- 1Laboratory of Psychobiology, Department of Psychobiology and Methods in Behavioral Science, Faculty of Psychology, Complutense University of Madrid, Madrid, Spain
- 2Institute of Agricultural Biology and Biotechnology (IBBA), National Research Council (CNR), Pisa, Italy
- 3Dipartimento di Scienze della Salute, Università di Firenze, Firenze, Italy
- 4Red de Trastornos Adictivos (RTA), Instituto de Salud Carlos III (ISCIII), Madrid, Spain
Introduction: Chronic alcohol consumption is known to cause gut dysbiosis (changes in microbiota composition and/or function, disruptive of the normal host–microbiota interactions). However, little is known about the changes that alcohol binge drinking induces in the gut microbiota. Here, we have tested the hypothesis that a protocol of alcohol binge drinking, known to induce neuroinflammation in previous studies, also promotes intestinal dysbiosis, and we explored how oleoylethanolamide (OEA, an acylethanolamide proven to counteract alcohol binge drinking-induced neuroinflammation) pretreatment modulates alcohol-induced dysbiosis.
Methods: Alcohol binges were forced by gavage three times per day during 4 consecutive days; OEA pretreatment (intraperitoneal or intragastric) was administered before each alcohol gavage. Stool microbiota composition was assessed by next-generation 16S rRNA gene sequencing, prior and after the 4-day alcohol binge protocol.
Results: Alcohol binge drinking reduced the richness of the gut microbiota and changed the microbial community, reducing Lactobacillus among other genera. Pretreatment with OEA in the alcohol-administered rats decreased the richness, evenness, and Shannon indices to a greater extent with respect to alcohol alone, also changing the community structure. Microbial interactions in the association network were further decreased following OEA administration in the alcohol group, with respect to the water administration. The synergistic interaction between alcohol binge and OEA was affected by the route of administration of OEA, since oral and i.p. administrations differently changed the community structure.
Conclusion: Results suggest that alcohol binge drinking produces a clear dysbiosis in animals; we observed that the well-known protective actions of OEA in the context of alcohol abuse might not be related to OEA-induced changes in alcohol-induced dysbiosis. These are observational results, and thus, further research will be needed for a complete understanding of the biological significance of the observed changes.
Highlights
● After an alcohol binge drinking protocol (T1) in rats, we observed reduced richness of the fecal microbiota, with a distinguishable bacterial community from T0 (no treatment) and from the T1 water group. Alcohol increased the levels of Clostridium sensu stricto, Romboutsia, Turicibacter, Bifidobacterium, Parabacteroides, and Enterococcus and decreased the levels of Lactobacillus, Clostridium XIVa, Clostridium XIVb, and Oscillibacter.
● OEA administration in the water group slightly changed the community structure with respect to the vehicle group but did not change the α-diversity. Microbial interactions in the association network were increased following OEA administration in the water group.
● Alcohol and OEA appeared to synergistically interact with the gut microbiota. Pretreatment with OEA in the alcohol-administered rats decreased the richness, evenness, and Shannon indices and changed the community structure to a greater extent in comparison with the alcohol without OEA group. Microbial interactions in the association network were further decreased following OEA administration in the alcohol group with respect to the water group.
● The route of OEA administration had a significant effect in the altered community structure induced by alcohol. Oral OEA reduced richness and i.p. OEA created a more fragmented association network, with more non-interconnected nodes, in comparison with vehicle administration in alcohol rats.
Introduction
Alcohol abuse is a risk factor for many diseases and mortality worldwide. Alcohol binge drinking is a pattern of alcohol abuse defined as the consumption of more than four or five drinks in about 2 h for women and men, respectively, resulting in blood alcohol levels (BAL) ≥80 mg/dl (NIAAA, 2004). In 2015, in the National Survey on Drug Use and Health, it was shown that 26.9% of U.S. adults older than 18 years old reported binge drinking in the past 30 days (SAMHSA, 2017). Other reports indicate that 38 million of U.S. adults drink alcohol in this pattern four times per month, consuming eight drinks on average, and that half of the 88,000 deaths resulting from alcohol consumption are due to binge drinking (Kanny et al., 2015).
Alcohol binge drinking appears to promote alterations in the gut–brain axis. We have previously demonstrated that the binge drinking protocol used in this study induces gut barrier alterations, with consequences in peripheral inflammation and immune activation, neuroinflammation, and behavioral changes. On one hand, binge drinking induces colonic inflammation and a decrement of tight junction (TJ) protein expression in the colonic barrier, an indication of its loss of integrity. As a result, the cell wall component of Gram-negative bacteria, i.e., lipopolysaccharide (LPS), is found in the blood after alcohol binges, and interestingly, we also observed the passage of bacteria from the intestinal lumen to other organs, including the mesenteric lymph nodes (MLNs) and spleen. This pattern of alcohol binge drinking strongly activates the innate and adaptive immune systems and induces peripheral inflammation and corticosterone rise in plasma (Antón et al., 2017; Antón et al., 2018). On the other hand, it is well-known that binge drinking induces neuroinflammation in the frontal cortex, which has been related to anxiety and depressive-like behavior during early withdrawal (Antón et al., 2017).
The microbiota–gut–brain axis is a bidirectional communication between the microorganisms present in the gut and the brain that uses the immune system and neural and neuroendocrine pathways (Cryan and Dinan, 2012). Gut microbiota is composed of a plethora of microorganisms (predominantly bacteria, but also archaea, viruses, fungi, and protozoa among others) that live in different niches of our gastrointestinal tract, especially in the colon, in a symbiotic relationship with the host, cooperating in different functions such as digestion, immunity, inflammation, and intestinal barrier regulation (Wang and Wang, 2016). Additionally, it has been proposed that the gut microbiota participates in the regulation of brain function, mood, and behavior through modulation of the gut–brain axis (Cryan and Dinan, 2012).
Alterations in this axis have been well documented in several brain disorders and conditions (Sherwin et al., 2018), including alcohol use disorders (AUD) (Rodriguez-Gonzalez and Orio, 2020). It has been recently shown that AUD leads to gut dysbiosis in humans by altering the composition and/or function of the microbiota (Litwinowicz et al., 2019). In experimental models, alcohol generally increases bacteria from the phyla Proteobacteria and Firmicutes and decreases bacteria from the phylum Bacteroidetes (Engen et al., 2015). Alcohol-induced gut dysbiosis, together with the disrupted intestinal barrier and the LPS leakage to the systemic circulation, is associated with alcoholic liver disease, which is a leading cause of mortality worldwide (Hartmann et al., 2015; Meroni et al., 2019), and with behavioral abnormalities in alcohol-dependent subjects (Leclercq et al., 2014; Temko et al., 2017). Indeed, alcohol-induced alterations in gut microbiota may account for withdrawal symptoms such as anxiety, depression, craving, and reward-seeking behaviors (Hillemacher et al., 2018). However, alcohol consumption does not always cause gut dysbiosis, highlighting that there may be other factors involved (Mutlu et al., 2012; Timary et al., 2015) and that patterns of alcohol consumption must be taken into account. The actions of binge drinking on neuroinflammation are very well known, and we recently described binge drinking-induced dysfunction of the gut barrier that may influence cortical neuroinflammation (Antón et al., 2018; Orio, 2020; Rodríguez-González and Orio, 2020). Further research is needed to understand whether the gut–brain axis alterations induced by this pattern of alcohol consumption involve also changes in the gut microbiota composition and possible modulatory pharmacotherapies. In this sense, addressing the gut microbiota composition could offer a new therapeutic option to combat alcohol-induced neuroinflammation and toxicity.
Previous studies in Orio’s laboratory showed that pharmacological pretreatment with oleoylethanolamide (OEA) before each binge episode prevents the alcohol-induced harmful effects on the gut–brain axis. OEA showed potent anti-inflammatory, antioxidant, and neuroprotective actions by blockade of Toll-like receptors (TLR)-4 and its agonists in the frontal cortex of rats. For example, 1) OEA ameliorates LPS-induced neuroinflammation in the frontal cortex and modulates motivated behaviors such as anhedonia (Sayd et al., 2015) and 2) OEA reduces alcohol binge-induced neuroinflammation in the frontal cortex and depressive-like behavior during abstinence (Antón et al., 2017). In Antón et al. (2018), we showed that OEA is able to reduce the leakage of bacterial components such as LPS (a TLR-4 agonist) from the gut to the blood and even the passage of whole bacteria to the MLNs, since it prevents alcohol-induced gut inflammation and decreases tight junction (TJ) proteins of the colonic barrier (Antón et al., 2018). These findings provide an exciting evidence of the participation of OEA in the dysregulation of the gut–brain axis induced by alcohol. However, the role of OEA in the modulation of microbiota composition, as part of the mentioned microbiota–gut–brain axis, has not been studied yet in the context of alcohol abuse.
Independently, Passani’s and de Filippo’s laboratories studied the effect of OEA administration on microbiota profile in healthy mice, showing that OEA changes the gut microbiota composition, expanding the microbial diversity, increasing Bacteroidetes, and decreasing Firmicutes, which is very similar to a lean-like phenotype fed with a fiber-rich diet (Di Paola et al., 2018). Thus, in the present study, we joined the expertise of the three previously mentioned research groups, to ascertain the specific effects of OEA pretreatment in the gut microbiota profile in the context of alcohol binge drinking consumption.
Materials and Methods
Animals
Forty-two young adult male Wistar rats (Envigo®, Barcelona, Spain) 8–9 weeks old, weighing around 160–220 g, were used. Rats were housed in cages in groups of three to four rats with a reverse 12-h light/dark cycle under standard temperature and humidity at the SPF Animal Care Facility of Complutense University of Madrid. Animals were allowed free access to standard food (A04 SAFE, Scientific Animal Food and Engineering, Augy, France) and tap water. Rats were maintained and daily surveilled for 10 days under constant conditions before the experiments. All studies were designed in compliance with the ARRIVE guidelines (Kilkenny et al., 2010) and adhered to the guidelines of the Animal Welfare Committee of Complutense University of Madrid (reference: PROEX 420/15) according to European legislation (2010/63/UE). The study groups were as follows: experimental groups: T0 rats (before any treatment) and T1 groups (after treatments): water + vehicle group, water + oral/i.p. OEA group, and alcohol + oral/i.p. OEA group.
Alcohol Binge Intoxications
Alcohol (30%, v/v) or vehicle (tap water) was intragastrically administered with specific cannulae (16G needle, Fisher Scientific, Waltham, MA, USA) at a maximum of three times/day during 4 consecutive days, following a standard paradigm of alcohol binge drinking (Obernier et al., 2002; Antón et al., 2017; Antón et al., 2018). The doses were titrated by blood alcohol levels (BAL) and by the observed behavioral signs related to alcohol intoxication (Antón et al., 2017; Antón et al., 2018). The average dose of alcohol per rat was 8.30 g/kg/day in this experiment.
The number of rats per group was initially higher in the alcohol-fed groups, since this protocol often induces mortality. In this experiment, the mortality was around 8%, similar to other studies (Obernier et al., 2002; Antón et al., 2017; Antón et al., 2018).
Drugs
OEA was synthesized as described in Giuffrida et al. (2000). OEA i.p. was dissolved in 5% Tween 80 in saline (vehicle) and administered at 5 mg/kg i.p., 10 min before the alcohol binges, except for the loading initial dose that was 10 mg/kg. Control rats were injected with vehicle i.p. This protocol of OEA administration has been proven to reduce binge drinking-induced peripheral inflammation and neuroinflammation (Antón et al., 2017; Antón et al., 2018).
OEA orally administered was dissolved in 1% carboxymethylcellulose in saline and administered at 20 mg/kg i.g. (3 ml/kg) 20 min before each alcohol gavage with specific cannulae (16G needle, Fisher Scientific, Waltham, MA, USA). This dose of OEA oral administration has been previously shown to prevent the binge drinking-induced bacterial translocation from the intestinal lumen to other organs, such as the MLNs, as well as the passage of LPS to the systemic blood (Antón et al., 2018).
BAL Determination
Blood samples were collected (20 µl) from the rat tail 2 h after the second alcohol administration every experimental day (at 3 p.m.). BAL were measured by enzymatic reaction using electrochemical detection with AM1 Alcohol Analyzer (Analox Instruments, London, UK). This protocol of alcohol binge drinking led to a sedation/ataxia behavior and relatively constant intoxicating BAL (average alcohol levels/day: 252.42 ± 165.58 mg/dl).
Fecal Sample Collection and Bacterial Genomic DNA Extraction
Feces were collected in sterile conditions before treatments (T0) and at the end of the 4-day protocol, 3 h after the last alcohol/water administration (T1), and stored at −80°C until nucleic acid extraction. Bacterial genomic DNA was extracted using DNeasy PowerLyzer PowerSoil Kit (Qiagen®, Hilden, Germany) following the instruction of the manufacturer. DNA quality was examined by gel electrophoresis.
16S Ribosomal DNA Sequencing
For microbiota analysis, the V3–V4 hypervariable regions of the bacterial 16S rRNA gene were amplified using a specific primer set (341F: 5′-CCTACGGGNGGCWGCAG-3′ and 805r: 5′-GACTACNVGGGTWTCTAATCC-3′) (Takahashi et al., 2014). Sequencing was performed with Illumina MiSeq (Illumina, San Diego, CA, USA) with V3 chemistry (600 cycles) with a PE 2X300 protocol at the IGA Technology Services (Udine, Italy) following their internal protocol. Raw reads data are available at the European Nucleotide Archive (ENA) database under accession number PRJEB44965.
Sequencing Data Processing and Statistical Analysis
Similar to previous reports (Butera et al., 2020; Tamburini et al., 2020), the obtained sequence libraries were pretreated by removing sequencing primer with CUTADAPT (Martin, 2011) and the low-quality ends at 5′ by using Sickle (Joshi and Fass, 2011) with a quality cutoff of 20. Pretreated sequences were then analyzed using MICCA v 1.7.2 (Albanese et al., 2015) to perform joining of forward and reverse reads and to perform amplicon sequence variance (ASV) picking using the UNOISE3 algorithm (Edgar, 2016). Taxonomy assignation to representative sequences of the identified ASVs was performed using RDP classifier and database v. 2.11 (Wang et al., 2007). Data were then analyzed using R software with a variety of dedicated packages. Phyloseq v.1.28.0 (McMurdie and Holmes, 2013) was used to import and handle ASV table and taxonomy files obtained through MICCA as well as to calculate PCoA ordination analysis (using the Bray–Curtis distance measure). Rarefaction to the smallest library size was used to normalize ASV counts prior to α-diversity analysis, which was performed using the microbiome package v 1.6.0 (Lahti et al., 2017). Other analyses were performed on the cumulative sum scaling (CSS transform, followed by log2 scaling) normalized ASV count table, as implemented in the metagenomeSeq version 1.26.3 (Paulson et al., 2013). PERMANOVA analysis was performed using the vegan package and using 9,999 permutations, and comparisons in α-diversity analysis were performed with the Wilcoxon rank-sum test. To identify plausible biomarkers of sample classes, we used linear discriminant analysis effect size (LEfSe) (Segata et al., 2011) on the CSS-transformed abundances. Network inferences were obtained with SPIEC-EASI (SParse InversE Covariance Estimation for Ecological Association Inference) (Kurtz et al., 2015) using the untransformed ASV count table. This method does not imply correlation analysis to construct microbiota networks; nevertheless, a connection between two nodes (i.e., an edge of the network) denotes a significant linear relationship between the abundance of two ASVs (i.e., nodes of the network). The microbiota interaction network was plotted with the igraph (Csardi and Nepusz, 2006) package.
Results
Effects of Alcohol Binges on Gut Microbiota Profile
We firstly aimed at assessing the effects of alcohol binge drinking on the gut microbiota. To do so, analysis was focused on the subset of animals that did not receive OEA pretreatment, and the effects in the alcohol groups were checked at two time points: before (T0) and after (T1) alcohol binge protocol. Diversity analysis results (reported in Figure 1) suggest that alcohol binge drinking had a major effect on the fecal microbiota. Alcohol lowered the richness of the community, which otherwise is significantly increasing from T0 to T1 in the control group (Figure 1A), but did not significantly affect the evenness nor the Shannon index (Figures 1B, C). In addition, alcohol caused a clearly distinguishable community composition in PCoA ordination analysis, with the alcohol-treated samples forming a distinct cluster along the first ordination axis, significantly different with respect to other samples (Figure 1D, see PERMANOVA reported as notation on the ordination graph).
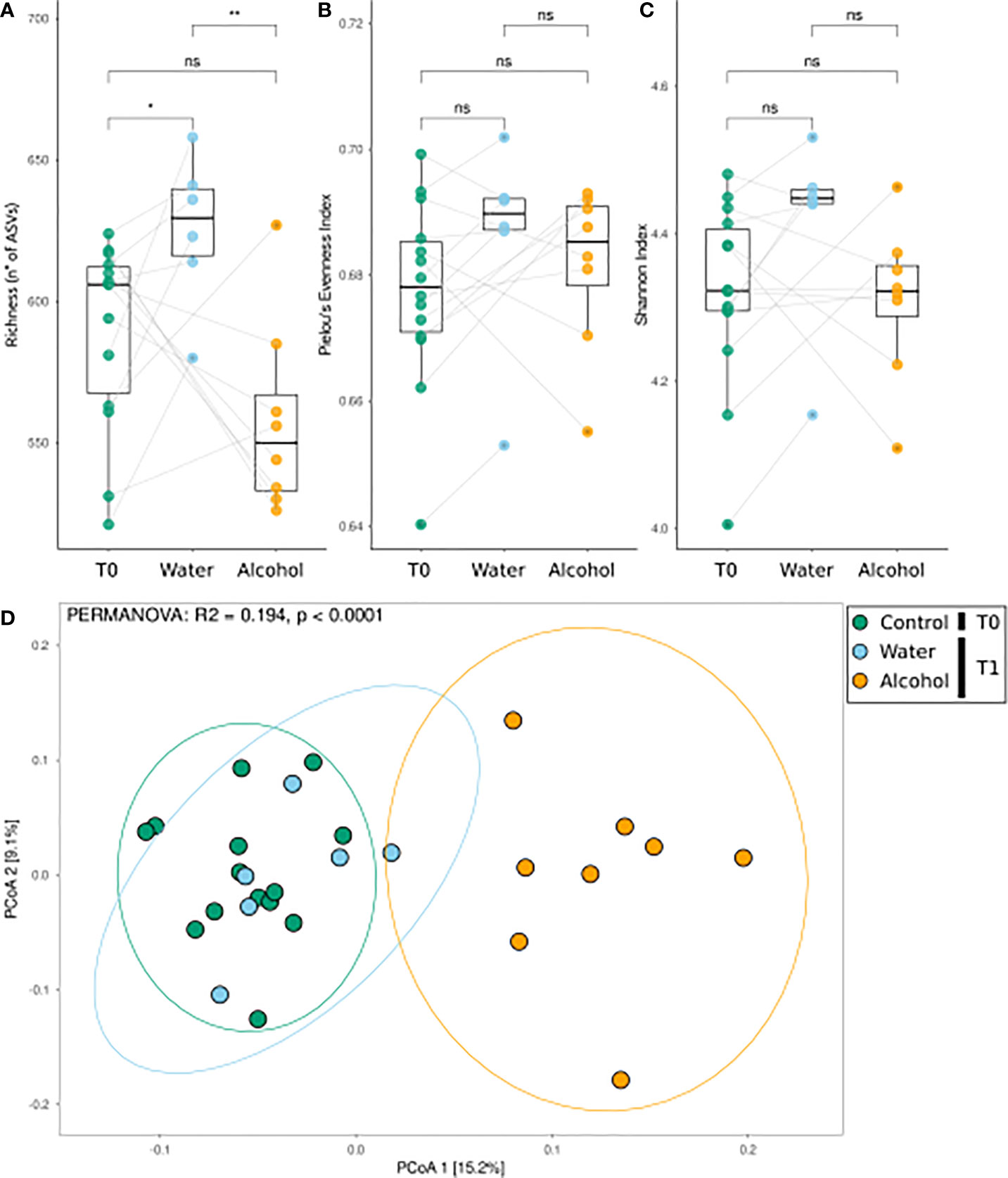
Figure 1 Bacterial community response to alcohol binge drinking. Analyses were performed on the non-OEA-treated sample subset, including the T1 alcohol- and water-administered rats, and the corresponding T0 samples. (A) Richness [number of different amplicon sequence variances (ASVs)], (B) Pielou’s evenness index (equitability of the distribution of relative abundances among ASVs), and (C) Shannon index (diversity of the community) as measures of α-diversity. Differences between groups were tested by the Wilcoxon rank-sum test and reported as notation on the plot (ns, p-value > 0.05; *p-value < 0.05; **p-value < 0.01). Dotted lines connect the data from the same animal at the two time points for each treatment. (D) β-Diversity analysis with PCoA ordination using the Bray–Curtis distance measure. T0 group, n = 14; water group, n = 6; alcohol group, n = 8. Notation above the plots reports the result of the PERMANOVA test.
As further confirmation of the strong effect of alcohol binge drinking on the fecal microbiota, we observed a high number of different genera at significantly different relative abundance values among the three sample groups (see Supplementary Figure 1 for an overall analysis of genera which significantly changed among the three groups, as tested by one-way ANOVA analysis).
LEfSe analysis (Figure 2) was used to discover biomarkers associated to each of the sample groups. To identify those most likely linked to a biological effect, we inspected their relative abundance (i.e., biomarkers with very low median relative abundance in a class are less indicative of a biological effect connected to that class) as well as their frequency distribution (i.e., those markers found in all samples of a class can be considered more likely connected to a biological effect, than those found in only few samples of a class). By doing so, we identify Clostridium sensu stricto, Romboutsia, Turicibacter, Bifidobacterium, Parabacteroides, and Enterococcus as valuable markers for the T1 alcohol group (Supplementary Figure 2), and these were found to have high relative abundance levels in ethanol-administrated rats. Conversely, Lactobacillus, Clostridium XIVa, Clostridium XIVb, and Oscillibacter were found as markers of T0 samples and displayed a significantly decreasing trend in relative abundance from T0 to T1 (Supplementary Figure 1). As such, they could be considered as markers of the normal weaning process. However, given that their decrease in relative abundance was more evident in ethanol-administrated rats, we can attribute it to a specific effect of ethanol on these genera.
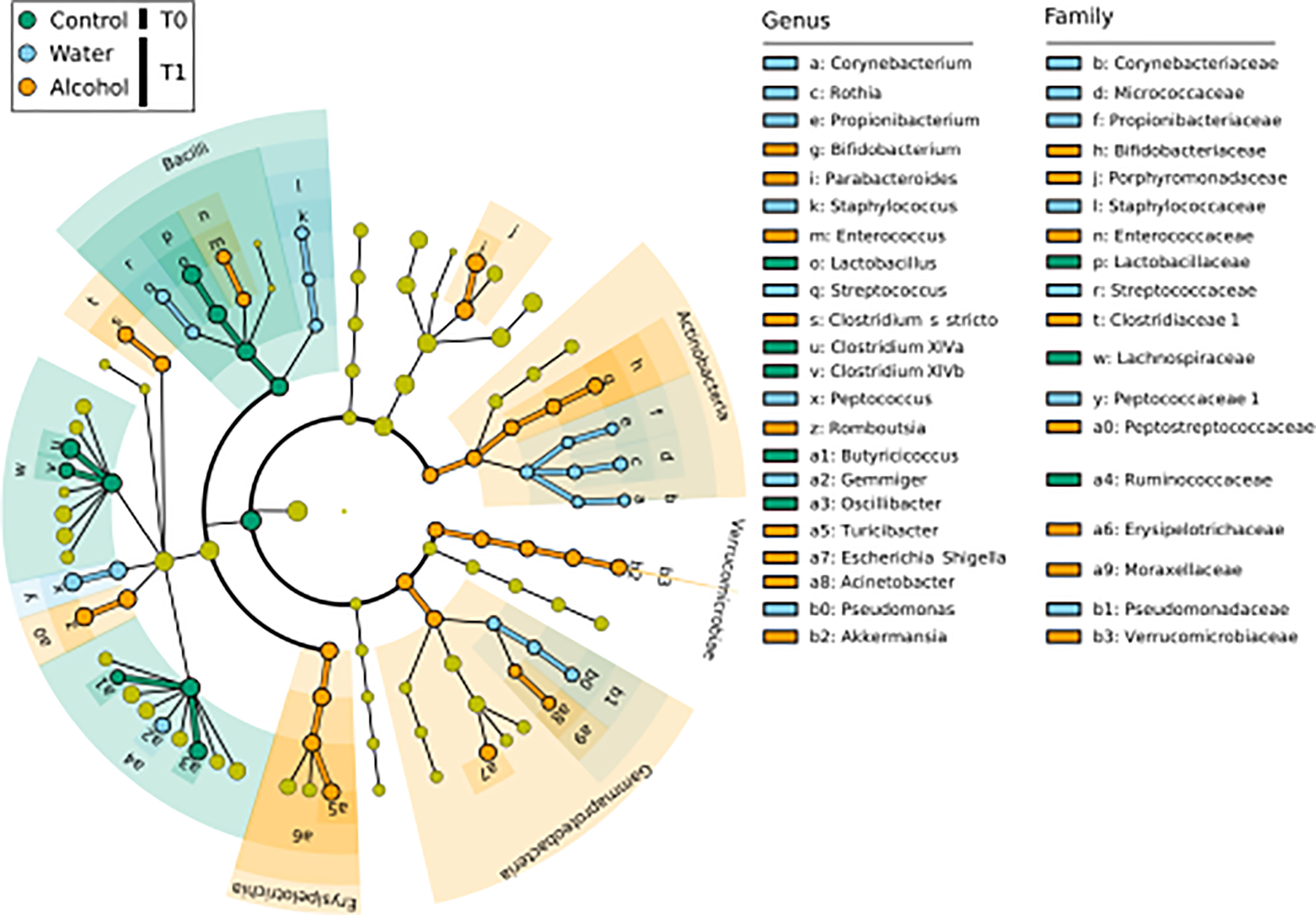
Figure 2 LEfSe biomarker identification for the comparison of genera relative abundance between the T1 ethanol-administered rats, the T1 water-administered rats, and the corresponding T0 samples. Significant biomarker taxon for each group was obtained with a Kruskal–Wallis test among classes (α = 0.05], and only biomarkers with a logarithmic linear discriminant analysis score higher than 2.0 were retained. T0 group, n = 14; water group, n = 6; alcohol group, n = 8.
Effects of OEA Treatment on Gut Microbiota Profile
After assessing the overall effects of alcohol binge drinking on fecal microbiota, we aimed at analyzing the specific effect of OEA administration per se and in the context of alcohol binge drinking. Figure 3 reports microbiota α- and β-diversity analysis on all sample sets. Higher richness values were observed in the groups of rats receiving water and vehicle, showing no significant difference with respect to the groups of rats receiving water and OEA, but with a significant difference with respect to samples collected at T0 and to the alcohol-receiving rats (both vehicle- and OEA-treated ones). Alternatively, the lowest richness values were observed in the group of rats receiving alcohol and OEA (Figure 3A), with a statistically significant difference versus all the other sample groups. The evenness and Shannon indices showed similar trends as both were increased from T0 to T1 except for the group of rats receiving alcohol and OEA, which showed significantly lower values with respect to all other T1 samples and, interestingly, similar to those observed in T0 samples (untreated animals) (Figures 3B, C).
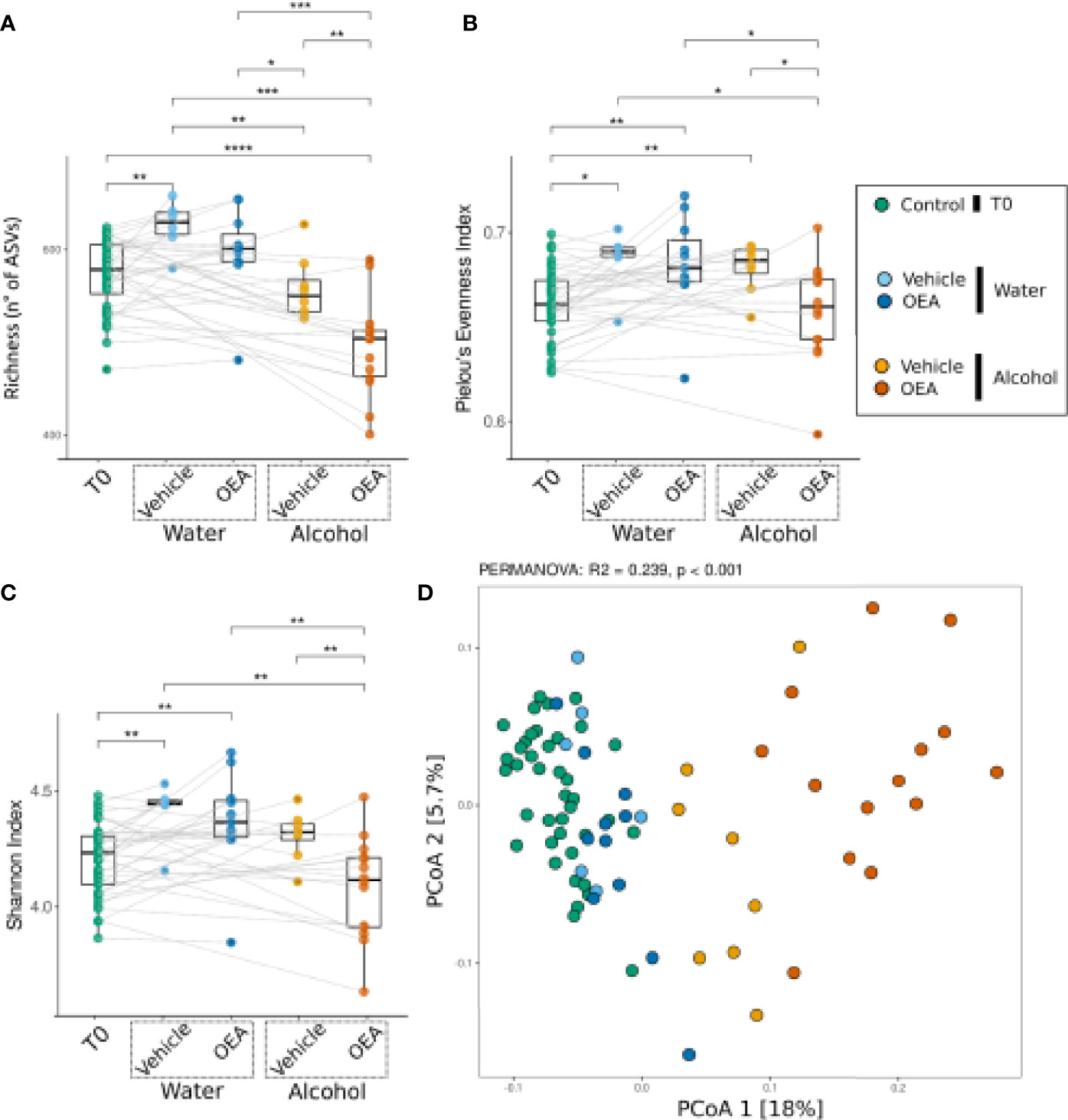
Figure 3 Bacterial community response [α-diversity: (A–C); β-diversity: (D)] to alcohol binge drinking and oleoylethanolamide (OEA) treatment. (A) Richness (number of different ASVs), (B) Pielou’s evenness index (equitability of the distribution of relative abundances among ASVs), and (C) Shannon index (diversity of the community) as measures of α-diversity. Differences between groups were tested by Wilcoxon rank-sum test and reported as notation on the plot (*p-value < 0.05; **p-value < 0.01, ***p-value < 0.001, ****p-value < 0.0001). Dotted lines connect the data from the same animal at the two time points for each treatment. (D) β-Diversity analysis with PCoA ordination among all samples, using the Bray–Curtis distance measure. Notation above the plots reports the result of the PERMANOVA test. T0 group, n = 40; water + vehicle group, n = 6; water + OEA group, n = 11; alcohol + vehicle group, n = 8; alcohol + OEA group, n = 14. Notation above the plots reports the result of the PERMANOVA test. Dotted lines connect the data from the same animal at the two time points for each treatment.
β-Diversity analysis (Figure 3D) indicated that the community was significantly different in the groups, as confirmed by PERMANOVA results (see notation above the ordination plot in Figure 3D). As previously observed, water samples overlap with T0 samples (pairwise PERMANOVA between T0 and water samples were significant but showed low R2 values; T0 vs. water + vehicle: R2 = 0.055, p-value: 0.001; T0 vs. water + OEA: R2 = 0.062, p-value: 0.001), showing a separation along the second ordination axis between the vehicle- and OEA-treated groups (pairwise PERMANOVA: water + vehicle vs. water + OEA: R2 = 0.098, p-value: 0.024). Regarding alcohol-treated samples (Figure 3D), they are clearly separated from the others along the first ordination axis (representing 18% of total data variance), also showing a difference between OEA and vehicle treatment (pairwise PERMANOVA: alcohol + vehicle vs. alcohol + OEA: R2 = 0.142, p-value: 0.001).
To further assess the effects of alcohol binge drinking and the effect of OEA administration in the gut microbiota, we used network analysis to evaluate if the complex interactions between ASVs in each group of samples were different (Figure 4 and Table 1). Overall, the microbial networks in the vehicle-treated samples were most similar (Figures 4A, C, E). In both water and alcohol groups, the higher frequency of node degree was 4 and both networks were made of a single connected component (Figure 4E, Table 1), with the alcohol network resulting as slightly more complex, displaying a higher number of nodes and edges. On the other hand, OEA deeply affected the microbial network structure both in the water and in the alcohol groups, in an opposite direction. The microbial network in the OEA + water group (Figures 4D, E, Table 1) was the most complex, even in respect to the vehicle networks, with more relations between ASVs (the value of node degree with higher frequency was 5) and with the highest overall number of nodes (ASVs) and edges (significant relations). Notwithstanding, in the alcohol group, OEA administration (Figures 4B, E, Table 1) had a different effect on the microbial community, leading also to its fragmentation (see the number of components in Table 1). In brief, in this network, the value of node degree with higher frequency was 2 and a substantially higher frequency of nodes with node degree equal to 1 or 0 was observed, with respect to the other networks. This means that, in the alcohol + OEA network, 63% of the nodes are linked with only one or two other nodes, whereas 3% of the nodes are not related with any other nodes (unconnected nodes).
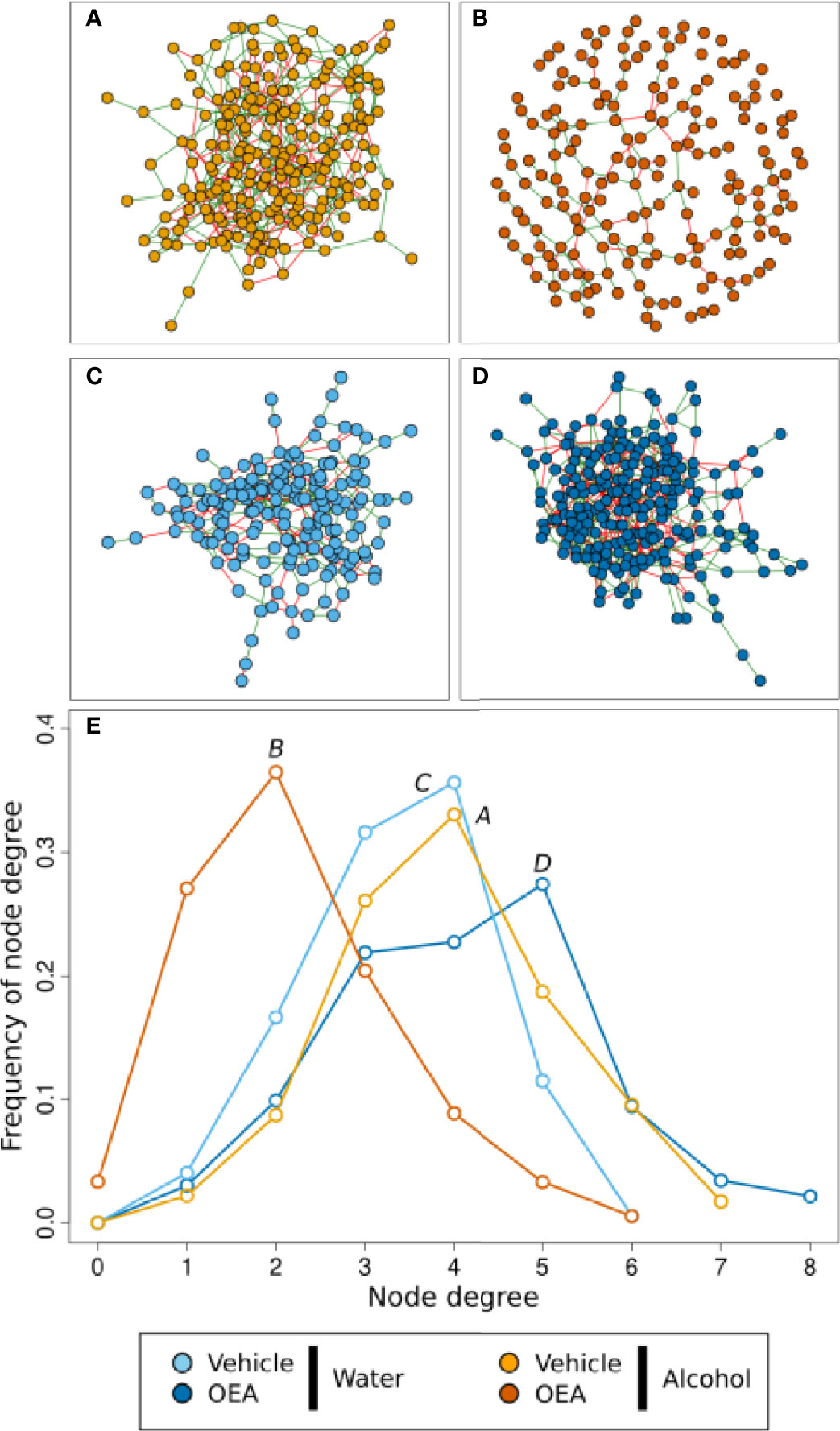
Figure 4 Microbiota association network on the T1 sample subset. (A–D) Graphic representation of inferred networks for each group, as indicated by the color of nodes. The graphical representation of the association network is composed of nodes (i.e., circles) connected by edges (i.e., the segments between nodes). Each node represents an ASV of the community, while each edge represents the presence of a significant association between two ASVs. There is a positive association when edges are green, while there is a negative association when edges are red. (E) Frequency distribution of the node degree statistic of the four inferred networks. In this representation, we report the frequencies (i.e., % of total) of nodes in the network for each value of node degree (i.e., a statistic representing the number of nodes connected to each node). Each network is represented as a different line, color coded as reported in the legend. Water + vehicle group, n = 6; water + OEA group, n = 11; alcohol + vehicle group, n = 8; alcohol + OEA group, n = 14.

Table 1 Properties of the networks reported in Figure 4.
These results suggest that OEA supplementation has a significant different outcome with respect to bacterial community structure and complexity, when combined either with water or alcohol administration. The 10 most abundant genera for each OEA-receiving sample group are reported in Table 2. Samples are ordered based on magnitude of the difference in relative abundance between the water and the alcohol sample groups, with one-way ANOVA to test for statistically significant differences. The genera Bacteroides, Escherichia/Shigella, and Parabacteroides were significantly more abundant (with a difference higher than 5%) in the alcohol group, while Clostridium XIVa, Lactobacillus, and Clostridium IV genera were significantly more abundant (with a difference higher than 5%) in the water group.
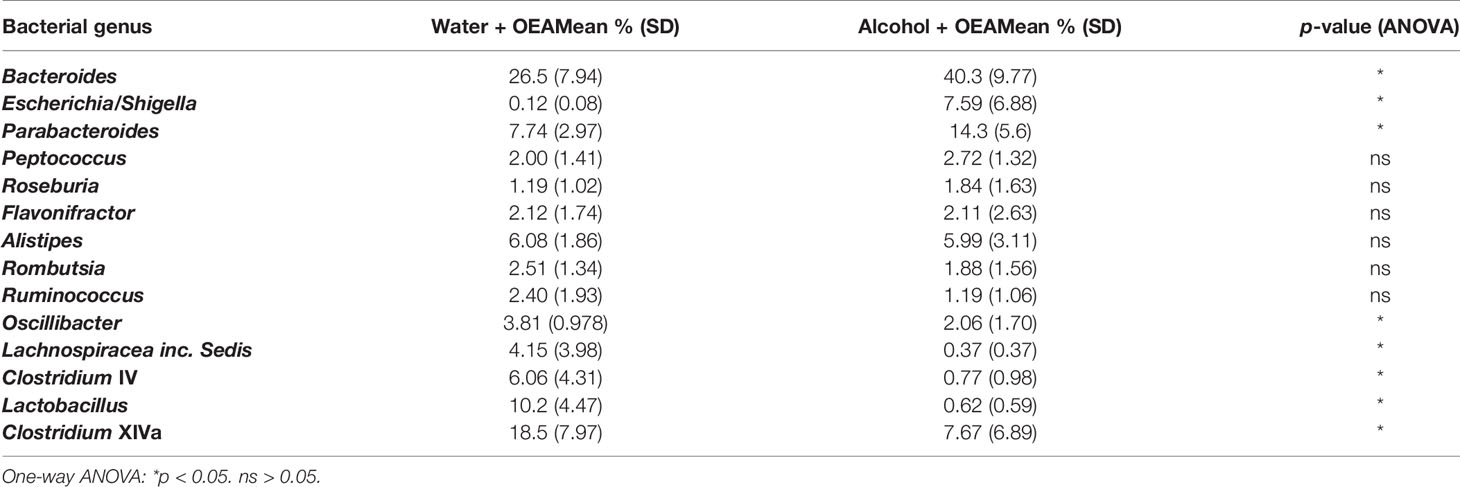
Table 2 The 10 most differentiating genera in OEA-supplemented samples (water + OEA and alcohol + OEA).
We next evaluated the effect of alcohol binge drinking and OEA pretreatment on the community composition at the genus level by identifying taxa found at significant different abundance, using LEfSe analysis. As shown in Figure 5, comparing alcohol-receiving samples with either OEA or vehicle pretreatment, numerous different taxa showed significantly different relative abundance in the two sample classes. Among them, members of the Firmicutes phylum and, in particular, four genera (Butyricicoccus, Clostridium IV, Gemmiger, and Ruminococcus) of the Ruminococcaceae family, as well as the genus Bifidobacterium of the Actinobacteria phylum, were more abundant in the vehicle group. The OEA-treated samples, conversely, had higher relative abundance of taxa among the Proteobacteria (i.e., Escherichia/Shigella and Pseudomonas) and Bacteroidetes (genus Bacteroides) phyla. At the genus levels, Corynebacterium, Staphylococcus, Aerococcus, Enterococcus, Blautia, and Peptococcus were more abundant in the OEA pretreatment group, whereas the genera Bifidobacterium, Clostridium sensu stricto, and Turicibacter were more abundant in the non-OEA pretreatment sample groups.
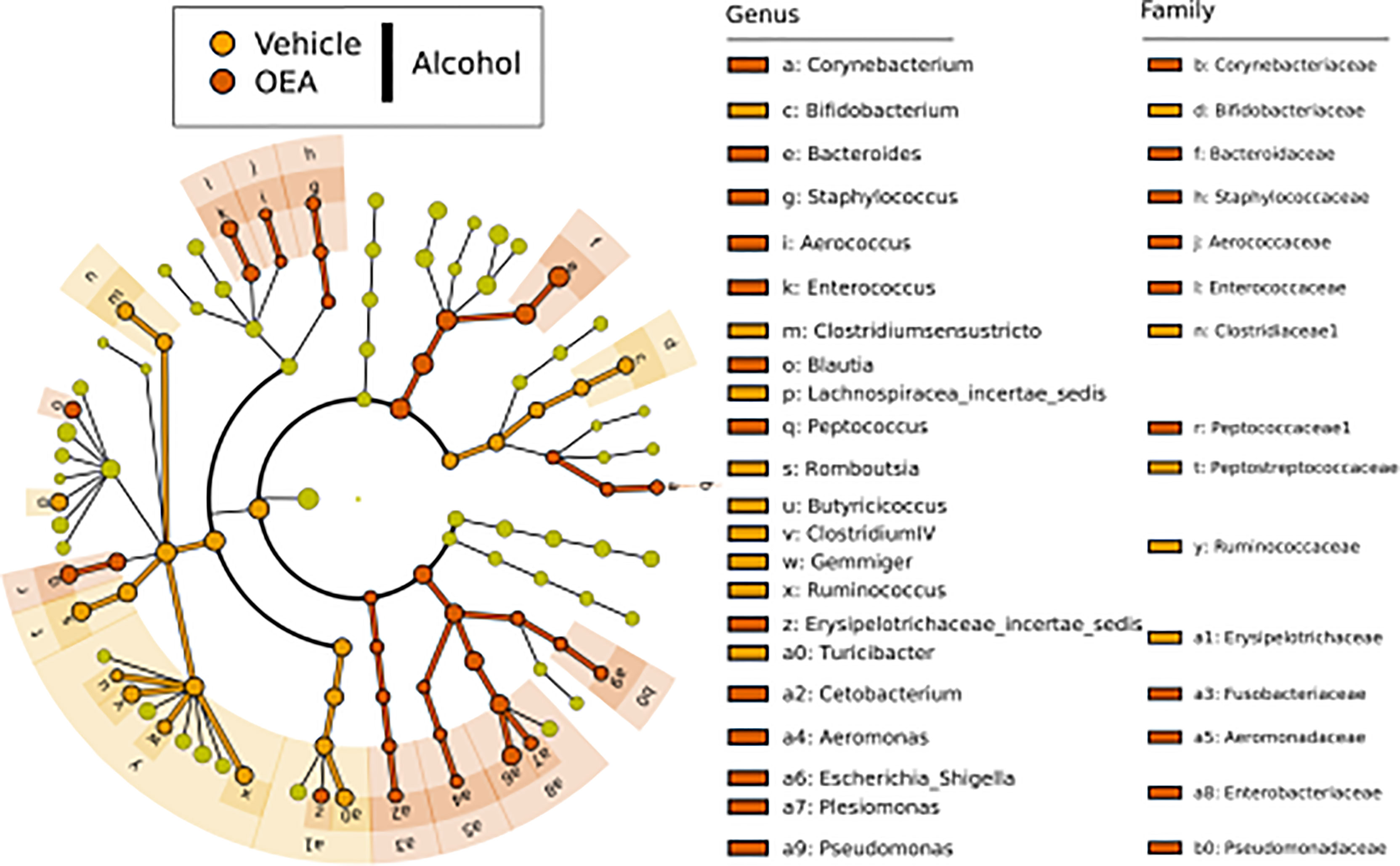
Figure 5 LEfSe biomarker identification for the comparison of genera relative abundance between the OEA-supplemented vs. the non-OEA-supplemented group of alcohol-intoxicated rats. Significant biomarker taxon for each group was obtained with a Kruskal–Wallis test among classes (α = 0.05), and only biomarkers with a logarithmic linear discriminant analysis score higher than 2.0 were retained. Alcohol + vehicle group, n = 8; alcohol + OEA group, n = 14.
Effects of OEA Route of Administration (i.p. vs. Oral) on Gut Microbiota Profile
Having assessed the general effect of OEA on gut microbiota, we finally aimed to discern whether different routes of OEA administration (oral vs. i.p.) had a differential impact on gut microbiota in the context of alcohol binge drinking. Figure 6 reports the diversity analysis on the alcohol-administrated rats receiving oral or i.p. OEA, which are compared with non-OEA alcohol-receiving rats in T1 and with the respective control rats in T0. The separation of samples in the PCoA ordination analysis (Figure 6A) indicated that oral and i.p. OEA administration in alcohol-intoxicated animals had a different effect on the structure of microbiota with respect to the vehicle group (ANOSIM test; R2 = 0.50; p = 1.00e−4). This was then confirmed by the pairwise PERMANOVA, which indicated that all the three groups of samples had a significantly different microbial community between each other. The highest differences were found between the vehicle and the oral OEA (R2 = 0.2322; p = 0.0006) or the i.p. OEA (R2 = 0.1423; p = 0.0021). The difference between the two administration routes was significant (R2 = 0.1367; p = 0.0042), which suggests that the OEA administration route may have a fundamental role in shaping the microbiota in alcohol-treated rats.
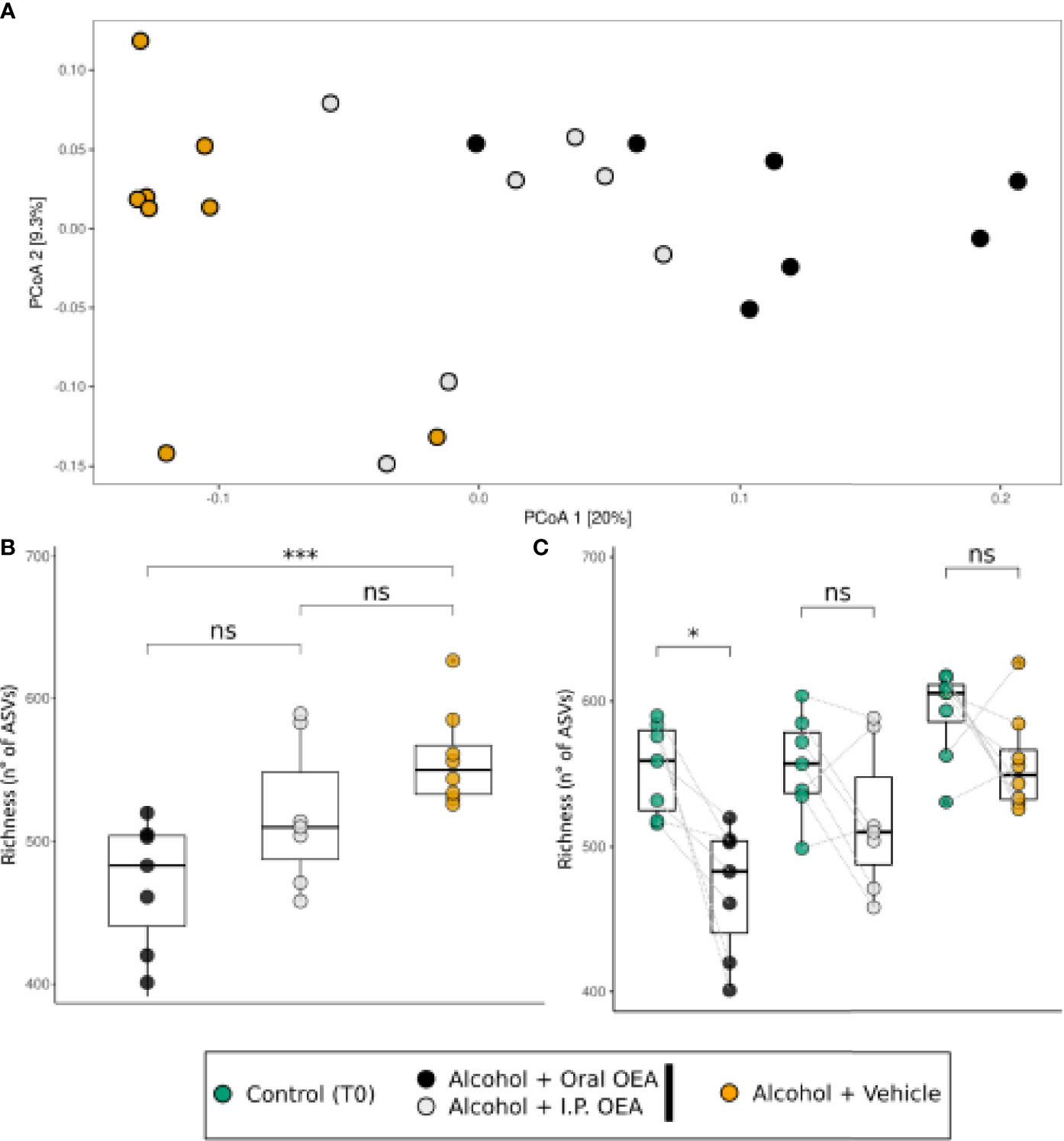
Figure 6 Bacterial community response to different routes of administration of OEA in alcohol-intoxicated rats. (A) β-Diversity analysis with PCoA ordination among the oral and i.p. OEA administration route, in comparison with the non-OEA-receiving rats. Ordination was constructed using the Bray–Curtis distance measure. (B) Richness (number of different ASVs) in the three sample groups. (C) Richness (number of different ASVs) in the three sample groups, in comparison with their respective T0 samples. Differences between groups were tested by Wilcoxon rank-sum test [paired test in panel (C)] and the resulting p-value was reported as notation on the plot (ns, p-value > 0.05; *p-value < 0.05; ***p-value < 0.001). Dotted lines connect the data from the same animal at the two time points for each treatment. T0 group, n = 22; alcohol + vehicle group, n = 8; alcohol + oral OEA group, n = 7; alcohol + i.p. OEA group, n = 7.
A similar finding can be observed also for the α-diversity measure of ASV richness (Figure 6B). The richness index appears to decrease in the i.p. and oral OEA in comparison with the vehicle group, but the only significant difference was found with respect to the oral OEA. Thus, oral OEA is also the only one showing a significant decrease in richness from T0 to T1 (Figure 6C).
We further assessed the different effects of oral and i.p. OEA in the alcohol-administered group by evaluating microbiota interactions using network analysis (Figure 7). The microbial network induced by i.p. OEA pretreatment (Figure 7A) is distinguishable from the one induced by oral OEA pretreatment (Figure 7B). The i.p. OEA network was sparser, with roughly 19 nodes less than the oral OEA one, but with almost half the edges (142 vs. 246) (Table 3). Consequently, the network has a high number of non-interconnected node modules, composed of 13 different components and a frequency distribution of node degree statistic dramatically skewed toward low values (Figure 7C and Table 3). Interestingly, the same effect was not found for the sample group with water administration (Figure 7C), in which there were little differences between i.p. and oral OEA administration. This difference is likely not linked to general ASV richness reduction (i.e., less total ASVs in the community would have less interactions and less nodes); as in Figure 6, we showed an opposite trend (higher richness in i.p. OEA with respect to oral OEA with no significant differences).
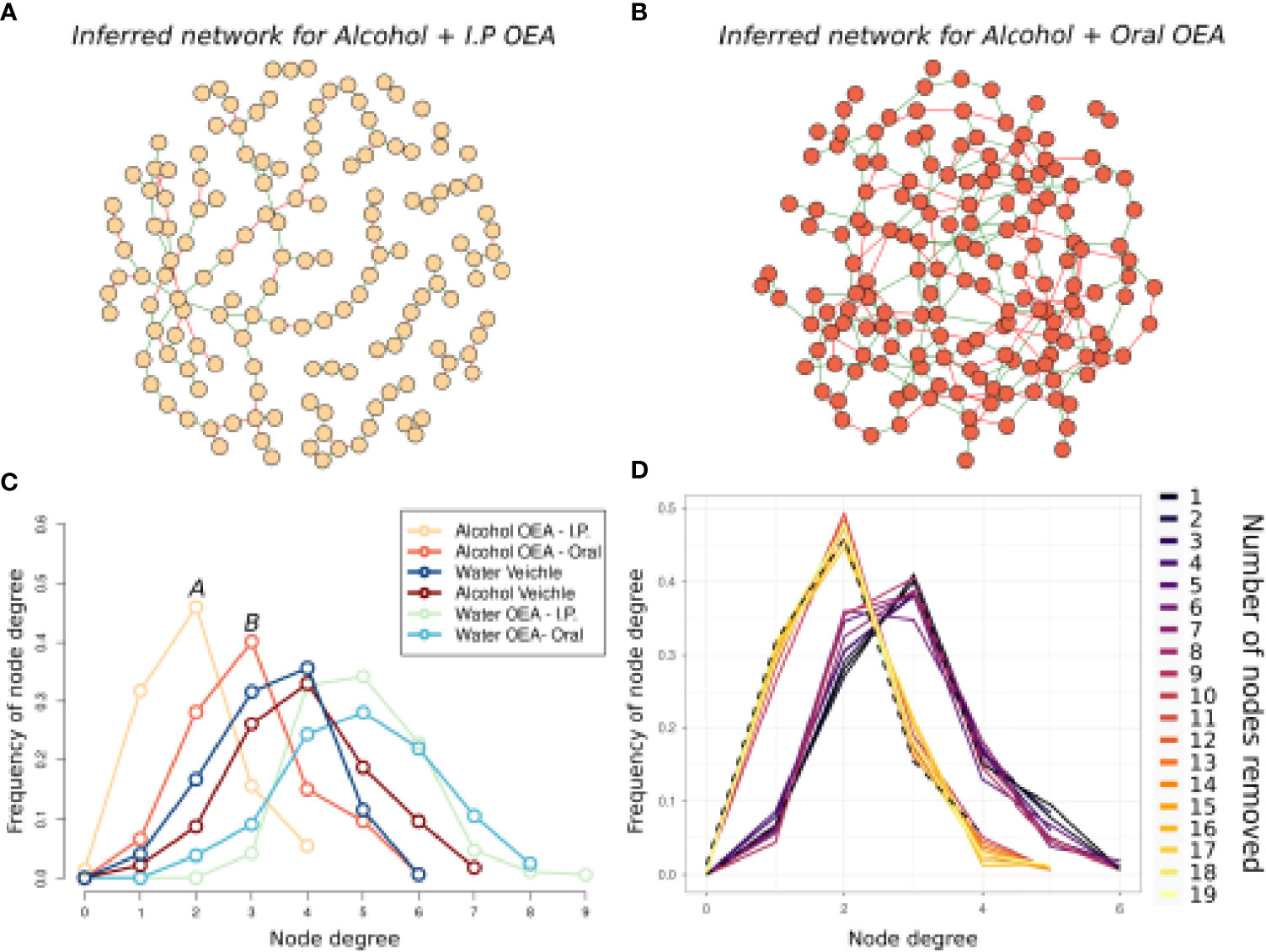
Figure 7 Microbiota association network on the alcohol-intoxicated samples, comparing the oral and i.p. administration routes of OEA. Graphic representation of inferred networks for the i.p. OEA (A) and oral OEA (B)-receiving rat groups, as indicated by the color of nodes and title. The graphical representation of the association network is composed of nodes (i.e., circles) connected by edges (i.e., the segments between nodes). Each node represents an ASV of the community, while each edge represents the presence of a significant association between two ASVs; there is a positive association when edges are green, while there is a negative association when edges are red. (C) Frequency distribution of the node degree statistic of all the inferred networks. In this representation, we report the frequencies (i.e., % of total) of nodes in the network for each value of node degree (i.e., a statistic representing the number of nodes connected to each node). Each network is represented as a different line, color coded as reported in the legend. (D) Frequency distribution of the node degree statistic in the oral OEA network, during the “attack” on node betweenness. This is graphically represented as in (C), but in this case, a single line for each iteration of the test is represented, and they all represent the oral OEA network from which a node is removed every iteration. Dotted lines represent i) the starting frequency distribution of the “undisturbed” oral OEA network (on the right) and ii) the frequency distribution of the i.p. OEA network. Each line represents the frequency distribution of node degree of a different network, reobtained after removal of one node (and recalculation) of the previous network. After the removal of 11 nodes, the frequency distribution of node degree in the oral OEA community resembles well the frequency distribution of the node degree in the i.p. OEA community. Alcohol + oral OEA group, n = 7; alcohol + i.p. OEA group, n = 7.

Table 3 Properties of the networks reported in Figure 7.
To elucidate possible mechanisms, we simulated the changes between the i.p. and oral networks by gradually removing 19 ASVs from the data (i.e., the difference in total nodes between the two networks) of the oral OEA samples, recalculating the network, and measuring the frequency distribution of node degree statistic (Figure 7D and Supplementary Figure 3). We simulated two mechanisms of community reassembly following i.p. OEA administration: a random loss of ASVs (Supplementary Figure 3) and a “targeted” loss of ASVs in decreasing order of node betweenness (i.e., in decreasing importance for the network structure). We were able to reproduce the same frequency distribution of node degree observed for the i.p. network, by the removal of 11 ASVs in decreasing betweenness order in the community of the oral sample, while we did not always reproduce the same distribution when nodes were randomly removed (in 20 repeated simulations). These results indicate that the difference between the community in the oral and i.p. sample groups seems to be at the single ASV level, likely originating from the loss of some specific and highly interacting ASVs and not from random ASV loss.
Discussion
In this paper, we describe the changes that alcohol binge drinking promotes in the gut microbiota during a 4-day protocol versus control (water) animals and the influence of i.p. and oral OEA administration in healthy conditions and in alcohol-induced dysbiosis.
Our results indicate that alcohol binge drinking reduces the bacterial α-diversity (richness of ASVs) and creates a clearly distinguishable community in ordination analysis (different β-diversity), with main differences observed from T0 (no treatment) to T1 (last day of binge) in the binge drinking group only. Also, alcohol binge drinking promotes some bacterial community compositional differences at the genus and family levels. Remarkably, alcohol binge drinking promotes a significant decrease in Lactobacillus, Clostridium, and Oscillibacter genera and the increment of Bifidobacterium, Turicibacter, Parabacteroides, and Romboutsia. An alcohol-induced decrement of Lactobacillus was previously reported in animal models using different patterns of alcohol consumption (Yan et al., 2011; Hartmann et al., 2013; Xiao et al., 2018; Kosnicki et al., 2019; Lee et al., 2019; Lamas-paz et al., 2020). Regarding α- and β-diversity, other studies using different alcohol models have obtained diverging results, some showing alcohol-induced reduction in α-diversity and/or changes in β-diversity (Mutlu et al., 2009; Bull-Otterson et al., 2013; Lee et al., 2019; Samuelson et al., 2019; Zallar et al., 2019) and others without significant changes in α- or β-diversity (Neyrinck et al., 2016; Reyes et al., 2020). At the time of the present study, the specific impact of alcohol on gut microbiota when consumed in a binge drinking pattern remained elusive. A recent study has shown that mice under a drinking in the dark (DID) alcohol binge model neither show changes in α-diversity (Shannon, Simpson, and Chao1 indices) or β-diversity nor show significant changes in bacteria community composition at the phylum or family level (Reyes et al., 2020). As mentioned before, in the present study, we observed alterations in both α- and β-diversity with a 4-day alcohol binge drinking protocol in rats. Differences in the protocol and/or the animal species may account for these discrepancies.
It is very interesting to note that changes in bacterial composition have been observed in different neurological disorders, although their causal roles remain elusive, and observations often remain limited to a correlational level. For example, a reduced abundance of Bifidobacterium and an increment of Clostridia were observed in autism, while a reduced abundance of Bifidobacterium and Lactobacillus was observed in depression (reviewed in Cryan et al., 2019). Additionally, the use of psychobiotics (probiotics that when consumed promote a beneficial effect on mental health; Dinan et al., 2013) has been shown to improve concentration and decrease anxiety and depression symptomatology both in preclinical and clinical studies (reviewed in Scriven et al., 2018). The specific consequences of alcohol binge-induced dysbiosis presented in this study are at present unknown as our results are descriptive and, thus, do not allow for mechanistic speculation about possible implications of these bacterial changes in a healthy status.
Previous experiments in Orio’s lab indicated that OEA pretreatment before each alcohol gavage has protective actions against multiple alcohol-induced effects, such as neuroinflammation, leaky gut, anhedonia, or depressive-like behavior in abstinence (Sayd et al., 2015; Antón et al., 2017; Antón et al., 2018; Orio et al., 2019; Orio, 2020). The actions of OEA were particularly interesting in the gut, since this biolipid was able to strongly reduce gut inflammation and colonic TJ disruption, providing protection against the bacteria translocation and also reducing alcohol-induced alterations in the immune system. Here, we aimed to fully characterize the actions of OEA in the gut by studying its impact on microbiota in the context of binge drinking. We hypothesized that some of the protective actions of OEA in the gut–brain axis could be mediated by amelioration of alcohol-induced gut dysbiosis. Results obtained in the present study are intriguing, since the effects of OEA significantly differ when this biolipid is administered in control or in alcohol-treated animals. Surprisingly, pretreatment with OEA in the alcohol-administered rats decreased the α-diversity by a reduction in evenness and Shannon indices, as well as changed β-diversity. Additionally, OEA pretreatment induced major changes in Bacteroides, Clostridium, Escherichia/Shigella, and Rombustia genera and promoted a fragmentation of the microbial network in the alcohol group. The latter evidence can be interpreted as indicative of a detrimental effect of the administration of OEA in conjunction with alcohol treatment. In fact, a more complex interaction network (i.e., with a frequency distribution skewed toward a higher degree) is indicative of a more diverse and more functional community in the intestinal environment, whereas shorter average path length values can be interpreted as increasing the speed at which the network responds to perturbations (Faust and Raes, 2012). It is to note that the α-diversity indices in the combined OEA and alcohol group are more similar to untreated rats (T0) than any other groups.
Nevertheless, the effect of OEA in physiological conditions (no administration of alcohol) appears to be different, showing no changes in α-diversity and only a slight change in β-diversity. At the community composition level, OEA increased Bacteroides and reduced Lactobacillus, among other genera. These results agree with our previous results using 10 mg/kg i.p. OEA during 11 consecutive days (Di Paola et al., 2018). In the mentioned paper, OEA increased Bacteroidetes phylum (Bacteroides) and decreased Firmicutes (Lactobacillus) and changed β-diversity; OEA, though, did not induce significant changes in α-diversity (Di Paola et al., 2018). As commented in the discussion of the cited paper, these results suggest that OEA treatment promotes a shift in gut microbiota toward a “lean-like phenotype”, mimicking the effects of a low-fat and high-polysaccharide/fiber-rich diet. Intriguingly, contrary to what was previously observed, OEA treatment without alcohol administration led to opposite changes in the microbial interaction network, which could be interpreted as positive. OEA treatment in this case increased the node degree value with higher frequency, skewing its distribution to the right, and increased total nodes and edges in comparison with the control group.
These results suggest that OEA may ameliorate the balance of gut bacteria in physiological conditions, but not under alcohol intoxications. Although unexpected, those results may also indicate that the beneficial effects of OEA pretreatment in the context of alcohol binge drinking are not mediated by changes in gut bacterial composition. Whether bacteria change their activity in different settings (with and without alcohol or its products, in conjunction to OEA pretreatment or not) is for us at present unknown. Other mechanisms, such as reduction of colonic inflammation, tight junction disruption and bacterial translocation, regulation of the immune system, antioxidant actions, and/or reduction of neuroinflammation, have been proposed to explain the protective actions of OEA in alcohol binge (Orio et al., 2019; Orio, 2020; Rodriguez-Gonzalez and Orio, 2020).
It is intriguing that the water group varies from T0 to T1. The reasons for the α-diversity changes from T0 to T1 in this control group are not fully understood. It has been suggested elsewhere that they could reflect the normal aging of animals (Flemer et al., 2017), although our experiment was too short to question this hypothesis. It is to note that the present experiment was designed to depict the specific effects of OEA in gut microbiota, and because of that, all animals in T1, including the water group, were injected with vehicle or with OEA. This means that, with this experimental design, we cannot discard the effect of the vehicle in the water-administered animals. Indeed, significant effects of vehicle in gut microbiota were found in some studies using long-lasting oral treatments, although the effects were considered detrimental. For example, continued oral administration of 1% Tween 80 or carboxymethylcellulose, as dietary emulsifiers, induced microbiota alterations and changed β-diversity when given in drinking water for 12 weeks (Chassaing et al., 2015; Holder et al., 2019). Our experiment was designed to question the specific effects of OEA in gut microbiota, and although the time of treatments was shorter, our experimental design did not allow to discard or confirm any vehicle effect.
Another interesting finding of this study is that the route of administration of OEA may affect differently the gut microbiota. Results indicate that oral OEA, but not i.p. OEA, significantly reduced richness in comparison with the vehicle + alcohol group, as well as oral and i.p. OEA differently changed bacterial community structure with respect to the mentioned group. Although the biological significance of many of the bacterial changes observed in this study is still unknown, we observed that i.p. OEA administration resulted in a more fragmented network with more non-interconnected nodes, suggesting a more detrimental effect on the microbial network with respect to oral administration. This difference in network community may be due to the loss of some specific highly interacting ASVs. It is to note that we previously observed differences between oral and i.p. OEA pretreatment in the context of alcohol binge drinking using the same animal model. As mentioned before, we showed that i.p. OEA was able to prevent the alcohol binge drinking-induced neuroinflammation in the frontal cortex, colonic inflammation, and decrement of gut tight junction proteins. However, oral OEA resulted to be more effective in preventing alcohol-induced gut bacteria translocation to MLNs and spleen and the passage of the high inflammatory component, LPS, to the systemic circulation (Antón et al., 2018).
In conclusion, to the best of our knowledge, this is the first paper studying the effect of alcohol binge drinking on fecal microbiota using this model and exploring the potential protective effect of pretreatment with the biolipid OEA on dysbiosis induced by binge drinking. They are both unexplored fields, and some of the results we have obtained are different from our initial hypothesis, which has caused difficulties in interpreting the results and understanding the biological meaning. Some of these difficulties arise from possible limitations of this study. For example, we did not have the possibility of measuring any biological marker (i.e., proinflammatory cytokines TNF-α or IL-1β in plasma) to correlate with the gut microbiota changes; this would have helped us to better understand the biological meaning of the observed microbial changes. Also, here, we used fecal samples as proxies for colonic microbiota content. Despite differences in the composition between fecal and mucosal communities being increasingly investigated, feces remain the most used sample source for gut microbiota analysis because it is naturally collected and non-invasive and can be obtained repeatedly (Tang et al., 2020). However, we cannot rule out that a similar analysis performed on samples of small intestine could produce different results than those presented here. In fact, it has been shown that chronic alcohol consumption may have a different impact on microbiota composition structure in the colon and in the jejunum (Fan et al., 2019). In conclusion, we carried out observational experiments, and despite the limitations, the observed results set the basis for future research to understand the biological meaning of these alterations.
To sum up, our results suggest that the protective actions of OEA in the context of alcohol abuse are not related to changes in alcohol-induced dysbiosis. Other mechanisms previously identified such as the prevention of leaky gut and anti-inflammatory actions may account for the protective effects.
Data Availability Statement
Datasets are uploaded in the European Nucleotide Archive (ENA) database with accession number PRJEB44965.
Ethics Statement
The animal study was reviewed and approved by the Animal Welfare Committee of Complutense University of Madrid (reference: PROEX 420/15).
Author Contributions
AR-G, MM, and LO were in charge of the experiment design and activity related to alcohol binge drinking in laboratory animals and sample collection. FV, CF, and MBP were in charge of microbiota analyses and statistical measures. AR-G, FV, and LO drafted the manuscript. All authors contributed to the article and approved the submitted version.
Funding
This work was supported by FEDER (European Union)/Ministerio de Ciencia e Innovación/Agencia Estatal de Investigación (Spain) (grant number: Retos 2018; RTI2018-099535-B-I00 to LO). AR-G was a recipient of a fellowship from Consejería de Educación, Juventud y Deporte (Comunidad de Madrid/Fondo Social Europeo). MBP was supported by JPI Nutricog, grant “Ambrosiac”.
Conflict of Interest
The authors declare that the research was conducted in the absence of any commercial or financial relationships that could be construed as a potential conflict of interest.
Publisher’s Note
All claims expressed in this article are solely those of the authors and do not necessarily represent those of their affiliated organizations, or those of the publisher, the editors and the reviewers. Any product that may be evaluated in this article, or claim that may be made by its manufacturer, is not guaranteed or endorsed by the publisher.
Acknowledgments
We thank Dr. Fernando Rodríguez de Fonseca for providing OEA used in the pilot studies.
Supplementary Material
The Supplementary Material for this article can be found online at: https://www.frontiersin.org/articles/10.3389/fcimb.2021.731910/full#supplementary-material
Supplementary Figure 1 | Comparison of genera relative abundance between the T1 alcohol administered rats, the T1 water administered rats, and the corresponding T0 samples. Only the genera with significant (with p-value < 0.05) difference in one way ANOVA were reported.
Supplementary Figure 2 | Best subset of identified microbiota markers, as reported in Figure 2. Histograms report relative abundance distribution among individual samples, grouping samples in the T1 alcohol and T1 water administered rats, and the corresponding T0 rats. The best marker were identified by evaluating their prevalence (i.e. the presence in multiple samples of a class) and relative abundance (i.e. by evaluating mean and median group abundances, reported as solid and dashed lines, respectively).
Supplementary Figure 3 | Microbiota association network on the alcohol intoxicated samples, comparing oral and i.p administration route of OEA. Image reports frequency distribution of the node degree statistic in the oral OEA network, during the removal of random nodes. Dotted lines represent i) the starting frequency distribution of the “undisturbed” oral OEA network (on the right,), ii) the frequency distribution of the i.p. OEA network. Each line represents the frequency distribution of node degree of a different network, re-obtained after removal of one node (and recalculation) of the previous network. The procedure was repeated 20 times. As none of the repetition showed a change in the shape of frequency of node degree distribution from the undisturbed network, we conclude that the loss of specific ASVs from the community led to the observed changes in microbial association network between the oral and the i.p. samples community.
References
Albanese, D., Fontana, P., De Filippo, C., Cavalieri, D., Donati, C. (2015). MICCA: A Complete and Accurate Software for Taxonomic Profiling of Metagenomic Data. Sci. Rep. 5, 9743. doi: 10.1038/srep09743
Antón, M., Alen, F., Heras, R., Serrano, A., Pavon, F. J., Leza, J. C., et al. (2017). Oleoylethanolamide Prevents Neuroimmune HMGB1/TLR4/NF-kB Danger Signaling in Rat Frontal Cortex and Depressive-Like Behavior Induced by Ethanol Binge Administration. Addict. Biol. 22, 724–741. doi: 10.1111/adb.12365
Antón, M., Rodríguez-González, A., Ballesta, A., González, N., Pozo, A., Fonseca, F. R., et al. (2018). Alcohol Binge Disrupts the Rat Intestinal Barrier: The Partial Protective Role of Oleoylethanolamide. Brit. J. Pharmacol. 175, 4464–4479. doi: 10.1111/bph.14501
Bull-Otterson, L., Feng, W., Kirpich, I., Wang, Y., Qin, X., Liu, Y., et al. (2013). Metagenomic Analyses of Alcohol Induced Pathogenic Alterations in the Intestinal Microbiome and the Effect of Lactobacillus Rhamnosus GG Treatment. PloS One 8, e53028. doi: 10.1371/journal.pone.0053028
Butera, A., Di Paola, M., Vitali, F., De Nitto, D., Covotta, F., Borrini, F., et al. (2020). IL-13 mRNA Tissue Content Identifies Two Subsets of Adult Ulcerative Colitis Patients With Different Clinical and Mucosa-Associated Microbiota Profiles. J. Crohns Colitis 14 (3), 369–380. doi: 10.1093/ecco-jcc/jjz154
Chassaing, B., Koren, O., Goodrich, J. K., Poole, A. C., Srinivasan, S., Ley, R. E., et al. (2015). Dietary Emulsifiers Impact the Mouse Gut Microbiota Promoting Colitis and Metabolic Syndrome. Nature 519 (7541), 92–96. doi: 10.1038/nature14232
Cryan, J. F., Dinan, T. G. (2012). Mind-Altering Microorganisms: The Impact of the Gut Microbiota on Brain and Behaviour. Nat. Rev. Neurosci. 13, 701–712. doi: 10.1038/nrn3346
Cryan, J., O'Riordan, K. J., Cowan, C. S. M., Sandhu, K. V., Bastiaanssen, T. F. S., Boehme, M., et al. (2019). The Microbiota-Gut-Brain Axis. Physiol. Rev. 99, 1877–2013. doi: 10.1152/physrev.00018.2018
Csardi, G., Nepusz, T. (2006). The Igraph Software Package for Complex Network Research. InterJournal Complex Syst. 1695 (5), 1–9.
Dinan, T. G., Stanton, C., Cryan, J. F. (2013). Psychobiotics: A Novel Class of Psychotropic. Biol. Psychiatry 74, 720–726. doi: 10.1016/j.biopsych.2013.05.001
Di Paola, M., Bonechi, E., Provensi, G., Costa, A., Clarke, G., Ballerini, C., et al. (2018). Oleoylethanolamide Treatment Affects Gut Microbiota Composition and the Expression of Intestinal Cytokines in Peyer’s Patches of Mice. Sci. Rep. 8, 1–12. doi: 10.1038/s41598-018-32925-x
Edgar, R. C. (2016). UNOISE2: Improved Error-Correction for Illumina 16S and ITS Amplicon Sequencing. BioRxiv, 081257. doi: 10.1101/081257
Engen, P. A., Green, S. J., Voigt, R. M., Forsyth, C. B., Keshavarzian, A. (2015). The Gastrointestinal Microbiome: Alcohol Effects on the Composition of Intestinal Microbiota. Alcohol Res. Curr. Rev. 37, 223.
Fan, Y., Ya-E, Z., Ji-Dong, W., Yu-Fan, L., Ying, Z., Ya-Lun, S., et al. (2018). Comparison of Microbial Diversity and Composition in Jejunum and Colon of the Alcohol-Dependent Rats. J. Microbiol. Biotechnol. 28 (11), 1883–1895. doi: 10.4014/jmb.1806.06050
Faust, K., Raes, J. (2012). Microbial Interactions: From Networks to Models. Nat. Rev. Microbiol. 10, 538–550. doi: 10.1038/nrmicro2832
Flemer, B., Gaci, N., Borrel, G., Sanderson, I. R., Chaudhary, P. P., Tottey, W., et al. (2017). Fecal Microbiota Variation Across the Lifespan of the Healthy Laboratory Rat. Gut Microbes 8 (5), 428–439. doi: 10.1080/19490976.2017.1334033
Giuffrida, A., Rodríguez de Fonseca, F., Piomelli, D. (2000). Quantification of Bioactive Acylethanolamides in Rat Plasma by Electrospray Mass Spectrometry. Anal. Biochem. 280, 87–93. doi: 10.1006/abio.2000.4509
Hartmann, P., Chen, P., Wang, H. J., Wang, L., McCole, D. F., Brandl, K., et al. (2013). Deficiency of Intestinal Mucin-2 Ameliorates Experimental Alcoholic Liver Disease in Mice. Hepatology 58, 108–119. doi: 10.1002/hep.26321
Hartmann, P., Seebauer, C. T., Schnabl, B. (2015). Alcoholic Liver Disease: The Gut Microbiome and Liver Cross Talk. Alcohol. Clin. Exp. Res. 39, 763. doi: 10.1111/acer.12704
Hillemacher, T., Bachmann, O., Kahl, K. G., Frieling, H. (2018). Alcohol, Microbiome, and Their Effect on Psychiatric Disorders. Prog. Neuropsychopharmacol. Biol. Psychiatry 85, 105–115. doi: 10.1016/j.pnpbp.2018.04.015
Holder, M. K., Peters, N. V., Whylings, J., Fields, C. T., Gewirtz, A. T., Chassaing, B., et al. (2019). Dietary Emulsifiers Consumption Alters Anxiety-Like and Social-Related Behaviors in Mice in a Sex-Dependent Manner. Sci. Rep. 9 (1), 172. doi: 10.1038/s41598-018-36890-3
Joshi, N. A., Fass, J. N. (2011). Sickle: A Sliding-Window, Adaptive, Quality-Based Trimming Tool for FastQ Files (Version 1.33) [Software].
Kanny, D., Brewer, R. D., Mesnick, J. B., Paulozzi, L. J., Naimi, T. S., Lu, H. (2015). Vital Signs: Alcohol Poisoning Deaths–United States 2010-2012. Morb. Mortal. Wkly. Rep. 63, 1238.
Kilkenny, C., Browne, W., Cuthill, I. C., Emerson, M., Altman, D. G. (2010). Animal research: Reporting in vivo experiments: The ARRIVE guidelines. Brit. J. Pharmacol. 160, 1577–1579. doi: 10.1111/j.1476-5381.2010.00872.x
Kosnicki, K. L., Penprase, J. C., Cintora, P., Torres, P. J., Harris, G. L., Brasser, S. M., et al. (2019). Effects of Moderate, Voluntary Ethanol Consumption on the Rat and Human Gut Microbiome. Addict. Biol. 24, 617–630. doi: 10.1111/adb.12626
Kurtz, Z. D., Müller, C. L., Miraldi, E. R., Littman, D. R., Blaser, M. J., Bonneau, R. A. (2015). Sparse and Compositionally Robust Inference of Microbial Ecological Networks. PloS Comput. Biol. 11 (5), e1004226. doi: 10.1371/journal.pcbi.1004226
Lahti, L., Shetty, S. (2017). “Tools for Microbiome Analysis in R. Microbiome Package Version 1.7.21,” in Bioconductor. Available at: http://microbiome.github.com/microbiome.
Lamas-paz, N., Sydor, S., Lamas-Paz, A., Morán, L., Salinas, B., Peng, J., et al. (2020). Intestinal Epithelial Cell-Derived Extracellular Vesicles Modulate Hepatic Injury via the Gut-Liver Axis During Acute Alcohol Injury. Front. Pharmacol. 11, 603771. doi: 10.3389/fphar.2020.603771
Leclercq, S., Matamoros, S., Cani, P. D., Neyrinck, A. M., Jamar, F., Starkel, P., et al. (2014). Intestinal Permeability, Gut-Bacterial Dysbiosis, and Behavioral Markers of Alcohol-Dependence Severity. Proc. Natl. Acad. Sci. U. S. A. 111, 4485. doi: 10.1073/pnas.1415174111
Lee, J., Ha, J. S., Park, H., Lee, E. (2019). Alteration of Gut Microbiota Composition by Short-Term Low-Dose Alcohol Intake Is Restored by Fermented Rice Liquor in Mice. Food Res. Int. 128, 108800. doi: 10.1016/j.foodres.2019.108800
Litwinowicz, K., Choroszy, M., Waszczuk, E. (2019). Changes in the Composition of the Human Intestinal Microbiome in Alcohol Use Disorder: A Systematic Review. Am. J. Drug Alcohol Abuse 46, 4–12. doi: 10.1080/00952990.2019.1669629
Martin, M. (2011). Cutadapt Removes Adapter Sequences From High-Throughput Sequencing Reads. EMBnet J. 17 (1), 10–12. doi: 10.14806/ej.17.1.200
McMurdie, P. J., Holmes, S. (2013). Phyloseq: An R Package for Reproducible Interactive Analysis and Graphics of Microbiome Census Data. PloS One 8, e61217. doi: 10.1371/journal.pone.0061217
Meroni, M., Longo, M., Dongiovanni, P. (2019). Alcohol or Gut Microbiota: Who Is the Guilty? Int. J. Mol. Sci. 20:4568. doi: 10.3390/ijms20184568
Mutlu, E. A., Gillevet, P. M., Rangwala, H., Sikaroodi, M., Naqvi, A., Engen, P. A., et al. (2012). Colonic Microbiome Is Altered in Alcoholism. Am. J. Physiol. Gastrointest. Liver Physiol. 302, 966. doi: 10.1152/ajpgi.00380.2011
Mutlu, E., Keshavarzian, A., Engen, P., Forsyth, C. B., Sikaroodi, M., Gillevet, P. (2009). Intestinal Dysbiosis: A Possible Mechanism of Alcohol-Induced Endotoxemia and Alcoholic Steatohepatitis in Rats. Alcohol. Clin. Exp. Res. 33, 1836–1846. doi: 10.1111/j.1530-0277.2009.01022
National Institute on Alcohol Abuse and Alcoholism [NIAAA]. (2004). NIAAA Council Approves Definition of Binge Drinking (Bethesda: National Institutes of Health).
Neyrinck, A. M., Etxeberria, U., Taminiau, B., Daube, G., Van Hul, M., Everard, A., et al. (2016). Rhubarb Extract Prevents Hepatic Inflammation Induced by Acute Alcohol Intake, an Effect Related to the Modulation of the Gut Microbiota. Mol. Nutr. Food Res. 61 (1), 1–21. doi: 10.1002/mnfr.201500899
Obernier, J. A., Bouldin, T. W., Crews, F. T. (2002). Binge Ethanol Exposure in Adult Rats Causes Necrotic Cell Death. Alcohol. Clin. Exp. Res. 26, 547–557. doi: 10.1111/j.1530-0277.2002.tb02573.x
Orio, L. (2020). The Multifaceted Potential of the Lipid Transmitter Oleoylethanolamide to Treat Alcohol-Induced Neuroinflammation and Alcohol Use Disorders. Neural Regen. Res. 15, 71–72. doi: 10.4103/1673-5374.264457
Orio, L., Alen, F., Pavón, F. J., Serrano, A., García-Bueno, B. (2019). Oleoylethanolamide, Neuroinflammation, and Alcohol Abuse. Front. Mol. Neurosci. 11:490. doi: 10.3389/fnmol.2018.00490
Paulson, J. N., Talukder, H., Pop, M., Bravo, H. C. (2013) Metagenomeseq: Statistical Analysis for Sparse High-Throughput Sequencing. Available at: http://cbcb.umd.edu/software/metagenomeSeq.
Reyes, R. E. N., Al Omran, A. J., Davies, D. L., Asatryan, L. (2020). Antibiotic-Induced Disruption of Commensal Microbiome Linked to Increases in Binge-Like Ethanol Consumption Behavior. Brain Res. 1747, 147067. doi: 10.1016/j.brainres.2020.147067
Rodriguez-Gonzalez, A., Orio, L. (2020). Microbiota and Alcohol Use Disorder: Are Psychobiotics a Novel Therapeutic Strategy? Curr. Pharm. Des. 26, 2426–2437. doi: 10.2174/1381612826666200122153541
SAMHSA. (2017). Key Substance Use and Mental Health Indicators in the United States: Results From the 2016 National Survey on Drug Use and Health.
Samuelson, D. R., Gu, M., Shellito, J. E., Molina, P. E., Taylor, C. M., Luo, M., et al. (2019). Intestinal Microbial Products From Alcohol-Fed Mice Contribute to Intestinal Permeability and Peripheral Immune Activation. Alcohol. Clin. Exp. Res. 43, 2122. doi: 10.1111/acer.14176
Sayd, A., Anton, M., Alen, F., Caso, J. R., Pavon, J., Leza, J. C., et al. (2015). Systemic Administration of Oleoylethanolamide Protects From Neuroinflammation and Anhedonia Induced by LPS in Rats. Int. J. Neuropsychopharmacol. 18 (6), pii:pyu111. doi: 10.1093/ijnp/pyu111
Scriven, M., Dinan, T. G., Cryan, J. F., Wall, M. (2018). Neuropsychiatric Disorders: Influence of Gut Microbe to Brain Signalling. Diseases 6 (3):78. doi: 10.3390/diseases6030078
Segata, N., Izard, J., Waldron, L., Gevers, D., Miropolsky, L., Garrett, W. S., et al. (2011). Metagenomic Biomarker Discovery and Explanation. Genome Biol. 12 (6), 1–18. doi: 10.1186/gb-2011-12-6-r60
Sherwin, E., Dinan, T. G., Cryan, J. F. (2018). Recent Developments in Understanding the Role of the Gut Microbiota in Brain Health and Disease. Ann. N. Y. Acad. Sci. 1420, 5–25. doi: 10.1111/nyas.13416
Takahashi, S., Tomita, J., Nishioka, K., Hisada, T., Nishijima, M. (2014). Development of a Prokaryotic Universal Primer for Simultaneous Analysis of Bacteria and Archaea Using Next-Generation Sequencing. PloS One 9, e105592. doi: 10.1371/journal.pone.0105592
Tamburini, E., Doni, L., Lussu, R., Meloni, F., Cappai, G., Carucci, A., et al. (2020). Impacts of Anthropogenic Pollutants on Benthic Prokaryotic Communities in Mediterranean Touristic Ports. Front. Microbiol. 11, 1234. doi: 10.3389/fmicb.2020.01234
Tang, Q., Jin, G., Wang, G., Liu, T., Liu, X., Wang, B., et al. (2020). Current Sampling Methods for Gut Microbiota: A Call for More Precise Devices. Front. Cell Infect. Microbiol. 10, 151. doi: 10.3389/fcimb.2020.00151
Temko, J. E., Bouhlal, S., Farokhnia, M., Lee, M. R., Cryan, J. F., Leggio, L. (2017). The Microbiota, the Gut and the Brain in Eating and Alcohol Use Disorders: A 'Ménage À Trois'? Alcohol Alcohol. (Oxford Oxfordshire) 52, 403–413. doi: 10.1093/alcalc/agx024
Timary, P. D., Leclercq, S., Stärkel, P., Delzenne, N. (2015). A Dysbiotic Subpopulation of Alcohol-Dependent Subjects. Gut Microbes 6, 388–391. doi: 10.1080/19490976.2015.1107696
Wang, Q., Garrity, G. M., Tiedje, J. M., Cole, J. R. (2007). Naive Bayesian Classifier for Rapid Assignment of rRNA Sequences Into the New Bacterial Taxonomy. Appl. Environ. Microbiol. 16, 5261–5267. doi: 10.1128/AEM.00062-07
Wang, H., Wang, Y. (2016). Gut Microbiota-Brain Axis. Chin. Med. J. 129, 2373–2380. doi: 10.4103/0366-6999.190667
Xiao, H., Ge, C., Feng, G., Li, Y., Li, H., Luo, D., et al. (2018). Gut Microbiota Modulates Alcohol Withdrawal-Induced Anxiety in Mice. Toxicol. Lett. 287, 23–30. doi: 10.1016/j.toxlet.2018.01.021
Yan, A. W., Fouts, D. E., Brandl, J., Stärkel, P., Torralba, M., Schott, E., et al. (2011). Enteric Dysbiosis Associated With a Mouse Model of Alcoholic Liver Disease. Hepatology 3 (1), 96–105. doi: 10.1002/hep.24018
Keywords: fecal microbiota, alcohol, OEA, microbiome, substance abuse, binge, dysbiosis
Citation: Rodríguez-González A, Vitali F, Moya M, De Filippo C, Passani MB and Orio L (2021) Effects of Alcohol Binge Drinking and Oleoylethanolamide Pretreatment in the Gut Microbiota. Front. Cell. Infect. Microbiol. 11:731910. doi: 10.3389/fcimb.2021.731910
Received: 28 June 2021; Accepted: 26 October 2021;
Published: 23 November 2021.
Edited by:
Edoardo Puglisi, Catholic University of the Sacred Heart, ItalyReviewed by:
Shiyu Tao, Huazhong Agricultural University, ChinaMaria de los Angeles Serradell, Consejo Nacional de Investigaciones Científicas y Técnicas (CONICET), Argentina
Copyright © 2021 Rodríguez-González, Vitali, Moya, De Filippo, Passani and Orio. This is an open-access article distributed under the terms of the Creative Commons Attribution License (CC BY). The use, distribution or reproduction in other forums is permitted, provided the original author(s) and the copyright owner(s) are credited and that the original publication in this journal is cited, in accordance with accepted academic practice. No use, distribution or reproduction is permitted which does not comply with these terms.
*Correspondence: Laura Orio, lorio@psi.ucm.es
†These authors have contributed equally to this work