- 1College of Life Science and Technology, Beijing University of Chemical Technology, Beijing, China
- 2State Key Laboratory of Pathogen and Biosecurity, Beijing Institute of Microbiology and Epidemiology, Beijing, China
- 3Laboratory Animal Center, Academy of Military Medical Sciences, Beijing, China
Hypervirulent Klebsiella pneumoniae (hvKp) can cause life-threatening community-acquired infections among healthy young individuals and is thus of concern for global dissemination. In this study, a mouse model of acute primary hvKp pneumonia was established via aerosolized intratracheal (i.t.) inoculation, laying the foundation for conducting extensive studies related to hvKp. Subsequently, a time-course transcriptional profile was created of the lungs from the mouse model at 0, 12, 24, 48 and 60 hours post-infection (hpi) using RNA Sequencing (RNA-Seq). RNA-Seq data were analyzed with the use of Mfuzz time clustering, weighted gene co-expression network analysis (WGCNA) and Immune Cell Abundance Identifier for mouse (ImmuCellAI-mouse). A gradual change in the transcriptional profile of the lungs was observed that reflected expected disease progression. At 12 hpi, genes related to acute phase inflammatory response increased in expression and lipid metabolism appeared to have a pro-inflammatory effect. At 24 hpi, exacerbation of inflammation was observed and active IFN-γ suggested that signaling promoted activation and recruitment of macrophages occurred. Genes related to maintaining the structural integrity of lung tissues showed a sustained decrease in expression after infection and the decrease was especially marked at 48 hpi. TNF, IL-17, MAPK and NF-kB signaling pathways may play key roles in the immunopathogenesis mechanism at all stages of infection. Natural killer (NK) cells consistently decreased in abundance after infection, which has rarely been reported in hvKp infection and could provide a new target for treatment. Genes Saa1 and Slpi were significantly upregulated during infection. Both Saa1, which is associated with lipopolysaccharide (LPS) that elicits host inflammatory response, and Slpi, which encodes an antimicrobial protein, have not previously been reported in hvKp infections and could be important targets for subsequent studies. To t our knowledge, this paper represents the first study to investigate the pulmonary transcriptional response to hvKp infection. The results provide new insights into the molecular mechanisms underlying the pathogenesis of hvKp pulmonary infection that can contribute to the development of therapies to reduce hvKp pneumonia.
Introduction
Klebsiella pneumoniae is a Gram-negative commensal bacterium and opportunistic pathogen found ubiquitously in the environment. It often colonizes on human mucosal surfaces and causes various nosocomial infections in the respiratory tract, lung, urinary tract, wound sites and blood (Podschun and Ullmann, 1998). Moreover, it is one of the few Gram-negative bacteria capable of causing primary pneumonia (Restuccia and Cunha, 1984) and is a major cause of hospital-acquired pneumonia (Magill et al., 2018). K. pneumoniae strains are classified into two distinct pathotypes: classic K. pneumoniae (cKp) and hypervirulent K. pneumoniae (hvKp) (Catalán-Nájera et al., 2017; Russo and Marr, 2019). The majority of infections caused by K. pneumoniae come from the “classical” strains of cKp (Shon et al., 2013). In contrast to cKp, hvKp is more virulent and capable of causing severe organ or life-threatening infections, such as pneumonia, hepatic abscess, meningitis and necrotizing fasciitis, in healthy individuals from community settings (Fang et al., 2007; Lederman and Crum, 2005; Patel et al., 2014; Pomakova et al., 2012). Although community-acquired pneumonia (CAP) due to hvKp is uncommon (Choby et al., 2020), it is noteworthy that bacterial CAP due to hvKp has higher mortality and respiratory failure rates compared with Streptococcus pneumoniae, the leading cause of CAP globally (Lin et al., 2010). In the past few decades, hvKp has spread globally and the incidence of infections has been increasing steadily (Lederman and Crum, 2005; Pomakova et al., 2012). Hence, a better understanding of hvKp pulmonary infection is warranted.
Mechanisms of hvKp infection pathogenesis and hvKp-host interactions are complex. Lipopolysaccharide (LPS), a major component of the outer membrane of K. pneumoniae (Whitfield and Trent, 2014), is recognized by the key pattern recognition receptor, toll-like receptor 4 (TLR4), which triggers the innate immune response (Akira et al., 2006; Scott et al., 2017). One of the most prominent bacterial phenotypes associated with hvKp is overproduction of capsule polysaccharide (CPS) (Yu et al., 2008), which leads to a hypermucous phenotype. Overproduction of CPS impairs complement-mediated bacterial killing and phagocytosis via neutrophils and macrophages, which directly correlates with host resistance to hvKp (Cheng et al., 2010; Catalina et al., 2013). Macrophages are essential for controlling K. pneumoniae replication and regulating the inflammatory response at different tissue sites, such as the lung, liver and spleen (Olonisakin et al., 2021). Monocytes are heterogeneous cells capable of displaying proinflammatory or immuno-regulatory phenotypes, depending on the nature of the microenvironment at the site of infection (Peñaloza et al., 2019). Inflammatory monocytes are required to clear K. pneumoniae from the lung (Xiong et al., 2016), while anti-inflammatory monocytes are recruited to the lung at later stages of K. pneumoniae infection and appear to play a beneficial role by mediating the clearance of apoptotic neutrophils (Poe et al., 2013). Overall, these results indicate that hvKp infection may induce discriminatory gene expression patterns in different types of host cells with different effects on specific cell types.
The lung is both a major immune organ in vertebrates and an important target organ for hvKp infection. Thus, a detailed description of hvKp pulmonary infection is necessary to explore the mechanisms of hvKp-host interactions. RNA-Seq, a recently developed method for high-throughput transcriptome sequencing (Hong et al., 2017), can reveal dynamic changes in host gene expression during pathogen infection and has been used to study various viral infections and diseases (He et al., 2017; Arun et al., 2018). Based on the above, we performed time-course RNA sequencing of lung tissues from mice with primary hvKp pneumonia to investigate transcription profile changes in hvKp-infected lungs and to screen for genes or other valuable research targets. This study promotes our understanding of pathogenesis associated with hvKp pulmonary infection.
Materials and Methods
Bacterial Strain and Growth Conditions
While no molecular diagnostic nor microbiological consensus exists for the definition of hypervirulence (Harada and Doi, 2018; Shan et al., 2006), the NTUH-K2044 hvKp strain has been recognized as hypervirulent given it possesses the magA and rmpA genes, belongs to capsular serotype K1, has high virulence and hypermucoviscosity (Fang et al., 2004; Chuang et al., 2006; Yu et al., 2006; Yeh et al., 2007). In this study, hvKp strain NTUH-K2044 was inoculated into Brain Heart Infusion (BHI) broth (BD Biosciences, Lawrence, KS) and grown overnight at 37°C with continuous shaking at 220 rpm. The overnight culture was diluted with BHI at 1:200 and incubated at 37°C with shaking for 3 h to achieve OD600 = 1.5. Then the culture was further diluted and incubated for another 2.5 h. The final culture was centrifuged, washed and resuspended in phosphate-buffered saline (PBS) to achieve OD600 = 1.0, a concentration of about 6 x 108 CFU/mL. The actual infection dose in each experiment was determined by serial dilution plating on BHI agar.
Mice Infection
Female C57BL/6Cnc mice aged 6-8 weeks were purchased from Vital River Laboratories (Beijing, China). Mice were exposed to aerosolized hvKp (2 x 104 CFU in 50 μL PBS per mouse) via aerosolized intratracheal inoculation as previously described (Feng et al., 2019). Briefly, each mouse was deeply anesthetized with pentobarbital sodium, a Micro Sprayer (Huironghe Company, Beijing, China) was inserted into the tracheal bifurcation of the mouse and hvKp aerosol was then generated by the Micro Sprayer and sprayed into the lung. Following exposure, survival was monitored twice daily for 14 days. Animals were randomly divided into four infection groups and one control group (five mice per group), and the infected mice were euthanized at 12, 24, 48 and 60 h post-infection (hpi) while the control group mice were euthanized immediately after delivery of PBS at the 0 h time point. The lungs of the mice were isolated and divided into two parts for subsequent histopathologic examination or total RNA extraction. All animal experiments were performed in the Laboratory Animal Center of Academy of Military Medical Science (AMMS), approved by the Animal Care and Use Committee (IACUC) of AMMS, and the ethical approval number was IACUC-DWZX-2020-050.
Histopathological Validation
Collected lungs were fixed in 4% paraformaldehyde, and the fixed tissues sliced, mounted on slides, and stained with hematoxylin-eosin (HE). Pathological changes in tissue slices were observed by light microscopy (BX60, Olympus, Japan). Tissue sections were evaluated by a trained pathologist (blind to treatment) according to the following scores: 0, no pathological lesions; 1, minimal; 2, mild; 3, moderate; 4, severe. The degree of pathological lesions was related to the distribution of lesions as follows: inflammatory cell infiltration, edema, congestion, and tissue necrosis. Pathology scores were assessed using one-way multilevel ANOVA in SAS 9.3.
RNA Extraction, Library Preparation, and Sequencing
The collected lungs were submerged in RNAlater stabilization solution (Invitrogen, Carlsbad, CA, USA) and total RNA was extracted using an RNA purification kit (Invitrogen, Carlsbad, CA). The concentration and purity of the extracted RNA were measured using a Nanodrop 2000c spectrophotometer (Thermo Fisher, Waltham, Massachusetts, USA). Library construction and sequencing were conducted by the Novogene Company in Beijing, China. No less than 1 μg of RNA per sample was used as the input material for RNA sample preparation. Sequencing libraries were generated using NEBNext® Ultra TMRNA Library Prep Kit for Illumina® (NEB, Ipswich, MA, USA). The library preparations were sequenced using the Illumina sequencing platform (HiSeq™ 2500, Illumina, San Diego, California, USA), sequenced reads from all samples were mapped to the reference genome (mouse) using Hisat2 v2.0.5, and then fragments per kilobase of exon per million fragments mapped (FPKM) values were calculated for each gene based on gene length. FPKM (Florea et al., 2013) was used as the unit of measurement to estimate transcript abundance.
Identification of DEGs and Bioinformatics Analysis
To normalize the RNA-Seq data, differential expression analysis was performed using the edgeR package in R (Smyth, 2010). Triplicates of the RNA-Seq experiments were analyzed separately and resulting p-value were adjusted using Benjamini and Hochberg’s approach for controlling false discovery rate (FDR) (Love et al., 2014). The selection criteria for differentially expressed genes (DEGs) in this study was an adjusted p–value <0.05 and an absolute fold change >2 or <0.5. To assess quality of the data, principal component analysis (PCA) was used to examine the distribution of samples. Based on the Gene Ontology (GO) database (http://geneontology.org/) and Kyoto Encyclopedia of Genes and Genomes (KEGG) database (https://www.genome.jp/kegg/), the Clusterprofiler (Guangchuang et al., 2012) package of R was applied to perform functional enrichment and pathway analysis, with corrected p-value <0.05 considered significantly enriched.
Time Series Gene Clustering
Soft cluster analysis using the fuzzy C-Means algorithm in the Mfuzz package (Futschik and Carlisle, 2011; Kumar and Futschik, 2007) was conducted to assign genes to clusters according to the expression pattern of DEGs. The number of clusters was set to nine and the fuzzifier coefficient, M, was set to 1.71.
Weighted Gene Co-Expression Network Analysis (WGCNA)
To identify modules with different expression patterns, a weighted correlation network analysis (WGCNA) was conducted for the 15 lung samples using the WGCNA package (Langfelder and Horvath, 2009) in R. The WGCNA input data were normalized values for each transcript and correlations between any two genes were first collected and analyzed by Pearson correlation coefficients to form a similarity matrix (Ivliev et al., 2010). At the same time, the topological overlap matrix (TOM) method was employed to take both direct and indirect relationships into account. Then, the hierarchical clustering tree was used to generate a division of gene modules based on the TOM values between genes. The module with the highest correlation for the sample characteristics was selected for further analysis.
Immune Infiltration Analysis
Immune Cell Abundance Identifier (ImmuCellAI) is a tool to accurately estimate immune cell abundance from gene expression datasets, including RNA-Seq and microarray data (Miao et al., 2020). Immune Cell Abundance Identifier for mouse (ImmuCellAI-mouse) is used as a complement to ImmuCellAI to estimate the abundance of 36 immune cell (sub)types in mouse transcriptome data (Miao et al., 2021). ImmuCellAI-mouse simulated the process of flow cytometry analysis by adopting a hierarchical strategy, dividing 36 cell types into three layers. Layer 1 is composed of seven major immune cell types: B cells, monocytes, dendritic cells (DCs), natural killer (NK) cells, granulocytes, macrophages and T cells. Cells in layer 2 are subtypes of cells in the first layer, including subtypes of B cells (B1, follicular B, germinal center B, marginal zone B, memory B and plasma B cells), subtypes of DCs (cDC1, cDC2, MoDC and pDC cells), subtypes of granulocytes (basophil, eosinophil, mast cell and neutrophils), subtypes of macrophages (M1 and M2 macrophages) and subtypes of T cells (CD4 T, CD8 T, NKT and gamma-delta T cells). Finally, cells in layer 3 are subtypes of CD4 T and CD8 T cells (including CD4+ naïve, CD4+ memory, Treg, T helper, CD8+ naïve, CD8+ central memory, CD8+ effector memory, cytotoxic and exhausted cells).
Validation of RNA-Seq by qRT-PCR
To validate the results of the RNA-Seq data, 12 DEGs were randomly selected for qRT-PCR validation based on their expression patterns at four time points. RNA samples were reverse-transcribed into cDNA using the TransScript One-Step gDNA Removal and cDNA Synthesis SuperMix (TransGen Biotech, Beijing, China). Primers used in this study have been previously published or can be found in PrimerBank (https://pga.mgh.harvard.edu/primerbank/); primer sequences are listed in Table 1. qRT-PCR was performed using the LightCycler 96 RT-PCR Detection System (Roche, Basel, Switzerland). Each reaction mixture was 20 μL in total, containing 10 μL of SYBR qPCR Master Mix (QIAGEN, Dusseldorf, Germany), 0.7 μL of each upstream and downstream primers (7 μM), 1 μL of cDNA template, and 7.6 μL of ddH2O. The following reaction procedure was used: 95°C for 120 s, then 42 cycles at 95°C for 15 s and 60°C for 30 s. Each experiment was performed in triplicate. The relative expression values of selected genes were calculated using the 2-ΔΔCt method and normalized against expression levels of the β-actin gene. Correlation between the RNA-Seq and qRT-PCR data was analyzed using Pearson’s correlation coefficient.
Results
Histopathological Changes in the Lungs
To explore the influence of hvKp on the survival time of mice, we recorded death and survival events for 14 days after infection. Mice started to die in large numbers 2.5 days after infection, with a mortality rate of 80%; at 4 days post-infection, mortality rate was 100% (Figure 1A). Therefore, we chose the five time points of 0, 12, 24, 48 and 60 hpi for subsequent experiments and analysis. Histopathological changes in HE-stained lung tissues revealed an intense and continuously increased inflammatory cell infiltration composed of neutrophils and monocytes during the infection (Figure 1B, 12-60 hpi). At the last stage of infection (60 hpi), most alveolar cavities were filled with edematous fluid, lung tissue was extensively hemorrhaged and tissue structures were locally destroyed (Figure 1A, 60 hpi). These pathological changes were absent in the lungs of control group mice (Figure 1C, 0 hpi). Histological scoring also revealed that the severity of lung lesions increased over time (Figure 1B). Histopathological validation indicated that hvKp caused excessive acute inflammation and severe lung injury in mice. Hence, the mouse model of acute primary hvKp pneumonia was successfully established, and all subsequent experiments were performed based on this animal model.
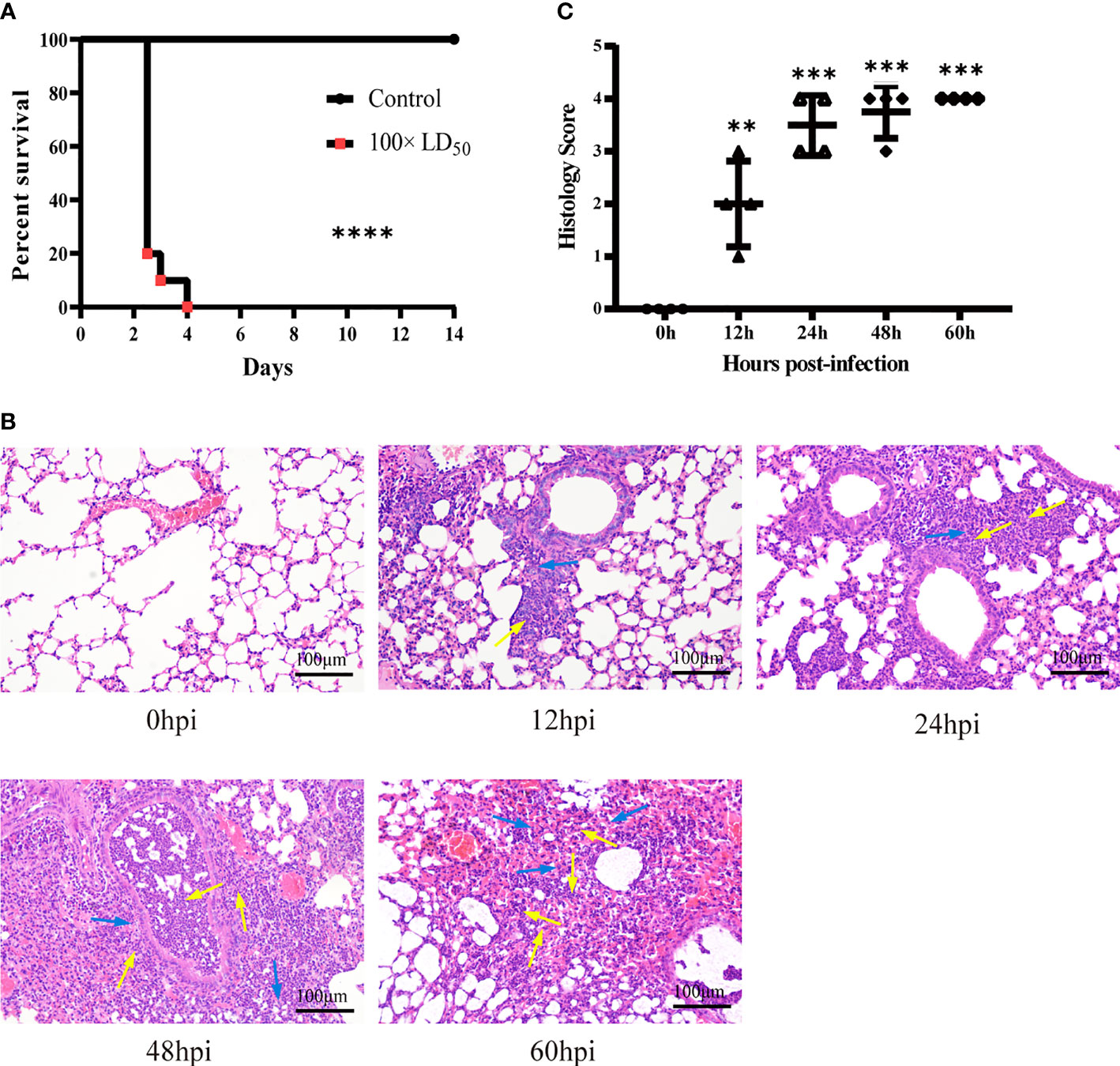
Figure 1 Histopathological analysis of lung tissue from mice infected with hvKp. Mice were challenged with 100 × LD50 (20000 CFU) hypervirulent Klebsiella pneumoniae strain (NTUH-K2044), and then the lungs were stained with hematoxylin-eosin (HE). (A) Survival curves of mice in the infected and control groups (n = 10 per group). ****p < 0.0001, compared with control group. (B) Pathological changes in the lungs at 0, 12, 24, 48 and 60 hours post-infection. Arrows show the infiltration of inflammatory cells; monocytes shown with blue arrows and neutrophils shown with yellow arrows (original magnification = 200×; scale bar = 100 μm). (C) Pathological scores of the lung sections; symbols show individual mouse lung scores, horizontal and vertical lines indicate mean and standard deviations of group. Scoring standard: 0, no pathological lesions; 1, minimal; 2, mild; 3, moderate; 4, severe. The degree of pathological lesions was related to the distribution of lesions as follows: inflammatory cell infiltration, edema, congestion, and tissue necrosis. **P < 0.01, ***P < 0.001, compared with 0hpi.
Overview of Transcriptome Analysis
We isolated hvKp infected lung tissue for RNA-Seq and recorded gene expression profiles at each time point. To assess the quality of the data, principal component analysis (PCA) was used to examine the distribution of samples (Figure 2A). The first principal component (PC1) accounted for 39.8% of the total expression variance for the top 1000 most variable genes. The expression matrices of the control and hvKp infected groups separated out along the PC1 axis, with the control group found at one end of the axis, the 12 and 24 hpi groups clustered together distinctly away from the control group, and the 48 and 60 hpi groups also clustered together and even further along the axis away from the control group. Thus, difference in gene expression profiles after hvKp infection indicate an altered transcriptional profile intrinsic to the lung.
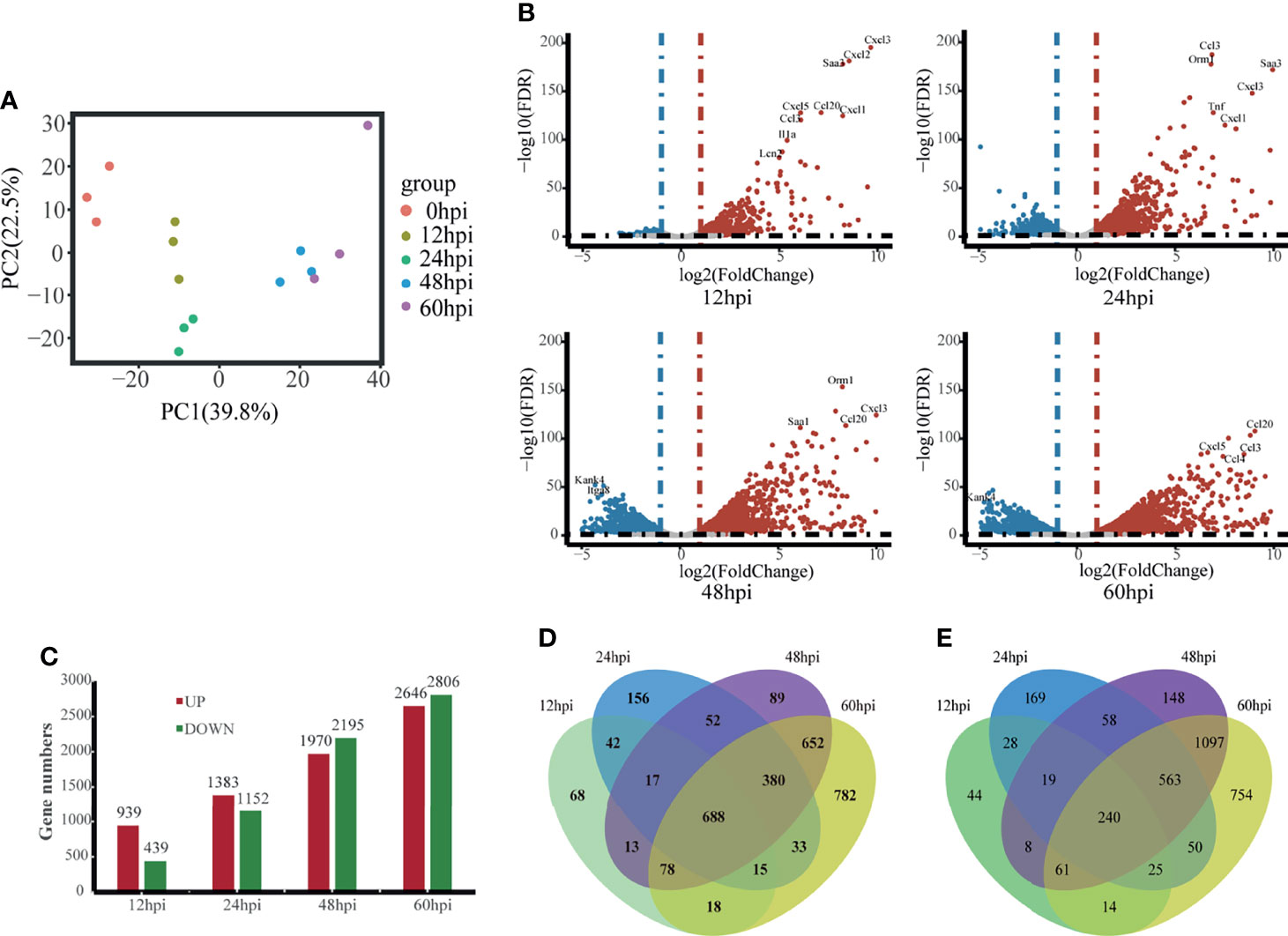
Figure 2 Overview of time-course transcriptome analysis based on the value of FPKM of all groups. (A) PCA of the normalized RNA-Seq data of lung tissues in response to hvKp infection. The same color represents the same stages in replicates. (B) Volcano plot of RNA-Seq transcriptome data displaying the pattern of gene expression. Significantly differentially expressed genes (DEGs, FDR P ≤ 0.05) are highlighted in red (up-regulated) or blue (down-regulated). Curated genes with specialized biological functions are indicated with labels. (C) Number of DEGs at different time points. (D) Venn diagram comparing the up-regulated DEGs. (E) Venn diagram comparing the Down-regulated DEGs. All transcriptome experiments were performed in biological triplicate.
Using the control group (0 hpi) as a reference, 6247 DEGs were identified across four time points after hvKp infection. At 12 hpi, 939 upregulated DEGs and 439 downregulated DEGs were detected, and by 60 hpi, 2647 upregulated DEGs and 2806 downregulated DEGs were detected. Overall, the number of upregulated and downregulated DEGs increased over time (Figures 2B, C) and a total of 688 genes were upregulated and 240 genes downregulated in common for all time points (Figures 2D, E). The fold-change and FDR of the top 20 genes in common with the most significant expression changes in upregulated genes are shown in Table 2. Some of these significantly upregulated genes, such as Csf3, Timp1, Slc39a14 and Cxcl3, are associated with innate immune responses including immune cell differentiation and migration, while some genes, such as Aoah, Slpi and Saa1, are closely associated with inflammatory responses caused by Gram-negative bacteria. Numerous genes significantly upregulated at 12 hpi are associated with inflammatory response, such as chemokines (e.g., Cxcl3, Cxcl2, Cxcl5, Cxcl1, Ccl20 and Ccl3), cytokines IL1α and other acute phase molecules (e.g., Saa3 and Lcn2; Figure 2B). Genes associated with the control of cytoskeleton formation, such as Kank4 and Itga8, were significantly down-regulated at later stages (≥48 hpi). All results suggest a gradual change in the transcription profile of the lung over time.
Analysis of Expression Patterns of DEGs
To get a more holistic view, DEGs were clustered into nine clusters according to their temporal expression patterns (Figure 3A) and the functional processes associated with each temporal cluster evaluated in a GO enrichment analysis (Figure 3B). Few functional processes were commonly enriched, indicating that the gene sets identified by Mfuzz have unique functions. We also found that identified processes were in keeping with the molecular pathophysiology of disease progression.
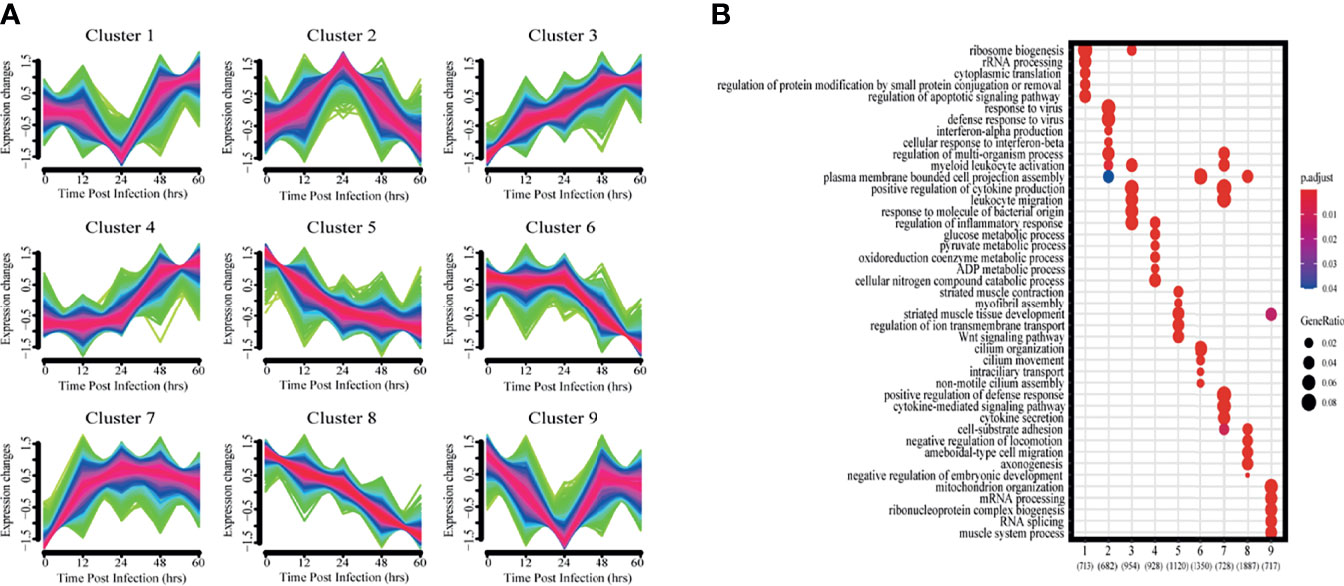
Figure 3 Clustering analysis of expression patterns based on DEGs. (A) Clustering by Mfuzz identified nine distinct temporal patterns of gene expression. Red indicates the variation of gene is more conformed to the center of the cluster, followed by blue, and finally green. (B) Heatmap shows the significance of the gene ontology (GO) terms in the biological processes describing each of the nine clusters. The number of enriched genes belonging to each cluster is shown in parentheses.
Clusters 1 and 9 showed a trend of decreasing gene expression levels before reaching a low point at 24 hpi and then increasing. GO analysis showed that 713 and 717 genes in clusters 1 and 9, respectively, were enriched for biological processes related to ribosomes, transcription and translation, including rRNA or mRNA processing, cytoplasmic translation and ribonucleoprotein complex biogenesis.
Cluster 2 gene expression levels peaked at 24 hpi. GO analysis revealed that these 682 genes enrich for biological processes related to interferon immune responses, such as interferon-α production and cellular responses to interferon-β.
Clusters 3 and 7 had gene expression levels that began increasing immediately after infection. GO analysis showed that the 954 and 728 genes in clusters 3 and 7, respectively, were enriched for biological processes related to immune response regulation, including leukocyte activation and migration, positive regulation of cytokine production, cytokine-mediated signaling pathways, and cytokine secretion.
Cluster 4 gene expression levels increased starting at 24 hpi. GO analysis revealed that the 928 genes enrich for biological processes related to glycolysis metabolism, such as glucose, pyruvate, oxidative cofactors and ADP metabolism.
Clusters 5 and 8 gene expression levels decreased after infection. GO analysis revealed that the 1120 and 1887 genes in clusters 5 and 8, respectively, were enriched for biological processes related to normal physiological processes in the organism, including regulation of ion transport across membranes, the Wnt signaling pathway, and cell matrix adhesion.
Cluster 6 gene expression levels decreased continuously after 24 hpi. GO analysis revealed a total of 1350 genes enriched for biological processes associated with ciliary tissue, such as ciliary motility, ciliary transport, and non-motile cilia assembly.
Functional Modules Identified by Network Analysis
WGCNA was performed with the detected DEGs. The dynamic tree cutting algorithm in the WGCNA package was used to process the hierarchical clustering tree, and a total of 28 different modules were finally obtained. The gray modules are the default modules and include discarded genes that could not be clustered, and the rest of the modules were named by randomly assigned colors (Figure 4A). Next, we calculated the correlation between the infection process (hpi) and the module genes (Figure 4B). To assess the importance of modules for our study, enrichment analysis was performed for modules with correlation coefficients greater than 0.5.
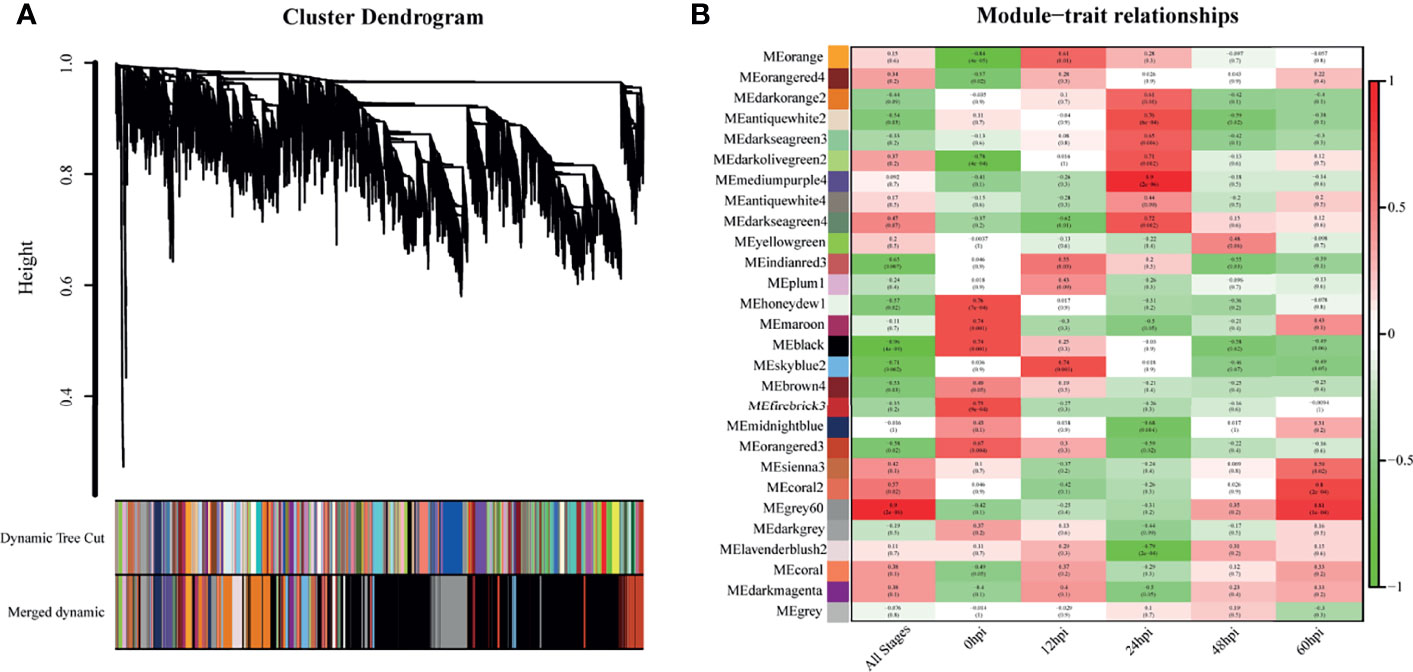
Figure 4 Genes modules in weighted gene co-expression network analysis (WGCNA). (A) Hierarchical clustering tree (Cluster Dendrogram) indicates the co-expression modules identified by WGCNA at different infection stages. The branches correspond to modules of highly interconnected groups of genes. The height (y-axis) indicates the co-expression distance and the x-axis corresponds to genes. Colors represent the 28 different modules along with gray indicating genes that could not be assigned to any module. (B) A heatmap chart showing module-trait relationships. Red denotes a positive correlation and Blue indicates a negative correlation between the module and infection stage.
The skyblue2 module was highly positively correlated with 12 hpi. GO analysis revealed that this module was mainly enriched in lipid-related biological processes including lipid biosynthetic processes, lipid metabolic processes and catalytic activity. KEGG analysis revealed that the main enriched pathways are related to metabolic regulation such as metabolic pathways, steroid biosynthesis and fatty acid metabolism (Figure 5A).
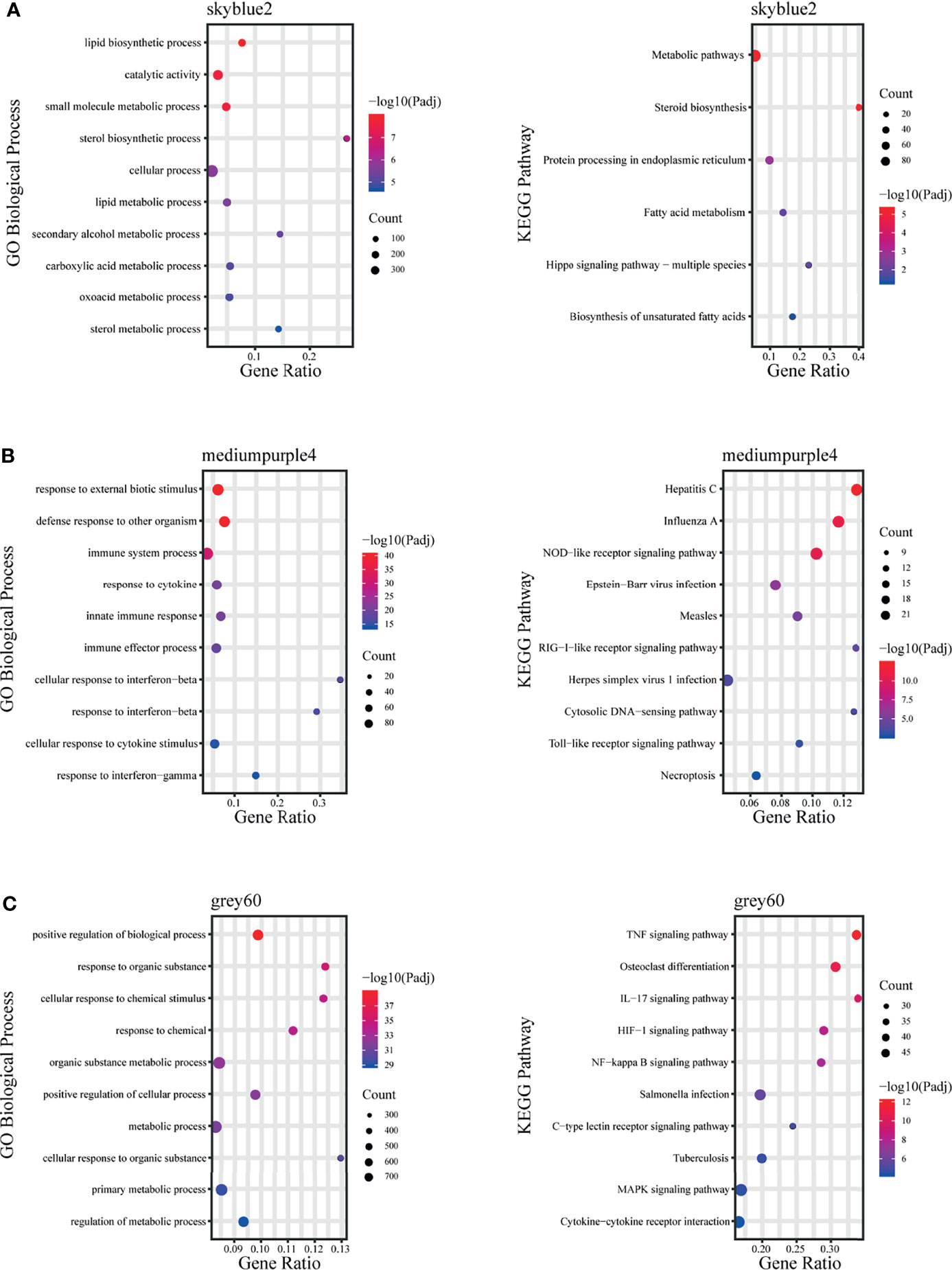
Figure 5 Fold enrichment of top-level overrepresented GO terms (biological process) and KEGG terms within modules that are highly positively correlated with different infection stages. (A) skyblue2 module, (B) mediumpurple4 module, (C) grey60 module.
The mediumpurple4 module played a key role at 24 hpi. GO analysis revealed that this module was mainly enriched in biological processes related to response to cytokines, including IFN-γ and IFN-β, and in intrinsic immune response to pathogens. KEGG analysis revealed that the main enriched pathways are related to pattern recognition receptors (PRRs), such as NOD-like receptor, RIG-I-like receptor and Toll-like receptor signaling pathways (Figure 5B).
The grey60 module was highly positively correlated with all time points, suggesting a key role at all stages. GO analysis revealed that this module was mainly enriched in biological processes, including positive regulation of biological process, response to organic substances and cellular response to chemical stimuli. KEGG analysis revealed enrichment mainly in signaling pathways such as TNF, IL-17, NF-kB, MAPK and HIF-1 (Figure 5C).
Immune Cell Abundance Analysis
To explore immune cell infiltration in lung tissue after infection, we used ImmuCellAI-mouse (Miao et al., 2021) to investigate variation in the infiltration of 36 immune cells among samples from different time points. ImmuCellAI-mouse divided the 36 cell types into three layers using a hierarchical strategy. Abundant immune cell populations with various kinds in each sample were shown in Figure 6A. As shown in Figure 6B, for the seven major immune cell types in layer 1, an overall decreasing trend with time occurred for B, NK and T cells, while an overall increasing trend occurred for granulocytes, macrophages and monocytes. This suggests innate immunity plays a dominant role after infection. Given this, we further considered the abundance of the 10 subtypes from the three major innate immune cells. Levels of neutrophils, M1 and M2 macrophages increased significantly (Figure 6C).
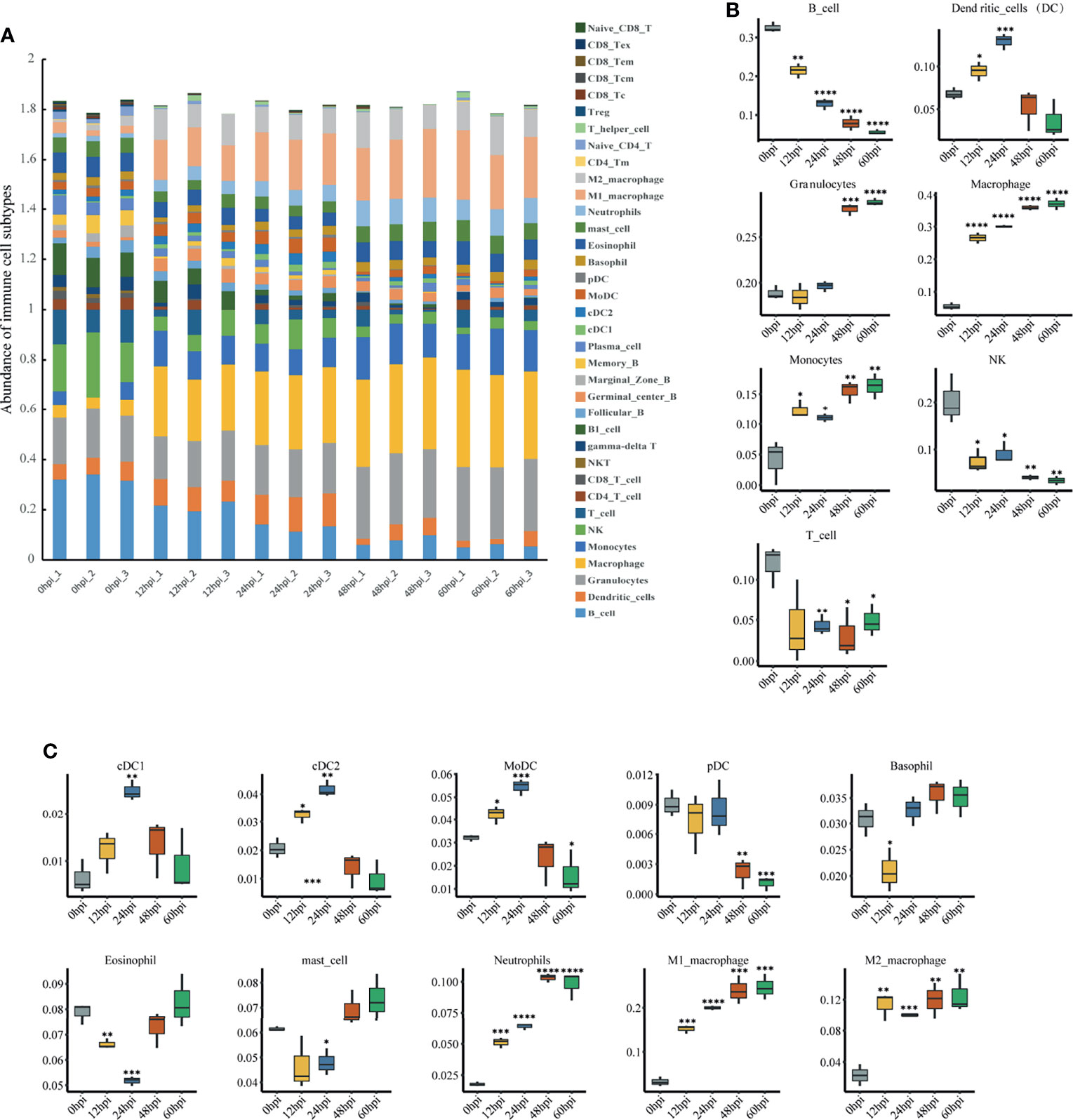
Figure 6 Immune cell infiltration analysis via ImmuCellAI-mouse. (A) Abundance of 36 immune cell types in all samples. (B) Abundance of the seven major immune cell types in layer 1 at different infection stages. (C) Abundance of 10 subtypes of the three major innate immune cell types in layer 2 at different infection stages. *P < 0.05, **P < 0.01, ***P < 0.001, ****P < 0.0001, compared with 0 hpi.
Quantitative RT-PCR Validation
To validate the reproducibility and repeatability of DEGs identified from transcriptome sequencing, 12 DEGs were randomly selected for qRT-PCR validation based on their expression patterns at four time points, namely, AA467197, Adamts4, Cyp27a1, Faim2, Igsf6, Serpine1, Fgfr4, Hpcal4, Timp1, Colq, Lbh and Slc38a5 (Figure 7A). These genes were significantly differentially expressed and consistently upregulated or downregulated with gene expression changes based on RNA-Seq. Correlation was measured using log2 (fold changes) between RNA-Seq and qRT-PCR; a high correlation coefficient (R2) of 0.9548 (Figure 7B) confirmed reliability of the transcriptome sequencing data.
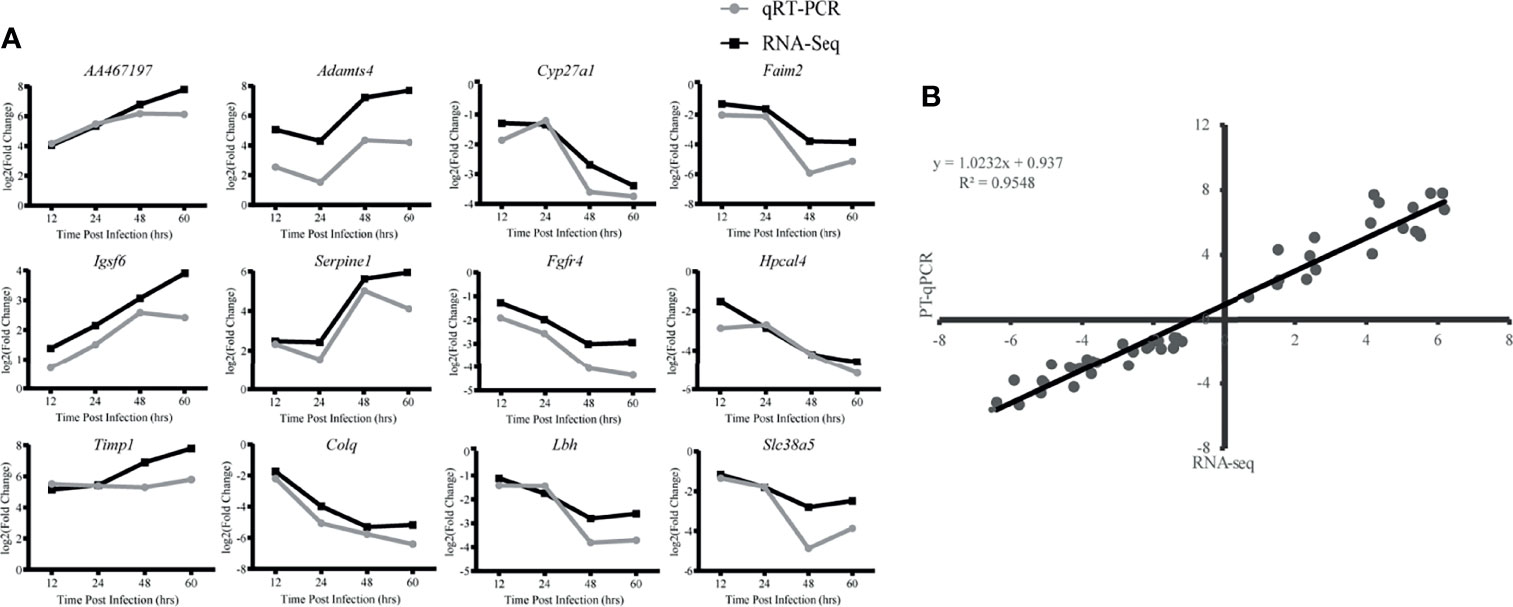
Figure 7 Validation of RNA-Seq data by qRT-PCR. (A) Twelve differentially expressed genes (DEG) were selected for verification. Relative quantity of gene expression (fold change) for each gene was calculated with the comparative 2-ΔΔCT method. The y-axis shows the fold changes of different infection stages compared to the beginning point, with positive values indicating up-regulation and negative values indicating down-regulation. Each data point was obtained from three biological replicates. (B) Correlation of fold change analyzed by data obtained using qRT-PCR (x axis) with RNA-Seq platform (y axis). Correlation analysis was performed using GraphPad software 8.0.
Discussion
Massive inflammation characterized by polymorphonuclear neutrophils and edema is a typical feature of pneumonia in mice caused by K. pneumoniae (Bengoechea and Pessoa, 2018). In this study, typical symptoms of pneumonia caused by K. pneumoniae were observed in mice within 60 hours after exposure, successfully validating the hvKp pulmonary infection mouse model. Using this model, we then investigated transcriptome profile changes in the lungs of infected mice at different time points to increase our understanding of hvKp pulmonary infection and to screen for genes or targets of research value.
Infection is a dynamic process, and temporal expression pattern clustering can effectively characterize gene expression. To get insight into the gene expression pattern during hvKp pulmonary infection, multiple bioinformatics analysis methods including Mfuzz time clustering, WGCNA and ImmuCellAI-mouse were comprehensively applied in this study. Mfuzz can cluster DEGs from different time points to show their progressive expression dynamics. WGCNA, based on gene co-expression clustering, screens gene modules of interest for further analysis. Recent research has emphasized the potential of this approach for grouping genes into functional modules to reveal the regulatory mechanisms behind complex traits (Behdani and Bakhtiarizadeh, 2017; Mohammad et al., 2018). The ImmuCellAI algorithm can evaluate the abundance of immune cell infiltration in gene expression profiles, and flow cytometry results confirm a higher accuracy compared to other commonly used algorithms, including CIBERSORT and TIMER (Li et al., 2017; Miao et al., 2020; Newman et al., 2015). ImmuCellAI-mouse as a supplement to ImmuCellAI is a comprehensive method for estimating the abundance of immune cells in mouse with a high accuracy (Miao et al., 2021).
At the early stage of infection (12 hpi), an overview of transcriptome analysis showed a rapid increase in the expression of genes encoding pro-inflammatory mediators (chemokines, cytokines and other acute phase molecules), suggesting the triggering of an acute inflammatory response. The skyblue2 module, which was highly associated with 12 hpi in WGCNA analysis, is closely related to the regulation of lipid metabolism, indicating that lipid metabolism plays a key role at 12 hpi. Metabolic regulation is important during host infection and has a significant impact on the immune response (Nhan et al., 2019). Both anti-inflammatory and pro-inflammatory immune responses require energy and metabolic regulation for their development (Kirthana and Ajay, 2014). Thus, to overcome the stresses induced by pathogens, the host needs to regulate relevant metabolic pathways early in the infection. Lipids are not only a very efficient source of energy, but also a key signaling mediator, thus being treated as ‘bioactive lipids’ (Escribá, 2006; Shimizu, 2009). Bioactive lipids play a vital role in immune regulation, inflammation and maintenance of homeostasis within the body (Shimizu, 2009). In the event of tissue infection, innate immune cells are recruited to the infected site and rapidly generate classical eicosanoids, a family of bioactive lipids involved in immunity and inflammation, whose main roles include amplifying or reducing inflammation, coordinating leukocyte recruitment, cytokine and chemokine production, antibody formation, cell proliferation and migration, and antigen presentation (Funk, 2001; Nathan, 2002; Harizi et al., 2008). Accordingly, we speculate that lipid metabolism may reflect a pro-inflammatory effect in the early stage of hvKp pulmonary infection and may have some clinical significance. However, further studies are needed to fully understand the underlying mechanisms.
Cluster2, whose gene expression level peaked at 24 hpi in the Mfuzz analysis, and the mediumpurple4 module, which is highly correlated with 24 hpi, are both closely associated with interferons according to GO analysis. The KEGG analysis for the mediumpurple4 module associates it with PRRs signaling pathways. Cellular immunity is essential to clear pathogens and type II interferon IFN-γ is a key molecule in promoting cellular immunity. A complex interaction between immune cell activity and IFN-γ leads to initiation of a cascade of pro-inflammatory responses through coordinated integration of other signals involving cytokines and PRRs, such as TNF-α, LPS and type I interferons (Kak et al., 2018). IFN-γ is an essential activator of antibacterial macrophages (Nathan, 1983). Glycolytic metabolism, which also promotes the survival, differentiation, and effector function of activated macrophages (Van den Bossche et al., 2015), had a sustained rise in the expression of its associated genes in cluster 4 starting from 24 hpi. Correspondingly, macrophage abundance in ImmuCellAI-mouse analysis continued to increase at 24 hpi after an already significant increase.
In the immune cell infiltration analysis, neutrophils and monocytes consistently increased after infection. Although neutrophil migration and activation are essential for infection clearance, excessive neutrophil recruitment and aberrant activation can lead to severe host tissue injury and may ultimately lead to death (Weber et al., 2015). This was reflected in our pathological findings, where after lung immune cells were significantly activated and recruited, local damage to lung tissue structures followed at 48 hpi. However, the abundance of NK cells continued to decrease. This finding is contrary to a previous study that found no change in NK cells in the lung during the first two days after K. pneumoniae infection (Xu et al., 2014). It is also dissimilar to responses observed with most bacteria, such as Streptococcus pneumoniae (van den Boogaard et al., 2016), Shigella flexneri (Le-Barillec et al., 2005), Pseudomonas aeruginosa (Wesselkamper et al., 2008), and Staphylococcus aureus (Small et al., 2008), where NK cells remain constant or increase after pulmonary infection. This suggests the decrease in pulmonary NK cells may be specific to hvKp infection. NK cells account for the highest percentage of resident lymphocytes in the lung, unlike other tissues (Wang et al., 2012; Sun et al., 2013), suggesting that NK cells are essential for the pulmonary immune response when pathogens invade. Although NK cells are traditionally well known for their critical protective role in antiviral innate immunity (Lodoen and Lanier, 2006), growing evidence indicates that NK cells are closely associated with fighting bacterial infections, with both beneficial or detrimental effects on the organism possible. For example, Dunn and North (Dunn and North, 1991) showed that early production of IFN-γ by NK cells is essential for resistance to L. monocytogenes. NK cells also contribute to host defense against K. pneumoniae (Xu et al., 2014). In contrast, other studies have shown that NK cell depletion leads to enhanced clearance of L. monocytogenes (Teixeira and Kaufmann, 1994) and a significant increase in survival for Streptococcus pneumoniae lung-infected mice (Christaki et al., 2015). These contrasting results could be related to the diversity of infection routes, bacterial strains and mouse strains and this possibility should be followed up. Interestingly, NK cells are also found to be reduced in paraquat dichloride induced lung injury, and in vivo NK cell depletion reduces macrophage and neutrophil infiltration, resulting in attenuated lung injury (Wu et al., 2020). Therefore, we hypothesize that hvKp lung infection decreases NK cells for attenuated inflammatory cell infiltration. The role of NK cells in severe hvKp lung infections, which could provide new targets for therapy, deserves further investigation and clarification.
During infection biological processes associated with immune response regulation, such as leukocyte activation and migration, cytokine production, cytokine-mediated signaling pathways and cytokine secretion were continuously activated. While biological processes associated with normal physiological processes of the organism, such as the regulation of ion transport across the membrane, the Wnt signaling pathway and cell matrix adhesion were continuously inhibited. This suggests that the organism undergoes a violent and sustained inflammatory response after infection and that the structures of the lungs are damaged. The grey60 module was highly positively correlated with all infection time points, indicating that the module genes play a key role in the infection process. KEGG analysis showed that grey60 module genes were closely associated with TNF, IL-17, HIF-1, NF-kB, and MAPK signaling pathways. TNF and IL-17 signaling pathways have important functions in host defense and disease pathogenesis (Gaffen, 2009; Kalliolias and Ivashkiv, 2016). NF-kB and MAPK are signaling pathways help mediate lung inflammatory responses (Lavoie et al., 2010; Wang et al., 2013). LPS is a potent stimulator that triggers MAPK and NF-kB signaling pathways (Li et al., 2015; Zhang et al., 2015). NF-kB is a key target of the p38 MAPK signaling pathway after LPS binding of TLR4/MYD88 to initiate the intracellular pathway (Shi et al., 2019; Zhou et al., 2019). P38MAPK/MK2 can regulate LPS-induced gene expression by controlling NF-kB p65 hyperphosphorylation and nuclear translocation (Gorska et al., 2007; Ehlting et al., 2011). Given the prominent role of these signaling pathways in hvKp pulmonary infection, further elucidation of the mechanisms of these signaling pathways in hvKp immunopathogenesis could provide novel insights for emergency treatment.
Some of the top 20 upregulated genes during the infection process are associated with the innate immune response, while others are closely associated with the inflammatory response induced by Gram-negative bacteria. One of them, Saa1 encodes serum amyloid A1 (SAA1), an acute phase protein in the inflammatory response and can be an antimicrobial agent by acting as a direct modulator of bacteria (Buck et al., 2016; Sun and Ye, 2016). Inducible expression of SAA1 in the acute phase may protect the host from Gram-negative bacterial infection by reducing LPS-induced tissue damage (Cheng et al., 2017). Lcn2 encodes Lipocalin-2 (LCN2), a key antimicrobial protein, whose primary antibacterial function is to bind and sequester bacterial iron carriers, thereby depriving bacteria of the iron that provides them with nutrients (Goetz et al., 2002). Previous work found LCN2 protein is upregulated in the lung after K. pneumoniae infection (Chan et al., 2009), and our findings support this. Aoah encodes a mammalian enzyme named acyloxyacyl hydrolase (AOAH) that can inactivate LPS in host tissues (Munford et al., 2009) and promotes recovery from lung inflammation caused by K. pneumoniae (Zou et al., 2017). Slpi encodes secretory leucocyte peptidase inhibitor (SLPI) that is secreted from lung tissue, exhibits antibacterial and antifungal properties and is an essential respiratory host defense protein similar to antimicrobial peptides (Gomez et al., 2009; Majchrzak-Gorecka et al., 2016). Irak3 encodes interleukin-1 receptor-associated kinase 3 (IRAK3; also known as IRAK-M), which has been rarely studied in bacterial pulmonary infections. However, IRAK3 is known to be involved in the regulation of LPS tolerance (van ‘t Veer et al., 2007; Yu et al., 2017), whereby cells or organisms exposed to LPS enter a non-responsive state and are unable to respond to further LPS stimuli, thus preventing inflammatory overload (Biswas and Lopez-Collazo, 2009; Hotchkiss et al., 2013). IRAK-M mRNA was upregulated in the lungs of WT mice with K. pneumoniae (Hoogerwerf et al., 2012) and our findings are consistent with this. Among these five genes involved in the antimicrobial response to bacterial infection, Saa1, associated with the LPS cell wall component of Gram-negative bacteria that causes host inflammatory response, and Slpi, which encodes an antimicrobial protein, have not previously been reported in hvKp lung infection. Given their persistently high expression levels and multiple functions in hvKp infected lungs, they are expected to be good therapeutic targets for intervention in hvKp-induced lung injury and deserve to be investigated in depth.
Our findings suggest that hvKp causes a primary acute inflammation in the lung that increases over time, leading to the damage of lung structures. Clarifying the role of NK cells in severe hvKp lung infections could provide new targets for therapy. Saa1 and Slpi, significantly upregulated during infection and associated with the immune response, have not been reported in hvKp infections and could be important targets for subsequent studies. Given that disease progression may depend on immune cells, further explorations at the level of a single cell type are warranted to elucidate the cellular associations of the phenomena found in this study. In conclusion, our work both validates the results of previous studies on K. pneumoniae pulmonary infections and provides new insights on hvKp pulmonary infections that have promise for the development of therapeutic approaches to reduce hvKp lung inflammation.
Data Availability Statement
The data presented in the study are deposited in the Gene Expression Omnibus (GEO) repository, accession number GSE199546. The original contributions presented in the study are publicly available. This data can be found here: https://www.ncbi.nlm.nih.gov/geo/query/acc.cgi?acc=GSE199546.
Ethics Statement
The animal study was reviewed and approved by Animal Care and Use Committee, Academy of Military Medical Science.
Author Contributions
DZ, TF, and WY conceived and designed the experiments. XZ, CC, TQ, LH, and LZ performed the experiments. XZ, JG, YZ, XS, and ML analyzed and interpreted the results. XZ and JG wrote the manuscript. All authors contributed to the article and approved the submitted version.
Conflict of Interest
The authors declare that the research was conducted in the absence of any commercial or financial relationships that could be construed as a potential conflict of interest.
Publisher’s Note
All claims expressed in this article are solely those of the authors and do not necessarily represent those of their affiliated organizations, or those of the publisher, the editors and the reviewers. Any product that may be evaluated in this article, or claim that may be made by its manufacturer, is not guaranteed or endorsed by the publisher.
Acknowledgments
We thank Professor Hui Wang (Peking University People’s Hospital) for kindly providing the hypervirulent K. pneumoniae strain NTUH-K2044.
References
Akira, S., Uematsu, S., Takeuchi, O. (2006). Pathogen Recognition and Innate Immunity. Cell 124, 783–801. doi: 10.1016/j.cell.2006.02.015
Arun, S., Gokhlesh, K., Mansour, E. M. (2018). Transcriptome Analysis Based on RNA-Seq in Understanding Pathogenic Mechanisms of Diseases and the Immune System of Fish: A Comprehensive Review. Int. J. Mol. Sci. 19, 245. doi: 10.3390/ijms19010245
Behdani, E., Bakhtiarizadeh, M. R. (2017). Construction of an Integrated Gene Regulatory Network Link to Stress-Related Immune System in Cattle. Genetica 145, 441–454. doi: 10.1007/s10709-017-9980-z
Bengoechea, J. A., Pessoa, S. J. (2018). Klebsiella Pneumoniae Infection Biology: Living to Counteract Host Defences. FEMS Microbiol. Rev. doi: 10.1093/femsre/fuy043
Biswas, S. K., Lopez-Collazo, E. (2009). Endotoxin Tolerance: New Mechanisms, Molecules and Clinical Significance. Trends Immunol. 30, 475–487. doi: 10.1016/j.it.2009.07.009
Buck, M., Gouwy, M., Wang, J., Snick, J., Opdenakker, G., Struyf, S., et al. (2016). Structure and Expression of Different Serum Amyloid A (SAA) Variants and Their Concentration-Dependent Functions During Host Insults. Curr. Med. Chem. 23, 1725–1755. doi: 10.2174/0929867323666160418114600
Catalán-Nájera, J. C., Garza-Ramos, U., Barrios-Camacho, H. (2017). Hypervirulence and Hypermucoviscosity: Two Different But Complementary Klebsiella Spp. Phenotypes? Virulence 8, 1111–1123. doi: 10.1080/21505594.2017.1317412
Catalina, M., Victoria, C., David, M., Enrique, L., Camino, P. G., Tomás, J., et al. (2013). Role of Bacterial Surface Structures on the Interaction of Klebsiella Pneumoniae With Phagocytes. PloS One 8, e56847. doi: 10.1371/journal.pone.0056847
Chan, Y. R., Liu, J. S., Pociask, D. A., Zheng, M., Mietzner, T. A., Berger, T., et al. (2009). Lipocalin 2 Is Required for Pulmonary Host Defense Against Klebsiella Infection. J. Immunol. 182, 4947–4956. doi: 10.4049/jimmunol.0803282
Cheng, H. Y., Chen, Y. S., Wu, C. Y., Chang, H. Y., Lai, Y. C., Peng, H. L. (2010). RmpA Regulation of Capsular Polysaccharide Biosynthesis in Klebsiella Pneumoniae CG43. J. Bacteriol. 192, 3144–3158. doi: 10.1128/JB.00031-10
Cheng, N., Liang, Y., Du, X., Ye, R. (2017). Serum Amyloid A Promotes LPS Clearance and Suppresses LPS-Induced Inflammation and Tissue Injury. EMBO Rep. doi: 10.15252/embr.201745517
Choby, J. E., Howard-Anderson, J., Weiss, D. S. (2020). Hypervirulent Klebsiella Pneumoniae – Clinical and Molecular Perspectives. J. Internal Med. 287, 283-300. doi: 10.1111/joim.13007
Christaki, E., Diza, E., Giamarellos-Bourboulis, E. J., Papadopoulou, N., Pistiki, A., Droggiti, D. I., et al. (2015). NK and NKT Cell Depletion Alters the Outcome of Experimental Pneumococcal Pneumonia: Relationship With Regulation of Interferon-γ Production. J. Immunol. Res., 532717. doi: 10.1155/2015/532717
Chuang, Y. P., Fang, C. T., Lai, S. Y., Chang, S. C., Wang, J. T. (2006). Genetic Determinants of Capsular Serotype K1 of Klebsiella Pneumoniae Causing Primary Pyogenic Liver Abscess. J. Infect. Dis. 193, 645–654. doi: 10.1086/499968
Dunn, P. L., North, R. J. (1991). Early Gamma Interferon Production by Natural Killer Cells Is Important in Defense Against Murine Listeriosis. Infect. Immun. 59, 2892–2900. doi: 10.1128/iai.59.9.2892-2900.1991
Ehlting, C., Ronkina, N., Böhmer, O., Albrecht, U., Bode, K. A., Lang, K. S., et al. (2011). Distinct Functions of the Mitogen-Activated Protein Kinase-Activated Protein (MAPKAP) Kinases MK2 and MK3: MK2 Mediates Lipopolysaccharide-Induced Signal Transducers and Activators of Transcription 3 (STAT3) Activation by Preventing Negative Regulatory Eff. J. Biol. Chem. 286, 4113–4124. doi: 10.1074/jbc.M111.235275
Escribá, P. (2006). Membrane-Lipid Therapy: A New Approach in Molecular Medicine. Trends Mol. Med. 12, 34–43. doi: 10.1016/j.molmed.2005.11.004
Fang, C. T., Chuang, Y. P., Shun, C. T., Chang, S. C., Wang, J. T. (2004). A Novel Virulence Gene in Klebsiella Pneumoniae Strains Causing Primary Liver Abscess and Septic Metastatic Complications. J. Exp. Med. 199, 697–705. doi: 10.1084/jem.20030857
Fang, C. T., Lai, S. Y., Yi, W. C., Po-Ren, H., Liu, K. L., Chang, S. C. (2007). Klebsiella Pneumoniae Genotype K1: An Emerging Pathogen That Causes Septic Ocular or Central Nervous System Complications From Pyogenic Liver Abscess. Clin. Infect. Dis. Off. Publ. Infect. Dis. Soc. Am. 284–293. doi: 10.1086/519262
Feng, J., Hu, X., Fu, M., Dai, L., Xiong, X. (2019). Enhanced Protection Against Q Fever in BALB/c Mice Elicited by Immunization of Chloroform-Methanol Residue of Coxiella Burnetii via Intratracheal Inoculation. Vaccine 37, 6076–6084. doi: 10.1016/j.vaccine.2019.08.041
Florea, L., Song, L., Salzberg, S. L. (2013). Thousands of Exon Skipping Events Differentiate Among Splicing Patterns in Sixteen Human Tissues. F1000 Res. 2, 188. doi: 10.12688/f1000research.2-188.v1
Funk, C. D. (2001). Prostaglandins and Leukotrienes: Advances in Eicosanoid Biology. Science 294, 1871–1875. doi: 10.1126/science.294.5548.1871
Futschik, M. E., Carlisle, B. (2011). Noise-Robust Soft Clustering Of Gene Expression Time-Course Data. J. Bioinf. Comput. Biol. doi: 10.1142/s0219720005001375
Gaffen, S. L. (2009). Structure and Signalling in the IL-17 Receptor Family. Nat. Rev. Immunol. 9, 556–567. doi: 10.1038/nri2586
Goetz, D. H., Borregaard, N., Bluhm, M. E., Raymond, K. N., Strong, R. K. (2002). The Neutrophil Lipocalin NGAL Is a Bacteriostatic Agent That Interferes With Siderophore-Mediated Iron Acquisition. Mol. Cell. 10, 1033–1043. doi: 10.1016/S1097-2765(02)00708-6
Gomez, S. A., Argüelles, C., Guerrieri, D., Tateosian, N. L., Amiano, N. O., Slimovich, R., et al. (2009). Secretory Leukocyte Protease Inhibitor: A Secreted Pattern Recognition Receptor for Mycobacteria. Am. J. Respir. Crit. Care Med. 179, 247. doi: 10.1164/rccm.200804-615OC
Gorska, M. M., Liang, Q., Stafford, S. J., Goplen, N., Dharajiya, N., Guo, L., et al. (2007). MK2 Controls the Level of Negative Feedback in the NF-κb Pathway and Is Essential for Vascular Permeability and Airway Inflammation. J. Exp. Med. 204, 1637–1652. doi: 10.1084/jem.20062621
Guangchuang, Y., Li-Gen, W., Yanyan, H., Qing-Yu, H. (2012). Clusterprofiler: An R Package for Comparing Biological Themes Among Gene Clusters. Omics: J. Integr. Biol. 16, 284-287. doi: 10.1089/omi.2011.0118
Harada, S., Doi, Y. (2018). Hypervirulent Klebsiella Pneumoniae: A Call for Consensus Definition and International Collaboration. J. Clin. Microbiol. 56, e00959-18. doi: 10.1128/JCM.00959-18
Harizi, H., Corcuff, J. B., Gualde, N. (2008). Arachidonic-Acid-Derived Eicosanoids: Roles in Biology and Immunopathology. Trends Mol. Med. 14, 461–469. doi: 10.1016/j.molmed.2008.08.005
He, Y., Wang, A., Chen, S., Wu, Z., Zhang, J., Wang, M., et al. (2017). Differential Immune-Related Gene Expression in the Spleens of Duck Tembusu Virus-Infected Goslings. Vet. Microbiol. 39, 39-47. doi: 10.1016/j.vetmic.2017.08.002
Hong, S. N., Joung, J. G., Bae, J. S., Lee, C. S., Koo, J. S., Park, S. J., et al. (2017). RNA-Seq Reveals Transcriptomic Differences in Inflamed and Noninflamed Intestinal Mucosa of Crohn’s Disease Patients Compared With Normal Mucosa of Healthy Controls. Inflamm. Bowel Dis. 23, 1098–1108. doi: 10.1097/MIB.0000000000001066
Hoogerwerf, J. J., van der Windt, G. J., Blok, D. C., Hoogendijk, A. J., De Vos, A. F., van ‘t Veer, C., et al. (2012). Interleukin-1 Receptor-Associated Kinase M-Deficient Mice Demonstrate an Improved Host Defense During Gram-Negative Pneumonia. Mol. Med. (Cambridge Mass) 18, 1067–1075. doi: 10.2119/molmed.2011.00450
Hotchkiss, R. S., Monneret, G., Payen, D. (2013). Sepsis-Induced Immunosuppression: From Cellular Dysfunctions to Immunotherapy. Nat. Rev. Immunol. 13, 862–874. doi: 10.1038/nri3552
Ivliev, A. E., t Hoen, P. A., Sergeeva, M. G. (2010). Coexpression Network Analysis Identifies Transcriptional Modules Related to Proastrocytic Differentiation and Sprouty Signaling in Glioma. Cancer Res. 70, 10060–10070. doi: 10.1158/0008-5472.CAN-10-2465
Kak, G., Raza, M., Tiwari, B. K. (2018). Interferon-Gamma (IFN-γ): Exploring Its Implications in Infectious Diseases. Biomol. Concepts 9, 64–79. doi: 10.1515/bmc-2018-0007
Kalliolias, G. D., Ivashkiv, L. B. (2016). TNF Biology, Pathogenic Mechanisms and Emerging Therapeutic Strategies. Nat. Rev. Rheumatol. doi: 10.1038/nrrheum.2015.169
Kirthana, G., Ajay, C. (2014). Metabolic Regulation of Immune Responses. Annu. Rev. Immunol. 32, 609–634. doi: 10.1146/annurev-immunol-032713-120236
Kumar, L., Futschik, M. (2007). Mfuzz: A Software Package for Soft Clustering of Microarray Data. Bioinformation. doi: 10.6026/97320630002005
Langfelder, P., Horvath, S. (2009). Langfelder P, Horvath S. WGCNA: An R Package for Weighted Correlation Network Analysis. BMC Bioinform. 9, 559. doi: 10.1186/1471-2105-9-559
Lavoie, J. P., Thompson, D., Hamilton, E., Debrue, M., Hickey, G. (2010). Effects of a MAPK P38 Inhibitor on Lung Function and Airway Inflammation in Equine Recurrent Airway Obstruction. Equine Veterinary J. 40, 577–583. doi: 10.2746/042516408X284646
Le-Barillec, K., Magalhaes, J. G., Corcuff, E., Thuizat, A., Sansonetti, P. J., Phalipon, A., et al. (2005). Roles for T and NK Cells in the Innate Immune Response to Shigella Flexneri. J. Immunol. 175, 1735–1740. doi: 10.4049/jimmunol.175.3.1735
Lederman, E. R., Crum, N. F. (2005). Pyogenic Liver Abscess With a Focus on Klebsiella Pneumoniae as a Primary Pathogen: An Emerging Disease With Unique Clinical Characteristics. Am. J. Gastroenterol. 100, 322. doi: 10.1111/j.1572-0241.2005.40310.x
Li, T., Fan, J., Wang, B., Traugh, N., Chen, Q., Liu, J. S., et al. (2017). TIMER: A Web Server for Comprehensive Analysis of Tumor-Infiltrating Immune Cells. Cancer Res. 77, e108–e110. doi: 10.1158/0008-5472.CAN-17-0307
Lin, Y. T., Jeng, Y. Y., Chen, T. L., Fung, C. P. (2010). Bacteremic Community-Acquired Pneumonia Due to Klebsiella Pneumoniae: Clinical and Microbiological Characteristics in Taiwa-2008. BMC Infect. Dis. 10, 307. doi: 10.1186/1471-2334-10-307
Li, W., Qiu, X., Jiang, H., Zhi, Y., Fu, J., Liu, J. (2015). Ulinastatin Inhibits the Inflammation of LPS-Induced Acute Lung Injury in Mice via Regulation of AMPK/NF-κb Pathway. Int. Immunopharmacol. 29, 560–567. doi: 10.1016/j.intimp.2015.09.028
Lodoen, M. B., Lanier, L. L. (2006). Natural Killer Cells as an Initial Defense Against Pathogens. Curr. Opin. Immunol. 18, 391–398. doi: 10.1016/j.coi.2006.05.002
Love, M. I., Huber, W., Anders, S. (2014). Moderated Estimation of Fold Change and Dispersion for RNA-Seq Data With Deseq2. Genome Biol. 15, 550. doi: 10.1186/s13059-014-0550-8
Magill, S. S., O’Leary, E., Janelle, S. J., Thompson, D. L., Dumyati, G., Nadle, J., et al. (2018). Changes in Prevalence of Health Care-Associated Infections in U.S. Hospitals N. Engl. J. Med. 379, 1732–1744. doi: 10.1056/NEJMoa1801550
Majchrzak-Gorecka, M., Majewski, P., Grygier, B., Murzyn, K., Cichy, J. (2016). Secretory Leukocyte Protease Inhibitor (SLPI), a Multifunctional Protein in the Host Defense Response. Cytokine Growth Factor Rev. 79–93. doi: 10.1016/j.cytogfr.2015.12.001
Miao, Y. R., Xia, M., Luo, M., Luo, T., Yang, M., Guo, A. Y. (2021). ImmuCellAI-Mouse: A Tool for Comprehensive Prediction of Mouse Immune Cell Abundance and Immune Microenvironment Depiction. Bioinformatics. doi: 10.1093/bioinformatics/btab711
Miao, Y. R., Zhang, Q., Lei, Q., Luo, M., Xie, G. Y., Wang, H., et al. (2020). ImmuCellAI: A Unique Method for Comprehensive T-Cell Subsets Abundance Prediction and Its Application in Cancer Immunotherapy. Adv. Sci. (Weinheim Baden-Wurttemberg Germany) 7, 1902880. doi: 10.1002/advs.201902880
Mohammad, R., Bakhtiarizadeh, B., Hosseinpour, M., Shahhoseini, A., Korte, P. (2018). Weighted Gene Co-Expression Network Analysis of Endometriosis and Identification of Functional Modules Associated With Its Main Hallmarks. Front. Genet. 9, 453. doi: 10.3389/fgene.2018.00453
Munford, R., Lu, M., Varley, A. (2009). Chapter 2 Kill the Bacteria … and Also Their Messengers? Adv. Immunol. 103, 29–48. doi: 10.1016/S0065-2776(09)03002-8
Nathan, C. (1983). Identification of Interferon-Gamma as the Lymphokine That Activates Human Macrophage Oxidative Metabolism and Antimicrobial Activity. J. Exp. Med. 158, 670–689. doi: 10.1084/jem.158.3.670
Nathan, C. (2002). Points of Control in Inflammation. Nature: Int. Weekly J. Sci. 420, 846–852. doi: 10.1038/nature01320
Newman, A. M., Liu, C. L., Green, M. R., Gentles, A. J., Feng, W., Xu, Y., et al. (2015). Robust Enumeration of Cell Subsets From Tissue Expression Profiles. Nat. Methods 12, 453–457. doi: 10.1038/nmeth.3337
Nhan, J. D., Turner, C. D., Anderson, S. M., Yen, C. A., Dalton, H. M., Cheesman, H. K., et al. (2019). Redirection of SKN-1 Abates the Negative Metabolic Outcomes of a Perceived Pathogen Infection. Proc. Natl. Acad. Sci. U. S. A. 116, 22322–22330. doi: 10.1073/pnas.1909666116
Olonisakin, T. F., Suber, T., Gonzalez-Ferrer, S., Xiong, Z., Peñaloza, H. F., van der Geest, R., et al. (2021). Stressed Erythrophagocytosis Induces Immunosuppression During Sepsis Through Heme-Mediated STAT1 Dysregulation. J. Clin. Invest. 4, 131. doi: 10.1172/JCI137468
Patel, P. K., Russo, T. A., Karchmer, A. W. (2014). Hypervirulent Klebsiella Pneumoniae. Open Forum Infect. Dis., ofu028. doi: 10.1093/ofid/ofu028
Peñaloza, H. F., Alvarez, D., Muñoz-Durango, N., Schultz, B. M., González, P. A., Kalergis, A. M., et al. (2019). The Role of Myeloid-Derived Suppressor Cells in Chronic Infectious Diseases and the Current Methodology Available for Their Study. J. Leukocyte Biol. 105, 857–872. doi: 10.1002/JLB.MR0618-233R
Podschun, R., Ullmann, U. (1998). Klebsiella Spp. As Nosocomial Pathogens: Epidemiology, Taxonomy, Typing Methods, and Pathogenicity Factors. Clin. Microbiol. Rev. 11, 589–603. doi: 10.1128/CMR.11.4.589
Poe, S. L., Arora, M., Oriss, T. B., Yarlagadda, M., Isse, K., Khare, A., et al. (2013). STAT1-Regulated Lung MDSC-Like Cells Produce IL-10 and Efferocytose Apoptotic Neutrophils With Relevance in Resolution of Bacterial Pneumonia. Mucosal Immunol. 6, 189–199. doi: 10.1038/mi.2012.62
Pomakova, D. K., Hsiao, C. B., Beanan, J. M., Olson, R., Macdonald, U., Keynan, Y., et al. (2012). Clinical and Phenotypic Differences Between Classic and Hypervirulent Klebsiella Pneumonia: An Emerging and Under-Recognized Pathogenic Variant. Eur. J. Clin. Microbiol. Infect. Dis. 31, 981–989. doi: 10.1007/s10096-011-1396-6
Restuccia, P. A., Cunha, B. A. (1984). Klebsiella. Infection Control Ic 5, 343. doi: 10.1017/S0195941700060549
Russo, T. A., Marr, C. M. (2019). Hypervirulent Klebsiella Pneumoniae. Clin. Microbiol. Rev. 32, e00001-19. doi: 10.1128/CMR.00001-19
Scott, A. J., Oyler, B. L., Goodlett, D. R., Ernst, R. K. (2017). Lipid A Structural Modifications in Extreme Conditions and Identification of Unique Modifying Enzymes to Define the Toll-Like Receptor 4 Structure-Activity Relationship. Biochim. Biophys. Acta (BBA) Mol. Cell Biol. Lipids. doi: 10.1016/j.bbalip.2017.01.004
Shan, Y., Lambrecht, R. W., Donohue, S. E., Bonkovsky, H. L. (2006). Role of Bach1 and Nrf2 in Up-Regulation of the Heme Oxygenase-1 Gene by Cobalt Protoporphyrin. FASEB J.: Off. Publ. Fed. Am. Societies Exp. Biol. 20, 2651–2653. doi: 10.1096/fj.06-6346fje
Shimizu, T. (2009). Lipid Mediators in Health and Disease: Enzymes and Receptors as Therapeutic Targets for the Regulation of Immunity and Inflammation. Annu. Rev. Pharmacol. Toxicol. doi: 10.1146/annurev.pharmtox.011008.145616
Shi, H., Wang, X.-L., Quan, H.-F., Yan, L., Pei, X.-Y., Wang, R., et al. (2019). Effects of Betaine on LPS-Stimulated Activation of Microglial M1/M2 Phenotypes by Suppressing TLR4/NF-κb Pathways in N9 Cells. Molecules 24, 367. doi: 10.3390/molecules24020367
Shon, A. S., Bajwa, R., Russo, T. A. (2013). Hypervirulent (Hypermucoviscous) Klebsiella Pneumoniae: A New and Dangerous Breed. Virulence 4, 107–118. doi: 10.4161/viru.22718
Small, C. L., McCormick, S., Gill, N., Kugathasan, K., Santosuosso, M., Donaldson, N., et al. (2008). NK Cells Play a Critical Protective Role in Host Defense Against Acute Extracellular Staphylococcus Aureus Bacterial Infection in the Lung. J. Immunol. 180, 5558–5568. doi: 10.4049/jimmunol.180.8.5558
Smyth, G. K. (2010). Edger: A Bioconductor Package for Differential Expression Analysis of Digital Gene Expression Data. Bioinformatics 26, 139. doi: 10.1093/bioinformatics/btp616
Sun, H., Sun, C., Tian, Z., Xiao, W. (2013). NK Cells in Immunotolerant Organs. Cell. Mol. Immunol. 10, 202–212. doi: 10.1038/cmi.2013.9
Sun, L., Ye, R. D. (2016). Serum Amyloid A1: Structure, Function and Gene Polymorphism. Gene 583, 48–57. doi: 10.1016/j.gene.2016.02.044
Teixeira, H. C., Kaufmann, S. H. (1994). Role of NK1. 1+ Cells in Experimental Listeriosis. NK1+ Cells Are Early IFN-Gamma Producers But Impair Resistance to Listeria Monocytogenes Infection. J. Immunol. 152, 1873–1882.
van ‘t Veer, C., van den Pangaart, P. S., van Zoelen, M. A. D., de Kruif, M., Birjmohun, R. S., Stroes, E. S., et al. (2007). Induction of IRAK-M Is Associated With Lipopolysaccharide Tolerance in a Human Endotoxemia Model. J. Immunol. (Baltimore Md.: 1950) 179, 7110–7120. doi: 10.4049/jimmunol.179.10.7110
van den Boogaard, F. E., van Gisbergen, K. P., Vernooy, J. H., Medema, J. P., Roelofs, J. J., van Zoelen, M. A., et al. (2016). Granzyme A Impairs Host Defense During Streptococcus Pneumoniae Pneumonia. Am. J. Physiol. Lung Cell. Mol. Physiol. 311, L507–L516. doi: 10.1152/ajplung.00116.2016
Van den Bossche, J., Baardman, J., de Winther, M. P. (2015). Metabolic Characterization of Polarized M1 and M2 Bone Marrow-Derived Macrophages Using Real-Time Extracellular Flux Analysis. J. Visualized Experiments: JoVE. doi: 10.3791/53424
Wang, J., Li, F., Zheng, M., Sun, R., Wei, H., Tian, Z. (2012). Lung Natural Killer Cells in Mice: Phenotype and Response to Respiratory Infection. Immunology 137, 37–47. doi: 10.1111/j.1365-2567.2012.03607.x
Wang, H.-W., Yang, W., Lu, J.-Y., Li, F., Sun, J.-Z. (2013). N-Acetylcysteine Administration Is Associated With Reduced Activation of NF-kB and Preserves Lung Dendritic Cells Function in a Zymosan-Induced Generalized Inflammation Model. J. Clin. Immunol. doi: 10.1007/s10875-012-9852-3
Weber, A., Rieber, N., Skokowa, J., Hartl, D. (2015). Neutrophils: Between Host Defence, Immune Modulation, and Tissue Injury. PloS Pathogens 11, e1004651. doi: 10.1371/journal.ppat.1004651
Wesselkamper, S. C., Eppert, B. L., Motz, G. T., Lau, G. W., Hassett, D. J., Borchers, M. T. (2008). NKG2D Is Critical for NK Cell Activation in Host Defense Against Pseudomonas Aeruginosa Respiratory Infection. J. Immunol. 181, 5481–5489. doi: 10.4049/jimmunol.181.8.5481
Whitfield, C., Trent, M. S. (2014). Biosynthesis and Export of Bacterial Lipopolysaccharides. Annu. Rev. Biochem. 83, 99–128. doi: 10.1146/annurev-biochem-060713-035600
Wu, M., Zhou, C., Li, M., Yu, H., Zhao, D., Xue, W., et al. (2020). Depletion of NK Cells Attenuates Paraquat-Induced Acute Lung Injury by Manipulating Macrophage Polarization. Int. Immunopharmacol. 86, 106698. doi: 10.1016/j.intimp.2020.106698
Xiong, H., Keith, J. W., Samilo, D. W., Carter, R. A., Leiner, I. M., Pamer, E. G. (2016). Innate Lymphocyte/Ly6C(hi) Monocyte Crosstalk Promotes Klebsiella Pneumoniae Clearance. Cell 165, 679–689. doi: 10.1016/j.cell.2016.03.017
Xu, X., Weiss, I. D., Zhang, H. H., Singh, S. P., Wynn, T. A., Wilson, M. S., et al. (2014). Conventional NK Cells can Produce IL-22 and Promote Host Defense in Klebsiella Pneumoniae Pneumonia. J. Immunol. 192, 1778–1786. doi: 10.4049/jimmunol.1300039
Yeh, K. M., Kurup, A., Siu, L. K., Koh, Y. L., Fung, C. P., Lin, J. C., et al. (2007). Capsular Serotype K1 or K2, Rather Than magA and Rmpa, Is a Major Virulence Determinant for Klebsiella Pneumoniae Liver Abscess in Singapore and Taiwan. J. Clin. Microbiol. 45, 466–471. doi: 10.1128/JCM.01150-06
Yu, W. L., Ko, W. C., Cheng, K. C., Lee, H. C., Ke, D. S., Lee, C. C., et al. (2006). Association Between rmpA and magA Genes and Clinical Syndromes Caused by Klebsiella Pneumoniae in Taiwan. Clin. Infect. Dis.: Off. Publ. Infect. Dis. Soc. Am. 42, 1351–1358. doi: 10.1086/503420
Yu, W. L., Ko, W. C., Cheng, K. C., Lee, C. C., Lai, C. C., Chuang, Y. C. (2008). Comparison of Prevalence of Virulence Factors for Klebsiella Pneumoniae Liver Abscesses Between Isolates With Capsular K1/K2 and Non-K1/K2 Serotypes. Diagn. Microbiol. Infect. Dis. 62, 1–6. doi: 10.1016/j.diagmicrobio.2008.04.007
Yu, S., Liu, X., Zhang, N., Yang, S., Mao, C., Feng, S., et al. (2017). Protection of Lipopolysaccharide (LPS) Preconditioning Against Endotoxin-Induced Uveitis (EIU) in Rats Is Associated With Overexpression of Interleukin-1 Receptor-Associated Kinase M(IRAK-M). Ocular Immunol. Inflamm. 1, 943-950. doi: 10.1080/09273948.2017.1291842
Zhang, T. Z., Yang, S. H., Yao, J. F., Juan, D. U., Yan, T. H. (2015). Sangxingtang Inhibits the Inflammation of LPS-Induced Acute Lung Injury in Mice by Down-Regulating the MAPK/NF-κb Pathway. Chin. J. Natural Med. doi: 10.1016/s1875-5364(15)30094-7
Zhou, J., Deng, Y., Li, F., Yin, C., Shi, J., Gong, Q. (2019). Icariside II Attenuates Lipopolysaccharide-Induced Neuroinflammation Through Inhibiting TLR4/MyD88/NF-κb Pathway in Rats. Biomed. Pharmacother. 111, 315–324. doi: 10.1016/j.biopha.2018.10.201
Keywords: hypervirulent Klebsiella pneumoniae, molecular pathology, lung, time-course transcriptome, inflammation
Citation: Zheng X, Guo J, Cao C, Qin T, Zhao Y, Song X, Lv M, Hu L, Zhang L, Zhou D, Fang T and Yang W (2022) Time-Course Transcriptome Analysis of Lungs From Mice Infected With Hypervirulent Klebsiella pneumoniae via Aerosolized Intratracheal Inoculation. Front. Cell. Infect. Microbiol. 12:833080. doi: 10.3389/fcimb.2022.833080
Received: 13 December 2021; Accepted: 11 March 2022;
Published: 27 April 2022.
Edited by:
Charles Robert Brown, University of Missouri, United StatesReviewed by:
Daniel Alford Powell, University of Arizona, United StatesMichael Bachman, University of Michigan, United States
Copyright © 2022 Zheng, Guo, Cao, Qin, Zhao, Song, Lv, Hu, Zhang, Zhou, Fang and Yang. This is an open-access article distributed under the terms of the Creative Commons Attribution License (CC BY). The use, distribution or reproduction in other forums is permitted, provided the original author(s) and the copyright owner(s) are credited and that the original publication in this journal is cited, in accordance with accepted academic practice. No use, distribution or reproduction is permitted which does not comply with these terms.
*Correspondence: Tongyu Fang, fangty2008@163.com; Wenhui Yang, fionyoung@163.com
†These authors have contributed equally to this work