- Department of Production Animal Health, Faculty of Veterinary Medicine, University of Calgary, Calgary, AB, Canada
Bovine digital dermatitis (DD) is a significant cause of infectious lameness and economic losses in cattle production across the world. There is a lack of a consensus across different 16S metagenomic studies on DD-associated bacteria that may be potential pathogens of the disease. The goal of this meta-analysis was to identify a consistent group of DD-associated bacteria in individual DD lesions across studies, regardless of experimental design choices including sample collection and preparation, hypervariable region sequenced, and sequencing platform. A total of 6 studies were included in this meta-analysis. Raw sequences and metadata were identified on the NCBI sequence read archive and European nucleotide archive. Bacterial community structures were investigated between normal skin and DD skin samples. Random forest models were generated to classify DD status based on microbial composition, and to identify taxa that best differentiate DD status. Among all samples, members of Treponema, Mycoplasma, Porphyromonas, and Fusobacterium were consistently identified in the majority of DD lesions, and were the best genera at differentiating DD lesions from normal skin. Individual study and 16S hypervariable region sequenced had significant influence on final DD lesion microbial composition (P < 0.05). These findings indicate that members of Treponema, Mycoplasma, Porphyromonas, and/or Fusobacterium may have significant roles in DD pathogenesis, and should be studied further in respect to elucidating DD etiopathogenic mechanisms and developing more effective treatment and mitigation strategies.
Introduction
Bovine digital dermatitis (DD) is an infectious skin lesion that was first reported in Italy in 1974 (Cheli and Mortellaro, 1974), and is a significant cause of lameness in cattle across the world (Read and Walker, 1998). DD is a multifaceted disease, having both painful ulcerative lesions and chronic hyperkeratotic stages across the different morphological presentations of the lesions (Döpfer et al., 1997; Berry et al., 2012). Treatment and production costs associated with DD lead to significant losses for both beef and dairy producers mainly due to either decreased average daily gain and decreased milk production (Gomez et al., 2015; Davis-Unger et al., 2017; Kulow et al., 2017; Dolecheck et al., 2019). Prevalence of DD at the herd level is reported to be as high as 93% (Solano et al., 2016), but prevalence estimates vary based on a multitude of factors including parity, breed, housing type and hoof trimming frequency (Holzhauer et al., 2006; Solano et al., 2016; Bran et al., 2018; Yang et al., 2019). Current treatment and prevention strategies rely on topical applications and footbaths of broad spectrum antimicrobial agents, most commonly tetracycline or copper sulfate, and are largely ineffective at returning lesions to normal skin and preventing recurrence of active lesions (Krull et al., 2016; Jacobs et al., 2018).
Early attempts to determine etiological agents of DD pointed to Treponema as the main pathogen of these lesions. More recent evidence shifts the focus to studying the roles of both Treponema and additional anaerobic bacteria in lesion formation and progression, leading to a polymicrobial hypothesis of DD causation (Yano et al., 2010; Santos et al., 2012). The quantities of Treponema are dramatically higher in most DD lesions compared to healthy skin; however, there exists a wide variety of Treponema species and phylotypes across individual animals and between studies (Klitgaard et al., 2008; Klitgaard et al., 2013; Krull et al., 2014). Most commonly, Treponema phagedenis, T. medium, T. refringens, T. denticola, and T. pedis are identified in DD lesions, but presence and population dynamics of each species varies across studies (Krull et al., 2014; Zinicola et al., 2015a; Zinicola et al., 2015b).
Additional bacteria outside the genus Treponema likely play some role in DD pathogenesis based on their presence and abundance within DD lesions; however, the conclusions on which of these bacteria potentially are involved can vary dramatically across studies of DD microbiota. In addition to Treponema, many recent metagenomic studies associate Mycoplasma abundance with DD lesions (Krull et al., 2014; Nielsen et al., 2016; Hesseling et al., 2019). Inconsistencies most notably arise between studies when implicating other bacterial genera in DD pathogenesis. Porphyromonas (Zinicola et al., 2015b; Nielsen et al., 2016), Fusobacterium (Nielsen et al., 2016; Moreira et al., 2018), and Dichelobacter (Krull et al., 2014; Moreira et al., 2018), among a few other genera are inconsistently associated with DD lesion microbiota and pathogenesis. It is currently unknown if these major differences between metagenomic studies are due to differences in sequencing, bioinformatic processing, sample size or if DD lesion microbiota differs across beef and dairy cattle breeds.
Comparing conclusions between metagenomic studies can lead to erroneous interpretations, as variation in study objectives and analytical processing can influence findings and result in contradictory comparisons between study outcomes. Many metagenomic studies process individual sequencing reads into operational taxonomic units, which are not directly comparable across experiments and can be a source of variation when drawing comparisons between studies (Callahan et al., 2017). Primer bias in deep amplicon sequencing can also have a significant impact on observed bacterial populations, as certain primers used in the amplification process before sequencing might not amplify all bacteria at equal rates, but the extent of this impact is not determined yet in DD-specific microbial communities. In addition, different study designs, experimental conditions, and statistical analyses can significantly alter the results and conclusions on microbial composition (Goodrich et al., 2014). Therefore, a meta-analysis is required in order to draw controlled and quantitative conclusions across these metagenomic DD studies.
The differences in findings between DD metagenomic studies leads to a lack of consensus on which bacteria may be potential pathogens, which is a limiting factor for future DD pathogenesis research. The goal of this meta-analysis was to use a consistent analytical approach on pooled sequences from individual DD metagenomic studies to identify a DD-associated microbiota that consistently and accurately differentiates DD lesions from normal skin. Identifying a consistent DD-associated microbiota, regardless of individual animal variation, 16S hypervariable region sequenced, cattle breed, and other study design choices would provide strong evidence for a potential core DD microbiota and initiate and accelerate future targeted research efforts toward determining etiopathogenesis mechanisms of DD.
Materials and Methods
Data Collection and Selection Criteria
Searches for publicly available data were performed on the NCBI Sequence Read Archive (SRA) database and the European Nucleotide Archive using the search term “digital dermatitis”, and limiting search results by “BioProject”. BioProject accession numbers (study identifiers) containing high throughput sequencing reads and associated metadata were collected. For inclusion in the meta-analysis, all studies had to have publicly available sequencing reads before September 1, 2020. Studies were required to be a bovine DD metagenomic study, and have conducted deep amplicon sequencing of a 16S rRNA hypervariable region using universal bacterial primers on samples originating from bovine skin. Raw data (DNA sequences and associated quality scores) and sufficient metadata (containing information differentiating samples by DD diagnosis) had to be publicly available for inclusion in the meta-analysis. Additional information on sample collection was obtained for each study. All samples were collected with biopsy punches from feet cleaned with water or chlorhexidine of either live animals or at a slaughterhouse. Depth and exact location of skin lesion samples were not universally available across all studies. Other relevant details concerning experimental processing of samples before sequencing are described in Table 1. Fastq files were all downloaded from the NCBI SRA database (www.ncbi.nlm.nih.gov/sra).
Sequence Processing
Raw fastq files that contained reads from both the V1V2 and Treponema-specific V3V4 16S rRNA regions were processed with Bowtie2 v.2.3.5.1 (Langmead and Salzberg, 2012) in order to separate reads by hypervariable region. Afterwards, sequences were processed using the DADA2 R package v.1.14.1 (Callahan et al., 2016). Quality filtering and base call error models were conducted on each study independently, in order to account for differences in error rates between sequencing runs. Reads were truncated after the average Phred score dropped below 30, and any reads with ambiguous nucleotides were removed. The recommended DADA2 settings for each sequencing platform (Illumina and LS454) were used to infer amplicon sequence variants (ASVs). Taxonomy was assigned using the DADA2 naïve Bayesian classifier against the SILVA v.138 database (Quast et al., 2013). Prior to analysis, all ASVs classified as Eukaryota, Mitochondria, or Chloroplast were removed.
Data Analysis
Due to the lack of consistent information across studies on individual lesion stages, all samples included in analysis were categorized as “DD positive” or “DD negative”. DD positive samples were defined as any sample from DD lesion skin at any stage of the disease. DD negative samples were classified as any sample from skin with no visible DD lesion present. Any samples that were obtained from treated lesions were removed from downstream analysis. For diversity analyses, samples with less than 1500 reads were removed, and the remaining samples were rarefied down to the minimum sequencing depth. Observed ASV count, Chwfi 2ao1 richness estimate, Fisher’s alpha and Shannon’s diversity index were used to evaluate diversity (richness and evenness) within DD positive and negative samples. Analysis of variance (ANOVA) followed by post hoc Tukey’s Honest Significant Difference (TukeyHSD) test determined any significant differences of diversity between DD statuses. For beta diversity analyses, ASVs were grouped at phylum, family, and genus taxonomic levels, and Bray-Curtis dissimilarities between samples were explored using Principal Coordinates (PCoA) analysis. Permutational ANOVA (PERMANOVA) with 999 permutations, followed by a test for multivariate homogeneity of group dispersions (BETADISP) was performed to identify differences in microbial composition between DD negative and DD positive samples.
The influence of different study variables and experimental designs on microbial composition was measured exclusively on DD positive samples by repeating the diversity analysis methods described above. Alpha diversity and relative abundance plots evaluated the effect of different 16S rRNA hypervariable regions sequenced, and beta diversity measured the variation in microbial composition by individual study, 16S rRNA hypervariable region, and sequencing platform. All diversity metrics and statistical analyses were conducted using the vegan package (v. 2.5.6) in R (Dixon, 2003).
Random forest modeling was used to predict DD status based on microbial composition, and identify the microbiota that best differentiates DD positive from DD negative samples. Prior to modeling, ASVs were grouped at phylum, family, and genus ranks and rare taxa (present in less than 1% of samples) were removed. Sample ASV counts were normalized using a centered log ratio transformation, with a pseudocount of 1 applied to all ASVs. Randomly generated training sample sets were made of 70% of each DD negative and positive samples, and testing sample sets contained the remaining 30% of samples in each group. Random forest models containing 1000 trees were trained using 100 repeats of 10-fold cross validation, and the number of variables sampled at each node was optimized as part of the caret R package v.6.0.86 (Kuhn, 2008). Model performance was evaluated based on accuracy of classification and kappa score, which was determined by constructing a confusion matrix based on the testing sample set. Relative variable importance along with relative abundance of each taxa was used to identify the microbiota that differentiate DD positive from DD negative samples. A P value of less than 0.05 was considered statistically significant for all comparisons. All analysis and figures were completed in R version 3.5.3.
Results
Study Selection and Characteristics
A total of 24 BioProject IDs were identified upon the initial search. Next, 12 studies were removed because they did not conduct deep amplicon sequencing of a 16S rRNA hypervariable region. Four studies were excluded because they were not from bovine skin/lesion samples, or did not have any raw fastq files associated with the BioProject ID. One study was removed because it did not use universal bacterial primers for sequencing. Finally, one study was removed due to a lack of metadata to differentiate samples by DD status. Included in the meta-analysis were 6 studies (Table 1), which all provided sufficient metadata to differentiate the DD status (DD negative samples, n = 37; DD positive samples, n = 190). The total number of raw sequences available for meta-analysis was 16,659,674, and after quality filtering, denoising, and merging, 8,827,942 reads were taxonomically classified. These samples were further categorized by sequencing platform, operation type (dairy, beef, or mixed, if known), 16S rRNA hypervariable region, DNA extraction kit, country of sample origin, and if the sample was known to come from an animal treated for DD. Samples that came from treated lesions (n = 16) were removed from the meta-analysis in order to focus solely on the differences in microbial populations between DD positive and DD negative skin.
Characterization of Microbiota in DD Positive and DD Negative Skin
For all measures of alpha diversity tested, DD positive skin microbiota had significantly lower (P < 0.05) diversity, richness, and evenness when compared to DD negative skin microbiota (Table 2). Bray-Curtis distances between samples showed a significant difference (P < 0.05) in microbial composition between DD negative and DD positive skin (Figure 1 and Table 3), regardless of the taxonomic rank tested. However, BETADISP was significant (P < 0.05) at the phylum level (Table 3). For these Bray-Curtis distances, the percent of variation explained by DD status increased as taxonomic rank lowered from phylum to genus (Table 3). When ASVs were grouped at the genus level, approximately 20.5% of the variation in microbial composition could be explained by DD status (Table 3). DD positive samples cluster in two major groups based on Bray-Curtis dissimilarities, and are visually separated by high and low Spirochaetaceae relative abundance (Figure S1).

Table 2 Alpha diversity estimatesa of DD negative and DD positive skin microbiota.
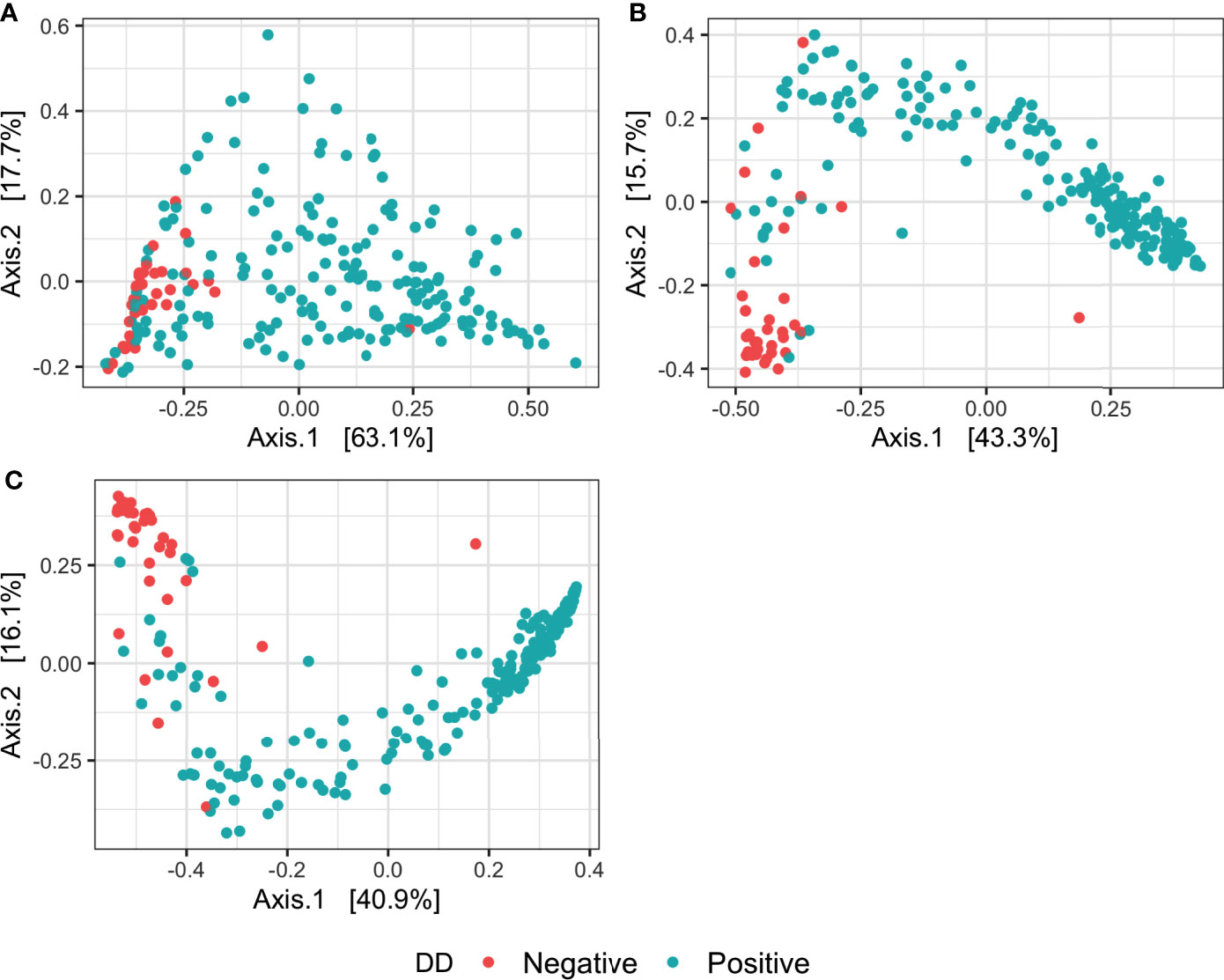
Figure 1 Principal coordinates analysis of Bray-Curtis distances of the microbiota in DD negative and DD positive skin. Bray-Curtis distances were calculated on samples with ASVs grouped at three different taxonomic levels: (A) Phylum, (B) Family, (C) Genus.

Table 3 Permutation analysis of variance and analysis of multivariate homogeneity of group dispersions on Bray-Curtis distances of DD negative and DD positive skin microbiota grouped at different taxonomic levels.
Distinct bacterial populations were found when DD negative skin microbiota was compared to DD positive skin microbiota. At the phylum level, Spirochaetota relative abundance was noticeably higher in DD positive skin, which was present at roughly 3% in DD negative skin to 35% relative abundance in DD positive skin (Figure 2A). There were also higher relative abundances of Fusobacteriota, Bacteroidota, and Campilobacterota in DD positive skin (Figure 2A). DD negative skin was dominated primarily by members of Firmicutes, Actinobacteriota, and Proteobacteria (Figure 2A). Additional trends emerged when increasing the taxonomic resolution to family and genus levels, as shown in Figures 2B, C. The families Spirochaetaceae and Fusobacteriaceae were higher in relative abundance in DD positive skin, similarly to their phylum level equivalents. In addition, families Mycoplasmataceae and Porphyromonadaceae were higher in relative abundance in DD positive skin compared to DD negative skin. At genus level grouping, Treponema dominated the DD positive skin microbiota; however, there were 4 other groups that also showed considerably higher relative abundance in DD positive skin compared to DD negative skin. Fusobacterium and Peptoanaerobacter were both relatively absent in DD negative skin, but were present at roughly 3% and 5% relative abundance in DD positive skin, respectively. Porphyromonas and Mycoplasma were present in DD negative skin at roughly 3% and 1%, respectively, but both had higher relative abundance in DD positive skin at greater than 6% relative abundance. In terms of bacterial prevalence, Treponema, Porphyromonas, Mycoplasma, Fusobacterium, and Peptoanaerobacter were present in the majority of DD positive skin samples, and were more prevalent in DD positive samples relative to DD negative skin samples (Table S1). Mycoplasma, Treponema, and Porphyromonas were all present in at least 94% of DD positive samples; whereas, Peptoanaerobacter and Fusobacterium were present in 81% and 63% of DD positive samples, respectively (Table S1). However, Treponema were also present in approximately 86% of DD negative samples, along with Porphyromonas and Peptoanaerobacter also present in more than 60% of DD negative samples (Table S1). Amnipila, Ezakiella, and Campylobacter, are genera that had low relative abundances at less than 1% in DD positive skin (Figure 2C), but were all present in greater than 75% of DD positive samples, while absent in the majority of DD negative skin samples (Table S1).
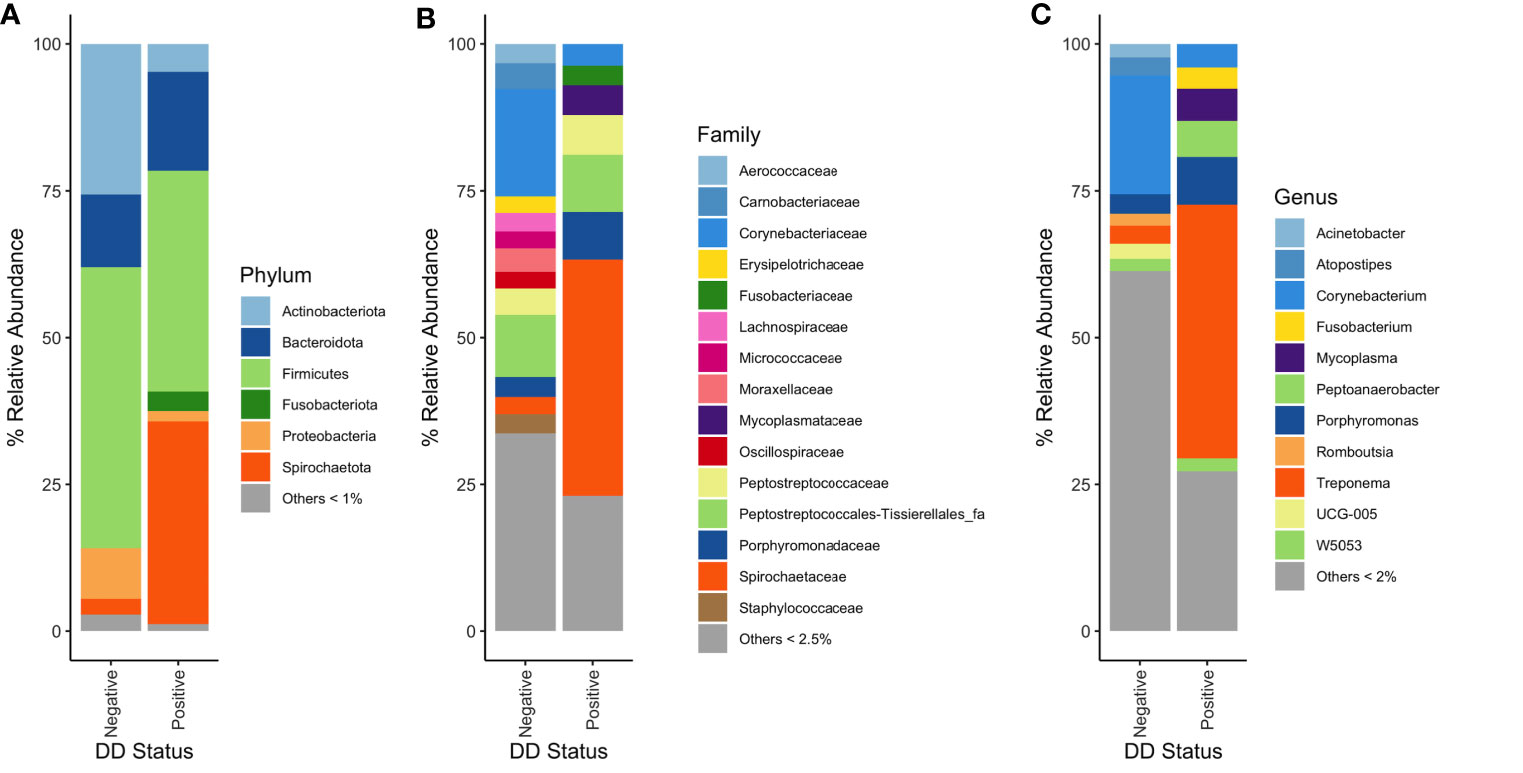
Figure 2 Mean percent relative abundances of microbiota in DD negative and DD positive skin. (A) Taxa were grouped at the phylum level, and all taxa that had less than 1% relative abundance were grouped. Taxa were grouped at the (B) family and (C) genus level, and all taxa that had less than 2.5% and 2% relative abundance, respectively, were grouped.
Random forests classifiers built at each taxonomic rank all had at least 90% accuracy in classifying DD status based on microbial composition (Table 4). When bacterial taxonomy was grouped at the genus level, models had the highest accuracy at 97.06% and a kappa score of 0.82 (Table 4). Of the top 30 important genera in the random forest model, 23 were associated with DD negative skin (Figures 3A–C). Mycoplasma was the third ranked genus in the model, and was the first ranked DD positive-associated bacteria in the model. Six additional genera were designated as important markers of DD positive skin microbiota, of these, only Treponema, Fusobacterium, and Porphyromonas had relative abundances of greater than 3% in DD lesions, whereas the remaining 3 genera had a relative abundance of roughly 0.1% or less in DD positive skin.

Table 4 Evaluation of random forest model performance on classifying DD status from microbial composition at three taxonomic levels.
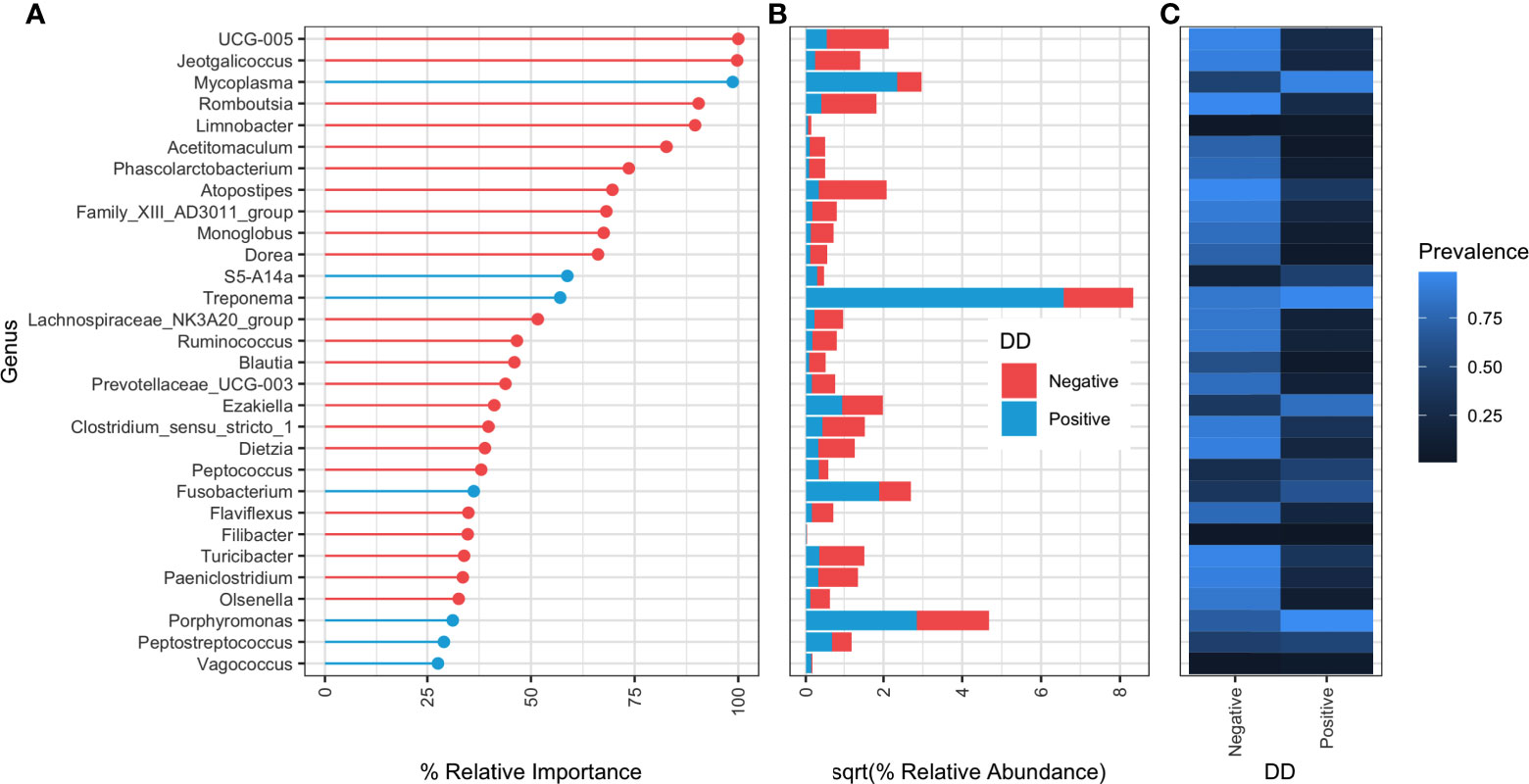
Figure 3 Relative importance ranking of random forest classifier at genus level taxonomy. (A) Percent relative importance of top 30 genera to the random forest classifier. Genera are coloured red if they have higher relative abundance in DD negative skin, and blue if they have a higher relative abundance in DD positive skin. (B) Square-root transformed relative abundances in DD positive and negative skin. Relative abundances were square-root transformed for easier visualization of large differences in relative abundance between genera. (C) Proportion of samples containing at least one sequence count of each genera.
Study Variables Influencing the Microbiota of DD Positive Skin
Permutational analysis of variance on Bray-Curtis distances identified BioProject (individual studies) as the largest source of variation (20%) in microbial composition between DD positive samples, regardless of taxonomic levels tested (Figure 4 and Table 5). The average relative abundances of non-rare taxa were relatively similar across studies, although one study detected distinctly lower relative abundance of Spirochaetaceae in DD positive samples (Figure S2). There were 3 different 16S rRNA hypervariable regions sequenced, and was another significant variable influencing the microbiota, accounting for roughly 11% of the differences between samples (Table 5). Sequencing platform, either Illumina or Life Sciences 454 pyrosequencing explained the lowest amount of variation of all categories tested (Table 5). All study variables, except for BioProject at phylum level grouping, displayed significant heterogenous dispersion across groups (P < 0.05) as identified in Table 6.
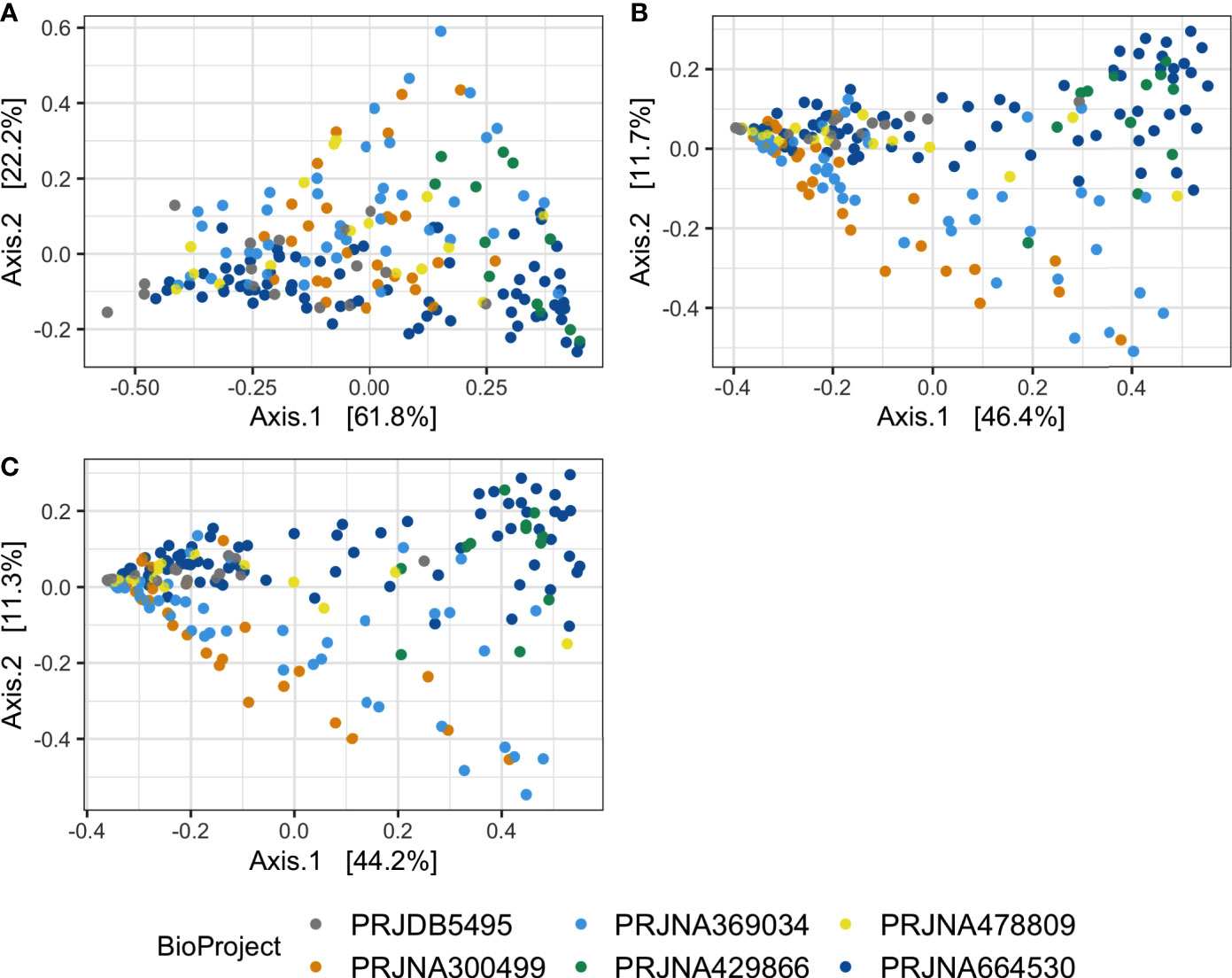
Figure 4 Principal coordinates analysis on Bray-Curtis distances of DD positive skin microbiota. Bray-Curtis distances were calculated on DD positive skin samples in which ASVs were grouped at (A) phylum, (B) family, and (C) genus level classifications.

Table 5 Study variable PERMANOVA analysis on Bray-Curtis distances of DD positive skin microbiota grouped at different taxonomic levels.

Table 6 Study variable BETADISP analysis of Bray-Curtis distances between DD positive skin microbiota grouped at different taxonomic levels.
There were significant differences (P < 0.05) in richness, evenness, and diversity within DD positive samples from the V3V4 region compared to both V1V2 and V1V3 for all diversity measures evaluated (Table 7). No significant differences in any alpha diversity measurement existed between samples from V1V2 and V1V3 hypervariable regions (Table 7). In DD lesions from V1V3 amplification, there was a notable absence of Fusobacterium compared to the other two hypervariable regions, which show a Fusobacterium relative abundance of 2-4% in DD positive skin (Figures 5A, B). Although the proportions varied across sequencing regions, DD positive skin appeared to be dominated by Treponema, regardless of 16S rRNA hypervariable region (Figure 5B). Other bacterial genera with a relative abundance higher than 2% that were common across all sequencing regions in DD positive skin were Mycoplasma, Peptoanaerobacter, and Porphyromonas (Figure 5B). Although no DD negative skin samples were acquired from the V1V3 region, microbiota of DD negative skin from V1V2 and V3V4 sequencing both contained a community dominated by members of the phyla Firmicutes, Actinobacteriota, Bacteroidota, and Proteobacteria (Figure 5A).

Table 7 Alpha diversity estimates* of DD positive skin microbiota across different 16S rRNA hypervariable regions**.
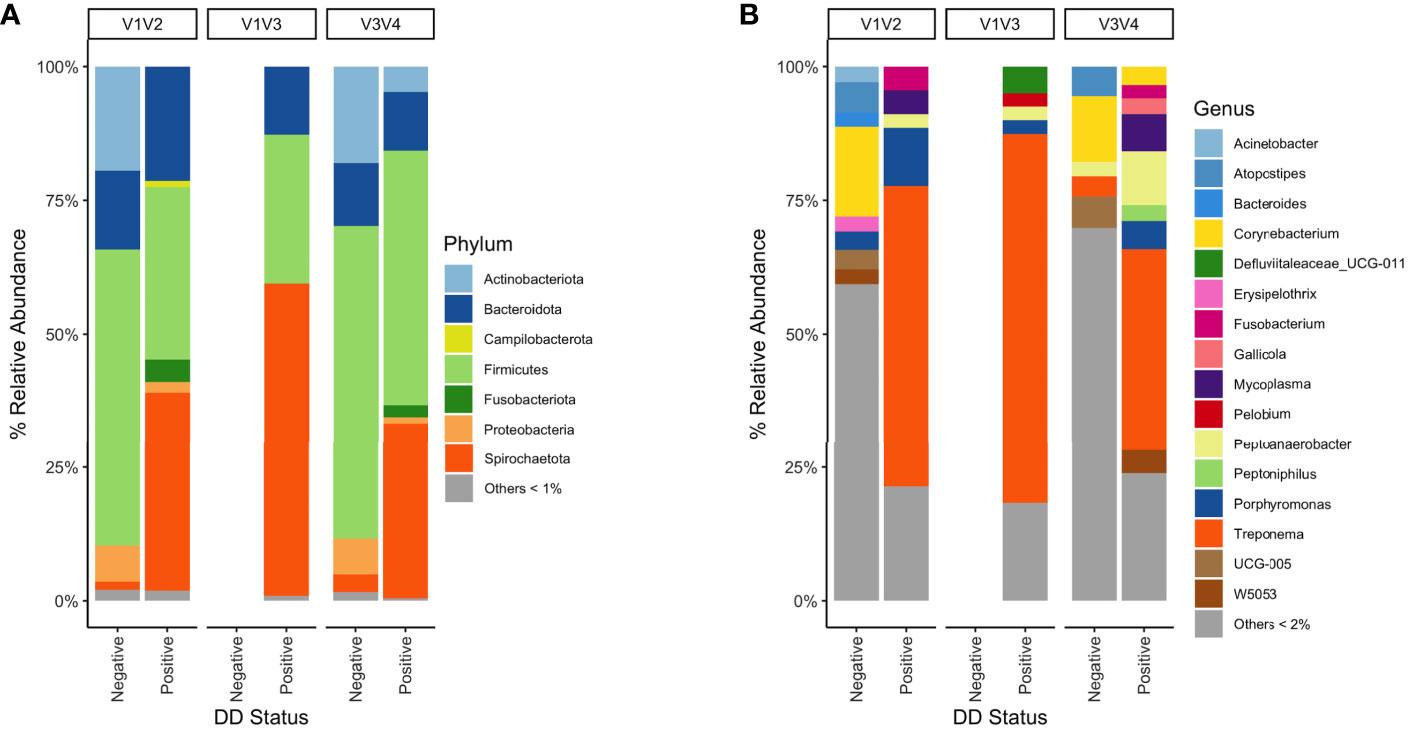
Figure 5 Mean percent relative abundance of DD negative skin and DD lesions from 16S rRNA hypervariable regions V1V2, V1V3, and V3V4. (A) Taxa were grouped at the phylum level, and all taxa that had less than 1% relative abundance were grouped. Taxa were grouped at the (B) genus level, and all taxa that had less than 2% relative abundance were grouped.
Discussion
In this meta-analysis, Treponema, Mycoplasma, Porphyromonas, and Fusobacterium were the genera that best differentiated DD positive skin from DD negative skin, indicating their possible role in DD pathogenesis. These genera were present in the majority of DD affected animals, and were relatively more abundant in DD positive skin microbiota compared to DD negative skin. Whereas DD-associated microbiota and conclusions on which bacteria may have a role in DD etiology is varied across literature, the findings of this study identified a relatively consistent bacterial consortium in DD affected skin, regardless of study variables and experimental design choices. We also identify a consistent DD-associated microbiota across dairy and beef cattle breeds, providing justification for extrapolating dairy DD knowledge to beef cattle DD.
Although it was not possible to identify any taxa in 100% of animals with DD, there are strong associations of Treponema, Mycoplasma, Fusobacterium, and Porphyromonas with DD positive skin. This core group of genera we identified agrees best with the outcome of two previous metagenomic studies (Nielsen et al., 2016; Caddey et al., submitted for publication), in which they identify the same microbiota that best differentiates DD lesions from healthy skin. We identified other bacterial groups that displayed strong associations with DD skin, mainly members of Firmicutes, but these were left out of the primary core group because of their relatively low rank in the random forest model and low relative abundance in DD positive skin. However, low abundant taxa can have the capability to modulate community structures as keystone taxa (Banerjee et al., 2018), and ignoring taxa because of their low abundance may result in an incomplete picture of DD pathogenesis. Although it is unknown if low abundance taxa play a role in DD microbial community structure formation, we know Dichelobacter nodosus drives pathogenesis of ovine foot rot and only has a relative abundance of less than 2% in diseased tissue (Maboni et al., 2017), so it is possible that low abundant taxa may contribute to DD pathogenesis.
Treponema has been the most common genus implied as an etiologic agent of DD. Consistent with this study, virtually every other metagenomic study of DD observes a higher relative abundance of Treponema in most DD lesions compared to normal skin (Krull et al., 2014; Zinicola et al., 2015b; Marcatili et al., 2016; Nielsen et al., 2016; Hesseling et al., 2019). Conversely, it is not uncommon to see some healthy skin samples to also have high proportions of at least one species of Treponema (Beninger et al., 2018; Caddey et al., submitted for publication). Currently, there is little knowledge of pathogenesis mechanisms of individual Treponema spp., but T. pedis, T. medium, and T. phagedenis are some of the most commonly identified across DD literature (Klitgaard et al., 2013; Beninger et al., 2018), and may have pathogenic potential as identified in murine infection models (Elliott et al., 2007; Arrazuria et al., 2020).
Mycoplasma are more recently implicated in DD etiology (Krull et al., 2014; Nielsen et al., 2016), and they have been identified in this meta-analysis as the best DD-associated genus at differentiating disease status. There is a lack of understanding of which Mycoplasma spp. are important to DD development, due to a lack of culture isolates or species-specific identification methods, thus we only have short 16S rRNA amplicon-based identifications which do not give a definitive species identification. The major focus in literature tends to be on M. fermentans in DD pathogenesis, whose presence has been determined by PCR (Nielsen et al., 2016) and by bacterial culture from DD (Wyss et al., 2005). No DD studies have identified an abundance or presence of M. bovis, a major pathogen of bovine respiratory disease (Griffin et al., 2010). The lack of type cultures of Mycoplasma spp. isolated from DD lesions impairs our ability to further understand their roles in DD pathogenesis.
There is previous literature showing Fusobacterium as differentiating DD lesions from healthy skin (Sullivan et al., 2015; Nielsen et al., 2016; Moreira et al., 2018), but is suggested to have a larger role in chronic lesions due to it being more prevalent in those lesions (Hesseling et al., 2019). We did not identify Fusobacterium in any of the samples from V1V3 amplification. This could suggest that Fusobacterium is not important for lesion formation, and perhaps impacts severity of lesions as a secondary invader. However, there could be potential confounding effects that may have caused Fusobacterium to be absent in V1V3 reads, such as that only active lesion stages were sequenced, or there could be potential primer mismatches with DD-specific strains resulting in poor amplification efficiency. Two different Fusobacterium spp. have been identified and are both present in the majority of DD lesions of beef cattle (Caddey et al., submitted for publication); therefore, interest should remain on Fusobacterium as a potential pathogenic agent of DD. Porphyromonas is also a possible contributor to DD pathogenesis that is detectable in all active lesions in one study (Caddey et al., submitted for publication), but along with Fusobacterium, its superficial location in the dermis has led some studies to limit their conclusions on its potential involvement (Moreira et al., 2018). However, in periodontal disease, which has similar higher level microbial community structure to DD, Porphyromonas plays a significant role in influencing the metabolic activity of Treponema species (Tan et al., 2014). Additionally, Porphyromonas and Fusobacterium species generate mixed-species biofilms as a mechanism to impair the bovine innate immune system (Lockhart et al., 2017). While it is not evident that the same microbial strategies are involved in DD pathogenesis, it provides significant motivation to identify interactions between DD-associated species to determine a framework of the synergistic mechanisms used as pathogenesis mechanisms.
From the key group of DD-associated bacteria we mentioned in this study, multiple species within Treponema, Porphyromonas, and Mycoplasma have been identified within DD microbiota (Zinicola et al., 2015b; Nielsen et al., 2016; Beninger et al., 2018). Due to the unreliability of species level classifications of the 16S rRNA hypervariable regions sequenced in this meta-analysis, comparisons at the species level were not performed (Johnson et al., 2019). Methods to reliably quantify species-level dynamics across DD lesions is essential to validating these metagenomic results, and account for differences in 16S rRNA copy number and primer specificity between individual taxa.
One of the more common species implicated in DD lesion formation, D. nodosus, the etiologic agent of ovine foot rot, were present in less than 30% of DD samples in this meta-analysis (Rasmussen et al., 2012; Krull et al., 2014; Moreira et al., 2018). Insufficient amplification of D. nodosus isolates through one pair of universal 16S rRNA primers has been shown (Calvo-Bado et al., 2011), and could be a potential reason for D. nodosus absence in the majority of samples in this study. Dichelobacter nodosus is primarily suggested to have a potential role in early establishment of DD lesions, and then appears relatively rare in later lesion stages (Rasmussen et al., 2012; Krull et al., 2014), which could explain our inability to identify D. nodosus as clinically relevant. Further investigations of D. nodosus populations is warranted for early DD lesions, in order to characterize its potential role in epithelial infiltration and facilitation of skin colonization. Some studies not included in this meta-analysis have identified bacteria that show strong associations to DD lesion microbiota, such as Candidatus Aemobophilus asiaticus, which was present in large abundance in two studies (Zinicola et al., 2015a; Zinicola et al., 2015b), but not detected in this meta-analysis. Similarly, Guggenheimella bovis has been implicated in a potential DD pathogenesis role (Schlafer et al., 2008) but also was not identifiable in this meta-analysis.
Machine learning classifiers can help shed light on the complexity of polymicrobial infections that have major individual variation. These models appraise individual bacterial population dynamics with respect to overall community structure instead of the traditional statistical approach which primarily study individual taxa in isolation (Topçuoğlu et al., 2020). Random forest models provide a relative variable importance ranking, and in this meta-analysis, Mycoplasma was the best DD-associated bacteria at differentiating DD status, instead of Treponema, which is historically the most often group implicated in DD etiology. The relative variable importance is not a function of biological relevance of each organism, but rather scores variables based on their reduction of randomness in model prediction, and therefore we cannot conclude a relative ranking among DD-associated bacteria in their importance to DD pathogenesis.
Each of the studies included in this meta-analysis had significant differences in the microbial compositions of their samples based on PERMANOVA analysis. However, this could be due to the fact there was significant heterogenous dispersion between groups, so we cannot conclude that there are significant differences in the microbial composition between studies and study variables. The skin microbial composition and diversity of DD lesions is quite variable across individuals (Moreira et al., 2018), which is one of the reasons why it has been difficult to pin down a causative agent. This individual animal variation may be causing the heterogenous dispersions among groups tested for study bias.
In our meta-analysis, there were 2 studies that contained two-thirds of the total samples. These large studies may have skewed our results, but that is unlikely, given that our principal coordinates analysis appeared to show more diversity within studies than between studies, and it’s likely that the massive variation in Treponema abundance between samples was the source of this variation within studies. Observing the bacterial population differences between the 16S rRNA hypervariable regions identified minimal differences in the core bacterial group identified as potential DD pathogens, furthering evidence suggesting that these two large studies did not skew our results. An additional concern in study bias includes the significantly greater diversity in samples from V3V4 16S rRNA hypervariable regions compared to both V1V2 and V1V3. There are at least two potential reasons for this: 1) the samples that used V3V4 sequencing were also the only samples to include skin samples from beef cattle breeds rather than just dairy breeds; 2) V3V4 primers may amplify a wider range of bovine DD skin microbiota. DD in beef cattle is a recent field of study, as DD is emerging in those populations (Orsel et al., 2018), and since dairy and beef animals have separate housing environments and genetics, there is potential for their skin microbiota to have significant differences.
This meta-analysis has identified, through a consistent analytical approach of skin microbiota across multiple studies, that Treponema, Mycoplasma, Porphyromonas, and Fusobacterium were key genera that differentiated DD lesions from normal skin. These genera are consistently associated with the majority of skin samples of DD lesions. Based on abundance data, Mycoplasma best differentiates DD lesions from normal skin and should be a priority focus in future research on DD pathogenesis. Treponema are the most abundant bacteria in DD, but have also been identified in low amounts in most DD negative skin samples. Further analysis on the individual species of Treponema, as well as species of the other potential DD pathogens, is warranted to further understand their roles in DD lesions. In addition, longitudinal analysis of bacterial population dynamics throughout DD lesion progression would aid in elucidating potential causative roles of the DD-associated bacteria identified in this meta-analysis. This study is an accumulation of current understanding of DD microbiology, and provides strong evidence to standardize future research to focus primarily on the potential DD pathogens identified in this study.
Data Availability Statement
The original contributions presented in the study are included in the article/Supplementary Material. Further inquiries can be directed to the corresponding author.
Author Contributions
BC and JB conceptualized and designed the study. BC collected sequencing data and performed all data analysis. BC wrote the manuscript and JB edited and reviewed the manuscript. All authors contributed to the article and approved the submitted version.
Funding
Funding was supplied by Alberta Agriculture and Forestry (grant number 2018F129R), the Canadian Dairy Commission under the Workplace Development Initiative, and the Simpson Ranch Grant at the University of Calgary Faculty of Veterinary Medicine.
Conflict of Interest
The authors declare that the research was conducted in the absence of any commercial or financial relationships that could be construed as a potential conflict of interest.
Supplementary Material
The Supplementary Material for this article can be found online at: https://www.frontiersin.org/articles/10.3389/fcimb.2021.685861/full#supplementary-material
References
Arrazuria, R., Knight, C. G., Lahiri, P., Cobo, E. R., Barkema, H. W., De Buck, J. (2020). Treponema Spp. Isolated From Bovine Digital Dermatitis Display Different Pathogenicity in a Murine Abscess Model. Microorganisms 8, 1507. doi: 10.3390/microorganisms8101507
Banerjee, S., Schlaeppi, K., van der Heijden, M. G. A. (2018). Keystone Taxa as Drivers of Microbiome Structure and Functioning. Nat. Rev. Microbiol. 16, 567–576. doi: 10.1038/s41579-018-0024-1
Beninger, C., Naqvi, S. A., Naushad, S., Orsel, K., Luby, C., Derakhshani, H., et al. (2018). Associations Between Digital Dermatitis Lesion Grades and the Quantities of Four Treponema Species. Vet. Res. 49, 111. doi: 10.1186/s13567-018-0605-z
Berry, S. L., Read, D. H., Famula, T. R., Mongini, A., Döpfer, D. (2012). Long-Term Observations on the Dynamics of Bovine Digital Dermatitis Lesions on a California Dairy After Topical Treatment With Lincomycin HCl. Vet. J. 193, 654–658. doi: 10.1016/j.tvjl.2012.06.048
Bran, J. A., Daros, R. R., von Keyserlingk, M. A. G., LeBlanc, S. J., Hötzel, M. J. (2018). Cow- and Herd-Level Factors Associated With Lameness in Small-Scale Grazing Dairy Herds in Brazil. Prev. Vet. Med. 151, 79–86. doi: 10.1016/j.prevetmed.2018.01.006
Callahan, B. J., McMurdie, P. J., Holmes, S. P. (2017). Exact Sequence Variants Should Replace Operational Taxonomic Units in Marker-Gene Data Analysis. ISME J. 11, 2639–2643. doi: 10.1038/ismej.2017.119
Callahan, B. J., Mcmurdie, P. J., Rosen, M. J., Han, A. W., Johnson, A. J. A., Holmes, S. P. (2016). DADA2: High-Resolution Sample Inference From Illumina Amplicon Data. Nat. Methods 13, 581–583. doi: 10.1038/nmeth.3869.DADA2
Calvo-Bado, L. A., Oakley, B. B., Dowd, S. E., Green, L. E., Medley, G. F., Ul-Hassan, A., et al. (2011). Ovine Pedomics: The First Study of the Ovine Foot 16S rRNA-Based Microbiome. ISME J. 5, 1426–1437. doi: 10.1038/ismej.2011.25
Cheli, R., Mortellaro, C. (1974). La dermatite digitale del bovino. Proceedings of the 8th International Conference on Diseases of Cattle. Piacenza, Milan, Italy. p. 208–213.
Davis-Unger, J., Pajor, E., Schwartzkopf-Genswein, K., Marti, S., Dorin, C., Spackman, E., et al. (2017). Economic Impacts of Lameness in Feedlot Cattle. Transl. Anim. Sci. 1, 467–479. doi: 10.2527/tas2017.0052
Dixon, P. (2003). VEGAN, a Package of R Functions for Community Ecology. J. Veg. Sci. 14, 927–930. doi: 10.1111/j.1654-1103.2003.tb02228.x
Dolecheck, K. A., Overton, M. W., Mark, T. B., Bewley, J. M. (2019). Use of a Stochastic Simulation Model to Estimate the Cost Per Case of Digital Dermatitis, Sole Ulcer, and White Line Disease by Parity Group and Incidence Timing. J. Dairy Sci. 102, 715–730. doi: 10.3168/jds.2018-14901
Döpfer, D., Koopmans, A., Meijer, F. A., Szakáll, I., Schukken, Y. H., Klee, W., et al. (1997). Histological and Bacteriological Evaluation of Digital Dermatitis in Cattle, With Special Reference to Spirochaetes and Campylobacter Faecalis. Vet. Rec. 140, 620–623. doi: 10.1136/vr.140.24.620
Elliott, M. K., Alt, D. P., Zuerner, R. L. (2007). Lesion Formation and Antibody Response Induced by Papillomatous Digital Dermatitis-Associated Spirochetes in a Murine Abscess Model. Infect. Immun. 75, 4400–4408. doi: 10.1128/IAI.00019-07
Gomez, A., Cook, N. B., Socha, M. T., Döpfer, D. (2015). First-Lactation Performance in Cows Affected by Digital Dermatitis During the Rearing Period. J. Dairy Sci. 98, 4487–4498. doi: 10.3168/jds.2014-9041
Goodrich, J. K., Di Rienzi, S. C., Poole, A. C., Koren, O., Walters, W. A., Caporaso, J. G., et al. (2014). Conducting a Microbiome Study. Cell 158, 250–262. doi: 10.1016/j.cell.2014.06.037
Gotoh, Y., Chiba, K., Sekiyama, Y., Okada, K., Hayashi, T., Misawa, N. (2020). 16s rRNA-Based Amplicon Analysis of Changes in the Bacterial Population in the Lesions of Papillomatous Digital Dermatitis in Dairy Cattle After Topical Treatment With Allyl Isothiocyanate. Microbiol. Immunol. 64, 416–423. doi: 10.1111/1348-0421.12786
Griffin, D., Chengappa, M. M., Kuszak, J., McVey, D. S. (2010). Bacterial Pathogens of the Bovine Respiratory Disease Complex. Vet. Clin. North Am. Food Anim. Pract. 26, 381–394. doi: 10.1016/j.cvfa.2010.04.004
Hesseling, J., Legione, A. R., Stevenson, M. A., McCowan, C. I., Pyman, M. F., Finochio, C., et al. (2019). Bovine Digital Dermatitis in Victoria, Australia. Aust. Vet. J. 97, 404–413. doi: 10.1111/avj.12859
Holzhauer, M., Hardenberg, C., Bartels, C. J. M., Frankena, K. (2006). Herd- and Cow-Level Prevalence of Digital Dermatitis in the Netherlands and Associated Risk Factors. J. Dairy Sci. 89, 580–588. doi: 10.3168/jds.S0022-0302(06)72121-X
Jacobs, C., Orsel, K., Mason, S., Barkema, H. W. (2018). Comparison of Effects of Routine Topical Treatments in the Milking Parlor on Digital Dermatitis Lesions. J. Dairy Sci. 101, 5255–5266. doi: 10.3168/jds.2017-13984
Johnson, J. S., Spakowicz, D. J., Hong, B. Y., Petersen, L. M., Demkowicz, P., Chen, L., et al. (2019). Evaluation of 16S rRNA Gene Sequencing for Species and Strain-Level Microbiome Analysis. Nat. Commun. 10, 5029. doi: 10.1038/s41467-019-13036-1
Klitgaard, K., Boye, M., Capion, N., Jensen, T. K. (2008). Evidence of Multiple Treponema Phylotypes Involved in Bovine Digital Dermatitis as Shown by 16S rRNA Gene Analysis and Fluorescence In Situ Hybridization. J. Clin. Microbiol. 46, 3012–3020. doi: 10.1128/JCM.00670-08
Klitgaard, K., Bretó, A. F., Boye, M., Jensen, T. K. (2013). Targeting the Treponemal Microbiome of Digital Dermatitis Infections by High-Resolution Phylogenetic Analyses and Comparison With Fluorescent in Situ Hybridization. J. Clin. Microbiol. 51, 2212–2219. doi: 10.1128/JCM.00320-13
Krull, A. C., Shearer, J. K., Gorden, P. J., Cooper, V. L., Phillips, G. J., Plummera, P. J. (2014). Deep Sequencing Analysis Reveals Temporal Microbiota Changes Associated With Development of Bovine Digital Dermatitis. Infect. Immun. 82, 3359–3373. doi: 10.1128/IAI.02077-14
Krull, A. C., Shearer, J. K., Gorden, P. J., Scott, H. M., Plummer, P. J. (2016). Digital Dermatitis: Natural Lesion Progression and Regression in Holstein Dairy Cattle Over 3 Years. J. Dairy Sci. 99, 3718–3731. doi: 10.3168/jds.2015-10535
Kuhn, M. (2008). Building Predictive Models in R Using the Caret Package. J. Stat. Software 28, 1–26. doi: 10.18637/jss.v028.i05
Kulow, M., Merkatoris, P., Anklam, K. S., Rieman, J., Larson, C., Branine, M., et al. (2017). Evaluation of the Prevalence of Digital Dermatitis and the Effects on Performance in Beef Feedlot Cattle Under Organic Trace Mineral Supplementation. J. Anim. Sci. 95, 3435–3444. doi: 10.2527/jas.2017.1512
Langmead, B., Salzberg, S. L. (2012). Fast Gapped-Read Alignment With Bowtie 2. Nat. Methods 9, 357–359. doi: 10.1038/nmeth.1923
Lockhart, J. S., Buret, A. G., Ceri, H., Storey, D. G., Anderson, S. J., Morck, D. W. (2017). Mixed Species Biofilms of Fusobacterium Necrophorum and Porphyromonas Levii Impair the Oxidative Response of Bovine Neutrophils In Vitro. Anaerobe 47, 157–164. doi: 10.1016/j.anaerobe.2017.05.008
Maboni, G., Blanchard, A., Frosth, S., Stewart, C., Emes, R., Tötemeyer, S. (2017). A Distinct Bacterial Dysbiosis Associated Skin Inflammation in Ovine Footrot. Sci. Rep. 7, 45220. doi: 10.1038/srep45220
Marcatili, P., Nielsen, M. W., Sicheritz-Pontén, T., Jensen, T. K., Schafer-Nielsen, C., Boye, M., et al. (2016). A Novel Approach to Probe Host-Pathogen Interactions of Bovine Digital Dermatitis, a Model of a Complex Polymicrobial Infection. BMC Genomics 17, 987. doi: 10.1186/s12864-016-3341-7
Moreira, T. F., Facury Filho, E. J., Carvalho, A. U., Strube, M. L., Nielsen, M. W., Klitgaard, K., et al. (2018). Pathology and Bacteria Related to Digital Dermatitis in Dairy Cattle in All Year Round Grazing System in Brazil. PLoS One 13, e0193870. doi: 10.1371/journal.pone.0193870
Nielsen, M. W., Strube, M. L., Isbrand, A., Al-Medrasi, W. D. H. M., Boye, M., Jensen, T. K., et al. (2016). Potential Bacterial Core Species Associated With Digital Dermatitis in Cattle Herds Identified by Molecular Profiling of Interdigital Skin Samples. Vet. Microbiol. 186, 139–149. doi: 10.1016/j.vetmic.2016.03.003
Orsel, K., Plummer, P., Shearer, J., De Buck, J., Carter, S. D., Guatteo, R., et al. (2018). Missing Pieces of the Puzzle to Effectively Control Digital Dermatitis. Transbound Emerg. Dis. 65, 186–198. doi: 10.1111/tbed.12729
Quast, C., Pruesse, E., Yilmaz, P., Gerken, J., Schweer, T., Yarza, P., et al. (2013). The SILVA Ribosomal RNA Gene Database Project: Improved Data Processing and Web-Based Tools. Nucleic Acids Res. 41, 590–596. doi: 10.1093/nar/gks1219
Rasmussen, M., Capion, N., Klitgaard, K., Rogdo, T., Fjeldaas, T., Boye, M., et al. (2012). Bovine Digital Dermatitis: Possible Pathogenic Consortium Consisting of Dichelobacter Nodosus and Multiple Treponema Species. Vet. Microbiol. 160, 151–161. doi: 10.1016/j.vetmic.2012.05.018
Read, D. H., Walker, R. L. (1998). Papillomatous Digital Dermatitis (Footwarts) in California Dairy Cattle: Clinical and Gross Pathologic Findings. J. Vet. Diagn. Investig. 10, 67–76. doi: 10.1177/104063879801000112
Santos, T. M. A., Pereira, R. V., Caixeta, L. S., Guard, C. L., Bicalho, R. C. (2012). Microbial Diversity in Bovine Papillomatous Digital Dermatitis in Holstein Dairy Cows From Upstate New York. FEMS Microbiol. Ecol. 79, 518–529. doi: 10.1111/j.1574-6941.2011.01234.x
Schlafer, S., Nordhoff, M., Wyss, C., Strub, S., Hübner, J., Gescher, D. M., et al. (2008). Involvement of Guggenheimella Bovis in Digital Dermatitis Lesions of Dairy Cows. Vet. Microbiol. 128, 118–125. doi: 10.1016/j.vetmic.2007.09.024
Solano, L., Barkema, H. W., Mason, S., Pajor, E. A., LeBlanc, S. J., Orsel, K. (2016). Prevalence and Distribution of Foot Lesions in Dairy Cattle in Alberta, Canada. J. Dairy Sci. 99, 6828–6841. doi: 10.3168/jds.2016-10941
Sullivan, L. E., Evans, N. J., Blowey, R. W., Grove-White, D. H., Clegg, S. R., Duncan, J. S., et al. (2015). A Molecular Epidemiology of Treponemes in Beef Cattle Digital Dermatitis Lesions and Comparative Analyses With Sheep Contagious Ovine Digital Dermatitis and Dairy Cattle Digital Dermatitis Lesions. Vet. Microbiol. 178, 77–87. doi: 10.1016/j.vetmic.2015.04.011
Tan, K. H., Seers, C. A., Dashper, S. G., Mitchell, H. L., Pyke, J. S., Meuric, V., et al. (2014). Porphyromonas Gingivalis and Treponema Denticola Exhibit Metabolic Symbioses. PLoS Pathog. 10, e1003955. doi: 10.1371/journal.ppat.1003955
Topçuoğlu, B. D., Lesniak, N. A., Ruffin, M. T., Wiens, J., Schloss, P. D. (2020). A Framework for Effective Application of Machine Learning to Microbiome-Based Classification Problems. MBio 11, e00434–e00420. doi: 10.1101/816090
Wyss, C., Dewhirst, F. E., Paster, B. J., Thurnheer, T., Luginbühl, A. (2005). Guggenheimella Bovis Gen. Nov., Sp. Nov., Isolated From Lesions of Bovine Dermatitis Digitalis. Int. J. Syst. Evol. Microbiol. 55, 667–671. doi: 10.1099/ijs.0.63116-0
Yang, D. A., Johnson, W. O., Müller, K. R., Gates, M. C., Laven, R. A. (2019). Estimating the Herd and Cow Level Prevalence of Bovine Digital Dermatitis on New Zealand Dairy Farms: A Bayesian Superpopulation Approach. Prev. Vet. Med. 165, 76–84. doi: 10.1016/j.prevetmed.2019.02.014
Yano, T., Moe, K. K., Yamazaki, K., Ooka, T., Hayashi, T., Misawa, N. (2010). Identification of Candidate Pathogens of Papillomatous Digital Dermatitis in Dairy Cattle From Quantitative 16S rRNA Clonal Analysis. Vet. Microbiol. 143, 352–362. doi: 10.1016/j.vetmic.2009.12.009
Zinicola, M., Higgins, H., Lima, S., Machado, V., Guard, C., Bicalho, R. (2015a). Shotgun Metagenomic Sequencing Reveals Functional Genes and Microbiome Associated With Bovine Digital Dermatitis. PLoS One 10, e0133674. doi: 10.1371/journal.pone.0133674
Keywords: cattle, digital dermatitis, microbiota, skin microbiome, Treponema, meta-analysis
Citation: Caddey B and De Buck J (2021) Meta-Analysis of Bovine Digital Dermatitis Microbiota Reveals Distinct Microbial Community Structures Associated With Lesions. Front. Cell. Infect. Microbiol. 11:685861. doi: 10.3389/fcimb.2021.685861
Received: 27 March 2021; Accepted: 16 June 2021;
Published: 16 July 2021.
Edited by:
Paul Plummer, Iowa State University, United StatesReviewed by:
David Alt, United States Department of Agriculture (USDA), United StatesMichelle B. Visser, University at Buffalo, United States
Copyright © 2021 Caddey and De Buck. This is an open-access article distributed under the terms of the Creative Commons Attribution License (CC BY). The use, distribution or reproduction in other forums is permitted, provided the original author(s) and the copyright owner(s) are credited and that the original publication in this journal is cited, in accordance with accepted academic practice. No use, distribution or reproduction is permitted which does not comply with these terms.
*Correspondence: Jeroen De Buck, jdebuck@ucalgary.ca