- 1FG16 – Mycotic and Parasitic Agents and Mycobacteria, Robert Koch Institute, Berlin, Germany
- 2Department of Molecular Parasitology, Humboldt-Universität zu Berlin, Berlin, Germany
- 3School of Biological Sciences, University of Edinburgh, Edinburgh, United Kingdom
Rodents, in particular Mus musculus, have a long and invaluable history as models for human diseases in biomedical research, although their translational value has been challenged in a number of cases. We provide some examples in which rodents have been suboptimal as models for human biology and discuss confounders which influence experiments and may explain some of the misleading results. Infections of rodents with protozoan parasites are no exception in requiring close consideration upon model choice. We focus on the significant differences between inbred, outbred and wild animals, and the importance of factors such as microbiota, which are gaining attention as crucial variables in infection experiments. Frequently, mouse or rat models are chosen for convenience, e.g., availability in the institution rather than on an unbiased evaluation of whether they provide the answer to a given question. Apart from a general discussion on translational success or failure, we provide examples where infections with single-celled parasites in a chosen lab rodent gave contradictory or misleading results, and when possible discuss the reason for this. We present emerging alternatives to traditional rodent models, such as humanized mice and organoid primary cell cultures. So-called recombinant inbred strains such as the Collaborative Cross collection are also a potential solution for certain challenges. In addition, we emphasize the advantages of using wild rodents for certain immunological, ecological, and/or behavioral questions. The experimental challenges (e.g., availability of species-specific reagents) that come with the use of such non-model systems are also discussed. Our intention is to foster critical judgment of both traditional and newly available translational rodent models for research on parasitic protozoa that can complement the existing mouse and rat models.
Introduction
Gregor Mendel introduced the basic concept of a “model organism” when he reported his experiments on plant hybrids. He picked peas as a model because they had clear experimental advantages for addressing his question: “At the very outset special attention was devoted to the Leguminosae on account of their peculiar floral structure (…) this led to the result that the genus Pisum was found to possess the necessary qualifications.” (Mendel, 1866) Since then this approach for model selection for a particular purpose is widely used, meaning that a model organism should be accessible, experimentally tractable, have short generation times, be affordable to maintain and breed, possess clearly identifiable features that are to be studied, and more recently be genetically tractable (which includes access to a sequenced genome), to name a few (Rand, 2008). Given the importance of model systems in biology, the history and diversity of model organisms has been extensively reviewed (Conn, 2008; Hunter, 2008; Müller and Grossniklaus, 2010; Ankeny and Leonelli, 2011; Bolker, 2012; Alfred and Baldwin, 2015).
The available model organisms span a great taxonomic range, and for many questions single-celled organisms such as bacteria (Escherichia coli) or yeasts (Saccharomyces cerevisiae) are sufficient. However, in many biomedical studies which aim to translate findings to humans, non-mammals are not applicable as translational models (Hau, 2008). Yet, shorter phylogenetic distances and anatomical similarities are no guarantee for translational success, as research on primates has demonstrated (detailed in section The CD28 Superagonist Antibody “Disaster”). Given the high relevance of protozoa for human and animal health and our own scientific interests in these parasites, we will concentrate on such infections in rodents. While their value as translational models is not without dispute (see section Protozoan Parasites) they have been, still are, and will continue to be, invaluable for both basic biological questions in host-parasite interactions as well as pre-clinical studies. Their importance for scientific progress is demonstrated, for example by the essential role laboratory mice played in the discovery of dendritic cells (see references in Steinman, 2012) and macrophages (reviewed by Gordon, 2007). The value of rodents, and mice in particular, has also been highlighted for leading to fundamental insights in infection biology (Buer and Balling, 2003; Vidal et al., 2008; Douam et al., 2015). Given the historical and current importance of rodents, we here explore benefits and limitations of, e.g., inbred or outbred mice, lab rodents, or rodents from the wild, etc., as well as possible external confounders, such as breeding conditions in lab facilities or interactions in an ecosystem, that might have great impact on research results. With this review, we encourage experimentalists, particularly in translational medicine, to consider a broad set of potential rodent models in order to identify and use the best available system for specific studies. We aim to provide a foundation and useful references for such decisions.
Protozoan Parasites
Infections with protozoan parasites cause substantial illness and economic loss in humans worldwide (see Tables 1, 2 for details; Fletcher et al., 2012; Murray et al., 2012; Andrews et al., 2014; Robertson et al., 2014; Kassebaum et al., 2016). These parasites with high impact on humans mostly are the Amoeba, e.g., Entamoeba spp.; the flagellates, e.g., Trichomonas spp., Giardia spp., Leishmania spp., and Trypanosoma spp., and the large group of Apicomplexans, which contain, e.g., Plasmodium spp., Toxoplasma gondii, and Cryptosporidium spp. They are most often transmitted to their host either via ingestion of contaminated food, water, or via a vector (e.g., mosquitoes or flies; see Table 2). Here we provide a brief overview of some parasitic protozoa which substantially impact humans, and of which many are referred to in our examples used in following sections.
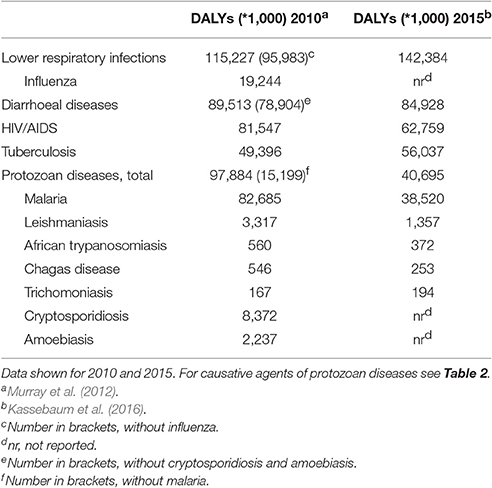
Table 1. Global “Disability-Adjusted Life-Years,” DALYs, for high impact infectious diseases, with several examples from protozoan parasites.
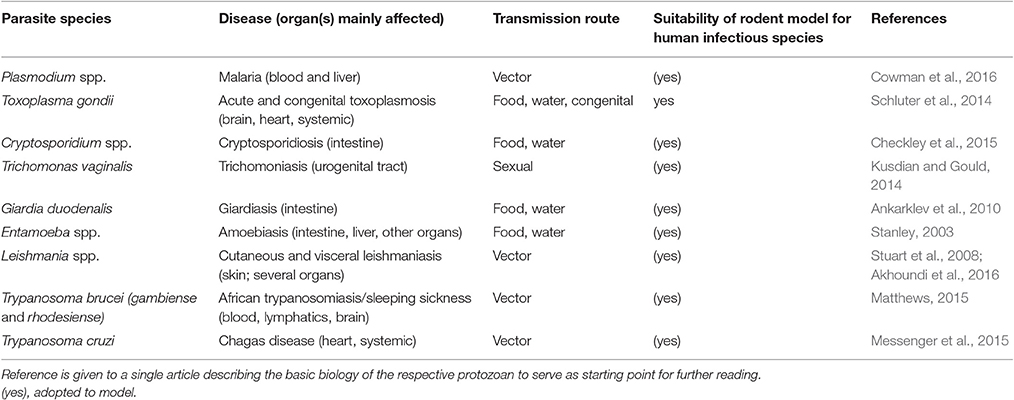
Table 2. The diseases caused, transmission routes, and suitability of rodents as models for human disease are listed for selected protozoan parasites.
The significance of protozoan infections for global human health is here exemplified by data (see Table 1) where the impact of infections by the most devastating protozoan parasites is expressed as “disability-adjusted life-years” (DALYs, used by the World Health Organization (WHO) and others as a measure of disease impact). These diseases ranked second in importance across all infectious diseases, behind lower respiratory infections, and before AIDS and tuberculosis. The great majority of this impact can be attributed to malaria alone (85% caused by Plasmodium spp.). However, the collective disease burden of the other protozoa evaluated was also substantial and in the range of influenza (Murray et al., 2012). While many of these figures, including those for malaria, are fortunately on the decline, this disease was still ranked among the top 20 leading diseases as identified by the WHO worldwide in 2015 (Kassebaum et al., 2016). In addition to these human health concerns, protozoan parasites cause significant losses in many species of domestic animals (Perry and Grace, 2009; Torgerson and Macpherson, 2011; Fitzpatrick, 2013; Torgerson, 2013) and are in some cases a conservation concern for wildlife (Pedersen et al., 2007).
While many of these parasites have a very restricted host range (infecting a single host species and/or tissue), other extremes such as T. gondii, a zoonotic parasite assumed to infect all nucleated cells in all warm-blooded animals, exist. Consequently, there is a mixture of more or less natural relationships between the parasites and the rodent hosts when the latter are used as translational models for human infections (see Table 2). Many parasite genera contain species which naturally infect rodents (e.g., Plasmodium, Giardia, Cryptosporidium), although in most cases the very same species do not also infect humans. For other parasite species, the rodent model has been made susceptible, frequently by genetic means, to human relevant parasites (e.g., P. falciparum or C. parvum/C. hominis). In malaria research, rodents have been successfully used as models, but the suitability of the mouse to mimic severe human malaria has been questioned (Langhorne et al., 2011). In leishmaniasis research, rodents are acknowledged for contributing to a better understanding of the immune response to the parasite (Lipoldová and Demant, 2006) but other authors point out limitations and the lack of suitability of certain mouse strains to study specific parasite genotypes (Mears et al., 2015). Research on human sleeping sickness (T. brucei) has benefitted largely from mouse models (Antoine-Moussiaux et al., 2008; Giroud et al., 2009; Magez and Caljon, 2011) but criticism has been raised that more suitable animal models should be applied to address sleeping sickness in livestock (T. congolense and T. vivax; Morrison et al., 2016).
Why are Mice and Rats such Popular Models?
Biomedical research depends heavily on model organisms and the majority of these are rodents, particularly in infectious disease and immunological research. A few numbers illustrate this impressively. For example, in the European Union alone, 75% of all animals used for “experimental and other scientific purposes” in 2011 were house mice (Mus spp. 61%) and rats (Rattus spp. 14%; The Commission to the Council and the European Parliament, 2013). Other rodents (gerbils, hamsters, different species of mice, and other rodents) only constitute 0.47% of animals used (Figure 1A). In Germany, 91.5% of animals used in research on infectious diseases were rodents, with the vast majority being Mus musculus (88.6%; Figure 1B). Similar numbers are reported in the United Kingdom, with 82% of all research using rodents, again dominated by M. musculus (74.6%; UK Home Office, 2015). While these numbers also include animals that were used as donors, e.g., for blood or organs and thus for in vitro experimentation these data nevertheless illustrate the dominance and importance of rodents, in particular laboratory inbred mice, as model organisms.
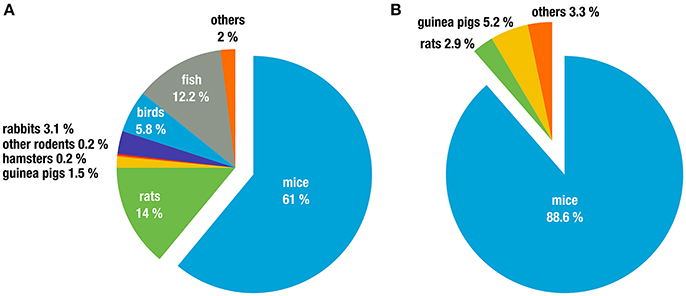
Figure 1. Animal classes used in experiments for (A) all life sciences disciplines in the 27 EU member states (2011 in %). Data taken from (The Commission to the Council and the European Parliament, 2013), and (B) research on infection biology only, in Germany (2011 in %). Data taken from (German Federal Ministry of Food and Agriculture, 2011).
Another informative figure shows that the number of publications where mice and rats were mentioned in the title dominates all other model organisms included (Figure 2), e.g., Arabidopsis, Drosophila, S. cerevisiae, Caenorhabditis elegans, Xenopus, zebrafish, Neurospora, and Dictyostelium discoideum. It is presumably no coincidence that a sharp increase in these “mouse publications” was seen in the 1990s, given that it was when embryonic stem cell manipulation met homologous recombination of the mouse genome. This resulted in the generation of defined gene knock-out mice (Figure 2), a finding which was later rewarded with the Nobel Prize in Physiology (Mak, 2007). The importance of this discovery for scientific progress in infection biology cannot be overestimated. However, in rats no such methods were available until relatively recently (Tong et al., 2010; van Boxtel and Cuppen, 2010), which is reflected in the drastic increase of mouse models and a relatively stable use of rats from 1990s until now. It is likely due to the highly developed genetic tools in mice, together with the more than 450 inbred mouse strains established since the first strain (DBA/2) was developed by Clarence Cook Little, that mice, and in particular the C57BL/6 strain, are the most popular animal model (Beck et al., 2000; Festing and Fisher, 2000). However, with the advent of CRISPR/Cas9 gene modifications in rats this will likely change (Hu et al., 2013; Li D. et al., 2013; Li W. et al., 2013), since this method has worked so far in almost all organisms tried and it can most likely also be applied to wild rodents. The historic establishment of tools for mice combined with the fact that 99% of genes are conserved between the human and the mouse genomes (Waterston et al., 2002) has made and will continue to make the mouse an obvious choice for translational efforts, i.e., research to understand the basics of and find treatments for human diseases. In addition, it will be exciting to see contributions from so far poorly explored model systems.
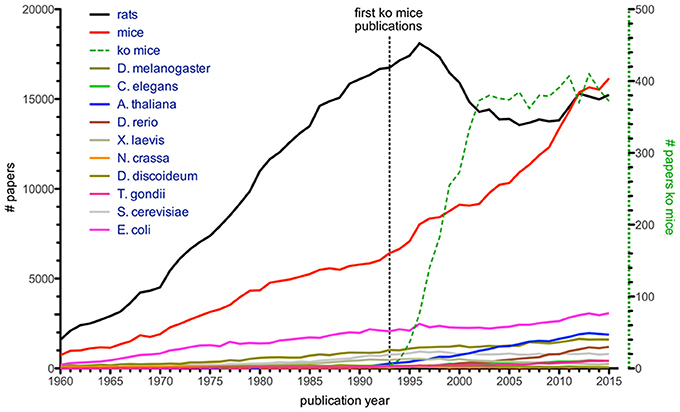
Figure 2. Number of citations with organism's name in title (Dietrich et al., 2014), based on Web of Science entries for a given year. Numbers for single-celled model organisms like T. gondii, S. cerevisiae, and E. coli are given for comparison. Green dashed line (with corresponding y-axis on the right) illustrates articles mentioning knock-out mice, with first papers appearing in the early 1990s.
The Mice We Use in Experiments — Who Are They and How Do They Live?
Here we will briefly cover definitions of nomenclature for referring to different types of mice, from laboratory to “wild” rodents.
Classical inbred mice are defined as either being “produced using at least 20 consecutive generations of sister x brother or parent x offspring matings” or “traceable to a single ancestral pair in the 20th or subsequent generation.” (“Nomenclature of Inbred Mice,” defined by the Mouse Genomic Nomenclature Committee). However, it can be noted that 20 generations of inbreeding does not lead to fixed alleles in the entire genome, although for most phenotypes no differences are detected after this threshold (Chia et al., 2005). Different inbred populations exist and are referred to as strains (whereas outbred populations are often referred to as stocks). Inbred strains are genetically highly homogenous, well-defined, and often with genomes and SNP data available. In addition, extensive descriptions of (mutant) strains are available in the Mouse Phenome Database (Grubb et al., 2014) or the International Mouse Phenotyping Consortium database (Koscielny et al., 2014; see Table S1 for links) and should be consulted when planning experiments.
Wild-derived inbred strains are “descendants of mice captured in wild populations during the mid to late 20th century and represent several different Mus species from around the world” (Lutz et al., 2012). These mice are considered suitable for, e.g., evolutionary studies and gene mapping, but notably do not represent the genetic diversity of wild animals.
Outbred stocks are defined as “a closed population (for at least four generations) of genetically variable animals that is bred to maintain maximum heterozygosity” (Chia et al., 2005), meaning that each individual is genetically different from the others. Once established, the goal is to keep the genetic variability between generations to a minimum which is achieved by using, e.g., a certain number of breeding pairs (Chia et al., 2005). We onwards refer to inbred and outbred M. musculus models as lab mice, if nothing else is specified.
Recombinant Inbred Strains, RIS, are a collection of mice established by inbreeding two existing inbred strains into a set of strains (often called set or panel). Each such strain is genetically homogenous, but “parallel” strains produced from the same two well-defined ancestral strains are genetically more different from each other than either of the two ancestors (Chia et al., 2005). One advantage of using a RIS set compared to pure inbred strains is that phenotypic differences (e.g., pathogen or drug susceptibility) can be fairly easily assigned to specific genotypes (Guénet et al., 2015), and obtaining high-quality quantitative data on transcripts and proteins is feasible (Chick et al., 2016). Other options for lab mice, such as genetic crosses, will be covered in the section Humanized Mice: Rodents Which Mimic the Human Immune System.
We refer to wild rodents (including wild mice, e.g., species of Mus) as rodents which breed without direct intervention or manipulation by humans, in their natural habitats, e.g., farmland, forests or cities (Singleton and Krebs, 2007). Such populations may in some cases be under experimental study and manipulated, for example by regular trapping, diet manipulations and drug treatment, and will here still be considered as wild populations.
Mouse Housing Influences Experimental Outcome
Almost all animal research facilities can house rodents in specific pathogen-free, SPF, barrier facilities. This standard includes regular screening for a large set of common pathogens (in order to detect contamination), and commonly autoclaving cages, bedding, water, food and other housing related materials to assure hygienic and controlled housing, as well as controlled light/dark cycles (Hedrich and Nicklas, 2012). For details see respective lab manuals (Ayadi et al., 2011; Hedrich, 2012). Animal psychological status has been shown to influence variability in experimental studies, including examples of more reproducible results from “happier” mice, which display less anxiety or depression-associated behavior as a result of increased animal welfare (Bayne and Würbel, 2012). Although, wild mice can run several 100 m per night (Latham and Mason, 2004) including a means for physical activity (e.g., running wheels) is not standard in animal housing. Moreover, it is debated whether such so-called enrichment of housing is always required, beneficial, or adequate for the outcome of an experiment (Bayne and Würbel, 2012), given that after decades of breeding and selection lab mice in many respects show different behaviors to wild mice (Latham and Mason, 2004).
Recent publications highlight the important role of microbiota in rodents (and humans). Even though SPF animals are the most commonly used rodents in experiments (Fiebiger et al., 2016), gut microbiota are not homogenous (e.g., in composition or bacterial numbers) in such research settings and the extent of this variation has only recently emerged. Microbiota differ between vendors and mouse strains (Hufeldt et al., 2010; Ericsson et al., 2015; Hilbert et al., 2017), different shipments from the same vendor (Hoy et al., 2015), between research animal facilities (Rausch et al., 2016) and even between rooms in the same breeding facility (Rogers et al., 2014). Determining factors for gut microbiota differences under SPF housing conditions without experimental perturbations have been analyzed, and apart from vendor, the fodder and treatment thereof is important (Rausch et al., 2016). Therefore, housing conditions strongly influence mouse microbiota. Data also suggest a general difference between inbred lab mice and wild mice in that the proportion of Firmicutes vs. Bacteroides vary, with wild rodents being dominated by Firmicutes and vice versa (Weldon et al., 2015).
Misleading Results due to Inappropriate Analysis or an Inappropriate Model
“If you have cancer and you are a mouse, we can take good care of you” (Kolata, 1998). This famous sentence from Judah Folkman (the “father” of tumor angiogenesis) makes this point: any model - animal or even mathematical - only returns the output it is capable of producing. A translational mouse model that lacks human feature X will never give a response in X, no matter how important that particular feature is in the context of a human disease. While the mouse model has been very successful for understanding the general principles of the mammalian immune system and infectious disease (e.g., Buer and Balling, 2003), it is important to be aware of, and acknowledge, the intrinsic benefits and limitations in any model chosen for a specific experiment. However, before we focus on biological confounders we want to consider that failures in the transition from preclinical studies to humans may also be due to poorly designed or performed studies (see Couzin-Frankel, 2013; Justice and Dhillon, 2016). To illustrate that problems of very different character can challenge the suitability of translational rodent models, we will first discuss two past examples from different disciplines (sepsis and immunology) that caused vibrant discussions in the scientific community and were subsequently analyzed in great detail. They can thus provide valuable insights of general importance for scientists with different research interests. In the section Non-genetic Confounders in Rodent Infections with Protozoan Parasites we will then turn to confounders in translational rodent models of infections with protozoan parasites.
Analysis, Re-Analysis and Meta-Analysis — Three Studies and Three Conclusions
Due to its importance for human health, research on sepsis in mouse models is heavily funded, but its translational success has so far been disappointing (van der Worp et al., 2010). Few papers in biomedical research have therefore raised such an excitement and storm of replies and counter-replies as the Seok et al. (2013) study on sepsis. They analyzed transcriptomic data from various mouse models of human inflammatory diseases, and human samples in particular from septic shock, and concluded that “genomic responses in mouse models poorly mimic human inflammatory diseases” (Seok et al., 2013). However, re-analysis of the very same data subsequently concluded the opposite, and these authors simply replaced “poorly” with “greatly” in the article's title of their reply (Takao and Miyakawa, 2015). This discussion is still ongoing, with a recent paper (Weidner et al., 2016) pointing out that the data from Seok et al. per se are good enough to compare the transcriptional responses of certain (but not all) mouse models to humans, but that the analytical tools used in the two first papers were inappropriate. The authors' conclusions were that gene set enrichment analysis (GSEA) is more appropriate than gene-to-gene comparisons, which require setting an arbitrary threshold for the determination of differentially expressed genes (as opposed to identification by statistical means). Those tools were used differently by various authors for re-analyses of the original data sets, thus leading to opposite conclusions (Seok et al., 2013; Shay et al., 2013; Takao and Miyakawa, 2015; Warren et al., 2015). A further level of complexity that makes conclusions derived from transcriptomic comparisons challenging is that for many genes there is no correlation between mRNA levels and protein quantities (see section Transcriptomes Do Not Necessarily Predict Protein Levels). Although transcriptome analysis is a fairly easily accessible and promising technique, these examples illustrate that such relatively young tools require close evaluation of the entire work-flow. Understanding and considering the physics or chemistry behind the method and to critically assess appropriate analysis methods is a community task when new scientific methods are being established.
The CD28 Superagonist Antibody “Disaster”
It is well known that substantial differences between the mouse and human immune systems exist (Mestas and Hughes, 2004; Zschaler et al., 2014; Sellers, 2017) and that they need to be considered when using mice as preclinical models of human disease (e.g., Beura et al., 2016). In 2006, a small human phase I clinical trial aimed at alleviating rheumatoid arthritis tested a humanized monoclonal antibody, TGN1412, directed against the human T cell receptor CD28. However, instead of improving the autoimmune condition, it resulted in devastating consequences (reviewed by Hunig, 2016). It was anticipated from laboratory mouse studies that injection of the antibody would result in the preferential production of regulatory T cells, followed by a downregulation of active T cells. However, all six volunteers had to be hospitalized and at least four of them suffered multiple organ dysfunctions. TGN1412 had caused an immediate “cytokine storm” in these patients due to substantial TNF-α release, followed by dramatically increased plasma concentrations of several cytokines. This “cytokine release syndrome” (CRS) was caused by a strong activation of CD4+ effector memory T cells, which eventually caused severe tissue damage. But why had preclinical studies in primate models, namely cynomolgus and rhesus monkeys, not indicated any signs of problems? What was unknown in 2006 was that those primates' CD4+ effector memory T-cells do not express CD28 whereas humans do (Eastwood et al., 2010). In this particular case, the monkeys were a poor model for humans, despite their phylogenetically close relationship.
And why had the human response not been seen in the numerous rodent experiments performed prior to the trial? Interestingly, later experiments have demonstrated that at least two drastically modified lab mouse models can indeed be good models for the TGN1412 experiments. The first example is linked to the fact that, as is the case for most immunological experiments, mice in the initial studies had been raised and kept under SPF conditions. Thereby, they had no exposure to microbial antigens that would elicit CD4+ memory T cells. Thus, CRS was not initiated upon TGN1412 treatment as it was in humans. Consequently, when TGN1412 was later given to non-laboratory “dirty” mammals (including rodents, see section Getting the Rodent Model “Dirty”) exposed to prior environmental microbial stimuli, they experienced similar syndromes as the human volunteers (Eastwood et al., 2010). The second alternative model consists of humanized mice (see section Humanized Mice: Rodents Which Mimic the Human Immune System). A recent study made use of mice which had been reconstituted with human peripheral blood mononuclear cells. Injecting TGN1412 into those animals recapitulated a number of the disastrous immunological outcomes also seen in the initial human trial (Weissmuller et al., 2016). Importantly, the transplanted human cells also included a small amount of effector memory cells. Therefore, both the use of “dirty” and humanized mice better mimicked human biology than the rodent models which were used in the pre-clinical studies (although both these models have gained interest more recently and were, if at all, very new ideas at that time).
Non-genetic Confounders in Rodent Infections with Protozoan Parasites
Lab rodent models have been essential for understanding molecular, cellular, and immunological responses; however, most of the variability inherent in natural populations is not captured by them (Pedersen and Babayan, 2011; Beura et al., 2016). Even so, sources of variation which influence experimental outcomes in lab experiments have also been identified in these very models. Reports based on lab experiments have often not accounted for such variability and instead ascribed differences between experimental groups to the aspect under study.
Microbiota as a General Confounder for Rodent Experiments
Recently, the role of microbiota as a confounder for experimental outcomes in various scientific fields has gained increasing interest (Servick, 2016), largely due to the development of next-generation sequencing and related methods. Common approaches to study microbiota include sequencing fecal content from lab mice and germ-free mice (discussed in detail in Fiebiger et al., 2016), fecal transplants, antibiotics treatment, probiotics, addition of a specific bacterium, and infectious agents. For biomedical research it is noteworthy that microbiota influences host susceptibility to drugs. One example are proteins encoded by drug processing genes, DPGs, which are responsible for uptake, distribution, metabolism, and excretion of xenobiotics such as drugs (Aplenc and Lange, 2004; Klaassen et al., 2011). DPGs in mouse liver display different expression patterns depending on the microbiota status of the animal (Fiebiger et al., 2016). Some authors have linked microbiota differences to subsequent variation in brain activity and changes in social behavior, a concept referred to as the gut-brain axis (e.g., Foster and McVey Neufeld, 2013; Mayer et al., 2015; Gacias et al., 2016) which is proposed to depend on several factors, including the immune system. Variation in microbiota is known to influence both local and systemic immune function by altering the balance of Th1/Th2 cell composition, influencing re-localization of neutrophils, or affecting macrophage polarization (Denny et al., 2016; Lopes et al., 2016). Taken together, it is therefore not surprising that differences in microbiota can have a substantial impact on protozoan parasite infections in the gut and elsewhere. It is also easy to imagine a situation in which genetically modified mice obtained from one breeder or lab and control mice from other sources leads to unintended differences in microbiota composition with resulting influence on the outcome of infection experiments.
Protozoan Infection Experiments Are Influenced by Microbiota
One early study pointing to the importance of microbiota for the establishment of a protozoan infection used germ-free mice which were infected with the intestinal parasite G. duodenalis (Torres et al., 1992). The authors demonstrated that the microbiota influences the establishment and nature of intestinal infection with regards to severity and parasite reproductive success. A later study showed that female mice with the same genetic background were either susceptible or resistant to G. duodenalis infection (Singer and Nash, 2000). Differences were due to the origin (vendor) of the animals, and the same was true for immunodeficient mice. Co-housing led to resistance in all animals, whereas treatment with antibiotics made all animals equally susceptible to intestinal infection. It was therefore concluded that the microbiota determined the outcome of infection. These studies have recently been complemented with more in-depth investigations of the microbial community, showing changes in the amount of microbiota and its composition upon G. duodenalis infection in mice (Barash et al., 2016). Hence, not only do microbiota influence infection outcome but the parasite in turn alters the gut microbiota. These studies emphasize the complexity of gastrointestinal parasite infections. Further analysis of microbiota-parasite-host cohabitation will likely reveal interactions such as competition for nutrients or synergies in metabolism.
The complexity of microbiome influences is not limited to gut microbiota. Skin microbiota has also been shown to influence the outcome of cutaneous leishmaniasis in mice. Its causative agent, L. major, differently induced skin lesions, edema, and necrosis in germ-free mice compared to SPF mice upon intradermal infection (Naik et al., 2012). Germ-free mice displayed less disease severity, but also reduced levels of IFN-γ and IL-17A from ɑβ T cells in the infected skin area compared to SPF reared animals. By orally administering antibiotics, the gut microbiota, but not skin microbiota, changed without influencing cytokine production. However, introduction of a skin commensal bacterium, Staphylococcus epidermis, did rescue IL-17A production in the skin. The authors concluded that local cytokine production was specifically linked to skin microbiota. In a different study, L. major infection altered the gut microbiota of infected animals (but differently depending on mouse strain) (Lamour et al., 2015). Infection changed how gut microbiota correlated with systemic functions such as urine metabolites, plasma metabolites, and the immune system. Such findings also highlight that simple correlations between microbiota and protozoan parasites may not be adequate to elucidate the dynamic role of microbiota during infection.
Interestingly, the microbiota does not only affect the site of infection but can also influence how host and parasite interact at other sites. Recent work provided evidence that the severity of malaria infection with rodent Plasmodium spp. can also depend on vendor. Differences in disease severity correlate with differences in microbiota composition (Villarino et al., 2016) or bacterial transcription profiles (Stough et al., 2016), demonstrating systemic effects by the microbiota. A study from 2014 also reported a mechanism for such correlations, describing production of anti-Plasmodium spp. antibodies in response to gut colonization, specifically by E. coli O86:B7 but not by the reference E. coli K12 (Yilmaz et al., 2014). These studies demonstrate that infections in specific compartments which are not colonized by commensal bacteria are nevertheless influenced and such effects must be considered in planning experiments and interpreting results.
Excursion 1: A Reductionist In vitro Approach Using Organoids
Protozoa-host interaction studies have largely been restricted to more or less suitable rodent models, cell lines (often cancer-derived), and short-lived primary tissue cultures from biopsy or surgery. Recent advances in stem cell research have paved the way for the development of self-renewing and complex tissue-like culture systems, so-called organoids, which mimic organs in their main functions and structural features (Willyard, 2015). Major advantages include that host-parasite interactions can be investigated in a primary, long-lived, organ-like tissue from the organism of choice, including humans, in real time (Klotz et al., 2012). Organoids have been developed from the gastro-intestinal tract (GIT), including stomach, gut and liver, and also from kidney and brain (Clevers, 2016). Importantly, organoids lack tissue-specific immune cells and in the GIT the microbiota, and therefore complexity is low compared to in vivo settings. However, this feature allows the researcher to construct an experimental setup with exactly the desired level of complexity, adding for instance the Mouse Intestinal Bacterial Collection (Lagkouvardos et al., 2016), human microbiota from biopsies, and/or a set of cytokines or immune cells of interest. For elucidating the role of individual actors during a parasitic infection, organoids are promising alternatives to animal models, cell culture systems, and the use of human biopsy material.
Sex and Age
Two long-known factors that influence infection success by parasites are sex and age. The most obvious differences between the sexes are hormones (Roberts et al., 2001; Klein, 2004; Bernin and Lotter, 2014) but X chromosome-linked mutations (van Lunzen and Altfeld, 2014; Garenne, 2015) and sex-specific behavior can also affect the outcome of infectious diseases. A prominent example in protozoan infections is glucose-6-phosphate dehydrogenase (G6PD) deficiency, which protects humans of both sexes to different extents from clinical outcomes of infections with P. falciparum (Shah et al., 2016). A previously developed humanized mouse model of G6PD deficiency (Rochford et al., 2013) has recently been used in screening efforts to identify malaria transmission-blocking drugs (Wickham et al., 2016). A second example involves X-linked immunodeficiency in the B-cell responses due to mutations in the Bruton's tyrosine kinase. The mutation causes a sex-specific effect which leads to X-linked agammaglobulinaemia (XLA). Human patients and mice bearing a similar mutation (CBA/N) are more prone to develop chronic giardiasis (Skea and Underdown, 1991; Van der Hilst et al., 2002).
In rodent models of, e.g., Plasmodium spp., Cryptosporidium spp., and Leishmania spp. infection age significantly influences susceptibility (Adam et al., 2003), parasite reproductive success (Rhee et al., 1999), and severity of disease (Muller et al., 2008). In the case of Cryptosporidium (Rhee et al., 1999), hamsters displayed age-dependent differences (within the first 2 months of life) in infection persistence measured by time for shedding oocysts, whereas mice did not. The results demonstrate that rodents can be used to study cryptosporidosis, but simultaneously suggest that generalizations of these results to other species are difficult, and translational success is not obvious. For leishmaniasis, recent work showed mouse age-specific differences in the induction of adaptive immunity. Animals were exposed to a vaccine candidate based on genetically modified L. donovani and aged mice (~16 months) had a less pronounced adaptive immune response compared to young mice (~2 months) upon L. major challenge after vaccination (Bhattacharya et al., 2016). In Babesia microti infection of lab mice between the ages of 2 and 18 months (Vannier et al., 2004), one of three strains (DBA/2 mice) mimicked patterns seen in humans in which susceptibility and an inability to clear infection increased with age. The other two strains displayed smaller differences in susceptibility and no change in infection clearance, illustrating possibilities to use rodents as models for human babesiosis, but alerting to possible issues with interpretation and translation of results.
Given these examples it seems obvious to consider age and sex aspects when planning rodent experiments. However, it is not unusual to use only male or only female mice (Flórez-Vargas et al., 2016) based on convenience, local availability, costs, legal issues (more animals required when both sexes are examined; Clayton and Collins, 2014) or research area. In particular in infectious disease research there is a strong bias toward using female mice (Flórez-Vargas et al., 2016). One reason for this is presumably that they are less aggressive and thus cheaper since they can be housed in (experimental) groups in a single cage whereas this is challenging for male mice. Likewise, younger mice are cheaper to obtain since housing cost are lower. Thus, convenience rather than scientific reasoning might influence the choice of sex or age in many studies.
Models for All Purposes — from Fixed Alleles to Complex Ecology
Even before the first draft of the mouse genome was published in 2002 (Waterston et al., 2002), scientists were aware of the relative genetic homogeneity of the lab mouse compared to wild mouse populations (Guenet and Bonhomme, 2003). Inbreeding over almost a century fixed alleles in currently available lab mice, which now represent just a fraction of the genetic variability found in nature. Although a desirable feature for some questions, this variability can be of great importance in studies of host-parasite interactions. Genetic variability might be the reason for different host susceptibility together with, e.g., the confounders discussed above (see section Non-genetic Confounders in Rodent Infections with Protozoan Parasites). In many cases allelic variations of a gene involved in immune responses were identified as the cause of infection outcome.
When Immune Responses Depend on Genetics — Selected Examples
Allelic variation at Lsh and H2 loci is involved in the opposite outcome of the acquired immune response in L. donovani infection between e.g., CBA and BALB/c mice (Loeuillet et al., 2016). Another emerging example is the role that the inflammasome has in sensing protozoan infections (reviewed in Zamboni and Lima-Junior, 2015). During T. gondii infection in rodents, sequence differences in the pathogen sensor Nlrp1 accounts for species-specific inflammasome induction - and thus outcome - in lab mice (Ewald et al., 2014) and rat strains (Cirelli et al., 2014). Phenotypic differences between model animals can also be due to polymorphisms in the inflammasome pathway effectors, e.g., IL-18 and IL-1β. A study on a wild, natural population of field voles (Microtus agrestis) found associations with polymorphisms of IL-1β, IL-2, and IL-12β and differential susceptibility to pathogen infection (Turner et al., 2011), with the impact on susceptibility being comparable to parameters like sex and body weight. The parasites considered in the study were mostly nematodes, cestodes, and B. microti, making the influence of these cytokines' polymorphisms in other protozoan parasite infections a likely scenario.
Recently, polymorphisms in immunity-related GTPases (IRGs) affecting the rodent immune responses to T. gondii were described in a study comparing the DNA sequences of several inbred and wild-derived mice (Lilue et al., 2013). Remarkably, the authors showed that while the sequences of all the examined lab mice were highly conserved, genes in the wild-derived mice were extremely diverse, comparable to the diversity of MHC genes. One of those genes, the highly polymorphic Irgb2-b1 from a wild-derived M. musculus, when expressed in C57BL/6 fibroblasts, was sufficient to confer resistance (i.e., prevent cell lysis) to so-called virulent strains of T. gondii. While 1–10 parasites of these strains can kill a lab mouse, IRG-polymorphic wild-derived mice are resistant to infection by much higher numbers of the same T. gondii strain. Apart from highlighting extensive sequence variability of wild-derived but not classical inbred laboratory strains in these gene loci, this work emphasizes that the definition of virulence is heavily dependent on the animal of choice and that its definition should always be accompanied by stating the experimental conditions. In the following section we move from traditional, genetically homogenous and inbred mice to mice manipulated to resemble aspects of the human immune system, and then to genetic crosses between inbred and wild-derived mice. Lastly, we will turn our attention to “dirty mice” and wild rodents in natural settings.
Humanized Mice: Rodents Which Mimic the Human Immune System
Designing an immune system with human features within the mouse—generating humanized mice—has recently emerged as an approach to expand the areas where lab mice can be used to model disease. Generation of humanized mice is based on immunodeficient animals (e.g., SCID, Rag2−/−) whose innate and adaptive immune systems are severely compromised and the animals are instead characterized by increased survival of transplanted human hematopoietic cells (Kaushansky et al., 2014; Good et al., 2015), which produce a large number of different human immune cells in the mouse. Depending on further needs, these mice can be populated with, for example human red blood cells and/or CD34+ hematopoietic stem cells that further give rise to T cells and antigen presenting cells (APC). Recent advances in the development of humanized mice offer the possibility to study human infectious diseases which could previously not be investigated in mice, in the mouse model (Brehm et al., 2013). Even though the use of rodent-infecting Plasmodium spp. such as P. berghei has greatly contributed to understanding the parasite's biology and general principles of protective immune mechanisms in mammals (Craig et al., 2012), it is promising that human-infecting P. falciparum now can be researched in lab mice (Kaushansky et al., 2014). The basic strategy for generating humanized mice, and adaptations of it lead to the generation of mice in which the blood stages of human malaria parasite life cycles could be established (Kaushansky et al., 2014; Good et al., 2015).
More advanced models with engrafted human hepatocytes (FRG-NOD huHep) have been further used to establish the complete development of the pre-erythrocytic liver stage of P. falciparum after mosquito bite, including formation of exo-erythrocytic merozoites, subsequently infectious to human red blood cells in the same mouse (Vaughan et al., 2012). Although, the development of the mature sexual stages (gametocytes) that are necessary to complete the parasite life cycle is still inefficient, it seems possible that complete P. falciparum (and other human Plasmodium spp.) life cycles could be routinely maintained using humanized mice. Recently, such mice were also used to conduct a genetic cross between two P. falciparum strains in those animals, something that so far was only possible in non-human primates, including chimpanzees (Vaughan et al., 2015).
These examples and others from several other infectious agents (Ernst, 2016) suggest that humanized mice will continue to contribute to a new repertoire of mouse translational models. Understanding host specificity factors for a given human pathogen is crucial for the design of susceptible humanized mice, and methods to identify such factors are described in detail by Douam et al. (2015). However promising, the establishment of these mice is relatively new and already several limitations are known (described in more detail in Ernst, 2016), which limit the extent to which the models actually mimic the human immune system. For instance, several mouse cytokines differ largely in their sequences between mouse and human, and IL-13 has no effect on human cells. This might explain the low proportions of certain human immune cell types in humanized mice. In addition, signaling and adhesion molecules are different between humans and mice and, importantly, the expression of murine and not human major histocompatibility complexes impairs the function of T cells. Attempts have been made to account for some of these limitations (Ernst, 2016) but so far humanized mice are probably best considered as a promising, but yet developing, tool in translational research.
Even so, host-specificity has limited the choice of model systems for studying protozoan parasites. The Plasmodium spp. examples and initial attempts with L. major infection in humanized mice (Wege et al., 2012) hold promise for future possibilities to investigate also other human-specific protozoan parasite species in lab mice.
Mixing the Known — Recombinant Inbred Strains and the Collaborative Cross
In order to document an influence of genetic heterogeneity on experimental results, models beyond inbred animals are required (Phifer-Rixey and Nachman, 2015; Chow, 2016). This is also true in translational research, where humans represent a genetically diverse population. When the aim is to find differences across the genome, as in genome-wide association studies (GWAS) where a given phenotype is thought to be linked to genetics, outbred stocks of mice or rats are not a solution since they are “a genetically ill-defined set of laboratory mice that are often used erroneously in toxicology, pharmacology and basic research” (Chia et al., 2005). In addition, their usefulness is limited for practical reasons, e.g., individual phenotypic variability requires larger sample sizes than necessary with inbred strains, or the study will lack statistical power to correlate experimental differences with certain genotypes (see Chia et al., 2005; Festing, 2014). In order to address the problem of limited genetic diversity but avoid the ill-defined genetic composition of wild animals, numerous mouse collections besides the RIS (see section The Mice We Use in Experiments — Who Are They and How Do They Live) have been established.
The concept for producing RIS sets has been extended by the Complex Traits Consortium to produce more genetically variable sets (Chia et al., 2005). These are known as Collaborative Crosses (CC) and are based on a set of 8 defined and sequenced founder strains, including three wild-derived strains of Mus and five traditional inbred strains. Although the set of strains is genetically diverse, each CC strain is at least 90% homogenous and hence genetically well-defined. The CCs were designed specifically for complex trait analysis (Churchill et al., 2004; Threadgill and Churchill, 2012) and the derived Diversity Outbred (DO) population (Churchill et al., 2012) has resulted in an even more genetically diverse mouse population (see Figure 3). Other derivatives of CC's concept exist, like the Heterogeneous Stock mice (Valdar et al., 2006) or the Hybrid Mouse Diversity Panel (Bennett et al., 2010). Besides GWAS studies, which map determinants for non-infectious diseases, CC animals have recently been used to map susceptibility or pathogenesis determinants in bacterial and viral infection models (Durrant et al., 2011; Ferris et al., 2013; Rasmussen et al., 2014; Vered et al., 2014; Gralinski et al., 2015; Lore et al., 2015; Smith et al., 2016). However, no data for parasite infections have been reported so far, and their large potential for exploring how host genotype influences infections needs to be explored in the future. Nevertheless, CC and DO mice also have limitations (Phifer-Rixey and Nachman, 2015). They are all derived from subspecies, which limits the genetic variation and may cause partial hybrid sterility in crosses. This, in turn, might have resulted in the elimination of genetic variation at these genomic loci. However, it is expected that further crosses will improve these models on the genetic level.
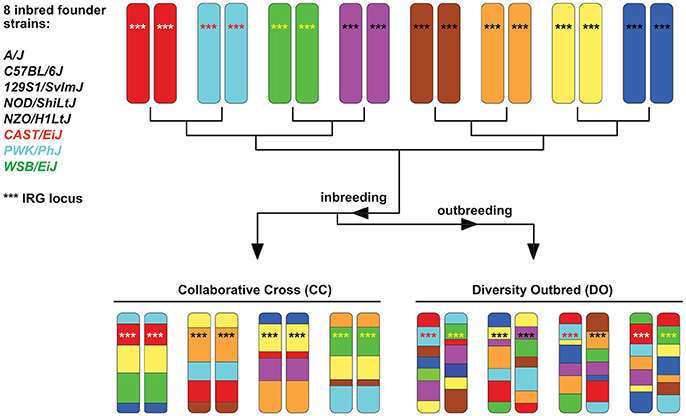
Figure 3. Scheme explaining the principle of Collaborative Cross (CC) and Diversity Outbred (DO) sets (based on Chick et al., 2016). Initially, they all derived from 8 inbred founder strains. Chromosome 11 is given as an example, with the IRG locus (see section When immune responses depend on genetics — selected examples) indicated by ***. The locus is highly homogenous in 5 of the 8 founder strains (black ***) but highly polymorphic in the 3 wild-derived strains CAST, PWK, and WSB, indicated by differently colored *** in the chromosomes.
Yet, these mice will never approach the genetic diversity found in the human population or wild animal populations. In addition, individuals in natural populations encounter seasonal and spatial variability in the environment, as well as differences in climate and food availability. Wild animals are also exposed to and infected with a vast array of parasites and other pathogens, harbor different microbiota, and individuals vary in their demography, behavior and genetic composition. While it is possible to add key elements of natural variation into the above described rodent model systems, there is an increasing interest in moving beyond the controlled laboratory setting to a more realistic scenario.
Excursion 2: Metabolic Disease in Lab Mice and Humans — Is Ecological Complexity Better than SPF Facilities?
Around 25% of the world population has a metabolic syndrome, defined by the International Diabetes Federation as either diabetes / prediabetes, abdominal obesity, high cholesterol or high blood pressure. Animal studies provide important information on these conditions (Bäckhed et al., 2004; Turnbaugh et al., 2006). The role of microbiota has gained interest also in this field because of potential new treatments which can manipulate the microbiome community and function (Borody and Khoruts, 2012). Of interest here are the large numbers of studies conducted in rodents which demonstrate, for instance, alterations in body weight and insulin sensitivity which correlate with changes in microbiota upon antibiotics treatment in these model rodents (Bäckhed et al., 2004; Turnbaugh et al., 2006). Although similar data also exists from human studies and therefore support that translation of results from rodent models to humans in this case is possible, a recent contribution to this topic questions the rodent model to mimic metabolic disease and microbiota correlations in humans. In a study performed on 57 overweight and obese adult men, the systemic effects of two antibiotic treatments compared to placebo were investigated (Reijnders et al., 2016). Changes in microbiota composition (detected by 16S rRNA microarray analysis) were observed for specific antibiotics against gram-positive bacteria, but no differences were seen with the broad-spectrum antibiotic. On most other readouts, the authors did not see significant responses to antibiotics treatment. Hence, changes in microbiota composition did not correlate with changes in systemic functions in humans (e.g., insulin sensitivity, energy metabolism and gut permeability; Reijnders et al., 2016), which is in contrast with rodent data (Bäckhed et al., 2004; Turnbaugh et al., 2006). Reijnders et al. (2016) discuss hypotheses for these discrepancies, mentioning treatment duration and the method of antibiotic intake (capsules or in water). In addition, a possibly important difference between the described rodent studies and the human study is the fact that humans are a “wild” population. The genetic heterogeneity and environmental influences in the human population of 57 men are indeed different from the SPF bred rodents. The effects of both previous and current microbe colonization and/or infections do influence immune responses in an individual and constitute an important difference between wild and controlled laboratory populations (reviewed in Tao and Reese, 2017). Possibly, the use of a “dirty” or wild rodent population would be a more suitable choice when the aim is to investigate correlations in a highly complex biological system.
Getting the Rodent Model “Dirty”
In the continuum of approaches that can be employed to better understand protozoan parasite infection and immunity, “dirty” animals taken from the wild or laboratory animals exposed to wild cage-mates have emerged as a promising model (Maizels and Nussey, 2013). Arguing for such translational models, recent results demonstrate that inbred mice reared in SPF conditions have the immunological phenotype of neonatal humans, lacking effector-differentiated and mucosally distributed memory T cells (Beura et al., 2016). In contrast, “dirty” M. musculus brought in from either a pet shop or from feral barn populations had immune responses more similar to adult humans, with high levels of memory CD8+ cells, likely due to diverse microbial exposure and infection. These changes in both the innate and adaptive immune cellular responses and immune gene expression could also be recapitulated by co-housing previously SPF inbred mice with pet shop mice. While about 20% of the SPF mice died due to microbial infection, the immune response of those that survived also resembled adult humans within 4–8 weeks, with effector-differentiated and mucosal memory T cells. In addition, within that short time frame, co-housed mice responded similarly to the wild-caught pet shop mice in terms of infection, such that they were significantly more resistant, amongst other pathogens, to challenge with the cerebral malaria model P. berghei (Beura et al., 2016). Another research group has aimed to make their inbred laboratory mouse strains “dirty” by giving them sequential infection with mouse herpes virus, influenza and an intestinal helminth in order to test how this more natural pattern of exposure to pathogens may affect immune variation and expression after vaccination (Reese et al., 2016). They found that co-infected mice had different immune gene signatures, cytokine expression and antibody levels in the blood both before and after yellow fever virus vaccination compared with their SPF lab mice controls. These expression patterns resembled those of pet store-raised mice. While getting the traditional sterile laboratory mouse models dirty may pose logistical challenges, such results should encourage researchers to revisit abandoned vaccine candidates as well as to establish different routines for testing new ones.
Benefits of Using Wild Mice
Studies on dirty mice with the benefits described above still lack other aspects of natural variation that are important (Pedersen and Babayan, 2011). Thus, there is a need for wild model organisms that permit robust studies of the individual and environmental variation inherent in natural populations (including humans). Populations of wild mice vary in many of the same ways as humans (e.g., age, sex, condition, resources, parasite exposure, infection/co-infection, genetics, etc.), yet can provide a tractable, experimental system to test the importance of natural variability on infection, immunity and disease control. There are several key epidemiological features in wild mouse populations that closely resemble human infection dynamics, such as having great variation in infection probability, burdens and disease severity across individuals. Moreover, wild mice are commonly found chronically infected with parasites, suggesting either a high frequency of re-infection, long-lasting infections, or both (Pedersen and Babayan, 2011; Knowles et al., 2013).
One approach to start a research program on wild rodents is to study the traditional laboratory mouse species (M. musculus) in the wild (Potter et al., 1986; Viney et al., 2015). Abolins et al. (2011) found that the immune function of wild-caught M. musculus was significantly greater than lab-reared C57BL/6 mice, such that after immunization with a novel antigen wild-caught mice had higher concentrations of total IgG and IgE, produced higher and more avid concentrations of antigen-specific IgG, and had greater activation of T helper cells, macrophages and dendritic cells than lab-reared mice. While wild M. musculus offer a great parallel to lab-reared mice and can serve as comparisons for protozoan infection in the laboratory, many studies do not exhaustively sample for ectoparasites and protozoans and their true infection status is not well described. Commonly, wild M. musculus are reported to be infected with mainly ectoparasites and a few nematodes (mostly Syphacia spp. pinworms; e.g., Weldon et al., 2015); however there are records of natural infections with Giardia muris, Spironucleus muris, and Encephalitozoon cuniculi (Baker, 1998), Eimeria spp. (Ball and Lewis, 1984), Cryptosporidium spp. (Backhans et al., 2013), and T. gondii (Kijlstra et al., 2008).
Rodents beyond Wild Mice Are Natural Hosts of a Wide Variety of Protozoa
Beyond M. musculus, there are several well-studied wild rodents that are both commonly infected with protozoan parasites and also offer tractable wild model systems for both longitudinal and experimental studies of infection and immunity. In North America, much work has focused on white-footed mice and deer mice (Peromyscus leucopus and P. maniculatus), both because they are very abundant and widespread, but also because they are competent reservoirs of important emerging zoonotic pathogens (e.g., Hantavirus and Borrelia spp.; Bedford and Hoekstra, 2015). In Europe, wood mice (Apodemus sylvaticus; e.g., Knowles et al., 2013), yellow-necked mice (A. flavicollis; e.g., Ferrari et al., 2004), bank voles (Myodes glareolus; e.g., Withenshaw et al., 2016), and field voles (M. agrestis; Smith et al., 2005; Turner et al., 2014) have been commonly studied as models for wild host-pathogen interactions and are all regularly infected with protozoan parasites. For example, wild populations of A. sylvaticus in the United Kingdom have been found to be infected with C. parvum, C. muris (Chalmers et al., 1997); > five species of Eimeria (Ball and Lewis, 1984; Higgs and Nowell, 2000); Babesia sp. and Hepatozoon sp. (Turner, 1986); two species of Trypanosoma (Noyes et al., 2002), Frenkelia microti (Svobodova et al., 2004) and T. gondii (Jackson and Siim, 1986). It is very likely that this list is a far from exhaustive.
The benefits of using wild rodent-parasite models to better understand protozoan infection dynamics include the ability to: (i) conduct longitudinal field experiments which follow marked individuals throughout their lives while measuring infection status, physiological and demographic metrics (Knowles et al., 2013; Pedersen and Antonovics, 2013; Turner et al., 2014), and crucially (ii) test the efficacy of disease control interventions at the individual and population level in an ecologically relevant environment (Knowles et al., 2013; Pedersen and Antonovics, 2013). For example, in a population of wild field voles (M. agrestis) the researchers repeatedly treated one population with a standard insecticide to reduce the prevalence of fleas, and in turn, found that this reduced the prevalence of vector-transmitted Trypanosoma spp. by ~33% (Smith et al., 2005). In addition, in experimental field studies of both P. maniculatus and P. leucopus in the US, and A. sylvaticus in the UK, anthelmintic treatment was used to reduce nematode burdens within specific, marked animals. The treatment was found to unexpectedly increase the prevalence and/or intensity of co-infecting Eimeria spp. suggesting strong antagonistic within-host interactions between a worm and a protozoon (Knowles et al., 2013; Pedersen and Antonovics, 2013).
Research on wild rodents benefit from the extensive immunological toolbox developed in lab mice (Pedersen and Babayan, 2011). In wild populations of A. sylvaticus, innate immune responsiveness, as measured by splenocyte tumor necrosis factor responses to toll-like receptor (TLR) agonists, was found to correlate positively with Eimeria spp. fecal oocysts counts, most strongly with receptors TLR7 and TLR9 (Jackson et al., 2009). More recently, the availability of genomes for wild rodents has enabled the ability to measure immunological expression in wild rodent populations. A recent investigation of wild field voles measured expression of a wide range of innate and adaptive responses by cultured and stimulated splenocytes. Importantly, repeated measures from peripheral blood samples of IFN-y, Gata3 and IL-10 expression enabled the authors to test for correlations with specific parasite infections (Jackson et al., 2014). Taken together, wild rodents reach large sample sizes, can be repeatedly recaptured using live traps, marked and followed before and after interventions, and are commonly infected with protozoan parasites. Studying the dynamics of protozoan infections in wild rodents is a valuable resource for expanding our knowledge in infection biology and might thus be a useful addition for translational research on human protozoan infections.
A Case for Going Wild: Do T. gondii-Induced Behavioral Changes Exist in Natural Habitats?
How relevant are findings which suggest parasite influences on lab mouse behavior when performed in lab environments? The so-called “manipulation hypothesis” of a T. gondii infection in rodents suggests that infection leads to subsequent changes in the animal's behavior, with one consequence being that they lose their fear for feline odor (e.g., fur or urine). Cats and other felids are the only definite hosts where sexual reproduction of T. gondii can take place. Therefore, at first sight it makes sense that such “manipulated” infected rodents would experience more fatal encounters with a cat than non-infected ones, thereby increase the chance for T. gondii to sexually reproduce with another strain from a second subsequent infected prey.
The advantage or necessity of this scenario for parasite sexual reproduction in the wild has been called into question (Worth et al., 2013), but here we focus on the fact that all reported experiments were done exclusively in lab animals (Worth et al., 2014). At first sight this might not seem problematic since M. musculus and T. gondii naturally occur together. However, it is well known, but not necessarily well appreciated, that behavioral studies of rodents can be influenced by the methods used, housing conditions, genetic background and whether they are lab or wild-derived animals (Wolff, 2003; Beckers et al., 2009; Fonio et al., 2012; Chalfin et al., 2014; Newman et al., 2015). Even differences in the microbiota can have profound effects (Hsiao et al., 2013; see section Microbiota as a General Confounder for Rodent Experiments). Moreover, lab mice have been selected for decades for docile behavior, while wild mice show anxious behavior under natural conditions (Latham and Mason, 2004; Yoshiki and Moriwaki, 2006; Fonio et al., 2012; Chalfin et al., 2014).
How well does the manipulation hypothesis apply to the natural situation of predator (cat) and prey (T. gondii-infected mouse or rat)? Ecological observations might explain some of the observed discrepancies, i.e., no behavioral differences were found in one study but were indeed found in another (Worth et al., 2014). Several studies indicate that predation risk of wild or wild-derived small rodents depends more on habitat characteristics (e.g., ability to hide) than on whether the rodent senses a present predator by its odor or even by its physical presence (Orrock, 2004; Powell and Banks, 2004; Verdolin, 2006). Aversive responses to predator odor can also differ dramatically between individual lab rats (Hogg and File, 1994) and, importantly, between lab mice and wild mice (Coulston et al., 1993; Hebb et al., 2004). Thus, experiments with “fearless” lab mice in non-natural terrains may not accurately reflect the behavioral changes induced by a parasite like T. gondii under natural conditions.
Experimental Challenges and Available Resources for Non-traditional Rodent Models
Having provided a number of reasons for considering wild rodents as alternatives, we will briefly address the experimental challenges. Approaching studies on non-model rodents is demanding but the toolbox has improved significantly compared to a decade ago (Pedersen and Babayan, 2011; Zimmerman et al., 2014). Method development in the fields of genomics, transcriptomics and proteomics together with increasing affordability provide better prerequisites for research on non-model alternatives (Jackson, 2015). Next-generation sequencing has led to constantly expanding genomic data. This is demonstrated by the nearly 4,000 eukaryotic genomes available on NCBI Genome (February, 2017) as compared to around 650 in 2013 (Ellegren, 2014).
Database Resources for Wild Rodent Genomes
Any resources available for lab mice are to varying extents useful starting-points for work on wild rodents. The Mouse Genomes Project is the biggest collection of genomic data on rodents (Table S1). Currently it consists of whole-genome assemblages and strain-specific gene annotations of 16 inbred and wild-derived mouse strains. A goal of this project is the classification of sequence variations between common laboratory strains compared to the reference strain C57BL/6J (Adams et al., 2015; Doran et al., 2016). All sequence reads, variants and assemblages can be useful references for highly recombinant outbred strains (Nicod et al., 2016) or wild rodent genomes. There are also increasing numbers of genomes and/or transcriptomes available for wild rodents (e.g., A. sylvaticus, M. glareolus, and M. agrestis; see Figure 4), with most of them being “work-in-progress” considering assemblage status and annotations (for details see Table S1 and links therein). The quality and coverage of these genomes vary and there is for instance no clear definition of a required coverage for referring to DNA sequences as a “genome” (Ellegren, 2014). However, they do provide a good source for homology searches for a gene-of-interest, primer design for PCR applications etc. Naturally, purification of DNA, RNA or proteins and functional PCR protocols may require protocol optimization when applied to new species but otherwise follow established schemes. Some database resources and other initiatives to promote such development are discussed below and summarized in Table S1.
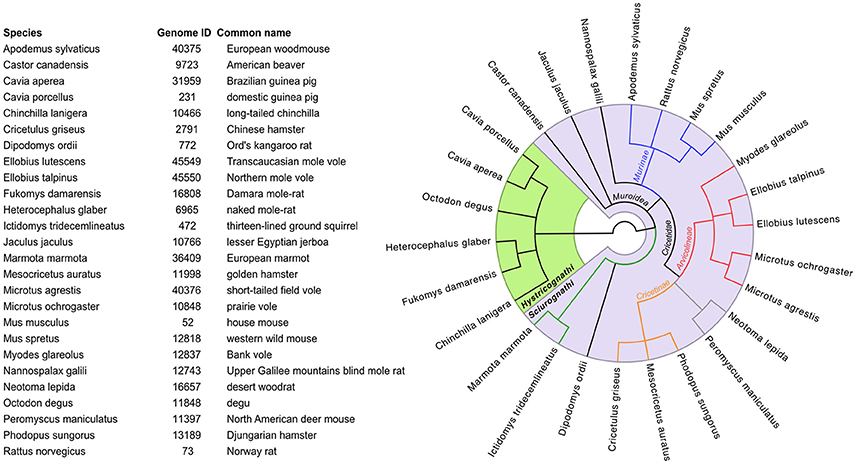
Figure 4. Names of rodents (with links in Table S2 to their available genome sequences at NCBI), together with their phylogenetic relationship (tree computed at https://www.ncbi.nlm.nih.gov/Taxonomy/CommonTree/wwwcmt.cgi).
While reference genomes are not a prerequisite for some studies they are, for instance, indispensable as a template in quantitative gene expression studies with high-throughput sequencing of RNA (RNA-seq; Vijay et al., 2013). However, bioinformatics pipelines are often developed for established model organisms and may require considerable adjustments for non-model organisms (Ekblom and Wolf, 2014). Although such limitations may hamper the speed of omics applications to non-model organisms, genomes of non-model rodents will serve as excellent resources for developing species-specific tools to measure, for example, expression of immunological responses to infection. Readers that are interested in considering genome sequencing for their own non-model organism are referred to a recent step-by-step introduction of the required workflows (Ekblom and Wolf, 2014) and to the Generic Model Organism Database (GMOD) initiative, which provides software tools and data models for subsequent representation of their annotated genomes and curated data of their model organism (O'Connor et al., 2008).
Transcriptomes Do Not Necessarily Predict Protein Levels
It should be emphasized that genomic and transcriptomic data, as valuable as they are, provide only indirect means with regards to proteomic output in response to infection. In general, the relationship between the concentration of a given transcript and its encoded protein(s) is difficult to predict just by RNA-seq or qPCR data (Liu et al., 2016). For example, Chick et al. (2016) used the currently most sensitive technology for abundance determination of both transcripts and proteins and applied them to CC and DO mice (see section Humanized Mice: Rodents Which Mimic the Human Immune System and Figure 3). They showed that for many genes the levels of the corresponding protein varied substantially in genetically divergent mice. Sex also influenced protein amounts within a given species (Chick et al., 2016). These recent data emphasize the importance of quantitative proteomic measures in general to complement or validate transcriptomic data, but also highlight that genetic diversity within mice influences the results.
Antibodies, Cytokines, and Protein Quantification
Antibody-based assays are still the cornerstone for qualitative and quantitative determination of immunological parameters like chemokines or cytokines but also other proteins of interest. Numerous well-defined and evaluated reagents exist for lab mice and rats but their usefulness for wild rodents with respect to cross-reactivity is largely unexplored and presumably quite low in many cases. The same applies to immunological effector molecules like cytokines, for which IFN-γ is a good example. IFN-γ is known to be highly species-specific, which made the production of a recombinant protein active with M. glareolus or Microtus spp. cell lines a prerequisite (Torelli et al, in preparation). Starting from genomic sequences and going to the purification of active recombinant protein from E. coli required less than half a year and will now provide the scientific community with this important cytokine.
Developing antibodies that (cross)react with wild rodents is certainly much more time and resource-consuming, but feasible. An alternative could be parallel (or selective) reaction monitoring (PRM, SRM) which are mass spectrometry-based methods that quantify unique, specific peptide sequences of a given protein (Rauniyar, 2015; Bourmaud et al., 2016). The method was recently used to quantify several cytokines and chemokines from human cells (Muqaku et al., 2015). The appealing aspect of this admittedly demanding method lies in the fact that by carefully selecting peptide sequences conserved between rodent species, they could be used across those species at relatively low cost, once established (Hüttenhain et al., 2009). Ideally, it could thus be regarded as a community effort. A database with corresponding peptides from human and mouse proteomes does exist (see Table S1) and constitutes a useful starting point (Peptide Atlas; Deutsch et al., 2008).
Given the availability of published genomic and transcriptomic data of wild rodent species (Table S2), work similar to the studies mentioned will hopefully expand the current toolbox for non-model rodents in the near future. A dedicated web site with information on such shared resources, but also on cross-reacting reagents such as polyclonal or monoclonal antibodies or commercial cytokines and other proteins tested in non-model rodents, although not yet existing, would greatly boost the interest and ease of use of non-model organisms in future studies.
Concluding Remarks
We are convinced that rodents will continue to be important translational models for research on protozoan parasites, given that appropriate considerations are made during experimental design. By providing some examples where translation from rodent disease models to human medicine has failed, and, more importantly, by pointing at identified reasons for inconclusive or misleading data, we wish to inspire readers to consider more than the most convenient model for future experiments. Making use of rich database-resources that are available for investigating, e.g., expected phenotypes of mice, will aid in this respect. We also hope that readers are encouraged to consider and control for various confounders such as microbiota influences and housing conditions in their experimental designs. While wild models pose some challenges, we have pointed out that these rodents possess distinct advantages with regards to genetic variability and environmental exposures that can reflect immunological responses to parasites in humans more adequately than current lab models. The increasing availability of genome and transcriptome datasets as well as improved methods for quantitative proteomics already show their impact on wild infection biology.
Author Contributions
TE, FT, CK, AP, and FS contributed to the text and approved its final version.
Funding
TE and FT are supported by and TE, FT, CK, and FS are members of the Research Training Group 2046 “Parasite Infections: From Experimental Models to Natural Systems,” funded by the Deutsche Forschungsgemeinschaft. Own cited work from FS and CK was supported by the Robert Koch Institute. AP is supported by a Chancellors Fellowship from the University of Edinburgh, and her cited work was supported by a NERC grant (NE/G006830/1 and NE/G007349/1) and a Wellcome Trust Centre for Infection, Immunity and Evolution Advanced Fellowship (no. 095831).
Conflict of Interest Statement
The authors declare that the research was conducted in the absence of any commercial or financial relationships that could be construed as a potential conflict of interest.
Acknowledgments
The authors thank Toni Aebischer and Ankur Midha for valuable comments and suggestions on the manuscript.
Supplementary Material
The Supplementary Material for this article can be found online at: http://journal.frontiersin.org/article/10.3389/fcimb.2017.00238/full#supplementary-material
References
Abolins, S. R., Pocock, M. J., Hafalla, J. C., Riley, E. M., and Viney, M. E. (2011). Measures of immune function of wild mice, Mus musculus. Mol. Ecol. 20, 881–892. doi: 10.1111/j.1365-294X.2010.04910.x
Adam, E., Pierrot, C., Lafitte, S., Godin, C., Saoudi, A., Capron, M., et al. (2003). The age-related resistance of rats to Plasmodium berghei infection is associated with differential cellular and humoral immune responses. Int. J. Parasitol. 33, 1067–1078. doi: 10.1016/s0020-7519(03)00176-0
Adams, D. J., Doran, A. G., Lilue, J., and Keane, T. M. (2015). The Mouse Genomes Project: a repository of inbred laboratory mouse strain genomes. Mamm. Genome 26, 403–412. doi: 10.1007/s00335-015-9579-6
Akhoundi, M., Kuhls, K., Cannet, A., Votypka, J., Marty, P., Delaunay, P., et al. (2016). A historical overview of the classification, evolution, and dispersion of Leishmania parasites and sandflies. PLoS Negl. Trop. Dis. 10:e0004349. doi: 10.1371/journal.pntd.0004349
Alfred, J., and Baldwin, I. T. (2015). The natural history of model organisms: new opportunities at the wild frontier. Elife 4:e06956. doi: 10.7554/eLife.06956
Andrews, K. T., Fisher, G., and Skinner-Adams, T. S. (2014). Drug repurposing and human parasitic protozoan diseases. Int. J. Parasitol. Drugs Drug Resist. 4, 95–111. doi: 10.1016/j.ijpddr.2014.02.002
Ankarklev, J., Jerlstrom-Hultqvist, J., Ringqvist, E., Troell, K., and Svard, S. G. (2010). Behind the smile: cell biology and disease mechanisms of Giardia species. Nat. Rev. Microbiol. 8, 413–422. doi: 10.1038/nrmicro2317
Ankeny, R. A., and Leonelli, S. (2011). What's so special about model organisms? Stud. Hist. Phil. Sci. 42, 313–323. doi: 10.2307/40985626
Antoine-Moussiaux, N., Magez, S., and Desmecht, D. (2008). Contributions of experimental mouse models to the understanding of African trypanosomiasis. Trends Parasitol. 24, 411–418. doi: 10.1016/j.pt.2008.05.010
Aplenc, R., and Lange, B. (2004). Pharmacogenetic determinants of outcome in acute lymphoblastic leukaemia. Br. J. Haematol. 125, 421–434. doi: 10.1111/j.1365-2141.2004.04932.x
Ayadi, A., Ferrand, G., Cruz, I. G. D., and Warot, X. (2011). Mouse breeding and colony management. Curr. Protoc. Mouse Biol. 1, 239–264. doi: 10.1002/9780470942390.mo100214
Backhans, A., Jacobson, M., Hansson, I., Lebbad, M., Lambertz, S. T., Gammelgard, E., et al. (2013). Occurrence of pathogens in wild rodents caught on Swedish pig and chicken farms. Epidemiol. Infect. 141, 1885–1891. doi: 10.1017/s0950268812002609
Bäckhed, F., Ding, H., Wang, T., Hooper, L. V., Koh, G. Y., Nagy, A., et al. (2004). The gut microbiota as an environmental factor that regulates fat storage. Proc. Natl. Acad. Sci. U.S.A. 101, 15718–15723. doi: 10.1073/pnas.0407076101
Baker, D. G. (1998). Natural pathogens of laboratory mice, rats, and rabbits and their effects on research. Clin. Microbiol. Rev. 11, 231–266.
Ball, S. J., and Lewis, D. C. (1984). Eimeria (Protozoa: Coccidia) in wild populations of some British rodents. J. Zool. 202, 373–381. doi: 10.1111/j.1469-7998.1984.tb05089.x
Barash, N. R., Maloney, J. G., Singer, S., and Dawson, S. C. (2016). Giardia alters commensal microbial diversity throughout the murine gut. bioRxiv. doi: 10.1101/087122
Bayne, K., and Würbel, H. (2012). “Mouse enrichment,” in The Laboratory Mouse, 2nd Edn., ed H. J. Hedrich (Boston, MA: Academic Press), 547–566.
Beck, J. A., Lloyd, S., Hafezparast, M., Lennon-Pierce, M., Eppig, J. T., Festing, M. F., et al. (2000). Genealogies of mouse inbred strains. Nat. Genet. 24, 23–25. doi: 10.1038/71641
Beckers, J., Wurst, W., and de Angelis, M. H. (2009). Towards better mouse models: enhanced genotypes, systemic phenotyping and envirotype modelling. Nat. Rev. Genet. 10, 371–380. doi: 10.1038/nrg2578
Bedford, N. L., and Hoekstra, H. E. (2015). Peromyscus mice as a model for studying natural variation. Elife 4:e06813. doi: 10.7554/eLife.06813
Bennett, B. J., Farber, C. R., Orozco, L., Kang, H. M., Ghazalpour, A., Siemers, N., et al. (2010). A high-resolution association mapping panel for the dissection of complex traits in mice. Genome Res. 20, 281–290. doi: 10.1101/gr.099234.109
Bernin, H., and Lotter, H. (2014). Sex bias in the outcome of human tropical infectious diseases: influence of steroid hormones. J. Infect. Dis. 209(Suppl. 3), S107–S113. doi: 10.1093/infdis/jit610
Beura, L. K., Hamilton, S. E., Bi, K., Schenkel, J. M., Odumade, O. A., Casey, K. A., et al. (2016). Normalizing the environment recapitulates adult human immune traits in laboratory mice. Nature 532, 512–516. doi: 10.1038/nature17655
Bhattacharya, P., Dey, R., Dagur, P. K., Joshi, A. B., Ismail, N., Gannavaram, S., et al. (2016). Live attenuated Leishmania donovani centrin knock out parasites generate non-inferior protective immune response in aged mice against visceral leishmaniasis. PLoS Negl. Trop. Dis. 10:e0004963. doi: 10.1371/journal.pntd.0004963
Bolker, J. (2012). Model organisms: there's more to life than rats and flies. Nature 491, 31–33. doi: 10.1038/491031a
Borody, T. J., and Khoruts, A. (2012). Fecal microbiota transplantation and emerging applications. Nat. Rev. Gastroenterol. Hepatol. 9, 88–96. doi: 10.1038/nrgastro.2011.244
Bourmaud, A., Gallien, S., and Domon, B. (2016). Parallel reaction monitoring using quadrupole-Orbitrap mass spectrometer: principle and applications. Proteomics 16, 2146–2159. doi: 10.1002/pmic.201500543
Brehm, M. A., Jouvet, N., Greiner, D. L., and Shultz, L. D. (2013). Humanized mice for the study of infectious diseases. Curr. Opin. Immunol. 25, 428–435. doi: 10.1016/j.coi.2013.05.012
Buer, J., and Balling, R. (2003). Mice, microbes and models of infection. Nat. Rev. Genet. 4, 195–205. doi: 10.1038/nrg1019
Chalfin, L., Dayan, M., Levy, D. R., Austad, S. N., Miller, R. A., Iraqi, F. A., et al. (2014). Mapping ecologically relevant social behaviours by gene knockout in wild mice. Nat. Commun. 5, 4569. doi: 10.1038/ncomms5569
Chalmers, R. M., Sturdee, A. P., Bull, S. A., Miller, A., and Wright, S. E. (1997). The prevalence of Cryptosporidium parvum and C. muris in Mus domesticus, Apodemus sylvaticus and Clethrionomys glareolus in an agricultural system. Parasitol. Res. 83, 478–482. doi: 10.1007/s004360050283
Checkley, W., White, A. C. Jr., Jaganath, D., Arrowood, M. J., Chalmers, R. M., Chen, X. M., et al. (2015). A review of the global burden, novel diagnostics, therapeutics, and vaccine targets for cryptosporidium. Lancet Infect. Dis. 15, 85–94. doi: 10.1016/s1473-3099(14)70772-8
Chia, R., Achilli, F., Festing, M. F. W., and Fisher, E. M. C. (2005). The origins and uses of mouse outbred stocks. Nat. Genet. 37, 1181–1186. doi: 10.1038/ng1665
Chick, J. M., Munger, S. C., Simecek, P., Huttlin, E. L., Choi, K., Gatti, D. M., et al. (2016). Defining the consequences of genetic variation on a proteome-wide scale. Nature 534, 500–505. doi: 10.1038/nature18270
Chow, C. Y. (2016). Bringing genetic background into focus. Nat. Rev. Genet. 17, 63–64. doi: 10.1038/nrg.2015.9
Churchill, G. A., Airey, D. C., Allayee, H., Angel, J. M., Attie, A. D., Beatty, J., et al. (2004). The Collaborative Cross, a community resource for the genetic analysis of complex traits. Nat. Genet. 36, 1133–1137. doi: 10.1038/ng1104-1133
Churchill, G. A., Gatti, D. M., Munger, S. C., and Svenson, K. L. (2012). The Diversity Outbred mouse population. Mamm. Genome 23, 713–718. doi: 10.1007/s00335-012-9414-2
Cirelli, K. M., Gorfu, G., Hassan, M. A., Printz, M., Crown, D., Leppla, S. H., et al. (2014). Inflammasome sensor NLRP1 controls rat macrophage susceptibility to Toxoplasma gondii. PLoS Pathog. 10:e1003927. doi: 10.1371/journal.ppat.1003927
Clayton, J. A., and Collins, F. S. (2014). Policy: NIH to balance sex in cell and animal studies. Nature 509, 282–283. doi: 10.1038/509282a
Clevers, H. (2016). Modeling development and disease with organoids. Cell 165, 1586–1597. doi: 10.1016/j.cell.2016.05.082
Coulston, S., Stoddart, D. M., and Crump, D. R. (1993). Use of predator odors to protect chick-peas from predation by laboratory and wild mice. J. Chem. Ecol. 19, 607–612. doi: 10.1007/bf00984995
Couzin-Frankel, J. (2013). When mice mislead. Science 342, 922–925. doi: 10.1126/science.342.6161.922
Cowman, A. F., Healer, J., Marapana, D., and Marsh, K. (2016). Malaria: biology and disease. Cell 167, 610–624. doi: 10.1016/j.cell.2016.07.055
Craig, A. G., Grau, G. E., Janse, C., Kazura, J. W., Milner, D., Barnwell, J. W., et al. (2012). The role of animal models for research on severe malaria. PLoS Pathog. 8:e1002401. doi: 10.1371/journal.ppat.1002401
Denny, J. E., Powell, W. L., and Schmidt, N. W. (2016). Local and long-distance calling: conversations between the gut microbiota and intra- and extra-gastrointestinal tract infections. Front. Cell. Infect. Microbiol. 6:41. doi: 10.3389/fcimb.2016.00041
Deutsch, E. W., Lam, H., and Aebersold, R. (2008). PeptideAtlas: a resource for target selection for emerging targeted proteomics workflows. EMBO Rep. 9, 429–434. doi: 10.1038/embor.2008.56
Dietrich, M. R., Ankeny, R. A., and Chen, P. M. (2014). Publication trends in model organism research. Genetics 198, 787–794. doi: 10.1534/genetics.114.169714
Doran, A. G., Wong, K., Flint, J., Adams, D. J., Hunter, K. W., and Keane, T. M. (2016). Deep genome sequencing and variation analysis of 13 inbred mouse strains defines candidate phenotypic alleles, private variation and homozygous truncating mutations. Genome Biol. 17, 167. doi: 10.1186/s13059-016-1024-y
Douam, F., Gaska, J. M., Winer, B. Y., Ding, Q., von Schaewen, M., and Ploss, A. (2015). Genetic dissection of the host tropism of human-tropic pathogens. Annu. Rev. Genet. 49, 21–45. doi: 10.1146/annurev-genet-112414-054823
Durrant, C., Tayem, H., Yalcin, B., Cleak, J., Goodstadt, L., de Villena, F. P., et al. (2011). Collaborative Cross mice and their power to map host susceptibility to Aspergillus fumigatus infection. Genome Res. 21, 1239–1248. doi: 10.1101/gr.118786.110
Eastwood, D., Findlay, L., Poole, S., Bird, C., Wadhwa, M., Moore, M., et al. (2010). Monoclonal antibody TGN1412 trial failure explained by species differences in CD28 expression on CD4+ effector memory T-cells. Br. J. Pharmacol. 161, 512–526. doi: 10.1111/j.1476-5381.2010.00922.x
Ekblom, R., and Wolf, J. B. W. (2014). A field guide to whole-genome sequencing, assembly and annotation. Evol. Appl. 7, 1026–1042. doi: 10.1111/eva.12178
Ellegren, H. (2014). Genome sequencing and population genomics in non-model organisms. Trends Ecol. Evol. 29, 51–63. doi: 10.1016/j.tree.2013.09.008
Ericsson, A. C., Davis, J. W., Spollen, W., Bivens, N., Givan, S., Hagan, C. E., et al. (2015). Effects of vendor and genetic background on the composition of the fecal microbiota of inbred mice. PLoS ONE 10:e0116704. doi: 10.1371/journal.pone.0116704
Ernst, W. (2016). Humanized mice in infectious diseases. Comp. Immunol. Microbiol. Infect. Dis. 49, 29–38. doi: 10.1016/j.cimid.2016.08.006
Ewald, S. E., Chavarria-Smith, J., and Boothroyd, J. C. (2014). NLRP1 is an inflammasome sensor for Toxoplasma gondii. Infect. Immun. 82, 460–468. doi: 10.1128/iai.01170-13
Ferrari, N., Cattadori, I. M., Nespereira, J., Rizzoli, A., and Hudson, P. J. (2004). The role of host sex in parasite dynamics: field experiments on the yellow-necked mouse Apodemus flavicollis. Ecol. Lett. 7, 88–94. doi: 10.1046/j.1461-0248.2003.00552.x
Ferris, M. T., Aylor, D. L., Bottomly, D., Whitmore, A. C., Aicher, L. D., Bell, T. A., et al. (2013). Modeling host genetic regulation of influenza pathogenesis in the collaborative cross. PLoS Pathog. 9:e1003196. doi: 10.1371/journal.ppat.1003196
Festing, M., and Fisher, E. (2000). Mighty mice - Clarence Little's brainwave gave biomedical researchers their best friend. Nature 404, 815–815. doi: 10.1038/35009167
Festing, M. F. W. (2014). Evidence should trump intuition by preferring inbred strains to outbred stocks in preclinical research. ILAR J. 55, 399–404. doi: 10.1093/ilar/ilu036
Fiebiger, U., Bereswill, S., and Heimesaat, M. M. (2016). Dissecting the interplay between intestinal microbiota and host immunity in health and disease: lessons learned from germfree and gnotobiotic animal models. Eur. J. Microbiol. Immunol. 6, 253–271. doi: 10.1556/1886.2016.00036
Fitzpatrick, J. L. (2013). Global food security: the impact of veterinary parasites and parasitologists. Vet. Parasitol. 195, 233–248. doi: 10.1016/j.vetpar.2013.04.005
Fletcher, S. M., Stark, D., Harkness, J., and Ellis, J. (2012). Enteric protozoa in the developed world: a public health perspective. Clin. Microbiol. Rev. 25, 420–449. doi: 10.1128/cmr.05038-11
Flórez-Vargas, O., Brass, A., Karystianis, G., and Bramhall, M. (2016). Bias in the reporting of sex and age in biomedical research on mouse models. Elife 5:e13615. doi: 10.7554/eLife.13615
Fonio, E., Golani, I., and Benjamini, Y. (2012). Measuring behavior of animal models: faults and remedies. Nat. Methods 9, 1167–1170. doi: 10.1038/nmeth.2252
Foster, J. A., and McVey Neufeld, K. A. (2013). Gut-brain axis: how the microbiome influences anxiety and depression. Trends Neurosci. 36, 305–312. doi: 10.1016/j.tins.2013.01.005
Gacias, M., Gaspari, S., Santos, P. M. G., Tamburini, S., Andrade, M., Zang, F., et al. (2016). Microbiota-driven transcriptional changes in prefrontal cortex override genetic differences in social behavior. Elife 5:e13442. doi: 10.7554/eLife.13442
Garenne, M. (2015). Demographic evidence of sex differences in vulnerability to infectious diseases. J. Infect. Dis. 211, 331–332. doi: 10.1093/infdis/jiu448
German Federal Ministry of Food and Agriculture (2011). Versuchstierzahlen 2011. Available online at: http://www.bmel.de/DE/Tier/Tierschutz/_texte/TierschutzTierforschung.html?docId=8596776#doc8596776bodyText11
Giroud, C., Ottones, F., Coustou, V., Dacheux, D., Biteau, N., Miezan, B., et al. (2009). Murine models for Trypanosoma brucei gambiense disease progression—from silent to chronic infections and early brain tropism. PLoS Negl. Trop. Dis. 3:e509. doi: 10.1371/journal.pntd.0000509
Good, M. F., Hawkes, M. T., and Yanow, S. K. (2015). Humanized mouse models to study cell-mediated immune responses to liver-stage malaria vaccines. Trends Parasitol. 31, 583–594. doi: 10.1016/j.pt.2015.06.008
Gordon, S. (2007). The macrophage: past, present and future. Eur. J. Immunol. 37(Suppl. 1), S9–S17. doi: 10.1002/eji.200737638
Gralinski, L. E., Ferris, M. T., Aylor, D. L., Whitmore, A. C., Green, R., Frieman, M. B., et al. (2015). Genome wide identification of SARS-CoV susceptibility loci using the Collaborative Cross. PLoS Genet. 11:e1005504. doi: 10.1371/journal.pgen.1005504
Grubb, S. C., Bult, C. J., and Bogue, M. A. (2014). Mouse phenome database. Nucleic Acids Res. 42, D825–D834. doi: 10.1093/nar/gkt1159
Guénet, J. L., Benavides, F., and Panthier, J. J. (2015). “The different categories of genetically standardized populations of laboratory mice,” in Genetics of the Mouse, eds J. L. Guénet, F. Benavides, J. J. Panthier, and X. Montagutelli (Berlin Heidelberg: Springer-Verlag), 319–359.
Guenet, J. L., and Bonhomme, F. (2003). Wild mice: an ever-increasing contribution to a popular mammalian model. Trends Genet. 19, 24–31. doi: 10.1016/S0168-9525(02)00007-0
Hau, J. (2008). “Animal models for human diseases: an overview,” in Sourcebook of Models for Biomedical Research, ed P. M. Conn (Totowa, NJ: Humana Press), 3–8.
Hebb, A. L., Zacharko, R. M., Gauthier, M., Trudel, F., Laforest, S., and Drolet, G. (2004). Brief exposure to predator odor and resultant anxiety enhances mesocorticolimbic activity and enkephalin expression in CD-1 mice. Eur. J. Neurosci. 20, 2415–2429. doi: 10.1111/j.1460-9568.2004.03704.x
Hedrich, H. J., and Nicklas, W. (2012). “Housing and Maintenance,” in The Laboratory Mouse, 2nd Edn., ed H. J. Hedrich (Boston, MA: Academic Press), 521–545.
Higgs, S., and Nowell, F. (2000). Population biology of Eimeria (Protozoa: Apicomplexa) in Apodemus sylvaticus: a capture/recapture study. Parasitology 120(Pt 4), 355–363. doi: 10.1017/S0031182099005545
Hilbert, T., Steinhagen, F., Senzig, S., Cramer, N., Bekeredjian-Ding, I., Parcina, M., et al. (2017). Vendor effects on murine gut microbiota influence experimental abdominal sepsis. J. Surg. Res. 211, 126–136. doi: 10.1016/j.jss.2016.12.008
Hogg, S., and File, S. E. (1994). Responders and nonresponders to cat odor do not differ in other tests of anxiety. Pharmacol. Biochem. Behav. 49, 219–222.
Hoy, Y. E., Bik, E. M., Lawley, T. D., Holmes, S. P., Monack, D. M., Theriot, J. A., et al. (2015). Variation in taxonomic composition of the fecal microbiota in an inbred mouse strain across individuals and time. PLoS ONE 10:e0142825. doi: 10.1371/journal.pone.0142825
Hsiao, E. Y., McBride, S. W., Hsien, S., Sharon, G., Hyde, E. R., McCue, T., et al. (2013). Microbiota modulate behavioral and physiological abnormalities associated with neurodevelopmental disorders. Cell 155, 1451–1463. doi: 10.1016/j.cell.2013.11.024
Hufeldt, M. R., Nielsen, D. S., Vogensen, F. K., Midtvedt, T., and Hansen, A. K. (2010). Variation in the gut microbiota of laboratory mice is related to both genetic and environmental factors. Comp. Med. 60, 336–342.
Hunig, T. (2016). The rise and fall of the CD28 superagonist TGN1412 and its return as TAB08: a personal account. FEBS J. 283, 3325–3334. doi: 10.1111/febs.13754
Hunter, P. (2008). The paradox of model organisms - The use of model organisms in research will continue despite their shortcomings. EMBO Rep. 9, 717–720. doi: 10.1038/embor.2008.142
Hüttenhain, R., Malmström, J., Picotti, P., and Aebersold, R. (2009). Perspectives of targeted mass spectrometry for protein biomarker verification. Curr. Opin. Chem. Biol. 13, 518–525. doi: 10.1016/j.cbpa.2009.09.014
Hu, X., Chang, N., Wang, X., Zhou, F., Zhou, X., Zhu, X., et al. (2013). Heritable gene-targeting with gRNA/Cas9 in rats. Cell Res. 23, 1322–1325. doi: 10.1038/cr.2013.141
Jackson, J. A. (2015). Immunology in wild nonmodel rodents: an ecological context for studies of health and disease. Parasite Immunol. 37, 220–232. doi: 10.1111/pim.12180
Jackson, J. A., Friberg, I. M., Bolch, L., Lowe, A., Ralli, C., Harris, P. D., et al. (2009). Immunomodulatory parasites and toll-like receptor-mediated tumour necrosis factor alpha responsiveness in wild mammals. BMC Biol. 7:16. doi: 10.1186/1741-7007-7-16
Jackson, J. A., Hall, A. J., Friberg, I. M., Ralli, C., Lowe, A., Zawadzka, M., et al. (2014). An immunological marker of tolerance to infection in wild rodents. PLoS Biol. 12:e1001901. doi: 10.1371/journal.pbio.1001901
Jackson, M. H., and Siim, J. C. (1986). Toxoplasmosis in a wild rodent population of central Scotland and a possible explanation of the mode of transmission. J. Zool. 209, 549–557. doi: 10.1111/j.1469-7998.1986.tb03610.x
Justice, M. J., and Dhillon, P. (2016). Using the mouse to model human disease: increasing validity and reproducibility. Dis. Model. Mech. 9, 101–103. doi: 10.1242/dmm.024547
Kassebaum, N. J., Arora, M., Barber, R. M., Bhutta, Z. A., Brown, J., Carter, A., et al. (2016). Global, regional, and national disability-adjusted life-years (DALYs) for 315 diseases and injuries and healthy life expectancy (HALE), 1990–2015: a systematic analysis for the Global Burden of Disease Study 2015. Lancet 388, 1603–1658. doi: 10.1016/s0140-6736(16)31460-x
Kaushansky, A., Mikolajczak, S. A., Vignali, M., and Kappe, S. H. I. (2014). Of men in mice: the success and promise of humanized mouse models for human malaria parasite infections. Cell. Microbiol. 16, 602–611. doi: 10.1111/cmi.12277
Kijlstra, A., Meerburg, B., Cornelissen, J., De Craeye, S., Vereijken, P., and Jongert, E. (2008). The role of rodents and shrews in the transmission of Toxoplasma gondii to pigs. Vet. Parasitol. 156, 183–190. doi: 10.1016/j.vetpar.2008.05.030
Klaassen, C. D., Lu, H., and Cui, J. Y. (2011). Epigenetic regulation of drug processing genes. Toxicol. Mech. Methods 21, 312–324. doi: 10.3109/15376516.2011.562758
Klein, S. L. (2004). Hormonal and immunological mechanisms mediating sex differences in parasite infection. Parasite Immunol. 26, 247–264. doi: 10.1111/j.0141-9838.2004.00710.x
Klotz, C., Aebischer, T., and Seeber, F. (2012). Stem cell-derived cell cultures and organoids for protozoan parasite propagation and studying host-parasite interaction. Int. J. Med. Microbiol. 302, 203–209. doi: 10.1016/j.ijmm.2012.07.010
Knowles, S. C., Fenton, A., Petchey, O. L., Jones, T. R., Barber, R., and Pedersen, A. B. (2013). Stability of within-host-parasite communities in a wild mammal system. Proc. Biol. Sci. 280:20130598. doi: 10.1098/rspb.2013.0598
Kolata, G. (1998, May 3). A special report: a cautious awe greets drugs that eradicate tumors in mice. The New York Times.
Koscielny, G., Yaikhom, G., Iyer, V., Meehan, T. F., Morgan, H., Atienza-Herrero, J., et al. (2014). The International Mouse Phenotyping Consortium Web Portal, a unified point of access for knockout mice and related phenotyping data. Nucleic Acids Res. 42, D802–D809. doi: 10.1093/nar/gkt977
Kusdian, G., and Gould, S. B. (2014). The biology of Trichomonas vaginalis in the light of urogenital tract infection. Mol. Biochem. Parasitol. 198, 92–99. doi: 10.1016/j.molbiopara.2015.01.004
Lagkouvardos, I., Pukall, R., Abt, B., Foesel, B. U., Meier-Kolthoff, J. P., Kumar, N., et al. (2016). The Mouse Intestinal Bacterial Collection (miBC) provides host-specific insight into cultured diversity and functional potential of the gut microbiota. Nat. Microbiol. 1:16131. doi: 10.1038/nmicrobiol.2016.131
Lamour, S. D., Veselkov, K. A., Posma, J. M., Giraud, E., Rogers, M. E., Croft, S., et al. (2015). Metabolic, immune, and gut microbial signals mount a systems response to Leishmania major infection. J. Proteome Res. 14, 318–329. doi: 10.1021/pr5008202
Langhorne, J., Buffet, P., Galinski, M., Good, M., Harty, J., Leroy, D., et al. (2011). The relevance of non-human primate and rodent malaria models for humans. Malar. J. 10:23. doi: 10.1186/1475-2875-10-23
Latham, N., and Mason, G. (2004). From house mouse to mouse house: the behavioural biology of free-living Mus musculus and its implications in the laboratory. Appl. Anim. Behav. Sci. 86, 261–289. doi: 10.1016/j.applanim.2004.02.006
Li, D., Qiu, Z., Shao, Y., Chen, Y., Guan, Y., Liu, M., et al. (2013). Heritable gene targeting in the mouse and rat using a CRISPR-Cas system. Nat. Biotechnol. 31, 681–683. doi: 10.1038/nbt.2661
Li, W., Teng, F., Li, T., and Zhou, Q. (2013). Simultaneous generation and germline transmission of multiple gene mutations in rat using CRISPR-Cas systems. Nat. Biotechnol. 31, 684–686. doi: 10.1038/nbt.2652
Lilue, J., Müller, U. B., Steinfeldt, T., and Howard, J. C. (2013). Reciprocal virulence and resistance polymorphism in the relationship between Toxoplasma gondii and the house mouse. Elife 2:e01298. doi: 10.7554/eLife.01298
Lipoldová, M., and Demant, P. (2006). Genetic susceptibility to infectious disease: lessons from mouse models of leishmaniasis. Nat. Rev. Genet. 7, 294–305. doi: 10.1038/nrg1832
Liu, Y., Beyer, A., and Aebersold, R. (2016). On the dependency of cellular protein levels on mRNA abundance. Cell 165, 535–550. doi: 10.1016/j.cell.2016.03.014
Loeuillet, C., Banuls, A. L., and Hide, M. (2016). Study of Leishmania pathogenesis in mice: experimental considerations. Parasit. Vectors 9, 144. doi: 10.1186/s13071-016-1413-9
Lopes, M. E. M., Carneiro, M. B. H., Dos Santos, L. M., and Vieira, L. Q. (2016). Indigenous microbiota and Leishmaniasis. Parasite Immunol. 38, 37–44. doi: 10.1111/pim.12279
Lore, N. I., Iraqi, F. A., and Bragonzi, A. (2015). Host genetic diversity influences the severity of Pseudomonas aeruginosa pneumonia in the Collaborative Cross mice. BMC Genet. 16:106. doi: 10.1186/s12863-015-0260-6
Lutz, C. M., Linder, C. C., and Davisson, M. T. (2012). “Strains, Stocks and Mutant Mice,” in The Laboratory Mouse, 2nd Edn., ed H. J. Hedrich (Boston, MA: Academic Press), 37–56.
Magez, S., and Caljon, G. (2011). Mouse models for pathogenic African trypanosomes: unravelling the immunology of host–parasite–vector interactions. Parasite Immunol. 33, 423–429. doi: 10.1111/j.1365-3024.2011.01293.x
Maizels, R. M., and Nussey, D. H. (2013). Into the wild: digging at immunology's evolutionary roots. Nat. Immunol. 14, 879–883. doi: 10.1038/ni.2643
Mak, T. W. (2007). Gene targeting in embryonic stem cells scores a knockout in Stockholm. Cell 131, 1027–1031. doi: 10.1016/j.cell.2007.11.033
Matthews, K. R. (2015). 25 years of African trypanosome research: from description to molecular dissection and new drug discovery. Mol. Biochem. Parasitol. 200, 30–40. doi: 10.1016/j.molbiopara.2015.01.006
Mayer, E. A., Tillisch, K., and Gupta, A. (2015). Gut/brain axis and the microbiota. J. Clin. Invest. 125, 926–938. doi: 10.1172/JCI76304
Mears, E. R., Modabber, F., Don, R., and Johnson, G. E. (2015). The current in vivo models for the discovery and utility of new anti-leishmanial drugs targeting cutaneous leishmaniasis. PLoS Negl. Trop. Dis. 9:e0003889. doi: 10.1371/journal.pntd.0003889
Mendel, G. (1866). Versuche über Pflanzen-Hybriden. Verhandlungen des Naturforschenden Vereines in Brünn Vol. 4, 3–47. English version available online at: http://www.esp.org/foundations/genetics/classical/gm-65.pdf (May, 2017).
Messenger, L. A., Miles, M. A., and Bern, C. (2015). Between a bug and a hard place: Trypanosoma cruzi genetic diversity and the clinical outcomes of Chagas disease. Expert Rev. Anti Infect. Ther. 13, 995–1029. doi: 10.1586/14787210.2015.1056158
Mestas, J., and Hughes, C. C. W. (2004). Of mice and not men: differences between mouse and human immunology. J. Immunol. 172, 2731–2738. doi: 10.4049/jimmunol.172.5.2731
Morrison, L. J., Vezza, L., Rowan, T., and Hope, J. C. (2016). Animal African trypanosomiasis: time to increase focus on clinically relevant parasite and host species. Trends Parasitol. 32, 599–607. doi: 10.1016/j.pt.2016.04.012
Müller, B., and Grossniklaus, U. (2010). Model organisms — a historical perspective. J. Proteomics 73, 2054–2063. doi: 10.1016/j.jprot.2010.08.002
Muller, I., Hailu, A., Choi, B. S., Abebe, T., Fuentes, J. M., Munder, M., et al. (2008). Age-related alteration of arginase activity impacts on severity of leishmaniasis. PLoS Negl. Trop. Dis. 2:e235. doi: 10.1371/journal.pntd.0000235
Muqaku, B., Slany, A., Bileck, A., Kreutz, D., and Gerner, C. (2015). Quantification of cytokines secreted by primary human cells using multiple reaction monitoring: evaluation of analytical parameters. Anal. Bioanal. Chem. 407, 6525–6536. doi: 10.1007/s00216-015-8817-9
Murray, C. J., Vos, T., Lozano, R., Naghavi, M., Flaxman, A. D., Michaud, C., et al. (2012). Disability-adjusted life years (DALYs) for 291 diseases and injuries in 21 regions, 1990-2010: a systematic analysis for the Global Burden of Disease Study 2010. Lancet 380, 2197–2223. doi: 10.1016/s0140-6736(12)61689-4
Naik, S., Bouladoux, N., Wilhelm, C., Molloy, M. J., Salcedo, R., Kastenmuller, W., et al. (2012). Compartmentalized control of skin immunity by resident commensals. Science 337, 1115–1119. doi: 10.1126/science.1225152
Newman, A. E. M., Edmunds, N. B., Ferraro, S., Heffell, Q., Merritt, G. M., Pakkala, J. J., et al. (2015). Using ecology to inform physiology studies: implications of high population density in the laboratory. Am. J. Physiol. Regul. Integr. Comp. Physiol. 308, R449–R454. doi: 10.1152/ajpregu.00328.2014
Nicod, J., Davies, R. W., Cai, N., Hassett, C., Goodstadt, L., Cosgrove, C., et al. (2016). Genome-wide association of multiple complex traits in outbred mice by ultra-low-coverage sequencing. Nat. Genet. 48, 912–918. doi: 10.1038/ng.3595
Noyes, H. A., Ambrose, P., Barker, F., Begon, M., Bennet, M., Bown, K. J., et al. (2002). Host specificity of Trypanosoma (Herpetosoma) species: evidence that bank voles (Clethrionomys glareolus) carry only one T. (H.) evotomys 18S rRNA genotype but wood mice (Apodemus sylvaticus) carry at least two polyphyletic parasites. Parasitology 124(Pt 2), 185–190. doi: 10.1017/S0031182001001019
O'Connor, B. D., Day, A., Cain, S., Arnaiz, O., Sperling, L., and Stein, L. D. (2008). GMODWeb: a web framework for the Generic Model Organism Database. Genome Biol. 9:R102. doi: 10.1186/gb-2008-9-6-r102
Orrock, J. L. (2004). Rodent foraging is affected by indirect, but not by direct, cues of predation risk. Behav. Ecol. 15, 433–437. doi: 10.1093/beheco/arh031
Pedersen, A. B., and Antonovics, J. (2013). Anthelmintic treatment alters the parasite community in a wild mouse host. Biol. Lett. 9:20130205. doi: 10.1098/rsbl.2013.0205
Pedersen, A. B., and Babayan, S. A. (2011). Wild immunology. Mol. Ecol. 20, 872–880. doi: 10.1111/j.1365-294X.2010.04938.x
Pedersen, A. B., Jones, K. E., Nunn, C. L., and Altizer, S. (2007). Infectious diseases and extinction risk in wild mammals. Conserv. Biol. 21, 1269–1279. doi: 10.1111/j.1523-1739.2007.00776.x
Perry, B., and Grace, D. (2009). The impacts of livestock diseases and their control on growth and development processes that are pro-poor. Philos. Trans. R. Soc. Lond. B. Biol. Sci. 364, 2643–2655. doi: 10.1098/rstb.2009.0097
Phifer-Rixey, M., and Nachman, M. W. (2015). Insights into mammalian biology from the wild house mouse Mus musculus. Elife 4:e05959. doi: 10.7554/eLife.05959
Potter, M., Nadieau, J. H., and Cancro, M. P. (1986). The Wild Mouse in Immunology. Berlin Heidelberg: Springer
Powell, F., and Banks, P. B. (2004). Do house mice modify their foraging behaviour in response to predator odours and habitat? Anim. Behav. 67, 753–759. doi: 10.1016/j.anbehav.2003.08.016
Rand, M. S. (2008). “Selection of biomedical animal models,” in Sourcebook of Models for Biomedical Research, ed P. M. Conn (Totowa, NJ: Humana Press), 9–16.
Rasmussen, A. L., Okumura, A., Ferris, M. T., Green, R., Feldmann, F., Kelly, S. M., et al. (2014). Host genetic diversity enables Ebola hemorrhagic fever pathogenesis and resistance. Science 346, 987–991. doi: 10.1126/science.1259595
Rauniyar, N. (2015). Parallel reaction monitoring: a targeted experiment performed using high resolution and high mass accuracy mass spectrometry. Int. J. Mol. Sci. 16, 28566–28581. doi: 10.3390/ijms161226120
Rausch, P., Basic, M., Batra, A., Bischoff, S. C., Blaut, M., Clavel, T., et al. (2016). Analysis of factors contributing to variation in the C57BL/6J fecal microbiota across German animal facilities. Int. J. Med. Microbiol. 306, 343–355. doi: 10.1016/j.ijmm.2016.03.004
Reese, T. A., Bi, K., Kambal, A., Filali-Mouhim, A., Beura, L. K., Bürger, M. C., et al. (2016). Sequential infection with common pathogens promotes human-like immune gene expression and altered vaccine response. Cell Host Microbe 19, 713–719. doi: 10.1016/j.chom.2016.04.003
Reijnders, D., Goossens, G. H., Hermes, G. D. A., Neis, E. P., van der Beek, C. M., Most, J., et al. (2016). Effects of gut microbiota manipulation by antibiotics on host metabolism in obese humans: a randomized double-blind placebo-controlled trial. Cell Metab. 24, 63–74. doi: 10.1016/j.cmet.2016.06.016
Rhee, J. K., So, W. S., and Kim, H. C. (1999). Age-dependent resistance to Cryptosporidium muris (strain MCR) infection in golden hamsters and mice. Korean J. Parasitol. 37, 33–37.
Roberts, C. W., Walker, W., and Alexander, J. (2001). Sex-associated hormones and immunity to protozoan parasites. Clin. Microbiol. Rev. 14, 476–488. doi: 10.1128/cmr.14.3.476-488.2001
Robertson, L. J., Sprong, H., Ortega, Y. R., van der Giessen, J. W., and Fayer, R. (2014). Impacts of globalisation on foodborne parasites. Trends Parasitol. 30, 37–52. doi: 10.1016/j.pt.2013.09.005
Rochford, R., Ohrt, C., Baresel, P. C., Campo, B., Sampath, A., Magill, A. J., et al. (2013). Humanized mouse model of glucose 6-phosphate dehydrogenase deficiency for in vivo assessment of hemolytic toxicity. Proc. Natl. Acad. Sci. U.S.A. 110, 17486–17491. doi: 10.1073/pnas.1310402110
Rogers, G. B., Kozlowska, J., Keeble, J., Metcalfe, K., Fao, M., Dowd, S. E., et al. (2014). Functional divergence in gastrointestinal microbiota in physically-separated genetically identical mice. Sci. Rep. 4:5437. doi: 10.1038/srep05437
Schluter, D., Daubener, W., Schares, G., Gross, U., Pleyer, U., and Luder, C. (2014). Animals are key to human toxoplasmosis. Int. J. Med. Microbiol. 304, 917–929. doi: 10.1016/j.ijmm.2014.09.002
Sellers, R. S. (2017). Translating mouse models. Toxicol. Pathol. 45, 134–145. doi: 10.1177/0192623316675767
Seok, J., Warren, H. S., Cuenca, A. G., Mindrinos, M. N., Baker, H. V., Xu, W., et al. (2013). Genomic responses in mouse models poorly mimic human inflammatory diseases. Proc. Natl. Acad. Sci. U.S.A. 110, 3507–3512. doi: 10.1073/pnas.1222878110
Shah, S. S., Rockett, K. A., Jallow, M., Sisay-Joof, F., Bojang, K. A., Pinder, M., et al. (2016). Heterogeneous alleles comprising G6PD deficiency trait in West Africa exert contrasting effects on two major clinical presentations of severe malaria. Malar. J. 15, 64. doi: 10.1186/s12936-015-1045-0
Shay, T., Jojic, V., Zuk, O., Rothamel, K., Puyraimond-Zemmour, D., Feng, T., et al. (2013). Conservation and divergence in the transcriptional programs of the human and mouse immune systems. Proc. Natl. Acad. Sci. U.S.A. 110, 2946–2951. doi: 10.1073/pnas.1222738110
Singer, S. M., and Nash, T. E. (2000). The role of normal flora in Giardia lamblia infections in mice. J. Infect. Dis. 181, 1510–1512. doi: 10.1086/315409
Singleton, G. R., and Krebs, C. J. (2007). “The secret world of wild mice,” in The Mouse in Biomedical Research, 2nd Edn., eds M. T. Davisson, F. W. Quimby, S. W. Barthold, C. E. Newcomer, and A. L. Smith (Burlington, NJ: Academic Press), 25–51.
Skea, D. L., and Underdown, B. J. (1991). Acquired resistance to Giardia muris in X-linked immunodeficient mice. Infect. Immun. 59, 1733–1738
Smith, A., Telfer, S., Burthe, S., Bennett, M., and Begon, M. (2005). Trypanosomes, fleas and field voles: ecological dynamics of a host-vector-parasite interaction. Parasitology 131(Pt 3), 355–365. doi: 10.1017/S0031182005007766
Smith, C. M., Proulx, M. K., Olive, A. J., Laddy, D., Mishra, B. B., Moss, C., et al. (2016). Tuberculosis susceptibility and vaccine protection are independently controlled by host genotype. MBio 7:e01516. doi: 10.1128/mBio.01516-16
Steinman, R. M. (2012). Decisions about dendritic cells: past, present, and future. Annu. Rev. Immunol. 30, 1–22. doi: 10.1146/annurev-immunol-100311-102839
Stough, J. M. A., Dearth, S. P., Denny, J. E., LeCleir, G. R., Schmidt, N. W., Campagna, S. R., et al. (2016). Functional characteristics of the gut microbiome in C57BL/6 mice differentially susceptible to plasmodium yoelii. Front. Microbiol. 7:1520. doi: 10.3389/fmicb.2016.01520
Stuart, K., Brun, R., Croft, S., Fairlamb, A., Gürtler, R. E., McKerrow, J., et al. (2008). Kinetoplastids: related protozoan pathogens, different diseases. J. Clin. Invest. 118, 1301–1310. doi: 10.1172/JCI33945
Svobodova, M., Vorisek, P., Votypka, J., and Weidinger, K. (2004). Heteroxenous Coccidia (Apicomplexa: Sarcocystidae) in the populations of their final and intermediate hosts: European buzzard and small mammals. Acta Protozool. 43, 251–260.
Takao, K., and Miyakawa, T. (2015). Genomic responses in mouse models greatly mimic human inflammatory diseases. Proc. Natl. Acad. Sci. U.S.A. 112, 1167–1172. doi: 10.1073/pnas.1401965111
Tao, L., and Reese, T. A. (2017). Making mouse models that reflect human immune responses. Trends Immunol. 38, 181–193. doi: 10.1016/j.it.2016.12.007
The Commission to the Council and the European Parliament (2013). Seventh Report on the Statistics on the Number of Animals Used for Experimental and Other Scientific Purposes in the Member States of the European Union. Available online at: http://ec.europa.eu/environment/chemicals/lab_animals/reports_en.htm
Threadgill, D. W., and Churchill, G. A. (2012). Ten years of the Collaborative Cross. Genetics 190, 291–294. doi: 10.1534/genetics.111.138032
Tong, C., Li, P., Wu, N. L., Yan, Y., and Ying, Q. L. (2010). Production of p53 gene knockout rats by homologous recombination in embryonic stem cells. Nature 467, 211–213. doi: 10.1038/nature09368
Torgerson, P. R. (2013). One world health: socioeconomic burden and parasitic disease control priorities. Vet. Parasitol. 195, 223–232. doi: 10.1016/j.vetpar.2013.04.004
Torgerson, P. R., and Macpherson, C. N. (2011). The socioeconomic burden of parasitic zoonoses: global trends. Vet. Parasitol. 182, 79–95. doi: 10.1016/j.vetpar.2011.07.017
Torres, M. R., Silva, M. E., Vieira, E. C., Bambirra, E. A., Sogayar, M. I., Pena, F. J., et al. (1992). Intragastric infection of conventional and germfree mice with Giardia lamblia. Braz. J. Med. Biol. Res. 25, 349–352.
Turnbaugh, P. J., Ley, R. E., Mahowald, M. A., Magrini, V., Mardis, E. R., and Gordon, J. I. (2006). An obesity-associated gut microbiome with increased capacity for energy harvest. Nature 444, 1027–1131. doi: 10.1038/nature05414
Turner, A. K., Begon, M., Jackson, J. A., Bradley, J. E., and Paterson, S. (2011). Genetic diversity in cytokines associated with immune variation and resistance to multiple pathogens in a natural rodent population. PLoS Genet. 7:e1002343. doi: 10.1371/journal.pgen.1002343
Turner, A. K., Beldomenico, P. M., Bown, K., Burthe, S. J., Jackson, J. A., Lambin, X., et al. (2014). Host-parasite biology in the real world: the field voles of Kielder. Parasitology 141, 997–1017. doi: 10.1017/s0031182014000171
Turner, C. M. (1986). Seasonal and age distributions of Babesia, Hepatozoon, Trypanosoma and Grahamella species in Clethrionomys glareolus and Apodemus sylvaticus populations. Parasitology 93(Pt 2), 279–289.
UK Home Office (2015). Annual Statistics of Scientific Procedures of Living Animals Great Britain 2015. Available online at: https://www.gov.uk/government/uploads/system/uploads/attachment_data/file/537708/scientific-procedures-living-animals-2015.pdf
Valdar, W., Solberg, L. C., Gauguier, D., Burnett, S., Klenerman, P., Cookson, W. O., et al. (2006). Genome-wide genetic association of complex traits in heterogeneous stock mice. Nat. Genet. 38, 879–887. doi: 10.1038/ng1840
van Boxtel, R., and Cuppen, E. (2010). Rat traps: filling the toolbox for manipulating the rat genome. Genome Biol. 11:217. doi: 10.1186/gb-2010-11-9-217
Van der Hilst, J. C., Smits, B. W., and van der Meer, J. W. (2002). Hypogammaglobulinaemia: cumulative experience in 49 patients in a tertiary care institution. Neth. J. Med. 60, 140–147.
van der Worp, H. B., Howells, D. W., Sena, E. S., Porritt, M. J., Rewell, S., O'Collins, V., et al. (2010). Can animal models of disease reliably inform human studies? PLoS Med. 7:e1000245. doi: 10.1371/journal.pmed.1000245
van Lunzen, J., and Altfeld, M. (2014). Sex differences in infectious diseases-common but neglected. J. Infect. Dis. 209(Suppl. 3), S79–S80. doi: 10.1093/infdis/jiu159
Vannier, E., Borggraefe, I., Telford, S. R. III, Menon, S., Brauns, T., Spielman, A., et al. (2004). Age-associated decline in resistance to Babesia microti is genetically determined. J. Infect. Dis. 189, 1721–1728. doi: 10.1086/382965
Vaughan, A. M., Mikolajczak, S. A., Wilson, E. M., Grompe, M., Kaushansky, A., Camargo, N., et al. (2012). Complete Plasmodium falciparum liver-stage development in liver-chimeric mice. J. Clin. Invest. 122, 3618–3628. doi: 10.1172/jci62684
Vaughan, A. M., Pinapati, R. S., Cheeseman, I. H., Camargo, N., Fishbaugher, M., Checkley, L. A., et al. (2015). Plasmodium falciparum genetic crosses in a humanized mouse model. Nat. Methods 12, 631–633. doi: 10.1038/nmeth.3432
Verdolin, J. L. (2006). Meta-analysis of foraging and predation risk trade-offs in terrestrial systems. Behav. Ecol. Sociobiol. 60, 457–464. doi: 10.1007/s00265-006-0172-6
Vered, K., Durrant, C., Mott, R., and Iraqi, F. A. (2014). Susceptibility to Klebsiella pneumonaie infection in collaborative cross mice is a complex trait controlled by at least three loci acting at different time points. BMC Genomics 15:865. doi: 10.1186/1471-2164-15-865
Vidal, S. M., Malo, D., Marquis, J. F., and Gros, P. (2008). Forward genetic dissection of immunity to infection in the mouse. Annu. Rev. Immunol. 26, 81–132. doi: 10.1146/annurev.immunol.26.021607.090304
Vijay, N., Poelstra, J. W., Kunstner, A., and Wolf, J. B. (2013). Challenges and strategies in transcriptome assembly and differential gene expression quantification. A comprehensive in silico assessment of RNA-seq experiments. Mol. Ecol. 22, 620–634. doi: 10.1111/mec.12014
Villarino, N. F., LeCleir, G. R., Denny, J. E., Dearth, S. P., Harding, C. L., Sloan, S. S., et al. (2016). Composition of the gut microbiota modulates the severity of malaria. Proc. Natl. Acad. Sci. U.S.A. 113, 2235–2240. doi: 10.1073/pnas.1504887113
Viney, M., Lazarou, L., and Abolins, S. (2015). The laboratory mouse and wild immunology. Parasite Immunol. 37, 267–273. doi: 10.1111/pim.12150
Warren, H., Tompkins, R., Moldawer, L., Seok, J., Xu, W., Mindrinos, M., et al. (2015). Mice are not men. Proc. Natl. Acad. Sci. U.S.A. 112, E345. doi: 10.1073/pnas.1414857111
Waterston, R. H., Lindblad-Toh, K., Birney, E., Rogers, J., Abril, J. F., Agarwal, P., et al. (2002). Initial sequencing and comparative analysis of the mouse genome. Nature 420, 520–562. doi: 10.1038/nature01262
Wege, A. K., Florian, C., Ernst, W., Zimara, N., Schleicher, U., Hanses, F., et al. (2012). Leishmania major infection in humanized mice induces systemic infection and provokes a nonprotective human immune response. PLoS Negl. Trop. Dis. 6:e1741. doi: 10.1371/journal.pntd.0001741
Weidner, C., Steinfath, M., Opitz, E., Oelgeschläger, M., and Schönfelder, G. (2016). Defining the optimal animal model for translational research using gene set enrichment analysis. EMBO Mol. Med. 8, 831–838. doi: 10.15252/emmm.201506025
Weissmuller, S., Kronhart, S., Kreuz, D., Schnierle, B., Kalinke, U., Kirberg, J., et al. (2016). TGN1412 induces lymphopenia and human cytokine release in a humanized mouse model. PLoS ONE 11:e0149093. doi: 10.1371/journal.pone.0149093
Weldon, L., Abolins, S., Lenzi, L., Bourne, C., Riley, E. M., and Viney, M. (2015). The gut microbiota of wild mice. PLoS ONE 10:e0134643. doi: 10.1371/journal.pone.0134643
Wickham, K. S., Baresel, P. C., Marcsisin, S. R., Sousa, J., Vuong, C. T., Reichard, G. A., et al. (2016). Single-dose primaquine in a preclinical model of glucose-6-phosphate dehydrogenase deficiency: implications for use in malaria transmission-blocking programs. Antimicrob. Agents Chemother. 60, 5906–5913. doi: 10.1128/aac.00600-16
Willyard, C. (2015). The boom in mini stomachs, brains, breasts, kidneys and more. Nature 523, 520–522. doi: 10.1038/523520a
Withenshaw, S. M., Devevey, G., Pedersen, A. B., and Fenton, A. (2016). Multihost Bartonella parasites display covert host specificity even when transmitted by generalist vectors. J. Anim. Ecol. 85, 1442–1452. doi: 10.1111/1365-2656.12568
Wolff, J. O. (2003). Laboratory studies with rodents: facts or artifacts? Bioscience 53, 421–427. doi: 10.1641/0006-3568(2003)053[0421:LSWRFO]2.0.CO;2
Worth, A. R., Andrew Thompson, R. C., and Lymbery, A. J. (2014). Reevaluating the evidence for Toxoplasma gondii-induced behavioural changes in rodents. Adv. Parasitol. 85, 109–142. doi: 10.1016/B978-0-12-800182-0.00003-9
Worth, A. R., Lymbery, A. J., and Thompson, R. C. A. (2013). Adaptive host manipulation by Toxoplasma gondii: fact or fiction? Trends Parasitol. 29, 150–155. doi: 10.1016/j.pt.2013.01.004
Yilmaz, B., Portugal, S., Tran, T. M., Gozzelino, R., Ramos, S., Gomes, J., et al. (2014). Gut microbiota elicits a protective immune response against malaria transmission. Cell 159, 1277–1289. doi: 10.1016/j.cell.2014.10.053
Yoshiki, A., and Moriwaki, K. (2006). Mouse phenome research: implications of genetic background. ILAR J. 47, 94–102. doi: 10.1093/ilar.47.2.94
Zamboni, D. S., and Lima-Junior, D. S. (2015). Inflammasomes in host response to protozoan parasites. Immunol. Rev. 265, 156–171. doi: 10.1111/imr.12291
Zimmerman, L. M., Bowden, R. M., and Vogel, L. A. (2014). A vertebrate cytokine primer for eco-immunologists. Funct. Ecol. 28, 1061–1073. doi: 10.1111/1365-2435.12273
Keywords: wild rodent, protozoa, parasite, model organism, mouse, rat, translational research
Citation: Ehret T, Torelli F, Klotz C, Pedersen AB and Seeber F (2017) Translational Rodent Models for Research on Parasitic Protozoa—A Review of Confounders and Possibilities. Front. Cell. Infect. Microbiol. 7:238. doi: 10.3389/fcimb.2017.00238
Received: 23 February 2017; Accepted: 22 May 2017;
Published: 07 June 2017.
Edited by:
Kenneth Fields, University of Kentucky, United StatesReviewed by:
Aaron Conrad Ericsson, University of Missouri, United StatesLuciana Oliveira Andrade, Universidade Federal de Minas Gerais, Brazil
Copyright © 2017 Ehret, Torelli, Klotz, Pedersen and Seeber. This is an open-access article distributed under the terms of the Creative Commons Attribution License (CC BY). The use, distribution or reproduction in other forums is permitted, provided the original author(s) or licensor are credited and that the original publication in this journal is cited, in accordance with accepted academic practice. No use, distribution or reproduction is permitted which does not comply with these terms.
*Correspondence: Frank Seeber, c2VlYmVyZkBya2kuZGU=