- 1College of Veterinary Medicine, Huazhong Agricultural University, Wuhan, China
- 2Institute of Traditional Chinese Veterinary Medicine, College of Veterinary Medicine, Nanjing Agricultural University, Nanjing, China
- 3MOE Joint International Research Laboratory of Animal Health and Food Safety, College of Veterinary Medicine, Nanjing Agricultural University, Nanjing, China
- 4Department of Animal Nutrition and Feed Science, College of Animal Science and Technology, Huazhong Agricultural University, Wuhan, China
- 5Faculty of Veterinary and Animal Sciences, The Islamia University of Bahawalpur, Bahawalpur, Pakistan
Short-chain fatty acids (SCFA) are principal nutrient substrates of intestinal epithelial cells that regulate the epithelial barrier in yaks. Until now, metagenomics sequencing has not been reported in diarrheal yaks. Scarce information is available regarding the levels of fecal SCFA and diarrhea in yaks. So, our study aims to identify the potential pathogens that cause the emerging diarrhea and explore the potential relationship of short-chain fatty acids in this issue. We estimated diarrhea rate in yaks after collecting an equal number of fecal samples from affected animals. Metagenomics sequencing and quantitative analysis of SCFA were performed, which revealed 15%–25% and 5%–10% prevalence of diarrhea in yak’s calves and adults, respectively. Violin box plot also showed a higher degree of dispersion in gene abundance distribution of diarrheal yaks, as compared to normal yaks. We found 366,163 significant differential abundance genes in diarrheal yaks, with 141,305 upregulated and 224,858 downregulated genes compared with normal yaks via DESeq analysis. Metagenomics binning analysis indicated the higher significance of bin 33 (Bacteroidales) (p < 0.05) in diarrheal animals, while bin 10 (p < 0.0001), bin 30 (Clostridiales) (p < 0.05), bin 51 (Lactobacillales) (p < 0.05), bin 8 (Lachnospiraceae) (p < 0.05), and bin 47 (Bacteria) (p < 0.05) were significantly higher in normal yaks. At different levels, a significant difference in phylum (n = 4), class (n = 8), oder (n = 8), family (n = 16), genus (n = 17), and species (n = 30) was noticed, respectively. Compared with healthy yaks, acetic acid (p < 0.01), propionic acid (p < 0.01), butyric acid (p < 0.01), isobutyric acid (p < 0.01), isovaleric acid (p < 0.05), and caproic acid (p < 0.01) were all observed significantly at a lower rate in diarrheal yaks. In conclusion, besides the increased Staphylococcus aureus, Babesia ovata, Anaplasma phagocytophilum, Bacteroides fluxus, viruses, Klebsiella pneumonia, and inflammation-related bacteria, the decrease of SCFA caused by the imbalance of intestinal microbiota was potentially observed in diarrheal yaks.
Introduction
The long-haired bovine species, i.e., the yak, is an indispensable economic pillar on the Qing-Tibetan plateau (Li et al., 2014). There are approximately 15 million yaks in China, accounting for over 90% of the world’s yak population (Li et al., 2018; Li et al., 2020). At an average of 3,000–5,000 m above sea level, the yak is depicted as a symbolic animal dependent on herdsmen’s lives (Li et al., 2014). Yaks serve as transportations, especially in the mountainy zigzag areas (Li et al., 2017). Meat, butter, and milk from yaks are also considered essential food items for local Tibetans (Li et al., 2018). Its hide is used to make boots, rafts, aprons, leather bags, and leather harnesses (Li et al., 2020). While the long hairs and dungs are commonly used for livelihood purposes (Li et al., 2017).
Hongyuan is located on the eastern edge of the Qinghai-Tibetan plateau and northwest of Sichuan province, with a northern latitude of 31°51′–33°33′ and eastern longitude of 101°51′–103°22′. In this continental plateau, the temperature ranges from −22.8°C to 24.6°C, with an average temperature of 2.9°C and 860.8 mm precipitation annually. According to the latest reports, animal husbandry is the primary industry with 75,383 yak population in this region. About 7,765 kg of yak’s meat has also been produced annually, accounting for 96.23% of the total meat production in Hongyuan (Bureau of Statistics of Hongyuan County, http://www.hongyuan.gov.cn/hyxrmzf/c100057/zwgk.shtml). However, an emerging endemic diarrheal disease in yaks during the past few years (usually from May to August) has caused deaths to animals and caused a huge constraint on the development of the local economy.
Bovine diarrhea is a common disease throughout farms with worldwide distribution. It has been causing a heavy economic loss concerning fertility rate, milk production, and animal growth (Han et al., 2017). In yaks, diarrhea-causing pathogens, i.e., Cryptosporidium parvum, bovine viral diarrhea (BVD) virus, and Escherichia coli have been reported previously (Deng et al., 2015; Qi et al., 2015; Li et al., 2018). Although many measures have been employed to improve hygiene and feeding management with extensive drugs, the problem is still at its peak (Cho et al., 2013).
Intestinal tract is colonized by a large and diverse type of microbial microbiota (Xu et al., 2019). This community produces extensive metabolic products in the intestine, which interact intimately with host cells to maintain physiological processes and functions, i.e., nutrition absorption, host metabolism, and immunity (Backhed et al., 2012; Xu et al., 2019; Wei et al., 2020). Mainly, microbiota benefits the host through intestinal epithelium by protecting it and producing beneficial metabolites, which helps in food digestion and against pathogenic invasion. The gut microbiome can convert fermentable dietary fibers into short-chain fatty acids (SCFA) that provide additional energy to the host (Blaut, 2015). These SCFA are organic carboxylic acids with less than 6 carbon atoms of the acetate; propionate and butyrate are the most abundant extension in the intestine (Alam et al., 2000). A previous study reported that diets containing alfalfa meal and commodity-concentrated fiber could drop diarrhea rate via metabolic interactions between hindgut microbiota and SCFA in piglets (Liu et al., 2018). These SCFA act as ligands for G-protein coupled receptors by activating anti-inflammatory signaling (Parada Venegas et al., 2019). Metagenomics sequencing is commonly utilized in microbial organisms, as it provides the accurate classification of microbiota species and annotation to the bacteria at a functional level rather than functional prediction (Mukhuba et al., 2019; Luan et al., 2020; Tian et al., 2020).
It is generally accepted that microbiota composition and function contribute to the host’s health status (Long et al., 2020). Previously, dysfunctional gut microbiota was related to diseases like human inflammatory bowel disease, diabetes, and cardiovascular disease (Wang et al., 2011; Wei et al., 2020). The imbalance of such intestinal microbiota may cause diarrhea due to the growing conditional pathogens, mucosal barrier damage, immunity dropping, and intestinal permeability (Long et al., 2020). However, it is still unclear how the changed microbiota can cause the emerging diarrheal disease in yaks. Hence, this study was conducted to explore such potential pathogens and short-chain fatty acid changes in diarrheal yaks.
Materials and Methods
Sample Collection
We visited 10 family yak farms with diarrhea outbreaks during June and July 2019 (Table 1) in Hongyuan, Sichuan, China. The prevalence of diarrhea in farms was estimated by consulting animal owners as these bovines were free ranged, having grasslands without concentrated feed on the plateau. A total of 100 fresh fecal samples were collected from diarrheal (n = 60) and healthy (n = 40) yak calves in 2~3 months. All the fecal samples were frozen immediately in liquid nitrogen and then transported to the laboratory of Huazhong Agricultural University, Wuhan, China. Samples were kept at −80°C for further analysis.
DNA Extraction and Mixing of Samples
The genomic DNA from fecal samples were extracted by QIAamp Fast DNA Stool Mini Kit (QIAGEN, Venlo, NL) following the manufacturer’s instructions. Genomic DNA samples were stored at −20°C before further assessment. The quantity and quality of extractions were measured using a NanoDrop ND-1000 spectrophotometer (Thermo Fisher Scientific, Waltham, MA, USA), agarose gel electrophoresis, and Quant-iT PicoGreen dsDNA Assay Kit (Invitrogen). Every 10 samples from different farms were then mixed and gained 6 diarrheal group samples (D1, D2, D3, D4, D5, D6) and 4 normal group samples (NA, NB, NC, ND).
Library Construction and Sequencing
Metagenome shotgun sequencing libraries (400 bp) were constructed using Illumina TruSeq Nano DNA LT Library Preparation Kit. Each library was sequenced by employing the Illumina HiSeq X-ten platform (Illumina, USA) with PE150 strategy (Shanghai, China).
Sequence Analysis
Further analysis to achieve quality-filtered reads, the sequencing adapters were removed from raw sequencing reads by using Cutadapt (v1.2.1) (Martin, 2011). Low-quality reads were trimmed by performing a sliding window algorithm. Reads were aligned to the host genome via BWA (http://bio-bwa.sourceforge.net/) to remove host gene contamination (Li and Durbin, 2009). Quality-filtered reads were then de novo assembled to construct the metagenome for each mixed sample based on the iterative De Bruijn graph assembler for sequencing data with highly uneven depth (IDBA-UD) (Peng et al., 2012). All coding regions (CDS) of metagenomic scaffolds longer than 300 bp were predicted by MetaGeneMark (http://exon.gatech.edu/GeneMark/metagenome) (Zhu et al., 2010). CDS sequences samples were clustered by CD-HIT at 90% protein sequence identity to obtain a nonredundant gene catalog (Fu et al., 2012). Gene abundance in each sample was estimated (http://soap.genomics.org.cn/) based on aligned read number. The lowest common ancestor taxonomy of the nonredundant genes was obtained by aligning them against the NCBI-NT database by BLASTN (p-value <0.001). Similarly, the functional profiles of the nonredundant genes were obtained and annotated in public databases including the Golang (GO), Kyoto Encyclopedia of Genes and Genomes (KEGG), Evolutionary genealogy of genes were done through: Non-supervised Orthologous Groups (EggNOG), and Carbohydrate-Active enZYme (CAZy) by utilizing DIAMOND (Buchfink) alignment algorithm (Buchfink et al., 2015). The normalization of reads per sample was performed prior to statistical analysis to ensure that bias is not caused by sampling at different depths.
Comparing the Difference of Intestinal Microbiota Between Normal and Diarrheal Yaks
Based on the taxonomic and functional profiles of nonredundant genes, linear discriminant analysis effect size (LEfSe) was performed to detect differentially abundant taxa and functions across the groups using default parameters (Segata et al., 2011). Beta diversity analysis was performed to investigate microbial communities’ compositional and functional variations across diarrheal and healthy yak samples through Bray–Curtis distance metrics (Bray and Curtis, 1957). Visualization was done via principal coordinate analysis (PCoA), nonmetric multidimensional scaling (NMDS), and unweighted pair-group method with arithmetic mean (UPGMA) hierarchical clustering (Ramette, 2007). Differential gene (upregulated gene and downregulated gene) abundance analysis was performed via DESeq at fold change ≥2 and p-value <0.01 (Backhed et al., 2015). Metagenomics binning analysis was carried out using Maxbin2 and Maxbat2 (Sieber et al., 2018) at >50% genome completeness and <10% contamination rate.
Extraction of Fatty Acids From Fecal Samples
Firstly, 20 mg from each sample was taken out and combined with 1 ml phosphoric acid (0.5% v/v) in a sterile 2-ml EP tube and then mixed thoroughly via vortex and ultrasonication for 10 and 5 min, respectively. Secondly, a 0.1-ml sample was taken out and then placed in a sterile 1.5-ml EP tube along with 0.5 ml MTBE (CAS No. 1634-04-4). The final product was mixed thoroughly via vortex and ultrasonication for 3 and 5 min, respectively. Thirdly, at 12,000 rpm, the sample was centrifuged at 4°C for 10 min. After taking out 0.2 ml from the supernatant, 10 extracted samples from the same group were mixed and vortexed for 1 min. Finally, a 0.2-ml mixture was taken out and transferred into a vial for sample detection and further analysis using GC-MS/MS (Agilent).
Qualitative and Quantitative Analysis of SCFA
Total ion current (TIC) and standard quality of all mixture samples were detected through GC-MS/MS (Agilent) using the procedures and parameters shown in Table 2. Quality control analysis of the samples was carried out to ensure the method’s validity. The standard quality of the samples was checked thrice to measure the instrument stability. This standard quality was tested in every ten samples to monitor the repeatability of the analysis process. Qualitative and quantitative analyses of SCFA were performed by Agilent MassHunter. Standard curves for all SCFA were generated by detecting standard quality control samples, which were caproic acid (CAS No. 64-19-7), isovaleric acid (CAS No. 79-09-4), valeric acid (CAS No. 79-31-2), butyric acid (CAS No. 107-92-6), propionic acid (CAS No. 503-74-2), acetic acid (CAS No. 109-52-4), isobutyric acid (CAS No. 142-62-1), 2-methylpentanoic acid (CAS No. 97-61-0), MTBE (CAS No. 1634-04-4), and phosphoric acid (CAS No. 7664-38-2).
Statistical Analysis
The prevalence of diarrhea in different farms was analyzed through IBM SPSS Statistics (SPSS 22.0) using Chi-square (results were followed up as upper and lower limits of prevalence). Quantitative analyses of SCFA were expressed as means ± standard deviation (SD). Whereas, the difference of SCFA among the groups was analyzed via Wilcoxon test and fold changes through piloting SPSS (IBM, 22.0). p-Values <0.05 were considered statistically significant. t-Test was also performed to compare intestinal microbiota differences using IBM SPSS Statistics. Annotated analysis was performed via MetaPhlAn2 (http://huttenhower.sph.harvard.edu/metaphlan2, Version 2.0) compared with the database (Kanehisa et al., 2014). Functional profile analysis was performed by annotating against the GO, KEGG, EggNOG, and CAZy databases (Kanehisa et al., 2006; Cantarel et al., 2009; Kanehisa et al., 2014; Powell et al., 2014).
Results
Data Deposition
The raw sequences data were deposited in the BioSample database with the accession number: SAMN16091789-SAMN16091798.
Prevalence of Diarrhea in Yaks
Diarrhea in yaks was found in all farms (10/10), especially in calf farms (100%), while 80% (8/10) of farms reported diarrhea in adult yaks. The overall prevalence of diarrhea ranged from 0% to 4% and 15% to 20%. While in calves and adults, the prevalence ranged from 15% to 25% and 5% to 10%, respectively (Table 1). A significant difference was observed in both upper (p < 0.001) and lower limits of prevalence (p < 0.01) (Supplementary Figure S2).
Sequencing Data of Yak Microbiota Samples and Gene Abundance Distribution
Overall, 445,199,120 total reads and 445,089,080 clean reads were obtained from diarrheal yaks, while 285,976,660 and 285,951,940 total and clean reads were obtained from normal yaks. Moreover, 66.30 and 42.67 Gb clean bases were found in diarrheal and normal yak groups, respectively. The Q20 and Q30 in both groups was more than 97% and 92%, which confirmed reliable and accurate base recognitions (Bolger et al., 2014). No significant difference (p > 0.05) was observed in total reads, clean reads, Q20, and Q30. However, a significant difference was found in GC content between diarrheal (44.69%~46.08%) and normal yaks (46.12%~46.38%) (p < 0.05) (Supplementary Figure S3). According to the violin box plot, the degree of dispersion in gene abundance distribution was higher in diarrheal than normal yaks (Supplementary Figure S4).
Species Composition and Abundance Analysis
The abundance of Firmicutes and Proteobacteria was found to be significantly lower in yaks with diarrhea than in normal yaks (p < 0.05) (Supplementary Figure S5).
Firmicutes and Bacteroidales were found primarily in both groups (Supplementary Figure S6). Principal component analysis (PCA) found the left-side location of D1, D2, D3, D4, D5, and D6 groups. While NA, NB, NC, and ND groups were located on the right side in two-dimensional graphic representation. Samples in normal yaks were concentrically distributed as compared with the diarrheal yaks (Supplementary Figure S7A). Compared with normal animals, Bacteroidetes (p < 0.01) and Apicomplexa (p < 0.05) were significantly higher in diarrheal yaks, while Firmicutes (p < 0.05) and Euryarchaeota (p < 0.001) were significant at lower levels (Figure 1).

Figure 1 Comparing intestinal microbiota difference between different yaks at phylum level. D, diarrheal group samples; N, normal group samples.
Clostridia and Bacteroidia were observed mainly in normal yaks at the class level, while Bacteroidia, Clostridia, and Bacilli were dominated in diarrheal animals (Supplementary Figure S8). PCA showed that diarrheal and normal yak samples were infrequent and together, respectively (Supplementary Figure S7B). Compared with normal animals, Aconoidasida (p < 0.05) was significantly higher in diarrheal yaks, while Clostridia (p < 0.05), Methanobacteria (p < 0.001), Flavobacteriia (p < 0.001), Deltaproteobacteria (p < 0.001), Alphaprotebacteria (p < 0.01), and Cytophagia (p < 0.001) were significantly lower in number (Supplementary Figure S9).
At the order level, Clostridiales was higher in normal yaks, while Bacteroidales was higher in diarrheal animals (Supplementary Figure S10). PCA showed that the samples in diarrheal animals were far from each other than normal animals (Supplementary Figure S7C). In comparison with normal animals, Bacteroidales (p < 0.01) and Piroplasmida (p < 0.05) were significantly higher, while Clostridiales (p < 0.05), Methanobacteriales (p < 0.001), Flavobacteriales (p < 0.001), Cytophagales (p < 0.001), Spirochaetales (p < 0.001), and Marinilabiliales (p < 0.001) were significantly lower in diarrheal yaks (Supplementary Figure S11).
At the family level, Ruminococcaceae and Lachnospiraceae were found mainly in normal yaks, while Bacteroidaceae was found in diarrheal yaks with high abundance (Supplementary Figure S12). PCA showed that samples in diarrheal animals were located far from each other than normal animals (Supplementary Figure S7D). Compared with normal animals, Bacteroidaceae (p < 0.001), Staphylococcaceae (p < 0.05), and Babesiidae (p < 0.05) were higher in diarrheal yaks, while Ruminococcaceae (p < 0.05), Rikenellaceae (p < 0.001), Clostridiaceae (p < 0.05), Eubacteriaceae (p < 0.001), Methanobacteriaceae (p < 0.001), Oscillospiraceae (p < 0.001), Paenibacillaceae (p < 0.001), Flavobacteriaceae (p < 0.001), Muribaculaceae (p < 0.001), Spirochaetaceae (p < 0.001), Clostridiales Family XIII. Incertae Sedis (p < 0.001), Erysipelotrichaceae (p < 0.05), and Eggerthellaceae (p < 0.001) were remarkably lower (Supplementary Figure S13).
Bacteroides and Clostridium were found higher at the genus level in normal yaks, while Bacteroides was the main genus in diarrheal yaks (Supplementary Figure S14). PCA indicated that diarrheal samples were located far from normal animals (Supplementary Figure S7E). Compared with normal animals, Bacteroides (p < 0.001), Staphylococcus (p < 0.05), Blautia (p < 0.05), Babesia (p < 0.05), and Butyricicossus (p < 0.05) were outstandingly higher in diarrheal yaks, while Clostridium (p < 0.01), Alistipes (p < 0.001), Ruminococcus (p < 0.001), Eubacterium (p < 0.001), Methanobrevibacter (p < 0.001), Oscilllibacter (p < 0.001), Butyrivibrio (p < 0.001), Bacillus (p < 0.05), Paenibacillus (p < 0.001), Anaerotruncu (p < 0.001), Roseburia (p < 0.05), Treponema (p < 0.01), and Lachnoclostridium (p < 0.05) were lower (Figure 2).
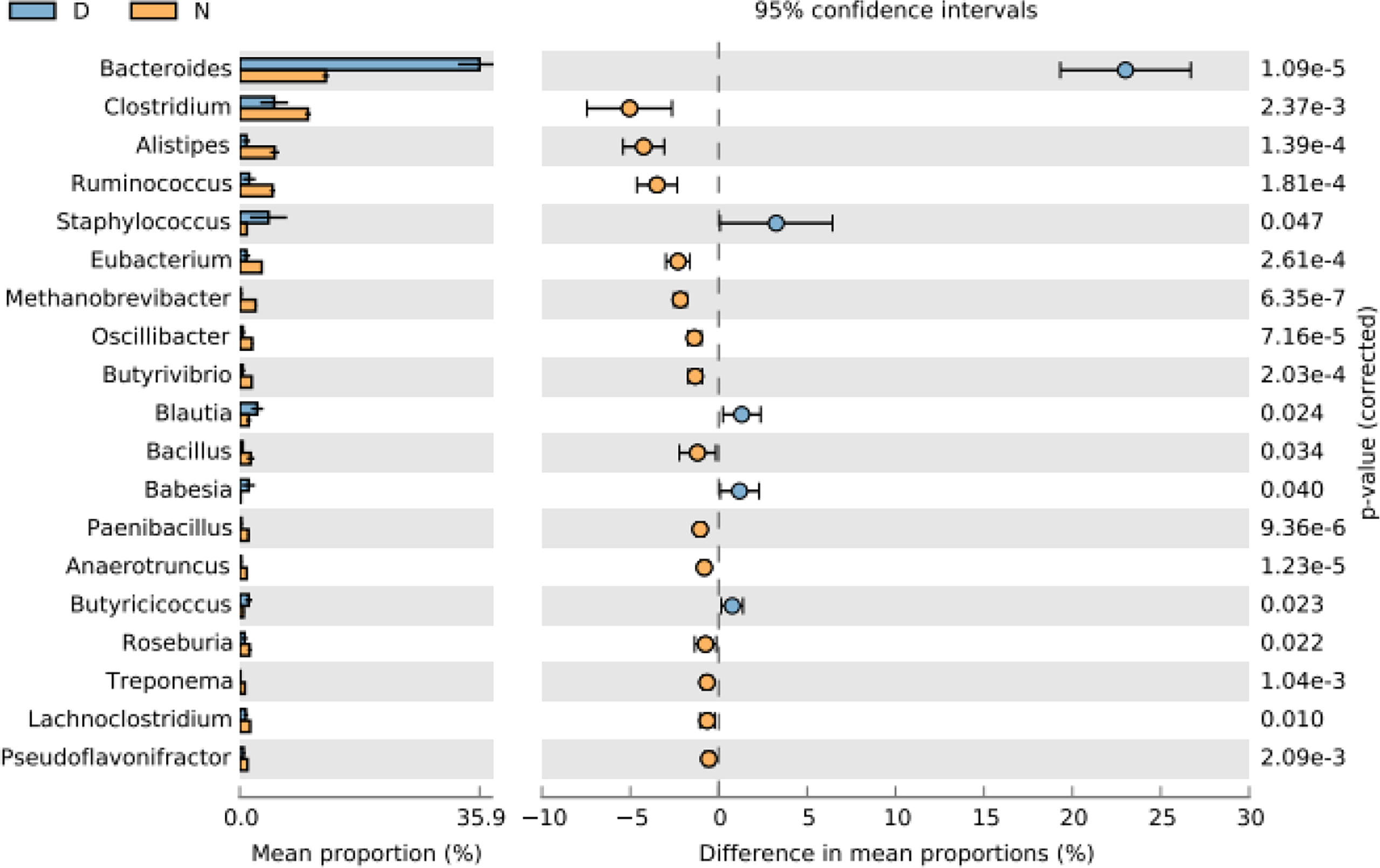
Figure 2 Comparing intestinal microbiota difference between different yaks at genus level. D, diarrheal group samples; N, normal group samples.
At the species level, Firmicutes bacterium CAG:110 was found to be most abundant in normal yaks, while Staphylococcus aureus was the main species in diarrheal yaks (Supplementary Figure S15). PCA indicated that samples in diarrheal animals located separately as compared with normal animals (Supplementary Figure S7F). Compared with normal animals, Staphylococcus aureus (p < 0.05), Bacteroides coprophilus (p < 0.01), Bacteroides plebeius (p < 0.01), Butyricicoccus pullicaecorum (p < 0.01), Babesia ovata (p < 0.05), Fusobacterium mortiferum (p < 0.05), [Ruminococcus] gnavus (p < 0.01), Anaplasma phagocytophilum (p < 0.05), Bacteroides fluxus (p < 0.05), Firmicutes bacterium CAG:424 (p < 0.05), viruses (p < 0.05), Fournierella massiliensis (p < 0.05), Bacteroides vulgatus (p < 0.05), and Klebsiella pneumoniae (p < 0.05) were higher in diarrheal yaks, while Firmicutes bacterium CAG:110 (p < 0.01), Clostridiales bacterium (p < 0.001), Ruminococcaceae bacterium (p < 0.001), Clostridium sp. CAG:413 (p < 0.001), Clostridia bacterium (p < 0.001), Firmicutes bacterium CAG:137 (p < 0.001), Methanobrevibacter olleyae (p < 0.001), Bacteroidales bacterium WCE2008 (p < 0.001), Ruminococcus flavefaciens (p < 0.001), Methanobrevibacter ruminantium (p < 0.001), Bacteroidete bacterium (p < 0.001), Anaerotruncus sp. Cag:390 (p < 0.001), Clostridium sp. CAG:448 (p < 0.001), Firmicutes bacterium (p < 0.01), Firmicutes bacterium CAG:124 (p < 0.001), and Firmicutes bacterium CAG:170 (p < 0.001) were significantly lower in number (Figure 3).
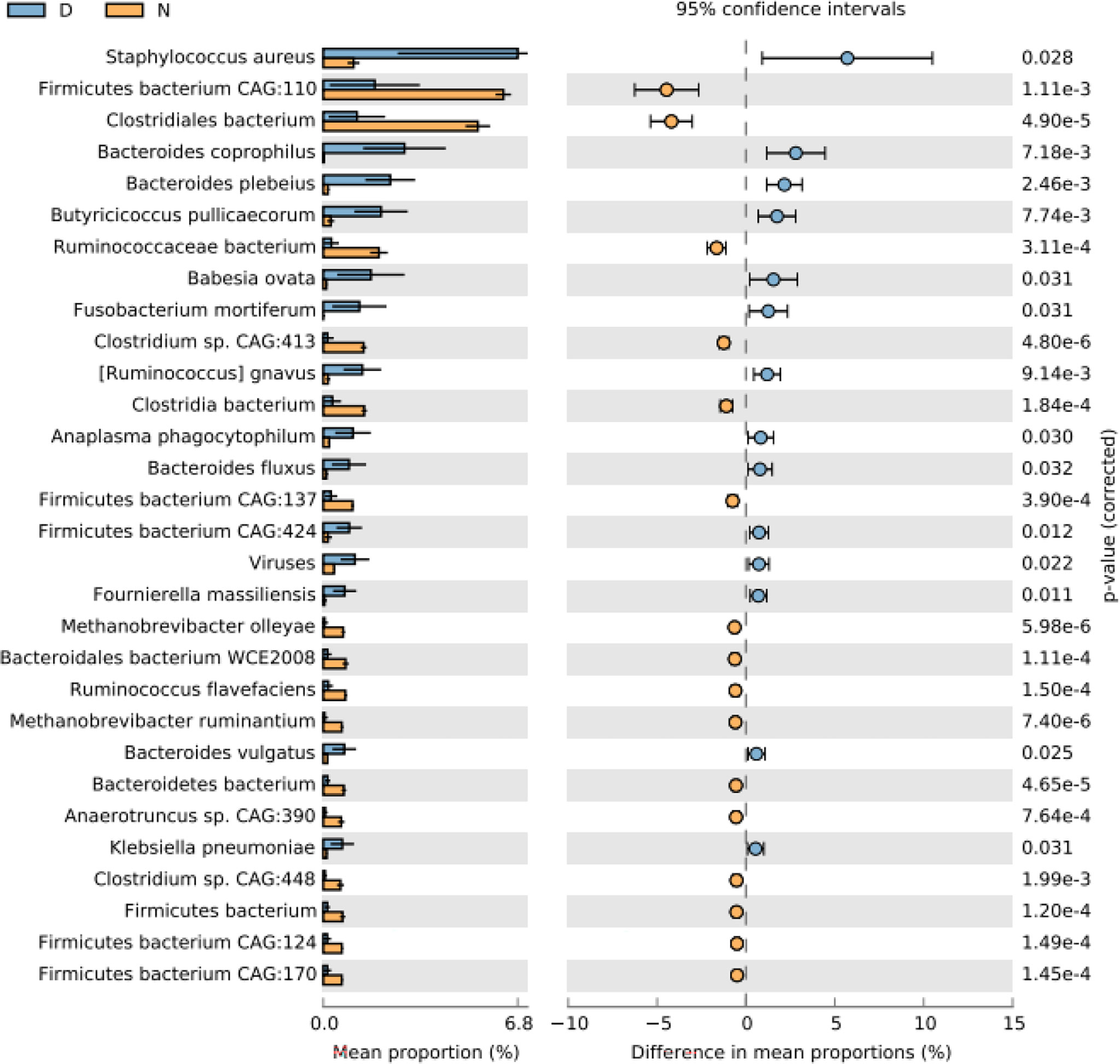
Figure 3 Comparing intestinal microbiota difference between different yaks at species level. D, diarrheal group samples; N, normal group samples.
The statistics of significant compositional species compared with normal animals are shown in Figure 4. The circus map indicated that the phylum level of the two groups is mainly consisted of Firmicutes, while a significant difference was found in the case of Fusobacteria, Bacteroidetes, and Proteobacteria in both groups. While no significant difference was found in Bacteroidia, Bacilli, and Gammaproteo at the species level (Figure 5).
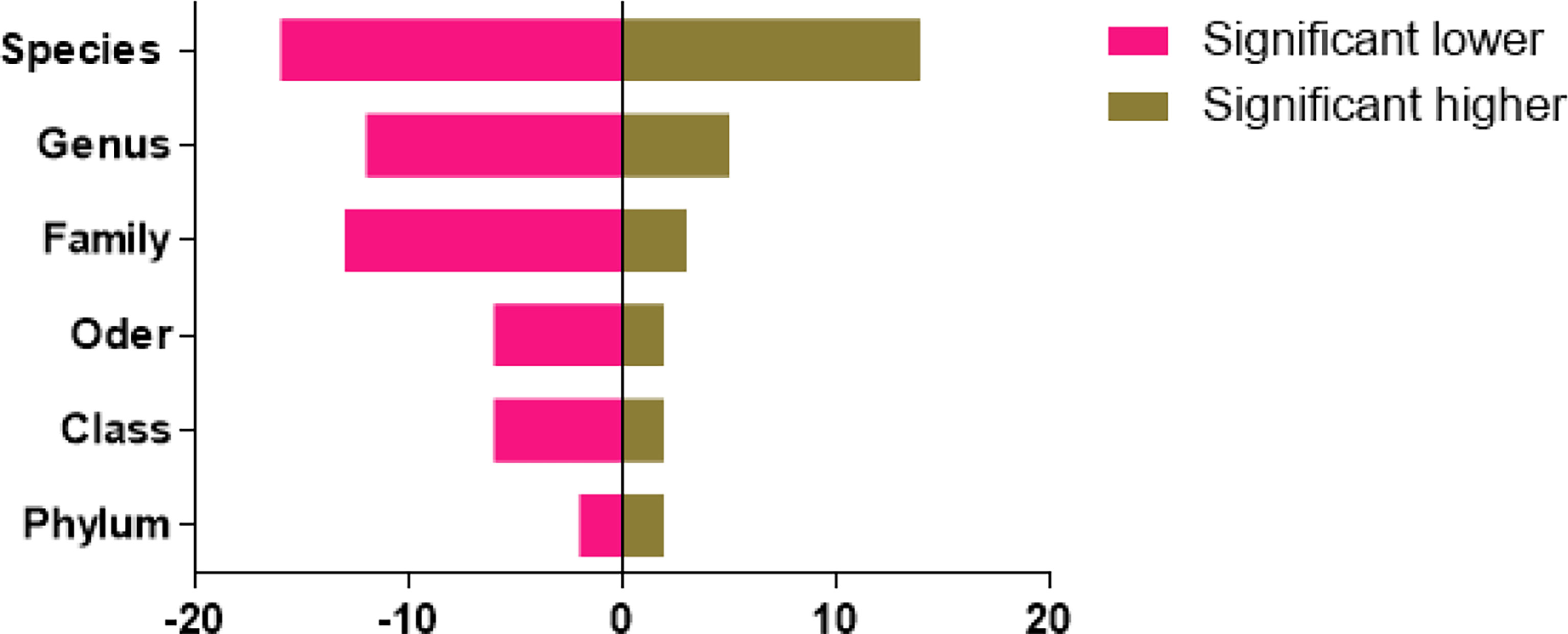
Figure 4 Statistics of significant species composition of diarrheal animals in different levels compared with normal animals (x-axis: difference numbers; y-axis: different levels).
Functional Analysis of Intestinal Yak Microbiota
Annotated scores of one having HSP >60 bits were selected for analyzing relative abundance at different functional levels (Qin et al., 2012; Karlsson et al., 2012; Karlsson et al., 2013; Backhed et al., 2015). In total, 354,990, 486,219, 778,943, 867,820, 366,984, and 188,719 nonredundant genes were found in the GO, eggnog, KEGG, NR, Swissport, and CAZy databases, respectively. In KEGG, nonredundant genes were related to cellular community and energy metabolism and 40 more metabolic pathways in all yaks. Nonsignificantly, lower nervous system, development, and nucleotide metabolism were found in diarrheal yaks (Figure 6).
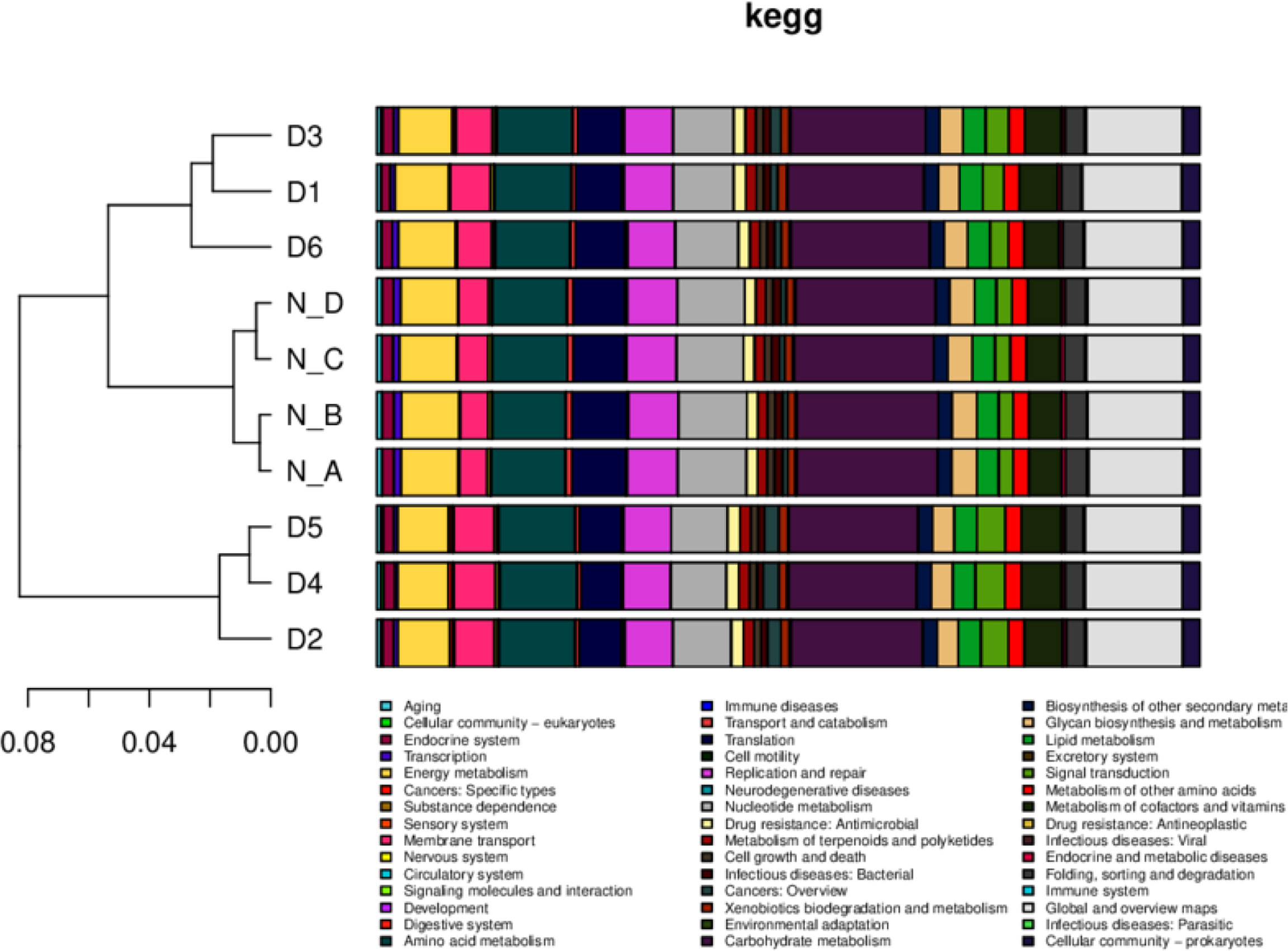
Figure 6 The relative functional abundance analysis. KEGG. D1, D2, D3, D4, D5, and D6 represent the diarrheal samples; N_A, N_B, N_C, and N_D represent the normal samples.
In eggNOG, about 24 cell metabolic pathways, i.e. wall biogenesis, chromatin structure and dynamics, were reported in all animals. In secondary metabolites biosynthesis, signal transduction mechanisms were non significantly higher in diarrheal animals, while translation, ribosomal structure and biogenesis were higher in healthy yaks (Figure 7). In CAZy, carbohydrate-binding modules, glycosyltransferases, glycoside hydrolases, polysaccharide lyases, auxiliary activities and carbohydrate esterase were found in both groups. Moreover, glycoside hydrolases were found higher in normal yaks, while glycosyltransferases were higher in diarrhea yaks non significantly (Figure 8).
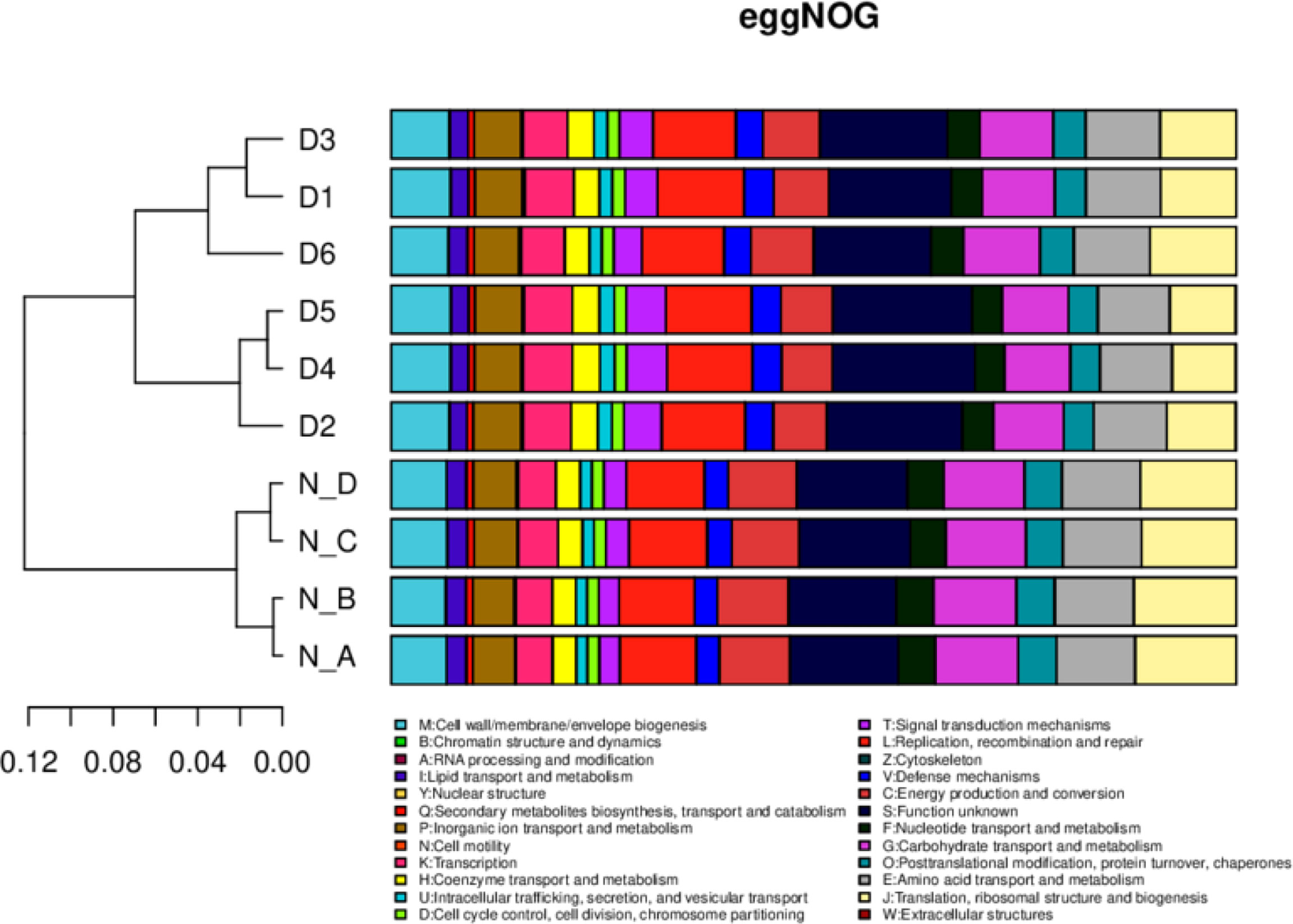
Figure 7 The functional relative abundance analysis. eggNOG. D1, D2, D3, D4, D5, and D6 represent the diarrheal samples; N_A, N_B, N_C, and N_D represent the normal samples.
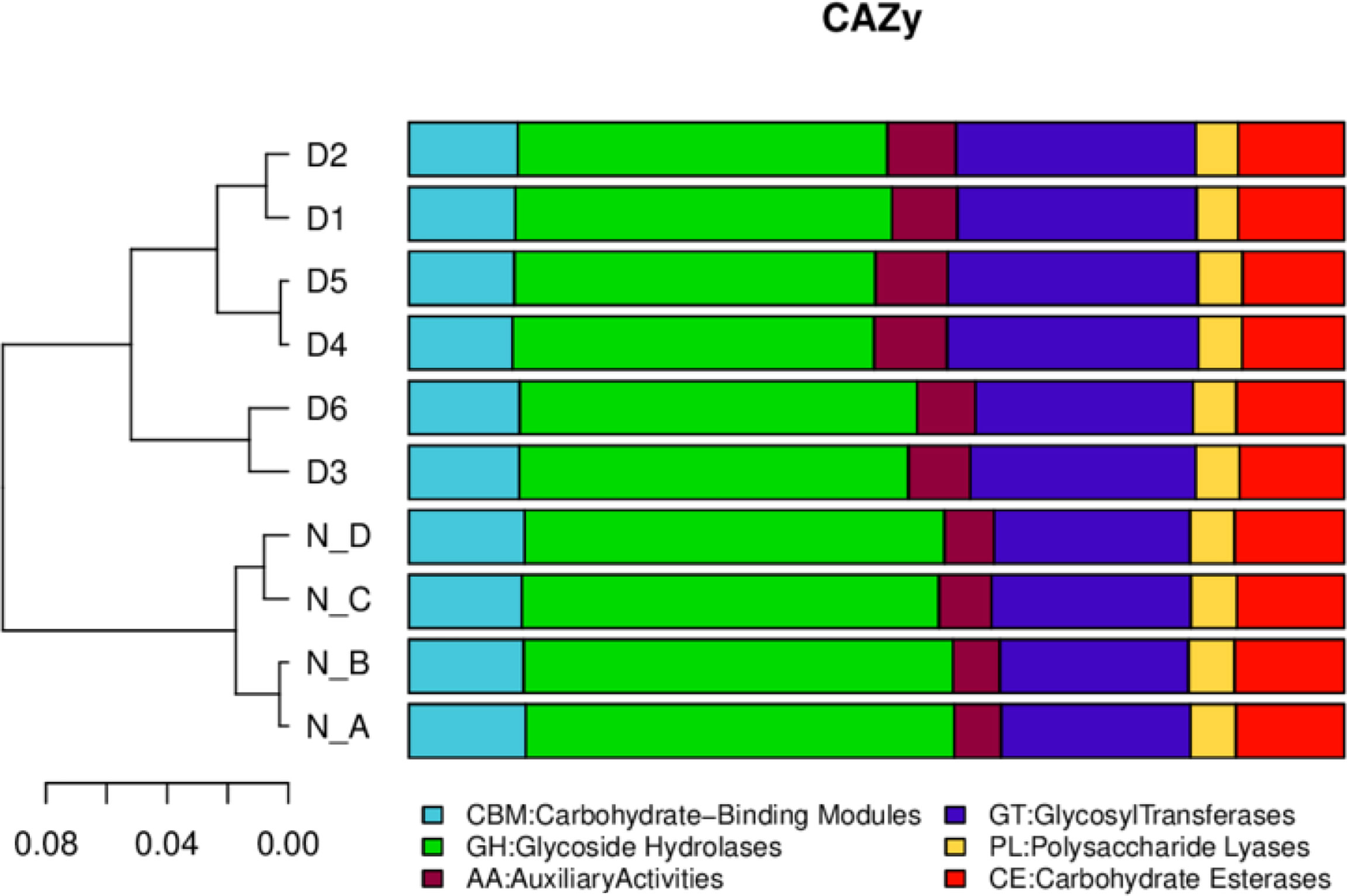
Figure 8 The functional relative abundance analysis. CAZy. D1, D2, D3, D4, D5, and D6 represent the diarrheal samples; N_A, N_B, N_C, and N_D represent the normal samples.
Among 366,163 significant differential abundance genes,141,305 were upregulated and 224,858 were downregulated in diarrheal yaks compared with normal yaks via DESeq analysis (Supplementary Figure S16A). Metagenomics binning analysis with bin 33 (Bacteroidales) (p < 0.05) was significantly higher in diarrheal animals, while bin 10 (p < 0.0001), bin 30 (Clostridiales) (p < 0.05), bin 51 (Lactobacillales) (p < 0.05), bin 8 (Lachnospiraceae) (p < 0.05), and bin 47 (Bacteria) (p < 0.05) was significantly higher in normal animals (Supplementary Figures S17, S18). In the current study, 6 out of 7 SCFA were uncovered significantly lower in diarrheal yaks from 100 mixed fecal samples by employing GC-MS/MS (p < 0.05) (Figure 9). Statistical analysis showed that SCFA acetic acid (53.8%) accounted for most of the acetate (50%–70%) in the intestine, which represents the major KEGG signal pathway (Figure 10).
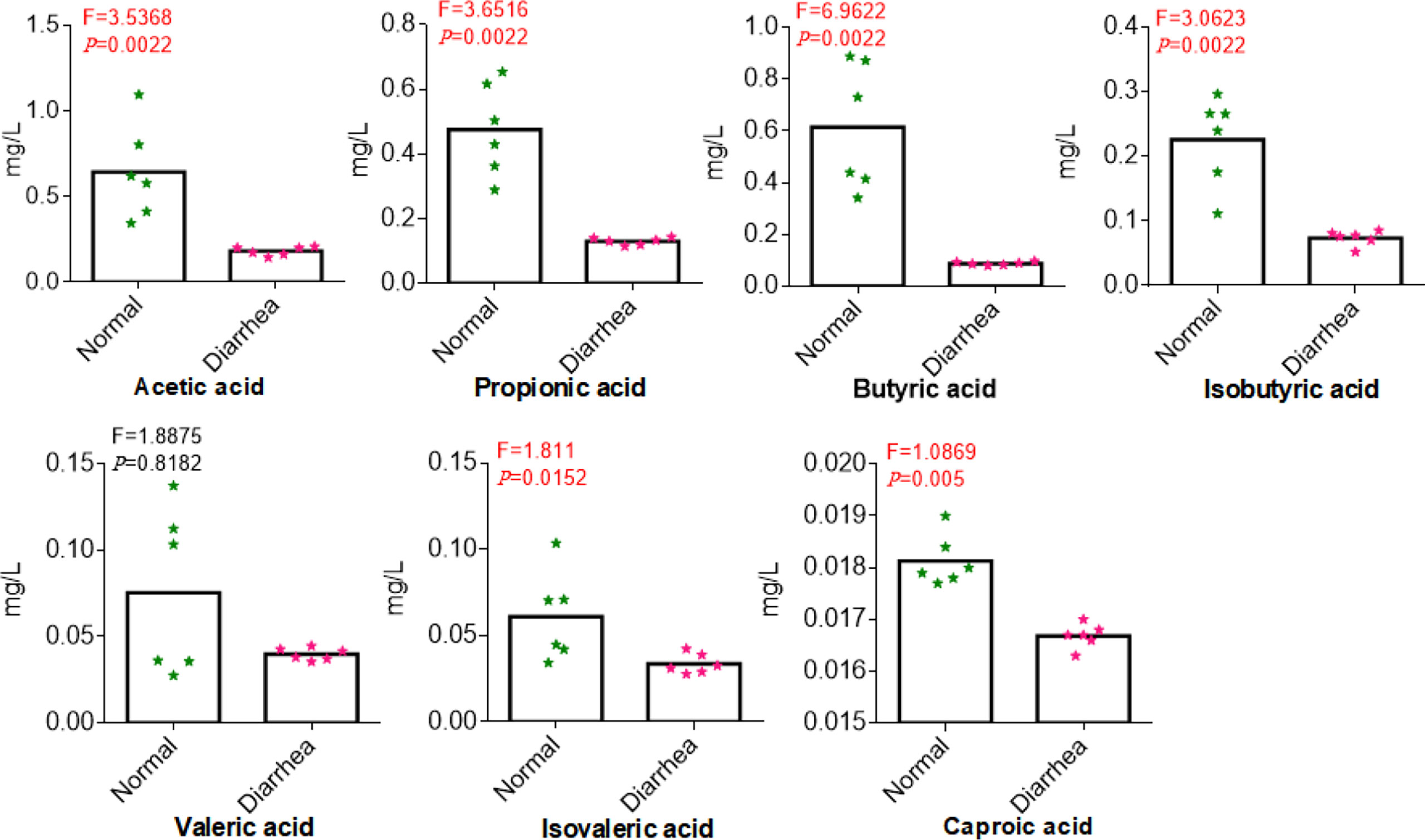
Figure 9 Comparing the concentrations of SCFA in normal and diarrhea groups. The x-axis corresponds to SCFA, and the y-axis corresponds to the concentration of SCFA.
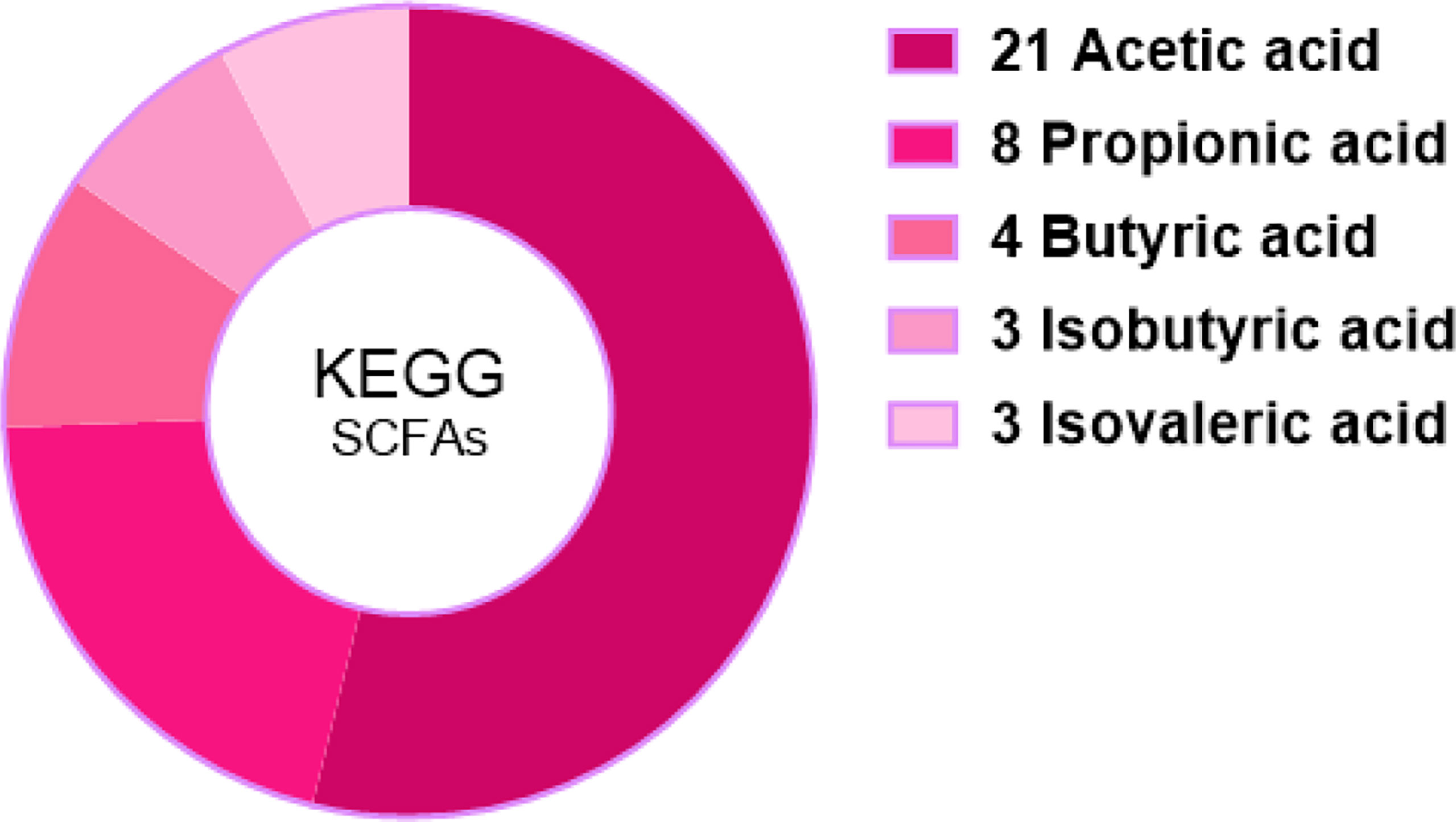
Figure 10 Statistic analysis of SCFA-relevant KEGG signal pathways in the intestinal metabolism through kanehisa laboratories.
DESeq analysis was employed to uncover significant differential abundance genes between two yak groups at fold change ≥2 and p-value <0.01 (Anders and Huber, 2010). There were 366,163 significantly essential differential abundance genes in diarrheal yaks compared with normal yaks, with 141,305 upregulated and 224,858 downregulated (Supplementary Figure S16A). Differential abundant genes were compared against the cluster of orthologous protein database. Most of the genes were related to amino acid metabolism, replication, recombination, cell wall biogenesis, carbohydrate transportation and metabolism, translation, ribosomal structure, and biogenesis (Supplementary Figure S16B). Annotation of abundant differential genes in the KEGG path showed the relationship of the gene with metabolism (Supplementary Figure S19A). Enrichment analysis of abundant differential genes in the KEGG pathway revealed that 24 genes showed a significant performance, such as carbohydrate metabolism gene, the global and overview map gene, the amino acid metabolism gene, etc. The enrichment factor in the x-axis represented the significant enrichment level of differentially expressed abundant genes. Lipopolysaccharide biosynthesis was at the highest level of differentially expressed abundant genes (Supplementary Figure S19B). Most significantly, different genes were related to the ribosomes (p < 0.001), peptidoglycan biosynthesis (p < 0.001), and homologous recombination (p < 0.001).
Binning Analysis of the Metagenome of Intestinal Microbiota in Yaks
Metagenomics binning analysis revealed 9 bins with genome completeness >50% and contamination rate <10% in yaks (Table 3). The genome completeness was 93.10% to 99.31% containing Bacteria, Selenomonadales, Clostridiales, Firmicutes, Lactobacillus, and Bacteroidetes. Through heat map of 76 bins, bin 33 (p < 0.05) was significantly higher in the diarrheal group, while bin 10 (p < 0.0001), bin 30 (p < 0.05), bin 51 (p < 0.05), bin 8 (p < 0.05), and bin 47 (p < 0.05) was significantly higher in the normal group (Figure 5; Supplementary Figure S20). The genomics of those bins were Bacteroidales (bin 33), Clostridiales (bin 30), Lactobacillales (bin 51), Lachnospiraceae (bin 8), and Bacteria (bin 47).
Quantitative Analysis of SCFA in Yaks
Sample quality control analysis showed that the TIC from different samples nearly overlapped completely, which indicated that the current data were repeatable and reliable (Supplementary Figure S20A). The TIC from yak mixture samples showed several single waves without overlapping, which revealed valid results of SCFA (Supplementary Figure S20B). In the present study, all the correlation coefficients (R2) of each equation of linear regressions were over 0.994≈1.000, which ensured the accuracy of SCFA values (Table 4).
Discussion
In Norway, about US$10 million loss was noted due to calves death affected by diarrhea in 2006 (Østerås et al., 2007). As an agricultural country, the development of animal husbandry is important, especially in Hongyuan (China), like plateau areas. In our study, the prevalence of diarrhea in yaks was estimated at about 15%–25% and 5%–10% in calves and adults, respectively (Table 1). Diarrhea in yaks was significantly higher in yak calves (Supplementary Figure S2), which was in line with the widely accepted knowledge that morbidity of diarrhea in calves is more serious (Dore et al., 2019). Therefore, discovering the potential causes of this emerging diarrhea is urgent and meaningful, especially on the remote plateau.
The intestinal microbiota is also considered an additional organ, which comprises billions of microorganisms. The intestinal microbiota is important in the synthesis and metabolism of nutrients, hormones, and vitamins, playing a role in drug utilization, pathogen fortification, and immune system maturation (Blaut, 2015; Mangiola et al., 2016; Rinninella et al., 2019). Therefore, the imbalance of intestinal microbiota may lead to serious diseases. Previously, we performed high-throughput sequencing of intestinal microflora from diarrheal yaks. Our study found 41 genera of bacteria in perinatal healthy yaks, while 145 genera of bacteria were only tested in healthy perinatal yaks (Han et al., 2017). Moreover, 212 genera of fungus were found in healthy yaks, and 373 and 208 genera of fungus were found in calves and diarrheal adult yaks, respectively (Li et al., 2018). However, 16s RNA sequencing was limited to the genus level. In the current study, metagenomics sequencing was employed to explore the potential pathogens of diarrhea in yaks. Violin box plot also showed the higher gene abundance in diarrhea yaks in concern with the degree of dispersion than normal yaks (Supplementary Figure S4). Such results may predict the different microbiota compositions in diarrheal and normal animals. We found more significantly lower species composition in diarrheal yaks (Supplementary Figure S5).
Staphylococcus aureus is a commonly known bacterium related to human and animal foodborne diseases (Kroning et al., 2020). This pathogen also causes orthopedic implant-associated infection, especially methicillin-resistant bacteria (Czuban et al., 2020). As infected animals are commonly treated with antimicrobial agents, thus serious antimicrobial resistance is becoming a public health concern worldwide (Kroning et al., 2020). Diseases such as gastroenteritis, nausea, vomiting, abdominal cramps, etc., are usually seen in infected individuals (Wang et al., 2020). The increase of Staphylococcus aureus in diarrheal yaks may indicate a potential threat for local herdsmen. Bacteroides coprophilus was previously reported as proinflammatory in ankylosing spondylitis (Zhou et al., 2020), which may infer with an inflammatory status of diarrhea yaks. Bacteroides plebeius was previously found significantly higher in type 2 diabetes mellitus patients (Wang et al., 2020), also regarded as a biomarker of this disease. Thus, the increase of Bacteroides plebeius in diarrhea animals means the abnormal glucose metabolism in yaks.
The butyrate-producing bacteria Butyricicoccus pullicaecorum is commonly linked with inflammatory conditions of the intestinal ecosystem (Andrade et al., 2020), which may cause inferred inflammatory response during diarrhea in yaks. Though Babesia ovata is a low pathogenic species, its infection may lead to severe damage in cattle when coinfected with Theileria orientalis (Nguyen et al., 2019). A previous study reported that the prevalence of T. orientalis in yaks was 9.7% on the plateau (Li et al., 2017). The infection of T. orientalis may be the main reason for bloody diarrhea in yaks (Supplementary Figure S1). Fusobacterium mortiferum was usually isolated from Crohn’s and Behcet’s patients (Garcia-Saura et al., 2019). Also, Ruminococcus gnavus is a Crohn’s disease-associated pathobiont (Yu et al., 2020), which was in line with the diarrhea symptoms in yaks. Anaplasma phagocytophilum is a commonly reported emerging tick-borne zoonotic pathogen causing anaplasmosis (Adamska, 2020). This bacterium primarily infects host neutrophils, which break the first-line immune defensive barrier in mammalians (Tang et al., 2020). The infected animals show typically anemia (Langenwalder et al., 2020), which reveal that A. phagocytophilum may contribute to diarrhea in yaks. Bacteroides fluxus is a pathogenic species of Bacteroides that displays numerous and high rates of antibiotic resistance. Higher abundance of Bacteroides fluxus means this bacterium plays a potential role in diarrhea. Firmicutes bacterium was associated with lipogenesis metabolism in animals with nonalcoholic fatty liver disease (Paul et al., 2019). The increased Firmicutes bacterium (CAG:424) in diarrheal yaks may cause dyslipidemia. Bovine viral diarrhea and Rotavirus were also reported in yaks (Li et al., 2018; Yan et al., 2020), which could infer that the increased abundance of these viruses may cause diarrhea in yaks.
Fournierella massiliensis is a new human-associated member of the family Ruminococcaceae (Togo et al., 2017), which may have little relationship with diarrhea. Bacteroides vulgatus was the main cause of polycystic ovary syndrome through disrupted ovarian functions and aggravated insulin resistance (Qi et al., 2019). This means increment of Bacteroides vulgatus in yaks may cause diarrhea via affecting glycol metabolism. Klebsiella pneumonia causes many infections, i.e., pneumonia, urinary tract infection, meningitis, and bacteremia (Furlan et al., 2020), which indicates the infection status of diarrheal yaks. While Firmicutes bacterium CAG:110 (p < 0.01), Clostridiales bacterium (p < 0.001), Ruminococcaceae bacterium (p < 0.001), Clostridium sp. CAG:413 (p < 0.001), Clostridia bacterium (p < 0.001), Firmicutes bacterium CAG:137 (p < 0.001), Methanobrevibacter olleyae (p < 0.001), Bacteroidales bacterium WCE2008 (p < 0.001), Ruminococcus flavefaciens (p < 0.001), Methanobrevibacter ruminantium (p < 0.001), Bacteroidete bacterium (p < 0.001), Anaerotruncus sp. Cag:390 (p < 0.001), Clostridium sp. CAG:448 (p < 0.001), Firmicutes bacterium (p < 0.01), Firmicutes bacterium CAG:124 (p < 0.001), and Firmicutes bacterium CAG:170 (p < 0.001) were significantly lower (Figure 8C). Firmicutes bacterium CAG:110 was found to be potentially associated with swine feed efficiency variation in cecum microbiota via the utilization of dietary polysaccharides and dietary protein (Quan et al., 2020). It means the dropped abundance of Firmicutes bacterium CAG:110 decreases feed efficiency. Firmicutes bacterium CAG:137, Firmicutes bacterium (p < 0.01), Firmicutes bacterium CAG:124 (p < 0.001), and Firmicutes bacterium CAG:170 (p < 0.001) belong to host energy uptake or storage-limiting related Firmicutes, which are one of the most abundant bacteria in animals and human beings (Ley et al., 2005). The lower of Firmicutes bacteria may linked to glycol metabolism, which may further cause diarrhea.
Butyrate-producing Clostridiales bacterium is associated with protecting the host from colorectal cancer, immune, and metabolic disorders (Pichler et al., 2020). It means dropped Clostridiales bacterium in yaks may contribute to diarrhea. Ruminococcus flavefaciens works with noncellulolytic Treponema or Butyrivibrio species that can accelerate cellulose digestion (Cheng et al., 1991). The lower Ruminococcus flavefaciens in diarrheal yaks may decrease the cellulose efficiency. Previously, more deficient Ruminococcaceae bacterium was found in hospitalized patients, cirrhosis (Paul et al., 2019), and diarrhea foals (Schoster et al., 2017). The deceased of this bacterium may provide an insight that Ruminococcaceae bacterium has a relationship with diarrhea in yaks. CAG:413, CAG:448 and Clostridia bacterium belonged to the Clostridium genus, recognized as a beneficial bacteria to the host (Guo et al., 2020). The lower of this three Clostridium spp. may promote diarrhea in yaks. Methanobrevibacter olleyae and Methanobrevibacter ruminantium composed the M. ruminantium clade, which belongs to the ruminant Methanobrevibacter genus (Kelly et al., 2016). These two bacteria with other Methanobrevibacter spp. compose the rumen methanogenic community (Danielsson et al., 2012), which indicates that diarrheal yaks also have decreased methane production. Bacteroidales bacterium WCE2008 is a Bacteroidales species accepted as “beneficial” microbes (Anderson, 1996). A previously dropped abundance of Bacteroidales was found in pediatric patients with CD (Anderson, 1996), which may infer that the imbalance of this bacterium is related to diarrhea in yaks. Higher abundance of Bacteroidete bacterium is related to healthy lean of host, as it can generate three main SCFA, butyrate, acetate, and propionate (Davis, 2018). The decreased Bacteroidete bacterium in animals contribute to diarrhea. Anaerotruncus can utilize cheese whey to produce acetic and butyric acids (Gao et al., 2016). The decreased Anaerotruncus sp. CAG:390 in diarrhea may affect fatty acid metabolism in ruminants.
Microbiota is a key regulator of digestion, extraction, synthesis, and absorption of many nutrients and metabolites, i.e., bile acids, lipids, amino acids, vitamins, and SCFA (Rinninella et al., 2019). The SCFA are not only principal nutrient substrates of intestinal epithelial cells but also can regulate the epithelial barrier (Mazzawi et al., 2019). Previously, concentrations of SCFA was related with diarrhea-predominant irritable bowel syndrome patients (Mazzawi et al., 2019). SCFA could mitigate adenine-induced chronic kidney disease (Mikami et al., 2020); preoperative fecal levels of SCFA had an important impact on the occurrence of postoperative infectious complications in patients with esophageal cancer (Motoori et al., 2020). SCFA in fecal samples was commonly used to approximate gut levels, which can infer the relationship between intestinal SCFA production and fecal levels (Haenen et al., 2013). It reveals that the imbalance of gut microbiota dropped the levels of SCFA in diarrhea, so it can be supposed that SCFA has immunological and regulatory functions (Farup et al., 2016), activating anti-inflammatory signaling via acting as ligands of G-protein-coupled receptors, e.g., GPR109A, GPR41, and GPR43 (Parada Venegas et al., 2019). The current results are in line with diarrhea-dominant IBS with lower levels of SCFA (Huda-Faujan et al., 2010). Among the common SCFA, acetate (C2), propionate (C3), and butyrate (C4) are the most in number, produced by anaerobic fermentation of dietary fibers in the intestine (Parada Venegas et al., 2019). Those three SCFA accounted for 90% of SCFA produced by gut microbiota, which depict the beneficial effects on intestinal epithelial cells and immune cells in the intestinal mucosa (Rechkemmer and Vonengelhardt, 1988; Chen et al., 2020).
Acetate was reported to mediate joint inflammation in a murine gout model via inflammasome assembly and IL-1β (Vieira et al., 2015). Propionic acid was found to be increased in gut-associated Treg cells (relates to systemic immune reaction and disease amelioration) (Duscha et al., 2020). Butyrate is a primary energy source for colonocytes and can maintain intestinal homeostasis through anti-inflammatory actions via inhibiting nuclear factor-kappa β and histone deacetylation by promoting epithelial barrier function (Morrison and Preston, 2016; Parada Venegas et al., 2019). Previously, a lower abundance of butyrate-producing bacteria and fecal butyrate were found in stroke patients as higher risk factors (Zeng et al., 2019). Bacteroidetes from Firmicutes mainly produce acetate and propionate, while butyrate is mainly produced by phylum Firmicutes, i.e., Faecalibacterium prausnitzii, Clostridium leptum, Eubacterium rectale, and Roseburia spp (Louis et al., 2010).. In a previous study, Firmicutes phylum was found clearly lower in diarrheal yaks (p < 0.05) (Han et al., 2017). Genus of Clostridium_IV (p < 0.01) and Clostridium_XI (p < 0.05) were found significantly lower in diarrheal yaks except Clostridium XVIII (p < 0.01). The genera of Bacteroides (p < 0.05) and Faecalibacterium (p < 0.05) were found significantly higher in diarrhea yaks, while no significant difference was found in the genera of Eubacterium, Eubacterium, and Roseburia (Han et al., 2017). However, among all those genera, Clostridium_IV and Clostridium_XI were the dominant (Han et al., 2017), which may uncover that the decreasing of clostridium may cause the drop of SCFA (C2-C4). In the current study, isobutyric acid, isovaleric acid, and caproic acid were found significantly lower in diarrheal animals (p < 0.05), which was in line with patients suffering from cirrhosis and neuromyelitis optica spectrum disorders (Gong et al., 2019; Jin et al., 2019). As SCFA plays a critical role in mucosal integrity and immune response (Wang et al., 2019). So, the dropping of SCFA (C4-C6) may damage mucosal and inflammation response. SCFA generation bacteria of Anaerotruncus sp. CAG:390, Clostridiales bacterium and Butyricicoccus pullicaecorum are lower in number in diarrheal yaks. Although Fusobacterium mortiferum producing butyric and acetic acids increase significantly (Thompson et al., 1992). However, it could not affect the dropping trend of SCFA in diarrheal animals. Moreover, the abundance of SCFA acetic acid (53.85%) in the current study was the primary level of acetate (50%–70%) in the intestine (Lavelle and Sokol, 2020).
Conclusion
In conclusion, we estimated the prevalence of emerging diarrhea in yak calves was 15%–25% and 5%–10% in adult yaks. Besides the high prevalence of Staphylococcus aureus, Babesia ovata, Anaplasma phagocytophilum, Bacteroides fluxus, viruses, Klebsiella pneumonia, and inflammation-related bacteria, the decrease of SCFA was observed in diarrheal yaks. Our results will give insights into the prevention and treatment of emerging diarrhea issue in yaks on the plateau.
Data Availability Statement
The datasets presented in this study can be found in online repositories. The names of the repository/repositories and accession number(s) can be found in the article/Supplementary Material.
Ethics Statement
Ethical review and approval were not required for the animal study because only fresh faecal samples were collected from diarrheal animals so no ethical permission was required. Written informed consent was obtained from the owners for the participation of their animals in this study.
Author Contributions
KL, JiL, and DQ designed the research. KL, ZZ, JuL, LP, YW, and AL conducted the experiments. KL analyzed the data. KL and MS prepared the manuscript. MK revised and finalized the manuscript. All authors listed have made a substantial, direct, and intellectual contribution to the work and approved it for publication.
Funding
The current research was supported by the China Postdoctoral Science Foundation (2020M672378), China Agriculture Research System (CARS-37), and Tibet Autonomous Region Science Fund (ZDZX2018000043).
Conflict of Interest
The authors declare that the research was conducted in the absence of any commercial or financial relationships that could be construed as a potential conflict of interest.
Publisher’s Note
All claims expressed in this article are solely those of the authors and do not necessarily represent those of their affiliated organizations, or those of the publisher, the editors and the reviewers. Any product that may be evaluated in this article, or claim that may be made by its manufacturer, is not guaranteed or endorsed by the publisher.
Supplementary Material
The Supplementary Material for this article can be found online at: https://www.frontiersin.org/articles/10.3389/fcimb.2022.805481/full#supplementary-material
References
Østerås, O., Gjestvang, M. S., Vatn, S., Sølverød, L. (2007). Perinatal Death in Production Animals in the Nordic Countries – Incidence and Costs. Acta Veterinaria Scandinavica 49 (1), 1–4. doi: 10.1186/1751-0147-49-S1-S14
Adamska, M. (2020). The Role of Different Species of Wild Ungulates and Ixodes Ricinus Ticks in the Circulation of Genetic Variants of Anaplasma Phagocytophilum in a Forest Biotope in North-Western Poland. Ticks Tick Borne Dis. 11, 101465. doi: 10.1016/j.ttbdis.2020.101465
Alam, N., Meier, R., Schneider, H., Sarker, S., Bardhan, P., Mahalanabis, D., et al. (2000). Partially Hydrolyzed Guar Gum–Supplemented Oral Rehydration Solution in the Treatment of Acute Diarrhea in Children. J. Pediatr. Gastroenterol. Nutr. 31, 503–507. doi: 10.1097/00005176-200011000-00010
Anders, S., Huber, W. (2010). Differential Expression Analysis for Sequence Count Data. Genome Biol. 11, R106. doi: 10.1186/gb-2010-11-10-r106
Anderson, P. C. (1996). Clinical Immunology: Principles and Practice. J. Am. Med. Assoc. 275, 1208–1209. doi: 10.1001/jama.1996.03530390076043
Andrade, J. C., Almeida, D., Domingos, M., Seabra, C. L., Machado, D., Freitas, A. C., et al. (2020). Commensal Obligate Anaerobic Bacteria and Health: Production, Storage, and Delivery Strategies. Front. Bioeng. Biotechnol. 8, 550. doi: 10.3389/fbioe.2020.00550
Backhed, F., Fraser, C. M., Ringel, Y., Sanders, M. E., Sartor, R. B., Sherman, P. M., et al. (2012). Defining a Healthy Human Gut Microbiome: Current Concepts, Future Directions, and Clinical Applications. Cell Host Microbe 12, 611–622. doi: 10.1016/j.chom.2012.10.012
Backhed, F., Roswall, J., Peng, Y., Feng, Q., Jia, H., Kovatcheva-Datchary, P., et al. (2015). Dynamics and Stabilization of the Human Gut Microbiome During the First Year of Life. Cell Host Microbe 17, 690–703. doi: 10.1016/j.chom.2015.04.004
Blaut, M. (2015). Gut Microbiota and Energy Balance: Role in Obesity. Proc. Nutr. Soc. 74, 227–234. doi: 10.1017/S0029665114001700
Bolger, A. M., Lohse, M., Usadel, B. (2014). Trimmomatic: A Flexible Trimmer for Illumina Sequence Data. Bioinformatics 30, 2114–2120. doi: 10.1093/bioinformatics/btu170
Bray, J. R., Curtis, J. T. (1957). An Ordination of the Upland Forest Communities of Southern Wisconsin. Ecol. Monogr. 27, 326–349. doi: 10.2307/1942268
Buchfink, B., Xie, C., Huson, D. H. (2015). Fast and Sensitive Protein Alignment Using DIAMOND. Nat. Methods 12, 2. doi: 10.1038/nmeth.3176
Cantarel, B. L., Coutinho, P. M., Rancurel, C., Bernard, T., Lombard, V., Henrissat, B. (2009). The Carbohydrate-Active EnZymes Database (CAZy): An Expert Resource for Glycogenomics. Nucleic Acids Res. 37, D233–D238. doi: 10.1093/nar/gkn663
Chen, X. F., Chen, X., Tang, X. (2020). Short-Chain Fatty Acid, Acylation and Cardiovascular Diseases. Clin. Sci. (Lond.) 134, 657–676. doi: 10.1042/CS20200128
Cheng, K. J., Forsberg, C. W., Minato, H., Costerton, J. W. (1991). “24 - Microbial Ecology and Physiology of Feed Degradation Within the Rumen,” in Physiological Aspects of Digestion and Metabolism in Ruminants. Eds. Tsuda, T., Sasaki, Y., Kawashima, R. (San Diego: Academic Press), 595–624. doi: 10.1016/B978-0-12-702290-1.50031-X
Cho, Y.-I., Han, J.-I., Wang, C., Cooper, V., Schwartz, K., Engelken, T., et al. (2013). Case-Control Study of Microbiological Etiology Associated With Calf Diarrhea. Vet. Microbiol. 166, 375–385. doi: 10.1016/j.vetmic.2013.07.001
Czuban, M., Kulka, M. W., Wang, L., Koliszak, A., Achazi, K., Schlaich, C., et al. (2020). Titanium Coating With Mussel Inspired Polymer and Bio-Orthogonal Chemistry Enhances Antimicrobial Activity Against Staphylococcus Aureus. Mater. Sci. Eng. C. Mater. Biol. Appl. 116, 111109. doi: 10.1016/j.msec.2020.111109
Danielsson, R., Schnurer, A., Arthurson, V., Bertilsson, J. (2012). Methanogenic Population and CH(4) Production in Swedish Dairy Cows Fed Different Levels of Forage. Appl. Environ. Microbiol. 78, 6172–6179. doi: 10.1128/AEM.00675-12
Davis, H. C. (2018). Can the Gastrointestinal Microbiota be Modulated by Dietary Fibre to Treat Obesity? Ir J. Med. Sci. 187, 393–402. doi: 10.1007/s11845-017-1686-9
Deng, M., Ji, S., Fei, W., Raza, S., He, C., Chen, Y., et al. (2015). Prevalence Study and Genetic Typing of Bovine Viral Diarrhea Virus (BVDV) in Four Bovine Species in China. PloS One 10, e0121718. doi: 10.1371/journal.pone.0121718
Dore, V., Foster, D. M., Ru, H., Smith, G. W. (2019). Comparison of Oral, Intravenous, and Subcutaneous Fluid Therapy for Resuscitation of Calves With Diarrhea. J. Dairy Sci. 102, 11337–11348. doi: 10.3168/jds.2019-16970
Duscha, A., Gisevius, B., Hirschberg, S., Yissachar, N., Stangl, G. I., Eilers, E., et al. (2020). Propionic Acid Shapes the Multiple Sclerosis Disease Course by an Immunomodulatory Mechanism. Cell 180, 1067–106+. doi: 10.1016/j.cell.2020.02.035
Farup, P. G., Rudi, K., Hestad, K. (2016). Faecal Short-Chain Fatty Acids - a Diagnostic Biomarker for Irritable Bowel Syndrome? BMC Gastroenterol. 16, 51. doi: 10.1186/s12876-016-0446-z
Fu, L., Niu, B., Zhu, Z., Wu, S., Li, W. (2012). CD-HIT: Accelerated for Clustering the Next-Generation Sequencing Data. Bioinformatics 28, 3150–3152. doi: 10.1093/bioinformatics/bts565
Furlan, J. P. R., Savazzi, E. A., Stehling, E. G. (2020). Genomic Insights Into Multidrug-Resistant and Hypervirulent Klebsiella Pneumoniae Co-Harboring Metal Resistance Genes in Aquatic Environments. Ecotoxicol. Environ. Saf. 201, 110782. doi: 10.1016/j.ecoenv.2020.110782
Gao, J., Liu, G., Li, H., Xu, L., Du, L., Yang, B. (2016). Predictive Functional Profiling Using Marker Gene Sequences and Community Diversity Analyses of Microbes in Full-Scale Anaerobic Sludge Digesters. Bioproc. Biosyst. Eng. 39, 1115–1127. doi: 10.1007/s00449-016-1588-7
Garcia-Saura, A. G., Zapata-Perez, R., Hidalgo, J. F., Cabanes, J., Gil-Ortiz, F., Sanchez-Ferrer, A. (2019). An Uncharacterized FMAG_01619 Protein From Fusobacterium Mortiferum ATCC 9817 Demonstrates That Some Bacterial Macrodomains can Also Act as Poly-ADP-Ribosylhydrolases. Sci. Rep. 9, 3230. doi: 10.1038/s41598-019-39691-4
Gong, J. L., Qiu, W., Zeng, Q., Liu, X. Y., Sun, X. B., Li, H. J., et al. (2019). Lack of Short-Chain Fatty Acids and Overgrowth of Opportunistic Pathogens Define Dysbiosis of Neuromyelitis Optica Spectrum Disorders: A Chinese Pilot Study. Multiple Sclerosis J. 25, 1316–1325. doi: 10.1177/1352458518790396
Guo, Z., Hu, B., Han, H., Lei, Z., Shimizu, K., Zhang, L., et al. (2020). Metagenomic Insights Into the Effects of Nanobubble Water on the Composition of Gut Microbiota in Mice. Food Funct. 11, 7175–7182. doi: 10.1039/D0FO01592J
Haenen, D., Zhang, J., Souza da Silva, C., Bosch, G., van der Meer, I., van Arkel, J., et al. (2013). A Diet High in Resistant Starch Modulates Microbiota Composition, SCFA Concentrations, and Gene Expression in Pig Intestine. J. Nutr. 143, 274–283. doi: 10.3945/jn.112.169672
Han, Z., Li, K., Shahzad, M., Zhang, H., Luo, H., Qiu, G., et al. (2017). Analysis of the Intestinal Microbial Community in Healthy and Diarrheal Perinatal Yaks by High-Throughput Sequencing. Microb. Pathog. 111, 60–70. doi: 10.1016/j.micpath.2017.08.025
Huda-Faujan, N., Abdulamir, A. S., Fatimah, A., Anas, O., Shuhaimi, M., Yazid, A., et al. (2010). The Impact of the Level of the Intestinal Short Chain Fatty Acids in Inflammatory Bowel Disease Patients Versus Healthy Subjects. Open Biochem. J. 4, 53–58. doi: 10.2174/1874091X01004010053
Jin, M., Kalainy, S., Baskota, N., Chiang, D., Deehan, E. C., McDougall, C., et al. (2019). Faecal Microbiota From Patients With Cirrhosis has a Low Capacity to Ferment non-Digestible Carbohydrates Into Short-Chain Fatty Acids. Liver Int. 39, 1437–1447. doi: 10.1111/liv.14106
Kanehisa, M., Goto, S., Hattori, M., Aoki-Kinoshita, K. F., Itoh, M., Kawashima, S., et al. (2006). From Genomics to Chemical Genomics: New Developments in KEGG. Nucleic Acids Res. 34, D354–D357. doi: 10.1093/nar/gkj102
Kanehisa, M., Goto, S., Sato, Y., Kawashima, M., Furumichi, M., Tanabe, M. (2014). Data, Information, Knowledge and Principle: Back to Metabolism in KEGG. Nucleic Acids Res. 42, D199–D205. doi: 10.1093/nar/gkt1076
Karlsson, F. H., Fak, F., Nookaew, I., Tremaroli, V., Fagerberg, B., Petranovic, D., et al. (2012). Symptomatic Atherosclerosis is Associated With an Altered Gut Metagenome. Nat. Commun. 3, 1245. doi: 10.1038/ncomms2266
Karlsson, F. H., Tremaroli, V., Nookaew, I., Bergstrom, G., Behre, C. J., Fagerberg, B., et al. (2013). Gut Metagenome in European Women With Normal, Impaired and Diabetic Glucose Control. Nature 498, 99–103. doi: 10.1038/nature12198
Kelly, W. J., Li, D., Lambie, S. C., Cox, F., Attwood, G. T., Altermann, E., et al. (2016). Draft Genome Sequence of the Rumen Methanogen Methanobrevibacter Olleyae YLM1. Genome Announc 4 (2), e00232–16. doi: 10.1128/genomeA.00232-16
Kroning, I. S., Haubert, L., Kleinubing, N. R., Jaskulski, I. B., Scheik, L. K., Ramires, T., et al. (2020). New Spa Types, Resistance to Sanitisers and Presence of Efflux Pump Genes in Staphylococcus Aureus From Milk. Int. Dairy J. 109, 104712. doi: 10.1016/j.idairyj.2020.104712
Langenwalder, D. B., Silaghi, C., Nieder, M., Pfeffer, M., von Loewenich, F. D. (2020). Co-Infection, Reinfection and Superinfection With Anaplasma Phagocytophilum Strains in a Cattle Herd Based on ankA Gene and Multilocus Sequence Typing. Parasit. Vectors 13, 157. doi: 10.1186/s13071-020-04032-2
Lavelle, A., Sokol, H. (2020). Gut Microbiota-Derived Metabolites as Key Actors in Inflammatory Bowel Disease. Nat. Rev. Gastroenterol. Hepatol. 17, 223–237. doi: 10.1038/s41575-019-0258-z
Ley, R. E., Backhed, F., Turnbaugh, P., Lozupone, C. A., Knight, R. D., Gordon, J. I. (2005). Obesity Alters Gut Microbial Ecology. Proc. Natl. Acad. Sci. U. S. A. 102, 11070–11075. doi: 10.1073/pnas.0504978102
Li, H., Durbin, R. (2009). Fast and Accurate Short Read Alignment With Burrows-Wheeler Transform. Bioinformatics 25, 1754–1760. doi: 10.1093/bioinformatics/btp324
Li, K., Gao, J., Shahzad, M., Han, Z., Nabi, F., Liu, M., et al. (2014). Seroprevalence of Toxoplasma Gondii Infection in Yaks (Bos Grunniens) on the Qinghai-Tibetan Plateau of China. Vet. Parasitol. 205, 354–356. doi: 10.1016/j.vetpar.2014.07.014
Li, J.-K., Li, K., Han, Z.-Q., Zhang, H., Wang, X.-Q., Luo, H.-Q., et al. (2018). Serological Survey of Bovine Viral Diarrhoea Virus Among Yaks (Bos Poephagus Grunniens) in Hongyuan of Sichuan, China. Pakistan J. Zool 51.1. doi: 10.17582/journal.pjz/2018.50.4.sc7
Li, S., Liu, J., Liu, A., Li, Y., Wang, S., Wang, S., et al. (2017). Molecular Investigation of Piroplasma Infection in White Yaks (Bos Grunniens) in Gansu Province, China. Acta Trop. 171, 220–225. doi: 10.1016/j.actatropica.2017.04.009
Li, K., Li, Z., Zeng, Z., Li, A., Mehmood, K., Shahzad, M., et al. (2020). Prevalence and Molecular Characterization of Cryptosporidium Spp. In Yaks (Bos Grunniens) in Naqu, China. Microb. Pathog. 144, 104190. doi: 10.1016/j.micpath.2020.104190
Li, K., Mehmood, K., Zhang, H., Jiang, X., Shahzad, M., Dong, X., et al. (2018). Characterization of Fungus Microbial Diversity in Healthy and Diarrheal Yaks in Gannan Region of Tibet Autonomous Prefecture. Acta Trop. 182, 14–26. doi: 10.1016/j.actatropica.2018.02.017
Li, K., Shahzad, M., Zhang, H., Jiang, X., Mehmood, K., Zhao, X., et al. (2018). Socio-Economic Burden of Parasitic Infections in Yaks From 1984 to 2017 on Qinghai Tibetan Plateau of China-A Review. Acta Trop. 183, 103–109. doi: 10.1016/j.actatropica.2018.04.011
Liu, B., Wang, W., Zhu, X., Sun, X., Xiao, J., Li, D., et al. (2018). Response of Gut Microbiota to Dietary Fiber and Metabolic Interaction With SCFA in Piglets. Front. Microbiol. 9, 2344. doi: 10.3389/fmicb.2018.02344
Li, K., Wang, X. Q., Shahzad, M., Zhang, H., Zhao, X. D., Jiang, X., et al. (2018). Antibiotic Resistance and Screening of the Resistant Genes of Escherichia Coli (E-Coli) Isolated From Diarrheal Yak Calves in Sichuan Province, China. Trop. Biomed 35, 478–486. doi: 10.3390/ani11061712
Li, K., Zhang, L., Zhang, H., Lei, Z., Luo, H., Mehmood, K., et al. (2017). Epidemiological Investigation and Risk Factors of Echinococcus Granulosus in Yaks (Bos Grunniens), Tibetan Pigs and Tibetans on Qinghai Tibetan Plateau. Acta Trop. 173, 147–152. doi: 10.1016/j.actatropica.2017.06.019
Long, C. X., Shao, H. Q., Luo, C. Y., Yu, R., Tan, Z. J. (2020). Bacterial Diversity in the Intestinal Mucosa of Dysbiosis Diarrhea Mice Treated With Qiweibaizhu Powder. Gastroenterol. Res. Pract. 2020, 9420129. doi: 10.1155/2020/9420129
Louis, P., Young, P., Holtrop, G., Flint, H. (2010). Diversity of Human Colonic Butyrate-Producing Bacteria Revealed by Analysis of the Butyryl-CoA:acetate CoA-Transferase Gene. Environ. Microbiol. 12, 304–314. doi: 10.1111/j.1462-2920.2009.02066.x
Luan, Z., Sun, G., Huang, Y., Yang, Y., Yang, R., Li, C., et al. (2020). Metagenomics Study Reveals Changes in Gut Microbiota in Centenarians: A Cohort Study of Hainan Centenarians. Front. Microbiol. 11, 1474. doi: 10.3389/fmicb.2020.01474
Mangiola, F., Ianiro, G., Franceschi, F., Fagiuoli, S., Gasbarrini, G., Gasbarrini, A. (2016). Gut Microbiota in Autism and Mood Disorders. World J. Gastroenterol. 22, 361–368. doi: 10.3748/wjg.v22.i1.361
Martin, M. (2011). Cutadapt Removes Adapter Sequences From High-Throughput Sequencing Reads. EMBnetjournal 17, 3. doi: 10.14806/ej.17.1.200
Mazzawi, T., Hausken, T., Hov, J. R., Valeur, J., Sangnes, D. A., El-Salhy, M., et al. (2019). Clinical Response to Fecal Microbiota Transplantation in Patients With Diarrhea-Predominant Irritable Bowel Syndrome is Associated With Normalization of Fecal Microbiota Composition and Short-Chain Fatty Acid Levels. Scand J. Gastroenterol. 54, 690–699. doi: 10.1080/00365521.2019.1624815
Mikami, D., Kobayashi, M., Uwada, J., Yazawa, T., Kamiyama, K., Nishimori, K., et al. (2020). Short-Chain Fatty Acid Mitigates Adenine-Induced Chronic Kidney Disease via FFA2 and FFA3 Pathways. Biochim. Biophys. Acta Mol. Cell Biol. Lipids 1865, 158666. doi: 10.1016/j.bbalip.2020.158666
Morrison, D. J., Preston, T. (2016). Formation of Short Chain Fatty Acids by the Gut Microbiota and Their Impact on Human Metabolism. Gut Microbes 7, 189–200. doi: 10.1080/19490976.2015.1134082
Motoori, M., Tanaka, K., Sugimura, K., Miyata, H., Saito, T., Miyazaki, Y., et al. (2020). Impact of Preoperative Fecal Short Chain Fatty Acids on Postoperative Infectious Complications in Esophageal Cancer Patients. BMC Gastroenterol. 20, 7. doi: 10.1186/s12876-020-01217-y
Mukhuba, M., Roopnarain, A., Moeletsi, M. E., Adeleke, R. (2019). Metagenomic Insights Into the Microbial Community and Biogas Production Pattern During Anaerobic Digestion of Cow Dung and Mixed Food Waste. J. Chem. Technol. Biotechnol. 95, 151–162. doi: 10.1002/jctb.6217
Nguyen, T. T., Dang-Trinh, M. A., Higuchi, L., Mosqueda, J., Hakimi, H., Asada, M., et al. (2019). Initiated Babesia Ovata Sexual Stages Under In Vitro Conditions Were Recognized by Anti-CCp2 Antibodies, Showing Changes in the DNA Content by Imaging Flow Cytometry. Pathogens 8 (3), 104. doi: 10.3390/pathogens8030104
Parada Venegas, D., de la Fuente, M. K., Landskron, G., Gonzalez, M. J., Quera, R., Dijkstra, G., et al. (2019). Short Chain Fatty Acids (SCFA)-Mediated Gut Epithelial and Immune Regulation and Its Relevance for Inflammatory Bowel Diseases. Front. Immunol. 10, 277. doi: 10.3389/fimmu.2019.00277
Paul, C., Filippidou, S., Jamil, I., Kooli, W., House, G. L., Estoppey, A., et al. (2019). “Chapter Three - Bacterial Spores, From Ecology to Biotechnology,” in Advances in Applied Microbiology, vol. 106. Eds. Gadd, G. M., Sariaslani, S. (Academic Press), 79–111. doi: 10.1016/bs.aambs.2018.10.002
Peng, Y., Leung, H. C., Yiu, S. M., Chin, F. Y. (2012). IDBA-UD: A De Novo Assembler for Single-Cell and Metagenomic Sequencing Data With Highly Uneven Depth. Bioinformatics 28, 1420–1428. doi: 10.1093/bioinformatics/bts174
Pichler, M. J., Yamada, C., Shuoker, B., Alvarez-Silva, C., Gotoh, A., Leth, M. L., et al. (2020). Butyrate Producing Colonic Clostridiales Metabolise Human Milk Oligosaccharides and Cross Feed on Mucin via Conserved Pathways. Nat. Commun. 11, 3285. doi: 10.1038/s41467-020-17075-x
Powell, S., Forslund, K., Szklarczyk, D., Trachana, K., Roth, A., Huerta-Cepas, J., et al. (2014). eggNOG V4.0: Nested Orthology Inference Across 3686 Organisms. Nucleic Acids Res. 42, D231–D239. doi: 10.1093/nar/gkt1253
Qi, M., Cai, J., Wang, R., Li, J., Jian, F., Huang, J., et al. (2015). Molecular Characterization of Cryptosporidium Spp. And Giardia Duodenalis From Yaks in the Central Western Region of China. BMC Microbiol. 15, 108. doi: 10.1186/s12866-015-0446-0
Qin, J., Li, Y., Cai, Z., Li, S., Zhu, J., Zhang, F., et al. (2012). A Metagenome-Wide Association Study of Gut Microbiota in Type 2 Diabetes. Nature 490, 55–60. doi: 10.1038/nature11450
Qi, X., Yun, C., Sun, L., Xia, J., Wu, Q., Wang, Y., et al. (2019). Gut Microbiota–Bile Acid–Interleukin-22 Axis Orchestrates Polycystic Ovary Syndrome. Nat. Med. 25, 1225–1233. doi: 10.1038/s41591-019-0509-0
Quan, J., Wu, Z., Ye, Y., Peng, L., Wu, J., Ruan, D., et al. (2020). Metagenomic Characterization of Intestinal Regions in Pigs With Contrasting Feed Efficiency. Front. Microbiol. 11, 32. doi: 10.3389/fmicb.2020.00032
Ramette, A. (2007). Multivariate Analyses in Microbial Ecology. FEMS Microbiol. Ecol. 62, 142–160. doi: 10.1111/j.1574-6941.2007.00375.x
Rechkemmer, G., Vonengelhardt, W. (1988). Concentration-Dependence and PH-Dependence of Short-Chain Fatty-Acid Absorrption in the Proximal and Distal Colon of Guinea-Pig (CAVIA-PORCELLUS). Comp. Biochem. Physiol. a-Physiol 91, 659–663. doi: 10.1016/0300-9629(88)90944-9
Rinninella, E., Raoul, P., Cintoni, M., Franceschi, F., Miggiano, G. A. D., Gasbarrini, A., et al. (2019). What is the Healthy Gut Microbiota Composition? A Changing Ecosystem Across Age, Environment, Diet, and Diseases. Microorganisms 7 (1), 14. doi: 10.3390/microorganisms7010014
Schoster, A., Staempfli, H. R., Guardabassi, L. G., Jalali, M., Weese, J. S. (2017). Comparison of the Fecal Bacterial Microbiota of Healthy and Diarrheic Foals at Two and Four Weeks of Life. BMC Vet. Res. 13, 144. doi: 10.1186/s12917-017-1064-x
Segata, N., Izard, J., Waldron, L., Gevers, D., Miropolsky, L., Garrett, W. S., et al. (2011). Metagenomic Biomarker Discovery and Explanation. Genome Biol. 12, R60. doi: 10.1186/gb-2011-12-6-r60
Sieber, C. M. K., Probst, A. J., Sharrar, A., Thomas, B. C., Hess, M., Tringe, S. G., et al. (2018). Recovery of Genomes From Metagenomes via a Dereplication, Aggregation and Scoring Strategy. Nat. Microbiol. 3, 836–843. doi: 10.1038/s41564-018-0171-1
Tang, H., Zhu, J., Wu, S., Niu, H. (2020). Identification and Characterization of an Actin Filament-Associated Anaplasma Phagocytophilum Protein. Microb. Pathog. 147, 104439. doi: 10.1016/j.micpath.2020.104439
Thompson, J., Nguyen, N. Y., Robrish, S. A. (1992). Sucrose Fermentation by Fusobacterium Mortiferum ATCC 25557: Transport, Catabolism, and Products. J. Bacteriol 17 (10), 3227–3235. doi: 10.1128/jb.174.10.3227-3235.1992
Tian, N., Guo, X., Wang, M., Chen, C., Cui, H., Zhang, L., et al. (2020). Bacterial Community Diversity of Shilixiang Baijiu Daqu Based on Metagenomics. J. Food Biochem. 44 (10), e13410. doi: 10.1111/jfbc.13410:e13410
Togo, A. H., Durand, G., Khelaifia, S., Armstrong, N., Robert, C., Cadoret, F., et al. (2017). Fournierella Massiliensis Gen. Nov., Sp Nov., a New Human-Associated Member of the Family Ruminococcaceae. Int. J. Syst Evol Microbiol. 67, 1393–1399. doi: 10.1099/ijsem.0.001826
Vieira, A. T., Macia, L., Galvao, I., Martins, F. S., Canesso, M. C., Amaral, F. A., et al. (2015). A Role for Gut Microbiota and the Metabolite-Sensing Receptor GPR43 in a Murine Model of Gout. Arthritis Rheumatol. 67, 1646–1656. doi: 10.1002/art.39107
Wang, Z., Klipfell, E., Bennett, B. J., Koeth, R., Levison, B. S., Dugar, B., et al. (2011). Gut Flora Metabolism of Phosphatidylcholine Promotes Cardiovascular Disease. Nature 472, 57–63. doi: 10.1038/nature09922
Wang, T. Y., Zhang, X. Q., Chen, A. L., Zhang, J., Lv, B. H., Ma, M. H., et al. (2020). A Comparative Study of Microbial Community and Functions of Type 2 Diabetes Mellitus Patients With Obesity and Healthy People. Appl. Microbiol. Biotechnol. 104, 7143–7153. doi: 10.1007/s00253-020-10689-7
Wang, J. J., Zhang, Q. M., Ni, W. W., Zhang, X., Li, Y., Li, A. L., et al. (2019). Modulatory Effect of Lactobacillus Acidophilus KLDS 1.0738 on Intestinal Short-Chain Fatty Acids Metabolism and GPR41/43 Expression in Beta-Lactoglobulin-Sensitized Mice. Microbiol. Immunol. 63, 303–315. doi: 10.1080/09168451.2018.1495551
Wang, Z., Zhu, J., Li, W., Li, R., Wang, X., Qiao, H., et al. (2020). Antibacterial Mechanism of the Polysaccharide Produced by Chaetomium Globosum CGMCC 6882 Against Staphylococcus Aureus. Int. J. Biol. Macromol. 159, 231–235. doi: 10.1016/j.ijbiomac.2020.04.269
Wei, H., Li, X., Tang, L., Yao, H., Ren, Z., Wang, C., et al. (2020). 16s rRNA Gene Sequencing Reveals the Relationship Between Gut Microbiota and Ovarian Development in the Swimming Crab Portunus Trituberculatus. Chemosphere 254, 126891. doi: 10.1016/j.chemosphere.2020.126891
Xu, G., Xing, W., Li, T., Xue, M., Ma, Z., Jiang, N., et al. (2019). Comparative Study on the Effects of Different Feeding Habits and Diets on Intestinal Microbiota in Acipenser Baeri Brandt and Huso Huso. BMC Microbiol. 19, 297. doi: 10.1186/s12866-019-1673-6
Yan, N., Li, R., Wang, Y., Zhang, B., Yue, H., Tang, C. (2020). High Prevalence and Genomic Characteristics of G6P 1 Bovine Rotavirus A in Yak in China. J. Gen. Virol. 101, 701–711. doi: 10.1099/jgv.0.001426
Yu, S., Balasubramanian, I., Laubitz, D., Tong, K., Bandyopadhyay, S., Lin, X., et al. (2020). Paneth Cell-Derived Lysozyme Defines the Composition of Mucolytic Microbiota and the Inflammatory Tone of the Intestine. Immunity 53, 398–416 e8. doi: 10.1016/j.immuni.2020.07.010
Zeng, X., Gao, X., Peng, Y., Wu, Q., Zhu, J., Tan, C., et al. (2019). Higher Risk of Stroke Is Correlated With Increased Opportunistic Pathogen Load and Reduced Levels of Butyrate-Producing Bacteria in the Gut. Front. Cell Infect. Microbiol. 9, 4. doi: 10.3389/fcimb.2019.00004
Zhou, C., Zhao, H., Xiao, X. Y., Chen, B. D., Guo, R. J., Wang, Q., et al. (2020). Metagenomic Profiling of the Pro-Inflammatory Gut Microbiota in Ankylosing Spondylitis. J. Autoimmun 107, 102360. doi: 10.1016/j.jaut.2019.102360
Keywords: yak, diarrhea, gut microbiota, metagenomics, SCFA
Citation: Li K, Zeng Z, Liu J, Pei L, Wang Y, Li A, Kulyar MF-e-A, Shahzad M, Mehmood K, Li J and Qi D (2022) Effects of Short-Chain Fatty Acid Modulation on Potentially Diarrhea-Causing Pathogens in Yaks Through Metagenomic Sequencing. Front. Cell. Infect. Microbiol. 12:805481. doi: 10.3389/fcimb.2022.805481
Received: 30 October 2021; Accepted: 25 February 2022;
Published: 23 March 2022.
Edited by:
Mingqun Lin, The Ohio State University, United StatesReviewed by:
Almagul Kushugulova, Nazarbayev University, KazakhstanSelvasankar Murugesan, Sidra Medicine, Qatar
Copyright © 2022 Li, Zeng, Liu, Pei, Wang, Li, Kulyar, Shahzad, Mehmood, Li and Qi. This is an open-access article distributed under the terms of the Creative Commons Attribution License (CC BY). The use, distribution or reproduction in other forums is permitted, provided the original author(s) and the copyright owner(s) are credited and that the original publication in this journal is cited, in accordance with accepted academic practice. No use, distribution or reproduction is permitted which does not comply with these terms.
*Correspondence: Kun Li, lik2014@sina.com; Jiakui Li, lijk210@sina.com; Desheng Qi, qds@mail.hzau.edu.cn