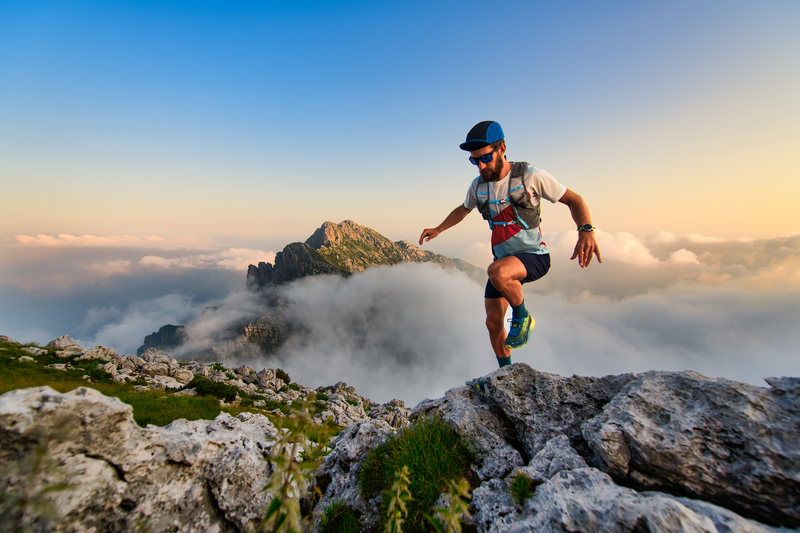
95% of researchers rate our articles as excellent or good
Learn more about the work of our research integrity team to safeguard the quality of each article we publish.
Find out more
PERSPECTIVE article
Front. Cell. Infect. Microbiol. , 31 July 2020
Sec. Microbiome in Health and Disease
Volume 10 - 2020 | https://doi.org/10.3389/fcimb.2020.00388
This article is part of the Research Topic New Techniques in Microbiome Research View all 12 articles
Even as the field of microbiome research has made huge strides in mapping microbial community composition in a variety of environments and organisms, explaining the phenotypic influences on the host by microbial taxa—both known and unknown—and their specific functions still remain major challenges. A pressing need is the ability to assign specific functions in terms of enzymes and small molecules to specific taxa or groups of taxa in the community. This knowledge will be crucial for advancing personalized therapies based on the targeted modulation of microbes or metabolites that have predictable outcomes to benefit the human host. This perspective article advocates for the combined use of standards-free metabolomics and activity-based protein profiling strategies to address this gap in functional knowledge in microbiome research via the identification of novel biomolecules and the attribution of their production to specific microbial taxa.
A number of studies have shown that the microbiome composition in an individual's gut and other body sites is inherently dynamic and changes over time due to many factors, such as dietary changes, medical interventions (e.g., antibiotic use), other environmental exposures, childhood maturation, normal aging, and illness. A widespread approach in microbiome research has been to associate different diseases with alterations in the microbiome (dysbiosis), but directionality is often unknown and it is not clear if these changes are causal or simply associative (Olesen and Alm, 2016). Fluctuations in community composition do not necessarily indicate changes in community function or metabolic activity (Whidbey et al., 2019). In order to be able to design microbiome-modulation based therapies to improve human health, a deeper functional knowledge is required and comprising of (A) complete biochemical characterization of microbiome metabolites, (B) the proteins involved in their production, conversion or transport, (C) the microbial populations responsible for producing, utilizing or otherwise interacting with these molecules, and (D) their effect on host physiology.
The Human Microbiome Project characterized the microbial communities present in multiple body habitats in a large cohort of healthy subjects with both 16S and shotgun metagenomic data. Although extensive variability was observed in the taxonomic diversity, metabolic pathways were evenly encoded across both individual and body habitats, revealing functional plasticity in these ecosystems (Human Microbiome Project Consortium, 2012). The variation between individuals can arise from a large number of co-varying factors (e.g., host lifestyle, diet, cultural habits, host genetics, age, disease states, maternal transmission, family members, local environment etc.), (Schmidt et al., 2018). Population based analyses have shown that known factors that correlate with shifts in microbiome composition and structure collectively explain only a fraction of the interindividual variance (Falony et al., 2016; Zhernakova et al., 2016), underscoring the complexity of molecular mechanisms that likely govern host-microbiome interactions. The chemical space spanning these interactions is massive, dynamically changing and shaped by multiple, often confounding, factors. Humans are continually exposed to a large number of substances that are foreign to the body. Human milk is an important first “exposure” for breast-fed infants, rich in biologically active components (oligosaccharides, hormones, lipids etc.) and harboring its own microbiome. The milk microbiome has recently attracted much scientific attention, given its role in early establishment of the infant gut microbiome and maternal health (McGuire and McGuire, 2017; Ramani et al., 2018; Moossavi et al., 2019). Other exposures include a variety of chemical compounds present in the foods we eat and xenobiotics such as pharmaceutical drugs, cosmetics, and environmental pollutants. Some of these molecules that may not be bioactive in their original form make their way through the digestive tract and are bio-transformed by the gut microbiota into products that are biologically active and may have a beneficial or detrimental effect to the host. Enterohepatic circulation of drugs, bile acids, and other chemicals through biliary excretion, gut microbial biotransformation, and intestinal reabsorption can result in altered pharmacology and toxicology (Klaassen and Cui, 2015; Winston and Theriot, 2020). Many commonly prescribed drugs are known to be metabolically altered by the microbiome, significantly impacting their biological activity. Thus, the interindividual variability in gut microbial composition means that a drug's efficacy or toxicity can vary depending on an individual's unique microbiome.
Microorganisms interact with each other and the host physiology via small molecule metabolites. These include exogeneous small molecules, metabolites produced by the host, microbial biotransformation products and molecules synthesized de novo by the microbes. Metabolomics is a powerful tool for characterizing the diverse array of small molecule metabolites that take part in the complex interplay between the microbiota, host, and environment.
In addition to direct characterization of microbial metabolites, which are the downstream products of metabolism, it is imperative to link these metabolites back to enzymes and other functional proteins expressed by the microbiota and that interact with these molecules in some fashion. Although many core functions can be performed by a number of different microbial members of the community (functional redundancy), other specialized functions have been attributed to specific taxa. For example, the Cgr2 protein from a single species in the gut, Eggerthella lenta has been found to inactivate digoxin, a plant toxin, and a cardiac drug (Koppel et al., 2018). Speculations about the genesis of microbial metabolites can be made through employing conventional omics approaches such as metagenomics, metatranscriptomics, global metaproteomics, and metabolomics, to find correlative relationships, but abundance measurements do not establish a direct functional connection. Distinguishing active populations within the microbiome is important from a metabolic perspective because there may be microbial candidates that have all the prerequisites for a given activity, but conventional methods cannot determine if the system is functionally competent. The value of function based approaches is illustrated in the case of E. lenta where the mere presence of the microbe in the gut was not found to correlate with levels of drug inactivation (Saha et al., 1983; Haiser et al., 2013). Chemoproteomic tools that require activity for a protein to appear in the final readout can be used to investigate the functionally active proteome.
Next generation standards-free metabolomics can provide comprehensive coverage of the metabolome from a variety of sample types including feces, blood plasma, milk etc. Candidate metabolite features that are capable of indirectly or directly modulating the host phenotype can be selected from the metabolome using a variety of strategies that include statistical significance using comparative study design, pathway, and systems analysis (Guijas et al., 2018). Standards-free metabolomics can enable comprehensive, putative compound identification, greatly accelerating the selection of metabolites implicated in a disease state for example, which have shown strong correlation to dysbiosis in the microbiome, indicating their possible involvement in causing the host phenotype. These molecules are then targets for activity-based probe design for use in profiling aliquots of the same or similar samples to identify the microbes that make the enzymes or transporters that act on the molecules of interest. One can envision that a similar workflow could be used to understand the effect of a drug or a dietary compound of interest on the microbiome and host, where activity-based probes can be custom-designed for the drug and standards-free metabolomics along with molecular networking strategies can be used to profile downstream products of microbial and host metabolism of the compound. In this perspective review, we provide a detailed vision for the integration of metabolomics and activity-based protein profiling for identifying novel molecules in microbiomes and the organisms responsible for their synthesis or metabolism.
An in-depth understanding of the human microbiome's effect on host physiology at the biomolecular level will require tools to predict and measure molecules metabolized by the microbes. Untargeted mass spectrometry-based metabolomics measurements enable thousands of metabolite signals to be measured from a sample, which is helpful when investigating a very diverse and largely uncharacterized chemical space like the human gut. Comprehensive compound identification is a significant, long-standing bottleneck faced by the metabolomics community. Accurate identification of small molecule structures will be fundamental to understanding the role of various metabolites in modulating biological processes in the microbes and the host. In recent years, there has been growing interest in integrating ion mobility spectrometry (IMS) into current MS-based analytical methods (Lanucara et al., 2014; May and McLean, 2015; Paglia et al., 2015; Metz et al., 2017; Dodds and Baker, 2019). Using a multidimensional analytical platform such as LC-IMS-MS/MS, which combines IMS and tandem mass spectrometry, not only provides improved separation and dynamic range of detection but also gives the user an additional dimension of structural information for high confidence identifications. IMS is capable of separating stereoisomers and isobaric compounds and measures the physical-chemical property of collision cross section (CCS), which has been shown to be highly reproducible (Stow et al., 2017). For features detected in the human microbiome, for example, defined by relative retention time, CCS, m/z, and mass fragmentation patterns in LC-IMS-MS/MS, a putative identification can be made, or a candidate list narrowed, using reference values of known molecules (Paglia and Astarita, 2017; King et al., 2019; Nuñez et al., 2019). Armed with multiple pieces of experimental information on an unknown molecule, the next step is to query in-house reference libraries. Traditionally, these have been determined experimentally through analyses of authentic reference materials: purified and concentrated compounds of interest are analyzed for relevant chemical properties (Castle et al., 2006; Sumner et al., 2007). The number of standards that can be analyzed by any single laboratory is inherently limited due to a variety of reasons including cost, availability of authentic reference materials, and instrument time. As a work-around, commercial reference libraries and freely available online spectral databases exist to aid researchers in metabolite identification. However, even as databases with reference spectra continue to grow, the metabolome coverage represented is still only a fraction of all the possible molecules that can be detected in biological and environmental samples. Building these reference libraries experimentally is slow and expensive, particularly when considering chemical space has been estimated to contain up to 1060 unique molecules (Dobson, 2004). Community wide sharing and curation of metabolomics data and associated metadata, reference databases, computational tool development, and knowledge dissemination will continue to be crucial for accelerating metabolomics research (Wang et al., 2016, 2020; Picache et al., 2020) but the challenge of identifying unknown molecules, especially those for which reference standards do not exist, remains a major roadblock. As a result, there has been growing interest in what has been termed “standards free” approaches, wherein reference values are determined through in silico methods, including quantum chemical simulations (Paglia et al., 2014; Yesiltepe et al., 2018; Colby et al., 2019), machine learning (Allen et al., 2014; Hufsky et al., 2014; Dührkop et al., 2015; Wolfer et al., 2016; Zhou et al., 2016; Zhou Z. et al., 2017; Zhou Z.W. et al., 2017; Bach et al., 2018), deep learning (Gómez-Bombarelli et al., 2018; Kang and Cho, 2018; Colby et al., 2020), and quantitative structure-activity/property relationship (QSAR/QSPR) models (Wong and Burkowski, 2009; Schneider and Schneider, 2016; Miyao et al., 2017). These approaches dramatically accelerate the library-building process, enabling reference libraries that are orders-of-magnitude larger than those created from analysis of authentic reference material. For example, the IMS-derived molecular property of CCS has been experimentally determined for only 1,884 unique molecules (Colby et al., 2019). In silico methods, by comparison, have yielded a predicted CCS library containing over 53 million molecules (Colby et al., 2020).
Compared to experimental reference values, the error inherent in in silico predictions does pose limitations to comprehensive, unambiguous identification. However, appropriately modeling this error, as well as the error associated with experimental measurements, enables significant downselection to candidate lists amenable to verification by authentic standards. Further, by leveraging libraries of much broader chemical space coverage, putative matches carry better approximations of false discovery, an until recently ignored metric among the metabolomics community with potentially problematic ramifications (Scheubert et al., 2017; Wang et al., 2018).
Though standards-free approaches to identification in metabolomics studies improve chemical space coverage, and by extension estimates of false discovery rates, libraries are still limited to known chemical space, or, as of this publication, 168 billion molecules, determined from the union of all publicly available databases, including ChEBI, ChEMBL, Enamine, PubChem, UNPD, HMDB, DSSTox, ZINC, KEGG, and GDB17, among others. The “chemical dark matter” that remains is uncharacterizable by techniques discussed thus far. Instead, “library free” approaches, wherein molecular structures can be predicted directly from experimental signatures (i.e., inverse-QSAR/QSPR), must be employed for these “unknown unknowns.” For example, SIRIUS 4 is able to predict chemical structure from mass fragmentation pattern without referencing a database (Dührkop et al., 2019). In addition, nascent advances in generative deep learning approaches have shown promise in Arnold et al. (2016) library free identification (Kadurin et al., 2017a,b; Blaschke et al., 2018; Dai et al., 2018; De Cao and Kipf, 2018; Gómez-Bombarelli et al., 2018; Gupta et al., 2018; Jin et al., 2018; Kang and Cho, 2018; Kim et al., 2018; Lim et al., 2018; Merk et al., 2018; Colby et al., 2020).
In addition, the use of in silico metabolism prediction tools will be valuable in expanding the aforementioned databases or chemical search-space to include putative biotransformations of metabolites that result from human or gut microbial metabolism of xenobiotic compounds (Djoumbou-Feunang et al., 2019). Computational strategies that access biosynthetic gene clusters encoded in metagenomic data will be useful for guiding the discovery of novel small molecules from the microbiome (Sugimoto et al., 2019).
The need to provide direct attribution of microbiota-derived metabolites to specific taxa has brought activity-based protein profiling (ABPP) to the forefront of microbiome science (Whidbey and Wright, 2019; Keller et al., 2020). ABPP exclusively selects for active proteins through function-dependent covalent labeling with small molecule activity-based probes (ABPs), (Cravatt et al., 2008). The ABP-labeled proteins can be subsequently analyzed using mass-spectrometry methods, SDS-PAGE, and live-cell imaging. A new ABP may be hypothetically tailored for any metabolic protein of interest by taking advantage of chemical reactivity or physical binding interactions. The modular nature of an ABP allows for flexibility in post-labeling analysis. ABPP will be an indispensable tool in understanding how microbiome metabolism modulates the host response to external factors, such as diet or environmental exposures.
ABPP acts as a complementary strategy to metaproteomics by circumventing some of the challenges for its application (Heyer et al., 2017; Lee et al., 2017). Metaproteomic profiling provides more functional clues than metagenomics or metatranscriptomics, but the current technologies are not as sensitive as sequencing-based methods. As a result, metaproteomic approaches are biased toward highly abundant proteins, which leaves significant gaps in knowledge. This is particularly relevant to microbiome samples, where certain important taxa are often underrepresented in a population. Sample fractionation and two-dimensional chromatography (capillary and microchip electrophoresis) have been employed to reduce sample complexity (Leary et al., 2013; Tanca et al., 2015; Xiong et al., 2015; Stepanova and Kasicka, 2016). However, this enrichment is costly and thereby limits the number of samples that can be analyzed, and biases the results due to the loss of information (Tanca et al., 2015). However, even with advances in sensitivity, metaproteomic profiles still cannot definitively determine the proportion of the functionally active proteome because many proteins require cofactors, substrates, and post-translational modifications to be functionally active. Because ABPP is inherently an enrichment strategy, it simultaneously retains low abundance proteins and acts as a method to distinguish activity. However, integrating abundance and activity profiles provides a rich representation of the proteome. For example, Wolan and coworkers have shown the potential of coupling ABPP and stable isotope labeling for the enrichment of targeted human and microbial proteins for metaproteomics study in colitis or inflammatory bowel disease mouse model (Mayers et al., 2017).
ABPP also promises to resolve the problem of poorly annotated metagenomes that plagues metaproteomic analyses. It is estimated that 40–70% of the protein coding genes of the human microbiome cannot currently be predicted (Prestat et al., 2014). This problem is exacerbated when the genes come from poorly characterized taxa (uncultured taxa can be up to 40% of metagenomic data). In many cases, genes of unknown function are excluded from analysis because a method that requires mapping to an annotated genome is employed. ABPP can help address this challenge, as ABP-labeling directly signifies protein function (Adam et al., 2004; Kamat et al., 2015; Xu et al., 2015; Martell et al., 2016; Ortega et al., 2018; Elahi et al., 2019). This is especially true when accompanying metabolomics data can provide added confidence (Jansen et al., 2020). ABPP can also identify viable whole cells in complex samples based on a certain function without needing any information beforehand, which may aid in the study of un-culturable microbes with poorly characterized genomes. ABPP has been coupled with fluorescence-assisted cell sorting (FACS) to identify and isolate single cell population responsible for enzyme activity (Whidbey et al., 2019). Hence, combining FACS with ABPP allows for the enrichment of functionally active cells from microbiome with reduced sample complexity. Moreover, these sorted cells can be submitted for further analysis using other omics techniques with a less complex system (Jansson and Baker, 2016).
In addition to the technical advantages of ABPP, the gut microbiome offers many opportunities for exciting conceptual applications because of the diverse chemical transformations mediated exclusively by microbes and their corresponding enzymes and transporters. Host enzymes primarily perform oxidative and conjugative reactions leading to hydrophilic and higher molecular weight metabolites for elimination. In contrast, microbial enzymes typically use reductive and hydrolytic metabolisms to facilitate microbial growth. Many of these reactions can be beneficial for the host, such as the breakdown of plant polysaccharides (indigestible by host enzymes) by complex carbohydrate-active enzymes (>5,000), (El Kaoutari et al., 2013), which can result in the formation of short-chain fatty acid products that can positively influence host health (Rios-Covian et al., 2016). Application of ABPs based on carbohydrates to delineate the role of fiber diet on gut microbiome has a tremendous potential to find robust probiotics in the future (Chauvigne-Hines et al., 2012; Wu et al., 2019).
Function-based profiling will undoubtedly aid in understanding how microbiome activity can bolster host resilience, but it will also be useful for comprehending how it can conversely increase susceptibility to disease. For example, the microbiome produces proteins that degrade the host-produced complex polysaccharide, mucin, whose deregulation is linked to ulcerative colitis (Pullan et al., 1994), and this process has been successfully characterized using ABPP (Tsai et al., 2013; Thuy-Boun and Wolan, 2019). ABPs have also been applied to study the microbiome's modification of other host-produced metabolites, such as bile salts (Zhuang et al., 2017; Parasar et al., 2019), which have implications in the onset of diseases including cholestatic and inflammatory diseases, diabetes, and obesity (Wahlstrom et al., 2016). Microbial enzymes such as proteases, hydrolases, and β-glucuronidases have been labeled using various ABPs and applied successfully in investigating the changes in gut microbiome activity in different disease models (Hatzios et al., 2016; Mayers et al., 2017; Zhuang et al., 2017; Parasar et al., 2019; Whidbey et al., 2019; Jariwala et al., 2020). Importantly, these analyses reveal that change in microbial enzyme activity does not faithfully correspond to gene abundance, which reiterates the necessity of function-based analyses such as ABPP for researchers to harness the chemistry of the microbiome.
The objective of this perspective is to highlight the tandem use of standards-free metabolomics and activity-based protein profiling to elucidate the metabolic function of specific taxa and the variety of enzymatic products and small molecule metabolites that they are capable of producing (Figure 1). Comprehensive, untargeted characterization of the metabolome can help identify bioactive metabolites that modulate host phenotype. Activity-based probes can be tailor made for metabolite targets (or dietary compounds or drugs) that have been detected and identified using standards-free metabolomics and implicated to have an impact on the health of the host and the microbiome. This opens up the possibility of categorizing gut microorganisms based on their functional products (enzymes and metabolites), under a defined set of host and environmental factors. We expect that the incorporation of experimental and computationally predicted molecular properties, as part of metabolomics workflows will result in improved detection and increased confidence identification. As researchers start to explore the immense chemical space of human microbiomes and encounter previously unknown molecules, the field will start to rely increasingly on computationally generated libraries containing multiple molecular descriptors such as retention time, CCS, accurate masses of precursor, and fragment ions etc. thus providing increasing confidence of a match as more of these predicted values match with experimentally measured values for a molecule of interest. In recent years, ABPP has emerged as a successful platform to functionally characterize proteins from incompletely annotated genomes and allow study of shifts in functional activity of microbiome in case of change due to external environment, disease, and exposure to chemicals. Standards-free metabolomics coupled with ABPP, ushers in a new era for deciphering the functionally relevant microorganisms in the microbiome. Determining these functional links provides a roadmap for unlocking the full potential of probiotics, developing personalized medicine for individuals based on their unique microbiome, and delineating the relationships between microbial metabolites and human health.
Figure 1. Schematic for the combined use of standards-free metabolomics (blue) and activity-based protein profiling (purple) workflows to functionally characterize biomolecules, the microbial populations involved and their effect on the host. The colored text boxes (blue, purple, or blue+purple) indicate the data and understanding gained from either one or a combination of both technologies.
SPC and TM conceptualized the work. SPC, NA, SMC, KB, and TM wrote the paper. All authors reviewed and edited the paper.
This work was supported by the National Institutes of Health, National Institute of Environmental Health Sciences Grant no. U2CES030170, National Institute of Child Health and Human Development Grant no. R01HD092297 and by the PNNL Laboratory Directed Research and Development program as a contribution of the Biomedical Resilience & readiness in AdVerse operating Environments (BRAVE) program. Battelle operates PNNL for the U.S. Department of Energy under contract DE-AC05-76RLO01830.
The authors declare that the research was conducted in the absence of any commercial or financial relationships that could be construed as a potential conflict of interest.
The authors thank Pacific Northwest National Laboratory (PNNL) Graphic Designer Nathan Johnson for making the figure.
Adam, G. C., Burbaum, J., Kozarich, J. W., Patricelli, M. P., and Cravatt, B. F. (2004). Mapping enzyme active sites in complex proteomes. J. Am. Chem. Soc. 126, 1363–1368. doi: 10.1021/ja038441g
Allen, F., Pon, A., Wilson, M., Greiner, R., and Wishart, D. (2014). CFM-ID: a web server for annotation, spectrum prediction and metabolite identification from tandem mass spectra. Nucleic Acids Res. 42, W94–W99. doi: 10.1093/nar/gku436
Arnold, J. W., Roach, J., and Azcarate-Peril, M. A. (2016). emerging technologies for gut microbiome research. Trends Microbiol. 24, 887–901. doi: 10.1016/j.tim.2016.06.008
Bach, E., Szedmak, S., Brouard, C., Böcker, S., and Rousu, J. (2018). Liquid-chromatography retention order prediction for metabolite identification. Bioinformatics 34, i875–i883. doi: 10.1093/bioinformatics/bty590
Blaschke, T., Olivecrona, M., Engkvist, O., Bajorath, J., and Chen, H. (2018). Application of generative autoencoder in de novo molecular design. Mol. Inf. 37:1700123. doi: 10.1002/minf.201700123
Castle, A. L., Fiehn, O., Kaddurah-Daouk, R., and Lindon, J. C. (2006). Metabolomics standards workshop and the development of international standards for reporting metabolomics experimental results. Brief Bioinf. 7, 159–165. doi: 10.1093/bib/bbl008
Chauvigne-Hines, L. M., Anderson, L. N., Weaver, H. M., Brown, J. N., Koech, P. K., Nicora, C. D., et al. (2012). Suite of activity-based probes for cellulose-degrading enzymes. J. Am. Chem. Soc. 134, 20521–20532. doi: 10.1021/ja309790w
Colby, S. M., Nuñez, J. R., Hodas, N. O., Corley, C. D., and Renslow, R. R. (2020). Deep learning to generate in silico chemical property libraries and candidate molecules for small molecule identification in complex samples. Anal. Chem. 92, 1720–1729. doi: 10.1021/acs.analchem.9b02348
Colby, S. M., Thomas, D. G., Nuñez, J. R., Baxter, D. J., Glaesemann, K. R., Brown, J. M., et al. (2019). ISiCLE: a quantum chemistry pipeline for establishing in silico collision cross section libraries. Anal. Chem. 91, 4346–4356. doi: 10.1021/acs.analchem.8b04567
Cravatt, B. F., Wright, A. T., and Kozarich, J. W. (2008). Activity-based protein profiling: from enzyme chemistry to proteomic chemistry. Annu. Rev. Biochem. 77, 383–414. doi: 10.1146/annurev.biochem.75.101304.124125
Dai, H., Tian, Y., Dai, B., Skiena, S., and Song, L. (2018). Syntax-directed variational autoencoder for structured data. arXiv [preprint] arXiv:1802.08786.
De Cao, N., and Kipf, T. (2018). MolGAN: An implicit generative model for small molecular graphs. arXiv [preprint] arXiv:1805.11973.
Djoumbou-Feunang, Y., Fiamoncini, J., Gil-de-la-Fuente, A., Greiner, R., Manach, C., and Wishart, D. S. (2019). BioTransformer: a comprehensive computational tool for small molecule metabolism prediction and metabolite identification. J. Cheminf. 11:2. doi: 10.1186/s13321-018-0324-5
Dodds, J. N., and Baker, E. S. (2019). Ion mobility spectrometry: fundamental concepts, instrumentation, applications, and the road ahead. J. Am. Soc. Mass Spectrom. 30, 2185–2195. doi: 10.1007/s13361-019-02288-2
Dührkop, K., Fleischauer, M., Ludwig, M., Aksenov, A. A., Melnik, A. V., Meusel, M., et al. (2019). SIRIUS 4: a rapid tool for turning tandem mass spectra into metabolite structure information. Nat. Methods 16, 299–302. doi: 10.1038/s41592-019-0344-8
Dührkop, K., Shen, H., Meusel, M., Rousu, J., and Böcker, S. (2015). Searching molecular structure databases with tandem mass spectra using CSI:FingerID. Proc. Nat. Acad. Sci. U.S.A. 112:12580. doi: 10.1073/pnas.1509788112
El Kaoutari, A., Armougom, F., Gordon, J. I., Raoult, D., and Henrissat, B. (2013). The abundance and variety of carbohydrate-active enzymes in the human gut microbiota. Nat. Rev. Microbiol. 11, 497–504. doi: 10.1038/nrmicro3050
Elahi, R., Ray, W. K., Dapper, C., Dalal, S., Helm, R. F., and Klemba, M. (2019). Functional annotation of serine hydrolases in the asexual erythrocytic stage of Plasmodium falciparum. Sci. Rep. 9:17532. doi: 10.1038/s41598-019-54009-0
Falony, G., Joossens, M., Vieira-Silva, S., Wang, J., Darzi, Y., Faust, K., et al. (2016). Population-level analysis of gut microbiome variation. Science 352, 560–564. doi: 10.1126/science.aad3503
Gómez-Bombarelli, R., Wei, J. N., Duvenaud, D., Hernández-Lobato, J. M., Sánchez-Lengeling, B., Sheberla, D., et al. (2018). Automatic chemical design using a data-driven continuous representation of molecules. ACS Cent. Sci. 4, 268–276. doi: 10.1021/acscentsci.7b00572
Guijas, C., Montenegro-Burke, J. R., Warth, B., Spilker, M. E., and Siuzdak, G. (2018). Metabolomics activity screening for identifying metabolites that modulate phenotype. Nat. Biotechnol. 36, 316–320. doi: 10.1038/nbt.4101
Gupta, A., Müller, A. T., Huisman, B. J., Fuchs, J. A., Schneider, P., and Schneider, G. (2018). Generative recurrent networks for de novo drug design. Mol. Inf. 37:1700111. doi: 10.1002/minf.201700111
Haiser, H. J., Gootenberg, D. B., Chatman, K., Sirasani, G., Balskus, E. P., and Turnbaugh, P. J. (2013). Predicting and manipulating cardiac drug inactivation by the human gut bacterium Eggerthella lenta. Science 341, 295–298. doi: 10.1126/science.1235872
Hatzios, S. K., Abel, S., Martell, J., Hubbard, T., Sasabe, J., Munera, D., et al. (2016). Chemoproteomic profiling of host and pathogen enzymes active in cholera. Nat. Chem. Biol. 12, 268–274. doi: 10.1038/nchembio.2025
Heyer, R., Schallert, K., Zoun, R., Becher, B., Saake, G., and Benndorf, D. (2017). Challenges and perspectives of metaproteomic data analysis. J. Biotechnol. 261, 24–36. doi: 10.1016/j.jbiotec.2017.06.1201
Hufsky, F., Scheubert, K., and Böcker, S. (2014). Computational mass spectrometry for small-molecule fragmentation. Trends Anal. Chem. 53, 41–48. doi: 10.1016/j.trac.2013.09.008
Human Microbiome Project Consortium (2012). Structure, function and diversity of the healthy human microbiome. Nature 486, 207–214. doi: 10.1038/nature11234
Jansen, R. S., Mandyoli, L., Hughes, R., Wakabayashi, S., Pinkham, J. T., Selbach, B., et al. (2020). Aspartate aminotransferase Rv3722c governs aspartate-dependent nitrogen metabolism in Mycobacterium tuberculosis. Nat. Commun. 11:1960. doi: 10.1038/s41467-020-15876-8
Jansson, J. K., and Baker, E. S. (2016). A multi-omic future for microbiome studies. Nat. Microbiol. 1:16049. doi: 10.1038/nmicrobiol.2016.49
Jariwala, P. B., Pellock, S. J., Goldfarb, D., Cloer, E. W., Artola, M., Simpson, J. B., et al. (2020). Discovering the microbial enzymes driving drug toxicity with activity-based protein profiling. ACS Chem. Biol. 15, 217–225. doi: 10.1021/acschembio.9b00788
Jin, W., Barzilay, R., and Jaakkola, T. (2018). Junction tree variational autoencoder for molecular graph generation. arXiv [preprint] arXiv:1802.04364.
Kadurin, A., Aliper, A., Kazennov, A., Mamoshina, P., Vanhaelen, Q., Khrabrov, K., et al. (2017a). The cornucopia of meaningful leads: applying deep adversarial autoencoders for new molecule development in oncology. Oncotarget 8:10883. doi: 10.18632/oncotarget.14073
Kadurin, A., Nikolenko, S., Khrabrov, K., Aliper, A., and Zhavoronkov, A. (2017b). druGAN: an advanced generative adversarial autoencoder model for de novo generation of new molecules with desired molecular properties in silico. Mol. Pharm. 14, 3098–3104. doi: 10.1021/acs.molpharmaceut.7b00346
Kamat, S. S., Camara, K., Parsons, W. H., Chen, D. H., Dix, M. M., Bird, T. D., et al. (2015). Immunomodulatory lysophosphatidylserines are regulated by ABHD16A and ABHD12 interplay. Nat. Chem. Biol. 11, 164–171. doi: 10.1038/nchembio.1721
Kang, S., and Cho, K. (2018). Conditional molecular design with deep generative models. J. Chem. Inf. Model. 59, 43–52. doi: 10.1021/acs.jcim.8b00263
Keller, L. J., Babin, B. M., Lakemeyer, M., and Bogyo, M. (2020). Activity-based protein profiling in bacteria: applications for identification of therapeutic targets and characterization of microbial communities. Curr. Opin. Chem. Biol. 54, 45–53. doi: 10.1016/j.cbpa.2019.10.007
Kim, K., Kang, S., Yoo, J., Kwon, Y., Nam, Y., Lee, D., et al. (2018). Deep-learning-based inverse design model for intelligent discovery of organic molecules. npj Comput. Mater. 4:67. doi: 10.1038/s41524-018-0128-1
King, A. M., Mullin, L. G., Wilson, I. D., Coen, M., Rainville, P. D., Plumb, R. S., et al. (2019). Development of a rapid profiling method for the analysis of polar analytes in urine using HILIC–MS and ion mobility enabled HILIC–MS. Metabolomics 15:17. doi: 10.1007/s11306-019-1474-9
Klaassen, C. D., and Cui, J. Y. (2015). Review: mechanisms of how the intestinal microbiota alters the effects of drugs and bile acids. Drug Metab. Dispos. 43, 1505–1521. doi: 10.1124/dmd.115.065698
Koppel, N., Bisanz, J. E., Pandelia, M. E., Turnbaugh, P. J., and Balskus, E. P. (2018). Discovery and characterization of a prevalent human gut bacterial enzyme sufficient for the inactivation of a family of plant toxins. Elife 7. doi: 10.7554/eLife.33953.044
Lanucara, F., Holman, S. W., Gray, C. J., and Eyers, C. E. (2014). The power of ion mobility-mass spectrometry for structural characterization and the study of conformational dynamics. Nat. Chem. 6, 281–294. doi: 10.1038/nchem.1889
Leary, D. H., Hervey, W. J., Deschamps, J. R., Kusterbeck, A. W., and Vora, G. J. (2013). Which metaproteome? The impact of protein extraction bias on metaproteomic analyses. Mol. Cell. Probes 27, 193–199. doi: 10.1016/j.mcp.2013.06.003
Lee, P. Y., Chin, S. F., Neoh, H. M., and Jamal, R. (2017). Metaproteomic analysis of human gut microbiota: where are we heading? J. Biomed. Sci. 24:36. doi: 10.1186/s12929-017-0342-z
Lim, J., Ryu, S., Kim, J. W., and Kim, W. Y. (2018). Molecular generative model based on conditional variational autoencoder for de novo molecular design. J. Cheminf. 10:31. doi: 10.1186/s13321-018-0286-7
Martell, J., Seo, Y., Bak, D. W., Kingsley, S. F., Tissenbaum, H. A., and Weerapana, E. (2016). Global cysteine-reactivity profiling during impaired insulin/IGF-1 signaling in C. elegans identifies uncharacterized mediators of longevity. Cell Chem. Biol. 23, 955–966. doi: 10.1016/j.chembiol.2016.06.015
May, J. C., and McLean, J. A. (2015). Ion mobility-mass spectrometry: time-dispersive instrumentation. Anal. Chem. 87, 1422–1436. doi: 10.1021/ac504720m
Mayers, M. D., Moon, C., Stupp, G. S., Su, A. I., and Wolan, D. W. (2017). Quantitative metaproteomics and activity-based probe enrichment reveals significant alterations in protein expression from a mouse model of inflammatory bowel disease. J. Proteome Res. 16, 1014–1026. doi: 10.1021/acs.jproteome.6b00938
McGuire, M. K., and McGuire, M. A. (2017). Got bacteria? The astounding, yet not-so-surprising, microbiome of human milk. Curr. Opin. Biotechnol. 44, 63–68. doi: 10.1016/j.copbio.2016.11.013
Merk, D., Friedrich, L., Grisoni, F., and Schneider, G. (2018). De novo design of bioactive small molecules by artificial intelligence. Mol. Inf. 37:1700153. doi: 10.1002/minf.201700153
Metz, T. O., Baker, E. S., Schymanski, E. L., Renslow, R. S., Thomas, D. G., Causon, T. J., et al. (2017). Integrating ion mobility spectrometry into mass spectrometry-based exposome measurements: what can it add and how far can it go? Bioanalysis 9, 81–98. doi: 10.4155/bio-2016-0244
Miyao, T., Funatsu, K., and Bajorath, J. (2017). Exploring differential evolution for inverse QSAR analysis. F1000Research 6:1285. doi: 10.12688/f1000research.12228.1
Moossavi, S., Sepehri, S., Robertson, B., Bode, L., Goruk, S., Field, C. J., et al. (2019). Composition and variation of the human milk microbiota are influenced by maternal and early-life factors. Cell Host Microbe 25, 324–335. doi: 10.1016/j.chom.2019.01.011
Nuñez, J. R., Colby, S. M., Thomas, D. G., Tfaily, M. M., Tolic, N., Ulrich, E. M., et al. (2019). Evaluation of in silico multifeature libraries for providing evidence for the presence of small molecules in synthetic blinded samples. J. Chem. Inform. Model. 59, 4052–4060. doi: 10.1021/acs.jcim.9b00444
Olesen, S. W., and Alm, E. J. (2016). Dysbiosis is not an answer. Nat. Microbiol. 1:16228. doi: 10.1038/nmicrobiol.2016.228
Ortega, C., Frando, A., Webb-Robertson, B. J., Anderson, L. N., Fleck, N., Flannery, E. L., et al. (2018). A global survey of ATPase activity in Plasmodium falciparum asexual blood stages and gametocytes. Mol. Cell. Proteomics 17, 111–120. doi: 10.1074/mcp.RA117.000088
Paglia, G., and Astarita, G. (2017). Metabolomics and lipidomics using traveling-wave ion mobility mass spectrometry. Nat. Protoc. 12, 797–813. doi: 10.1038/nprot.2017.013
Paglia, G., Kliman, M., Claude, E., Geromanos, S., and Astarita, G. (2015). Applications of ion-mobility mass spectrometry for lipid analysis. Anal. Bioanal. Chem. 407, 4995–5007. doi: 10.1007/s00216-015-8664-8
Paglia, G., Williams, J. P., Menikarachchi, L., Thompson, J. W., Tyldesley-Worster, R., Halldorsson, S., et al. (2014). Ion mobility derived collision cross sections to support metabolomics applications. Anal. Chem. 86, 3985–3993. doi: 10.1021/ac500405x
Parasar, B., Zhou, H., Xiao, X., Shi, Q., Brito, I. L., and Chang, P. V. (2019). Chemoproteomic profiling of gut microbiota-associated bile salt hydrolase activity. ACS Cent. Sci. 5, 867–873. doi: 10.1021/acscentsci.9b00147
Picache, J. A., May, J. C., and McLean, J. A. (2020). Crowd-sourced chemistry: considerations for building a standardized database to improve omic analyses. ACS Omega 5, 980–985. doi: 10.1021/acsomega.9b03708
Prestat, E., David, M. M., Hultman, J., Tas, N., Lamendella, R., Dvornik, J., et al. (2014). FOAM (Functional Ontology Assignments for Metagenomes): a Hidden Markov Model (HMM) database with environmental focus. Nucleic Acids Res. 42:e145. doi: 10.1093/nar/gku702
Pullan, R. D., Thomas, G. A., Rhodes, M., Newcombe, R. G., Williams, G. T., Allen, A., et al. (1994). Thickness of adherent mucus gel on colonic mucosa in humans and its relevance to colitis. Gut 35, 353–359. doi: 10.1136/gut.35.3.353
Ramani, S., Stewart, C. J., Laucirica, D. R., Ajami, N. J., Robertson, B., Autran, C. A., et al. (2018). Human milk oligosaccharides, milk microbiome and infant gut microbiome modulate neonatal rotavirus infection. Nat. Commun. 9:5010. doi: 10.1038/s41467-018-07476-4
Rios-Covian, D., Ruas-Madiedo, P., Margolles, A., Gueimonde, M., de Los Reyes-Gavilan, C. G., and Salazar, N. (2016). Intestinal short chain fatty acids and their link with diet and human health. Front. Microbiol. 7:185. doi: 10.3389/fmicb.2016.00185
Saha, J. R., Butler, V. P. Jr., Neu, H. C., and Lindenbaum, J. (1983). Digoxin-inactivating bacteria: identification in human gut flora. Science 220, 325–327. doi: 10.1126/science.6836275
Scheubert, K., Hufsky, F., Petras, D., Wang, M., Nothias, L.-F., Dührkop, K., et al. (2017). Significance estimation for large scale metabolomics annotations by spectral matching. Nat. Commun. 8:1494. doi: 10.1038/s41467-017-01318-5
Schmidt, T. S. B., Raes, J., and Bork, P. (2018). The human gut microbiome: from association to modulation. Cell 172, 1198–1215. doi: 10.1016/j.cell.2018.02.044
Schneider, P., and Schneider, G. (2016). De novo design at the edge of chaos: miniperspective. J. Med. Chem. 59, 4077–4086. doi: 10.1021/acs.jmedchem.5b01849
Stepanova, S., and Kasicka, V. (2016). Recent developments and applications of capillary and microchip electrophoresis in proteomic and peptidomic analyses. J. Sep. Sci. 39, 198–211. doi: 10.1002/jssc.201500973
Stow, S. M., Causon, T. J., Zheng, X., Kurulugama, R. T., Mairinger, T., May, J. C., et al. (2017). An interlaboratory evaluation of drift tube ion mobility–mass spectrometry collision cross section measurements. Anal. Chem. 89, 9048–9055. doi: 10.1021/acs.analchem.7b01729
Sugimoto, Y., Camacho, F. R., Wang, S., Chankhamjon, P., Odabas, A., Biswas, A., et al. (2019). A metagenomic strategy for harnessing the chemical repertoire of the human microbiome. Science 366:eaax9176. doi: 10.1126/science.aax9176
Sumner, L. W., Amberg, A., Barrett, D., Beale, M. H., Beger, R., Daykin, C. A., et al. (2007). Proposed minimum reporting standards for chemical analysis Chemical Analysis Working Group (CAWG) Metabolomics Standards Initiative (MSI). Metabolomics 3, 211–221. doi: 10.1007/s11306-007-0082-2
Tanca, A., Palomba, A., Pisanu, S., Addis, M. F., and Uzzau, S. (2015). Enrichment or depletion? The impact of stool pretreatment on metaproteomic characterization of the human gut microbiota. Proteomics 15, 3474–3485. doi: 10.1002/pmic.201400573
Thuy-Boun, P. S., and Wolan, D. W. (2019). A glycal-based photoaffinity probe that enriches sialic acid binding proteins. Bioorg. Med. Chem. Lett. 29, 2609–2612. doi: 10.1016/j.bmcl.2019.07.054
Tsai, C. S., Yen, H. Y., Lin, M. I., Tsai, T. I., Wang, S. Y., Huang, W. I., et al. (2013). Cell-permeable probe for identification and imaging of sialidases. Proc. Natl. Acad. Sci. U.S.A. 110, 2466–2471. doi: 10.1073/pnas.1222183110
Wahlstrom, A., Sayin, S. I., Marschall, H. U., and Backhed, F. (2016). Intestinal crosstalk between bile acids and microbiota and its impact on host metabolism. Cell Metab. 24, 41–50. doi: 10.1016/j.cmet.2016.05.005
Wang, M., Carver, J. J., Phelan, V. V., Sanchez, L. M., Garg, N., Peng, Y., et al. (2016). Sharing and community curation of mass spectrometry data with global natural products social molecular networking. Nat. Biotechnol. 34, 828–837. doi: 10.1038/nbt.3597
Wang, M., Jarmusch, A. K., Vargas, F., Aksenov, A. A., Gauglitz, J. M., Weldon, K., et al. (2020). Mass spectrometry searches using MASST. Nat. Biotechnol. 38, 23–26. doi: 10.1038/s41587-019-0375-9
Wang, X., Jones, D. R., Shaw, T. I., Cho, J. H., Wang, Y., Tan, H., et al. (2018). Target-decoy-based false discovery rate estimation for large-scale metabolite identification. J. Proteome Res. 17, 2328–2334. doi: 10.1021/acs.jproteome.8b00019
Whidbey, C., Sadler, N. C., Nair, R. N., Volk, R. F., DeLeon, A. J., Bramer, L. M., et al. (2019). A probe-enabled approach for the selective isolation and characterization of functionally active subpopulations in the gut microbiome. J. Am. Chem. Soc. 141, 42–47. doi: 10.1021/jacs.8b09668
Whidbey, C., and Wright, A. T. (2019). Activity-based protein profiling-enabling multimodal functional studies of microbial communities. Curr. Top. Microbiol. Immunol. 420, 1–21. doi: 10.1007/82_2018_128
Winston, J. A., and Theriot, C. M. (2020). Diversification of host bile acids by members of the gut microbiota. Gut Microbes 11, 158–171. doi: 10.1080/19490976.2019.1674124
Wolfer, A. M., Lozano, S., Umbdenstock, T., Croixmarie, V., Arrault, A., and Vayer, P. (2016). UPLC–MS retention time prediction: a machine learning approach to metabolite identification in untargeted profiling. Metabolomics 12:8. doi: 10.1007/s11306-015-0888-2
Wong, W. W., and Burkowski, F. J. (2009). A constructive approach for discovering new drug leads: using a kernel methodology for the inverse-QSAR problem. J. Cheminf. 1:4. doi: 10.1186/1758-2946-1-4
Wu, L., Armstrong, Z., Schroder, S. P., de Boer, C., Artola, M., Aerts, J. M., et al. (2019). An overview of activity-based probes for glycosidases. Curr. Opin. Chem. Biol. 53, 25–36. doi: 10.1016/j.cbpa.2019.05.030
Xiong, W., Giannone, R. J., Morowitz, M. J., Banfield, J. F., and Hettich, R. L. (2015). Development of an enhanced metaproteomic approach for deepening the microbiome characterization of the human infant gut. J. Proteome Res. 14, 133–141. doi: 10.1021/pr500936p
Xu, H., Majmudar, J. D., Davda, D., Ghanakota, P., Kim, K. H., Carlson, H. A., et al. (2015). Substrate-competitive activity-based profiling of ester prodrug activating enzymes. Mol. Pharm. 12, 3399–3407. doi: 10.1021/acs.molpharmaceut.5b00414
Yesiltepe, Y., Nuñez, J. R., Colby, S. M., Thomas, D. G., Borkum, M. I., Reardon, P. N., et al. (2018). An automated framework for NMR chemical shift calculations of small organic molecules. J. Cheminf. 10:52. doi: 10.1186/s13321-018-0305-8
Zhernakova, A., Kurilshikov, A., Bonder, M. J., Tigchelaar, E. F., Schirmer, M., Vatanen, T., et al. (2016). Population-based metagenomics analysis reveals markers for gut microbiome composition and diversity. Science 352, 565–569. doi: 10.1126/science.aad3369
Zhou, Z., Shen, X., Tu, J., and Zhu, Z. J. (2016). Large-scale prediction of collision cross-section values for metabolites in ion mobility-mass spectrometry. Anal. Chem. 88, 11084–11091. doi: 10.1021/acs.analchem.6b03091
Zhou, Z., Xiong, X., and Zhu, Z. J. (2017). MetCCS predictor: a web server for predicting collision cross-section values of metabolites in ion mobility-mass spectrometry based metabolomics. Bioinformatics 33, 2235–2237. doi: 10.1093/bioinformatics/btx140
Zhou, Z. W., Tu, J., Xiong, X., Shen, X. T., and Zhu, Z. J. (2017). LipidCCS: prediction of collision cross-section values for lipids with high precision to support ion mobility-mass spectrometry-based lipidomics. Anal. Chem. 89, 9559–9566. doi: 10.1021/acs.analchem.7b02625
Keywords: microbiome, function, standards-free metabolomics, ABPP, human health
Citation: Couvillion SP, Agrawal N, Colby SM, Brandvold KR and Metz TO (2020) Who Is Metabolizing What? Discovering Novel Biomolecules in the Microbiome and the Organisms Who Make Them. Front. Cell. Infect. Microbiol. 10:388. doi: 10.3389/fcimb.2020.00388
Received: 19 May 2020; Accepted: 25 June 2020;
Published: 31 July 2020.
Edited by:
Tao Lin, Baylor College of Medicine, United StatesReviewed by:
J. Christopher Fenno, University of Michigan, United StatesCopyright © 2020 Couvillion, Agrawal, Colby, Brandvold and Metz. This is an open-access article distributed under the terms of the Creative Commons Attribution License (CC BY). The use, distribution or reproduction in other forums is permitted, provided the original author(s) and the copyright owner(s) are credited and that the original publication in this journal is cited, in accordance with accepted academic practice. No use, distribution or reproduction is permitted which does not comply with these terms.
*Correspondence: Thomas O. Metz, dGhvbWFzLm1ldHpAcG5ubC5nb3Y=
Disclaimer: All claims expressed in this article are solely those of the authors and do not necessarily represent those of their affiliated organizations, or those of the publisher, the editors and the reviewers. Any product that may be evaluated in this article or claim that may be made by its manufacturer is not guaranteed or endorsed by the publisher.
Research integrity at Frontiers
Learn more about the work of our research integrity team to safeguard the quality of each article we publish.