- 1Institute of Statistics, National Yang Ming Chiao Tung University, Hsinchu, Taiwan
- 2Institute of Data Science and Engineering, National Yang Ming Chiao Tung University, Hsinchu, Taiwan
Background and Aims: Entacapone, one of the most common drugs distributed among patients with Parkinson’s disease, is a peripherally acting catechol-O-methyltransferase (COMT) inhibitor that is used in addition to levodopa to control symptoms. However, there have been negative effects reported against entacapone, namely, gastrointestinal (GI) problems and drowsiness. In this pilot study, we aim to examine the hypothesis that the discomfort induced by entacapone might be originated from the shift of microbial composition by adjusting the effect of levodopa.
Methods: The population in this pilot study consisted of 13 PD patients treated with levodopa only and 11 with both levodopa and entacapone. The 16S rRNA gene sequence data were processed, aligned, and categorized using the DADA2. Alpha diversity indices for Observed, Chao1, Shannon, and Simpson metrics were calculated with Phyloseq 1.32.0. Dissimilarities were calculated using unweighted unique fraction metrics (Unifrac), weighted Unifrac, and Canberra distance. Functional differences were calculated by PICRUSt2 based on the KEGG database.
Results: Results of 16S rRNA sequencing analysis showed that while entacapone did not influence the species richness, the composition of the microbial community shifted considerably. Relative abundances of bacteria related to constipation and other GI disorders also altered significantly. Functional enrichment analysis revealed changes in the metabolic activity of alanine, aspartate, and glutamate. These amino acids are related to common side effects of entacapone such as auditory hallucinations, fatigue, and nightmare.
Conclusion: Our findings provide testable hypothesis on the cause of unpleasant side effects of entacapone, which in the long run could possibly be reduced through gut microbiota manipulation.
Introduction
Parkinson’s disease (PD) is one of the most common neurodegenerative disorders in the world which currently cannot be prevented or cured (Lebouvier et al., 2009; Wang, 2021). Its clinical features are akinesia, bradykinesia, rigidity, and tremors, which are known to be directly related to the progressive death of dopaminergic neurons (Xia and Mao, 2012). Levodopa (LD), a substance that could cross the blood–brain barrier and further increase brain dopamine concentrations, is currently the most effective symptomatic agent for the treatment of PD (Haddad et al., 2018). The use of LD is often coupled with entacapone, a catechol-O-methyltransferase (COMT) inhibitor, to increase its effectiveness and to reduce the risk of motor complications in PD patients (Schrag, 2005).
Adjunctive use of entacapone with LD in PD is beneficial by preventing catechol-O-methyltransferase from breaking down and metabolizing LD, which extends and stabilizes the clinical response to LD (Kaakkola, 2000). Although the addition of entacapone combined with LD is beneficial, several bothersome side effects come along with the treatment such as abdominal pain, constipation, diarrhea, nausea, etc. Some may even experience serious side effects that require immediate medical attention (Chong and Mersfelder, 2000). In a meta-analysis done with 14 studies to compare the effectiveness and safety of adjuvant treatment of entacapone in PD, a trend towards increased patient withdrawal was found due to intolerable adverse events. Patients on entacapone had a higher frequency of adverse events than those on placebo (Li et al., 2017). Although it is hypothesized that these adverse events may be related to the increase of dopamine caused by entacapone in the corpus striatum (Li et al., 2017), the underlying mechanism is still unclear.
Research have shown that human microbiome plays a critical role in the development and progression of major human diseases such as gastrointestinal disorders, metabolic diseases, psychological diseases, etc. (Wang et al., 2017). Human cohort studies have also provided insights between use of drugs and altered microbial composition and functional profiles (Zhernakova et al., 2016; Jackson et al., 2018; Vila et al., 2020). Evidence showed that there is a direct metabolizing effect of bacteria by entacapone (Weersma et al., 2020). Conversely, entacapone also inhibits the growth rate of several bacterial species (Maier et al., 2018). Despite the evidence showing complicated bidirectional interactions between entacapone and gut microbes, the studies were either done in vitro or in vivo without eliminating the effect of LD (Unger et al., 2016; Maier et al., 2018; Weis et al., 2019; Zimmermann et al., 2019).
In this study, we investigated the potential effect of entacapone alone on the alteration of microbial composition and the association of this alteration with the ensued side effects. We present a case–control study where microbiota in PD patients treated with LD only (PD_L) and patients treated with entacapone in addition to LD (PD_LE) are analyzed. We ensured the study groups did not differ with respect to possible confounders and then employed tools for bacterial identification, evaluation of ecological diversities of the microbiome, and functional predictions.
Methods
Participant Recruitment and Data Collection
We adapted our data from the study of Weis et al. (2019), in which 34 PD patients and 25 healthy controls were recruited. Among the cohort, 13 patients (6 women, 7 men) treated with LD only (PD_L) and 11 patients (4 women, 7 men) treated with both LD and entacapone (PD_LE) were selected for our study. Both groups were age and sex matched. At the time of sampling, the mean age of PD_L group was 70.6 (sd = 8.8) years and 69.9 (sd = 5.39) years for the PD_LE group. Mean duration of the disease was 77.9 (sd = 50.9) and 120 (sd = 54.3) months for PD_L and PD_LE respectively. Sequencing library preparation of the V4 and V5 region of the bacterial 16S rRNA genes were performed using the 16S-specific primers 520 F (5′-AYTGGGYDTAAAGNG-3′) and 926 R (5′-CCGTCAATTCMTTTRAGTTT-3′). Unique, custom made index barcodes with sequencing adapter (CCATCTCATCCCTGCGTGTCTCCGACTCAG) were added to the amplicon targets. Details of diagnostic criteria for PD, fecal sample collection process, DNA isolation, library preparation and sequencing can be found in the previous reference (Weis et al., 2019).
Data Availability and Ethical Statement
Sequences analyzed in this study are accessible at the European Nucleotide Archive (ENA) under the accession number PRJEB30615. Subject data can be found in the supplementary information files in the paper of Weis et al. All data are open-access and de-identified. No ethical approval is required.
Processing of 16s rRNA Sequence Data
The 16S rRNA gene is highly conserved in bacteria. As a result, it is highly suited as a target gene for DNA sequencing for bacterial identification. The 16S rRNA gene sequence data was downloaded at the European Nucleotide Archive (ENA) under the accession number PRJEB30615 (Weis et al., 2019). Each sample was sequenced four times (paired-end reads) and we merged the sequences of four independent runs into a single dataset for all samples. The combined sequence reads were then processed with Trimmomatic v0.39 (Bolger et al., 2014) to remove the adaptors. The outputs were then processed, aligned, and categorized using the DADA2 1.16 (Callahan et al., 2016) pipeline. In brief, sequence reads were first filtered using recommended parameters of DADA2. Filtered reads were then de-replicated and de-noised using DADA2 default parameters. After building the amplicon sequence variant (ASV) table and removing chimeras, taxonomy was assigned using SILVA v132 natively implemented in DADA2. We used the addSpecies function in DADA2 to add species-level annotation with SILVA as reference. Sequence counts were normalized to relative abundances (Calculated by dividing the number of sequences that were assigned to a unique ASV by the total sequence count in the sample, which is also termed as Total-Sum Scaling) for later use. In addition to scaling, rarefying is another popular approach for normalization in literatures. However, rarefying was not adapted in this study due to the limitations of potentially reducing statistical power and the restriction of sample size of our dataset.
Statistical Analyses of 16s rRNA Sequence Data
Alpha diversity indices for Observed features, Chao1, Shannon, and Simpson metrics (Chao, 1984; Magurran, 1988; Rosenzweig, 1995) were calculated with Phyloseq 1.32.0 (McMurdie and Holmes, 2013). Dissimilarities (distance) between the microbiomes of PD_LE and PD_L samples were calculated using the following metrics to ensure that the choice of metrics did not affect the results: unweighted unique fraction metrics (Unifrac), weighted Unifrac (Lozupone et al., 2011), and Canberra distance (Lance and Williams, 1967). Beta diversity indices for weighted and unweighted unifrac were calculated with Phyloseq 1.32.0 (McMurdie and Holmes, 2013). Canberra distance was calculated with vegan 2.5.7. The differences between PD_LE and PD_L were tested. P-values for alpha diversity were calculated with ANOVA using stats 4.0.5 and beta diversity with ADONIS using vegan 2.5.7. The identification of significantly different phylum and genus between PD_LE and PD_L was performed using t-test. Variable comparison between the two groups were conducted by Fisher’s exact test and Wilcoxon rank sum test.
Functional Enrichment Analysis of Predicted Metagenomes
We used Phylogenetic Investigation of Communities by Reconstruction of Unobserved States (PICRUSt2) version 2.4.1 (Douglas et al., 2020) to infer metagenome composition in the samples, following the recommended pipeline of normalizing ASVs by copy number (to account for differences in number of copies of 16S rRNA between taxa), predicting functions using Kyoto Encyclopedia of Genes and Genomes (KEGG) (Kanehisa and Goto, 2000) orthologs, and grouping predicted pathways by KEGG hierarchical level 3. We tested PD_L and PD_LE differences for all metabolic pathways presented in all of our samples (N = 116 pathways) using the Statistical Analysis of Metagenomic Profiles (STAMP) software version 2.1.3 (Parks and Beiko, 2013). We compared PD_L versus PD_LE using White’s non-parametric t-test (two sided, replication = 1,000), with a Storey FDR <.05 as a cutoff for significance.
Results
Processing 16S Reads Using DADA2
Patients treated with LD and entacapone (n = 11) and those treated with LD only (n = 13) from Weis et al. (2019) were selected for our study. The general characteristics of these participants are summarized in Table 1. We downloaded the V4 and V5 regions of the bacterial 16S rRNA sequencing data (which was sequenced using an Ion Torrent PGM platform.) of the 24 samples to further characterize the microbiome. A total of 15,019,733 sequences with a mean of 625,822 sequences per sample (min: 85,858; max: 2,710,753 sequences) was obtained. The average read length was 293.28 base pairs, totaling 4.41 G bases. After filtering, denoising, and removing chimeras, we retained 11,608,066 (77.29% of initial) reads. We then assigned taxa to these reads. A total of 11,432 ASVs were identified, in which 15 phyla and 255 genera were assigned. The number and all detailed information of all taxonomic levels were in the Supplementary Materials.
Metadata Comparison Between Patients Treated With and Without Entacapone
Microbiome can be potentially affected by numerous factors. If the distribution of such variable differs between PD_LE and PD_L, then it is possible to find a significant difference in microbiota between the study groups that is simply an artifact of the related variable. Given the reason above, we have tested the data on all variables collected. If the difference between P_LE and P_L was significant, the variable would be considered as a potential confounder. Our results showed that none of the 12 variables (sex, age, calprotectin, smoker, constipation, disease duration months, Hoehn Yahr stage, phenotype, appendectomy, family history for neurodegenerative disorders, LD dose, other GI symptoms) were significantly different between P_LE and P_L (Table 1), indicating that these variables did not confound the causal relationship between entacapone and the following microbiome change.
Structural Diversity Measures
We compared the overall taxonomic diversity between PD_L and PD_LE using different metrics of alpha diversity that incorporate species richness and evenness. We estimated observed features (i.e., number of ASVs), and the Chao1, Shannon and Simpson indices from the ASV table. None of the measures mentioned above were significantly different between the groups (pObserved = 0.3348; pChao1 = 0.3384; pShannon = 0.9736; pSimpson = 0.8675) (Figure 1A). Non-parametric method (Kruskal–Wallis test) generated similar results, which was provided in the Supplementary Material. We next applied both phylogenetic (UniFrac) and non-phylogenetic (Canberra) methods to calculate the dissimilarities (distance) between the microbiomes of PD_LE and PD_L samples. In contrast to alpha-diversity, we found a significant change in community structure using Canberra distance metric (pCanberra = 0.0025). Using principle coordinate analysis (PCoA) based on Canberra distances, we found that the two highest-ranked dimensions, PCo1 and PCo2, explained 7 and 6.7% of variance respectively (Figure 1B). Significant difference was also found between the groups in the unweighted Unifrac measure (pUnweighted-unifrac = 0.004). The two highest-ranked dimensions, PCo1 and PCo2, explained 10.4 and 8.9% of variance respectively (Figure 1C). No significant difference was found in weighted UniFrac measure between the groups (pWeighted-unifrac = 0.1945) (Figure 1D).
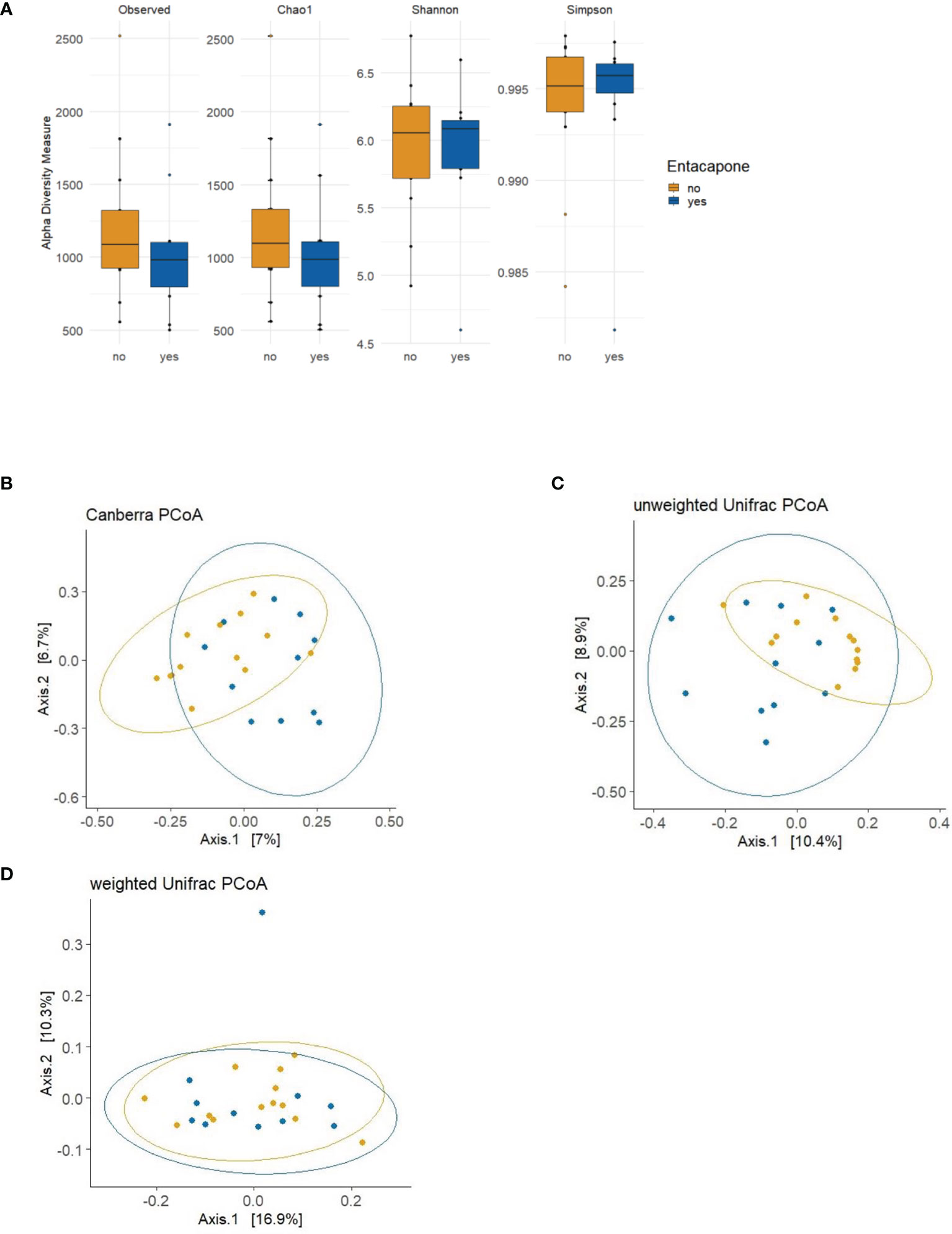
Figure 1 Alpha and beta diversity plots to visualize the difference in microbiota structure between the study groups PD_LE and PD_L. (A) The boxplots show the alpha diversity of the bacterial communities by means of observed amplicon sequence variants (ASVs), and Chao1, Shannon, and Simpson indexes. Median, and also lower and upper quartiles are shown on the plots. The PCoA plots show the following 3 distance measures: Canberra (B), unweighted unique fraction metric (UniFrac) (C), and weighted UniFrac (D). Statistically significant differences were found between the two groups using Canberra (ADONIS; p = 0.0025; R2 = 0.0546; perm = 9999) and unweighted UniFrac distance metrics (ADONIS; p = 0.004; R2 = 0.0628; perm = 9999). Blue: PD_LE, orange: PD_L. Each dot represents an individual sample. Ellipses are drawn at 95% confidence intervals.
Identification of Taxa That Differed Between Patients Treated With and Without Entacapone
Out of 11,432 ASVs detected in both PD_LE and PD_L, 108 were significantly different in relative abundance. Among the significant ASVs, 17 (15.7%) were classified as Actinobacteria and 91 (84.3%) were classified as Firmicutes. These two taxa were also the first two top phyla detected after running DADA2. Apart from the 3 ASVs without genus information, we were able to identify the rest 105 significant ASVs in 9 genera. It is worth noting that of all the significant ASVs, 86 (79.63%) were decreased and 22 (20.37%) were increased. All of the decreased ASVs were Firmicutes and 77.27% of the increased ASVs were Actinobacterium (Figure 2A). The increased genera were Eubacterium, Christensenellaceae_R-7_group, and Bifidobacterium. The decreased genera were Sellimonas, Lactobacillus, Intestinibacter, Faecalibacterium, Dorea, Blautia (Figures 2B, 3). Only 4 significant ASVs were classified in species level (Intestinibacter bartlettii, Dorea longicatena, Blautia obeum). Among the bacteria that were significantly different in abundance between PD_LE and PD_L, 5 genera were unique to our study when comparing to Weis et al. (2019). These were Lactobacillus, Intestinibacter, Dorea, Christensenellaceae_R_7_group, and Blautia (Figure 3). ANCOM was also conducted for sensitivity analysis and the results were provided in the Supplementary Material. The aforementioned 9 genera with additional 5 genera (Agathobacter, Clostridium_sensu_stricto_1, Lactonifactor, Romboutsia, and Streptococcus) were found significantly different between the groups.
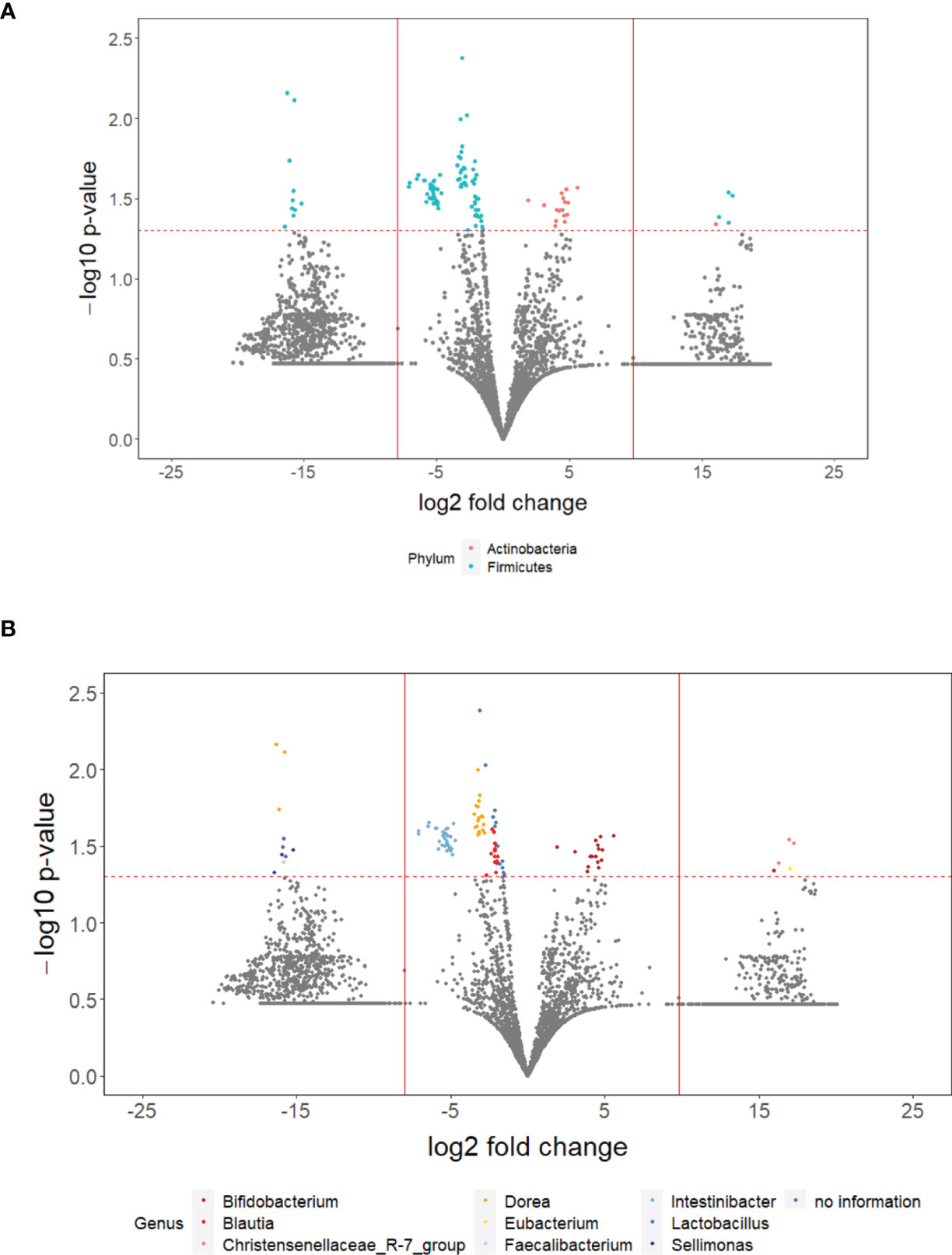
Figure 2 The overall difference of gut microbiota between PD_LE and PD_L individuals was assessed. A negligible number (1.635235 ∗ 10−9) was added to the abundance of every ASV to avoid the value of magnitude of fold change being infinitely large or small. This number was generated based on the one hundredth of the minimal relative abundance. Each point represents an ASV with its magnitude fold change in relative abundance (log2 of PD_LE/PD_L) on the x-axis and the value of statistical significance (−log10 of p-value) on the y-axis. The dashed red line shows where p = 0.05 with points above the line having p <0.05 and points below the line having p > 0.05. Significant ASVs are colored based on phylum (A) and genus (B). Points outside of the solid lines are ASVs with mean abundance of 0 in either PD_LE (left) or PD_L (right) group.
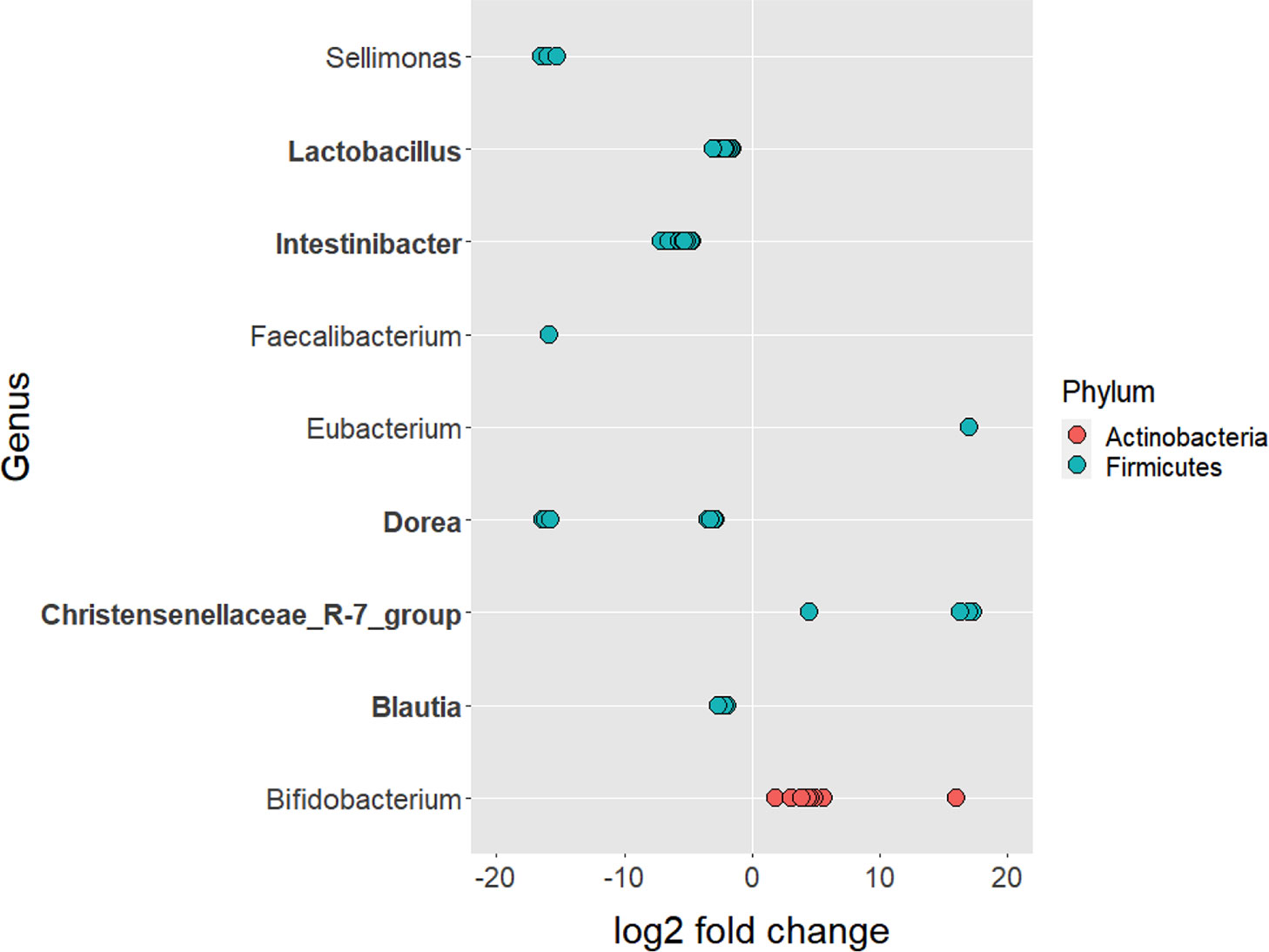
Figure 3 ASVs that significantly differed in abundance before and after the treatment of entacapone. 108 ASVs were identified as significantly different after the treatment of entacapone. 3 ASVs that were not assigned taxonomy to the genus level had a decrease in abundance. They were removed for plotting purposes. Genus and phylum level classification of the significant ASVs are provided in the plot. Genus in bold are unique to our study when compared with Weis et al. (2019).
Functional Prediction
To evaluate functional differences in the microbiomes of PD_LE versus PD_L, we used PICRUSt2 (Douglas et al., 2020), a computational tool that allows using 16S rRNA amplicon data to predict the functional potential of a bacterial community based on the KEGG database. We then tested the difference between PD_LE versus PD_L using STAMP. Among the 116 metabolic pathways tested, 10 were significantly different between PD_LE versus PD_L (Figure 4). According to the KEGG hierarchical level 2 classification, the 10 pathways are involved in carbohydrate metabolism, energy metabolism, lipid metabolism, amino acid metabolism, metabolism of other amino acids, metabolism of terpenoids and polyketides, biosynthesis of other secondary metabolites, xenobiotics biodegradation and metabolism.
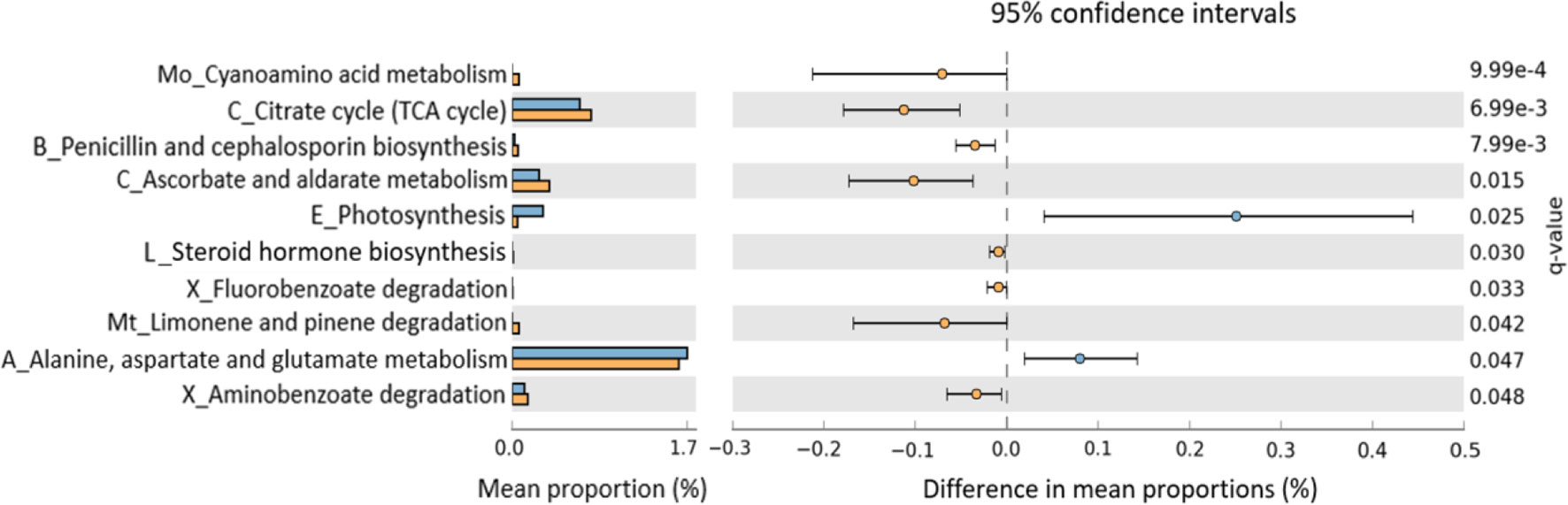
Figure 4 Predicted functional differences between PD_L and PD_LE microbiomes. A total of 10 metabolic pathways differed significantly between patients treated with and without entacapone. Pathways that were more abundant in PD_LE are on the positive side (blue circle with 95% confidence interval). Pathways that were more abundant in PD_L are on the negative side (orange circle). q-value, the Storey false discovery rate (FDR)-corrected P-value. Mean proportions are shown in stacks for PD_LE (blue) and PD_L (orange). Differences in mean proportions: mean proportion in PD_LE minus mean proportions in PD_L. Only metabolic pathways at the Kyoto Encyclopedia of Genes Genomes (KEGG) hierarchical level 1 were investigated to limit inclusion of nonbacterial pathways. Tests were conducted at KEGG hierarchical level 3, which included 116 pathways present in all samples. The letter(s) in front of each pathway name indicates the KEGG hierarchical level 2 for that pathway (A, amino acid metabolism; B, biosynthesis of other secondary metabolites; C, carbohydrate metabolism; E, energy metabolism; L, lipid metabolism; Mo, metabolism of other amino acids; Mt, metabolism of terpenoids and polyketides; X, xenobiotics biodegradation and metabolism); TCA, tricarboxylic acid.
Discussion
Being a common medication used in combination with LD for the treatment of Parkinson’s disease, microbiologists have gained interests in the interactions between entacapone and gut microbiome, resulting in research that has been conducted in the related field (Unger et al., 2016; Weis et al., 2019; Grün et al., 2020). Yet most of these in vivo studies overlooked the impact of LD on the microbial composition (Weis et al., 2019; Cirstea et al., 2020), which led to findings that could possibly be associated with LD instead of entacapone. In vitro studies such as high-throughput drug screening have also shown significant effect of entacapone on gut microbiome (Maier et al., 2018; Zimmermann et al., 2019). However, the selected bacteria only partially recapitulate the human microbiota. These test results do not fully represent the alteration of microbiome in the human gut. Because of the limitations in previous studies, the impact on microbiome solely by entacapone treatment in PD patients is still unclear. To the best of our knowledge, this is the first study investigating the influence of entacapone via 16S rRNA gene sequencing by adjusting the effect of LD on the human microbiota and examining how these microbial signatures may associate with adverse reactions to entacapone.
Our results revealed that entacapone alone had no effect on phylogenetic diversity and species richness; however, it was a major effect factor for the phylogenetic composition of these samples. The majority of bacteria significantly increased and decreased in abundance were Actinobacteria and Firmicutes respectively. Several GI disorders have been proved to be associated with the imbalance of bacteria under these phyla (Passos and Moraes-Filho, 2017; Dieterich et al., 2018; Binda et al., 2018; Rinninella et al., 2019), suggesting the possibility of their mediating effects on the relationship between entacapone treatment and the subsequent side effects. Significant differences in the metabolism between PD_LE and PD_L were suggested by the overrepresentation and underrepresentation of the predicted KEGG pathways associated with different metabolic process and biosynthesis in either group.
Several findings regarding the changes in the microbiome after entacapone treatment deserve consideration. First, our findings agree with the experimental results described below, suggesting the mediating role of microbial change towards side effects, especially constipation [such as Faecalibacterium prausnitzii, Bifidobacterium, Lactobacillus, and Eubacterium (Zoppi et al., 1998; Nourrisson et al., 2014; Mirghafourvand et al., 2016; Cao et al., 2017)], caused by the intake of entacapone. Recent studies using 16S rRNA-based microbiota profiling have demonstrated dysbiosis of gut microbiota in constipation (Ohkusa et al., 2019). Although the findings are inconsistent and currently no consensus exists, studies in constipation have found significant inter-individual differences between healthy individual and patients (Zhu et al., 2014; Mancabelli et al., 2017; Li et al., 2020). Interestingly, results of alpha-diversity analysis from these studies suggested similar community richness and diversity between the two groups (Li et al., 2020), which were consistent with our findings. Evidence from a mouse study showed the causal relationship between dysbiosis and chronic constipation. Mice received fecal microbiota from patients with constipation produced constipated features such as reduction of intestinal motility, lower frequency of pellet expulsion, less fecal water content, etc. (Cao et al., 2017). A major alteration in the microbial community was also found when compared to mice receiving fecal microbiota from healthy individuals (Cao et al., 2017). Their proportion of Firmicutes and the relative abundance of Lactobacillus were both significantly lower, which could also be seen in PD_LE in our study.
A second key observation from our study was that we not only confirmed the previous reported bacteria associating with COMT-inhibitors, we have also found unique microbial signatures that are highly related to GI disorders. Results from our analysis showed that 86.16% of the significantly decreased ASVs were classified in the Firmicutes phylum in PD_LE. At genus level, the relative abundance of Faecalibacterium decreased by 66.08% and Bifidobacterium doubled when comparing PD_LE to PD_L. These results corroborate with earlier reports (Unger et al., 2016; Weis et al., 2019; Grün et al., 2020), suggesting that entacapone itself is able to redistribute bacterial community profile in PD patients. Studies done by real-time quantitative PCR showed that the abundance of Faecalibacterium prausnitzii significantly reduced in PD patients on entacapone when compared to controls or participants without COMT-inhibitors (Unger et al., 2016; Grün et al., 2020). In another study conducting 16S rRNA analysis, the relative abundance of Faecalibacterium also significantly decreased in patients treated with entacapone when compared to the controls (Weis et al., 2019). On the other hand, increasing relative abundance of Bifidobacterium was seen in PD patients on a COMT-inhibitor therapy (Aho et al., 2019). Interestingly, the significant decrease of F. prausnitzii and increase of Bifidobacterium were both found in the fecal samples of constipated individuals (Zoppi et al., 1998; Nourrisson et al., 2014).
On comparison of our data with the study done by Weis et al. (2019), we have revealed several genera that were specifically altered by entacapone in addition to their findings. The altered concentrations of these bacteria were associated with individuals suffered from gastrointestinal disorders, mainly constipation. For example, the deficiencies of Lactobacillus were involved in reduced frequency of bowel movements in pregnant woman (Mirghafourvand et al., 2016). Intestinal abundance of Lactobacillus is positively correlated with Crohn’s disease patients (Wang et al., 2014; Lewis et al., 2015). Another study showed the increase of Dorea could alleviate symptoms in constipated mice (Wang et al., 2019). Species under Eubacterium increased significantly in mice underwent fecal microbiota transplant from constipated participants (Cao et al., 2017).
Results from functional prediction suggested that there was an increased activity in amino acid metabolism such as alanine, aspartate, and glutamate. Accumulating evidences have indicated that the dysregulation of these amino acids might involve in the pathology of fatigue, which is one of the major side effects of entacapone (Marquezi et al., 2003; Stout et al., 2007; Li et al., 2020). Whether entacapone induced microbiome alteration leads to dizziness and drowsiness via regulating the metabolic pathways of the above-mentioned amino acid is worth future investigation.
Several limitation merits to be noticed. First, our results do not prove causality between entacapone, microbiota, and the side effects that may ensue due to our cross-sectional data and the small sample size (the microbial differences between patients with constipation and without constipation, with GI or without GI disorder are provided in the Supplementary Material). However, the correlations are plausible and worth future investigation. To further understand the causal role of gut microbiota in the development of side effects, mediation analysis of properly designed longitudinal studies with sufficient amount of sample size would be highly recommended. Second, information such as medication history or diet habit was not provided in the original dataset. In future studies, all factors with potential to affect microbiome should be measured and correctly adjusted. Third, due to the restriction of sample size, several important factors such as disease duration were not adjusted. It is worth to note that patients with entacapone had longer disease duration, despite not significantly different between P_LE and P_L groups. This phenomenon could be partially explained by the fact that patients with longer disease duration had higher prevalence of levodopa resistance and were more likely to be prescribed entacapone. Disease duration should be adjusted in future studies when dataset with larger size is available. Fourth, as far as we know, mechanisms which explained the association between certain bacteria, such as Intestinibacter, and constipation or other GI disorders have not been investigated by previous literature. However, it was reported that metformin, a type 2 diabetes medication and known as its severe gastrointestinal adverse effects, also significantly lowers Intestinibacter abundance in colon microbiota. Based on the findings, researchers proposed a hypothesis that Intestinibacter might subsequently play a role in inducing gastrointestinal adverse effects (Olgun, 2017; Bryrup et al., 2019). Our finding with Intestinibacter slightly supported this hypothesis and more sophisticated studies are required to disentangle this question. Finally, the sample size is relatively small, leading to lower statistical power and less confounders that could be adjusted. Therefore, this study should be viewed as a pilot study. All conclusions drawn from this study should be examined by studies with larger sample size and with more sophisticated design and analysis.
In conclusion, our findings provide testable hypothesis on the cause of unpleasant side effects of entacapone, which in the long run could possibly be reduced through gut microbiota manipulation.
Data Availability Statement
Publicly available datasets were analyzed in this study. This data can be found here: European Nucleotide Archive (ENA) under the accession number PRJEB30615.
Author Contributions
SF came up with the original idea. SF, YH, and PW set up and performed the bioinformatics procedures. CL, YH, and PW conducted data analysis. SF wrote the first version of manuscript. SF, SL, and HW contributed to the paper. All authors listed have made a substantial, direct, and intellectual contribution to the work and approved it for publication.
Funding
This research was supported by The Ministry of Science and Technology, 107-2118-M-009 -002-MY2 (for SF and HW) and 111-2628-B-A49 -007- (for CL, YH, PW and SL), Taiwan.
Conflict of Interest
The authors declare that this research was conducted in the absence of commercial or financial relationships that could be construed as potential conflicts of interest.
Publisher’s Note
All claims expressed in this article are solely those of the authors and do not necessarily represent those of their affiliated organizations, or those of the publisher, the editors and the reviewers. Any product that may be evaluated in this article, or claim that may be made by its manufacturer, is not guaranteed or endorsed by the publisher.
Acknowledgments
We thank Dr. Weis’ team for their published data.
Supplementary Material
The Supplementary Material for this article can be found online at: https://www.frontiersin.org/articles/10.3389/fcimb.2022.837019/full#supplementary-material
References
Aho, V. T., Pereira, P. A., Voutilainen, S., Paulin, L., Pekkonen, E., Auvinen, P., et al. (2019). Gut Microbiota in Parkinson's Disease: Temporal Stability and Relations to Disease Progression. EBioMedicine 44, 691–707. doi: 10.1016/j.ebiom.2019.05.064
Binda, C., Lopetuso, L. R., Rizzatti, G., Gibiino, G., Cennamo, V., Gasbarrini, A. (2018). Actinobacteria: A Relevant Minority for the Maintenance of Gut Homeostasis. Digestive Liver Disease 50 (5), 421–428. doi: 10.1016/j.dld.2018.02.012
Bolger, A. M., Lohse, M., Usadel, B. (2014). Trimmomatic: A Flexible Trimmer for Illumina Sequence Data. Bioinformatics 30 (15), 2114–2120. doi: 10.1093/bioinformatics/btu170
Bryrup, T., Thomsen, C. W., Kern, T., Allin, K. H., Brandslund, I., Jørgensen, N. R., et al. (2019). Metformin-Induced Changes of the Gut Microbiota in Healthy Young Men: Results of a Non-Blinded, One-Armed Intervention Study. Diabetologia 62 (6), 1024–1035. doi: 10.1007/s00125-019-4848-7
Callahan, B. J., McMurdie, P. J., Rosen, M. J., Han, A. W., Johnson, A. J. A., Holmes, S. P. (2016). DADA2: High-Resolution Sample Inference From Illumina Amplicon Data. Nat. Methods 13 (7), 581–583. doi: 10.1038/nmeth.3869
Cao, H., Liu, X., An, Y., Zhou, G., Liu, Y., Xu, M., et al. (2017). Dysbiosis Contributes to Chronic Constipation Development via Regulation of Serotonin Transporter in the Intestine. Sci. Rep. 7 (1), 1–12. doi: 10.1038/s41598-017-10835-8
Chao, A. (1984). Nonparametric Estimation of the Number of Classes in a Population. Scandinavian J. Statistics 11 (4), 265–270. doi: 10.1016/j.jesp.2010.02.007
Chong, B. S., Mersfelder, T. L. (2000). Entacapone. Ann. Pharmacother. 34 (9), 1056–1065. doi: 10.1345/aph.19328
Cirstea, M. S., Yu, A. C., Golz, E., Sundvick, K., Kliger, D., Radisavljevic, N., et al. (2020). Microbiota Composition and Metabolism Are Associated With Gut Function in Parkinson's Disease. Movement Disord. 35 (7), 1208–1217. doi: 10.1002/mds.28052
Dieterich, W., Schink, M., Zopf, Y. (2018). Microbiota in the Gastrointestinal Tract. Med. Sci. 6 (4), 116. doi: 10.3390/medsci6040116
Douglas, G. M., Maffei, V. J., Zaneveld, J. R., Yurgel, S. N., Brown, J. R., Taylor, C. M., et al. (2020). PICRUSt2 for Prediction of Metagenome Functions. Nat. Biotechnol. 38 (6), 685–688. doi: 10.1038/s41587-020-0548-6
Grün, D., Zimmer, V. C., Kauffmann, J., Spiegel, J., Dillmann, U., Schwiertz, A., et al. (2020). Impact of Oral COMT-Inhibitors on Gut Microbiota and Short Chain Fatty Acids in Parkinson's Disease. Parkinsonism Related Disord. 70, 20–22. doi: 10.1016/j.parkreldis.2019.11.020
Haddad, F., Sawalha, M., Khawaja, Y., Najjar, A., Karaman, R. (2018). Dopamine and Levodopa Prodrugs for the Treatment of Parkinson’s Disease. Molecules. 23 (1), 40. doi: 10.3390/molecules23010040
Jackson, M. A., Verdi, S., Maxan, M. E., Shin, C. M., Zierer, J., Bowyer, R. C., et al. (2018). Gut Microbiota Associations With Common Diseases and Prescription Medications in a Population-Based Cohort. Nat. Commun. 9 (1), 1–8. doi: 10.1038/s41467-018-05184-7
Kaakkola, S. (2000). Clinical Pharmacology, Therapeutic Use and Potential of COMT Inhibitors in Parkinson’s Disease. Drugs. 59 (6), 1233–1250. doi: 10.2165/00003495-200059060-00004
Kanehisa, M., Goto, S. (2000). KEGG: Kyoto Encyclopedia of Genes and Genomes. Nucleic Acids Res. 28 (1), 27–30. doi: 10.1093/nar/28.1.27
Lance, G. N., Williams, W. T. (1967). Mixed-Data Classificatory Programs I - Agglomerative Systems. Aust. Comput. J. 1 (1), 15–20.
Lebouvier, T., Chaumette, T., Paillusson, S., Duyckaerts, C., Bruley des Varannes, S., Neunlist, M., et al. (2009). The Second Brain and Parkinson’s Disease. Eur. J. Neurosci. 30 (5), 735–741. doi: 10.1111/j.1460-9568.2009.06873.x
Lewis, J. D., Chen, E. Z., Baldassano, R. N., Otley, A. R., Griffiths, A. M., Lee, D., et al. (2015). Inflammation, Antibiotics, and Diet as Environmental Stressors of the Gut Microbiome in Pediatric Crohn’s Disease. Cell Host Microbe 18 (4), 489–500. doi: 10.1016/j.chom.2015.09.008
Li, H., Chen, J., Ren, X., Yang, C., Liu, S., Bai, X., et al. (2020). Gut Microbiota Composition Changes in Constipated Women of Reproductive Age. Front. Cell. Infection Microbiol. 10:557515. doi: 10.3389/fcimb.2020.557515
Li, J., Lou, Z., Liu, X., Sun, Y., Chen, J. (2017). Efficacy and Safety of Adjuvant Treatment With Entacapone in Advanced Parkinson’s Disease With Motor Fluctuation: A Systematic Meta-Analysis. Eur. Neurol. 78 (3-4), 143–153. doi: 10.1159/000479555
Lozupone, C., Lladser, M. E., Knights, D., Stombaugh, J., Knight, R. (2011). UniFrac: An Effective Distance Metric for Microbial Community Comparison. ISME J. 5 (2), 169–172. doi: 10.1038/ismej.2010.133
Magurran, A. E. (1988). Ecological Diversity and Its Measurement. (Princeton, NJ:Princeton University Press).
Maier, L., Pruteanu, M., Kuhn, M., Zeller, G., Telzerow, A., Anderson, E. E., et al. (2018). Extensive Impact of Non-Antibiotic Drugs on Human Gut Bacteria. Nature 555 (7698), 623–628. doi: 10.1038/nature25979
Mancabelli, L., Milani, C., Lugli, G. A., Turroni, F., Ferrario, C., van Sinderen, D., et al. (2017). Meta-Analysis of the Human Gut Microbiome From Urbanized and Pre-Agricultural Populations. Environ. Microbiol. 19 (4), 1379–1390. doi: 10.1111/1462-2920.13692
Marquezi, M. L., Roschel, H. A., dos Santos Costa, A., Sawada, L. A., Lancha, A. H., Jr. (2003). Effect of Aspartate and Asparagine Supplementation on Fatigue Determinants in Intense Exercise. Int. J. Sport Nutr. Exercise Metab. 13 (1), 65–75. doi: 10.1123/ijsnem.13.1.65
McMurdie, P. J., Holmes, S. (2013). Phyloseq: An R Package for Reproducible Interactive Analysis and Graphics of Microbiome Census Data. PloS One 8 (4), e61217. doi: 10.1371/journal.pone.0061217
Mirghafourvand, M., Rad, A. H., Charandabi, S. M. A., Fardiazar, Z., Shokri, K. (2016). The Effect of Probiotic Yogurt on Constipation in Pregnant Women: A Randomized Controlled Clinical Trial. Iranian Red Crescent Med. J. 18 (11), e39870. doi: 10.5812/ircmj.39870
Nourrisson, C., Scanzi, J., Pereira, B., NkoudMongo, C., Wawrzyniak, I., Cian, A., et al. (2014). Blastocystis Is Associated With Decrease of Fecal Microbiota Protective Bacteria: Comparative Analysis Between Patients With Irritable Bowel Syndrome and Control Subjects. PloS One 9 (11), e111868. doi: 10.1371/journal.pone.0111868
Ohkusa, T., Koido, S., Nishikawa, Y., Sato, N. (2019). Gut Microbiota and Chronic Constipation: A Review and Update. Front. Med. 6, 19. doi: 10.3389/fmed.2019.00019
Olgun, A. (2017). " Metformin-Resistant" Folic Acid Producing Probiotics or Folic Acid Against Metformin's Adverse Effects Like Diarrhea. Med. Hypotheses. 106, 33–34. doi: 10.1016/j.mehy.2017.07.009
Parks, D., Beiko, R. (2013). STAMP: Statistical Analysis of Metagenomic Profiles. Encyclopedia Metagenomics. New York, NY, USA:Springer 2013, 1–6. doi: 10.1007/978-1-4614-6418-1_780-1
Passos, M., Moraes-Filho, J. P. (2017). Intestinal Microbiota in Digestive Diseases. Arquivos Gastroenterologia 54, 255–262. doi: 10.1590/s0004-2803.201700000-31
Rinninella, E., Raoul, P., Cintoni, M., Franceschi, F., Miggiano, G. A. D., Gasbarrini, A., et al. (2019). What is the Healthy Gut Microbiota Composition? A Changing Ecosystem Across Age, Environment, Diet, and Diseases. Microorganisms 7 (1), 14. doi: 10.3390/microorganisms7010014
Rosenzweig, M. L. (1995). Species Diversity in Space and Time. (Cambridge, UK:Cambridge University Press).
Schrag, A. (2005). Entacapone in the Treatment of Parkinson's Disease. Lancet Neurol. 4 (6), 366–370. doi: 10.1016/S1474-4422(05)70098-3
Stout, J., Cramer, J., Zoeller, R., Torok, D., Costa, P., Hoffman, J., et al. (2007). Effects of β-Alanine Supplementation on the Onset of Neuromuscular Fatigue and Ventilatory Threshold in Women. Amino Acids 32 (3), 381–386. doi: 10.1007/s00726-006-0474-z
Unger, M. M., Spiegel, J., Dillmann, K. U., Grundmann, D., Philippeit, H., Bürmann, J., et al. (2016). Short Chain Fatty Acids and Gut Microbiota Differ Between Patients With Parkinson's Disease and Age-Matched Controls. Parkinsonism Related Disord. 32, 66–72. doi: 10.1016/j.parkreldis.2016.08.019
Vila, A. V., Collij, V., Sanna, S., Sinha, T., Imhann, F., Bourgonje, A. R., et al. (2020). Impact of Commonly Used Drugs on the Composition and Metabolic Function of the Gut Microbiota. Nat. Commun. 11 (1), 1–11. doi: 10.1038/s41467-019-14177-z
Wang, H. (2021). MicroRNAs, Parkinson’s Disease, and Diabetes Mellitus. Int. J. Mol. Sci. 22 (6), 2953. doi: 10.3390/ijms22062953
Wang, L., Chen, C., Cui, S., Lee, Y. K., Wang, G., Zhao, J., et al. (2019). Adhesive Bifidobacterium Induced Changes in Cecal Microbiome Alleviated Constipation in Mice. Front. Microbiol. 10, 1721. doi: 10.3389/fmicb.2019.01721
Wang, W., Chen, L., Zhou, R., Wang, X., Song, L., Huang, S., et al. (2014). Increased Proportions of Bifidobacterium and the Lactobacillus Group and Loss of Butyrate-Producing Bacteria in Inflammatory Bowel Disease. J. Clin. Microbiol. 52 (2), 398–406. doi: 10.1128/JCM.01500-13
Wang, B., Yao, M., Lv, L., Ling, Z., Li, L. (2017). The Human Microbiota in Health and Disease. Engineering 3 (1), 71–82. doi: 10.1016/J.ENG.2017.01.008
Weersma, R. K., Zhernakova, A., Fu, J. (2020). Interaction Between Drugs and the Gut Microbiome. Gut 69 (8), 1510–1519. doi: 10.1136/gutjnl-2019-320204
Weis, S., Schwiertz, A., Unger, M. M., Becker, A., Faßbender, K., Ratering, S., et al. (2019). Effect of Parkinson’s Disease and Related Medications on the Composition of the Fecal Bacterial Microbiota. NPJ Parkinson's Dis. 5 (1), 1–9. doi: 10.1038/s41531-019-0100-x
Xia, R., Mao, Z.-H. (2012). Progression of Motor Symptoms in Parkinson’s Disease. Neurosci. Bulletin 28 (1), 39–48. doi: 10.1007/s12264-012-1050-z
Zhernakova, A., Kurilshikov, A., Bonder, M. J., Tigchelaar, E. F., Schirmer, M., Vatanen, T., et al. (2016). Population-Based Metagenomics Analysis Reveals Markers for Gut Microbiome Composition and Diversity. Science 352 (6285), 565–569. doi: 10.1126/science.aad3369
Zhu, L., Liu, W., Alkhouri, R., Baker, R. D., Bard, J. E., Quigley, E. M., et al. (2014). Structural Changes in the Gut Microbiome of Constipated Patients. Physiol. Genomics 46 (18), 679–686. doi: 10.1152/physiolgenomics.00082.2014
Zimmermann, M., Zimmermann-Kogadeeva, M., Wegmann, R., Goodman, A. L. (2019). Mapping Human Microbiome Drug Metabolism by Gut Bacteria and Their Genes. Nature 570 (7762), 462–467. doi: 10.1038/s41586-019-1291-3
Keywords: Parkinson’s disease, microbiome, entacapone, constipation, drowsiness, levodopa
Citation: Fu S-C, Lee C-H, Hsieh Y-C, Wu P-H, Lin S-H and Wang H (2022) A Pilot Study Exploring the Association of Entacapone, Gut Microbiota, and the Subsequent Side Effects in Patients With Parkinson’s Disease. Front. Cell. Infect. Microbiol. 12:837019. doi: 10.3389/fcimb.2022.837019
Received: 16 December 2021; Accepted: 10 February 2022;
Published: 05 April 2022.
Edited by:
Intawat Nookaew, University of Arkansas for Medical Sciences, United StatesReviewed by:
Sawannee Sutheeworapong, King Mongkut’s University of Technology Thonburi, ThailandNatthaya Chuaypen, Chulalongkorn University, Thailand
Copyright © 2022 Fu, Lee, Hsieh, Wu, Lin and Wang. This is an open-access article distributed under the terms of the Creative Commons Attribution License (CC BY). The use, distribution or reproduction in other forums is permitted, provided the original author(s) and the copyright owner(s) are credited and that the original publication in this journal is cited, in accordance with accepted academic practice. No use, distribution or reproduction is permitted which does not comply with these terms.
*Correspondence: Sheng-Hsuan Lin, c2hlbmdsaW5Ac3RhdC5uY3R1LmVkdS50dw==; Hsiuying Wang, d2FuZ0BzdGF0Lm55Y3UuZWR1LnR3