrigues et al., 2019). The similarity scores and the antileishmanial properties of these antidepressant and antipsychotic drugs warrant the testing of the compounds to assess their antileishmanial propensity.
Furthermore, compound A4 devoid of the benzo[H]azepine also showed a chemical structural similarity score of 0.564 to CP-39,332, a serotonin–norepinephrine reuptake inhibitor. The successes emanating from other serotonin inhibitors for leishmaniasis treatment suggest A4 as a potential antileishmanial compound. In addition, A5 showed a similarity score of 0.538 to cefradine, a broad-spectrum antibiotic for the treatment of skin, chest, throat, and ear infections (Wishart et al., 2018). A recent study has revealed that patients exposed to antibiotics had a greater healing rate (Barakat et al., 2017), suggesting A5 to be explored as an antileishmanial agent. In lieu of the aforementioned, the potential leads A1, A2, A3, A4, A5, and A6 with diverse structural similarities with the antipsychotic and antibiotic agents are also proposed as potential antileishmanial agents via inhibition of sterol methyltransferase and are worthy of further experimental evaluation to assess their biological efficacy.
The study modeled a reasonable structure of LdSMT with good quality parameters, which has been made available to augment the structure-based drug design. In addition, small non-steroidal molecules with negligible toxicity with the potential to suppress LdSMT were identified and could be harmonized into non-commercial databases for the design of new biotherapeutic compounds. Furthermore, the de-novo design was employed in making available chemical structures of compounds which can be synthesized to ascertain their antileishmanial potency.
The renewed interest in polypharmacology drugs with the added advantage of overcoming drug resistance necessitates the investigation of these compounds against plausible targets involved in the ergosterol biosynthetic pathway of Leishmania parasites. Furthermore, coordinating these ligands to transition metals to find multimodality metallodrugs with the potential of inhibiting two or more enzymes in the ergosterol pathway may present a possible biotherapeutic route for leishmaniasis.
In-silico approaches were used to predict putative inhibitors targeting LdSMT by elucidating the 3D structure of LdSMT via Modeller followed by subjection of 22,26-azasterol to scaffold hopping and de-novo drug design. In all, six potential inhibitors labeled A1, A2, A3, A4, A5, and A6 were generated via de-novo design with binding affinities of −8.4, −7.5, −7.2, −7.0, −7.0, and −7.0 kcal/mol, respectively. The compounds A1 and A2 demonstrated comparable binding affinity to that of 22,26-azasterol (−7.6 kcal/mol), the main inhibitor of LdSMT. The study identified Tyr92 to be essential for ligand binding in the receptor binding pocket, and this was corroborated by MD simulation and MM-PBSA calculations. The physicochemical and pharmacological profiling showed that the compounds are drug-like and predicted as non-toxic. The predicted ligand quality metrics including Ki, LE, LE_Scale, FQ, LELP, BEI, and SEI were all within the acceptable range. These findings suggest that the compounds possess antileishmanial potential and warrant experimental corroboration.
The original contributions presented in the study are included in the article/Supplementary Material. Further inquiries can be directed to the corresponding author.
POS, SKK, and RA conceptualized the project. PS designed the project and predominantly undertook the computational analysis with inputs from SKK, RA, EB, WAM, and MDW. POS wrote the first draft of the manuscript. All the authors read, edited, and approved the manuscript before submission.
The authors declare that the research was conducted in the absence of any commercial or financial relationships that could be construed as a potential conflict of interest.
All claims expressed in this article are solely those of the authors and do not necessarily represent those of their affiliated organizations, or those of the publisher, the editors and the reviewers. Any product that may be evaluated in this article, or claim that may be made by its manufacturer, is not guaranteed or endorsed by the publisher.
The authors are grateful to the West African Centre for Cell Biology of Infectious Pathogens (WACCBIP), University of Ghana for the use of Zuputo, a DELL high-performance computing system, for this study. POS is grateful to the Ghana National Petroleum Corporation (GNPC) for supporting the postgraduate studies.
The Supplementary Material for this article can be found online at: https://www.frontiersin.org/articles/10.3389/fcimb.2022.859981/full#supplementary-material
Abad-Zapatero, C. (2007). Ligand Efficiency Indices for Effective Drug Discovery. Expert Opin. Drug Discov. 2, 469–488. doi: 10.1517/17460441.2.4.469
Abad-Zapatero, C., Perišic, O., Wass, J., Bento, A. P., Overington, J., Al-Lazikani, B., et al. (2010). Ligand Efficiency Indices for an Effective Mapping of Chemico-Biological Space: The Concept of an Atlas-Like Representation. Drug Discov. Today 15, 804–811. doi: 10.1016/j.drudis.2010.08.004
Abraham, M. J., Murtola, T., Schulz, R., Páll, S., Smith, J. C., Hess, B., et al. (2015). Gromacs: High Performance Molecular Simulations Through Multi-Level Parallelism From Laptops to Supercomputers. SoftwareX 1, 19–25. doi: 10.1016/J.SOFTX.2015.06.001
Agyapong, O., Asiedu, S. O., Kwofie, S. K., Miller, W. A., Parry, C. S., Sowah, R. A., et al. (2021). Molecular Modelling and De Novo Fragment-Based Design of Potential Inhibitors of Beta-Tubulin Gene of Necator Americanus From Natural Products. Inf. Med. Unlock. 26, 100734/1–100734/20. doi: 10.1016/j.imu.2021.100734
Ali, N., Ali, N. M., Fariba, J., Elaheh, H. (2012). The Efficacy of 5% Trichloroacetic Acid Cream in the Treatment of Cutaneous Leishmaniasis Lesions. J. Dermatolog. Treat. 23, 136–139. doi: 10.3109/09546634.2010.500322
Alkarkhi, A. F. M., Alqaraghuli, W. A. A. (2020). R Statistical Software. Appl. Stat. Environ. Sci. R. 1, 1–17. doi: 10.1016/b978-0-12-818622-0.00002-2
Anderson, R. J., Weng, Z., Campbell, R. K., Jiang, X. (2005). Main-Chain Conformational Tendencies of Amino Acids. Proteins Struct. Funct. Genet. 60, 679–689. doi: 10.1002/PROT.20530
Andrade-Neto, V. V., Pereira, T. M., Canto-Cavalheiro, M., Torres-Santos, E. C. (2016). Imipramine Alters the Sterol Profile in Leishmania Amazonensis and Increases Its Sensitivity to Miconazole. Parasitol. Vectors 9, 1–8. doi: 10.1186/S13071-016-1467-8
Azam, S. S., Abro, A., Raza, S., Saroosh, A. (2014). Structure and Dynamics Studies of Sterol 24-C-Methyltransferase With Mechanism Based Inactivators for the Disruption of Ergosterol Biosynthesis. Mol. Biol. Rep. 41, 4279–4293. doi: 10.1007/s11033-014-3299-y
Baell, J. B. (2016). Feeling Nature’s PAINS: Natural Products, Natural Product Drugs, and Pan Assay Interference Compounds (PAINS). J. Nat. Prod. 79, 616–628. doi: 10.1021/acs.jnatprod.5b00947
Baell, J. B., Nissink, J. W. M. (2017). Seven Year Itch: Pan-Assay Interference Compounds (PAINS) in 2017—Utility and Limitations. ACS Chem. Biol. 13, 36–44. doi: 10.1021/ACSCHEMBIO.7B00903
Baell, J., Walters, M. A. (2014). Chemistry: Chemical Con Artists Foil Drug Discovery. Nature 513, 481–483. doi: 10.1038/513481a
Banegas-Luna, A. J., Cerón-Carrasco, J. P., Puertas-Martín, S., Pérez-Sánchez, H. (2019). BRUSELAS: HPC Generic and Customizable Software Architecture for 3D Ligand-Based Virtual Screening of Large Molecular Databases. J. Chem. Inf. Model. 59, 2805–2817. doi: 10.1021/ACS.JCIM.9B00279
Banks, W. A. (2009). Characteristics of Compounds That Cross the Blood-Brain Barrier. BMC Neurol. 9, S3/1–S3/1. doi: 10.1186/1471-2377-9-S1-S3
Barakat, R., Aronson, N., Olsen, C. (2017). Microbiome Manipulation: Antibiotic Effects on Cutaneous Leishmaniasis Presentation and Healing. Open Forum Infect. Dis. 4, S122/1–S122/2. doi: 10.1093/OFID/OFX163.157
Basanagouda, M., Jadhav, V. B., Kulkarni, M. V., Nagendra Rao, R. (2011). Computer Aided Prediction of Biological Activity Spectra: Study of Correlation Between Predicted and Observed Activities for Coumarin-4-Acetic Acids. Indian J. Pharm. Sci. 73, 88–92. doi: 10.4103/0250-474X.89764
Behera, S. K., Sabarinath, T., Mishra, P. K. K., Deneke, Y., Kumar, A., Chandrasekar, S., et al. (2021). Immunoinformatic Study of Recombinant Liga/Bcon1-5 Antigen and Evaluation of Its Diagnostic Potential in Primary and Secondary Binding Tests for Serodiagnosis of Porcine Leptospirosis. Pathogens 10, 1–20. doi: 10.3390/pathogens10091082
Benet, L. Z., Hosey, C. M., Ursu, O., Oprea, T. I. (2016). BDDCS, the Rule of 5 and Drugability. Adv. Drug Deliv. Rev. 101, 89–98. doi: 10.1016/J.ADDR.2016.05.007
Benkert, P., Biasini, M., Schwede, T. (2011). Toward the Estimation of the Absolute Quality of Individual Protein Structure Models. Bioinformatics 27, 343–350. doi: 10.1093/BIOINFORMATICS/BTQ662
Berman, H. M., Westbrook, J., Feng, Z., Gilliland, G., Bhat, T. N., Weissig, H., et al. (2000). The Protein Data Bank. Nucleic Acids Res. 28, 235–242. doi: 10.1093/NAR/28.1.235
Boratyn, G. M., Camacho, C., Cooper, P. S., Coulouris, G., Fong, A., Ma, N., et al. (2013). BLAST: A More Efficient Report With Usability Improvements. Nucleic Acids Res. 41, 29–33. doi: 10.1093/NAR/GKT282
Broni, E., Kwofie, S. K., Asiedu, S. O., Miller, W. A., Wilson, M. D. (2021). A Molecular Modeling Approach to Identify Potential Antileishmanial Compounds Against the Cell Division Cycle (Cdc)-2-Related Kinase 12 (CRK12) Receptor of Leishmania Donovani. Biomolecules 11, 458/1–458/32. doi: 10.3390/biom11030458
Burley, S. K., Berman, H. M., Kleywegt, G. J., Markley, J. L., Nakamura, H., Velankar, S. (2017). Protein Data Bank (PDB): The Single Global Macromolecular Structure Archive. Methods Mol. Biol. 1607, 627–641. doi: 10.1007/978-1-4939-7000-1_26
Chang, M. W., Lindstrom, W., Olson, A. J., Belew, R. K. (2007). Analysis of HIV Wild-Type and Mutant Structures via in Silico Docking Against Diverse Ligand Libraries. J. Chem. Inf. Model. 47, 1258–1262. doi: 10.1021/CI700044S
Chanquia, S. N., Larregui, F., Puente, V., Labriola, C., Lombardo, E., García Liñares, G. (2019). Synthesis and Biological Evaluation of New Quinoline Derivatives as Antileishmanial and Antitrypanosomal Agents. Bioorg. Chem. 83, 526–534. doi: 10.1016/J.BIOORG.2018.10.053
Clark, R. L., Carter, K. C., Mullen, A. B., Coxon, G. D., Owusu-Dapaah, G., McFarlane, E., et al. (2007). Identification of the Benzodiazepines as a New Class of Antileishmanial Agent. Bioorg. Med. Chem. Lett. 17, 624–627. doi: 10.1016/J.BMCL.2006.11.004
Colovos, C., Yeates, T. O. (1993). Verification of Protein Structures: Patterns of Nonbonded Atomic Interactions. Protein Sci. 2, 1511–1519. doi: 10.1002/pro.5560020916
Congreve, M., Marshall, F. (2010). The Impact of GPCR Structures on Pharmacology and Structure-Based Drug Design. Br. J. Pharmacol. 159, 986–996. doi: 10.1111/J.1476-5381.2009.00476.X
Crentsil, J. A., Yamthe, L. R. T., Anibea, B. Z., Broni, E., Kwofie, S. K., Tetteh, J. K. A., et al. (2020). Leishmanicidal Potential of Hardwickiic Acid Isolated From Croton Sylvaticus. Front. Pharmacol. 11, 753. doi: 10.3389/fphar.2020.00753
Dahiya, R., Mohammad, T., Gupta, P., Haque, A., Alajmi, M. F., Hussain, A., et al. (2019). Molecular Interaction Studies on Ellagic Acid for Its Anticancer Potential Targeting Pyruvate Dehydrogenase Kinase 3. RSC. Adv. 9, 23302–23315. doi: 10.1039/C9RA02864A
Daina, A., Michielin, O., Zoete, V. (2017). SwissADME: A Free Web Tool to Evaluate Pharmacokinetics, Drug-Likeness and Medicinal Chemistry Friendliness of Small Molecules. Sci. Rep. 7, 42717/1–42717/13. doi: 10.1038/SREP42717
Dao Duong Thi, M., Helen Grant, M., Mullen, A. B., Tettey, J. N. A., MacKay, S. P., Clark, R. L. (2009). Metabolism of Two New Benzodiazepine-Type Anti-Leishmanial Agents in Rat Hepatocytes and Hepatic Microsomes and Their Interaction With Glutathione in Macrophages. J. Pharm. Pharmacol. 61, 399–406. doi: 10.1211/JPP/61.03.0017
da Silva Rodrigues, J. H., Miranda, N., Volpato, H., Ueda-Nakamura, T., Nakamura, C. V. (2019). The Antidepressant Clomipramine Induces Programmed Cell Death in Leishmania Amazonensis Through a Mitochondrial Pathway. Parasitol. Res. 118, 977–989. doi: 10.1007/s00436-018-06200-x
Das, B., TK Baidya, A., Mathew, A. T., Kumar Yadav, A., Kumar, R. (2022). Structural Modification Aimed for Improving Solubility of Lead Compounds in Early Phase Drug Discovery. Bioorg. Med. Chem. 56, 116614/1–116614/83. doi: 10.1016/j.bmc.2022.116614
Davies, M., Nowotka, M., Papadatos, G., Dedman, N., Gaulton, A., Atkinson, F., et al. (2015). ChEMBL Web Services: Streamlining Access to Drug Discovery Data and Utilities. Nucleic Acids Res. 43, 612–620. doi: 10.1093/NAR/GKV352
de Souza Neto, L. R., Moreira-Filho, J. T., Neves, B. J., Maidana, R. L. B. R., Guimarães, A. C. R., Furnham, N., et al. (2020). In Silico Strategies to Support Fragment-To-Lead Optimization in Drug Discovery. Front. Chem. 8, 93. doi: 10.3389/FCHEM.2020.00093
Dong, Y. W., Liao, M. L., Meng, X. L., Somero, G. N. (2018). Structural Flexibility and Protein Adaptation to Temperature: Molecular Dynamics Analysis of Malate Dehydrogenases of Marine Molluscs. Proc. Natl. Acad. Sci. U. S. A. 115, 1274–1279. doi: 10.1073/PNAS.1718910115
Douguet, D. (2010). E-LEA3D: A Computational-Aided Drug Design Web Server. Nucleic Acids Res. 38, 615–621. doi: 10.1093/NAR/GKQ322
Du, X., Li, Y., Xia, Y. L., Ai, S. M., Liang, J., Sang, P., et al. (2016). Insights Into Protein–Ligand Interactions: Mechanisms, Models, and Methods. Int. J. Mol. Sci. 17, 144/1–144/34. doi: 10.3390/IJMS17020144
Dundas, J., Ouyang, Z., Tseng, J., Binkowski, A., Turpaz, Y., Liang, J. (2006). CASTp: Computed Atlas of Surface Topography of Proteins With Structural and Topographical Mapping of Functionally Annotated Residues. Nucleic Acids Res. 34, 116–118. doi: 10.1093/nar/gkl282
Edwards, M. P., Price, D. A. (2010). Role of Physicochemical Properties and Ligand Lipophilicity Efficiency in Addressing Drug Safety Risks. Annu. Rep. Med. Chem. 45, 380–391. doi: 10.1016/S0065-7743(10)45023-X
Ertl, P., Schuffenhauer, A. (2009). Estimation of Synthetic Accessibility Score of Drug-Like Molecules Based on Molecular Complexity and Fragment Contributions. J. Cheminform. 1, 1–11. doi: 10.1186/1758-2946-1-8/TABLES/1
Eswar, N., Eramian, D., Webb, B., Shen, M. Y., Sali, A. (2008). Protein Structure Modeling With MODELLER. Methods Mol. Biol. 426, 145–159. doi: 10.1007/978-1-60327-058-8_8
Fage, C. D., Isiorho, E. A., Liu, Y., Wagner, D. T., Liu, H., Keatinge-Clay, A. T. (2015). The Structure of SpnF, a Standalone Enzyme That Catalyzes [4 + 2] Cycloaddition. Nat. Chem. Biol. 11, 256–258. doi: 10.1038/nchembio.1768
Farmer, J., Kanwal, F., Nikulsin, N., Tsilimigras, M. C. B., Jacobs, D. J. (2017). Statistical Measures to Quantify Similarity Between Molecular Dynamics Simulation Trajectories. Entropy 19, 646/1–646/17. doi: 10.3390/E19120646
Fisar, Z., Hroudová, J., Raboch, J. (2010). Inhibition of Monoamine Oxidase Activity by Antidepressants and Mood Stabilizers Alzheimer´sAlzheimer´s Disease View Project Mitochondrial Dysfunctions in Bipolar Affective Disorder View Project. Neuroendocrinol. Lett. 31, 645–656.
Fiser, A., Šali, A. (2003). Modeller: Generation and Refinement of Homology-Based Protein Structure Models. Methods Enzymol. 374, 461–491. doi: 10.1016/S0076-6879(03)74020-8
Ghorbani, M., Farhoudi, R. (2018). Leishmaniasis in Humans: Drug or Vaccine Therapy? Drug Des. Devel. Ther. 12, 25–40. doi: 10.2147/DDDT.S146521
Gil, C., Martinez, A. (2021). Is Drug Repurposing Really the Future of Drug Discovery or Is New Innovation Truly the Way Forward? Taylor. Fr. 16, 829–831. doi: 10.1080/17460441.2021.1912733
Goto, Y., Bhatia, A., Raman, V. S., Vidal, S. E. Z., Bertholet, S., Coler, R. N., et al. (2009). Leishmania Infantum Sterol 24-C-Methyltransferase Formulated With MPL-SE Induces Cross-Protection Against L. Major Infection. Vaccine 27, 2884–2890. doi: 10.1016/J.VACCINE.2009.02.079
Goto, Y., Bogatzki, L. Y., Bertholet, S., Coler, R. N., Reed, S. G. (2007). Protective Immunization Against Visceral Leishmaniasis Using Leishmania Sterol 24-C-Methyltransferase Formulated in Adjuvant. Vaccine 25, 7450–7458. doi: 10.1016/j.vaccine.2007.08.001
Grinter, S. Z., Zou, X. (2014). Challenges, Applications, and Recent Advances of Protein-Ligand Docking in Structure-Based Drug Design. Molecules 19, 10150–10176. doi: 10.3390/MOLECULES190710150
Gros, L., Castillo-Acosta, V. M., Jiménez, C. J., Sealey-Cardona, M., Vargas, S., Estévez, A. M., et al. (2006). New Azasterols Against Trypanosoma Brucei: Role of 24-Sterol Methyltransferase in Inhibitor Action. Antimicrob. Agents Chemother. 50, 2595–2601. doi: 10.1128/AAC.01508-05
Gupta, Y., Maciorowski, D., Zak, S. E., Jones, K. A., Kathayat, R. S., Azizi, S. A., et al. (2021). Bisindolylmaleimide IX: A Novel Anti-SARS-CoV2 Agent Targeting Viral Main Protease 3clpro Demonstrated by Virtual Screening Pipeline and in-Vitro Validation Assays. Methods 195, 57–71. doi: 10.1016/j.ymeth.2021.01.003
Haddad, Y., Adam, V., Heger, Z. (2020). Ten Quick Tips for Homology Modeling of High-Resolution Protein 3D Structures. PloS Comput. Biol. 16, e1007449/1–e1007449/19. doi: 10.1371/journal.pcbi.1007449
Held, M., Metzner, P., Prinz, J. H., Noé, F. (2011). Mechanisms of Protein-Ligand Association and Its Modulation by Protein Mutations. Biophys. J. 100, 701–710. doi: 10.1016/J.BPJ.2010.12.3699
Hernández-Bojorge, S. E., Blass-Alfaro, G. G., Rickloff, M. A., Gómez-Guerrero, M. J., Izurieta, R. (2020). Epidemiology of Cutaneous and Mucocutaneous Leishmaniasis in Nicaragua. Parasite. Epidemiol. Contr. 11, e00192/1–e00192/11. doi: 10.1016/j.parepi.2020.e00192
Hevener, K. E., Pesavento, R., Ren, J., Lee, H., Ratia, K., Johnson, M. E. (2018). Hit-To-Lead: Hit Validation and Assessment. Methods Enzymol. 610, 265–309. doi: 10.1016/bs.mie.2018.09.022
Hollingsworth, S. A., Dror, R. O. (2018). Molecular Dynamics Simulation for All. Neuron 99, 1129–1143. doi: 10.1016/J.NEURON.2018.08.011
Hopkins, A. L., Groom, C. R., Alex, A. (2004). Ligand Efficiency: A Useful Metric for Lead Selection. Drug Discov. Today 9, 430–431. doi: 10.1016/S1359-6446(04)03069-7
Hopkins, A. L., Keserü, G. M., Leeson, P. D., Rees, D. C., Reynolds, C. H. (2014). The Role of Ligand Efficiency Metrics in Drug Discovery. Nat. Rev. Drug Discov. 13, 105–121. doi: 10.1038/NRD4163
Huang, Q., Li, L. L., Yang, S. Y. (2010). PhDD: A New Pharmacophore-Based De Novo Design Method of Drug-Like Molecules Combined With Assessment of Synthetic Accessibility. J. Mol. Graph. Model. 28, 775–787. doi: 10.1016/J.JMGM.2010.02.002
Hughes, J. P., Rees, S. S., Kalindjian, S. B., Philpott, K. L. (2011). Principles of Early Drug Discovery. Br. J. Pharmacol. 162, 1239–1249. doi: 10.1111/j.1476-5381.2010.01127.x
Huo, L., Zhao, X., Acedo, J. Z., Estrada, P., Nair, S. K., Donk, W. A. (2020). Characterization of a Dehydratase and Methyltransferase in the Biosynthesis of Ribosomally Synthesized and Post-Translationally Modified Peptides in Lachnospiraceae. ChemBioChem 21, 190–199. doi: 10.1002/cbic.201900483
Hu, Y., Stumpfe, D., Bajorath, J. (2017). Recent Advances in Scaffold Hopping. J. Med. Chem. 60, 1238–1246. doi: 10.1021/ACS.JMEDCHEM.6B01437
Ikeogu, N. M., Akaluka, G. N., Edechi, C. A., Salako, E. S., Onyilagha, C., Barazandeh, A. F., et al. (2020). Leishmania Immunity: Advancing Immunotherapy and Vaccine Development. Microorganisms 8, 1201/1–1201/21. doi: 10.3390/MICROORGANISMS8081201
Islam, M. A., Pillay, T. S. (2020). Identification of Promising Anti-DNA Gyrase Antibacterial Compounds Using De Novo Design, Molecular Docking and Molecular Dynamics Studies. J. Biomol. Struct. Dyn. 38, 1798–1809. doi: 10.1080/07391102.2019.1617785
Jacquemard, C., Kellenberger, E. (2019). A Bright Future for Fragment-Based Drug Discovery: What Does It Hold? Expert Opin. Drug Discov. 14, 413–416. doi: 10.1080/17460441.2019.1583643
Jeon, B., Ruszczycky, M. W., Russell, W. K., Lin, G.-M., Kim, N., Choi, S., et al. (2017). Investigation of the Mechanism of the SpnF-Catalyzed [4+2]-Cycloaddition Reaction in the Biosynthesis of Spinosyn A. Proc. Natl. Acad. Sci. 114, 10408–10413. doi: 10.1073/pnas.1710496114
Karplus, M., Kuriyan, J. (2005). Molecular Dynamics and Protein Function. Proc. Natl. Acad. Sci. 102, 6679–6685. doi: 10.1073/PNAS.0408930102
Kaur, N. (2013). An Insight Into Medicinal and Biological Significance of Privileged Scaffold: 1,4-Benzodiazepine. Int. J. Pharma. Bio Sci. 4, 318–337.
Kidane, M. E., Vanderloop, B. H., Zhou, W., Thomas, C. D., Ramos, E., Singha, U., et al. (2017). Sterol Methyltransferase a Target for Anti-Amoeba Therapy: Towards Transition State Analog and Suicide Substrate Drug Design. J. Lipid Res. 58, 2310–2323. doi: 10.1194/JLR.M079418
Kim, S., Chen, J., Cheng, T., Gindulyte, A., He, J., He, S., et al. (2021). PubChem in 2021: New Data Content and Improved Web Interfaces. Nucleic Acids Res. 49, D1388–D1395. doi: 10.1093/NAR/GKAA971
Kranthi, K. R., Mathi, P., Prasad, M. V. V. V., Botlagunta, M., Ravi, M., Ramachandran, D. (2018). De Novo Design of Selective Sortase-A Inhibitors: Synthesis, Structural and In Vitro Characterization. Chem. Data Collect. 15, 126–133. doi: 10.1016/j.cdc.2018.04.007
Kshirsagar, U. A. (2015). Recent Developments in the Chemistry of Quinazolinone Alkaloids. Org. Biomol. Chem. 13, 9336–9352. doi: 10.1039/x0xx00000x
Kumari, R., Kumar, R., Lynn, A. (2014). G_Mmpbsa —A GROMACS Tool for High-Throughput MM-PBSA Calculations. J. Chem. Inf. Model. 54, 1951–1962. doi: 10.1021/ci500020m
Kwofie, S. K., Broni, E., Dankwa, B., Enninful, K. S., Kwarko, G. B., Darko, L., et al. (2020). Outwitting an Old Neglected Nemesis: A Review on Leveraging Integrated Data-Driven Approaches to Aid in Unraveling of Leishmanicides of Therapeutic Potential. Curr. Top. Med. Chem. 20, 349–366. doi: 10.2174/1568026620666200128160454
Kwofie, S., Broni, E., Yunus, F., Nsoh, J., Adoboe, D., Miller, W., et al. (2021). Molecular Docking Simulation Studies Identifies Potential Natural Product Derived-Antiwolbachial Compounds as Filaricides Against Onchocerciasis. Biomedicines 9, 1682/1–1682/33. doi: 10.3390/biomedicines9111682
Kwofie, S., Dankwa, B., Enninful, K., Adobor, C., Broni, E., Ntiamoah, A., et al. (2019). Molecular Docking and Dynamics Simulation Studies Predict Munc18b as a Target of Mycolactone: A Plausible Mechanism for Granule Exocytosis Impairment in Buruli Ulcer Pathogenesis. Toxins. (Basel). 11, 181/1–181/16. doi: 10.3390/toxins11030181
Lagunin, A., Stepanchikova, A., Filimonov, D., Poroikov, V. (2000). PASS: Prediction of Activity Spectra for Biologically Active Substances. Bioinformatics 16, 747–748. doi: 10.1093/BIOINFORMATICS/16.8.747
Larsson, P., Wallner, B., Lindahl, E., Elofsson, A. (2008). Using Multiple Templates to Improve Quality of Homology Models in Automated Homology Modeling. Protein Sci. 17, 990–1002. doi: 10.1110/ps.073344908
Laskowski, R. A., Luscombe, N. M., Swindells, M. B., Thornton, J. M. (1996). Protein Clefts in Molecular Recognition and Function. Protein Sci. 5, 2438–2452. doi: 10.1002/pro.5560051206
Laskowski, R. A., MacArthur, M. W., Thornton, J. M. (2012). PROCHECK : Validation of Protein-Structure Coordinates. International Tables for Crystallography 21, 684–687. doi: 10.1107/97809553602060000882
Liang, J., Woodward, C., Edelsbrunner, H. (1998). Anatomy of Protein Pockets and Cavities: Measurement of Binding Site Geometry and Implications for Ligand Design. Protein Sci. 7, 1884–1897. doi: 10.1002/pro.5560070905
Liao, K. H., Chen, K. B., Lee, W. Y., Sun, M. F., Lee, C. C., Chen, C. Y. C. (2014). Ligand-Based and Structure-Based Investigation for Alzheimer’s Disease From Traditional Chinese Medicine. Evid. Based Compl. Altern. Med. 2014, 364819/1–364819/16. doi: 10.1155/2014/364819
Lighthall, G. K., Parast, L. M., Rapoport, L., Wagner, T. H. (2010). Introduction of a Rapid Response System at a United States Veterans Affairs Hospital Reduced Cardiac Arrests. Anesth. Analg. 111, 679–686. doi: 10.1213/ANE.0B013E3181E9C3F3
Lima, M. L., Abengózar, M. A., Nácher-Vázquez, M., Martínez-Alcázar, M. P., Barbas, C., Tempone, A. G., et al. (2018). Molecular Basis of the Leishmanicidal Activity of the Antidepressant Sertraline as a Drug Repurposing Candidate. Antimicrob. Agents Chemother. 62, e01928–18/1-e01928-18/43. doi: 10.1128/AAC.01928-18
Lin, X., Li, X., Lin, X. (2020). A Review on Applications of Computational Methods in Drug Screening and Design. Molecules 25, 1375/1–1375/17. doi: 10.3390/molecules25061375
Lin, J. H., Yamazaki, M. (2003). Role of P-Glycoprotein in Pharmacokinetics: Clinical Implications. Clin. Pharmacokinet. 42, 59–98. doi: 10.2165/00003088-200342010-00003
Lionta, E., Spyrou, G., Vassilatis, D., Cournia, Z. (2014). Structure-Based Virtual Screening for Drug Discovery: Principles, Applications and Recent Advances. Curr. Top. Med. Chem. 14, 1923–1938. doi: 10.2174/1568026614666140929124445
Lipinski, C. A., Lombardo, F., Dominy, B. W., Feeney, P. J. (2001). Experimental and Computational Approaches to Estimate Solubility and Permeability in Drug Discovery and Development Settings. Adv. Drug Deliv. Rev. 46, 3–26. doi: 10.1016/S0169-409X(00)00129-0
Löbenberg, R., Amidon, G. L., Ferraz, H. G., Bou-Chacra, N. (2013). Mechanism of Gastrointestinal Drug Absorption and Application in Therapeutic Drug Delivery. Ther. Deliv. Methods A. Concise. Overv. Emerg. Areas. 8–22. doi: 10.4155/EBO.13.349/ASSET/IMAGES/LARGE/FIGURE6.JPEG
Lorente, S. O., Rodrigues, J. C. F., Jiménez, C. J., Joyce-Menekse, M., Rodrigues, C., Croft, S. L., et al. (2004). Novel Azasterols as Potential Agents for Treatment of Leishmaniasis and Trypanosomiasis. Antimicrob. Agents Chemother. 48, 2937–2950. doi: 10.1128/AAC.48.8.2937-2950.2004
Luer, M. S., Penzak, S. R. (2016). Pharmacokinetic Properties. Appl. Clin. Pharmacokinet. Pharmacodyn. Psychopharmacol. Agents. 3-27 doi: 10.1007/978-3-319-27883-4_1
Magaraci, F., Jimenez Jimenez, C., Rodrigues, C., Rodrigues, J. C. F., Vianna Braga, M., Yardley, V., et al. (2003). Azasterols as Inhibitors of Sterol 24-Methyltransferase in Leishmania Species and Trypanosoma Cruzi. J. Med. Chem. 46, 4714–4727. doi: 10.1021/jm021114j
Mak, K. K., Pichika, M. R. (2019). Artificial Intelligence in Drug Development: Present Status and Future Prospects. Drug Discov. Today 24, 773–780. doi: 10.1016/J.DRUDIS.2018.11.014
Mandal, S., Moudgil, N., Mandal, S. K. (2009). Rational Drug Design. Eur. J. Pharmacol. 625, 90–100. doi: 10.1016/j.ejphar.2009.06.065
Mavromoustakos, T., Durdagi, S., Koukoulitsa, C., Simcic, M., Papadopoulos, M. G., Hodoscek, M., et al. (2011). Strategies in the Rational Drug Design. Curr. Med. Chem. 18, 2517–2530. doi: 10.2174/092986711795933731
Meier, A., Söding, J. (2015). Automatic Prediction of Protein 3d Structures by Probabilistic Multi-Template Homology Modeling. PloS Comput. Biol. 11, e1004343/1–e1004343/20. doi: 10.1371/JOURNAL.PCBI.1004343
Mendonca Junior, F. J. B., Scotti, L., Ishiki, H., Botelho, S. P. S., Da Silva, M. S., Scotti, M. T. (2015). Benzo- and Thienobenzo- Diazepines: Multi-Target Drugs for CNS Disorders. Med. Chem. (Los. Angeles). 15, 630–647. doi: 10.2174/1389557515666150219125030
Messaoudi, A., Belguith, H., Ben Hamida, J. (2013). Homology Modeling and Virtual Screening Approaches to Identify Potent Inhibitors of VEB-1 β-Lactamase. Theor. Biol. Med. Model. 10, 22/1–22/10. doi: 10.1186/1742-4682-10-22
Mishra, S., Parmar, N., Chandrakar, P., Sharma, C. P., Parveen, S., Vats, R. P., et al. (2021). Design, Synthesis, In Vitro and In Vivo Biological Evaluation of Pyranone-Piperazine Analogs as Potent Antileishmanial Agents. Eur. J. Med. Chem. 221, 113516/1–113516/14. doi: 10.1016/j.ejmech.2021.113516
Momeni, A. Z., Aminjavaheri, M., Omidghaemi, M. R. (2003). Treatment of Cutaneous Leishmaniasis With Ketoconazole Cream. J. Dermatolog. Treat. 14, 26–29. doi: 10.1080/09546630305552
Mora Lagares, L., Minovski, N., Caballero Alfonso, A. Y., Benfenati, E., Wellens, S., Culot, M., et al. (2020). Homology Modeling of the Human P-Glycoprotein (ABCB1) and Insights Into Ligand Binding Through Molecular Docking Studies. Int. J. Mol. Sci. 21, 4058/1–4058/35. doi: 10.3390/ijms21114058
Morris, G. M., Ruth, H., Lindstrom, W., Sanner, M. F., Belew, R. K., Goodsell, D. S., et al. (2009). Software News and Updates AutoDock4 and AutoDockTools4: Automated Docking With Selective Receptor Flexibility. J. Comput. Chem. 30, 2785–2791. doi: 10.1002/JCC.21256
Mouchlis, V. D., Afantitis, A., Serra, A., Fratello, M., Papadiamantis, A. G., Aidinis, V., et al. (2021). Advances in De Novo Drug Design: From Conventional to Machine Learning Methods. Int. J. Mol. Sci. 22, 1–22. doi: 10.3390/IJMS22041676
Mukherjee, S., Mukherjee, B., Mukhopadhyay, R., Naskar, K., Sundar, S., Dujardin, J. C., et al. (2012). Imipramine Is an Orally Active Drug Against Both Antimony Sensitive and Resistant Leishmania Donovani Clinical Isolates in Experimental Infection. PloS Negl. Trop. Dis. 6, e1987/1–e1987/16. doi: 10.1371/JOURNAL.PNTD.0001987
Mukherjee, S., Xu, W., Hsu, F. F., Patel, J., Huang, J., Zhang, K. (2019). Sterol Methyltransferase Is Required for Optimal Mitochondrial Function and Virulence in Leishmania Major. Mol. Microbiol. 111, 65–81. doi: 10.1111/mmi.14139
Onakpoya, I. J., Heneghan, C. J., Aronson, J. K. (2016). Post-Marketing Withdrawal of 462 Medicinal Products Because of Adverse Drug Reactions: A Systematic Review of the World Literature. BMC Med. 14, 1–11. doi: 10.1186/S12916-016-0553-2
Pandey, P., Prasad, K., Prakash, A., Kumar, V. (2020). Insights Into the Biased Activity of Dextromethorphan and Haloperidol Towards SARS-CoV-2 NSP6: In Silico Binding Mechanistic Analysis. J. Mol. Med. 98, 1659–1673. doi: 10.1007/S00109-020-01980-1/FIGURES/10
Parasuraman, S. (2011). Prediction of Activity Spectra for Substances. J. Pharmacol. Pharmacother. 2, 52–53. doi: 10.4103/0976-500X.77119
Pathania, S., Singh, P. K., Narang, R. K., Rawal, R. K. (2021). Identifying Novel Putative ERK1/2 Inhibitors via Hybrid Scaffold Hopping –FBDD Approach. J. Biomol. Struct. Dyn. 39, 1–16. doi: 10.1080/07391102.2021.1889670
Pence, H. E., Williams, A. (2010). Chemspider: An Online Chemical Information Resource. J. Chem. Educ. 87, 1123–1124. doi: 10.1021/ED100697W
Pérez-Moreno, G., Sealey-Cardona, M., Rodrigues-Poveda, C., Gelb, M. H., Ruiz-Pérez, L. M., Castillo-Acosta, V., et al. (2012). Endogenous Sterol Biosynthesis Is Important for Mitochondrial Function and Cell Morphology in Procyclic Forms of Trypanosoma Brucei. Int. J. Parasitol. 42, 975–989. doi: 10.1016/j.ijpara.2012.07.012
Pettersen, E. F., Goddard, T. D., Huang, C. C., Couch, G. S., Greenblatt, D. M., Meng, E. C., et al. (2004). UCSF Chimera - A Visualization System for Exploratory Research and Analysis. J. Comput. Chem. 25, 1605–1612. doi: 10.1002/jcc.20084
Pountain, A. W., Weidt, S. K., Regnault, C., Bates, P. A., Donachie, A. M., Dickens, N. J., et al. (2019). Genomic Instability at the Locus of Sterol C24-Methyltransferase Promotes Amphotericin B Resistance in Leishmania Parasites. PloS Negl. Trop. Dis. 13, e0007052/1–e0007052/16. doi: 10.1371/journal.pntd.0007052
Prachayasittikul, V., Prachayasittikul, V. (2016). P-Glycoprotein Transporter in Drug Development. EXCLI J. 15, 113–118. doi: 10.17179/EXCLI2015-768
Rahman, F., Tabrez, S., Ali, R., Akand, S. K., Zahid, M., Alaidarous, M. A., et al. (2021). Virtual Screening of Natural Compounds for Potential Inhibitors of Sterol C-24 Methyltransferase of Leishmania Donovani to Overcome Leishmaniasis. J. Cell. Biochem. 122, 1216–1228. doi: 10.1002/JCB.29944
Reynolds, C. H., Bembenek, S. D., Tounge, B. A. (2007). The Role of Molecular Size in Ligand Efficiency. Bioorg. Med. Chem. Lett. 17, 4258–4261. doi: 10.1016/J.BMCL.2007.05.038
Reynolds, C. H., Reynolds, R. C. (2017). Group Additivity in Ligand Binding Affinity: An Alternative Approach to Ligand Efficiency. J. Chem. Inf. Model. 57, 3086–3093. doi: 10.1021/ACS.JCIM.7B00381
Richardson, J. L., Nett, I. R. E., Jones, D. C., Abdille, M. H., Gilbert, I. H., Fairlamb, A. H. (2009). Improved Tricyclic Inhibitors of Trypanothione Reductase by Screening and Chemical Synthesis. ChemMedChem 4, 1333–1340. doi: 10.1002/CMDC.200900097
Rinschen, M. M., Ivanisevic, J., Giera, M., Siuzdak, G. (2019). Identification of Bioactive Metabolites Using Activity Metabolomics. Nat. Rev. Mol. Cell Biol. 20, 353–367. doi: 10.1038/S41580-019-0108-4
Sakyi, P. O., Amewu, R. K., Devine, R. N. O. A., Bienibuor, A. K., Miller, W. A., Kwofie, S. K. (2021a). Unravelling the Myth Surrounding Sterol Biosynthesis as Plausible Target for Drug Design Against Leishmaniasis. J. Parasitol. Dis. 45, 1152–1171. doi: 10.1007/S12639-021-01390-1
Sakyi, P. O., Amewu, R. K., Devine, R. N. O. A., Ismaila, E., Miller, W. A., Kwofie, S. K. (2021b). The Search for Putative Hits in Combating Leishmaniasis: The Contributions of Natural Products Over the Last Decade. Nat. Prod. Bioprospect. 11, 489–544. doi: 10.1007/S13659-021-00311-2
Sander, T., Freyss, J., Von Korff, M., Rufener, C. (2015). DataWarrior: An Open-Source Program for Chemistry Aware Data Visualization and Analysis. J. Chem. Inf. Model. 55, 460–473. doi: 10.1021/CI500588J
Sasin, J. M., Bujnicki, J. M. (2004). COLORADO3D, a Web Server for the Visual Analysis of Protein Structures. Nucleic Acids Res. 1, 586–589. doi: 10.1093/NAR/GKH440
Satari, M. H., Primasari, A., Dharsono, H. D. A., Apriyanti, E., Suprijono, M. M., Herdiyati, Y., et al. (2021). Mode Action Prediction of Butein as Antibacterial Oral Pathogen Against Enterococcus Faecalis ATCC 29212 and an Inhibitor of MurA Enzyme: In Vitro and In Silico Study. Lett. Drug Des. Discov. 18, 744–753. doi: 10.2174/1570180818666210122163009
Sawale, R. T., Kalyankar, T. M., George, R., Deosarkar, S. D. (2016). Molar Refraction and Polarizability of Antiemetic Drug 4-Amino-5-Chloro-N-(2-(Diethylamino)Ethyl)-2 Methoxybenzamide Hydrochloride Monohydrate in {Aqueous-Sodium or Lithium Chloride} Solutions at 30 O C. J. Appl. Pharm. Sci. 6, 120–124. doi: 10.7324/JAPS.2016.60321
Schneider, P., Schneider, G. (2016). De Novo Design at the Edge of Chaos. J. Med. Chem. 59, 4077–4086. doi: 10.1021/ACS.JMEDCHEM.5B01849
Schultes, S., De Graaf, C., Haaksma, E. E. J., De Esch, I. J. P., Leurs, R., Krämer, O. (2010). Ligand Efficiency as a Guide in Fragment Hit Selection and Optimization. Drug Discov. Today Technol. 7, e157–e162. doi: 10.1016/J.DDTEC.2010.11.003
Sharma, M., Chauhan, K., Shivahare, R., Vishwakarma, P., Suthar, M. K., Sharma, A., et al. (2013). Discovery of a New Class of Natural Product-Inspired Quinazolinone Hybrid as Potent Antileishmanial Agents. J. Med. Chem. 56, 4374–4392. doi: 10.1021/jm400053v
Shen, M., Sali, A. (2006). Statistical Potential for Assessment and Prediction of Protein Structures. Protein Sci. 15, 2507–2524. doi: 10.1110/PS.062416606
Singh, S., McCoy, J. G., Zhang, C., Bingman, C. A., Phillips, G. N., Thorson, J. S. (2008). Structure and Mechanism of the Rebeccamycin Sugar 4′-O-Methyltransferase RebM. J. Biol. Chem. 283, 22628–22636. doi: 10.1074/jbc.M800503200
Sinha, S., Wang, S. M. (2020). Classification of VUS and Unclassified Variants in BRCA1 BRCT Repeats by Molecular Dynamics Simulation. Comput. Struct. Biotechnol. J. 18, 723–736. doi: 10.1016/J.CSBJ.2020.03.013
Sippl, M. J. (1993). Recognition of Errors in Three-Dimensional Structures of Proteins. Proteins Struct. Funct. Genet. 17, 355–362. doi: 10.1002/prot.340170404
Sterling, T., Irwin, J. J. (2015). ZINC 15 - Ligand Discovery for Everyone. J. Chem. Inf. Model. 55, 2324–2337. doi: 10.1021/ACS.JCIM.5B00559
Šudomová, M., Hassan, S. T. S., Khan, H., Rasekhian, M., Nabavi, S. M. (2019). A Multi-Biochemical and In Silico Study on Anti-Enzymatic Actions of Pyroglutamic Acid Against PDE-5, ACE, and Urease Using Various Analytical Techniques: Unexplored Pharmacological Properties and Cytotoxicity Evaluation. Biomolecules 9, 392–405. doi: 10.3390/BIOM9090392
Sun, H., Tawa, G., Wallqvist, A. (2012). Classification of Scaffold-Hopping Approaches. Drug Discov. Today 17, 310–324. doi: 10.1016/J.DRUDIS.2011.10.024
Tabrez, S., Rahman, F., Ali, R., Muhammad, F., Alshehri, B. M., Alaidarous, M. A., et al. (2021). Repurposing of FDA-Approved Drugs as Inhibitors of Sterol C-24 Methyltransferase of Leishmania Donovani to Fight Against Leishmaniasis. Drug Dev. Res. 82, 1154–1161. doi: 10.1002/DDR.21820
Talevi, A., Bellera, C. L. (2019). Challenges and Opportunities With Drug Repurposing: Finding Strategies to Find Alternative Uses of Therapeutics. Taylor. Fr. 15, 397–401. doi: 10.1080/17460441.2020.1704729
Tian, W., Chen, C., Lei, X., Zhao, J., Liang, J. (2018). CASTp 3.0: Computed Atlas of Surface Topography of Proteins. Nucleic Acids Res. 46, W363–W367. doi: 10.1093/nar/gky473
Tippmann, S. (2014). Programming Tools: Adventures With R. Nature 517, 109–110. doi: 10.1038/517109a
Torres-Santos, E. C., Andrade-Neto, V. V., Cunha-Júnior, E. F., Do Canto-Cavalheiro, M. M., Atella, G. C., De Almeida Fernandes, T., et al. (2016). Antileishmanial Activity of Ezetimibe: Inhibition of Sterol Biosynthesis, In Vitro Synergy With Azoles, and Efficacy in Experimental Cutaneous Leishmaniasis. Antimicrob. Agents Chemother. 60, 6844–6852. doi: 10.1128/AAC.01545-16
Trott, O., Olson, A. J. (2010). AutoDock Vina: Improving the Speed and Accuracy of Docking With a New Scoring Function, Efficient Optimization and Multithreading. J. Comput. Chem. 31, 455–461. doi: 10.1002/JCC.21334
Urbina, J. A., Visbal, G., Contreras, L. M., McLaughlin, G., Docampo, R. (1997). Inhibitors of Δ(24(25)) Sterol Methyltransferase Block Sterol Synthesis and Cell Proliferation in Pneumocystis Carinii. Antimicrob. Agents Chemother. 41, 1428–1432. doi: 10.1128/AAC.41.7.1428
Urbina, J. A., Vivas, J., Visbal, G., Contreras, L. M. (1995). Modification of the Sterol Composition of Trypanosoma (Schizotrypanum) Cruzi Epimastigotes by Δ24(25)-Sterol Methyl Transferase Inhibitors and Their Combinations With Ketoconazole. Mol. Biochem. Parasitol. 73, 199–210. doi: 10.1016/0166-6851(95)00117-j
van den Anker, J., Reed, M. D., Allegaert, K., Kearns, G. L. (2018). Developmental Changes in Pharmacokinetics and Pharmacodynamics. J. Clin. Pharmacol. 58, S10–S25. doi: 10.1002/JCPH.1284
Van Der Spoel, D., Lindahl, E., Hess, B., Groenhof, G., Mark, A. E., Berendsen, H. J. C. (2005). GROMACS: Fast, Flexible, and Free. J. Comput. Chem. 26, 1701–1718. doi: 10.1002/JCC.20291
Van Norman, G. A. (2019). Phase II Trials in Drug Development and Adaptive Trial Design. JACC Basic. To. Transl. Sci. 4, 428–437. doi: 10.1016/J.JACBTS.2019.02.005
Veber, D. F., Johnson, S. R., Cheng, H. Y., Smith, B. R., Ward, K. W., Kopple, K. D. (2002). Molecular Properties That Influence the Oral Bioavailability of Drug Candidates. J. Med. Chem. 45, 2615–2623. doi: 10.1021/JM020017N
Waterhouse, A., Bertoni, M., Bienert, S., Studer, G., Tauriello, G., Gumienny, R., et al. (2018). SWISS-MODEL: Homology Modelling of Protein Structures and Complexes. Nucleic Acids Res. 46, W296–W303. doi: 10.1093/NAR/GKY427
Wiederstein, M., Sippl, M. J. (2007). ProSA-Web: Interactive Web Service for the Recognition of Errors in Three-Dimensional Structures of Proteins. Nucleic Acids Res. 35, W407–W410. doi: 10.1093/nar/gkm290
Wishart, D. S., Feunang, Y. D., Guo, A. C., Lo, E. J., Marcu, A., Grant, J. R., et al. (2018). DrugBank 5.0: A Major Update to the DrugBank Database for 2018. Nucleic Acids Res. 46, D1074–D1082. doi: 10.1093/NAR/GKX1037
Wyllie, S., Thomas, M., Patterson, S., Crouch, S., De Rycker, M., Lowe, R., et al. (2018). Cyclin-Dependent Kinase 12 Is a Drug Target for Visceral Leishmaniasis. Nature 560, 192–197. doi: 10.1038/S41586-018-0356-Z
Xu, D., Zhang, Y. (2011). Improving the Physical Realism and Structural Accuracy of Protein Models by a Two-Step Atomic-Level Energy Minimization. Biophys. J. 101, 2525–2534. doi: 10.1016/j.bpj.2011.10.024
Keywords: leishmaniasis, 24-sterol methyltransferase, Leishmania donovani, de-novo drug design, molecular docking, molecular dynamics simulation
Citation: Sakyi PO, Broni E, Amewu RK, Miller WA III, Wilson MD and Kwofie SK (2022) Homology Modeling, de Novo Design of Ligands, and Molecular Docking Identify Potential Inhibitors of Leishmania donovani 24-Sterol Methyltransferase. Front. Cell. Infect. Microbiol. 12:859981. doi: 10.3389/fcimb.2022.859981
Received: 22 January 2022; Accepted: 28 April 2022;
Published: 02 June 2022.
Edited by:
Vipan Kumar, Guru Nanak Dev University, IndiaReviewed by:
Marcus Scotti, Federal University of Paraíba, BrazilCopyright © 2022 Sakyi, Broni, Amewu, Miller, Wilson and Kwofie. This is an open-access article distributed under the terms of the Creative Commons Attribution License (CC BY). The use, distribution or reproduction in other forums is permitted, provided the original author(s) and the copyright owner(s) are credited and that the original publication in this journal is cited, in accordance with accepted academic practice. No use, distribution or reproduction is permitted which does not comply with these terms.
*Correspondence: Samuel Kojo Kwofie, c2trd29maWVAdWcuZWR1Lmdo
Disclaimer: All claims expressed in this article are solely those of the authors and do not necessarily represent those of their affiliated organizations, or those of the publisher, the editors and the reviewers. Any product that may be evaluated in this article or claim that may be made by its manufacturer is not guaranteed or endorsed by the publisher.
Research integrity at Frontiers
Learn more about the work of our research integrity team to safeguard the quality of each article we publish.