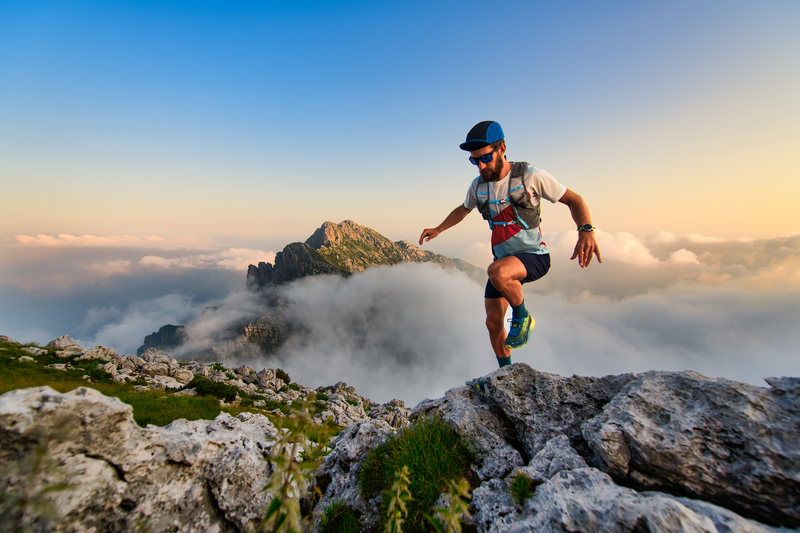
95% of researchers rate our articles as excellent or good
Learn more about the work of our research integrity team to safeguard the quality of each article we publish.
Find out more
ORIGINAL RESEARCH article
Front. Cell. Infect. Microbiol. , 05 December 2022
Sec. Intestinal Microbiome
Volume 12 - 2022 | https://doi.org/10.3389/fcimb.2022.1048519
Evidence supports associations between gut microbiota and cardiovascular protein levels in plasma. However, it is unclear whether these associations reflect a causal relationship. To reveal the causal relationship between gut microbiota and cardiovascular protein levels in plasma, we estimated their causal effects using two-sample Mendelian randomization (MR) analysis. Sensitivity analysis was also performed to assess the robustness of our results. Genome-wide association study (GWAS) of microbiomes in the MiBioGen study included 211 bacterial taxa (18,473 individuals), and GWAS of 90 cardiovascular proteins included 30,931 individuals. There were 196 bacterial taxa from five levels available for analysis. The following 14 causal relationships were identified: phylum Euryarchaeota and carbohydrate antigen 125 (β = 0.289), order Bacillales and CSF-1 (β = -0.211), genus Dorea and HSP-27 (β = 0.465), phylum Actinobacteria and IL-8 (β = 0.274), order Enterobacteriales and KIM-1 (β = -0.499), class Actinobacteria, genus Bifidobacterium, phylum Actinobacteria and LEP (β = -0.219, β = -0.201, and β = -0.221), genus Methanobrevibacter and NT-proBNP (β = 0.371), family Peptostreptococcaceae and SRC (β = 0.191), order Verrucomicrobiales, phylum Verrucomicrobia and TNF-R2 (β = 0.251 and β = 0.233), family Veillonellaceae and t-PA (β = 0.271), and class Erysipelotrichia and VEGF-D (β = 0.390). Sensitivity analysis showed no evidence of pleiotropy or heterogeneity. The results of the reverse MR analysis showed no reverse causality for any of the 13 gut microbes and 11 cardiovascular proteins. Mendelian randomization estimates provide strong evidence for a causal effect of gut microbiota-mediated alterations on cardiovascular protein expression.
Gut microbiota is a dynamic and complex ecological community of microorganisms that inhabit the human gut (O’Hara and Shanahan, 2006). Twin-based heritability estimates and macrogenome-wide association studies have challenged the conventional view of the gut microbiota as a purely environmental factor (Blekhman et al., 2015; Turpin et al., 2016; Liu et al., 2021). Gut microbiota affects immunity, neurology, psychiatric traits, metabolism, and even circulation of the body (Gilbert et al., 2018). Many disease states have been associated with changes in faecal microbiota, including cardiovascular disease (Brown and Hazen, 2015). Indeed, specific gut microbiota-dependent metabolites affect host metabolism and cardiovascular disease. For example, phenylacetyl glutamine promotes adverse cardiovascular phenotypes in the host by interacting with multiple adrenergic receptors (Nemet et al., 2020). Additionally, it has been suggested that gut microbiota directly influence plasma levels of proinflammatory factors including some cardiovascular proteins. For example, Lee et al. found that enterotoxigenic Bacteroides fragilis-secreted toxin induces IL-8 secretion from intestinal epithelial cells via the E-Cadherin/β-Catenin/NF-κB pathway (Lee et al., 2022). Engevik et al. found that F. nucleatum produces outer membrane vesicles that activate Toll-like receptor 4 to drive extracellular signal-regulated kinase, NF-κB, and proinflammatory cytokine expression (Engevik et al., 2021). Pallikkuth et al. studied rhesus monkeys as non-human primates, and found that highly abundant archaea and amoebae in aged animals had a direct correlation with plasma biomarkers of inflammation and immune activation, such as interleukin (IL)-6, IL-8, and TNF (Pallikkuth et al., 2021). However, most studies have examined only a few gut microbes and cardiovascular proteins. No comprehensive or systematic randomized controlled trials have been conducted on the causal relationship between gut microbes and cardiovascular protein expression in humans.
Typically, the gold standard to infer causality is a randomized controlled trial (RCT). Considering the difficulty in implementing RCTs, Mendelian randomization (MR) takes full advantage of this method. It is an epidemiological method that strengthens chance inferences by genetic variation as an instrumental variable for exposure (Burgess and Thompson, 2015). Because parental alleles are randomly assigned to offspring during meiosis in accordance with Mendel’s second law, the method minimizes the interference of important confounding factors such as the natural environment and socioeconomic level. The use of genetic variation as a valid instrumental variable in MR analysis relies on three core assumptions. First, genetic variation must be strongly correlated to exposure factors (association assumption). Second, genetic variation cannot be directly correlated to outcomes (exclusion restriction assumption). Third, genetic variation cannot be correlated to any possible confounding factors (independence assumption) (Emdin et al., 2017). To date, genetic studies have shown that host genetic variation affects the composition of the gut microbiota (Sanna et al., 2019). Therefore, in the present study, we performed two-sample MR analysis to fully explore whether gut microbiota abundance has a causal effect on cardiovascular protein levels and to identify specific pathogenic bacterial taxa.
Sample summary statistics employed GWAS meta-analysis of the gut microbiome in the MiBioGen study, the largest cohort, multi-ethnic, genome-wide gut microbiome meta-analysis to date. The meta-analysis included 18,340 individuals from 22 cohorts consisting of adults or adolescents and two cohorts consisting of children. There were four multiple ancestry samples. Single ancestry samples included 16 European cohorts, one Middle Eastern cohort, one East Asian cohort, one US Hispanic/Latin cohort, and one African American cohort. The microbial composition was analysed by targeting three different variable regions of the 16S rRNA gene: V1–V2 (n=3,716), V3–V4 (n=4,211), and V4 (n=10,413). A total of 211 taxa were included in this study (131 genuses, 35 families, 20 orders, 16 phyla, and nine classes) (Kurilshikov et al., 2021).
Cardiovascular protein phenotypes were obtained from a recent genomic analysis of 90 cardiovascular proteins in 30,931 individuals. The study included 15 cohorts (13 discovery datasets and two replication datasets). The analysis identified 451 protein quantitative trait loci associated with the plasma levels of 85 proteins (P< 5×10-8) (Folkersen et al., 2020).
Bacterial taxa were analysed at five levels (phylum, class, order, family, and genus). A unique taxonomic unit was defined as a feature. Considering that a gene cis region only constitutes a small proportion of the genome, we relaxed the conventional genome-wide significance P-value threshold for instrument selection to P<1×10-5 (Sanna et al., 2019). We implemented a series of additional quality control steps to select eligible instrumental variables (IVs). First, SNPs with allelic inconsistencies between the exposure and result samples (i.e., A/G vs. A/C) were excluded. Second, palindromic A/T or G/C alleles were excluded to avoid distortions in strand orientation or allelic coding. Third, SNPs within each bacterial taxonomic unit were clustered together and only independent SNPs were retained. The linkage disequilibrium (LD) threshold for clustering was set to r2< 0.01 and the size of the clustering window was set to 500 kb. A total of 1,000 Genome Project sequencing data (phase 3) were used to estimate LD. Fourth, horizontal pleiotropy effects, i.e., confounding effects caused by other diseases, may violate the second hypothesis in the MR analysis (SNPs are not associated with outcomes). Once horizontal pleiotropic effects were found, this instrumental variable was deleted. The MR-Egger regression test and MR-PRESSO test were applied to detect potential horizontal pleiotropy and to eliminate the effect of pleiotropy by removing outliers (Verbanck et al., 2018). Additionally, genomic coordinates for all correlation analyses were based on Ensembl GRCh37 reference during the coordination process (Yates et al., 2020), which removed ambiguous and duplicate SNPs. For markers without SNP information, SNP conversion was performed using the ieugwasr package for chr:pos (radius set to 0).
We performed MR analyses to investigate the causal relationships between gut microbiota and 90 cardiovascular proteins. The causal relationship between each pair of bacterial taxa and plasma metrics was examined using four MR methods: the inverse variance weighted (IVW) test (Burgess et al., 2013), weighted median estimator (WME) (Bowden et al., 2016), MR-Egger regression (Bowden et al., 2015), and MR-PRESSO (Verbanck et al., 2018). Each of the four statistical methods has its own model assumptions that are explained in detail in each study (Burgess et al., 2013; Bowden et al., 2015; Bowden et al., 2016; Verbanck et al., 2018). The IVW method is slightly stronger than the other methods under certain conditions (Bowden et al., 2016). Therefore, we performed MR estimation using IVW as the main method. To account for multiple testing, we implemented a Bonferroni-corrected allowed type I error rate (α) of 0.05/n (the effective number of independent bacterial taxa at the taxonomic level): phylum P = 5.56 × 10-3 (0.05/9), class P = 3.33 × 10-3 (0.05/15), order P=2.50×10-3(0.05/20), family P=1.56×10-3 (0.0 5/32), and genus P=4.20×10-4 (0.05/119). To assess the robustness of significant results, we performed several sensitivity analyses. The Cochrane Q test for IVW and MR-Egger regression was used to test for potential heterogeneity. Moreover, leave-one-out analyses were performed to determine whether the causal signal was driven by a single SNP. Additionally, to assess the strength of the selected SNPs, the F-statistic was calculated for each bacterial taxonomic unit using the following equation:
where R2 is the fraction of exposed variance explained by IVs, k is the number of IVs, and n is the sample size. F-statistic ≥ 10 indicates no strong evidence of weak instrument bias. IVs with an F-statistic of less than 10 were considered weak IVs and excluded (Pierce et al., 2011). Because the minor allele frequency was not provided for SNPs in gut microbes, we estimated the R2 values directly using the get_r_from_pn function of the TwoSampleMR package. Additionally, we performed reverse MR analysis to explore the reverse causality of cardiovascular proteins. MR analysis was performed in R (version 4.1.3) (http://www.r-project.org ) using the TwoSampleMR package (Hemani et al., 2018).
In the microbiome samples, we excluded three unknown families and 12 unknown genuses. Additionally, we found duplicate data for class Verrucomicrobiae and order Verrucomicrobiales, but fortunately, this did not affect the results. We therefore chose to retain the order Verrucomicrobiales with lower species relationships. Thus, nine phyla, 15 classes, 20 orders, 32 families, and 131 genuses bacterial taxa were included in the subsequent MR analysis. MR analyses by the IVW test are summarized in Supplementary Tables 1–3. We found 14 causal relationships between 13 bacterial characteristics and 11 cardiovascular protein indicators, including phylum Euryarchaeota and carbohydrate antigen 125 (CA-125) (P = 1.26 × 10-3), order Bacillales and colony-stimulating factor (CSF-1) (P = 5.07 × 10-4), genus Dorea and heat shock protein 27 (HSP-27) (P = 6.28 × 10-5), phylum Actinobacteria and interleukin-8 (IL-8) (P = 2.30 × 10-3), order Enterobacteriales and kidney injury molecule-1 (KIM-1) (P = 2.04 × 10-3), class Actinobacteria, genus Bifidobacterium, and the phylum Actinobacteria and leptin (LEP) (P = 1.79 × 10-3, P = 4.14 × 10-4, and P = 3.49 × 10-3, respectively), genus Methanobrevibacter and N-terminal pro-brain natriuretic peptide (NT-proBNP) (P = 3.75 × 10-4), family Peptostreptococcaceae and proto-oncogene tyrosine-protein kinase Src (SRC) (P = 5.49 × 10-4), order Verrucomicrobiales and phylum Verrucomicrobia, and tumor necrosis factor receptor 2 (TNF-R2) (P = 1.88×10-3 and P = 3.98×10-3, respectively), family Veillonellaceae and tissue plasminogen activator (t-PA) (P = 9.84 × 10-5), and class Erysipelotrichia and vascular endothelial growth factor D (VEGF-D) (P = 3.07 × 10-3). Among them, nine bacterial traits were positively correlated to IL-8, CA-125, TNF-R2, VEGF-D, SRC, t-PA, HSP-27, and NT-proBNP with regression coefficients ranging from 0.19 to 0.47. Five bacterial traits were negatively and causally correlated to LEP, CSF-1, and KIM-1 with regression coefficients ranging from -0.50 to -0.20. Notably, the causal effects of phylum and class Actinobacteria on LEP, and phylum and order Verrucomicrobia on TNF-R2 were consistent in direction (Figure 1). Scatter plots of the 14 causal IVW, WME, and MR-Egger are shown in Figure 2. The F-statistics for all IVs were greater than 10, indicating no evidence of weak instrument bias (Supplementary Table 4). The MR-Presso test and MR-Egger regression test did not show a horizontal multidirectional effect (p>0.05), whereas the statistics for the IVW test and MR-Egger regression showed no evidence of heterogeneity among the identified outcomes (P>0.05) (Table 1). Additionally, the leave-one-out sensitivity analysis showed that no single SNP significantly affected the causal association (Supplementary Figure 1). The reverse MR analysis showed no common SNPs for cardiovascular proteins (P< 1 × 10-5) and the identified bacterial features. Therefore, we did not find the presence of reverse causality.
Figure 1 Forest plot of causal relationships estimated for 13 microbiota and 11 cardiovascular proteins using four MR methods. IVW, inverse variance weighted; WME, weighted median estimator; MR-Egger, MR-Egger regression; MR-PRESSO, MR-PRESSO test; MR, Mendelian randomization.
Figure 2 Mendelian randomization estimation using multiple SNPs. Light blue, green, and dark blue lines represent the association between microbiota (exposure) and cardiovascular proteins (outcome) estimated by the IVW method, weighted median estimator, and MR-Egger regression, respectively. Vertical and horizontal black lines around each point show the 95% confidence interval for each polymorphism exposure association and polymorphism outcome association, respectively. SNP, single nucleotide polymorphism.
In this study, we performed MR analyses to assess the potential causal relationship between gut microbiota and 90 cardiovascular proteins. Using multi-cohort GWAS statistics of the gut microbiome and cardiovascular proteins, we identified 13 bacterial signatures that were causally associated with 11 cardiovascular proteins. Phylum Euryarchaeota has a high incidence and diversity in two body sites, the gut and oral cavity, as shown by Cai et al. Phylum Euryarchaeota, as an archaea may produce cancer-related metabolites that contribute to the human tumour microenvironment and carcinogenesis. This is consistent with our findings of a positive correlation between phylum Euryarchaeota and CA-125 (Cai et al., 2022). CSF-1 stimulates macrophage colonization and granulocyte function, and lowers blood cholesterol (Hume and MacDonald, 2012). He et al. found that order Bacillales lowers cholesterol (He et al., 2021). Unfortunately, this contradicts our results. Heat shock protein 27 (Hsp27), also known as heat shock protein beta-1 (HSPB1), is encoded by the HSPB1 gene. It has a molecular chaperone activity, contributes to heat resistance, inhibits apoptosis, and regulates cell development and differentiation (Van Montfort et al., 2001). Qiu et al. found that, after concurrent chemoradiotherapy of patients with non-small cell lung cancer, patients in the long-term progression-free survival group had increased faecal genus Dorea abundance (Qiu et al., 2022). Interestingly, we also found a positive causal relationship between genus Dorea and HSP-27 (β = 0.465). In a randomized controlled trial on the diet of patients with irritable bowel syndrome, serum proinflammatory IL-6 and IL-8 levels, and faecal abundance of actinomycetes, bifidobacteria, and E. faecalis were significantly lower in patients on the low-FODMAP diet (Hustoft et al., 2017). Coincidentally, Feng et al. reduced bacterial load with shen-ling-bai-zhu-san, which reduced bacterial abundance including Actinobacteria and inhibited interleukin (IL)-1β, IL-6, tumour necrosis factor-α, and IL-8 (Feng et al., 2020). In accordance with our results, a decrease in IL-8 may be partly caused by reduced abundance of phylum Actinobacteria. Many studies have reported that increased order Enterobacteriales may allow endotoxins to continuously enter circulation through an impaired intestinal barrier function, inducing a systemic inflammatory response to worsen the condition of patients with chronic kidney disease (Wu et al., 2021; Liu et al., 2022). Similarly, our study suggested that order Enterobacteriales was negatively correlated to plasma KIM-1 levels. Significantly elevated plasma levels of KIM-1 usually indicate early kidney injury. During this period, KIM-1 may be involved in early injury and repair of renal tubular epithelial cells and renal interstitial fibrosis through adhesion, clearance of apoptotic cells, and the immune response (Ichimura et al., 2012). Additionally, we found that phylum and class Actinobacteria was negatively correlated to LEP, a hormone produced mainly by adipocytes and intestinal epithelial cells in the small intestines, which regulates the energy balance by suppressing appetite and reducing fat storage in adipocytes (Machleidt and Lehnert, 2011). This is consistent with the findings of Yin et al. and Marques et al. who found that lysine restriction increases appetite by increasing the abundance of Actinobacteria, Saccharibacteria, and Synergistetes (Yin et al., 2017). Similarly, Marques et al. found lower levels of Actinobacteria spp. and Prevotella, but higher levels of Bifidobacterium and Lactobacillus in the intestinal flora of a high-fat-diet-induced obese rat model (Marques et al., 2016). These possible intermediates are caused by a decrease of LEP in plasma through Actinobacteria and Bifidobacterium. NT-proBNP is a hormone with an inactive N-terminal of 76 amino acids, which is cleaved from the molecule to release brain natriuretic peptide (Bhalla et al., 2004). Infusion of BNP at pathophysiological concentrations into hypertensive patients results in a progressive decrease in the left ventricular end-diastolic volume and left ventricular end-systolic volume as well as a reduction in cardiac preload (Atisha et al., 2004). Lv et al. found significant reductions in five bacterial species in the gut microbiota profile of early-onset preeclampsia women, including genus Methanobrevibacter (Lv et al., 2019). Thus, we hypothesized that plasma NT-proBNP decreases with the decrease in faecal genus Methanobrevibacter, which contributes to elevated blood pressure in patients. Jia et al. found an increase in the abundance of four bacteria, Lactobacillus, Actinomyces, Peptostreptococcaceae, and Alloscardovia, in the intestinal microbiota of intrahepatic cholangiocarcinoma patients (Jia et al., 2020). Furthermore, Liu et al. found that autocrine osteopontin promotes hepatic progenitor cell expansion and migration by binding to αv integrins and activating SRC activity to reduce membrane E-calmodulin and increase free cytoplasmic β-linked proteins. Moreover, this pathway plays a key role in the oncogenic transformation of hepatic progenitor cells (Liu et al., 2015). In combination with these previous studies, our findings led us to the hypothesis that the role of family Peptostreptococcaceae in the progression of tumours, including hepatocellular carcinoma, may be mediated through activation of SRC protein kinase, causing activation of downstream pathways. Tumour necrosis factor receptor 2 (TNFR2), also known as tumour necrosis factor receptor superfamily member 1B (TNFRSF1B) and CD120b, is one of two membrane receptors that bind tumour necrosis factor-α (TNFα) (Schall et al., 1990). In a study of the effect of intermittent fasting (IF) on diabetic retinopathy, Beli et al. found that the intestinal tract of IF mice had a reduction in both Bacteroidetes and Verrucomicrobia bacteria and that IF decreased retinal TNF-α expression compared with mice fed ad libitum (Beli et al., 2018). Therefore, understanding whether phylum and order Verrucomicrobia are also involved in reductions of TNF-α and its membrane receptor TNF-R2 may provide another novel insight into the causal relationship between Verrucomicrobia and TNF-R2. Sickle cell disease (SCD) is an inherited blood disorder that causes intravascular haemolysis, and slow blood flow can cause microthrombosis resulting in organ damage. In 14 healthy controls and 14 SCD subjects, severe ecological dysregulation was observed in SCD patients with many flora being abundant, including the family Veillonellaceae (Brim et al., 2021). t-PA is a protein involved in thrombus breakdown. It is a serine protease that catalyses conversion of fibrinogen to fibrinolytic enzymes, the main enzyme responsible for clot breakdown (Wardlaw et al., 2012). In agreement with our results, an increase in family Veillonellaceae positively led to an increase in plasma t-PA. Pindjakova et al. found that, compared with mice fed a hypercholesterolemic and proatherogenic diet, low-fat diet (Paigen diet)-fed mice, Gammaproteobacteria, Delataproteobacteria, and Erysipelotrichia were more abundant in obese mice fed a dietary, high-fat obesogenic, but non-inflammatory diet (Pindjakova et al., 2017). Unfortunately, we did not find any correlation between class Erysipelotrichia and VEGF-D for the time reported. We screened 11 plasma cardiovascular proteins and found 14 causal relationships between gut microbiota and these proteins.
In fact, in addition to the effect of gut microbiota on cardiovascular disease, we found that gut microbiota influences markers for tumour progression, endocrine metabolism, and renal organism on the basis of previous studies. However, it is undeniable that our study has certain shortcomings. Due to the limitations of data from gut microbes studies, the bacterial taxa mentioned in this article were only analysed at the genus and above level and not at the level of more specific species or strains. Our sample sizes of gut microbiota and cardiovascular proteins were not very large compared with some MR analyses with different aims. Moreover, the number of SNPs representative of each microbiota was relatively small. Further large-scale RCT clinical trials and studies of biological mechanisms are needed.
Overall, we comprehensively assessed the potential causal relationship between gut microbiota and cardiovascular protein expression. Combined with other studies, we partially confirmed our conclusions. Therefore, this study may provide new insights into microbially mediated alterations in cardiovascular protein levels.
The datasets presented in this study can be found in online repositories. The names of the repository/repositories and accession number(s) can be found in the article/Supplementary Material.
Studies involving human participants were reviewed and approved, and all studies were approved by their respective institutional review boards. No new data were collected and no new institutional review boards’ approval was required.
All authors contributed to the work presented in this paper. Conceptualisation, WZ and ZW. Resources, visualisation, and analysis, SZ, FZ and JD. writing—original draft preparation, WZ and SZ. writing—editing, ZW. supervision, ZW. project administration, WZ and ZW. funding acquisition, FZ and ZW. All authors approved the submitted version.
This work was supported by the National Natural Science Foundation of China (No. 81601692 and 81901969), the Technology Research from the Department of Education of Liaoning Province (No. JCZR2020013), 345 Talent Project of Shengjing hospital of China Medical University.
We thank Mitchell Arico from Liwen Bianji (Edanz) (https://www.liwenbianji.cn) for editing the language of a draft of this manuscript.
The authors declare that the research was conducted in the absence of any commercial or financial relationships that could be construed as a potential conflict of interest.
All claims expressed in this article are solely those of the authors and do not necessarily represent those of their affiliated organizations, or those of the publisher, the editors and the reviewers. Any product that may be evaluated in this article, or claim that may be made by its manufacturer, is not guaranteed or endorsed by the publisher.
The Supplementary Material for this article can be found online at: https://www.frontiersin.org/articles/10.3389/fcimb.2022.1048519/full#supplementary-material
Atisha, D., Bhalla, M. A., Morrison, L. K., Felicio, L., Clopton, P., Gardetto, N., et al. (2004). A prospective study in search of an optimal b-natriuretic peptide level to screen patients for cardiac dysfunction. Am. Heart J. 148 (3), 518–523. doi: 10.1016/j.ahj.2004.03.014
Beli, E., Yan, Y., Moldovan, L., Vieira, C. P., Gao, R., Duan, Y., et al. (2018). Restructuring of the gut microbiome by intermittent fasting prevents retinopathy and prolongs survival in db/db mice. Diabetes 67 (9), 1867–1879. doi: 10.2337/db18-0158
Bhalla, V., Willis, S., Maisel, A. S. (2004). B-type natriuretic peptide: the level and the drug–partners in the diagnosis of congestive heart failure. Congest Heart Fail 10 (1 Suppl 1), 3–27. doi: 10.1111/j.1527-5299.2004.03310.x
Blekhman, R., Goodrich, J. K., Huang, K., Sun, Q., Bukowski, R., Bell, J. T., et al. (2015). Host genetic variation impacts microbiome composition across human body sites. Genome Biol. 16 (1), 191. doi: 10.1186/s13059-015-0759-1
Bowden, J., Davey Smith, G., Burgess, S. (2015). Mendelian randomization with invalid instruments: effect estimation and bias detection through egger regression. Int. J. Epidemiol. 44 (2), 512–525. doi: 10.1093/ije/dyv080
Bowden, J., Davey Smith, G., Haycock, P. C., Burgess, S. (2016). Consistent estimation in mendelian randomization with some invalid instruments using a weighted median estimator. Genet. Epidemiol. 40 (4), 304–314. doi: 10.1002/gepi.21965
Brim, H., Taylor, J., Abbas, M., Vilmenay, K., Daremipouran, M., Varma, S., et al. (2021). The gut microbiome in sickle cell disease: Characterization and potential implications. PloS One 16 (8), e0255956. doi: 10.1371/journal.pone.0255956
Brown, J. M., Hazen, S. L. (2015). The gut microbial endocrine organ: bacterially derived signals driving cardiometabolic diseases. Annu. Rev. Med. 66, 343–359. doi: 10.1146/annurev-med-060513-093205
Burgess, S., Butterworth, A., Thompson, S. G. (2013). mendelian randomization analysis with multiple genetic variants using summarized data. Genet. Epidemiol. 37 (7), 658–665. doi: 10.1002/gepi.21758
Burgess, S., Thompson, S. G. (2015). Mendelian randomization: methods for using genetic variants in causal estimation. (London, UK: Chapman and Hall/CRC).
Cai, M., Kandalai, S., Tang, X., Zheng, Q. (2022). Contributions of human-associated archaeal metabolites to tumor microenvironment and carcinogenesis. Microbiol. Spectr. 10 (2), e0236721. doi: 10.1128/spectrum.02367-21
Emdin, C. A., Khera, A. V., Kathiresan, S. (2017). Mendelian randomization. Jama 318 (19), 1925–1926. doi: 10.1001/jama.2017.17219
Engevik, M. A., Danhof, H. A., Ruan, W., Engevik, A. C., Chang-Graham, A. L., Engevik, K. A., et al. (2021). Fusobacterium nucleatum secretes outer membrane vesicles and promotes intestinal inflammation. mBio 12 (2), e02706–20. doi: 10.1128/mBio.02706-20
Feng, J., Dai, W., Zhang, C., Chen, H., Chen, Z., Chen, Y., et al. (2020). Shen-ling-bai-zhu-san ameliorates inflammation and lung injury by increasing the gut microbiota in the murine model of streptococcus pneumonia-induced pneumonia. BMC Complement Med. Ther. 20 (1), 159. doi: 10.1186/s12906-020-02958-9
Folkersen, L., Gustafsson, S., Wang, Q., Hansen, D. H., Hedman Å, K., Schork, A., et al. (2020). Genomic and drug target evaluation of 90 cardiovascular proteins in 30,931 individuals. Nat. Metab. 2 (10), 1135–1148. doi: 10.1038/s42255-020-00287-2
Gilbert, J. A., Blaser, M. J., Caporaso, J. G., Jansson, J. K., Lynch, S. V., Knight, R. (2018). Current understanding of the human microbiome. Nat. Med. 24 (4), 392–400. doi: 10.1038/nm.4517
He, Q., Li, J., Ma, Y., Chen, Q., Chen, G. (2021). Probiotic potential and cholesterol-lowering capabilities of bacterial strains isolated from pericarpium citri reticulatae ‘Chachiensis’. Microorganisms 9 (6), 1224. doi: 10.3390/microorganisms9061224
Hemani, G., Zheng, J., Elsworth, B., Wade, K. H., Haberland, V., Baird, D., et al. (2018). The MR-base platform supports systematic causal inference across the human phenome. Elife 30 (7), e34408. doi: 10.7554/eLife.34408
Hume, D. A., MacDonald, K. P. (2012). Therapeutic applications of macrophage colony-stimulating factor-1 (CSF-1) and antagonists of CSF-1 receptor (CSF-1R) signaling. Blood 119 (8), 1810–1820. doi: 10.1182/blood-2011-09-379214
Hustoft, T. N., Hausken, T., Ystad, S. O., Valeur, J., Brokstad, K., Hatlebakk, J. G., et al. (2017). Effects of varying dietary content of fermentable short-chain carbohydrates on symptoms, fecal microenvironment, and cytokine profiles in patients with irritable bowel syndrome. Neurogastroenterol Motil. 29 (4), e12969. doi: 10.1111/nmo.12969
Ichimura, T., Brooks, C. R., Bonventre, J. V. (2012). Kim-1/Tim-1 and immune cells: shifting sands. Kidney Int. 81 (9), 809–811. doi: 10.1038/ki.2012.11
Jia, X., Lu, S., Zeng, Z., Liu, Q., Dong, Z., Chen, Y., et al. (2020). Characterization of gut microbiota, bile acid metabolism, and cytokines in intrahepatic cholangiocarcinoma. Hepatology 71 (3), 893–906. doi: 10.1002/hep.30852
Kurilshikov, A., Medina-Gomez, C., Bacigalupe, R., Radjabzadeh, D., Wang, J., Demirkan, A., et al. (2021). Large-Scale association analyses identify host factors influencing human gut microbiome composition. Nat. Genet. 53 (2), 156–165. doi: 10.1038/s41588-020-00763-1
Lee, C. G., Hwang, S., Gwon, S. Y., Park, C., Jo, M., Hong, J. E., et al. (2022). Bacteroides fragilis toxin induces intestinal epithelial cell secretion of interleukin-8 by the e-Cadherin/β-Catenin/NF-κB dependent pathway. Biomedicines 10 (4), 827. doi: 10.3390/biomedicines10040827
Liu, Y., Cao, L., Chen, R., Zhou, X., Fan, X., Liang, Y., et al. (2015). Osteopontin promotes hepatic progenitor cell expansion and tumorigenicity via activation of β-catenin in mice. Stem Cells 33 (12), 3569–3580. doi: 10.1002/stem.2072
Liu, J., Gao, L. D., Fu, B., Yang, H. T., Zhang, L., Che, S. Q., et al. (2022). Efficacy and safety of zicuiyin decoction on diabetic kidney disease: A multicenter, randomized controlled trial. Phytomedicine 100, 154079. doi: 10.1016/j.phymed.2022.154079
Liu, X., Tang, S., Zhong, H., Tong, X., Jie, Z., Ding, Q., et al. (2021). A genome-wide association study for gut metagenome in Chinese adults illuminates complex diseases. Cell Discovery 7 (1), 9. doi: 10.1038/s41421-020-00239-w
Lv, L. J., Li, S. H., Li, S. C., Zhong, Z. C., Duan, H. L., Tian, C., et al. (2019). Early-onset preeclampsia is associated with gut microbial alterations in antepartum and postpartum women. Front. Cell Infect. Microbiol. 9. doi: 10.3389/fcimb.2019.00224
Machleidt, F., Lehnert, H. (2011). [Central nervous system control of energy homeostasis]. Dtsch Med. Wochenschr 136 (11), 541–545. doi: 10.1055/s-0031-1274539
Marques, C., Meireles, M., Norberto, S., Leite, J., Freitas, J., Pestana, D., et al. (2016). High-fat diet-induced obesity rat model: a comparison between wistar and sprague-dawley rat. Adipocyte 5 (1), 11–21. doi: 10.1080/21623945.2015.1061723
Nemet, I., Saha, P. P., Gupta, N., Zhu, W., Romano, K. A., Skye, S. M., et al. (2020). A cardiovascular disease-linked gut microbial metabolite acts via adrenergic receptors. Cell 180 (5), 862–877.e822. doi: 10.1016/j.cell.2020.02.016
O’Hara, A. M., Shanahan, F. (2006). The gut flora as a forgotten organ. EMBO Rep. 7 (7), 688–693. doi: 10.1038/sj.embor.7400731
Pallikkuth, S., Mendez, R., Russell, K., Sirupangi, T., Kvistad, D., Pahwa, R., et al. (2021). Age associated microbiome and microbial metabolites modulation and its association with systemic inflammation in a rhesus macaque model. Front. Immunol. 12. doi: 10.3389/fimmu.2021.748397
Pierce, B. L., Ahsan, H., Vanderweele, T. J. (2011). Power and instrument strength requirements for mendelian randomization studies using multiple genetic variants. Int. J. Epidemiol. 40 (3), 740–752. doi: 10.1093/ije/dyq151
Pindjakova, J., Sartini, C., Lo Re, O., Rappa, F., Coupe, B., Lelouvier, B., et al. (2017). Gut dysbiosis and adaptive immune response in diet-induced obesity vs. Systemic Inflammation. Front. Microbiol. 8. doi: 10.3389/fmicb.2017.01157
Qiu, B., Xi, Y., Liu, F., Li, Y., Xie, X., Guo, J., et al. (2022). Gut microbiome is associated with the response to chemoradiotherapy in patients with non-small cell lung cancer. Int. J. Radiat. Oncol. Biol. Phys. S0360–3016 (22), 00749–0. doi: 10.1016/j.ijrobp.2022.07.032
Sanna, S., van Zuydam, N. R., Mahajan, A., Kurilshikov, A., Vich Vila, A., Võsa, U., et al. (2019). Causal relationships among the gut microbiome, short-chain fatty acids and metabolic diseases. Nat. Genet. 51 (4), 600–605. doi: 10.1038/s41588-019-0350-x
Schall, T. J., Lewis, M., Koller, K. J., Lee, A., Rice, G. C., Wong, G. H., et al. (1990). Molecular cloning and expression of a receptor for human tumor necrosis factor. Cell 61 (2), 361–370. doi: 10.1016/0092-8674(90)90816-w
Turpin, W., Espin-Garcia, O., Xu, W., Silverberg, M. S., Kevans, D., Smith, M. I., et al. (2016). Association of host genome with intestinal microbial composition in a large healthy cohort. Nat. Genet. 48 (11), 1413–1417. doi: 10.1038/ng.3693
Van Montfort, R., Slingsby, C., Vierling, E. (2001). Structure and function of the small heat shock protein/alpha-crystallin family of molecular chaperones. Adv. Protein Chem. 59, 105–156. doi: 10.1016/s0065-3233(01)59004-x
Verbanck, M., Chen, C. Y., Neale, B., Do, R. (2018). Detection of widespread horizontal pleiotropy in causal relationships inferred from mendelian randomization between complex traits and diseases. Nat. Genet. 50 (5), 693–698. doi: 10.1038/s41588-018-0099-7
Wardlaw, J. M., Murray, V., Berge, E., del Zoppo, G., Sandercock, P., Lindley, R. L., et al. (2012). Recombinant tissue plasminogen activator for acute ischaemic stroke: an updated systematic review and meta-analysis. Lancet 379 (9834), 2364–2372. doi: 10.1016/s0140-6736(12)60738-7
Wu, R., Ruan, X. L., Ruan, D. D., Zhang, J. H., Wang, H. L., Zeng, Q. Z., et al. (2021). Differences in gut microbiota structure in patients with stages 4-5 chronic kidney disease. Am. J. Transl. Res. 13 (9), 10056–10074.
Yates, A. D., Achuthan, P., Akanni, W., Allen, J., Allen, J., Alvarez-Jarreta, J., et al. (2020). Ensembl2022, 020. Nucleic Acids Res. 48 (D1), D682–d688. doi: 10.1093/nar/gkz966
Keywords: gut microbes, cardiovascular proteins, Mendelian randomization, causal effect, genome-wide association study
Citation: Zhang W, Zhang S, Zhao F, Du J and Wang Z (2022) Causal relationship between gut microbes and cardiovascular protein expression. Front. Cell. Infect. Microbiol. 12:1048519. doi: 10.3389/fcimb.2022.1048519
Received: 19 September 2022; Accepted: 17 November 2022;
Published: 05 December 2022.
Edited by:
Zhengwei Huang, Shanghai Jiao Tong University, ChinaCopyright © 2022 Zhang, Zhang, Zhao, Du and Wang. This is an open-access article distributed under the terms of the Creative Commons Attribution License (CC BY). The use, distribution or reproduction in other forums is permitted, provided the original author(s) and the copyright owner(s) are credited and that the original publication in this journal is cited, in accordance with accepted academic practice. No use, distribution or reproduction is permitted which does not comply with these terms.
*Correspondence: Zhe Wang, d3pfY211QDEyNi5jb20=
†These authors have contributed equally to this work
Disclaimer: All claims expressed in this article are solely those of the authors and do not necessarily represent those of their affiliated organizations, or those of the publisher, the editors and the reviewers. Any product that may be evaluated in this article or claim that may be made by its manufacturer is not guaranteed or endorsed by the publisher.
Research integrity at Frontiers
Learn more about the work of our research integrity team to safeguard the quality of each article we publish.