- 1Department of Food Science, Cornell University, Ithaca, NY, USA
- 2Department of Biological Statistics and Computational Biology, Cornell University, Ithaca, NY, USA
- 3Department of Biological Statistics and Computational Biology, Weill Institute for Cell and Molecular Biology, Cornell University, Ithaca, NY, USA
Our understanding of how pathogens shape their gene expression profiles in response to environmental changes is ever growing. Advances in Bioinformatics have made it possible to model complex systems and integrate data from variable sources into one large regulatory network. In these analyses, regulatory networks are typically broken down into regulatory motifs such as feed-forward loops (FFL) or auto-regulatory feedbacks, which serves to simplify the structure, while the functional implications of different regulatory motifs allow to make informed assumptions about the function of a specific regulatory pathway. Here we review the basic concepts of network features and use this language to break down the regulatory networks that govern the interactions between the main regulators of stress response, virulence, and transmission in Listeria monocytogenes. We point out the advantage that taking a “systems approach” could have for our understanding of gene functions, the detection of distant regulatory inputs, interspecies comparisons, and co-expression.
Introduction
Genetic studies used to be the main approach of studying regulatory mechanisms. These studies usually analyze small, closed regulatory systems, involving no more than four or five regulators and often only analyze a single regulatory mechanism involving a regulator and its regulatee. Advances in Bioinformatics have made it possible to model complex systems, including increasingly large regulatory networks in bacteria (Dufour and Donohue, 2012; van Helden et al., 2012), higher organisms (Middleton et al., 2012; Klinger et al., 2013), and chemical systems (Kamerlin et al., 2011, recently recognized with a Nobel Prize in Chemistry). The integrated analysis of all known regulatory interactions in an organism via a systems approach (Barabasi and Oltvai, 2004; Snoep et al., 2006) into one large network is possible, with data as diverse as RNA-seq, ChIP-seq, and microarray data as inputs (for a practical example see Bonneau et al., 2007). In these analyses, regulatory networks are typically broken down into regulatory motifs such as feed-forward loops (FFL) or autoregulatory feedbacks. Breaking down a network into these “building blocks” serves to simplify the structure, while the functional implications of different regulatory motifs allow the generation of informed assumptions about the function of a specific regulatory pathway. There are a number of examples on how network studies have been harnessed to discover new functions for known transcription factors (Bonneau et al., 2007), protein-protein interactions (Wichadakul et al., 2009), assign functions to genes with unknown functions (Bonneau et al., 2007), compare the same network across species and study their evolution and diversification (Wuchty et al., 2003), and compare different networks in the same organism (Xia et al., 2004; Yu and Gerstein, 2006).
The aim of this mini review is to give an overview of basic network motifs and their function, to use this framework to identify and explore different regulatory network motifs, and explore key regulatory networks in Listeria monocytogenes (L. monocytogenes). This approach will illustrate the benefits of taking a systems approach to a comprehensive analysis of large networks in L. monocytogenes and other bacterial pathogens.
In order to survive, bacteria must adapt to their environment, and to do so they express an array of regulatory factors responsible for mounting a specific and rapid response to changes in their surroundings. The foodborne pathogen L. monocytogenes has the ability to adapt to diverse conditions encountered in the extra-host environment (e.g., soil, food), the gastro-intestinal tract, and the extra- and intracellular environment encountered in different hosts. In order to overcome these changing conditions, L. monocytogenes expresses an arsenal of effector proteins encoded by genes that are tightly regulated by alternative σ (sigma) factors, transcriptional activators, transcriptional repressors and at the translational and post-translational levels. While transcriptional regulators are known to activate/repress a set of genes in response to a stimulus, in many cases the response is not as straightforward and adaptation to a particular stress often involves a network of regulators that can interact directly, or indirectly, through an activation cascade and/or coregulation.
In L. monocytogenes, several regulators involved in the control of gene expression have been identified and characterized in detail. The positive regulatory factor A (PrfA) regulates the expression of the vast majority of virulence genes (Scortti et al., 2007), therefore its own expression is tightly regulated at the transcriptional, translational and post-translational levels (reviewed in de las Heras et al., 2011) The concentration of PrfA and its affinity for the promoter will ultimately determine the strength of the PrfA response. This balance is achieved through the combination of different mechanisms such as basal transcriptional control (Chaturongakul et al., 2008), autoregulatory transcription loops (Scortti et al., 2007), and a translational thermoswitch that represses activation outside the host (Johansson et al., 2002) amongst others (reviewed in Freitag et al., 2009).
Another major input of regulation at the transcriptional level is achieved through the action of sigma factors, the promoter recognition subunits of RNA polymerase holoenzyme. In L. monocytogenes, σBregulates the expression of general stress response genes and therefore plays a crucial role in the survival of this bacterium in challenging environments (reviewed in O'Byrne and Karatzas, 2008). Besides σB, the L. monocytogenes genome encodes for two (in lineages I, II and IV isolates) to three (in lineage II isolates) additional alternative σ factors. σL regulates approximately 20 genes (Arous et al., 2004) and has been shown to be involved in low temperature resistance, salt and lactic acid stress (Chan et al., 2008; Raimann et al., 2009; Tessema et al., 2012). σH regulates approximately 50 genes (Chaturongakul et al., 2011) and appears to be involved in alkaline stress (Rea et al., 2004) and σC, an extra cytoplasmic σ factor specific to lineage II strains, has been shown to be activated by heat stress (Zhang et al., 2005). Other regulators involved in L. monocytogenes stress response include CtsR and HrcA, two negative regulators involved in heat shock stress (Nair et al., 2000). CodY is a nutrient responsive regulator with a possible role in mediating response to temperature stress (Bennett et al., 2007) and AgrA is a temperature dependent, autoregulatory protein involved in virulence (Autret et al., 2003; Garmyn et al., 2012). Additionally, over 15 two-component systems have been reported in L. monocytogenes, several of them involved in response to different stresses (Glaser et al., 2001; Williams et al., 2005; Chan et al., 2008).
Increasing evidence supports that there are many ways in which regulators interact to fine-tune L. monocytogenes gene expression in response to different environmental conditions. For example, overlaps in the regulons of PrfA, CtsR, HrcA, and σB, σC, σH, and σL have been shown (Chaturongakul et al., 2011). Similarly, the AgrA regulon has been shown to overlap with the PrfA, σB, σH, and CodY regulons (Garmyn et al., 2012). A number of more specific interactions between regulators have also been defined. For example, both CodY and σB have been shown to be involved in regulation of PrfA expression (Ollinger et al., 2009; Lobel et al., 2012). Additionally, increasing data is available on the role of non-coding RNA that interfere with gene regulation at the transcriptional, translational and post-translational level (reviewed in Mellin and Cossart, 2012). Overall, existing data support complex regulatory networks that allow L. monocytogenes to fine-tune its response of to the rapidly changing conditions and to integrate diverse stimuli to regulate specific phenotypic responses. Further studies of these networks are needed to understand their function under different conditions with a higher level of detail and resolution.
Network Features—an Overview of Concepts
Mathematical modeling allows for the identification of commonly used regulatory elements, or network motifs, which can be used as building blocks to understand larger network structures (reviewed in Alon, 2007; Tyson and Novak, 2010). These elements can be broken down into six motifs (Figure 1) which will be described below. These motifs have been described for bacteria (Shen-Orr et al., 2002) and yeast (Lee et al., 2002), some of them are more common and overrepresented whereas others are rare. In general, network motifs offer the possibility to study complicated regulatory systems on a higher level of abstraction.
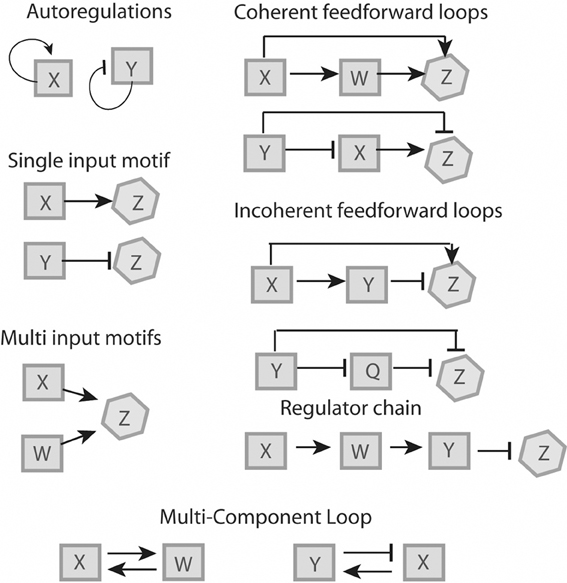
Figure 1. Regulatory features. Proteins “X” and “W” are positive regulators, proteins “Y” and “Q” are negative regulators and protein “Z” is a non-regulator. All six motifs represented can be recognized in L. monocytogenes (see Figure 2).
Single Input Motif
Under a specific condition, a positive (X) or negative (Y) transcription factor binds to a specific set of genes (operon Z), which are solely regulated by either X or Y. Functionally, single input motifs facilitate a fast and straightforward response, for example in reaction to a specific condition (Shen-Orr et al., 2002).
Multi-Input Motif
A set of transcriptional factors X and W are able to recognize and bind to the same promoter region of a set of genes (operon Z). A multi-input motif allows the coordination of gene expression in response to different signals (Shen-Orr et al., 2002).
Feed-Forward Loop
A transcriptional factor X regulates transcriptional factor W and both of them directly regulate a set of genes, operon Z. In a coherent FFL, both regulators have the same effect on Z (e.g., X activates W and both X and W activate Z). The coherent FFL serves as a signal-sensitive delay element that can be dose- or time-dependent (Mangan et al., 2003). In an incoherent FFL, both regulators have antagonistic roles on Z (e.g., X activates Y and Z, but Y represses Z). Incoherent FFL have been studied in detail, and they are thought to provide a biphasic behavior where phase one involves a rapid activation with a concomitant phase two of delayed inhibition (Mangan and Alon, 2003; Kim et al., 2008). These loops often serve to minimize noise, i.e., fluctuations in gene expression, therefore fine-tuning their regulatory response (Thattai and van Oudenaarden, 2001). In pathogens, these loops may allow for activation of specific genes that are required only in specific compartments, followed by rapid downregulation to prevent expression in a subsequent compartment where expression of a given protein may be detrimental.
Autoregulation
A transcriptional factor recognizes the promoter of its own gene. There are conceivable advantages of auto-regulation, such as fast reaction to stimuli and low biosynthetic cost of regulation (McAdams and Arkin, 1997; Thieffry et al., 1998; Becskei and Serrano, 2000; Guelzim et al., 2002; Lee et al., 2002; Shen-Orr et al., 2002).
Multi-Component Loop
Two or more regulatory factors are involved in a closed circuit. While multi-component loops have been described in yeast, they were initially thought to be absent in bacteria (Lee et al., 2002). However, recent studies have shown a few rare examples of multi-component loops in bacterial genetic networks (Ruiz et al., 2001; Kato et al., 2003).
Regulator Chain
Three or more regulators involved in the sequential activation of each other. Time-dependent events such as the cell cycle and developmental features such as spore formation often involve regulator chains (de Hoon et al., 2010).
Regulatory Network Features in L. monocytogenes
In this section, we apply the above principles to analyze regulatory network features in L. monocytogenes, focusing on the different interactions between σB and PrfA and their contribution to transcription and translation of genes with roles in virulence and stress response.
Single Input Motif
Examples of single input motifs are genes that are solely regulated by σB (e.g., uspL-1, uspL-2, uspL-3 Seifart et al., 2011, lmo2230 Kazmierczak et al., 2003; Utratna et al., 2012, gadD3 Wemekamp-Kamphuis et al., 2004; Oliver et al., 2009). While transcriptional regulation by PrfA of the core virulence genes plcA, hly, mpl, actA, plcB may also be viewed as a single input regulatory motif (Figure 2), transcription of these genes requires both σA as well as PrfA and thus should probably be considered a multi input motif. While some may not consider σA a regulator as it is the constitutively active housekeeping sigma factor, σA levels may still change, which would at least show minor effects on gene regulation.
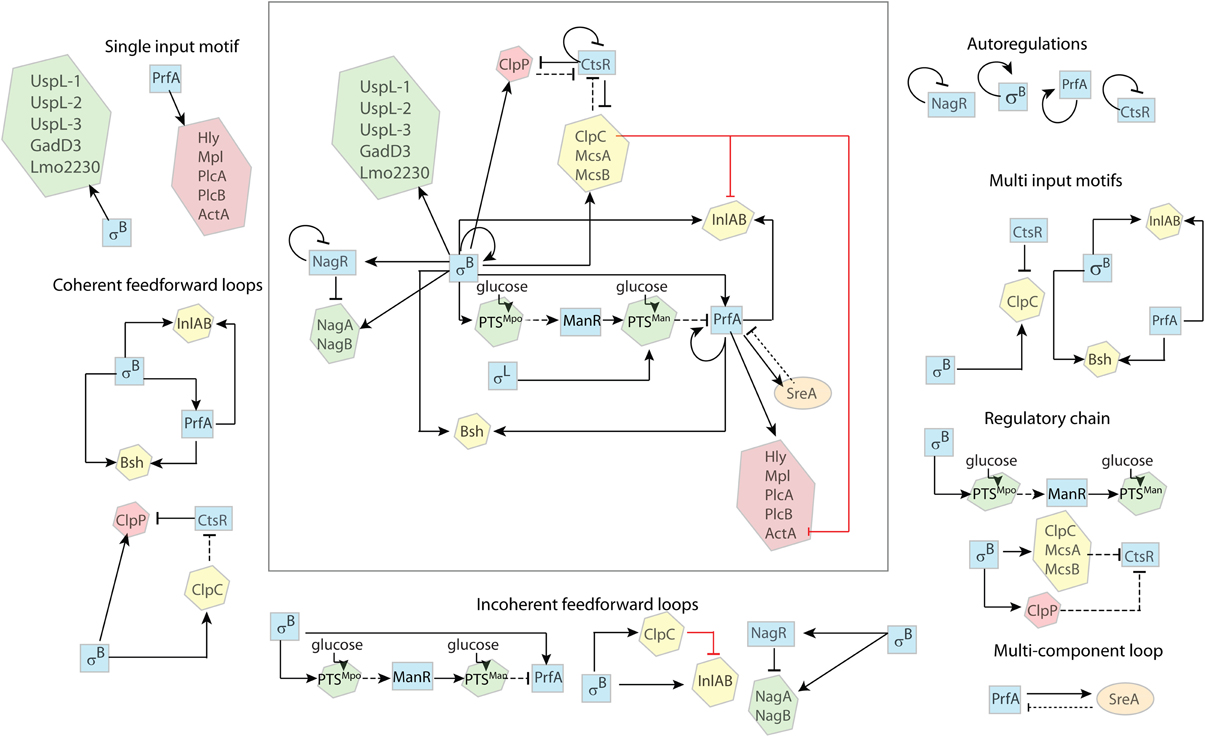
Figure 2. Regulatory features in L. monocytogenes. The regulatory network involves six transcriptional regulators; the alternative σ factors σB and σL, the transcription activators PrfA and ManR, and the transcription repressors CtsR and NagR (blue squares). Proteins not involved in transcription regulation are enclosed in hexagons. Proteins mostly active during environmental growth (green) include NagA, an N-acetyl-glucosamine-6-phosphate deacetylase, NagB, a glucosamine-6-phosphate deaminase, PTSMpo and PTSMan, two glucose PTSs; ClpC, a protease, InlA and InlB, two internalin proteins, and Bsh, a bile salt hydrolase, are involved in the early stages of infection (yellow); ClpP is a serine protease and Hly, Mpl, PlcA, PlcB, and ActA are virulence factors involved in the intracellular stage of infection (red). SreA is a trans-acting noncoding RNA. Solid arrows () indicate activation and crossed lines (
) indicate repression. Solid black lines indicate transcriptional regulation (i.e., regulation at the DNA level); dotted black lines indicate post-transcriptional regulation (i.e., regulation at the RNA level); dashed black lines indicate post-translational regulation (i.e., regulation at the protein level); red solid lines indicates unknown mechanism of regulation (i.e., transcriptional, post-transcriptional, or post-translational). The complex regulatory system is broken down into simpler regulatory features as described in the text.
Multi Input Motif
Overlapping regulons are common in bacteria and there are several examples in L. monocytogenes where multiple regulators regulate the same genes (Chaturongakul et al., 2011). For example, σB and PrfA co-regulate transcription of at least three genes, including the inlAB operon and bsh (Figure 2). Both inlAB and bsh have independent and distinct PrfA and σB dependent promoters (Lingnau et al., 1995; Dussurget et al., 2002; Kazmierczak et al., 2003). CtsR and σB also co-regulate transcription of the mcsA-mscB-clpC operon, which includes a σB dependent promoter (upstream of mcsA) and an independent σA dependent promoter with a CtsR binding site (upstream of ctsR itself) (Hu et al., 2007a).
Positive Autoregulation
PrfA, the main positive regulatory factor of virulence genes, can upregulate its own transcription (Figure 2). PrfA can be transcribed as a monocistronic mRNA from the two promoters directly upstream of the PrfA gene, p1prfA which is σA dependent and p2prfA which is σB and σA dependent (de las Heras et al., 2011). PrfA can also be transcribed as a bicistronic mRNA from the PrfA-dependent promoter upstream of plcA (Mengaud et al., 1991; Scortti et al., 2007), creating a positive autoregulatory feedback loop.
Another example of autoregulation is the transcription of sigB, which occurs from a σB-dependent promoter upstream of the L. monocytogenes rsbVW-sigB-rsbX operon (Kazmierczak et al., 2003) (Figure 2).
Negative Autoregulation
Examples for negative autoregulation in L. monocytogenes include regulation of CtsR and HrcA, both of which are negative regulators of heat shock proteins (Hu et al., 2007b) (Figure 2). Both CtsR (Nair et al., 2000) and HcrA (Hanawa et al., 2000) can bind to their own promoter and repress transcription of the ctsR-mcsA-mcsB-clpC and hcrA-grpA-dnaK operons respectively.
Coherent Feedforward Loops
L. monocytogenes has several coherent FFL that involve both σB and PrfA, including transcription of bsh and inlAB. bsh encodes a bile salt hydrolase, which contributes to the bacterial defense against bile salts (Dussurget et al., 2002; Jones et al., 2008). inlA encodes internalin A (InlA), a bacterial surface molecule that mediates the entry of L. monocytogenes into mammalian epithelial cells (Lingnau et al., 1995). The coherent FFL for both bsh and inlAB involves (i) direct transcriptional activation of these genes by σB and (ii) σB-dependent transcription of PrfA (Schwab et al., 2005), which in turn directly activates both bsh (Dussurget et al., 2002; Kazmierczak et al., 2003; Sue et al., 2003, 2004) and inlAB (McGann et al., 2007) transcription (Figure 2).
An example for a coherent FFL that works via inhibitory mechanisms is the regulation of ClpP, a heat shock protein that is involved in intracellular growth (Gaillot et al., 2000, 2001). This coherent FFL involves (i) upregulation of clpP transcript levels by σB, possibly through a putative σB-dependent promoter upstream of clpP (Wemekamp-Kamphuis et al., 2004) and (ii) σB-dependent transcription of the mcsA-mcsB-clpC operon (Gaillot et al., 2001) with ClpC, McsA, and McsB mediated post-translational inhibition of CtsR (Chaturongakul and Boor, 2006; Hu et al., 2007a), which relieves CtsR mediated transcriptional downregulation of clpP (Chaturongakul et al., 2011), resulting in increased ClpP levels (Figure 2). It is conceivable that this FFL serves to increase the level of ClpP under the acidic conditions encountered during gastrointestinal passage (via indication of the acid responsive σB), therefore priming the bacteria for more efficient subsequent intracellular growth.
Incoherent Feedforward Loops
One example of an incoherent FFL is represented by σB-dependent regulation of inlAB, which includes (i) positive transcriptional regulation of inlAB through σB (Kazmierczak et al., 2003) and (ii) indirect σB-dependent downregulation of inlAB expression, which involves σB activating the expression of ClpC (Hu et al., 2007a; Chaturongakul et al., 2011), which has been shown to downregulate, through an unknown mechanism, the transcription of inlA and inlB (Nair et al., 2000) (Figure 2).
A potentially very important example of an incoherent FFL can be found in the interaction between σB and PrfA. There is a σB-dependent direct upregulation of prfA transcription as well as a σB-dependent indirect post-translational inhibition of PrfA (Nadon et al., 2002; Ollinger et al., 2009). This incoherent FFL may facilitate rapid activation of PrfA, with a subsequent delayed inhibition of PrfA-dependent gene regulation to moderate the negative effects of prolonged activation of PrfA-dependent genes such as hly (Scortti et al., 2007), which may cause host cell lysis when overexpressed. This incoherent FFL includes (i) activation of prfA transcription via the σB-dependent p2prfA promoter (Nadon et al., 2002) and (ii) σB-dependent downregulation via a regulatory chain that involves mpoABCD (encoding PTSMpo), manR (encoding ManR), manLMN (encoding PTSMan), and prfA (encoding PrfA), as described in detail below (Figure 2). Ollinger et al. (2009) initially reported evidence for σB-dependent downregulation of PrfA by an unknown mechanism that did not involve downregulation of prfA transcription. Recently, Ake et al. (2011) showed that the the σA- and σB-induced mpo operon, which encodes the PTS complex PTSMpo, is involved in inactivation of PrfA through a cascade of post-translational and transcriptional regulation, providing a potential mechanism for σB-dependent downregulation of PrfA. This regulation involves PTSMpo itself, ManR, a transcriptional activator of the man operon, the PTSMan complex and PrfA (Raengpradub et al., 2008; Oliver et al., 2009, 2010; Ollinger et al., 2009; Tessema et al., 2009; Mujahid et al., 2013a). In the proposed model, upon uptake of glucose through PTSMpo, two subunits of PTSMpo become dephosphorylated and, then, prevent the inhibitory phosphorylation of ManR. The functional ManR then activates the transcription of the manLMN operon, which encodes for PTSMan. Upon uptake of glucose by PTSMan, the EIIABMan subunit becomes dephosphorylated, which inhibits PrfA by a mechanism not yet elucidated (Dalet et al., 2001; Arous et al., 2004; de las Heras et al., 2011; Mujahid et al., 2013b).
Incoherent FFL are functionally suited for the regulation of metabolic enzymes. Energy conservation warrants the transcription of catabolic enzymes only when the substrate is present. Therefore, the repressor of the respective enzymes is often co-translated in the same operon or at least under the control of the same transcription factor. The presence of the appropriate substrate then inactivates the repressor. An example for this is part of the chitin catabolism of L. monocytogenes. The chitin monomer GlcNAc is an ubiquitous source of carbon and nitrogen. It is used by many microorganisms (Resch et al., 2010) and can be exploited by bacteria in a dual way: it is either degraded into fructose-6-P and funneled into glycolysis for energy production, or it can be used anabolically in peptidoglycan synthesis (Bertram et al., 2011; Popowska et al., 2012). In L. monocytogenes, GlcNAc degradation is regulated by an incoherent FFL with (i) σB positively regulating transcription of genes that facilitate GlcNAC degradation (nagA and nagB) and (ii) σB positively regulating NagR, which negatively regulates transcription of nagA and nagB (Figure 2). Briefly, σB upregulates transcription of the nagABR operon (Raengpradub et al., 2008; Mujahid et al., 2013a). NagA and NagB are GlcNAc metabolic enzymes, and NagR is a transcriptional repressor that inhibits the transcription of nagABR unless the substrate (GlcNAc) for NagA and NagB is present. NagA (N-acetylglucosamine-6-phosphate deacetylase) and NagB (glucosamine-6-phosphate deaminase) facilitate the degradation of GlcNAc into fructose-6-P (Popowska et al., 2012).
Multi Component Loop
An example of a multi component loop in L. monocytogenes is the recently shown regulation involving (i) PrfA positively regulating sreA and (ii) SreA negatively regulating PrfA. SreA is a S-adenosylmethionine (SAM) riboswitch, and sreA transcription has been shown to be PrfA-dependent with a 7-fold increase during intracellular growth (Loh et al., 2009). Moreover, it has been shown that, in addition to controlling the expression of downstream genes, the SreA riboswitch also functions as a small noncoding RNA, acting post-transcriptionally to decrease the expression of PrfA (Loh et al., 2009; Mellin and Cossart, 2012).
Regulator Chain
Two examples of regulator chains in L. monocytogenes are (i) the σB-dependent repression of CtsR and (ii) the σB-dependent activation of PTSMan (see Figure 2). Briefly, the σB-dependent repression of CtsR involves σB-mediated transcriptional upregulation of McsA, McsB, ClpC, and ClpP (Hu et al., 2007a; Chaturongakul et al., 2011) and, as observed in B. subtilis (Kruger et al., 2001), subsequent degradation of CtsR by the ClpCP protease along with McsA and McsB. Similarly, σB also upregulates the transcription of mpoABCD (Raengpradub et al., 2008; Oliver et al., 2009, 2010; Ollinger et al., 2009; Mujahid et al., 2013b), which encodes PTSMpo. Upon glucose uptake by PTSMpo, one subunit, EIIBMpo, post-translationally activates the transcriptional regulator ManR, which then, activates the transcription of the manLMN operon (encoding PTSMan) (Ake et al., 2011).
Closing Remarks
A holistic systems approach to regulatory networks will be essential to provide new insights into gene regulation in L. monocytogenes. Studying complex regulatory interactions in motifs enables the detection of distant connections more easily as it shortens pathways into motifs without specifically naming all the intermediary steps. The abstraction to regulatory motifs also makes comparison across different species easier, since motif analysis will detect similarities in the hardwiring of a network regardless of the names of individual factors. Another important application of regulatory motifs in the analysis of regulatory networks is the study of co-expression. If two genes are co-regulated by the same transcription factor the degree of co-expression may vary (Yu et al., 2003). Experimental determination of co-expression for one motif will allow for informed assumptions about the degree of co-expression in a similarly wired regulatory network. However, one of the current challenges is the often missing information of the precise biological function of a network. Experimental confirmation of assumptions made from network analyses remains crucial.
As new genetics and “omics” data involving regulatory interactions in L. monocytogenes become available, the need to develop better tools to analyze these interactions on a large scale grows and a systems approach to understanding regulatory networks becomes feasible.
A better understanding of how transcriptional regulators affect the expression of downstream regulatees is key to understanding the biology of L. monocytogenes and other bacterial pathogens that have to transit rapidly changing environments to cause disease and will ultimately facilitate the development of better strategies to prevent and treat listeriosis.
Conflict of Interest Statement
The authors declare that the research was conducted in the absence of any commercial or financial relationships that could be construed as a potential conflict of interest.
References
Ake, F. M., Joyet, P., Deutscher, J., and Milohanic, E. (2011). Mutational analysis of glucose transport regulation and glucose-mediated virulence gene repression in Listeria monocytogenes. Mol. Microbiol. 81, 274–293. doi: 10.1111/j.1365-2958.2011.07692.x
Alon, U. (2007). Network motifs: theory and experimental approaches. Nat. Rev. Genet. 8, 450–461. doi: 10.1038/nrg2102
Arous, S., Buchrieser, C., Folio, P., Glaser, P., Namane, A., Hebraud, M., et al. (2004). Global analysis of gene expression in an rpoN mutant of Listeria monocytogenes. Microbiology 150(Pt 5), 1581–1590. doi: 10.1099/mic.0.26860-0
Autret, N., Raynaud, C., Dubail, I., Berche, P., and Charbit, A. (2003). Identification of the agr locus of Listeria monocytogenes: role in bacterial virulence. Infect. Immun. 71, 4463–4471. doi: 10.1128/IAI.71.8.4463-4471.2003
Barabasi, A. L., and Oltvai, Z. N. (2004). Network biology: understanding the cell's functional organization. Nat. Rev. Genet. 5, 101–113. doi: 10.1038/nrg1272
Becskei, A., and Serrano, L. (2000). Engineering stability in gene networks by autoregulation. Nature 405, 590–593. doi: 10.1038/35014651
Bennett, H. J., Pearce, D. M., Glenn, S., Taylor, C. M., Kuhn, M., Sonenshein, A. L., et al. (2007). Characterization of relA and codY mutants of Listeria monocytogenes: identification of the CodY regulon and its role in virulence. Mol. Microbiol. 63, 1453–1467. doi: 10.1111/j.1365-2958.2007.05597.x
Bertram, R., Rigali, S., Wood, N., Lulko, A. T., Kuipers, O. P., and Titgemeyer, F. (2011). Regulon of the N-acetylglucosamine utilization regulator NagR in Bacillus subtilis. J. Bacteriol. 193, 3525–3536. doi: 10.1128/JB.00264-11
Bonneau, R., Facciotti, M. T., Reiss, D. J., Schmid, A. K., Pan, M., Kaur, A., et al. (2007). A predictive model for transcriptional control of physiology in a free living cell. Cell 131, 1354–1365. doi: 10.1016/j.cell.2007.10.053
Chan, Y. C., Hu, Y., Chaturongakul, S., Files, K. D., Bowen, B. M., Boor, K. J., et al. (2008). Contributions of two-component regulatory systems, alternative sigma factors, and negative regulators to Listeria monocytogenes cold adaptation and cold growth. J. Food Prot. 71, 420–425.
Chaturongakul, S., and Boor, K. J. (2006). SigmaB activation under environmental and energy stress conditions in Listeria monocytogenes. Appl. Environ. Microbiol. 72, 5197–5203. doi: 10.1128/AEM.03058-05
Chaturongakul, S., Raengpradub, S., Palmer, M. E., Bergholz, T. M., Orsi, R. H., Hu, Y., et al. (2011). Transcriptomic and phenotypic analyses identify coregulated, overlapping regulons among PrfA, CtsR, HrcA, and the alternative sigma factors sigmaB, sigmaC, sigmaH, and sigmaL in Listeria monocytogenes. Appl. Environ. Microbiol. 77, 187–200. doi: 10.1128/AEM.00952-10
Chaturongakul, S., Raengpradub, S., Wiedmann, M., and Boor, K. J. (2008). Modulation of stress and virulence in Listeria monocytogenes. Trends Microbiol. 16, 388–396. doi: 10.1016/j.tim.2008.05.006
Dalet, K., Cenatiempo, Y., Cossart, P., and Hechard, Y. (2001). A sigma(54)-dependent PTS permease of the mannose family is responsible for sensitivity of Listeria monocytogenes to mesentericin Y105. Microbiology 147, 3263–3269.
de Hoon, M. J., Eichenberger, P., and Vitkup, D. (2010). Hierarchical evolution of the bacterial sporulation network. Curr. Biol. 20, R735–R745. doi: 10.1016/j.cub.2010.06.031
de las Heras, A., Cain, R. J., Bielecka, M. K., and Vazquez-Boland, J. A. (2011). Regulation of Listeria virulence: PrfA master and commander. Curr. Opin. Microbiol. 14, 118–127. doi: 10.1016/j.mib.2011.01.005
Dufour, Y. S., and Donohue, T. J. (2012). Signal correlations in ecological niches can shape the organization and evolution of bacterial gene regulatory networks. Adv. Microb. Physiol. 61, 1–36. doi: 10.1016/B978-0-12-394423-8.00001-9
Dussurget, O., Cabanes, D., Dehoux, P., Lecuit, M., Buchrieser, C., Glaser, P., et al. (2002). Listeria monocytogenes bile salt hydrolase is a PrfA-regulated virulence factor involved in the intestinal and hepatic phases of listeriosis. Mol. Microbiol. 45, 1095–1106. doi: 10.1046/j.1365-2958.2002.03080.x
Freitag, N. E., Port, G. C., and Miner, M. D. (2009). Listeria monocytogenes - from saprophyte to intracellular pathogen. Nat. Rev. Microbiol. 7, 623–628. doi: 10.1038/nrmicro2171
Gaillot, O., Bregenholt, S., Jaubert, F., Di Santo, J. P., and Berche, P. (2001). Stress-induced ClpP serine protease of Listeria monocytogenes is essential for induction of listeriolysin O-dependent protective immunity. Infect. Immun. 69, 4938–4943. doi: 10.1128/IAI.69.8.4938-4943.2001
Gaillot, O., Pellegrini, E., Bregenholt, S., Nair, S., and Berche, P. (2000). The ClpP serine protease is essential for the intracellular parasitism and virulence of Listeria monocytogenes. Mol. Microbiol. 35, 1286–1294. doi: 10.1046/j.1365-2958.2000.01773.x
Garmyn, D., Augagneur, Y., Gal, L., Vivant, A. L., and Piveteau, P. (2012). Listeria monocytogenes differential transcriptome analysis reveals temperature-dependent Agr regulation and suggests overlaps with other regulons. PLoS ONE 7:e43154. doi: 10.1371/journal.pone.0043154
Glaser, P., Frangeul, L., Buchrieser, C., Rusniok, C., Amend, A., Baquero, F., et al. (2001). Comparative genomics of Listeria species. Science 294, 849–852. doi: 10.1126/science.1063447
Guelzim, N., Bottani, S., Bourgine, P., and Kepes, F. (2002). Topological and causal structure of the yeast transcriptional regulatory network. Nat. Genet. 31, 60–63. doi: 10.1038/ng873
Hanawa, T., Kai, M., Kamiya, S., and Yamamoto, T. (2000). Cloning, sequencing, and transcriptional analysis of the dnaK heat shock operon of Listeria monocytogenes. Cell Stress Chaperones 5, 21–29. doi: 10.1379/1466-1268(2000)005%3C0021:CSATAO%3E2.0.CO;2
Hu, Y., Oliver, H. F., Raengpradub, S., Palmer, M. E., Orsi, R. H., Wiedmann, M., et al. (2007b). Transcriptomic and phenotypic analyses suggest a network between the transcriptional regulators HrcA and sigmaB in Listeria monocytogenes. Appl. Environ. Microbiol. 73, 7981–7991. doi: 10.1128/AEM.01281-07
Hu, Y., Raengpradub, S., Schwab, U., Loss, C., Orsi, R. H., Wiedmann, M., et al. (2007a). Phenotypic and transcriptomic analyses demonstrate interactions between the transcriptional regulators CtsR and Sigma B in Listeria monocytogenes. Appl. Environ. Microbiol. 73, 7967–7980. doi: 10.1128/AEM.01085-07
Johansson, J., Mandin, P., Renzoni, A., Chiaruttini, C., Springer, M., and Cossart, P. (2002). An RNA thermosensor controls expression of virulence genes in Listeria monocytogenes. Cell 110, 551–561. doi: 10.1016/S0092-8674(02)00905-4
Jones, B. V., Begley, M., Hill, C., Gahan, C. G., and Marchesi, J. R. (2008). Functional and comparative metagenomic analysis of bile salt hydrolase activity in the human gut microbiome. Proc. Natl. Acad. Sci. U.S.A. 105, 13580–13585. doi: 10.1073/pnas.0804437105
Kamerlin, S. C., Vicatos, S., Dryga, A., and Warshel, A. (2011). Coarse-grained (multiscale) simulations in studies of biophysical and chemical systems. Annu. Rev. Phys. Chem. 62, 41–64. doi: 10.1146/annurev-physchem-032210-103335
Kato, A., Latifi, T., and Groisman, E. A. (2003). Closing the loop: the PmrA/PmrB two-component system negatively controls expression of its posttranscriptional activator PmrD. Proc. Natl. Acad. Sci. U.S.A. 100, 4706–4711. doi: 10.1073/pnas.0836837100
Kazmierczak, M. J., Mithoe, S. C., Boor, K. J., and Wiedmann, M. (2003). Listeria monocytogenes sigma B regulates stress response and virulence functions. J. Bacteriol. 185, 5722–5734. doi: 10.1128/JB.185.19.5722-5734.2003
Kim, D., Kwon, Y. K., and Cho, K. H. (2008). The biphasic behavior of incoherent feed-forward loops in biomolecular regulatory networks. Bioessays 30, 1204–1211. doi: 10.1002/bies.20839
Klinger, B., Sieber, A., Fritsche-Guenther, R., Witzel, F., Berry, L., Schumacher, D., et al. (2013). Network quantification of EGFR signaling unveils potential for targeted combination therapy. Mol. Syst. Biol. 9, 673. doi: 10.1038/msb.2013.29
Kruger, E., Zuhlke, D., Witt, E., Ludwig, H., and Hecker, M. (2001). Clp-mediated proteolysis in Gram-positive bacteria is autoregulated by the stability of a repressor. EMBO J. 20, 852–863. doi: 10.1093/emboj/20.4.852
Lee, T. I., Rinaldi, N. J., Robert, F., Odom, D. T., Bar-Joseph, Z., Gerber, G. K., et al. (2002). Transcriptional regulatory networks in Saccharomyces cerevisiae. Science 298, 799–804. doi: 10.1126/science.1075090
Lingnau, A., Domann, E., Hudel, M., Bock, M., Nichterlein, T., Wehland, J., et al. (1995). Expression of the Listeria monocytogenes EGD inlA and inlB genes, whose products mediate bacterial entry into tissue culture cell lines, by PrfA-dependent and -independent mechanisms. Infect. Immun. 63, 3896–3903.
Lobel, L., Sigal, N., Borovok, I., Ruppin, E., and Herskovits, A. A. (2012). Integrative genomic analysis identifies isoleucine and CodY as regulators of Listeria monocytogenes virulence. PLoS Genet. 8:e1002887. doi: 10.1371/journal.pgen.1002887
Loh, E., Dussurget, O., Gripenland, J., Vaitkevicius, K., Tiensuu, T., Mandin, P., et al. (2009). A trans-acting riboswitch controls expression of the virulence regulator PrfA in Listeria monocytogenes. Cell 139, 770–779. doi: 10.1016/j.cell.2009.08.046
Mangan, S., and Alon, U. (2003). Structure and function of the feed-forward loop network motif. Proc. Natl. Acad. Sci. U.S.A. 100, 11980–11985. doi: 10.1073/pnas.2133841100
Mangan, S., Zaslaver, A., and Alon, U. (2003). The coherent feedforward loop serves as a sign-sensitive delay element in transcription networks. J. Mol. Biol. 334, 197–204. doi: 10.1016/j.jmb.2003.09.049
McAdams, H. H., and Arkin, A. (1997). Stochastic mechanisms in gene expression. Proc. Natl. Acad. Sci. U.S.A. 94, 814–819. doi: 10.1073/pnas.94.3.814
McGann, P., Wiedmann, M., and Boor, K. J. (2007). The alternative sigma factor sigma B and the virulence gene regulator PrfA both regulate transcription of Listeria monocytogenes internalins. Appl. Environ. Microbiol. 73, 2919–2930. doi: 10.1128/AEM.02664-06
Mellin, J. R., and Cossart, P. (2012). The non-coding RNA world of the bacterial pathogen Listeria monocytogenes. RNA Biol. 9, 372–378. doi: 10.4161/rna.19235
Mengaud, J., Dramsi, S., Gouin, E., Vazquez-Boland, J. A., Milon, G., and Cossart, P. (1991). Pleiotropic control of Listeria monocytogenes virulence factors by a gene that is autoregulated. Mol. Microbiol. 5, 2273–2283. doi: 10.1111/j.1365-2958.1991.tb02158.x
Middleton, A. M., Farcot, E., Owen, M. R., and Vernoux, T. (2012). Modeling regulatory networks to understand plant development: small is beautiful. Plant Cell 24, 3876–3891. doi: 10.1105/tpc.112.101840
Mujahid, S., Orsi, R. H., Boor, K. J., and Wiedmann, M. (2013b). Protein level identification of the Listeria monocytogenes sigma H, sigma L, and sigma C regulons. BMC Microbiol. 13:156. doi: 10.1186/1471-2180-13-156
Mujahid, S., Orsi, R. H., Vangay, P., Boor, K. J., and Wiedmann, M. (2013a). Refinement of the Listeria monocytogenes sigmaB regulon through quantitative proteomic analysis. Microbiology 159(Pt 6), 1109–1119. doi: 10.1099/mic.0.066001-0
Nadon, C. A., Bowen, B. M., Wiedmann, M., and Boor, K. J. (2002). Sigma B contributes to PrfA-mediated virulence in Listeria monocytogenes. Infect. Immun. 70, 3948–3952. doi: 10.1128/IAI.70.7.3948-3952.2002
Nair, S., Derre, I., Msadek, T., Gaillot, O., and Berche, P. (2000). CtsR controls class III heat shock gene expression in the human pathogen Listeria monocytogenes. Mol. Microbiol. 35, 800–811. doi: 10.1046/j.1365-2958.2000.01752.x
O'Byrne, C. P., and Karatzas, K. A. (2008). The role of sigma B (sigma B) in the stress adaptations of Listeria monocytogenes: overlaps between stress adaptation and virulence. Adv. Appl. Microbiol. 65, 115–140. doi: 10.1016/S0065-2164(08)00605-9
Oliver, H. F., Orsi, R. H., Ponnala, L., Keich, U., Wang, W., Sun, Q., et al. (2009). Deep RNA sequencing of L. monocytogenes reveals overlapping and extensive stationary phase and sigma B-dependent transcriptomes, including multiple highly transcribed noncoding RNAs. BMC Genomics 10:641. doi: 10.1186/1471-2164-10-641
Oliver, H. F., Orsi, R. H., Wiedmann, M., and Boor, K. J. (2010). Listeria monocytogenes {sigma} B has a small core regulon and a conserved role in virulence but makes differential contributions to stress tolerance across a diverse collection of strains. Appl. Environ. Microbiol. 76, 4216–4232. doi: 10.1128/AEM.00031-10
Ollinger, J., Bowen, B., Wiedmann, M., Boor, K. J., and Bergholz, T. M. (2009). Listeria monocytogenes sigmaB modulates PrfA-mediated virulence factor expression. Infect. Immun. 77, 2113–2124. doi: 10.1128/IAI.01205-08
Popowska, M., Osinska, M., and Rzeczkowska, M. (2012). N-acetylglucosamine-6-phosphate deacetylase (NagA) of Listeria monocytogenes EGD, an essential enzyme for the metabolism and recycling of amino sugars. Arch. Microbiol. 194, 255–268. doi: 10.1007/s00203-011-0752-3
Raengpradub, S., Wiedmann, M., and Boor, K. J. (2008). Comparative analysis of the sigma B-dependent stress responses in Listeria monocytogenes and Listeria innocua strains exposed to selected stress conditions. Appl. Environ. Microbiol. 74, 158–171. doi: 10.1128/AEM.00951-07
Raimann, E., Schmid, B., Stephan, R., and Tasara, T. (2009). The alternative sigma factor sigma(L) of L. monocytogenes promotes growth under diverse environmental stresses. Foodborne Pathog. Dis. 6, 583–591. doi: 10.1089/fpd.2008.0248
Rea, R. B., Gahan, C. G., and Hill, C. (2004). Disruption of putative regulatory loci in Listeria monocytogenes demonstrates a significant role for Fur and PerR in virulence. Infect. Immun. 72, 717–727. doi: 10.1128/IAI.72.2.717-727.2004
Resch, M., Schiltz, E., Titgemeyer, F., and Muller, Y. A. (2010). Insight into the induction mechanism of the GntR/HutC bacterial transcription regulator YvoA. Nucleic Acids Res. 38, 2485–2497. doi: 10.1093/nar/gkp1191
Ruiz, N., Peterson, C. N., and Silhavy, T. J. (2001). RpoS-dependent transcriptional control of sprE: regulatory feedback loop. J. Bacteriol. 183, 5974–5981. doi: 10.1128/JB.183.20.5974-5981.2001
Schwab, U., Bowen, B., Nadon, C., Wiedmann, M., and Boor, K. J. (2005). The Listeria monocytogenes prfAP2 promoter is regulated by sigma B in a growth phase dependent manner. FEMS Microbiol. Lett. 245, 329–336. doi: 10.1016/j.femsle.2005.03.025
Scortti, M., Monzo, H. J., Lacharme-Lora, L., Lewis, D. A., and Vazquez-Boland, J. A. (2007). The PrfA virulence regulon. Microbes Infect. 9, 1196–1207. doi: 10.1016/j.micinf.2007.05.007
Seifart, G. C., Izar, B., Pazan, F., Mohamed, W., Mraheil, M. A., Mukherjee, K., et al. (2011). Universal stress proteins are important for oxidative and acid stress resistance and growth of Listeria monocytogenes EGD-e in vitro and in vivo. PLoS ONE 6:e24965. doi: 10.1371/journal.pone.0024965
Shen-Orr, S. S., Milo, R., Mangan, S., and Alon, U. (2002). Network motifs in the transcriptional regulation network of Escherichia coli. Nat. Genet. 31, 64–68. doi: 10.1038/ng881
Snoep, J. L., Bruggeman, F., Olivier, B. G., and Westerhoff, H. V. (2006). Towards building the silicon cell: a modular approach. Biosystems 83, 207–216. doi: 10.1016/j.biosystems.2005.07.006
Sue, D., Boor, K. J., and Wiedmann, M. (2003). Sigma(B)-dependent expression patterns of compatible solute transporter genes opuCA and lmo1421 and the conjugated bile salt hydrolase gene bsh in Listeria monocytogenes. Microbiology 149(Pt 11), 3247–3256. doi: 10.1099/mic.0.26526-0
Sue, D., Fink, D., Wiedmann, M., and Boor, K. J. (2004). SigmaB-dependent gene induction and expression in Listeria monocytogenes during osmotic and acid stress conditions simulating the intestinal environment. Microbiology 150(Pt 11), 3843–3855. doi: 10.1099/mic.0.27257-0
Tessema, G. T., Moretro, T., Kohler, A., Axelsson, L., and Naterstad, K. (2009). Complex phenotypic and genotypic responses of Listeria monocytogenes strains exposed to the class IIa bacteriocin sakacin P. Appl. Environ. Microbiol. 75, 6973–6980. doi: 10.1128/AEM.00608-09
Tessema, G. T., Moretro, T., Snipen, L., Heir, E., Holck, A., Naterstad, K., et al. (2012). Microarray-based transcriptome of Listeria monocytogenes adapted to sublethal concentrations of acetic acid, lactic acid, and hydrochloric acid. Can. J. Microbiol. 58, 1112–1123. doi: 10.1139/w2012-091
Thattai, M., and van Oudenaarden, A. (2001). Intrinsic noise in gene regulatory networks. Proc. Natl. Acad. Sci. U.S.A. 98, 8614–8619. doi: 10.1073/pnas.151588598
Thieffry, D., Huerta, A. M., Perez-Rueda, E., and Collado-Vides, J. (1998). From specific gene regulation to genomic networks: a global analysis of transcriptional regulation in Escherichia coli. Bioessays 20, 433–440. doi: 10.1002/(SICI)1521-1878(199805)20:5<433::AID-BIES10>3.0.CO;2-2
Tyson, J. J., and Novak, B. (2010). Functional motifs in biochemical reaction networks. Annu. Rev. Phys. Chem. 61, 219–240. doi: 10.1146/annurev.physchem.012809.103457
Utratna, M., Cosgrave, E., Baustian, C., Ceredig, R., and O'Byrne, C. (2012). Development and optimization of an EGFP-based reporter for measuring the general stress response in Listeria monocytogenes. Bioeng. Bugs 3, 93–103. doi: 10.4161/bbug.19476
van Helden, J., Toussaint, A., and Thieffry, D. (2012). Bacterial molecular networks: bridging the gap between functional genomics and dynamical modelling. Methods Mol. Biol. 804, 1–11. doi: 10.1007/978-1-61779-361-5_1
Wemekamp-Kamphuis, H. H., Wouters, J. A., de Leeuw, P. P., Hain, T., Chakraborty, T., and Abee, T. (2004). Identification of sigma factor sigma B-controlled genes and their impact on acid stress, high hydrostatic pressure, and freeze survival in Listeria monocytogenes EGD-e. Appl. Environ. Microbiol. 70, 3457–3466. doi: 10.1128/AEM.70.6.3457-3466.2004
Wichadakul, D., McDermott, J., and Samudrala, R. (2009). Prediction and integration of regulatory and protein-protein interactions. Methods Mol. Biol. 541, 101–143. doi: 10.1007/978-1-59745-243-4_6
Williams, T., Bauer, S., Beier, D., and Kuhn, M. (2005). Construction and characterization of Listeria monocytogenes mutants with in-frame deletions in the response regulator genes identified in the genome sequence. Infect. Immun. 73, 3152–3159. doi: 10.1128/IAI.73.5.3152-3159.2005
Wuchty, S., Oltvai, Z. N., and Barabasi, A. L. (2003). Evolutionary conservation of motif constituents in the yeast protein interaction network. Nat. Genet. 35, 176–179. doi: 10.1038/ng1242
Xia, Y., Yu, H., Jansen, R., Seringhaus, M., Baxter, S., Greenbaum, D., et al. (2004). Analyzing cellular biochemistry in terms of molecular networks. Annu. Rev. Biochem. 73, 1051–1087. doi: 10.1146/annurev.biochem.73.011303.073950
Yu, H., and Gerstein, M. (2006). Genomic analysis of the hierarchical structure of regulatory networks. Proc. Natl. Acad. Sci. U.S.A. 103, 14724–14731. doi: 10.1073/pnas.0508637103
Yu, H., Luscombe, N. M., Qian, J., and Gerstein, M. (2003). Genomic analysis of gene expression relationships in transcriptional regulatory networks. Trends Genet. 19, 422–427. doi: 10.1016/S0168-9525(03)00175-6
Keywords: Listeria monocytogenes, regulatory network, PrfA, SigB, network motif
Citation: Guariglia-Oropeza V, Orsi RH, Yu H, Boor KJ, Wiedmann M and Guldimann C (2014) Regulatory network features in Listeria monocytogenes—changing the way we talk. Front. Cell. Infect. Microbiol. 4:14. doi: 10.3389/fcimb.2014.00014
Received: 29 October 2013; Accepted: 27 January 2014;
Published online: 14 February 2014.
Edited by:
Stephanie M. Seveau, The Ohio State University, USACopyright © 2014 Guariglia-Oropeza, Orsi, Yu, Boor, Wiedmann and Guldimann. This is an open-access article distributed under the terms of the Creative Commons Attribution License (CC BY). The use, distribution or reproduction in other forums is permitted, provided the original author(s) or licensor are credited and that the original publication in this journal is cited, in accordance with accepted academic practice. No use, distribution or reproduction is permitted which does not comply with these terms.
*Correspondence: Claudia Guldimann, Department of Food Science, Cornell University, 347 Stocking Hall, Ithaca, NY 14853, USA e-mail: cg445@cornell.edu