- Department of Immunology and Infectious Diseases, Harvard T.H. Chan School of Public Health, Boston, MA, United States
In the face of the unrelenting global burden of tuberculosis (TB), antibiotics remain our most effective tools to save lives and control the spread of Mycobacterium tuberculosis (Mtb). However, we confront a dual challenge in our use of antibiotics: simplifying and shortening the TB drug regimen while also limiting the emergence and propagation of antibiotic resistance. This task is now more feasible due to the increasing availability of bacterial genomic data at or near the point of care. These resources create an opportunity to envision how integration of bacterial genetic determinants of antibiotic response into treatment algorithms might transform TB care. Historically, Mtb drug resistance studies focused on mutations in genes encoding antibiotic targets and the resulting increases in the minimal inhibitory concentrations (MICs) above a breakpoint value. But recent progress in elucidating the effects of functional genetic diversity in Mtb has revealed various genetic loci that are associated with drug phenotypes such as low-level MIC increases and tolerance which predict the development of resistance and treatment failure. As a result, we are now poised to advance precision medicine approaches in TB treatment. By incorporating information regarding Mtb genetic characteristics into the development of drug regimens, clinical care which tailors antibiotic treatment to maximize the likelihood of success has come into reach.
Introduction
As the tuberculosis (TB) pandemic caused by Mycobacterium tuberculosis (Mtb) rages on, we must find new ways to augment the efficacy of antibiotics, our most powerful weapons in this war (The World Health Organization, 2021). Precision medicine represents a promising strategy, which would involve methods to mitigate the occurrence of treatment failure by predicting the antibiotic sensitivities of individual strains based on their genotype and modifying the drug regimen accordingly (Lange et al., 2020). Adopting precision medicine approaches such as tailored antibiotic treatment regimens informed by the results of next-generation molecular detection of mutations known to alter drug susceptibility could facilitate shortened treatment regimens and mitigate the development of resistance (Lange et al., 2020). However, such strategies require a roadmap of the relationship between bacterial variation, antibiotic sensitivities, and treatment outcomes. In this review, we will discuss advancements in our understanding of how Mtb genetic diversity affects antibiotic susceptibility, which has expanded conventional metrics and measures of drug sensitivity. We will highlight opportunities to apply this knowledge to bolster current efforts towards precision medicine for TB.
The genesis of our current understanding of drug resistance
The challenge of drug resistance in Mtb became clear almost immediately after the discovery of streptomycin (SM) in 1943 (Schatz et al., 1944). By 1947 two randomized controlled trials had been conducted to assess the efficacy of SM for TB (Marshall et al., 1948; Fox et al., 1954; Fox et al., 1999). In these trials, there was no long-term survival benefit to SM treatment; almost all patients receiving SM initially improved but most subsequently relapsed with drug resistant Mtb. These findings led to the recognition that Mtb with reduced antibiotic susceptibility would reliably emerge after a period of antibiotic selection, at least with a single agent.
In the late 1940’s and early 1950’s, drug susceptibility was measured by comparing the lowest concentration of a drug that inhibited the in vitro growth of strains isolated from patients to that of a reference strain, H37Rv, which had been derived from a lung lesion 50 years earlier (Mitchison, 1949). The presumption was that most Mtb strains at the time were drug sensitive by definition because they had not faced drug treatment before. More formally, the diagnostic assumption was that bacterial growth distributions in the presence of drug were normally distributed, and that all strains falling above this normal distribution were equally likely to fail SM treatment. This understanding simplified the technical complexity of standardizing measurements of drug responses and led the field to solutions for establishing resistance, such as the calibrated “minimum inhibitory concentration” (MIC), which was a comparative measure response in strains deemed “probably sensitive” (from newly diagnosed patients) and “probably resistant” (from treatment failures) (Fox et al., 1999). These approaches have now matured into the so-called proportion method whereby the critical concentration is the lowest concentration of drug, established by international convention, which inhibits 99% of the growth of a population of “phenotypically wild type” Mtb (The World Health Organization, 2018). The MIC is now defined as the lowest concentration of an antibiotic that inhibits visible bacterial growth of an individual strain and is often used by bench scientists (Schön et al., 2020). The MBC or minimum bactericidal concentration is the lowest concentration that kills 99% of the initial bacterial population and is utilized mostly in clinical settings (National Committee for Clinical Laboratory Standards, 1999). Even though the field recognizes a difference between low and high resistance to some antibiotics such as isoniazid (INH) or fluoroquinolones (Malik et al., 2012; Lempens et al., 2018), proportion methods such as these - or genetic proxies of proportion methods - remain the most commonly used approach to defining drug susceptibility in Mtb, and has had the effect of enforcing a dichotomized understanding of antibiotic resistance, where the “wildtype” is assumed to be more or less homogeneously sensitive and functionally drug naïve.
We note that that the assumption that “wildtype” Mtb is drug naïve was challenged early on by the increasing frequency of primary drug resistance, that is drug resistance in treatment naïve patients. By 1964, not even 20 years after the first SM trial, 11% of primary TB cases in Hong Kong were drug resistant (and 10% resistant to INH and/or SM in Kenya by 1974), reflecting remarkable drug pressure on the extant bacterial population (Hong Kong Government Tuberculosis ServiceBritish Medical Research Council Co-operative Investigation, 1964; An East African and British Medical Research Council Co-operative Investigation, 1978) even as measured by dichotomous assays. We propose that it is likely that the population distribution of other forms of altered drug susceptibility, not captured by conventional measures such as MIC assays and therefore not countered through subsequent regimen modifications, were also under this immense selective pressure and increasingly prevalent in the population.
Nonetheless, in a pragmatic field, the dichotomous definition of resistance became accepted as adequate where there was little capacity to measure drug susceptibility or tailor therapy based on bacterial features. Thus, as the modern short course chemotherapy regimens were developed through the 1980’s, length of treatment was established in part to minimize the effects of preexisting bacterial or host differences in drug responses that contributed to treatment failure. Radically simpler regimens were first tried in the 1950s - 3 months of INH alone, for example, which actually led to durable cure in nearly half of patients (46% or 62/134) (Blowers and Cooke, 1954), but the focus was on developing a universal regimen because there was little capacity to identify and act on predictors of short course therapy success or failure.
Given that drug regimens are designed around dichotomized measures of Mtb antibiotic resistance, in the genomic age the field has focused on delineating the bacterial genetic determinants that mediate MIC shifts above the critical concentration resistance breakpoint. This resulted in studies that identified and characterized antibiotic resistance determinants by zeroing in on individual mutations in the genes encoding drug targets or drug activators, such as single nucleotide polymorphisms in katG and inhA, rpoB, embB, pncA, and rpsL and rrs conferring resistance to INH, RIF, pyrazinamide (PZA), ethambutol (EMB), and SM respectively (Finken et al., 1993; Scorpio et al., 1997; Sreevatsan et al., 1997; Rozwarski et al., 1998; Ramaswamy et al., 2003; Hazbón et al., 2006; Juréen et al., 2008; Palomino and Martin, 2014). Such studies informed the development of GeneXpert MTB/RIF (Cepheid, Sunnyvale, CA, USA), the first test designed for rapid molecular detection of Mtb and RIF resistance (Boehme et al., 2010). Later advancements include Xpert MTB/XDR, which completes rapid susceptibility testing for additional first- and second-line drugs to partially address multidrug-resistant (MDR) and extensively drug-resistant (XDR) TB (Cao et al., 2021; Penn-Nicholson et al., 2022). These tests and other accessible rapid diagnostics represent a transformative first-step towards TB precision medicine. However, there is considerable space for improvement because drug resistance-conferring mutations and breakpoint MICs are not completely predictive of treatment outcomes (Liu et al., 2021). Therefore, the field has begun to consider the wider effect of Mtb genetic diversity on not only antibiotic resistance but other clinically-relevant antibiotic susceptibility phenotypes.
Moving beyond dichotomous measures of drug resistance
While the clinical TB field was focused on acquired, high-level drug resistance determined by MIC changes above a breakpoint value, the bench sciences have been rapidly expanding our understanding of the clinical implications of variation in MIC below the critical concentration and the associated phenotypes. This includes small increases in MIC below the resistance threshold and also tolerance and persistence phenotypes that have been reviewed previously (Lewis and Shan, 2017; Boldrin et al., 2020; Schrader et al., 2020). These also include a range of environmentally-driven alterations in drug susceptibility such as differentially culturable bacteria (Chengalroyen et al., 2016; Turapov et al., 2016), “fat and lazy” macrophage resident bacteria (Garton et al., 2008; Daniel et al., 2011), or bacterial subpopulations created by adaptive regulatory systems like the toxin-antitoxin systems (Slayden et al., 2018).
Historically, there has been little effort to incorporate these other forms of “drug conditioning” into clinical treatment frameworks because they have been somewhere between hard and impossible to measure in a clinical setting and of unclear clinical importance. However, there is data to suggest that we should pay attention to the spectrum of altered drug responses under high-level drug resistance, the mass of ice under the tip of the iceberg.
MIC measurements are accessible to clinical labs. Indeed, a range of MIC levels for Mtb clinical isolates were observed as early as 1953 when the methodology for the MIC determination of INH, SM, and para-aminosalicylic acid (PAS) was becoming standardized, but this was assumed part of the “wildtype”, drug sensitive distribution (Medical Research Council, 1953). However, in a landmark study, Colangeli et al. determined that putatively drug susceptible Mtb strains with higher MICs to RIF and INH are more likely to result in treatment failure (Colangeli et al., 2018). This observation indicates that small increases in MIC are clinically-relevant even though they have been historically overlooked and suggest a potential benefit of having the tools to identify these features.
With the advent of inexpensive sequencing technology, bacterial genomic data has also become widely available. This has been leveraged by methods such as candidate gene approaches to determine if mutations in known target genes of first-line drugs predict phenotypic antibiotic susceptibilities and bacterial genome-wide association studies (GWAS) to identify bacterial genetic variations associated with high-level drug resistance (Zhang et al., 2013; Coll et al., 2018; CRyPTIC consortium and the 100,000 genomes project, 2018; Hicks et al., 2018; Farhat et al., 2019; Lai and Ioerger, 2020). While the latter were originally undertaken to identify missing genetic determinants of MICs above breakpoint values, these studies have unearthed a suite of “stepping stone” mutations (Hicks et al., 2018; Safi et al., 2019; Hicks et al., 2020; Liu et al., 2022; Martini et al., 2022). These are mutations that at a minimum facilitate the acquisition of drug resistance but presumptively this occurs by reducing treatment efficacy. There have been few treatment cohorts with good metadata sufficiently powered to identify these factors through their direct association with treatment outcomes. However, the population genomic argument is that the selection in the population is de facto evidence of their benefit to the bacterium. We would argue that these bacterial factors will become even more important as we push to shorten treatment, though relative importance of specific factors may differ by regimen (Turkova et al., 2022).
The ultimate importance of identifying and parsing the genetic basis of these other forms of drug susceptibilities depends on the implications of and ability to act on this knowledge. We would argue that critical factors include (A) ability to modify drug regimen based on this information and (B) implications for new drugs – that is, mutations that do not alter drug responses in a target-specific fashion but broadly alter responses in a way that will compromise new regimens.
Non-canonical bacterial genetical determinants and low-level MIC shifts
The genetic basis of the low-level INH and RIF MIC shifts associated with treatment failure by Colangeli et al. was not defined. However, several studies have suggested a range of candidate variants. These include mutations in both known pathway genes and novel pathways. Mutations associated with antibiotic resistance differ in how much they modulate MIC, for example the katG S315T mutation is associated with large, resistance-level increases in the MIC of INH, while other mutations such as the fabG1 C-15T polymorphism upstream of the INH target inhA results in smaller MIC shifts and low-level resistance (Lavender et al., 2005; Palomino and Martin, 2014). As for non-canonical targets, a Lineage 1 (L1) subclade-defining mutation in ndh (R268H) was associated with a ~2-fold increase in INH MIC, deemed neutral by the authors but only because it did not meet a standardized definition of resistance despite being present in 9.5% of INH-resistant strains but in none of the susceptible isolates, consistent with the changes in drug susceptibility associated with treatment failure (Lee et al., 2001; Merker et al., 2020).
Additionally, non-target variants associated with drug resistance that may contribute to moderate yet clinically-relevant MIC changes were identified by bacterial population GWAS studies (Zhang et al., 2013; Coll et al., 2018; Hicks et al., 2018; Farhat et al., 2019; Lai and Ioerger, 2020). Mutations in dnaA, which are found in 3.2% of all clinical strains globally, have been linked to INH resistance and have ~2-fold increase in MIC (Hicks et al., 2020). Low-level INH MIC-shifts may be relevant for new and old regimens alike, where the new 4-month regimen (rifapentine, moxifloxacin) still contains an INH backbone (Dorman et al., 2021). Further, rnaseJ is highly mutated in drug-resistant clinical strains of Mtb (Martini et al., 2022). While deletion of the gene does not alter the MIC to RIF nor INH, it does increase multidrug tolerance (Martini et al., 2022). Mutations in resR (Rv1830), a gene undergoing positive selection in Mtb clinical isolates, result in a slight MIC increase to INH, but more interestingly leads to faster bacterial recovery from drug treatment (Liu et al., 2022). ResR belongs to a regulatory pathway with WhiB2 and WhiA, and mutations in these genes were associated with canonical drug resistance and relapse after antibiotic treatment (Liu et al., 2022). Around 1.5%-9.7% of drug-sensitive Mtb strains from high-burden TB countries carry mutations in these genes, but mutations are present in 22.2% of strains from patients who failed treatment in the global REMoxTB phase 3 regimen-shortening trial (Bryant et al., 2013; Jindani et al., 2014; Liu et al., 2022).
Low-level MIC shifts have also been observed for second-line drugs. Mutations in gidB are associated with low-level SM resistance, but patients infected with Mtb strains carrying the rrs mutation that confers high-level amikacin resistance may still benefit from SM treatment despite the presence of gidB variants (Spies et al., 2011; Wong et al., 2011; Cohen et al., 2015; Cohen et al., 2020). gidB mutation may also explain the 15% of SM-resistant Mtb strains that lack mutations in rrs and rpsL (Wong et al., 2011). Even though SM is no longer used in the treatment of TB, this example illustrates the diagnostic benefit of non-target genes that mediate low-level resistance (Cohen et al., 2020). Mutations that impair the monooxygenase Rv0565c are associated with low-level resistance to the second-line antibiotic ethionamide (ETH) (Hicks et al., 2019). Like loss-of-function (LOF) mutations in ald that result in D-cycloserine resistance, Rv0565c mutations are found exclusively in MDR strains of Mtb, suggesting selection by second-line drug regimens (Desjardins et al., 2016; Hicks et al., 2019). Interestingly, low-level resistance has also been described for BDQ and CFZ, mediated by mutations in non-target genes including the regulator of the MmpS5-MmpL5 efflux pump system and pepQ (Andries et al., 2014; Almeida et al., 2016), and is more prevalent than predicted by exposure to these drugs.
Mtb genetic variation and antibiotic susceptibility
The original framing of altered drug susceptibility in Mtb implicitly posited that there were not clinically relevant differences in antibiotic susceptibility between Mtb strains before antibiotic exposure. However, as the L1 subclade-defining mutation in ndh suggests, it has become clear that this is an oversimplified view (Oppong et al., 2019; Merker et al., 2020).
Numerous studies reviewed elsewhere have indicated that L2 and L4 strains are more associated with drug resistance, MDR, and XDR (Gygli et al., 2017; Shanmugam et al., 2022). Several studies have demonstrated that L2 Mtb strains acquire drug resistances more rapidly in vitro (Ford et al., 2013), and are also more likely to develop RIF resistance after becoming resistant to INH (Torres Ortiz et al., 2021). This may reflect factors such as different mutation rates or epistatic interactions with lineage- or sublineage-associated mutations (Borrell and Gagneux, 2011; Ford et al., 2013; Torres Ortiz et al., 2021).
Other epistatic effects have been described. Fenner et al. discovered that compared to other lineages, L1 strains are more likely to carry a specific a promoter mutation in inhA, a drug resistance determinant that encodes the enzymatic target of INH (Fenner et al., 2012). In addition, Mtb genetic background modulates the level of INH resistance conferred by other mutations known to mediate resistance, which suggests an epistatic interaction between drug resistance mutations and lineage genetic diversity (Fenner et al., 2012). Similarly, the essentiality of katG, the activator of INH, was shown to differ depending on strain genetic background (Carey et al., 2018). Further, the degree of essentiality for katG is associated with the katG mutation rate, suggesting that the epistatic interaction between katG essentiality and strain genetic background is associated with the development of drug resistance (Carey et al., 2018).
Torres et al. identified “pre-resistance” genomic loci and polymorphisms associated with increased risk for drug resistance acquisition which could inform precision medicine efforts to predict and preempt the occurrence (Torres Ortiz et al., 2021). Following the idea of pre-resistance, a subset of L2 strains carry an ancestral polymorphism in gidB, which could mediate some level of intrinsic SM resistance (Spies et al., 2011). Additionally, a subgroup of MDR L4 strains share a mutation in tlyA, a gene that mediates resistance to the second-line drug capreomycin (Walker et al., 2018; Merker et al., 2020). And fortunately, altered susceptibility mediated by clade-defining mutations is not always bad news. For example, a LOF mutation in whib7 shared by a subgroup of L1 strains results in increased macrolide sensitivity (Li et al., 2022). This subgroup is estimated to cause 43,000 cases of MDR-TB per year (Li et al., 2022; Edokimov et al., 2022). Further, specific clades of L1 and L4 carry LOF mutations in mmpl5 that could render them hypersusceptible to bedaquiline (BDQ) and clofazimine (CFZ) (Andries et al., 2014; Merker et al., 2020). Given the geographical distributions of Mtb lineage subgroups, these data underscore the need for drug susceptibility diagnostics that detect variants associated with the regional context (Manson et al., 2017).
Host-pathogen interactions shaping Mtb drug responses
In the cases of lineage or sublineage defining variants in genes like whib7 or mmpl5, Mtb strains have acquired altered sensitivity to drugs they have not seen before. This is often attributed to genetic drift. However, the alternative model is that there are some host environments which select for bacterial features that that are also advantageous to Mtb in the face of drug (Hicks et al., 2018; Safi et al., 2019). By identifying variants that alter Mtb antibiotic susceptibility in host-relevant contexts, the field has diversified the suite of candidate mutations that could improve molecular diagnostics.
Several studies have linked Mtb antibiotic sensitivity to features of the host environment such as carbon source availability. For example, Mtb glycerol starvation triggers a stress-resistance response that in turn promote multidrug antibiotic tolerance (Safi et al., 2019). This phenotype is mediated by transient frameshift mutations that impair the glycerol kinase-encoding gene glpK, mutations in which are associated with MDR and XDR Mtb strains (Bellerose et al., 2019; Safi et al., 2019). Nonsynonmoyous glpK mutations were identified in 6.6% of Mtb strains from a Peruvian cohort (Bellerose et al., 2019). Mutations in glpK accrue during in vitro drug treatment and human infection, so this serves as another example of how bacterial adaptation to the host may augment antibiotic susceptibility (Bellerose et al., 2019; Safi et al., 2019; Vargas and Farhat, 2020). Mutations in the transcription factor encoded by prpR are associated with drug resistant Mtb clinical strains (Hicks et al., 2018). prpR mutations mediate drug tolerance to INH, RIF, and the second-line antibiotic ofloxacin (OFL) only during macrophage infection or in liquid media supplemented with propionate (Hicks et al., 2018). prpR mutations are enriched in Chinese clinical isolates, with a prevalence of 8-10% (Hicks et al., 2018). The glycerol and propionate phenotypes are consistent with other studies that investigated Mtb drug tolerance and phosphoenolpyruvate starvation in the context of the nonreplicating state induced by hypoxia, a stress relevant to the lung environment that selects for resistance (Liu et al., 2016; Lim et al., 2021). Similarly, Dhar and McKinney discovered that cydC and the rv0096–rv0101 gene set are persistence genetic determinants that alter Mtb clearance in C57BL/6 mice treated with INH (Dhar and McKinney, 2010). The persistence phenotypes are dependent on the mouse tissue environment, suggesting an interaction between the Mtb physiological changes caused by mutations in these genes, INH, and components of the mouse tissue (Dhar and McKinney, 2010).
Bacterial variants and differences in host environments are likely to be compounded by host differences in the pharmacokinetics and pharmacodynamics of drug metabolism. It is clear that drug exposure matters. RIF concentrations 2 hours post-dosing is associated with TB treatment success (Ramachandran et al., 2017). Further, Chigutsa et al. determined that Mtb infection sterilization can be predicted by a nonlinear relationship between patient antibiotic concentrations and bacterial MICs (Chigutsa et al., 2015). Indeed, data suggest that altered susceptibility indicated by strain associated differences in MIC can be overcome with increased dosing (Ruesen et al., 2018).
Conclusion and discussion
Traditionally, Mtb antibiotic response studies focused on bacterial mutations in genes involved in the drug mechanism of action and MIC shifts above the resistance threshold. But examination of the larger role of Mtb genetic diversity and the associated clinically-relevant drug phenotypes has unearthed new avenues that can be exploited to develop the precision medicine toolkit and ultimately improve patient outcomes (Cohen et al., 2019) (Figure 1). By expanding the scope of molecular diagnostic tests, we can identify mutations in strains that allow us to predict antibiotic sensitivities and treatment failure, which direct us in optimizing the drug regimen and even dosing to maximize the likelihood of treatment success.
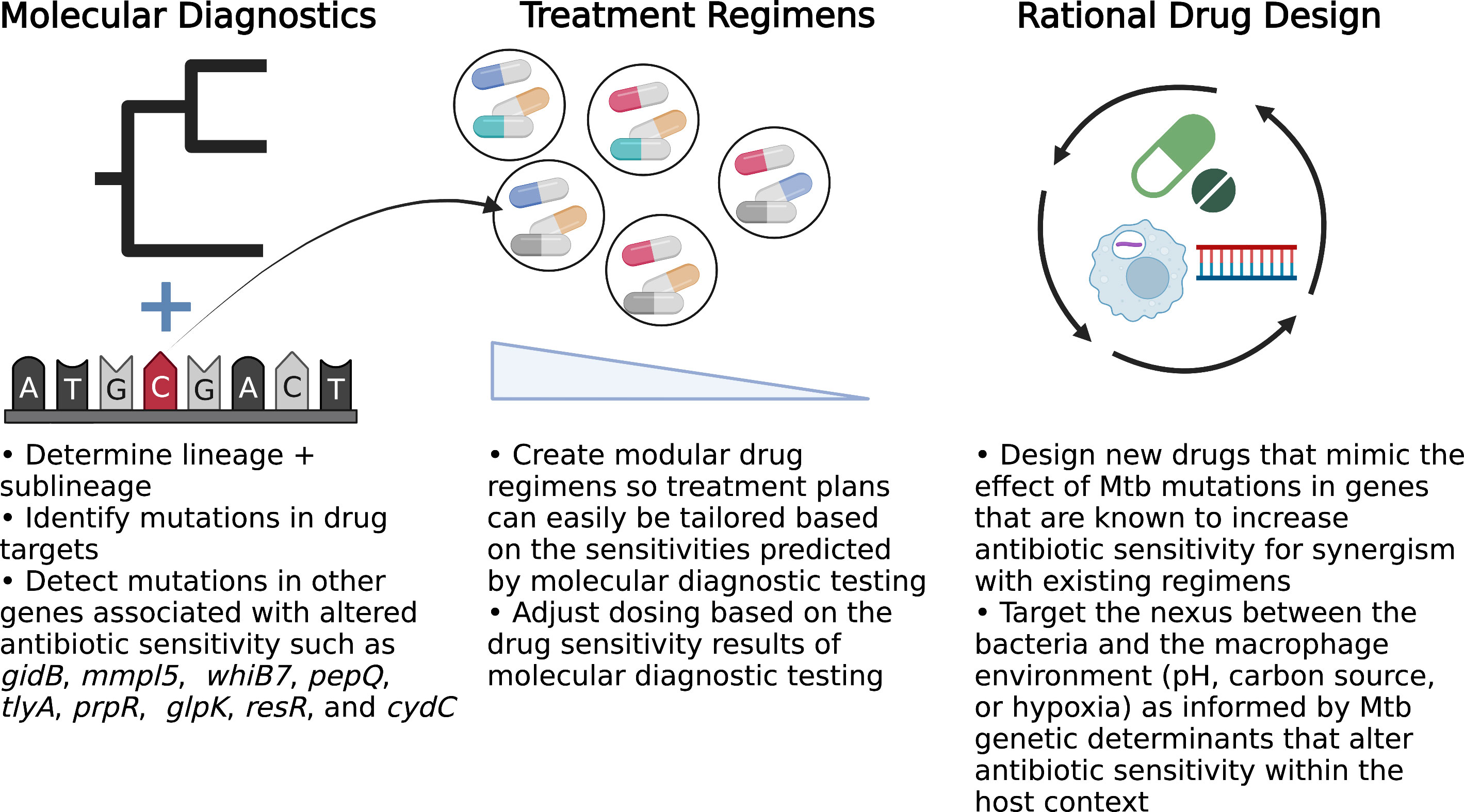
Figure 1 Opportunities to implement precision medicine approaches to improve TB treatment. BioRender.com.
We recognize that there are barriers to precision medicine in TB, including the cost of developing and implementing new tools and also epistatic interactions between diagnostic Mtb mutations and bacterial and host factors that could alter the phenotype penetrance and predictive power across populations. Therefore, we propose further research into understanding the prevalence and penetrance of potentially diagnostic mutations. This necessitates more studies that collect treatment outcome data and also WGS Mtb isolates. It is true that individual mutations may only have the power to predict resistance in a portion of strains, for example gyrA mutations only occur in 50-90% of fluoroquinolone-resistant clinical Mtb isolates (Brossier et al., 2010; Yin and Yu, 2010; Singh et al., 2015). To cover the lower end of the range, we should consider finding suites of mutations that together highly associate with resistance or clinical outcomes, a method that has already shown to be effective (Walker et al., 2015).
We can start by exploring mutations noted in a 2021 WHO catalogue of mutations associated with drug resistance, but in order to improve upon the growing collection of Mtb mutations with diagnostic potential, we should diversify our repertoire of phenotypes of clinical interest beyond just MIC (Walker et al., 2022). For example, further exploration into prevalent mutations in Mtb clinical isolates that modulate MBC, rather than just MIC, could reveal interesting predictors of host-relevant antibiotic tolerance phenotypes (Kalia et al., 2017; Sarathy et al., 2018; Dutta et al., 2019; Kreutzfeldt et al., 2022). Further, we should integrate Mtb strain characteristics with information regarding patient factors such as genetics, metabolism, environment, and geographic location to find novel ways to optimize treatment regimens and dosing. Given the presence of sublineage-defining mutations in genes associated with resistance and the endemic nature of these strains, even-high level patient information such as country or region of origin could inform which antibiotics may confer the maximum benefit (Manson et al., 2017; Walker et al., 2018; Hicks et al., 2020; Merker et al., 2020; Li et al., 2022). In the future, exploiting what we know and what we discover regarding host-pathogen interactions and altered antibiotic sensitivity can inform rational drug and rational drug regimen design. But in the meantime, more effort should go towards repurposing existing drugs by making TB antibiotic regimens and dosing modular so that treatment plans can be easily tuned for precise care.
We can no longer afford to take a one-size fits all approach to TB. It is our responsibility to leverage bench science advancement to improve patient outcomes via precision medicine.
Author contributions
SS, SF, and QL contributed to the conceptual framework of the review. SS wrote the first draft of the manuscript and SF wrote additional sections. SS, SF, and QL completed edits and revisions. All authors contributed to the article and approved the submitted version.
Funding
This study was funded by NIH/NIAID (P01AI132130, T32AI049928, T32AI132120).
Conflict of interest
The authors declare that the research was conducted in the absence of any commercial or financial relationships that could be construed as a potential conflict of interest.
Publisher's note
All claims expressed in this article are solely those of the authors and do not necessarily represent those of their affiliated organizations, or those of the publisher, the editors and the reviewers. Any product that may be evaluated in this article, or claim that may be made by its manufacturer, is not guaranteed or endorsed by the publisher.
References
Almeida, D., Ioerger, T., Tyagi, S., Li, S. Y., Mdluli, K., Andries, K., et al. (2016). Mutations in pepQ confer low-level resistance to bedaquiline and clofazimine in mycobacterium tuberculosis. Antimicrobial Agents chemotherapy 60 (8), 4590–4599. doi: 10.1128/AAC.00753-16
Andries, K., Villellas, C., Coeck, N., Thys, K., Gevers, T., Vranckx, L., et al. (2014). Acquired resistance of mycobacterium tuberculosis to bedaquiline. PloS One 9 (7), e102135. doi: 10.1371/journal.pone.0102135
An East African and British Medical Research Council Co-operative Investigation (1978). Tuberculosis in Kenya: A second national sampling survey of drug resistance and other factors, and a comparison with the prevalence data from the first national sampling survey. Tubercle 59 (3), 155–177. doi: 10.1016/0041-3879(78)90023-5
CRyPTIC consortium and the 100,000 genomes project (2018). Prediction of susceptibility to first-line tuberculosis drugs by DNA sequencing. New Engl. J. Med. 379 (15), 1403–1415. doi: 10.1056/NEJMoa1800474
Bellerose, M. M., Baek, S. H., Huang, C. C., Moss, C. E., Koh, E. I., Proulx, M. K., et al. (2019). Common variants in the glycerol kinase gene reduce tuberculosis drug efficacy. MBio 10 (4), e00663-19. doi: 10.1128/mBio.00663-19
Blowers, D. D., Cooke, D. J. (1954). Changes in isoniazid resistance of tubercle bacilli after cessation of treatment. Thorax 9, 254. doi: 10.1136/thx.9.4.254
Boehme, C. C., Nabeta, Hillemann, D., Nicol, M., Shenai, S., Krapp, F., et al. (2010). Rapid molecular detection of tuberculosis and rifampin resistance. New Engl. J. Med. 363 (11), 1005–1015. doi: 10.1056/NEJMoa0907847
Boldrin, F., Provvedi, R., Cioetto Mazzabò, L., Segafreddo, G., Manganelli, R. (2020). Tolerance and persistence to drugs: a main challenge in the fight against mycobacterium tuberculosis. Front. Microbiol. 11, 1924. doi: 10.3389/fmicb.2020.01924
Borrell, S., Gagneux, S. (2011). Strain diversity, epistasis and the evolution of drug resistance in mycobacterium tuberculosis. Clin. Microbiol. Infection 17 (6), 815–820. doi: 10.1111/j.1469-0691.2011.03556.x
Brossier, F., Veziris, N., Aubry, A., Jarlier, V., Sougakoff, W. (2010). Detection by GenoType MTBDR sl test of complex mechanisms of resistance to second-line drugs and ethambutol in multidrug-resistant mycobacterium tuberculosis complex isolates. J. Clin. Microbiol. 48 (5), 1683–1689. doi: 10.1128/JCM.01947-09
Bryant, J. M., Harris, S. R., Parkhill, J., Dawson, R., Diacon, A. H., van Helden, et al. (2013). Whole-genome sequencing to establish relapse or re-infection with mycobacterium tuberculosis: A retrospective observational study. Lancet Respir. Med. 1 (10), 786–792. doi: 10.1016/S2213-2600(13)70231-5
Cao, Y., Parmar, H., Gaur, R. L., Lieu, D., Raghunath, S., Via, N., et al. (2021). Xpert MTB/XDR: A 10-color reflex assay suitable for point-of-care settings to detect isoniazid, fluoroquinolone, and second-line-injectable-drug resistance directly from mycobacterium tuberculosis-positive sputum. J. Clin. Microbiol. 59 (3), e02314-20. doi: 10.1128/JCM.02314-20
Carey, A. F., Rock, J. M., Krieger, I. V., Chase, M. R., Fernandez-Suarez, M., Gagneux, S., et al. (2018). TnSeq of mycobacterium tuberculosis clinical isolates reveals strain-specific antibiotic liabilities. PloS Pathog. 14 (3), e1006939. doi: 10.1371/journal.ppat.1006939
Chengalroyen, M. D., Beukes, G. M., Gordhan, B. G., Streicher, E. M., Churchyard, G., Hafner, R., et al. (2016). Detection and quantification of differentially culturable tubercle bacteria in sputum from patients with tuberculosis. Am. J. Respir. Crit. Care Med. 194 (12), 1532–1540. doi: 10.1164/rccm.201604-0769OC
Chigutsa, E., Pasipanodya, J. G., Visser, M. E., Van Helden, D., Smith, J., Sirgel, F. A., et al. (2015). Impact of nonlinear interactions of pharmacokinetics and MICs on sputum bacillary kill rates as a marker of sterilizing effect in tuberculosis. Antimicrobial Agents chemotherapy 59 (1), 38–45. doi: 10.1128/AAC.03931-14
Cohen, K. A., Abeel, T., Manson McGuire, A., Desjardins, C. A., Munsamy, V., Shea, T., et al. (2015). Evolution of extensively drug-resistant tuberculosis over four decades: Whole genome sequencing and dating analysis of mycobacterium tuberculosis isolates from KwaZulu-natal. PloS Med. 12 (9), e1001880. doi: 10.1371/journal.pmed.1001880
Cohen, K. A., Manson, A. L., Desjardins, C. A., Abeel, T., Earl, A. M. (2019). Deciphering drug resistance in mycobacterium tuberculosis using whole-genome sequencing: Progress, promise, and challenges. Genome Med. 11 (1), 1–pp18. doi: 10.1186/s13073-019-0660-8
Cohen, K. A., Stott, K. E., Munsamy, V., Manson, A. L., Earl, A. M., Pym, A. S. (2020). Evidence for expanding the role of streptomycin in the management of drug-resistant mycobacterium tuberculosis. Antimicrobial Agents chemotherapy 64 (9), e00860–20. doi: 10.1128/AAC.00860-20
Colangeli, R., Jedrey, H., Kim, S., Connell, R., Ma, S., Chippada Venkata, U. D., et al. (2018). Bacterial factors that predict relapse after tuberculosis therapy. New Engl. J. Med. 379 (9), 823–833. doi: 10.1056/NEJMoa1715849
Coll, F., Phelan, J., Hill-Cawthorne, G. A., Nair, M. B., Mallard, K., Ali, S., et al. (2018). Genome-wide analysis of multi-and extensively drug-resistant mycobacterium tuberculosis. Nat. Genet. 50 (2), 307–316. doi: 10.1038/s41588-017-0029-0
Daniel, J., Maamar, H., Deb, C., Sirakova, T. D., Kolattukudy, E. (2011). Mycobacterium tuberculosis uses host triacylglycerol to accumulate lipid droplets and acquires a dormancy-like phenotype in lipid-loaded macrophages. PloS Pathog. 7 (6), e1002093. doi: 10.1371/journal.ppat.1002093
Desjardins, C. A., Cohen, K. A., Munsamy, V., Abeel, T., Maharaj, K., Walker, B. J., et al. (2016). Genomic and functional analyses of mycobacterium tuberculosis strains implicate ald in d-cycloserine resistance. Nat. Genet. , 48 (5), 544–551. doi: 10.1038/ng.3548
Dhar, N., McKinney, J. D. (2010). Mycobacterium tuberculosis persistence mutants identified by screening in isoniazid-treated mice. Proc. Natl. Acad. Sci. 107 (27), 12275–12280. doi: 10.1073/pnas.1003219107
Dorman, S. E., Nahid, Kurbatova, E. V., Phillips, Bryant, K., Dooley, K. E., et al. (2021). Four-month rifapentine regimens with or without moxifloxacin for tuberculosis. New Engl. J. Med. 384 (18), 1705–1718. doi: 10.1056/NEJMoa2033400
Dutta, N. K., Klinkenberg, L. G., Vazquez, M. J., Segura-Carro, D., Colmenarejo, G., Ramon, F., et al. (2019). Inhibiting the stringent response blocks mycobacterium tuberculosis entry into quiescence and reduces persistence. Sci. Adv. 5 (3), eaav2104. doi: 10.1126/sciadv.aav2104
Edokimov, K., Yamada, Y., Dary, C., Miow, Q. H., Hsu, L. Y., Ong, R. T. H., et al. (2022). Whole-genome sequencing of mycobacterium tuberculosis from Cambodia. Sci. Re 12 (1), 1–8. doi: 10.1038/s41598-022-10964-9
Farhat, M. R., Freschi, L., Calderon, R., Ioerger, T., Snyder, M., Meehan, C. J., et al. (2019). GWAS for quantitative resistance phenotypes in mycobacterium tuberculosis reveals resistance genes and regulatory regions. Nat. Commun. 10 (1), 1–pp11. doi: 10.1038/s41467-019-10110-6
Fenner, L., Egger, M., Bodmer, T., Altpeter, E., Zwahlen, M., Jaton, K., et al. (2012). Effect of mutation and genetic background on drug resistance in mycobacterium tuberculosis. Antimicrobial Agents chemotherapy 56 (6), 3047–3053. doi: 10.1128/AAC.06460-11
Finken, M., Kirschner, Meier, A., Wrede, A., Böttger, E. C. (1993). Molecular basis of streptomycin resistance in mycobacterium tuberculosis: Alterations of the ribosomal protein S12 gene and point mutations within a functional 16S ribosomal RNA pseudoknot. Mol. Microbiol. 9 (6), 1239–1246. doi: 10.1111/j.1365-2958.1993.tb01253.x
Ford, C. B., Shah, R. R., Maeda, M. K., Gagneux, S., Murray, M. B., Cohen, T., et al. (2013). Mycobacterium tuberculosis mutation rate estimates from different lineages predict substantial differences in the emergence of drug-resistant tuberculosis. Nat. Genet. 45 (7), 784–790. doi: 10.1038/ng.2656
Fox, W., Ellard, G. A., Mitchison, D. A. (1999). Studies on the treatment of tuberculosis undertaken by the British medical research council tuberculosis units, 1946–1986, with relevant subsequent publications. Int. J. Tuberculosis Lung Dis. 3 (10), S231–S279.
Fox, W., Sutherland, I., Daniels, M. (1954). A five-year assessment of patients in a controlled trial of streptomycin in pulmonary tuberculosis. Q. J. Med. 23 (91), 347–366.
Garton, N. J., Waddell, S. J., Sherratt, A. L., Lee, S. M., Smith, R. J., Senner, C., et al. (2008). Cytological and transcript analyses reveal fat and lazy persister-like bacilli in tuberculous sputum. PloS Med. 5 (4), e75. doi: 10.1371/journal.pmed.0050075
Gygli, S. M., Borrell, S., Trauner, A., Gagneux, S. (2017). Antimicrobial resistance in mycobacterium tuberculosis: Mechanistic and evolutionary perspectives. FEMS Microbiol. Rev. 41 (3), 354–373. doi: 10.1093/femsre/fux011
Hazbón, M. H., Brimacombe, M., Bobadilla del Valle, M., Cavatore, M., Guerrero, M. I., Varma-Basil, M., et al. (2006). Population genetics study of isoniazid resistance mutations and evolution of multidrug-resistant mycobacterium tuberculosis. Antimicrobial Agents chemotherapy 50 (8), 2640–2649. doi: 10.1128/AAC.00112-06
Hicks, N. D., Carey, A. F., Yang, J., Zhao, Y., Fortune, S. M. (2019). Bacterial genome-wide association identifies novel factors that contribute to ethionamide and prothionamide susceptibility in mycobacterium tuberculosis. MBio 10 (2), e00616–19. doi: 10.1128/mBio.00616-19
Hicks, N. D., Giffen, S. R., Culviner, H., Chao, M. C., Dulberger, C. L., Liu, Q., et al. (2020). Mutations in dnaA and a cryptic interaction site increase drug resistance in mycobacterium tuberculosis. PloS Pathog. 16 (11), e1009063. doi: 10.1371/journal.ppat.1009063
Hicks, N. D., Yang, J., Zhang, X., Zhao, B., Grad, Y. H., Liu, L., et al. (2018). Clinically prevalent mutations in mycobacterium tuberculosis alter propionate metabolism and mediate multidrug tolerance. Nat. Microbiol. 3 (9), 1032–1042. doi: 10.1038/s41564-018-0218-3
Hong Kong Government Tuberculosis ServiceBritish Medical Research Council Co-operative Investigation, A. (1964). Drug-resistance in patients with pulmonary tuberculosis presenting at chest clinics in Hong Kong. Tubercle 45 (2), 77–95. doi: 10.1016/S0041-3879(64)80064-7
Jindani, A., Harrison, T. S., Nunn, A. J., Phillips, Churchyard, G. J., Charalambous, S., et al. (2014). High-dose rifapentine with moxifloxacin for pulmonary tuberculosis. N Engl. J. Med. 371, 1599–1608. doi: 10.1056/NEJMoa1314210
Juréen, Werngren, J., Toro, J. C., Hoffner, S. (2008). Pyrazinamide resistance and pncA gene mutations in mycobacterium tuberculosis. Antimicrobial Agents chemotherapy 52 (5), 1852–1854. doi: 10.1128/AAC.00110-08
Kalia, N., Hasenoehrl, E. J., Ab Rahman, N. B., Koh, V. H., Ang, M. L., Sajorda, D. R., et al. (2017). Exploiting the synthetic lethality between terminal respiratory oxidases to kill mycobacterium tuberculosis and clear host infection. Proc. Natl. Acad. Sci. 114 (28), 7426–7431. doi: 10.1073/pnas.1706139114
Kreutzfeldt, K. M., Jansen, R. S., Hartman, T. E., Gouzy, A., Wang, R., Krieger, I. V., et al. (2022). CinA mediates multidrug tolerance in mycobacterium tuberculosis. Nat. Commun. 13 (1), 1–pp11. doi: 10.1038/s41467-022-29832-1
Lai, Y., Ioerger, T. R. (2020). Exploiting homoplasy in genome-wide association studies to enhance identification of antibiotic-resistance mutations in bacterial genomes. Evolutionary Bioinf. 16, 1176934320944932. doi: 10.1177/1176934320944932
Lange, C., Aarnoutse, R., Chesov, D., Van Crevel, R., Gillespie, S. H., Grobbel, H., et al. (2020). Perspective for precision medicine for tuberculosis. Front. Immunol. 11, 566608. doi: 10.3389/fimmu.2020.566608
Lavender, C., Globan, M., Sievers, A., Billman-Jacobe, H., Fyfe, J. (2005). Molecular characterization of isoniazid-resistant mycobacterium tuberculosis isolates collected in Australia. Antimicrobial Agents chemotherapy 49 (10), 4068–4074. doi: 10.1128/AAC.49.10.4068-4074.2005
Lee, A. S., Teo, A. S., Wong, S. Y. (2001). Novel mutations in ndh in isoniazid-resistant mycobacterium tuberculosis isolates. Antimicrobial Agents chemotherapy 45 (7), 2157–2159. doi: 10.1128/AAC.45.7.2157-2159.2001
Lempens, Meehan, C. J., Vandelannoote, K., Fissette, K., de Rijk, Van Deun, A., et al. (2018). Isoniazid resistance levels of mycobacterium tuberculosis can largely be predicted by high-confidence resistance-conferring mutations. Sci. Re 8 (1), 1–9. doi: 10.1038/s41598-018-21378-x
Lewis, K., Shan, Y. (2017). Why tolerance invites resistance. Science 355 (6327), 796–796. doi: 10.1126/science.aam7926
Lim, J., Lee, J. J., Lee, S. K., Kim, S., Eum, S. Y., Eoh, H. (2021). Phosphoenolpyruvate depletion mediates both growth arrest and drug tolerance of mycobacterium tuberculosis in hypoxia. Proc. Natl. Acad. Sci. 118 (35), e2105800118. doi: 10.1073/pnas.2105800118
Li, S., Poulton, N. C., Chang, J. S., Azadian, Z. A., Dejusus, M. A., Ruecker, N., et al. (2022). CRISPRi chemical genetics and comparative genomics identify genes mediating drug potency in Mycobacterium tuberculosis. Nature Microbiology 1–14. doi: 10.1101/2021.11.27.469863
Liu, Z., Gao, Y., Yang, H., Bao, H., Qin, L., Zhu, C., et al. (2016). Impact of hypoxia on drug resistance and growth characteristics of mycobacterium tuberculosis clinical isolates. PloS One 11 (11), e0166052. doi: 10.1371/journal.pone.0166052
Liu, Q., Yang, D., Qiu, B., Martinez, L., Ji, Y., Song, H., et al. (2021). Drug resistance gene mutations and treatment outcomes in MDR-TB: A prospective study in Eastern China. PloS Negl. Tro Dis. 15 (1), e0009068. doi: 10.1371/journal.pntd.0009068
Liu, Q., Zhu, J., Dulberger, C. L., Stanley, S., Wilson, S., Chung, E. S., et al. (2022). Tuberculosis treatment failure associated with evolution of antibiotic resilience. bioRxiv. doi: 10.1101/2022.03.29.486233
Malik, S., Willby, M., Sikes, D., Tsodikov, O. V., Posey, J. E. (2012). New insights into fluoroquinolone resistance in mycobacterium tuberculosis: Functional genetic analysis of gyrA and gyrB mutations. PloS One 7 (6), e39754. doi: 10.1371/journal.pone.0039754
Manson, A. L., Abeel, T., Galagan, J. E., Sundaramurthi, J. C., Salazar, A., Gehrmann, T., et al. (2017). Mycobacterium tuberculosis whole genome sequences from southern India suggest novel resistance mechanisms and the need for region-specific diagnostics. Clin. Infect. Dis. 64 (11), 1494–1501. doi: 10.1093/cid/cix169
Marshall, G., Blacklock, J. S., Cameron, C., Capon, N. B., Cruickshank, R., Gaddum, J. H., et al. (1948). Streptomycin treatment of tuberculous meningitis. Lancet 254, 582–596.
Martini, M. C., Hicks, N. D., Xiao, J., Alonso, M. N., Barbier, T., Sixsmith, J., et al. (2022). Loss of RNase J leads to multi-drug tolerance and accumulation of highly structured mRNA fragments in mycobacterium tuberculosis. PloS Pathog. 18 (7), e1010705. doi: 10.1371/journal.ppat.1010705
Medical Research Council (1953). Laboratory techniques for the determination of sensitivity of tubercle bacilli to isoniazid, streptomycin and PAS. Lancet 2, 213.
Merker, M., Kohl, T. A., Barilar, I., Andres, S., Fowler, W., Chryssanthou, E., et al. (2020). Phylogenetically informative mutations in genes implicated in antibiotic resistance in mycobacterium tuberculosis complex. Genome Med. 12 (1), 1–8. doi: 10.1186/s13073-020-00726-5
Mitchison, D. A. (1949). Tests for streptomycin sensitivity of tubercle bacilli in tween 80 albumin liquid medium. Lancet 254, 694–696. doi: 10.1016/S0140-6736(49)91327-6
National Committee for Clinical Laboratory Standards, Barry, A. L. (1999). Methods for determining bactericidal activity of antimicrobial agents: Approved guideline Vol. Vol. 19, No. 18) (Wayne, PA: National Committee for Clinical Laboratory Standards).
Oppong, Y. E., Phelan, J., Perdigão, J., Machado, D., Miranda, A., Portugal, I., et al. (2019). Genome-wide analysis of mycobacterium tuberculosis polymorphisms reveals lineage-specific associations with drug resistance. BMC Genomics 20 (1), 1–pp15. doi: 10.1186/s12864-019-5615-3
Palomino, J. C., Martin, A. (2014). Drug resistance mechanisms in mycobacterium tuberculosis. Antibiotics 3 (3), 317–340. doi: 10.3390/antibiotics3030317
Penn-Nicholson, A., Georghiou, S. B., Ciobanu, N., Kazi, M., Bhalla, M., David, A., et al. (2022). Detection of isoniazid, fluoroquinolone, ethionamide, amikacin, kanamycin, and capreomycin resistance by the xpert MTB/XDR assay: a cross-sectional multicentre diagnostic accuracy study. Lancet Infect. Dis. 22 (2), 242–249. doi: 10.1016/S1473-3099(21)00452-7
Ramachandran, G., Agibothu Kupparam, H. K., Vedhachalam, C., Thiruvengadam, K., Rajagandhi, V., Dusthackeer, A., et al. (2017). Factors influencing tuberculosis treatment outcome in adult patients treated with thrice-weekly regimens in India. Antimicrobial Agents chemotherapy 61 (5), e02464-16. doi: 10.1128/AAC.02464-16
Ramaswamy, S. V., Reich, R., Dou, S. J., Jasperse, L., Pan, X., Wanger, A., et al. (2003). Single nucleotide polymorphisms in genes associated with isoniazid resistance in mycobacterium tuberculosis. Antimicrobial Agents chemotherapy 47 (4), 1241–1250. doi: 10.1128/AAC.47.4.1241-1250.2003
Rozwarski, D. A., Grant, G. A., Barton, D. H., Jacobs, W. R., Jr., Sacchettini, J. C. (1998). Modification of the NADH of the isoniazid target (InhA) from mycobacterium tuberculosis. Science 279 (5347), 98–pp102. doi: 10.1126/science.279.5347.98
Ruesen, C., Riza, A. L., Florescu, A., Chaidir, L., Editoiu, C., Aalders, N., et al. (2018). Linking minimum inhibitory concentrations to whole genome sequence-predicted drug resistance in mycobacterium tuberculosis strains from Romania. Sci. Re 8 (1), 1–8. doi: 10.1038/s41598-018-27962-5
Safi, H., Gopal, Lingaraju, S., Ma, S., Levine, C., Dartois, V., et al. (2019). Phase variation in mycobacterium tuberculosis glpK produces transiently heritable drug tolerance. Proc. Natl. Acad. Sci. 116 (39), 19665–19674. doi: 10.1073/pnas.1907631116
Sarathy, J., Via, L. E., Weiner, D., Blanc, L., Boshoff, H., Eugenin, E. A., et al. (2018). Extreme drug tolerance of mycobacterium tuberculosis in caseum. Antimicrobial Agents chemotherapy 62 (2), e02266–e02217. doi: 10.1128/AAC.02266-17
Schatz, A., Bugle, E., Waksman, S. A. (1944). Streptomycin, a substance exhibiting antibiotic activity against gram-positive and gram-negative bacteria. Proc. Soc. Ex Biol. Med. 55 (1), 66–69. doi: 10.3181/00379727-55-14461
Schön, T., Werngren, J., Machado, D., Borroni, E., Wijkander, M., Lina, G., et al. (2020). Antimicrobial susceptibility testing of mycobacterium tuberculosis complex isolates–the EUCAST broth microdilution reference method for MIC determination Clin Microbiol Infect. 26(11), 1488–92. doi: 10.1016/j.cmi.2020.07.036
Schrader, S. M., Vaubourgeix, J., Nathan, C. (2020). Biology of antimicrobial resistance and approaches to combat it. Sci. Trans. Med. 12 (549), eaaz6992. doi: 10.1126/scitranslmed.aaz6992
Scorpio, A., Lindholm-Levy, Heifets, L., Gilman, R., Siddiqi, S., Cynamon, M., et al. (1997). Characterization of pncA mutations in pyrazinamide-resistant mycobacterium tuberculosis. Antimicrobial Agents chemotherapy 41 (3), 540–543. doi: 10.1128/AAC.41.3.540
Shanmugam, S. K., Kumar, N., Sembulingam, T., Ramalingam, S. B., Selvaraj, A., Rajendhiran, U., et al. (2022). Mycobacterium tuberculosis lineages associated with mutations and drug resistance in isolates from India. Microbiol. Spectr. 10 e01594-21. doi: 10.1128/spectrum.01594-21
Singh, Jain, A., Dixit, Prakash, S., Jaiswal, I., Venkatesh, V., et al. (2015). Prevalence of gyrA and b gene mutations in fluoroquinolone-resistant and-sensitive clinical isolates of mycobacterium tuberculosis and their relationship with MIC of ofloxacin. J. antibiotics 68 (1), 63–66. doi: 10.1038/ja.2014.95
Slayden, R. A., Dawson, C. C., Cummings, J. E. (2018). Toxin–antitoxin systems and regulatory mechanisms in mycobacterium tuberculosis. Pathog. Dis. 76 (4), fty039. doi: 10.1093/femspd/fty039
Spies, F. S., Ribeiro, A. W., Ramos, D. F., Ribeiro, M. O., Martin, A., Palomino, J. C., et al. (2011). Streptomycin resistance and lineage-specific polymorphisms in mycobacterium tuberculosis gidB gene. J. Clin. Microbiol. 49 (7), 2625–2630. doi: 10.1128/JCM.00168-11
Sreevatsan, S., Stockbauer, K. E., Pan, X. I., Kreiswirth, B. N., Moghazeh, S. L., Jacobs, W. R., Jr., et al. (1997). Ethambutol resistance in mycobacterium tuberculosis: Critical role of embB mutations. Antimicrobial Agents chemotherapy 41 (8), 1677–1681. doi: 10.1128/AAC.41.8.1677
The World Health Organization (2018) Technical report on critical concentrations for drug susceptibility testing of medicines used in the treatment of drug-resistant tuberculosis. Available at: https://www.who.int/publications/i/item/WHO-CDS-TB-2018.5.
The World Health Organization (2021) TB disease burden. Available at: https://www.who.int/publications/digital/global-tuberculosis-report-2021/tb-disease-burden.
Torres Ortiz, A., Coronel, J., Vidal, J. R., Bonilla, C., Moore, D. A., Gilman, R. H., et al. (2021). Genomic signatures of pre-resistance in mycobacterium tuberculosis. Nat. Commun. 12 (1), 1–pp13. doi: 10.21203/rs.3.rs-364747/v1
Turapov, O., O’Connor, B. D., Sarybaeva, A. A., Williams, C., Patel, H., Kadyrov, A. S., et al. (2016). Phenotypically adapted mycobacterium tuberculosis populations from sputum are tolerant to first-line drugs. Antimicrobial Agents chemotherapy 60 (4), 2476–2483. doi: 10.1128/AAC.01380-15
Turkova, A., Wills, G. H., Wobudeya, E., Chabala, C., Palmer, M., Kinikar, A., et al. (2022). Shorter treatment for nonsevere tuberculosis in African and Indian children. New Engl. J. Med. 386 (10), 911–922. doi: 10.1056/NEJMoa2104535
Vargas, R., Jr., Farhat, M. R. (2020). Antibiotic treatment and selection for glpK mutations in patients with active tuberculosis disease. Proc. Natl. Acad. Sci. 117 (8), 3910–3912. doi: 10.1073/pnas.1920788117
Walker, T. M., Kohl, T. A., Omar, S. V., Hedge, J., Elias, C. D. O., Bradley, et al. (2015). Whole-genome sequencing for prediction of mycobacterium tuberculosis drug susceptibility and resistance: a retrospective cohort study. Lancet Infect. Dis. 15 (10), 1193–1202. doi: 10.1016/S1473-3099(15)00062-6
Walker, T. M., Merker, M., Knoblauch, A. M., Helbling, Schoch, O. D., van der Werf, M. J., et al. (2018). A cluster of multidrug-resistant mycobacterium tuberculosis among patients arriving in Europe from the horn of Africa: A molecular epidemiological study. Lancet Infect. Dis. 18 (4), 431–440. doi: 10.1016/S1473-3099(18)30004-5
Walker, T. M., Miotto, Köser, C. U., Fowler, W., Knaggs, J., Iqbal, Z., et al. (2022). The 2021 WHO catalogue of mycobacterium tuberculosis complex mutations associated with drug resistance: a genotypic analysis. Lancet Microbe 3 (4), e265–e273. doi: 10.1016/S2666-5247(21)00301-3
Wong, S. Y., Lee, J. S., Kwak, H. K., Via, L. E., Boshoff, H. I., Barry, C. E., III (2011). Mutations in gidB confer low-level streptomycin resistance in mycobacterium tuberculosis. Antimicrobial Agents chemotherapy 55 (6), 2515–2522. doi: 10.1128/AAC.01814-10
Yin, X., Yu, Z. (2010). Mutation characterization of gyrA and gyrB genes in levofloxacin-resistant mycobacterium tuberculosis clinical isolates from guangdong province in China. J. Infection 61 (2), 150–154. doi: 10.1016/j.jinf.2010.05.001
Keywords: mycobacterium tuberculosis, antibiotic resistance, genetic diversity, precision medicine, bacterial genomics, molecular diagnostics, TB drug regimen, MIC
Citation: Stanley S, Liu Q and Fortune SM (2022) Mycobacterium tuberculosis functional genetic diversity, altered drug sensitivity, and precision medicine. Front. Cell. Infect. Microbiol. 12:1007958. doi: 10.3389/fcimb.2022.1007958
Received: 31 July 2022; Accepted: 14 September 2022;
Published: 03 October 2022.
Edited by:
Ben Gold, Weill Cornell Medicine, United StatesReviewed by:
Christina Stallings, Washington University in St. Louis, United StatesCamus Nimmo, Francis Crick Institute, United Kingdom
Greg Cook, University of Otago, New Zealand
Copyright © 2022 Stanley, Liu and Fortune. This is an open-access article distributed under the terms of the Creative Commons Attribution License (CC BY). The use, distribution or reproduction in other forums is permitted, provided the original author(s) and the copyright owner(s) are credited and that the original publication in this journal is cited, in accordance with accepted academic practice. No use, distribution or reproduction is permitted which does not comply with these terms.
*Correspondence: Sarah M. Fortune, sfortune@hsph.harvard.edu